DOI:
10.1039/C6IB00215C
(Review Article)
Integr. Biol., 2017,
9, 97-108
Next generation of network medicine: interdisciplinary signaling approaches
Received
24th October 2016
, Accepted 9th January 2017
First published on 16th January 2017
Abstract
In the last decade, network approaches have transformed our understanding of biological systems. Network analyses and visualizations have allowed us to identify essential molecules and modules in biological systems, and improved our understanding of how changes in cellular processes can lead to complex diseases, such as cancer, infectious and neurodegenerative diseases. “Network medicine” involves unbiased large-scale network-based analyses of diverse data describing interactions between genes, diseases, phenotypes, drug targets, drug transport, drug side-effects, disease trajectories and more. In terms of drug discovery, network medicine exploits our understanding of the network connectivity and signaling system dynamics to help identify optimal, often novel, drug targets. Contrary to initial expectations, however, network approaches have not yet delivered a revolution in molecular medicine. In this review, we propose that a key reason for the limited impact, so far, of network medicine is a lack of quantitative multi-disciplinary studies involving scientists from different backgrounds. To support this argument, we present existing approaches from structural biology, ‘omics’ technologies (e.g., genomics, proteomics, lipidomics) and computational modeling that point towards how multi-disciplinary efforts allow for important new insights. We also highlight some breakthrough studies as examples of the potential of these approaches, and suggest ways to make greater use of the power of interdisciplinarity. This review reflects discussions held at an interdisciplinary signaling workshop which facilitated knowledge exchange from experts from several different fields, including in silico modelers, computational biologists, biochemists, geneticists, molecular and cell biologists as well as cancer biologists and pharmacologists.
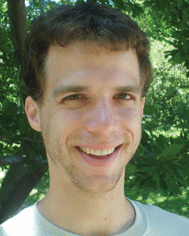
Tamas Korcsmaros
| Tamas Korcsmaros, is a Fellow at the Earlham Institute and the Institute of Food Research. Tamas combines computational and wet lab approaches to study signaling networks. He graduated at the Eotvos Lorand University (Hungary), where during his PhD years he established a research group, a bioinformatics training course and co-funded two network medicine companies. He organized several major conferences and workshops including the Interdisciplinary Signaling Workshop in 2014 together with Vicky Schneider and Giulio Superti-Furga. Using innovative approaches, this meeting provided a context for established scientists and early-stage researchers from different disciplines to discuss complex questions and suggest potential solutions. |
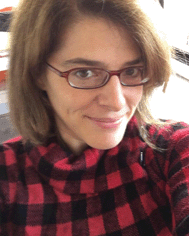
Maria Victoria Schneider
| Maria Victoria Schneider, is the Deputy Director of EMBL Australia Bioinformatics Resource (EMBL-ABR) and Associate Professor at the University of Melbourne, Australia. Vicky has a keen interest in data driven science, open science and best practice in data life cycle, tools and bioinformatics training. Previously, Vicky was part of the Earlham Institute Senior Management Team where she head the 361° Division and before that Vicky worked at EMBL-EBI. Prior to joining EMBL-EBI in 2007 Vicky held an Assistant Professor position at the University of Bern and EAWAG, with postdocs at the University of Zurich and University of Rome (Torvergata). |
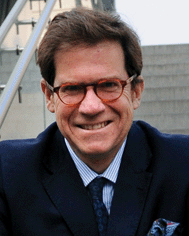
Giulio Superti-Furga
| Giulio Superti-Furga, is the Scientific Director of the Research Center for Molecular Medicine of the Austrian Academy of Sciences (CeMM), and Professor of Medical Systems Biology at the Medical University of Vienna. He performed studies in molecular biology at the University of Zurich, Genentech, and IMP Vienna, was post-doctoral fellow and Team Leader at EMBL, and co-founded the biotech companies Cellzome and Haplogen. His expertise is systems pharmacology. Among his scientific contributions are the elucidation of basic regulatory mechanisms of tyrosine kinases in human cancers, and the discovery of fundamental organization principles of the proteome and lipidome of higher organisms. |
Insight, innovation, integration
Network medicine approaches are inherently interdisciplinary, requiring a combination of computational and biological domain-specific knowledge and techniques. Despite initial expectations, network medicine has yet to have a major impact due to a lack of communication between the different disciplines required for success. Through an innovative 5-day workshop we explored and promoted the application of interdisciplinary approaches to the analysis of signaling networks. This review, inspired by the workshop, discusses literature illustrating the importance of multi-disciplinary approaches to problems of this kind, and identifies new opportunities for combining disciplines to further promote the impact of network medicine. To help improve access to such opportunities, we also provide advice and describe best practices for researchers aiming to deliver successful multidisciplinary projects in network medicine.
|
Introduction
A decade since the first proposal of the concept of network medicine,1 but except for a few landmark examples,2,3 network approaches are yet to revolutionize medicine. Despite an overall increase in both data quality and quantity, the number of fruitful systems-level projects is growing slowly compared to the number of newly available techniques. One possible reason for the delay in delivery of the promise offered by network medicine is the low number of truly multi-disciplinary projects and integrative approaches, involving scientists from different backgrounds.
More than ever, with the advent of high-throughput technologies and advances in bioinformatics and computational biology, signaling researchers are able to generate, access, analyze and interpret a variety of data-sets and integrate them at a scale not previously possible. This has contributed to the “big data problem” of signaling research and has led to the need of bringing expertise from different disciplines together. Experimentalists often do not have the necessary computational or systems biology background to exploit these new opportunities. Accordingly, they drown in data and concentrate on selected cases and more traditional analyses. Conversely, purely computational experts often lack the biological knowledge required to identify novel insights or design optimal studies, and often end up reporting on well-known phenomena. Involvement of diverse experts on the same project could help deliver better experimental design, data evaluation and interpretation.
In this review, we summarize and reflect on the impact of an interdisciplinary signaling workshop we organized in 2014 (http://2014.signalingworkshop.org) on supporting the promotion of multidisciplinary approaches to solve questions and problems in signaling biology. This review synthesizes multiple topics that are often the focus of dedicated reviews that would be published in corresponding specialist journal – by integrating these topics together in the same paper, we aim to help promote cross-disciplinary approaches and understanding.
Network medicine – a promising history
Albert-László Barabási1 introduced the term ‘network medicine’ to describe network-based approaches aiming to understand diseases at the systems-level. Likewise, Lemberger and Liu envisaged that ‘systems medicine is finally coming of age’.4 These authors suggested that networks can offer solutions for systems approaches on therapeutic and diagnostic innovation.5 Pawson and Linding extended the concept, and network medicine was defined as a pharmacological attempt that recognizes both the network connectivity and dynamics of signaling systems as components of drug targets.6 Aloy and colleagues identified protein–protein interactions in networks as suitable targets of drug-like compounds.7 Later, network medicine was described as a ‘think globally, act locally’ paradigm to understand disease phenotypes in order to develop local interventions that may cure a specific disease, but with a systems-level understanding of the cells’ global organization.8 Nowadays, network medicine is considered a resource for a variety of purposes: biomarker discovery, drug target prediction, drug side-effect analysis, drug repurposing and a tool to suggest new therapies.9 Recently, Menche and colleagues demonstrated the power of network approaches to understand disease pathogenesis.10 There are slightly different definitions to network medicine, often due to the diverse disciplines working on this topic. A collection of current definitions related to network medicine can be find in a review by Kirschner.11
Why network medicine requires interdisciplinary approaches?
