DOI:
10.1039/C6MB00732E
(Paper)
Mol. BioSyst., 2017,
13, 1109-1120
A metabolomic study on high-risk stroke patients determines low levels of serum lysine metabolites: a retrospective cohort study†
Received
26th October 2016
, Accepted 3rd April 2017
First published on 7th April 2017
Abstract
Identifying changes in serum metabolites during cerebral ischemia is an important approach for early diagnosis of thrombotic stroke. Herein, we highlight novel biomarkers for early diagnosis of patients at high risk of thrombotic stroke using high resolution metabolomics (HRM). In this retrospective cohort study, serum samples obtained from patients at risk of thrombotic stroke (n
=
62) and non-risk individuals (n
=
348) were tested using HRM, coupled with LC-MS/MS, to discriminate between metabolic profiles of control and stroke risk patients. Multivariate analysis and orthogonal partial least square-discriminant analysis (OPLS-DA) were performed to determine the top 5% metabolites within 95% group identities, followed by filtering with p-value <0.05 and annotating significant metabolites using a Metlin database. Mapping identified features from Kyoto Encyclopedia of Genes and Genomes (KEGG) and Mummichog resulted in 341 significant features based on OPLS-DA with p-value <0.05. Among these 341 features, nine discriminated the thrombotic stroke risk group from the control group: low levels of N6-acetyl-L-lysine, 5-aminopentanoate, cadaverine, 2-oxoglutarate, nicotinamide, L-valine, S-(2-methylpropionyl)-dihydrolipoamide-E and ubiquinone, and elevated levels of homocysteine sulfinic acid. Further analysis showed that these metabolite biomarkers are specifically related to stroke occurrence, and unrelated to other factors such as diabetes or smoking. Lower levels of lysine catabolites in thrombotic stroke risk patients, as compared to the control, supports targeting these compounds as novel biomarkers for early and non-invasive detection of a thrombotic stroke.
1. Introduction
Cerebral ischemia due to thrombotic occlusion causes transient or permanent reduction in cerebral blood flow, resulting in a brain stroke because of energy crisis and lack of oxygen. Subsequent energy depletion activates a cascade of molecular events, such as release of excess excitatory amino acids in extracellular space, free-radical formation, and inflammation.1 Therefore, several opportunistic biological markers are released into peripheral blood, providing new insights for metabolomics research for predicting ischemic lesions and clinical prognosis.
Recent progress in high resolution mass spectrometry has resulted in a metabolomics approach, currently receiving central attention in advanced research.2 It is now possible to identify biomarkers of thrombotic disorders in body fluids; for example, cerebrospinal fluid, serum, or blood.3 One of the major research challenges for an early diagnostic tool is to identify a subset of patients with an increased risk to develop thrombotic stroke, which has resulted in tremendous research in the last two decades.4 A majority of stroke biomarkers identified in previous studies were related to tissue damage, acute thrombosis, and inflammatory response.4 These biomarkers were mostly found as a result of cerebral damage.5 Nevertheless, these identified stroke biomarkers provided insights into the pathophysiology of ischemic or hemorrhagic stroke and helped in understanding the basic mechanisms of brain damage and/or recovery. However, use of stroke biomarkers is still in the research phase, and none of them has been used in clinical practice to date.
The role of the acidic amino acid glutamate as an excitatory neurotransmitter in the brain has been known for the past four decades.6 Under normal circumstances, the concentration of glutamate in blood and cerebrospinal fluids is lower than those of basic amino acids, lysine and arginine.7 However, during or after onset of cerebral ischemia, energy depletion results in loss of membrane potential and depolarization of neurons. This results in release of large amounts of glutamate from presynaptic neurons, and contributes to neuronal injury by excessive activation of N-methyl-D-aspartate (NMDA) and α-amino-3-hydroxy-5-methyl-4-isoxazolepropionic acid (AMPA) receptors, thus increasing intracellular Ca2+ and activating catabolic processes, thereby leading to cell death.1,8,9 Furthermore, nicotinamide, which is a precursor of nicotinamide adenine dinucleotide (NAD+),10 acts as a poly(ADP-ribose) polymerase (PARP) inhibitor, and helps to prevent DNA damage and cell death caused by PARP inappropriate activation in response to rising levels of nitric oxide (NO) during ischemic events.11 However, during ischemic strokes, nicotinamide is over consumed owing to inappropriate activation of PARP12 and ATPs are over utilized because of excessive activation of glutamate receptors, leading to cell death.13 Thus, glutamate and nicotinamide are important factors contributing to thrombotic ischemia. The excitotoxic activity of glutamate in a cerebral infarction can act as a diagnostic biomarker for ischemic lesion growth, final infarct volume, and early neurological deterioration during cerebral ischemia.4,14 However, the time required for an increase in glutamate levels during a stroke, and the window of opportunity to detect glutamate during the specific time of a stroke, was 6 hours in stable ischemic stroke patients,15 and even shorter (less than 2 hours) in animal stroke models.16 The short duration of higher levels of glutamate enables differentiating patients with an acute stroke at an early stage from the control group. However, no study has identified putative biomarkers that may serve as early diagnostic tools to identify a subset of patients with increased risk of developing thrombotic ischemia. Therefore, we attempted to identify biomarkers associated with alteration in glutamate levels in blood from patients that were at risk of thrombotic stroke and later diagnosed with stroke.
Previous research on lysine levels provided detailed information about the potential application of lysine in different human developmental and physiological programs,17,18 and reduced levels of lysine were documented to cause mental and physical disorders.19 Lysine and arginine are the two basic amino acids documented to be involved in protection against brain injury.20 Administration of L-arginine, a precursor of nitric oxide (NO), enhances intra-ischemic cerebral blood flow (CBF) and reduces brain damage, which has been shown to reduce ischemic infarction.21 Intravenous administration of lysine and arginine in experimental rat models was observed with suppression of glutamate-induced neuronal activity, thereby reducing effects of cerebral ischemic insults.20,22 Furthermore, lysine infusion resulted in increased plasma levels of arginine, suggesting an increased availability of arginine for NO synthesis.23 However, investigation of neuroprotective effects of lysine and arginine, or their combination during an acute phase of cerebral edema, showed that the mechanism of neuroprotection by lysine is distinct from that by arginine. Nonetheless, deficiency in dietary lysine might increase the risk of stroke, while combined ingestion of lysine with arginine may enhance neuroprotective effects.22 A similar study showed reduced stress-induced anxiety after prolonged treatment of a lysine and arginine combination in rats and humans.24,25 Furthermore, lysine catabolism is an important substrate for de novo synthesis of glutamate in the human brain,26–28 while nicotinamide plays an important substrate role in the catabolic pathway of lysine.28 Thus, lysine catabolism involves converting lysine to glutamate, and then to other stress-related metabolites, in response to brain ischemia at the expense of nicotinamide.
Thus, previous studies suggested that lysine catabolism might serve as an important biomarker for prediction of ischemic brain injury. However, no study has shown the clinical interpretation of changes in lysine metabolic profiles in stroke patients. Therefore, we performed a retrospective cohort study in risk patients later diagnosed as stroke patients, and hypothesized that determination of serum concentrations of these compounds is an important approach for understanding the altered metabolism and pathophysiology of brain injury before occurrence of a thrombotic stroke.