Network medicine studies are typically cross-disciplinary as they require the combination of multiple experimental (in vivo, ex vivo, or in vitro) and computational (in silico) data and analyses.12 Network medicine datasets are often used for mathematical modeling and computational simulation approaches, in many cases integrating one or more levels of complex dynamics (DNA and epigenetics, RNA and transcriptomics, proteins and proteomics, interaction networks, organelles, cells, tissues, organs, etc.).13 The result of these modeling analyses often provide information that can be used to adapt the process of collecting biological measurements on the system, with new insights emerging through a process of iterative proposal of further new models on the basis of collection of additional data.14 Thus, successful systems-level network medicine studies require the combination of expertise from different disciplines. Ideally, experimental researchers would be able to understand and use network-based approaches to studying signaling networks, in the same way they apply diverse experimental approaches to these studies e.g. FACS and qPCR.15 Multidisciplinary collaborations provide expertise in information science, computational biology and mathematics to ensure that patient data collected by physicians and clinical researchers can readily be assimilated and processed by other researchers.16 Supporting this, our automated literature survey pointed out an increase in the number of disciplines used in signaling network related publications in the last 6 and previous 10 years (Fig. 1). However, from more than 22
000 papers, we found only a few dozens mentioning more than four different approaches indicating a low though growing number of interdisciplinary studies. Here we list a few challenges as examples of why network medicine requires interdisciplinary signaling researchers and multidisciplinary collaboration of different experts.
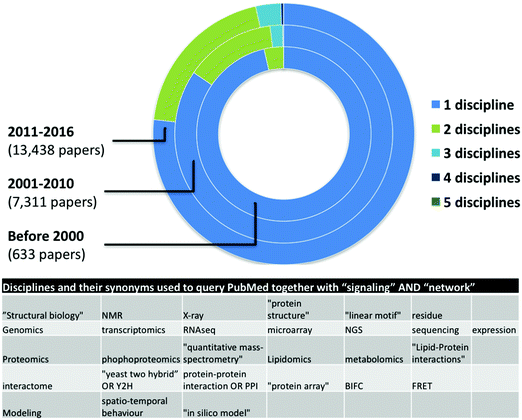 |
| Fig. 1 Number of papers with the words “signalling” AND “network” in PubMed abstracts. In January 2017, we measured the co-occurrences of different disciplines, like structural biology, genomics/transcriptomics, proteomics, modeling, and interactomics. We used 4–5 synonym keywords for each discipline (see table inset). This is not an exhaustive list but shows a clear trend. Though it is not visible in the figure, four different disciplines were mentioned in nine papers published between 2001–2010, and 45 papers published after 2011, while all the five disciplines were mentioned in one paper published between 2001–2010 and in two papers published after 2010. | |
Understanding the evolution, structure and mechanisms of normal signaling networks can point out components that play key roles in diseases.17 In many cases, these components have no direct relation to a particular disease but their stimulation or inhibition can have beneficial systems-level effect on the cellular network in normal cells, and leading to indirect benefits. For example, pharmacological modulation of key proteins of the signaling network can influence the robustness of the cells for therapeutic purposes, increasing robustness in healthy cells and decreasing robustness in cancerous cells during chemotherapy.18 In the early era of network biology, hubs (components of the signaling network having many interactions) received great interest as key components of the network.19 This was in part because such hubs, in protein–protein networks, were often well-characterized proteins. Several systems-level studies highlighted that hubs could be classified based on key dynamic features, such as specific binding interfaces or co-expressed interacting partners within a tissue or cell compartment.20,21 Studies on other, more global network parameters, such as the betweenness centrality (the average number of shortest paths going through the protein) showed the importance of bottleneck proteins, having sometimes low number of interactors but bridging two otherwise distinct parts of the network.22 Identification of key proteins necessitates detailed, well-curated signaling maps, integrated with dynamic (i.e., cell and context-specific) expression data and investigated by using advanced network analysis methods.9 One of the reasons to apply all these approaches is the fact that many of the post-translational modifications of proteins are stochastic, complicating the analysis of functional consequences. Developing and executing these complex workflows, understanding the structural biology, cell specificity and data analysis background as well as performing advanced network analyses are no longer simple protocols that can be found in a single textbook or learned in one training course.
Studying diseased signaling networks is also in the major focus of network medicine. Gene expression and sequencing studies on pathologically altered signaling networks has uncovered possible drug targets whose malfunction directly causes a disease. For example, during tumorigenesis when cells acquire the capability of continuous division and an often increased mutation rate,23 most of the (driver) mutations affect a limited number of central pathways.24 The use of network medicine tools and interdisciplinary approaches are essential if we aim to understand the systems-level change of the signaling network in later stages of tumorigenesis.25 Such pathological rewiring allows the appearance of other cancer hallmarks, including sustained angiogenesis and metastatic tissue invasion capabilities,26 as well as the deregulation of cellular metabolism and circumvention of immune responses.27 Tumor microenvironment is another key area where network approaches could offer valuable insights. For example intercellular networks between surface and secreted proteins have shown how certain fibroblasts turn into tumor growth helping, cancer associated fibroblasts.28 A key challenge for cancer related network medicine is to overcome the difficulties of therapeutically treating a pathologically rewired signaling network.29 In contrast to driver genes, functional analyses of GWAS data or gene enrichment studies are not enough to identify proteins essential in rewiring. Several publications have shown that changes in cross-talking (i.e., multi-pathway) proteins are important for the rewiring of signaling networks.25,30,31 Mutation even in one multi-pathway protein can have a systems-level effect as it can significantly alter the signaling flow, for example, by transducing a ‘death’ signal to a ‘survival’ transcription factor.26,32,33
Identification of multi-pathway proteins is a challenging task, requiring multi-disciplinary knowledge and detailed systems-level understanding of the cell type of interest. Accordingly, numerous drug developmental failures are unfortunately known where the targeted protein had undiscovered or underestimated cross-talk effects.34,35 Another challenge is that targeting a cross-talking protein may have an opposite effect in healthy cells as their signaling networks behave differently.18 On the contrary, pharmacological modification of a cross-talking protein in cancer therapy is not always useful as it may strengthen the robustness of cancer cells instead of weakening them.18,36 Similarly, targeting proteins in feedback loops can also have disadvantageous system effects if the signaling circuits of the target protein are not well explored.37 For example, a drug suppressing a negative feedback loop could, paradoxically, activate the targeted pathway.38 In conclusion, uncovering the systems properties of multi-pathway and feedback-associated proteins may facilitate the development of efficient, network pharmacological interventions.8,39 Identification of these promising target proteins requires the combination of structural biology, ‘omics’ datasets and advanced bioinformatics analyses or network modeling.
Network medicine of proteins
Enzymes and malfunctions of enzymes (enzymopathies) have been in the focus of medical biochemistry approaches for a long time. Recently, systems-level features of enzymes have been providing data and insight into the changes in signaling networks.40
Kinases
Kinases are one of the key enzymes in signaling cross-talk,33 and traditionally among the most pharmacologically targeted proteins of the cellular signaling networks.6 Kinase domains and their target motifs (i.e., specific aminoacid sequences in the substrate proteins) are well-known and comprehensively compiled in resources such as Phosphosite and dbPAF,41,42 computational algorithms, like NetworKIN and NetPhorest43,44 provide in silico resources of phosphorylation-modulated interaction networks. A proof-of-concept approach earlier showed that a complementary effort using both experimental validation and computational analysis is can successfully refine a phosphorylation network by combining linear phosphorylation motifs with protein–protein interactions, kinase-substrate co-expression, protein co-localizations and general pathway databases.43 Combining these in silico models with high-throughput, phospho-proteomic approaches has been shown to provide insights into signaling biology not only at a systemic, high-throughput level qualitatively but also quantitatively.45 Systems-level models of JNK and EphR signaling networks are examples of two such integrative approaches46,47 among others. Comparative phospho-proteomics have been connected to network evolution models to improve our understanding on the normal development and malignant alterations of signaling proteins.48,49 Linding and colleagues recently incorporated genomic sequencing data from next-generation sequencing (NGS) studies to predict the impact of disease mutations on cellular signaling networks.50,51
Phosphatases
It is important to highlight that less attention has been taken on the other player of the phosphorylation system: the protein phosphatases. As reviewed by Kholodenko and colleagues,52 protein phosphatases can play a dominant role in determining the spatio-temporal behavior of protein phosphorylation systems in the cell as both immediate and delayed negative regulators. Thus, pharmacological targeting of phosphatases can modify the signaling network at the systems-level. Despite their promising effect and high number, only a few protein tyrosine phosphatases are currently used as therapeutic targets.53,54 As a systems-level approach to aid this situation, Cesareni and colleagues55 compiled a cell specific phosphatome for growth pathways. They combined high-content siRNA screening that identified cell state specific phosphatases with pathway knowledge to perform a computational optimization approach based on the CellNOpt modeling tool.56 This effort resulted in a map of 41 phosphatases that affect key growth pathways and pointed out key cross-talking functions.55
Motifs
Kinases, phosphatases and other enzymes post-translationally modify their substrates based on a specific binding to target and/or protein regulatory motifs. Such motifs are typically short (less than ten residues) and often occur within intrinsically disordered regions.57 The ELM database collects and stores the most comprehensive repository of experimentally characterized peptide motifs.58 Prediction of binding motifs at the proteome level can be performed by using in silico tools like ANCHOR, which analyzes the sequence of a disordered region and estimates the energy of interaction of such a region with a general interaction partner.59 Combining interaction prediction, disordered region analysis and cancer related expression studies showed the importance of these approaches in examining the role of heat shock protein in signaling networks.60 Site-specific mutation analysis can provide validation of the functionality of predicted linear motifs.57 As linear motif-based interactions are sensitive to the expression of their binding partners, careful choice of experiments is necessary to avoid incorrect inferences about their functional relevance.61 The importance of short linear motifs from a network medicine perspective has been shown by Uyar et al. where the number of interactions mediated by a linear motif correlates with the likelihood that a mutation affecting that motif will be disease-related.62
Scaffold proteins
Scaffold proteins determine signaling flows and regulate cross-talks between pathways by binding and co-localizing with at least two members of one or more signaling pathways.63 Scaffolds can be efficient active members of a signaling circuit (i.e., a feed-back or feed-forward loop) by changing their own conformation upon repeated signaling events or allosterically modifying the enzymes binding to them.63,64 The effect of these modifications can range from small fine-tunings to significant changes in the signaling flow that activates different downstream events.65 It is not surprising that many scaffold proteins were found to be involved in the oncogenic rewiring of the signaling network66 and their mutation is implicated in different cancers, like colorectal cancer as in the case of AXIN.67 Despite the growing number of specific examples of the importance of scaffolds proteins in cellular networks,68,69 the scale and complexity of the current experimental approaches are not sufficient to explore the systems-level role of scaffold proteins in different disease states.70
Omics in network medicine
In the last two decades, omics technologies have made a great impact on medical research, turning biological research into a data-intensive science.71,72 These high-throughput methodologies are now routinely used to provide a top-down approach for understanding biological systems.73,74 The power of an omics approach in network medicine is due to its ability to detect context (e.g., cell, disease or treatment) specific data associated with signaling systems. The challenge of these approaches is that they often require either a computational biology expert or familiarity with sophisticated computational software solutions to extract biological insights from these data sets.75 A further complication is that genomic or transcriptomic data are often best interpreted in the context of the heterogeneous large-scale data sets that have already been deposited in publicly available repositories.75 Omics technologies can be found in an increasing number among the top-down approaches.76 Here, we present briefly genomics and lipidomics as selected examples of these approaches demonstrating their multi-disciplinary nature. We note that there are other relevant approaches that we are not highlighting here, such as metabolomics and phenomics, while proteomics has been mentioned in the previous section.
Genomics
Genomic approaches provide the highest number and variety of datasets on human diseases. These approaches include (1) whole-genome or exome sequencing identifying genetic mutations or copy number variations; (2) genome-wide association studies (GWASs) used to identify genetic variants associated with a disease; (3) microarray or RNAseq techniques for measuring the mRNA or miRNA expression of cells, comparing levels between states (transcriptomics); and (4) epigenomics analyses focusing on, for example, DNA methylation and its change during differentiation, ageing and cancer progression.75 Network medicine provides a highly effective framework to analyze genomic datasets and better understand complex diseases. Disease related genes may differ among affected individuals, but the affected pathway or network region is likely to be shared.75
Nowadays, next-generation sequencing on the single-cell level provides an opportunity to analyze networks in cancer cells based on data obtained from individual clones.71 To acquire the benefits of noise reduction, and incorporation on data tumor heterogeneity, that can be achieved from such single-cell approaches, requires sophisticated sample preparations from clinical researchers, specific sorting and sequencing infrastructures as well as advanced data evaluation tools, statistics and improved visualization solutions.77 For example, Bates and co-workers were able with a systems-level approach to validate a set of published prognostic biomarkers, and assess their effectiveness relative to known levels of intra-tumor heterogeneity for a cohort of clear cell renal cell carcinoma (ccRCC) patients.78
RNA-interference (RNAi) studies can provide valuable insights into the functional role and relevance of specific genetic variations or mutation. However, similarly to some compound based perturbation assays, RNAi experiments are often confounded by “off-target” effects due to signaling cross-talk and feedback processes.79 A possible solution is to interpret RNAi results in a network framework instead of focusing on single hits.79,80 Today, genetic engineering using the CRISPR-Cas9 system, can offer complementary validation strategies.81
Lipidomics
Lipidomics, the large-scale detection of protein–lipid interactions, is a recent and increasingly important ‘omics’ approach for building knowledge about the structure and function of signaling networks in healthy and diseased states. This has revealed the importance of altered lipid–protein interactions in regulatory circuits in several disorders. These interactions are attractive targets for pharmaceutical drug development. Challenges associated with detection of such interactions, especially at large scales, are being overcome by adopting chemical biology approaches to characterize in vivo assembled, stable protein–lipid complexes.82 A recent liposome microarray-based assay (LiMA) measures protein recruitment to membranes in a quantitative, automated, multiplexed and high-throughput manner.82 LiMA was recently used for large-scale testing of the lipid binding specificity of pleckstrin homology (PH) domains, using liposome arrays containing 122 different types of liposomes.82,83 Major signaling data repositories contain relatively few protein–lipid interactions, making network analysis of these interactions either very challenging, requiring extensive additional experimental work to characterize many additional interactions, or too simple, due to lack of existing open data on such interactions to build networks containing enough interactions to provide an accurate-enough description of cellular networks.84 Despite these issues, interest remains high in such approaches, as it is expected that network models for diseases where the actual change happens on the lipid level may provide many important insights into disease biology.85 An important success story for network approaches using lipidomics was recently reported,86 based on perturbation of cell membrane lipid metabolism by gene inactivation of macrophage enzymes involved in sphingomyelin/ceramide metabolism and quantitative analysis of 245 lipids. Network analysis revealed an unsuspected circular network structure that was reflective and predictive of the ability of mouse macrophages, and fibroblasts from patients suffering from lipid storage disorders, to mount an inflammatory response. This circular network, unprecedented among biological networks of co-regulated molecules, is evolutionarily conserved and likely to reflect not only biosynthetic relationships but also rapid homeostatic adaptation of perturbation. Without network analysis, these co-regulatory principles of cell membrane lipids would not have been discovered.
Modeling approaches in network medicine
Regardless of the focus and scale of the ‘omics’ approach or the structural and topological details of the signaling network, it is generally agreed that computational modeling is required to understand the dynamic behavior of a system. There are numerous reviews summarizing modeling methods for analyzing signaling networks (e.g.,15,87–91). Given that this topic is already so extensively reviewed elsewhere, here we only focus on multi-disciplinary aspects of the topic, listing a few specific examples of multi-disciplinary. For more general overviews of this topic, please refer to the reviews listed above.