2. Materials and methods
2.1 Chemicals and reagents
HPLC grade water (JT Baker, NJ, USA), acetonitrile (Tedia, NJ, USA), and formic acid (Fluka, MO, USA) were used in this study. All chemicals and reagents were of high purity and were stored at appropriate conditions. Standard solutions and serum samples were stored at −80 °C.
2.2 Study population
The study protocol was approved by the Korea University Institutional Review Board (KU-IRB-15-19-A-1). Study samples were obtained from the Korean Cancer Prevention Study-II (KCPS-II) biobank, Seoul and Gyeonggi, South Korea. KCPS-II is a prospective cohort biobank constructed in 2004–2011. Sampling was conducted according to the protocols approved by South Korea's Bioethics and Safety Act No. 9100. Blood was obtained from 410 people who consented to testing for research purposes at 11 comprehensive examination centers located in Seoul and Gyeonggi province in South Korea. Two groups were created based on their diagnosis as stroke or non-stroke patients, according to the International Classification of Disease (ICD) 10-code I63 (code for thrombotic stroke). To achieve this, 348 subjects, who did not develop a stroke later, were categorized as the control group. Among these, 78 persons were diabetic, 82 were ex-smokers, and 58 were current smokers. Additionally, 62 subjects were categorized as stroke risk patients who were later diagnosed with thrombotic stroke, according to ICD 10-code I63, on their first hospitalization in a 7 year retrospective cohort study with an outcome period from 2006 to 2010. Among the stroke risk group 19 were diabetic, 16 were ex-smokers, and 14 were current smokers. Details such as sex, age, body mass index (BMI), fasting blood sugar (FBS), total cholesterol, blood pressure, and smoking status of the control group and stroke risk patients are provided in Table 1. For validity of our results, an independent population of 400 people named as “Test set,” was used. Test set consisted of stroke risk patients (n = 99) and a control group (n = 301) who did not develop stroke.
Table 1 Study population characteristics
Characteristic |
Control [n = 348]
[mean ± SD]
|
TRSTR [n = 62]
[mean ± SD]
|
SD: standard deviation. Shows significant difference with control (student T test, p < 0.05). |
Sex |
Male (n) |
212 |
42 |
Female (n) |
136 |
20 |
Age (year) |
61.93 ± 3.12 |
63.34 ± 3.41a |
Fasting blood sugar (mg dL−1) |
98.86 ± 21.47 |
104.63 ± 30.51 |
BMI (kg m−2) |
24.23 ± 2.65 |
24.95 ± 2.97 |
Waist size (M) (cm) |
85.63 ± 7.06 |
88.89 ± 7.43a |
Waist size (F) (cm) |
80.87 ± 8.10 |
82.83 ± 9.27 |
Total cholesterol (mg dL−1) |
201.4 ± 36.63 |
196.95 ± 33.80 |
Triglycerides (mg dL−1) |
146.34 ± 91.83 |
158.31 ± 83.24 |
High density lipoproteins (M) (mg dL−1) |
49.09 ± 10.76 |
46.93 ± 11.90 |
High density lipoproteins (F) (mg dL−1) |
56.01 ± 12.51 |
52.3 ± 12.67 |
Systolic blood pressure (mmHg) |
126.11 ± 15.31 |
129.94 ± 16.50 |
Diastolic blood pressure (mmHg) |
76.72 ± 11.12 |
78.6 ± 9.95 |
Diabetes (n) |
78 |
19 |
Ex-smoker (n) |
82 |
16 |
Current-smoker (n) |
58 |
14 |
2.3 Sample preparation for metabolite extraction
Fifty microliter aliquots of serum were first treated with 195 μL of acetonitrile and 5 μL of a mixture of 3 stable isotope standards ([3-methyl-13C]-caffeine, [dimethyl-D6]-N,N-diethyl-M-toluamide, and [13C5, 15N]-L-methionine) (1
:
4, v/v), and centrifuged at 13
000 × g for 10 minutes at 4 °C for protein precipitation and extraction of metabolites. Supernatants containing the polar metabolites were collected for LC-MS/MS.29
2.4 Analysis of metabolites by LC-MS/MS
An Ultra Performance Liquid Chromatography system (Agilent 1260 Infinity Quaternary, Agilent, Santa Clara, CA, USA) coupled with an Agilent LC-MS/MS Q-TOF 6550 (Agilent, Santa Clara, CA, USA) mass spectrometer was used. Analytical separation was achieved using a Hypersil Gold C-18 (100 × 2.1 mm) 1.9 μm analytical column (Thermo, Waltham, USA) heated to 45 °C. Two different mobile phases were used for the analysis. Mobile phase A consisted of 0.1% formic acid in water, and mobile phase B consisted of 0.1% formic acid in acetonitrile. The analytes were eluted at a flow rate of 0.4 mL min−1 at 45 °C with solvent A and solvent B. The solvent gradient started with 5% solvent B (95% solvent A) for 1 min, followed by a linear increase to 45% B for 9 min, then to 90% B in 3 min, maintained at this concentration for additional 2 min, followed by return to the initial conditions. The total run time was 15 min. Analytes were detected using an electrospray ionization detector operated at the same conditions for positive and negative ion modes: a curtain gas of 35 psi, gas temperature of 250 °C supplied at 14 mL min−1, and sheath gas temperature of 250 °C supplied at a flow rate of 11 mL min−1. All samples were run in triplicate, and data for each ionization technique were acquired in the positive ion mode.30
2.5 Metabolic profiling
LC-MS data were acquired and processed using a software package (apLCMS) to obtain ion intensities.31 The apLCMS provided 7968 m/z (mass/charge ratio) within a range of ions set from 50 to 1000 with a resolution of 20
000. Accurate masses of features discriminating between the control group and stroke serum were visually compared using an orthogonal partial least squares-discriminant analysis (OPLS-DA) using Matlab software.32 The discriminatory m/z values after OPLS-DA were used to identify the top 5% features in the 95% range of group identities. An online database, METLIN, for annotation of features,33 was followed and the features were mapped to the Kyoto Encyclopedia of Genes and Genomes (KEGG) database to determine important metabolic pathways.34 In addition, significant m/z values after OPLS-DA with filtering of p-value less than 0.05 were annotated by Mummichog to create a potential metabolic network model.35
2.6 Data annotation and pathway analysis using KEGG and Mummichog
The identified metabolites, annotated using the Metlin Mass Spectrometry Database, were queried into the human metabolomics pathway (KEGG), and the detected m/z features that matched known human intermediary metabolites were observed as black dots in the map.36 Total detected m/z features (Lref) and the significant m/z features (Lsig) were arranged as two datasets and annotated by Mummichog to create a potential metabolic network model.35 From the m/z features in Lsig, matching metabolites were determined by Mummichog, and later, a reference metabolic network for all modules that can be produced by these metabolites was also established. The predicted metabolites listed in these modules were colored according to the fold change. This program has been successfully used to aid the identification of a metabolite activity network in immune response to virus.35 Moreover, discriminatory metabolites of thrombotic stroke risk patients and control groups were further classified for their accuracy and specificity using receiver operating characteristic (ROC) curves (MedCalc, Ostend, Belgium).37 ROC curves were used to search for an optimal cut-off value with the highest sensitivity and specificity, and Youden's index was used to identify a new cut-off value. Youden's index maximized the difference between sensitivity and specificity and between real-positive and false-positive subjects.38
3. Results
3.1 Subject characteristics
The subjects in this pilot study were from a cohort of thrombotic stroke risk patients and a control group living in South Korea. Demographic and phenotypic characteristics of the included subsets are described in Table 1. As matching feature tests were included in the design of this study, we observed no statistical differences between the control and stroke group of the pilot subset regarding the following characteristics: distribution of sex, body mass index (BMI), fasting blood sugar (FBS), total cholesterol, and blood pressure. Age criteria for selection was between 58–68 years; however, we detected a slight statistical difference in age among stroke risk patients and the control group as shown in Table 1.