Commonly-applied modeling approaches used to analyze cellular signaling include differential equation modeling, data driven modeling (such as clustering, principal components analysis, or partial least squares), modular response analysis, Boolean networks, and Bayesian inference modeling.87,88 These approaches have emerged as standard tools for systems-level research in signaling networks to derive biological insights from large-scale experiments. The scientists able to make the greatest impact using these methods typically have experience both in experimental biology and computational approaches.15 Thus, modeling itself has become a multi-disciplinary approach. Modeling approaches have the greatest impact when they address a clear biological question, and where the experimental measurements required for the modeling method can be exploited to provide valuable feedback on how to deliver the computational aspects of the work.87 This feedback often involves the optimization of experimental methods for data generation to reduce experimental noise and the number of erroneous signals (e.g., false-positives and false-negatives) in large data sets.87
To provide an interface between modeling approaches and experimental data validation, in the last few years, integrated and multi-layered databases have been built.92 Databases such as SignaLink contain manually curated, integrated and predicted interaction data of signaling pathways, their regulators (e.g., scaffolds) and modifier enzymes (e.g., phosphatases), as well as transcriptional and post-transcriptional regulators.68 The SignaLink website (http://signalink.org) is specifically designed to be as accessible as possible for experimental researchers, making it easier for them to use and develop computational network models. These resources, due to the non-trivial but biologically meaningful connections they provide (e.g., miRNA regulating transcription factors coordinating more than one pathway as master regulators), are able to drive network medicine projects, focusing on drug discovery93,94 and novel disease gene identification.10 As multiple signaling network resources are available with different focuses and levels of granularity, it is often unclear which should be used, either alone or in combination. The recently launched OmniPath gateway (http://omnipathdb.org) contains a systematic analysis of public resources on literature-curated human signaling interactions to facilitate to application of these databases.95 OmniPath applies a duel approach to help both experimental and computational researchers by providing a ready-to-use integrated dataset and an interactive Python module for advanced users.95
In pioneering work using Boolean logic models for modeling of cellular signaling, Saez-Rodriguez and co-workers developed CellNOpt, a discrete logic modeling approach for analyzing responses of signaling networks to stimuli (e.g., drugs or compounds).56 They were able to refine and update the wiring of a hepatocyte signaling network by training a literature-curated network against experimental data. Refined network increased its predictive power, identifying several new interactions that improved the match of model to data. Importantly, these novel interactions were supported by literature or their own follow-up experiments.56 To further increase the success of building predictive logic models from prior knowledge, CellNOpt has been implemented as a Cytoscape plug-in, enabling non-computational users to access this software, and develop similar models.96
A promising application of logical mathematical models is to convert them to genetic interaction networks to increase our understanding in disease network phenotypes.97 Knowledge of genetic interactions in the context of a disease phenotype can provide important insights into possible personalized treatment strategies, particularly in cancer.97,98 Modeling the dynamics of signalling processes behind regulatory genomics and systems plasticity features of cancer cells increases our understanding how they can evolve into different phenotypes, invade other tissues and evade drug therapies.99 Calzone and colleagues (2015) suggested a methodology for converting a logical mathematical model with a set of initial conditions into the corresponding genetic interaction network characterizing the behavior of all single and double mutants in terms of phenotype probabilities.97 The predictive power of this integrative modeling has recently been experimentally validated through a multi-disciplinary collaboration.100
To employ complex modeling approaches, a single focused research group is not enough, as expertise and data from different disciplines are needed to apply these approaches effectively. A promising and potent solution may lie in crowdsourcing to construct and analyze signaling networks by outsourcing the task to a relevant community.101 An already successful analogy for a community-driven approach has been carried out with metabolic networks.102 Several groups worked together to create a consensus reconstruction of a comprehensive metabolic network (Recon2), which can be used for computational modeling.102 Using similar approaches for building signaling networks will hopefully have a major impact on our understanding of healthy systems, and their malfunction in diseased states. Recently, in the context of the HPN-DREAM network inference challenge, an effective crowdsourcing approach were published using phosphoprotein data from cancer cell lines as well as in silico data from a nonlinear dynamical model to score more than 2000 networks submitted by challenge participants.103
Interdisciplinary research successes in network medicine
Here we highlight examples where the combination of different disciplines already proved to be a success in various areas of network medicine.
Hepatotoxicity is a major cause of drug development failures in the pre-clinical, clinical and post-approval stages.104 Lauffenburger and colleagues found that hepatic cytotoxicity responses are regulated by a multi-pathway signaling network balance.105 Using a systems modeling approach, they explored the signaling pathways that underlie the connections among hepatocellular stress, inflammation and hepatotoxicity. Phosphoprotein signaling data of human hepatocytes showed that activity balance of intertwined pro-survival and pro-death pathways (AKT and MAPK, respectively) determined the outcome of the therapy.105 Fortunately, with in silico and in vivo studies, they found that therapeutic modulation of cross-talks between these pathways as well as specific pathway inhibitors could antagonize drug and inflammation-induced hepatotoxicity.105
Upon infection, viral-induced rewiring of host cells is the result of a highly complex interplay between viral and host proteins. Due to the limited size of the viral genomes, viruses hijack and manipulate host proteins to utilize any accessible host mechanism.106 Superti-Furga and colleagues performed a systems-level study to identify common and virus-specific strategies by investigating the host targets of viral proteins.3 Using viral open reading frames (viORF) from 30 viral species (such as Ebola, human cytomegalovirus, and hepatitis C), they experimentally identified 579 host targets. Computational and network analyses of the host targets pointed out clear distinctions in preferences between the different viral groups: RNA viruses targeted the JAK–STAT and chemokine signaling pathways, while DNA viruses targeted cancer related pathways, enriched with MAPK signaling components.3 They also highlighted and experimentally validated PIK3CA as a multi-pathway protein, controlling cross-talks between multiple pathways as a key viral target. With a complex in silico approach and mass-spectrometry measurements, they classified the viORFs according to their influence on the host network. This network-based approach identified viral-specific and general rewiring strategies, thus a rationale for selecting specific intracellular pathways for pan-viral or virus-specific antiviral therapies.3
Signaling rewiring is a critical step in tumorigenesis, and understanding how pharmacological treatments affect the rewired cellular systems is essential for the development of successful anti-cancer therapies.9 The team of Mike Yaffe integrated advanced computational modeling (such as principal component analysis and partial least-squares regression modeling) with multiple types of experimental data and found that the most effective strategy for killing aggressive triple-negative breast cancer cells in vitro and in vivo is a time- and order-dependent combination of the EGF receptor kinase inhibitor erlotinib followed by doxorubicin.2 They found that due to an oncogenic rewiring, the active EGFR-mediated signaling inhibits the apoptotic process through caspase-8, which minimizes the effect of DNA damage induced by doxorubicin. Simultaneous inhibition of EGFR (by erlotinib) and doxorubicin treatment did not modify the rate of cell death substantially. Computational analyses of signaling networks and phenotypes of drug-treated cells highlighted that subsequent addition of the drugs in well-defined intervals could significantly enhance the treatment efficacy.2 After they validated this in cell culture and mice experiments, they continued the investigation to understand the underlying cellular mechanisms. Quantitative high-throughput reverse-phase protein microarrays and quantitative western blotting were used to measure the levels or activation states of 35 signaling proteins within multiple signaling pathways at 12 time points following exposure to erlotinib and doxorubicin both individually and in combination. In order to evaluate the generated data, they constructed a multifactorial data-driven mathematical model relating signaling inputs to phenotypic outputs. In total, their data set comprised more than 45
000 measurements of molecular signals and 2000 measurements of cellular responses.2
When previewing the work of Yaffe and colleagues, Erler and Linding argued that the reason why the drug development field so far lacked such studies was due to two major reasons: (1) lack of systems approaches, with most studies focusing on individual targets and the identification of specific mutations; (2) only in the last decade has it become technologically feasible to monitor thousands of molecular signals and to integrate them computationally to gain biological insight into drug mechanisms and efficacy.6 We share this opinion, and additionally believe that such studies have been lacking due to a lack of researchers and research communities trained to work in multi-disciplinary teams.