3.2 Separation of control and thrombotic stroke risk patients by OPLS-DA
Metabolic profiles of thrombotic stroke risk patients appeared differently from those of the healthy control group patients. Mass spectra were processed using apLCMS and resulted in 7968 features. In order to determine discriminatory metabolic difference between control and thrombotic stroke risk patients, m/z of the 7968 were analyzed using OPLS-DA with autoscaling. Fig. 1a shows the score plot that represents the separation of control and thrombotic strokes. Fig. 1b represents the loading plot. The green dots indicate 7968 features. The gold circles represent the top 5% of these features (403 m/z) in the range of 95% group identities that are most closely associated with control or thrombotic stroke. Thus, using OPLS-DA, the top 5% discriminatory metabolites were considered for discriminating important features between thrombotic stroke vs. control.39
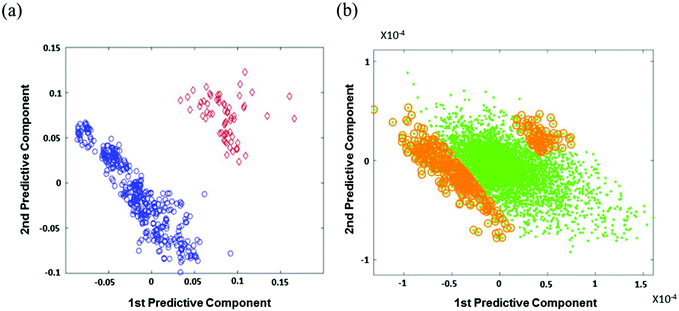 |
| Fig. 1 Discrimination between features of control and stroke serum by OPLS-DA. OPLS-DA results comparing metabolites from control group (blue circles) and stroke risk patients (red diamonds). (a) Score plot of control and stroke risk patient metabolic data showing a clear separation of groups. (b) The green dots signify metabolites with 95% association with the first two principal components. The gold circles represent the top 5% of these metabolites (403 m/z) that are most closely associated with control or stroke serum. | |
3.3 Annotation of features and pathway analysis
The extracted significant features after filtering with p-value (<0.05) were 341 m/z. These significantly expressed features in thrombotic stroke serum were annotated by their m/z values using the Metlin database or Mummichog. The following seven positive adducts were used: [M + H]+, [M + Na]+, [M + NH4]+, [M + H–H2O]+, [M + K]+, [M + HCOONa]+, and [M + HCOOK]+ with a confidence limit of 10 ppm to increase sensitivity of identification of compounds and to filter out irrelevant compounds that may have been included in the search. The Metlin database generates a list of possible metabolites that match the m/z values, as well as KEGG numbers that are used to map these metabolites to the metabolic pathway of the organism. ESI,† Fig. S1b shows the human metabolic pathway of thrombotic stroke from KEGG. The black dots on the colored pathways represent the potentially affected metabolites linked to thrombotic stroke. Among the 341 significant features, 41 metabolites were found to be involved in the KEGG human metabolic pathway. ESI,† Fig. S1b shows the top ten pathways with the largest number of compounds, which were candidate pathways for further analysis.
3.4 Lysine catabolism increased in thrombotic stroke risk patients
Previous studies have suggested lysine homeostasis as an important phenomenon for human brain functions. Therefore, we selectively analyzed the lysine catabolic pathway in KEGG and Mummichog, as shown in Fig. 2a. Compounds with black downwards arrows represent the levels of metabolites that decreased significantly (p-value <0.05) in thrombotic stroke risk patients compared to control group patients. Fig. 2b–e shows the relative concentration of lysine and its degraded metabolites, assessed using their respective signal intensities from Q-TOF. This assessment showed that the parent compound L-lysine (m/z 164.14, [M + NH4]+), along with its catabolites N6-acetyl-L-lysine (m/z 206.15, [M + NH4]+), 5-aminopentanoate(m/z 118.09, [M + H]+), and cadaverine (m/z 125.11, [M + Na]+) were lower in the serum obtained from thrombotic stroke risk patients, as shown in Fig. 2b–e, suggesting that lysine catabolism was higher in patients who were at risk or at early stage of thrombotic stroke. The distribution of these metabolites in control and stroke risk patients, when assessed by ROC analysis, further supported the lower levels of lysine catabolites in stroke risk patients. As shown in ESI,† Fig. S2a and Table S1, the AUC of all metabolites was greater than 0.5. Furthermore, as shown in Fig. 2a, 2-oxoglutarate acted as a substrate of glutamate during lysine degradation process. We assessed the relative concentration of 2-oxoglutarate, which was found to be lower in thrombotic stroke risk patients, as shown in ESI,† Fig. S3a, with an AUC of 0.716 (ESI,† Fig. S2a and Table S1). This result supports the elevated lysine catabolism phenomena, as stroke risk patients showed higher consumption of 2-oxoglutarate, compared to the control, during catabolism process of lysine at the expense of glutamate production. However, we could not detect glutamate in serum obtained from either thrombotic stroke risk patients or the control group. This can be explained by results from a previous study, where duration of increase in glutamate was shown to be only 6 hours after onset of ischemic stroke.15 However, another possibility might be the serum, which was obtained before stroke occurrence in this retrospective cohort study.