The power of interdisciplinarity and how to achieve it
We are in a moment in history, when there is a shift towards studying cell–cell interactions in an organ or organs and actual organisms as well as their contextual ecosystems, rather than the traditional investigation of individual cells. Such studies require the integration of a variety of complementary approaches such as: (1) chemical genetics (random mutagenesis of the genomes of near-haploid cells); (2) functional analysis by focused gene inactivation (RNAi and genome editing); (3) mechanistic understanding with structural biology approaches (NMR, crystallography, computational predictions, etc.); (4) genomics and transcriptional profiling (including miRNA analysis); (5) functional and phospho-proteomics (affinity purification and mass spectrometry); (6) lipidomics (isolation and mass spectrometry analysis of lipid content and protein–lipid interactions); (7) computational network analysis; (8) in silico modeling of biological processes (logic modeling, differential equations, etc.); (9) chemical proteomics and compound screening; and finally (10) integration and validation with patient data from clinical practice (Fig. 2). Computational analysis of multi-parametric high-content imaging of cells and cell–cell interactions, including subcellular structures, shapes, “social” pattern, offers to link molecular data to phenotypic manifestations. With the combined and integrated use of these approaches we can identify (1) new targets for known drugs, (2) previously unknown mechanisms of drug resistance, (3) effector genes for the compounds (genes required for the drug to exert its action), (4) mechanisms of synergy between compounds, (5) mechanisms of drug transport, and in a few cases (6) new medical use of existing drugs.
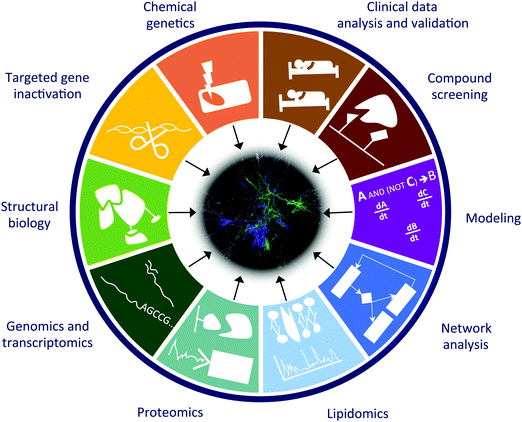 |
| Fig. 2 Scientific approaches that facilitate the understanding of signaling networks and promote network medicine analyses. Combination of methods used in different approaches could provide a higher level of understanding of systems of interest, compared to analyses conducted using just one approach. | |
A recently established and successful framework to facilitate interdisciplinary approaches to solve complex biomedical questions is the concept of Challenges (i.e., collaborative scientific competitions).107 This is based on crowdsourcing, open innovation and to foster the creation and dissemination of well-curated data repositories.107 In agreement with the success of crowdsourcing and Challenges, a previous study found that teams are 37.7% more likely than solo authors to insert novel combinations into familiar knowledge domains (in an analysis of 17.9 million papers spanning all scientific fields).108 Forming interdisciplinary teams is a challenging task, and several background factors could help it, for example people with lower H-index or from lower-tier institutions team up more, and higher tenure and prior co-authorship increase likelihood of collaboration.109 These data suggest that scientific meetings and networking as well as a general methodological understanding to each other's discipline are needed to establish successful interdisciplinary teams. Further indicators and general assessment of successful multi-disciplinary teamworks can be found in the review of Wooten and colleagues.110
To be able to effectively carry out interdisciplinary research projects, it is essential that postgraduate (and above) life science bench researchers are given the training and experience needed for them to be able to have familiarity in formulating precise questions that can be tackled computationally. This will be greatly facilitated by providing opportunities for researchers to meet and learn from each other, with these encounters supported by expert facilitation. Accordingly, in July 2014, we organized a five-day interdisciplinary workshop on signaling (http://2014.signalingworkshop.org) to bring together, in an environment designed to promote informal interpersonal interactions, experts from a wide range of backgrounds (in silico modelers, computational biologists, biochemists, geneticists, molecular and cell biologists as well as cancer biologists and pharmacologists). This workshop provided a context for participants to discuss the state-of-the-art in their respective fields, providing a forum for established scientists to share their perspectives and expertise, as well as enabling early stage researchers to present their projects, research questions and large-scale experiments. Experimentalists were asked to submit a key problem that required interdisciplinary approaches, and the problem was discussed in subgroups led by senior scientists with specific relevant expertise.
A key outcome to emerge from these studies and events is the collecting and mapping of training needs, and the collaborative definition of training programs that can effectively address the presented issues, and provide a genuinely supportive environment for building the interdisciplinarity skills of all scientists. The increasing number of multi-disciplinary projects showing the power of interdisciplinary approaches and their translational success in clinics will hopefully soon change the current funding environment, which already encourages interdisciplinary research but consistently provides lower funding to these proposals.111 Together, as a joint effort of researchers of different disciplines, open to understand and work with others, we will be able to meet the interdisciplinary requirements of network medicine, and provide excellent opportunities for the application of such approaches for the next generation of researchers.
Acknowledgements
The authors are grateful for the discussions with the participants of the Interdisciplinary Signaling Workshop (held in Visegrad, Hungary between 21–25 July 2014) and with the current and former members of the NetBiol and Korcsmaros groups. Specifically, we thank the help of Padhmanand Sudhakar, Zoltan Dul, Dezso Modos, Jo Brooks, Aidan Budd, Amanda Demeter, Marton Olbei and Chris Bennett in the preparation of the manuscript. The work of the authors were supported by a research grant from the Hungarian Science Foundation [OTKA NK78012], by a fellowship to TK in computational biology at Earlham Institute (Norwich, UK) in partnership with the Institute of Food Research (Norwich, UK), and strategically supported by Biotechnological and Biosciences Research Council, UK (BB/J004529/1).
References
- A.-L. Barabási, N. Engl. J. Med., 2007, 357, 404–407 CrossRef PubMed.
- M. J. Lee, A. S. Ye, A. K. Gardino, A. M. Heijink, P. K. Sorger, G. MacBeath and M. B. Yaffe, Cell, 2012, 149, 780–794 CrossRef CAS PubMed.
- A. Pichlmair, K. Kandasamy, G. Alvisi, O. Mulhern, R. Sacco, M. Habjan, M. Binder, A. Stefanovic, C.-A. Eberle, A. Goncalves, T. Bürckstümmer, A. C. Müller, A. Fauster, C. Holze, K. Lindsten, S. Goodbourn, G. Kochs, F. Weber, R. Bartenschlager, A. G. Bowie, K. L. Bennett, J. Colinge and G. Superti-Furga, Nature, 2012, 487, 486–490 CrossRef CAS PubMed.
- E. T. Liu and T. Lemberger, Mol. Syst. Biol., 2007, 3, 94 CrossRef PubMed.
- A. Henney and G. Superti-Furga, Nature, 2008, 455, 730–731 CrossRef CAS PubMed.
- T. Pawson and R. Linding, FEBS Lett., 2008, 582, 1266–1270 CrossRef CAS PubMed.
- A. Zanzoni, M. Soler-López and P. Aloy, FEBS Lett., 2009, 583, 1759–1765 CrossRef CAS PubMed.
- A.-L. Barabási, N. Gulbahce and J. Loscalzo, Nat. Rev. Genet., 2011, 12, 56–68 CrossRef PubMed.
- P. Csermely, T. Korcsmáros, H. J. M. Kiss, G. London and R. Nussinov, Pharmacol. Ther., 2013, 138, 333–408 CrossRef CAS PubMed.
- J. Menche, A. Sharma, M. Kitsak, S. D. Ghiassian, M. Vidal, J. Loscalzo and A.-L. Barabási, Science, 2015, 347, 1257601 CrossRef PubMed.
- M. Kirschner, Methods Mol. Biol., 2016, 1386, 3–15 Search PubMed.
- D. K. Arrell and A. Terzic, Clin. Pharmacol. Ther., 2010, 88, 120–125 CrossRef CAS PubMed.
- P. Prathipati and K. Mizuguchi, Curr. Top. Med. Chem., 2016, 16, 1009–1025 CrossRef CAS PubMed.
- H. V. Westerhoff, S. Nakayama, T. D. G. A. Mondeel and M. Barberis, Drug Discovery Today: Technol., 2015, 15, 23–31 CrossRef PubMed.
- K. A. Janes and D. A. Lauffenburger, J. Cell Sci., 2013, 126, 1913–1921 CrossRef CAS PubMed.