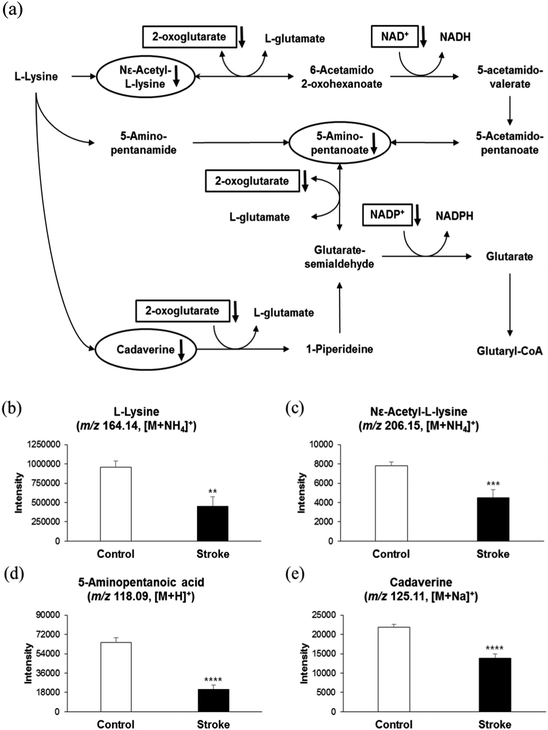 |
| Fig. 2 Lysine catabolic pathway from KEGG and Mummichog, and relative intensities of lysine and its catabolites in control and stroke risk patients. (a) Pathway of lysine catabolism obtained from the KEGG (ellipse) and Mummichog (rectangle). The arrows represent compounds that are detected in lower concentrations in stroke risk patients. (b) L-Lysine (m/z 164.14, [M + NH4]+) in control versus stroke risk group, extracted from raw data. (c–e) Metabolites from KEGG in control versus stroke risk group. (c) N6-Acetyl-L-lysine (m/z 206.15, [M + NH4]+), (d) 5-aminopentanoate (m/z 118.09, [M + H]+), and (e) cadaverine (m/z 125.11, [M + Na]+). * Significant difference (p < 0.05). | |
Furthermore, we evaluated the importance of nicotinamide in our study because nicotinamide, as shown in Fig. 2a, is utilized in all catabolic pathways of lysine as a source of energy. We observed a lower intensity of nicotinamide in stroke risk patients, as shown in ESI,† Fig. S3b, with an AUC of 0.653 (ESI,† Fig. S2a and Table S1). This finding is in accordance with previous results showing that lysine is efficiently catabolized during thrombotic ischemic events for production of glutamate in brain cells.28 Thus, our findings of lower concentrations of lysine catabolites, 2-oxoglutarate, and nicotinamide suggest an increased excitotoxic activity of glutamate.20,22,40 In addition, 2-oxoglutarate acts as a substrate of glutamate in the valine catabolism pathway, as shown in ESI,† Fig. S4a. Consistent with our previous results, we observed a lower concentration of valine (m/z 118.09, [M + H]+) in thrombotic stroke risk patients, as shown in ESI,† Fig. S4b, while its catabolite, S-(2-methylpropionyl)-dihydrolipoamide-E (m/z 278.13, [M + H]+), was found at higher concentrations (ESI,† Fig. S4c) with an AUC of 0.713 for valine and 0.716 for S-(2-methylpropionyl)-dihydrolipoamide-E (ESI,† Fig. S2b and Table S1). Similarly, concentrations of 2-oxoglutarate in the valine catabolic pathway were found at lower concentrations in the thrombotic stroke risk patients, as compared to those in control group patients, as shown in ESI,† Fig. S4d. This result suggests that valine, along with lysine, is degraded in the early neurological deterioration process for the production of glutamate, causing glutamate excitotoxicity because of increased production of glutamate.
3.5 Low serum levels of lysine catabolites are specifically related to stroke
As mentioned in the methods section 2.2 in this study, 348 subjects, consisting of 78 diabetic, 82 ex-smokers, and 58 current smokers, were categorized as the control group, which did not develop a stroke later during a 7-year cohort. Similarly, among 62 stroke risk patients who were later diagnosed with thrombotic stroke, 19 were diabetic, 16 were ex-smokers, and 14 were current smokers. Thus, we presumed that a low level of lysine catabolites and elevation in homocysteine sulfinic acid or other metabolites might be due to diabetes mellitus or smoking. In order to exclude the possibility of distortion of data by the diabetes metabolic effect, we separately analyzed the concentrations of lysine and its catabolites in the control group with and without diabetes mellitus (DM), and those in stroke risk patients with and without DM. As shown in Fig. 3a–d, the concentrations of lysine and its catabolites were significantly low in diabetic, as well as in non-diabetic subjects, who were at risk of developing a stroke, while diabetic and non-diabetic subjects in the control group who did not develop a stroke later, showed high intensities of lysine and lysine catabolites. These results suggest that elevated lysine catabolism correlates with pre stroke phenomena, and DM does not alter lysine catabolism. Similarly, we separately analyzed the concentration of lysine and its catabolites in the control group with and without smokers, and in stroke risk patients with and without smokers. As shown in Fig. 4a–d, concentrations of lysine and its catabolites were significantly lower in smoker or non-smoker subjects who were at risk of stroke development, as compared to those in smokers and non-smokers in the control group. These results further confirm that lysine catabolism is specifically related to stroke occurrence and unrelated to other factors such as DM or smoking.
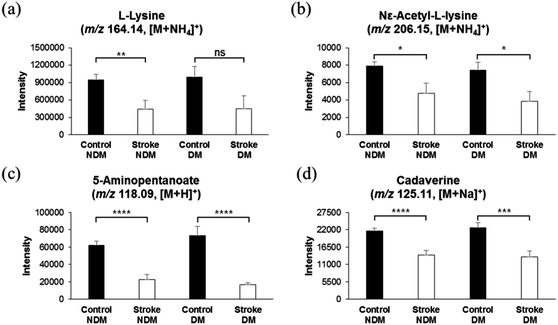 |
| Fig. 3 Relative concentrations of lysine and its catabolites in control group and stroke risk patients in relation to DM. (a–d) Comparison of relative concentrations of lysine and its catabolites in control subjects with and without DM and in stroke risk patients with and without DM. L-Lysine (m/z 164.14, [M + NH4]+), (b) N6-acetyl-L-lysine (m/z 206.15, [M + NH4]+), (c) 5-aminopentanoate (m/z 118.09, [M + H]+), and (d) cadaverine (m/z 125.11, [M + Na]+). DM stands for diabetic mellitus, and NDM stands for non-diabetic subjects. * Significant difference (p < 0.05). | |
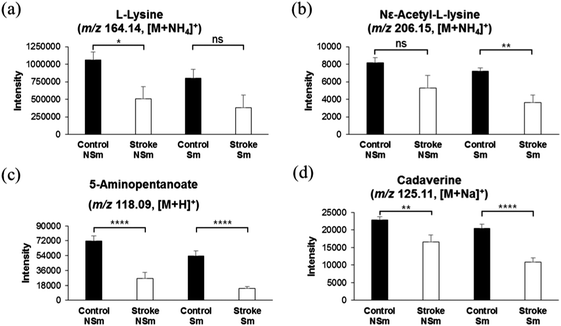 |
| Fig. 4 Relative concentrations of lysine and its catabolites in control group and stroke risk patients in relation to smoking. (a–d) Comparison of relative concentrations of lysine and its catabolites in smoker and non-smoker control subjects and in smoker and non-smoker stroke risk patients. L-Lysine (m/z 164.14, [M + NH4]+), (b) N6-acetyl-L-lysine (m/z 206.15, [M + NH4]+), (c) 5-aminopentanoate (m/z 118.09, [M + H]+), and (d) cadaverine (m/z 125.11, [M + Na]+). Sm stands for smoker, and NSm stands for non-smoker subjects. * Significant difference (p < 0.05). | |
Similar results were obtained with 2-oxoglutarate, which acts as a substrate of glutamate in the lysine and valine catabolism pathway, and nicotinamide, which is utilized in the lysine catabolic pathway as a source of energy. As shown in ESI,† Fig. S3, concentrations of both compounds, 2-oxoglutarate and nicotinamide, were significantly lower in stroke risk subjects with and without DM (ESI,† Fig. S3c and d) or smoking (ESI,† Fig. S3e and f), as compared to those in the control group.
3.6 Elevated homocysteine sulfinic acid and low ubiquinone levels are in agreement with other studies
Numerous studies have revealed the significant relationship between elevated plasma homocysteine concentrations and cardiovascular disease, especially stroke.41,42 Elevated plasma homocysteine promotes oxidative stress, leading to cell damage and/or death by mitogenic effects on vascular smooth muscles, and cytotoxic and thrombogenic effects on vascular endothelium.43 Especially, the acidic derivative of homocysteine, L-homocysteine sulfinic acid, has been shown to be a selective agonist of metabotropic glutamate receptors in rat brain cells, explaining pathogenesis of homocysteine-mediated damage during cerebral ischemia.44 Surprisingly, in support of previous studies, we found elevated levels of L-homocysteine sulfinic acid (m/z 168.03, [M + H]+) in serum samples of stroke risk patients, as compared with those of the control group, as shown in Fig. 5a. An AUC of 0.651 (ESI,† Fig. S2c and Table S1) was observed, suggesting a synergistic effect of L-homocysteine sulfinic acid on glutamate receptors along with glutamate, thereby increasing the excitotoxicity phenomena. Furthermore, as shown in Fig. 5c, the control group with DM or non-DM did not show any difference in the levels of L-homocysteine sulfinic acid, and the stroke risk patients with or without DM showed significantly higher intensities of L-homocysteine sulfinic acid, as compared to the control group. A similar phenomenon was observed with the smokers, as shown in Fig. 5e, suggesting the specific association of elevated L-homocysteine sulfinic acid levels with stroke occurrence.
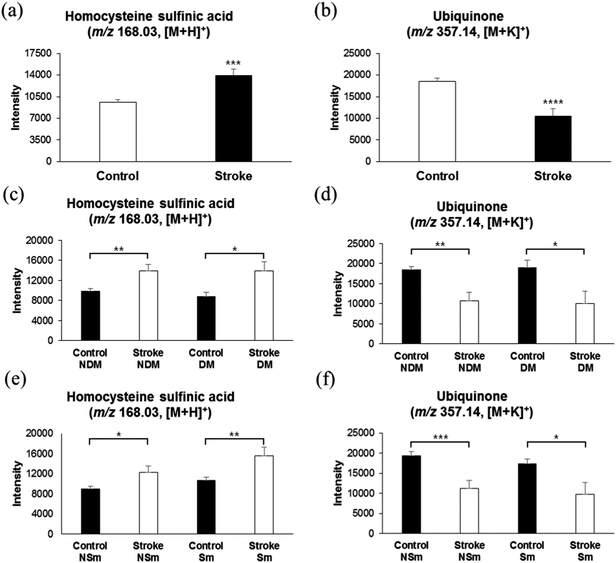 |
| Fig. 5 Intensities of homocysteine sulfinic acid and ubiquinone in control and stroke risk patients, and their correlation with diabetes metabolic or smoking. (a) Relative concentrations of homocysteine sulfinic acid (m/z 168.03, [M + H]+) in control and stroke risk patients. (b) Relative intensity of ubiquinone (m/z 357.14, [M + K]+) in control and stroke risk patients. (c and d) Comparison of relative intensities of homocysteine sulfinic acid and ubiquinone in diabetic and non-diabetic control subjects and diabetic and non-diabetic stroke risk patients. (e and f) Comparison of relative intensities of homocysteine sulfinic acid and ubiquinone in smoker and non-smoker control subjects and in smoker and non-smoker stroke risk patients. * Significant difference (p < 0.05). | |
A majority of antioxidants are reduced immediately after an acute ischemic stroke.45 Anti-oxidant therapy has long been investigated as a means to reduce the extent of injury resulting from ischemic stroke.46 Coenzyme Q10, also known as ubiquinone, is a naturally occurring anti-oxidant which has been shown to reduce the damage in several neurodegenerative diseases, such as Huntington's disease and Parkinson's disease, in animal models.47,48 In agreement with previous observations, we also found low intensities of ubiquinone (m/z 357.14, [M + K]+) in thrombotic stroke patients, possibly due to oxidative stress during early ischemia, as shown in Fig. 5b with an AUC of 0.677 (ESI,† Fig. S2c and Table S1). Such low plasma antioxidant activity has been shown to be associated with high lesion volume and neurological impairment in strokes.49 Furthermore, as shown in Fig. 5d and f, consistent with the results of lysine and homocysteine analyses, ubiquinone is specifically associated with the stroke risk group, and is not altered by DM or smoking.
3.7 Validation of candidate biomarkers in an independent population
To ensure the consistency of increased catabolism of lysine and other candidate metabolites in stroke risk patients, we validated our results in an independent propulsion (test set, n = 400), consisting of stroke risk patients who were later diagnosed as stroke patients (n = 99) and a control group (n = 301). The serum sample and statistical analysis was conducted in the same way as described in method sub-sections 2.3 to 2.6. As shown in ESI,† Fig. S5a and b, our test set resulted in exactly the same manner as those from OPLSDA results in Fig. 1a and b, and a clear separation was observed between control and thrombotic stroke risk patients. Furthermore, as shown in Fig. 6a and b, we observed a lower level of lysine and its catabolite, 5-aminopentanoate, in serum samples of stroke risk patients from the test set which is in accordance with our previous result (Fig. 2). Similar results, as shown in Fig. 6c–e, were obtained for 2-oxoglutarate, L-valine, and ubiquinone. However, we could not detect L-homocysteine sulfinic acid in our validity population due to unknown reasons.