- D. Vandamme, W. Fitzmaurice, B. Kholodenko and W. Kolch, QJM, 2013, 106, 891–895 CrossRef CAS PubMed.
- C. Corbi-Verge and P. M. Kim, Cell Commun. Signaling, 2016, 14, 8 CrossRef PubMed.
- H. Kitano, Nat. Rev. Drug Discovery, 2007, 6, 202–210 CrossRef CAS PubMed.
- A.-L. Barabási and Z. N. Oltvai, Nat. Rev. Genet., 2004, 5, 101–113 CrossRef PubMed.
- J.-D. J. Han, N. Bertin, T. Hao, D. S. Goldberg, G. F. Berriz, L. V. Zhang, D. Dupuy, A. J. M. Walhout, M. E. Cusick, F. P. Roth and M. Vidal, Nature, 2004, 430, 88–93 CrossRef CAS PubMed.
- P. M. Kim, L. J. Lu, Y. Xia and M. B. Gerstein, Science, 2006, 314, 1938–1941 CrossRef CAS PubMed.
- H. Yu, P. M. Kim, E. Sprecher, V. Trifonov and M. Gerstein, PLoS Comput. Biol., 2007, 3, e59 Search PubMed.
- I. P. Tomlinson, M. R. Novelli and W. F. Bodmer, Proc. Natl. Acad. Sci. U. S. A., 1996, 93, 14800–14803 CrossRef CAS.
- M. A. Ali and T. Sjöblom, Mol. Biosyst., 2009, 5, 902–908 RSC.
- J. J. Hornberg, F. J. Bruggeman, H. V. Westerhoff and J. Lankelma, BioSystems, 2006, 83, 81–90 CrossRef CAS PubMed.
- D. Hanahan and R. A. Weinberg, Cell, 2000, 100, 57–70 CrossRef CAS PubMed.
- D. Hanahan and R. a Weinberg, Cell, 2011, 144, 646–674 CrossRef CAS PubMed.
- B. Bozóky, A. Savchenko, P. Csermely, T. Korcsmáros, Z. Dúl, F. Pontén, L. Székely and G. Klein, Int. J. Cancer, 2013, 133, 286–293 CrossRef PubMed.
- M. Halasz, B. N. Kholodenko, W. Kolch and T. Santra, Sci. Signaling, 2016, 9, ra114 CrossRef PubMed.
- D. Kim, O. Rath, W. Kolch and K.-H. Cho, Oncogene, 2007, 26, 4571–4579 CrossRef CAS PubMed.
- A. Torkamani and N. J. Schork, Genome Res., 2009, 19, 1570–1578 CrossRef CAS PubMed.
- M. Mimeault and S. K. Batra, Pharmacol. Rev., 2010, 62, 497–524 CrossRef CAS PubMed.
- T. Korcsmáros, I. J. Farkas, M. S. Szalay, P. Rovó, D. Fazekas, Z. Spiró, C. Böde, K. Lenti, T. Vellai and P. Csermely, Bioinformatics, 2010, 26, 2042–2050 CrossRef PubMed.
- J. Jia, F. Zhu, X. Ma, Z. Cao, Z. W. Cao, Y. Li, Y. X. Li and Y. Z. Chen, Nat. Rev. Drug Discovery, 2009, 8, 111–128 CrossRef CAS PubMed.
- P. Rajasethupathy, S. J. Vayttaden and U. S. Bhalla, Curr. Opin. Chem. Biol., 2005, 9, 400–406 CrossRef CAS PubMed.
- H. Kitano, Nat. Rev. Cancer, 2004, 4, 227–235 CrossRef CAS PubMed.
- N. V. Sergina, M. Rausch, D. Wang, J. Blair, B. Hann, K. M. Shokat and M. M. Moasser, Nature, 2007, 445, 437–441 CrossRef CAS PubMed.
- T. M. Varusai, W. Kolch, B. N. Kholodenko and L. K. Nguyen, Mol. BioSyst., 2015, 11, 2750–2762 RSC.
- S. I. Berger and R. Iyengar, Bioinformatics, 2009, 25, 2466–2472 CrossRef CAS PubMed.
- H. V. Westerhoff, M. Verma, M. Nardelli, M. Adamczyk, K. van Eunen, E. Simeonidis and B. M. Bakker, Biochem. Soc. Trans., 2010, 38, 1189–1196 CrossRef CAS PubMed.
- P. V. Hornbeck, I. Chabra, J. M. Kornhauser, E. Skrzypek and B. Zhang, Proteomics, 2004, 4, 1551–1561 CrossRef CAS PubMed.
- S. Ullah, S. Lin, Y. Xu, W. Deng, L. Ma, Y. Zhang, Z. Liu and Y. Xue, Sci. Rep., 2016, 6, 23534 CrossRef CAS PubMed.
- R. Linding, L. J. Jensen, G. J. Ostheimer, M. A. T. M. van Vugt, C. Jørgensen, I. M. Miron, F. Diella, K. Colwill, L. Taylor, K. Elder, P. Metalnikov, V. Nguyen, A. Pasculescu, J. Jin, J. G. Park, L. D. Samson, J. R. Woodgett, R. B. Russell, P. Bork, M. B. Yaffe and T. Pawson, Cell, 2007, 129, 1415–1426 CrossRef CAS PubMed.
- M. L. Miller, L. J. Jensen, F. Diella, C. Jørgensen, M. Tinti, L. Li, M. Hsiung, S. A. Parker, J. Bordeaux, T. Sicheritz-Ponten, M. Olhovsky, A. Pasculescu, J. Alexander, S. Knapp, N. Blom, P. Bork, S. Li, G. Cesareni, T. Pawson, B. E. Turk, M. B. Yaffe, S. Brunak and R. Linding, Sci. Signaling, 2008, 1, ra2 CrossRef PubMed.
- H. Horn, E. M. Schoof, J. Kim, X. Robin, M. L. Miller, F. Diella, A. Palma, G. Cesareni, L. J. Jensen and R. Linding, Nat. Methods, 2014, 11, 603–604 CrossRef CAS PubMed.
- C. Bakal, R. Linding, F. Llense, E. Heffern, E. Martin-Blanco, T. Pawson and N. Perrimon, Science, 2008, 322, 453–456 CrossRef CAS PubMed.
- C. Jørgensen, A. Sherman, G. I. Chen, A. Pasculescu, A. Poliakov, M. Hsiung, B. Larsen, D. G. Wilkinson, R. Linding and T. Pawson, Science, 2009, 326, 1502–1509 CrossRef PubMed.
- C. S. H. Tan, B. Bodenmiller, A. Pasculescu, M. Jovanovic, M. O. Hengartner, C. Jørgensen, G. D. Bader, R. Aebersold, T. Pawson and R. Linding, Sci. Signaling, 2009, 2, ra39 CrossRef PubMed.
- C. S. H. Tan, A. Pasculescu, W. A. Lim, T. Pawson, G. D. Bader and R. Linding, Science, 2009, 325, 1686–1688 CrossRef CAS PubMed.
- P. Creixell, E. M. Schoof, J. T. Erler and R. Linding, Nat. Biotechnol., 2012, 30, 842–848 CrossRef CAS PubMed.
- J. T. Erler and R. Linding, Cell, 2012, 149, 731–733 CrossRef CAS PubMed.
- L. K. Nguyen, D. Matallanas, D. R. Croucher, A. von Kriegsheim and B. N. Kholodenko, FEBS J., 2013, 280, 751–765 CrossRef CAS PubMed.
- A. Alonso, J. Sasin, N. Bottini, I. Friedberg, I. Friedberg, A. Osterman, A. Godzik, T. Hunter, J. Dixon and T. Mustelin, Cell, 2004, 117, 699–711 CrossRef CAS PubMed.
- R.-J. He, Z.-H. Yu, R.-Y. Zhang and Z.-Y. Zhang, Acta Pharmacol. Sin., 2014, 35, 1227–1246 CrossRef CAS PubMed.