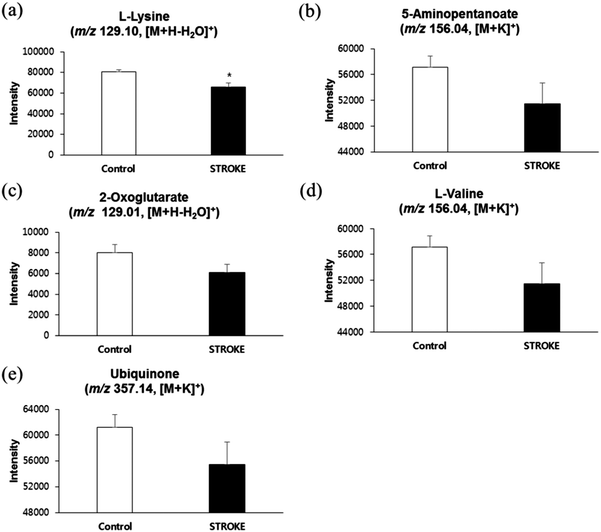 |
| Fig. 6 Relative concentrations of lysine and other candidate metabolites in test set (a–e) comparison of relative concentrations of our candidate biomarkers in control subjects and stroke risk patients from test set. L-Lysine (m/z 129.10, [M + H–H2O]+), (b) 5-aminopentanoate (m/z 156.04, [M + K]+), (c) 2-oxoglutarate (m/z 129.01, [M + H–H2O]+), (d) L-valine (m/z 156.04, [M + K]+) and (e) ubiquinone (m/z 357.14, [M + K]+). * Significant difference (p < 0.05). | |
4. Discussion
Using high resolution metabolomics in this retrospective cohort study, we identified specific serum metabolites in thrombotic stroke risk patients. In our analysis, we identified a small interrelated subset of metabolites. Our data demonstrate that reduction in concentrations of lysine catabolites is associated with stroke outcomes. Such an elevated catabolism process of lysine can increase production and uncontrolled activation of the excitatory neurotransmitter glutamate in postsynaptic membranes of neurons in the central nervous system.22,28,50 To our knowledge, this is the first cohort study to report association of lysine with stoke. However, further investigation is required to support our findings.
Glutamate is an excitatory amino acid and an important excitatory neurotransmitter in the central nervous system. Previously, several studies in animal models of cerebral ischemia have shown elevated levels of glutamate.16,51,52 Excitotoxity caused by such an elevated level of glutamate triggers oxidative stress through activation of nitric oxide synthase, inflammation through expression of inflammatory cytokines, and endothelial damage through matrix metalloproteinases (MMP) release.4 For instance, glutamate production with shorter survival has been shown as a diagnostic biomarker during cerebral ischemia.15 In our analysis however, we could not detect glutamate in serum samples obtained from thrombotic stroke risk patients, but we detected lysine as an important precursor of glutamate at the early neurological deterioration; as previously, lysine was shown as a substrate of glutamate in human brain tissues.28 Our data show that four different lysine pathways were affected in stroke risk patients, based on data from KEGG and Mummichog, and all those pathways interestingly involve the conversion of 2-oxoglutarate to glutamate during catabolism of lysine. Furthermore, in each of the catabolic pathways, nicotinamide is utilized as a source of energy. Our findings showed lower concentrations of lysine catabolites and nicotinamide in thrombotic stroke risk patents, in accordance with previous studies, which showed that lysine is efficiently catabolized during stroke for production of glutamate (Fig. 7).
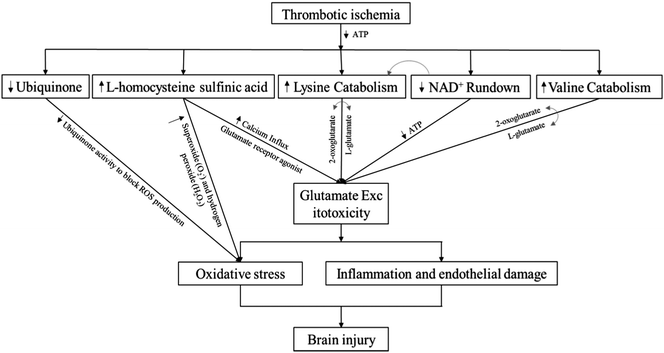 |
| Fig. 7 Schematic diagram of identified interrelated subsets of metabolites and their impact on stroke events. | |
The increased risk for diabetes in smoking men and women is around 50%.53 Many patients with type 1 and type 2 diabetes mellitus are also at risk for macrovascular complications, coronary heart disease (CHD), stroke, and peripheral vascular disease.53 Recently, branched-chain and aromatic amino acids were shown to be highly associated with risk of developing diabetes.54 Cigarette smoking is associated with worsening of metabolic control in diabetic patients. In short, smoking and diabetes cause a multitude of metabolic changes in serum metabolites in adults. However, whether it was exposure or effect of smoking or a surrogate of the DM, our results found negligible effects of these factors with lysine and valine catabolism in stroke risk patients. Diabetic, as well as non-diabetic subjects who were at risk of development of stroke, showed similar (low) intensities of lysine and lysine catabolites. Similarly, there was no difference observed between lysine levels of smokers or non-smokers.
In addition to the novel lysine metabolites, we found that homocysteine sulfinic acid levels increased significantly in stroke risk patients, as compared with those in the control, which is concordant with the well-described oxidative stress phenomena during stroke.42 Hyperhomocysteinemia may lead to thrombotic vascular disease through its role in oxidative injury to vascular endothelial cells, impaired production of NO, and adhesion of platelets to endothelial cells.55 Animal studies have shown that abnormal activation of glutamate receptors (mGluR) can cause oxidative injuries in cerebral ischemia by provoking an exacerbated NMDA-induced excitotoxicity.56 However, this phenomenon was not observed in mGluR1 knockout mice, and an additional study showed that noncompetitive mGluR5 antagonists can inhibit NMDA-induced apoptosis in primary cortical neuron cultures, thereby indicating the involvement of mGluR.57,58 Interestingly, screening for acidic homocysteine derivatives, especially L-homocysteine sulfinic acid, showed that these derivatives can potently and specifically activate mGluRs expression in cloned rats in vitro.59 Thus, at sufficiently high concentrations, acidic homocysteine derivatives can modulate activities of mGluRs, predisposing individuals to the neurotoxic consequences of stroke. Herein, measurement of L-homocysteine sulfinic acid levels supported this hypothesis, and we observed an elevated concentration of L-homocysteine sulfinic acid in serum samples of stroke risk patients, as compared to those of control group patients. This result indicates an important role of the acidic homocysteine derivative for predisposing stokes in risk patients.
Several factors, including smoking, contribute to increased plasma homocysteine levels.60 However, our study showed a negligible role of smoking with elevation of L-homocysteine sulfinic acid levels in stroke risk patients. Furthermore, levels of ubiquinone, a naturally occurring anti-oxidant, were also reduced in stroke risk patients, which, along with elevated homocysteine levels, promote oxidative stress during stroke events and may lead to cell damage.49 Thus, our findings support the results from previous studies that homocysteine and ubiquinone act as oxidative stress biomarkers during a stroke (Fig. 7). We also observed a significantly lower intensity of valine in stroke risk patients in comparison with the non-stroke control (ESI,† Fig. S4). This is in accordance with a previous study where reductions in levels of branched chain amino acids (valine, leucine, and isoleucine) were observed in plasma and CSF samples obtained from patients with acute stroke vs. control, and such reduction in the concentrations of branched chain amino acids was shown to be associated with stroke severity and worse neurological outcomes.61
Fig. 7 represents the schematic diagram of our identified interrelated subset of metabolites, based on their impacts on stroke events. A decrease in ubiquinone level inhibits the suppression of reactive oxygen species (ROS), resulting in oxidative stress.62 Increase in homocysteine sulfinic acid level increases calcium influx,63 and acts as a glutamate receptor agonist, thereby increasing glutamate excitotoxicity.44 Furthermore, hyperhomocysteinemia results in oxidative stress.64 Lysine or valine catabolism pathways involve conversion of 2-oxoglutarate to glutamate, thereby enhancing glutamate excitotoxity,28,61 while NAD+ is utilized in the catabolic pathway of lysine.28 On the basis of correlation patterns of our identified metabolites with lysine (ESI,† Fig. S6) and their impact on stroke events, we now know that low levels of lysine and its metabolites, along with elevated level of homocysteine sulfinic acid, can synergize to initiate excitotoxity in stroke risk patients. However, these results should be validated with a larger stroke population at early stages of stroke, instead of those at risk.
5. Conclusions
In summary, our study showed that lysine degradation metabolites are novel biomarkers for a stroke, which may not only help in identifying patients at risk for stroke, but can also help in understanding the pathophysiology of glutamate excitotoxity during a thrombotic stroke. In addition, in support of previous studies, homocysteine sulfinic acid, ubiquinone, and valine were also observed as potential biomarkers for an ischemic stroke. Moreover, these metabolite biomarkers are specifically related to stroke occurrence, and are not related to other factors such as DM or smoking. Thus, they can be used for early stage disease risk identification, early diagnosis, pathological mechanism research, and drug target screening. Further studies are required to validate and generalize the applicability of lysine as a novel biomarker in the management of ischemic stroke patients.
Author contributions
YHP, LY, and AK designed the study. YHP and LY carried out the metabolomics experiments and comprehensive data analysis, while SH and SHJ organized the cohort study. YHP and AK prepared the manuscript. All authors read and approved the final manuscript.
Conflicting interests
The authors declare no conflict of interest in this study.
Funding
This research is financially supported by Korea Health Industry Development Institute grant (HI14C2686) from Korea University.
Acknowledgements
We thank the Korean Cancer Prevention Study-II (KCPS-II) biobank, South Korea, for providing us with study samples. We thank Carl Medriano for insights and comments on the manuscript. We thank Karan Uppal at Emory University for providing software to run the analysis. We thank the Korea Health Industry Development Institute grant (HI14C2686) by Korea University for financially supporting this research.
References
- U. Dirnagl, C. Iadecola and M. A. Moskowitz, Trends Neurosci., 1999, 22, 391–397 CrossRef CAS PubMed.
- K. Dettmer, P. A. Aronov and B. D. Hammock, Mass Spectrom. Rev., 2007, 26, 51–78 CrossRef CAS PubMed.
- H. Zetterberg, D. H. Smith and K. Blennow, Nat. Rev. Neurol., 2013, 9, 201–210 CrossRef CAS PubMed.
- M. Castellanos and J. Serena, Cerebrovasc. Dis., 2007, 24(Suppl 1), 7–15 CrossRef CAS PubMed.
- M. A. Reynolds, H. J. Kirchick, J. R. Dahlen, J. M. Anderberg, P. H. McPherson, K. K. Nakamura, D. T. Laskowitz, G. E. Valkirs and K. F. Buechler, Clin. Chem., 2003, 49, 1733–1739 CAS.
- J. L. Johnson, Prog. Neurobiol., 1978, 10, 155–202 CrossRef CAS PubMed.
- F. Nishimura, M. Nishihara, M. Mori, K. Torii and M. Takahashi, Brain Res., 1995, 691, 217–222 CrossRef CAS PubMed.
- R. P. Simon, J. H. Swan, T. Griffiths and B. S. Meldrum, Science, 1984, 226, 850–852 CAS.
- D. W. Choi, J. Y. Koh and S. Peters, J. Neurosci., 1988, 8, 185–196 CAS.
- C. L. Crowley, C. M. Payne, H. Bernstein, C. Bernstein and D. Roe, Cell Death Differ., 2000, 7, 314–326 CrossRef CAS PubMed.
- M. Yuan, C. Siegel, Z. Zeng, J. Li, F. Liu and L. D. McCullough, Exp. Neurol., 2009, 217, 210–218 CrossRef CAS PubMed.
- E. H. Lo, P. Bosque-Hamilton and W. Meng, Stroke, 1998, 29, 830–836 CrossRef CAS PubMed.
- P. Belenky, K. L. Bogan and C. Brenner, Trends Biochem. Sci., 2007, 32, 12–19 CrossRef CAS PubMed.
- J. Serena, R. Leira, J. Castillo, J. M. Pumar, M. Castellanos and A. Davalos, Stroke, 2001, 32, 1154–1161 CrossRef CAS PubMed.
- A. Davalos, J. Castillo, J. Serena and M. Noya, Stroke, 1997, 28, 708–710 CrossRef CAS PubMed.
- K. Takagi, M. D. Ginsberg, M. Y. Globus, W. D. Dietrich, E. Martinez, S. Kraydieh and R. Busto, J. Cereb. Blood Flow Metab., 1993, 13, 575–585 CrossRef CAS PubMed.
- M. Smriga, S. Ghosh, Y. Mouneimne, P. L. Pellett and N. S. Scrimshaw, Proc. Natl. Acad. Sci. U. S. A., 2004, 101, 8285–8288 CrossRef CAS PubMed.
- M. Smriga, M. Kameishi, H. Uneyama and K. Torii, J. Nutr., 2002, 132, 3744–3746 CAS.
- K. Omura, N. Yamanaka, S. Higami, O. Matsuoka and A. Fujimoto, Pediatrics, 1976, 57, 102–105 CAS.
- K. K. Ebenezar, V. Sathish and T. Devaki, Mol. Cell. Biochem., 2003, 247, 163–169 CrossRef CAS PubMed.
- E. Morikawa, Z. Huang and M. A. Moskowitz, Am. J. Physiol., 1992, 263, H1632–1635 CAS.
- T. Kondoh, M. Kameishi, H. N. Mallick, T. Ono and K. Torii, Front. Integr. Neurosci., 2010, 4, 18 Search PubMed.
- T. Kato, M. Sano and N. Mizutani, Eur. J. Pediatr., 1987, 146, 56–58 CrossRef CAS PubMed.
- M. Smriga and K. Torii, Nutr. Neurosci., 2003, 6, 125–128 CrossRef CAS PubMed.
- M. Smriga, T. Ando, M. Akutsu, Y. Furukawa, K. Miwa and Y. Morinaga, Biomed. Res., 2007, 28, 85–90 CrossRef CAS PubMed.
- Y. F. Chang, J. Neurochem., 1978, 30, 355–360 CrossRef CAS PubMed.
- Y. F. Chang, Biochem. Biophys. Res. Commun., 1976, 69, 174–180 CrossRef CAS PubMed.
- F. Papes, M. J. Surpili, F. Langone, J. R. Trigo and P. Arruda, FEBS Lett., 2001, 488, 34–38 CrossRef CAS PubMed.
- J. M. Johnson, T. Yu, F. H. Strobel and D. P. Jones, Analyst, 2010, 135, 2864–2870 RSC.
- A. Lozano, M. A. Martinez-Uroz, M. J. Gomez-Ramos, M. M. Gomez-Ramos, M. Mezcua and A. R. Fernandez-Alba, Anal. Bioanal. Chem., 2012, 402, 935–943 CrossRef CAS PubMed.