- F. Sacco, P. F. Gherardini, S. Paoluzi, J. Saez-Rodriguez, M. Helmer-Citterich, A. Ragnini-Wilson, L. Castagnoli and G. Cesareni, Mol. Syst. Biol., 2012, 8, 603 CrossRef PubMed.
- J. Saez-Rodriguez, L. G. Alexopoulos, J. Epperlein, R. Samaga, D. A. Lauffenburger, S. Klamt and P. K. Sorger, Mol. Syst. Biol., 2009, 5, 331 CrossRef PubMed.
- P. Tompa, N. E. Davey, T. J. Gibson and M. M. Babu, Mol. Cell, 2014, 55, 161–169 CrossRef CAS PubMed.
- H. Dinkel, K. Van Roey, S. Michael, N. E. Davey, R. J. Weatheritt, D. Born, T. Speck, D. Krüger, G. Grebnev, M. Kuban, M. Strumillo, B. Uyar, A. Budd, B. Altenberg, M. Seiler, L. B. Chemes, J. Glavina, I. E. Sánchez, F. Diella and T. J. Gibson, Nucleic Acids Res., 2014, 42, D259–D266 CrossRef CAS PubMed.
- B. Mészáros, I. Simon and Z. Dosztányi, PLoS Comput. Biol., 2009, 5, e1000376 Search PubMed.
- V. Vojisavljevic and E. Pirogova, Med. Biol. Eng. Comput., 2016, 54, 1831–1844 Search PubMed.
- D. G. Gibson, Nat. Methods, 2014, 11, 521–526 CrossRef CAS PubMed.
- B. Uyar, R. J. Weatheritt, H. Dinkel, N. E. Davey and T. J. Gibson, Mol. Biosyst., 2014, 10, 2626–2642 RSC.
- A. Zeke, M. Lukács, W. A. Lim and A. Reményi, Trends Cell Biol., 2009, 19, 364–374 CrossRef CAS PubMed.
- B. N. Kholodenko, Nat. Rev. Mol. Cell Biol., 2006, 7, 165–176 CrossRef CAS PubMed.
- R. P. Bhattacharyya, A. Reményi, B. J. Yeh and W. A. Lim, Annu. Rev. Biochem., 2006, 75, 655–680 CrossRef CAS PubMed.
- J. H. Ha, M. Yan, R. Gomathinayagam, M. Jayaraman, S. Husain, J. Liu, P. Mukherjee, E. P. Reddy, Y. S. Song and D. N. Dhanasekaran, Oncotarget, 2016, 7, 72845–72859 Search PubMed.
- N. Parveen, M. U. Hussain, A. A. Pandith and S. Mudassar, Med. Oncol., 2011, 28(suppl 1), S259–S267 CrossRef PubMed.
- D. Fazekas, M. Koltai, D. Türei, D. Módos, M. Pálfy, Z. Dúl, L. Zsákai, M. Szalay-Bek, K. Lenti, I. J. Farkas, T. Vellai, P. Csermely, T. Korcsmáros and M. Szalay-Bekő, BMC Syst. Biol., 2013, 7, 7 CrossRef PubMed.
- F. Ramírez and M. Albrecht, Trends Cell Biol., 2010, 20, 2–4 CrossRef PubMed.
- M. Pálfy, A. Reményi and T. Korcsmáros, Trends Cell Biol., 2012, 22, 447–456 CrossRef PubMed.
- E. Wang, J. Zou, N. Zaman, L. K. Beitel, M. Trifiro and M. Paliouras, Semin. Cancer Biol., 2013, 23, 279–285 CrossRef CAS PubMed.
- D. Day and L. L. Siu, Genome Med., 2016, 8, 115 CrossRef PubMed.
- C. Russell, A. Rahman and A. R. Mohammed, Ther. Delivery, 2013, 4, 395–413 CrossRef CAS PubMed.
- S.-K. Yan, R.-H. Liu, H.-Z. Jin, X.-R. Liu, J. Ye, L. Shan and W.-D. Zhang, Chin. J. Nat. Med., 2015, 13, 3–21 Search PubMed.
- B. Berger, J. Peng and M. Singh, Nat. Rev. Genet., 2013, 14, 333–346 CrossRef CAS PubMed.
- J. M. Buescher and E. M. Driggers, Cancer Metab., 2016, 4, 4 CrossRef PubMed.
- L. de Vargas Roditi and M. Claassen, Curr. Opin. Biotechnol., 2014, 34C, 9–15 Search PubMed.
- S. Gulati, P. Martinez, T. Joshi, N. J. Birkbak, C. R. Santos, A. J. Rowan, L. Pickering, M. Gore, J. Larkin, Z. Szallasi, P. A. Bates, C. Swanton and M. Gerlinger, Eur. Urol., 2014, 66, 936–948 CrossRef CAS PubMed.
- J. L. Wilson, M. T. Hemann, E. Fraenkel and D. A. Lauffenburger, Semin. Cancer Biol., 2013, 23, 213–218 CrossRef CAS PubMed.
- J. L. Wilson, S. Dalin, S. Gosline, M. Hemann, E. Fraenkel and D. A. Lauffenburger, Integr. Biol., 2016, 8, 761–774 RSC.
- J. A. Doudna and E. Charpentier, Science, 2014, 346, 1258096 CrossRef PubMed.
- A.-E. Saliba, I. Vonkova, S. Ceschia, G. M. Findlay, K. Maeda, C. Tischer, S. Deghou, V. van Noort, P. Bork, T. Pawson, J. Ellenberg and A.-C. Gavin, Nat. Methods, 2014, 11, 47–50 CrossRef CAS PubMed.
- K. Yang and X. Han, Trends Biochem. Sci., 2016, 41, 954–969 CrossRef CAS PubMed.
- M. J. Gerl, V. Bittl, S. Kirchner, T. Sachsenheimer, H. L. Brunner, C. Lüchtenborg, C. Özbalci, H. Wiedemann, S. Wegehingel, W. Nickel, P. Haberkant, C. Schultz, M. Krüger and B. Brügger, PLoS One, 2016, 11, e0153009 Search PubMed.
- G. Balogh, M. Péter, G. Liebisch, I. Horváth, Z. Török, E. Nagy, A. Maslyanko, S. Benko, G. Schmitz, J. L. Harwood and L. Vígh, Biochim. Biophys. Acta, 2010, 1801, 1036–1047 CrossRef CAS PubMed.
- M. S. Köberlin, B. Snijder, L. X. Heinz, C. L. Baumann, A. Fauster, G. I. Vladimer, A.-C. Gavin and G. Superti-Furga, Cell, 2015, 162, 170–183 CrossRef PubMed.
- B. Kholodenko, M. B. Yaffe and W. Kolch, Sci. Signaling, 2012, 5, re1 CrossRef PubMed.
- K. A. Janes and M. B. Yaffe, Nat. Rev. Mol. Cell Biol., 2006, 7, 820–828 CrossRef CAS PubMed.
- C. Terfve and J. Saez-Rodriguez, Adv. Exp. Med. Biol., 2012, 736, 19–57 CrossRef CAS PubMed.
- E. Gonçalves, J. Bucher, A. Ryll, J. Niklas, K. Mauch, S. Klamt, M. Rocha and J. Saez-Rodriguez, Mol. Biosyst., 2013, 9, 1576–1583 RSC.
- D. Cohen, I. Kuperstein, E. Barillot, A. Zinovyev and L. Calzone, Methods Mol. Biol., 2013, 1021, 107–125 Search PubMed.
- T. Santra, W. Kolch and B. N. Kholodenko, PLoS Comput. Biol., 2014, 10, e1003385 Search PubMed.
- I. J. Farkas, T. Korcsmáros, I. A. Kovács, Á. Mihalik, R. Palotai, G. I. Simkó, K. Z. Szalay, M. Szalay-Beko, T. Vellai, S. Wang and P. Csermely, Sci. Signaling, 2011, 4, pt3 CrossRef PubMed.
- D. Modos, J. Brooks, D. Fazekas, E. Ari, T. Vellai, P. Csermely, T. Korcsmaros and K. Lenti, Sci. Rep., 2016, 6, 38588 CrossRef PubMed.