- S. K. Cribbs, Y. Park, D. M. Guidot, G. S. Martin, L. A. Brown, J. Lennox and D. P. Jones, AIDS Res. Hum. Retroviruses, 2014, 30, 579–585 CrossRef CAS PubMed.
- J. R. Roede, K. Uppal, Y. Park, K. Lee, V. Tran, D. Walker, F. H. Strobel, S. L. Rhodes, B. Ritz and D. P. Jones, PloS One, 2013, 8, e77629 CAS.
- C. A. Smith, G. O'Maille, E. J. Want, C. Qin, S. A. Trauger, T. R. Brandon, D. E. Custodio, R. Abagyan and G. Siuzdak, Ther. Drug Monit., 2005, 27, 747–751 CrossRef CAS PubMed.
- H. Ogata, S. Goto, K. Sato, W. Fujibuchi, H. Bono and M. Kanehisa, Nucleic Acids Res., 1999, 27, 29–34 CrossRef CAS PubMed.
- S. Li, Y. Park, S. Duraisingham, F. H. Strobel, N. Khan, Q. A. Soltow, D. P. Jones and B. Pulendran, PLoS Comput. Biol., 2013, 9, e1003123 CAS.
- Y. H. Park, Y. P. Shi, B. Liang, C. A. Medriano, Y. H. Jeon, E. Torres, K. Uppal, L. Slutsker and D. P. Jones, Malar. J., 2015, 14, 122 CrossRef PubMed.
- F. Schoonjans, A. Zalata, C. E. Depuydt and F. H. Comhaire, Comput Methods Programs Biomed, 1995, 48, 257–262 CrossRef CAS PubMed.
- M. D. Ruopp, N. J. Perkins, B. W. Whitcomb and E. F. Schisterman, Biom. J., 2008, 50, 419–430 CrossRef PubMed.
- R. De Sotto, C. Medriano, Y. Cho, K. S. Seok, Y. Park and S. Kim, Ecotoxicol. Environ. Saf., 2016, 127, 127–134 CrossRef CAS PubMed.
- V. V. Krylov, S. S. Petrikov, A. Solodov, S. A. Badygov and E. D. Mekhia Mekhia, Intensive Care Med. Exp., 2015, 3, A853 CrossRef.
- T. Okura, K. Miyoshi, J. Irita, D. Enomoto, T. Nagao, M. Kukida, A. Tanino, K. Kudo, Z. Pei and J. Higaki, Sci. Rep., 2014, 4, 5663 CAS.
- G. J. Hankey and J. W. Eikelboom, Curr. Opin. Neurol., 2001, 14, 95–102 CrossRef CAS PubMed.
- J. H. Yoo, C. S. Chung and S. S. Kang, Stroke, 1998, 29, 2478–2483 CrossRef CAS PubMed.
- Q. Shi, J. E. Savage, S. J. Hufeisen, L. Rauser, E. Grajkowska, P. Ernsberger, J. T. Wroblewski, J. H. Nadeau and B. L. Roth, J. Pharmacol. Exp. Ther., 2003, 305, 131–142 CrossRef CAS PubMed.
- A. Cherubini, M. C. Polidori, M. Bregnocchi, S. Pezzuto, R. Cecchetti, T. Ingegni, A. di Iorio, U. Senin and P. Mecocci, Stroke, 2000, 31, 2295–2300 CrossRef CAS PubMed.
- I. Margaill, M. Plotkine and D. Lerouet, Free Radical Biol. Med., 2005, 39, 429–443 CrossRef CAS PubMed.
- M. F. Beal, R. T. Matthews, A. Tieleman and C. W. Shults, Brain Res., 1998, 783, 109–114 CrossRef CAS PubMed.
- C. W. Shults, M. F. Beal, D. Fontaine, K. Nakano and R. H. Haas, Neurology, 1998, 50, 793–795 CrossRef CAS PubMed.
- J. S. Leinonen, J. P. Ahonen, K. Lonnrot, M. Jehkonen, P. Dastidar, G. Molnar and H. Alho, Stroke, 2000, 31, 33–39 CrossRef CAS PubMed.
- J. C. Watkins and H. J. Olverman, Trends Neurosci., 1987, 10, 265–272 CrossRef CAS.
- J. Castillo, A. Davalos, J. Naveiro and M. Noya, Stroke, 1996, 27, 1060–1065 CrossRef CAS PubMed.
- M. Y. Globus, R. Busto, E. Martinez, I. Valdes and W. D. Dietrich, Stroke, 1990, 21, III43–III46 CAS.
- B. Eliasson, Prog. Cardiovasc. Dis., 2003, 45, 405–413 CrossRef CAS PubMed.
- T. J. Wang, M. G. Larson, R. S. Vasan, S. Cheng, E. P. Rhee, E. McCabe, G. D. Lewis, C. S. Fox, P. F. Jacques, C. Fernandez, C. J. O'Donnell, S. A. Carr, V. K. Mootha, J. C. Florez, A. Souza, O. Melander, C. B. Clish and R. E. Gerszten, Nat. Med., 2011, 17, 448–453 CrossRef CAS PubMed.
- L. A. Harker, R. Ross, S. J. Slichter and C. R. Scott, J. Clin. Invest., 1976, 58, 731–741 CrossRef CAS PubMed.
- G. L. Collingridge and R. A. Lester, Pharmacol. Rev., 1989, 41, 143–210 CAS.
- A. G. Mukhin, S. A. Ivanova and A. I. Faden, Neuroreport, 1997, 8, 2561–2566 CrossRef CAS PubMed.
- F. Ferraguti, C. Pietra, E. Valerio, C. Corti, C. Chiamulera and F. Conquet, Neuroscience, 1997, 79, 1–5 CrossRef CAS PubMed.
-
S.
Ramakrishnan
,
K. N.
Sulochana
,
S.
Lakshmi, R. Selvi and N. Angayarkanni, Indian J. Biochem. Biophys., 2006, 43, 275–283 Search PubMed.
- P. O'Callaghan, R. Meleady, T. Fitzgerald and I. Graham, Eur. Heart J., 2002, 23, 1580–1586 CrossRef.
- W. T. Kimberly, Y. Wang, L. Pham, K. L. Furie and R. E. Gerszten, Stroke, 2013, 44, 1389–1395 CrossRef CAS PubMed.
- D. Lee, M. S. Shim, K. Y. Kim, Y. H. Noh, H. Kim, S. Y. Kim, R. N. Weinreb and W. K. Ju, Invest. Ophthalmol. Visual Sci., 2014, 55, 993–1005 CAS.
- P. I. Ho, D. Ortiz, E. Rogers and T. B. Shea, J. Neurosci. Res., 2002, 70, 694–702 CrossRef CAS PubMed.
- J. Loscalzo, J. Clin. Invest., 1996, 98, 5–7 CrossRef CAS PubMed.
Footnotes |
† Electronic supplementary information (ESI) available: Fig. S1–S5 and Table S1. See DOI: 10.1039/c6mb00732e |
‡ These authors contributed equally to this work. |
|
This journal is © The Royal Society of Chemistry 2017 |
Click here to see how this site uses Cookies. View our privacy policy here.