- D. Türei, T. Korcsmáros and J. Saez-Rodriguez, Nat. Methods, 2016, 13, 966–967 CrossRef PubMed.
- C. Terfve, T. Cokelaer, D. Henriques, A. MacNamara, E. Goncalves, M. K. Morris, M. van Iersel, D. A. Lauffenburger and J. Saez-Rodriguez, BMC Syst. Biol., 2012, 6, 133 CrossRef CAS PubMed.
- L. Calzone, E. Barillot and A. Zinovyev, Integr. Biol., 2015, 7, 921–929 RSC.
- T. Cuppens, S. Tuyaerts and F. Amant, Sarcoma, 2015, 2015, 243298 CrossRef PubMed.
- W. Kolch, M. Halasz, M. Granovskaya and B. N. Kholodenko, Nat. Rev. Cancer, 2015, 15, 515–527 CrossRef CAS PubMed.
- M. Chanrion, I. Kuperstein, C. Barrière, F. El Marjou, D. Cohen, D. Vignjevic, L. Stimmer, P. Paul-Gilloteaux, I. Bièche, S. D. R. Tavares, G.-F. Boccia, W. Cacheux, D. Meseure, S. Fre, L. Martignetti, P. Legoix-Né, E. Girard, L. Fetler, E. Barillot, D. Louvard, A. Zinovyev and S. Robine, Nat. Commun., 2014, 5, 5005 CrossRef CAS PubMed.
- E. Bilal, T. Sakellaropoulos, I. N. Melas, D. E. Messinis, V. Belcastro, K. Rhrissorrakrai, P. Meyer, R. Norel, A. Iskandar, E. Blaese, J. J. Rice, M. C. Peitsch, J. Hoeng, G. Stolovitzky, L. G. Alexopoulos, C. Poussin and Challenge Participants, Bioinformatics, 2015, 31, 484–491 CrossRef CAS PubMed.
- I. Thiele, N. Swainston, R. M. T. Fleming, A. Hoppe, S. Sahoo, M. K. Aurich, H. Haraldsdottir, M. L. Mo, O. Rolfsson, M. D. Stobbe, S. G. Thorleifsson, R. Agren, C. Bölling, S. Bordel, A. K. Chavali, P. Dobson, W. B. Dunn, L. Endler, D. Hala, M. Hucka, D. Hull, D. Jameson, N. Jamshidi, J. J. Jonsson, N. Juty, S. Keating, I. Nookaew, N. Le Novère, N. Malys, A. Mazein, J. A. Papin, N. D. Price, E. Selkov, M. I. Sigurdsson, E. Simeonidis, N. Sonnenschein, K. Smallbone, A. Sorokin, J. H. G. M. van Beek, D. Weichart, I. Goryanin, J. Nielsen, H. V. Westerhoff, D. B. Kell, P. Mendes and B. Ø. Palsson, Nat. Biotechnol., 2013, 31, 419–425 CrossRef CAS PubMed.
- S. M. Hill, L. M. Heiser, T. Cokelaer, M. Unger, N. K. Nesser, D. E. Carlin, Y. Zhang, A. Sokolov, E. O. Paull, C. K. Wong, K. Graim, A. Bivol, H. Wang, F. Zhu, B. Afsari, L. V. Danilova, A. V. Favorov, W. S. Lee, D. Taylor, C. W. Hu, B. L. Long, D. P. Noren, A. J. Bisberg, B. HPN-DREAM Consortium, G. B. Mills, J. W. Gray, M. Kellen, T. Norman, S. Friend, A. A. Qutub, E. J. Fertig, Y. Guan, M. Song, J. M. Stuart, P. T. Spellman, H. Koeppl, G. Stolovitzky, J. Saez-Rodriguez, S. Mukherjee, C. Cai, D. E. Carlin, A. Carlon, L. Chen, M. F. Ciaccio, T. Cokelaer, G. Cooper, C. J. Creighton, S.-M.-H. Daneshmand, A. de la Fuente, B. Di Camillo, L. V. Danilova, J. Dutta-Moscato, K. Emmett, C. Evelo, M.-K. H. Fassia, A. V. Favorov, E. J. Fertig, J. D. Finkle, F. Finotello, S. Friend, X. Gao, J. Gao, J. Garcia-Garcia, S. Ghosh, A. Giaretta, K. Graim, J. W. Gray, R. Großeholz, Y. Guan, J. Guinney, C. Hafemeister, O. Hahn, S. Haider, T. Hase, L. M. Heiser, S. M. Hill, J. Hodgson, B. Hoff, C. H. Hsu, C. W. Hu, Y. Hu, X. Huang, M. Jalili, X. Jiang, T. Kacprowski, L. Kaderali, M. Kang, V. Kannan, M. Kellen, K. Kikuchi, D.-C. Kim, H. Kitano, B. Knapp, G. Komatsoulis, H. Koeppl, A. Krämer, M. B. Kursa, M. Kutmon, W. S. Lee, Y. Li, X. Liang, Z. Liu, Y. Liu, B. L. Long, S. Lu, X. Lu, M. Manfrini, M. R. A. Matos, D. Meerzaman, G. B. Mills, W. Min, S. Mukherjee, C. L. Müller, R. E. Neapolitan, N. K. Nesser, D. P. Noren, T. Norman, B. Oliva, S. O. Opiyo, R. Pal, A. Palinkas, E. O. Paull, J. Planas-Iglesias, D. Poglayen, A. A. Qutub, J. Saez-Rodriguez, F. Sambo, T. Sanavia, A. Sharifi-Zarchi, J. Slawek, A. Sokolov, M. Song, P. T. Spellman, A. Streck, G. Stolovitzky, S. Strunz, J. M. Stuart, D. Taylor, J. Tegnér, K. Thobe, G. M. Toffolo, E. Trifoglio, M. Unger, Q. Wan, H. Wang, L. Welch, C. K. Wong, J. J. Wu, A. Y. Xue, R. Yamanaka, C. Yan, S. Zairis, M. Zengerling, H. Zenil, S. Zhang, Y. Zhang, F. Zhu, Z. Zi, G. B. Mills, J. W. Gray, M. Kellen, T. Norman, S. Friend, A. A. Qutub, E. J. Fertig, Y. Guan, M. Song, J. M. Stuart, P. T. Spellman, H. Koeppl, G. Stolovitzky, J. Saez-Rodriguez and S. Mukherjee,
Nat. Methods, 2016, 13, 310–318 CrossRef PubMed.
- N. Kaplowitz, Drug Saf., 2001, 24, 483–490 CrossRef CAS PubMed.
- B. D. Cosgrove, L. G. Alexopoulos, T. Hang, B. S. Hendriks, P. K. Sorger, L. G. Griffith and D. A. Lauffenburger, Mol. Biosyst., 2010, 6, 1195–1206 RSC.
- N. E. Davey, G. Travé and T. J. Gibson, Trends Biochem. Sci., 2011, 36, 159–169 CrossRef CAS PubMed.
- J. Saez-Rodriguez, J. C. Costello, S. H. Friend, M. R. Kellen, L. Mangravite, P. Meyer, T. Norman and G. Stolovitzky, Nat. Rev. Genet., 2016, 17, 470–486 CrossRef CAS PubMed.
- B. Uzzi, S. Mukherjee, M. Stringer and B. Jones, Science, 2013, 342, 468–472 CrossRef CAS PubMed.
- A. Lungeanu, Y. Huang and N. S. Contractor, J. Informetr., 2014, 8, 59–70 CrossRef PubMed.
- K. C. Wooten, R. M. Rose, G. V. Ostir, W. J. Calhoun, B. T. Ameredes and A. R. Brasier, Eval. Health Prof., 2014, 37, 33–49 CrossRef PubMed.
- L. Bromham, R. Dinnage and X. Hua, Nature, 2016, 534, 684–687 CrossRef CAS PubMed.
|
This journal is © The Royal Society of Chemistry 2017 |