The microbial colonization of activated carbon block point-of-use (PoU) filters with and without chlorinated phenol disinfection by-products†
Received
27th April 2017
, Accepted 5th July 2017
First published on 6th July 2017
Abstract
Activated carbon block (ACB)-based point-of-use (PoU) drinking water filters are widely used to remove residual disinfectant, heavy metals, and disinfection by-products (DBPs) from household tap water. The filters are not designed to remove bacteria, which can grow in the filter. Based on previous research, we hypothesized that more biofilm would form in PoU filters due to the presence of chlorinated phenolic DBPs, resulting in higher bacterial concentrations in filtered water. Therefore, studies were conducted by operating a manifold system with three sets of ACB PoU filters that received chloraminated tap water spiked with (50 and 100 ng L−1 added) and without (0 ng L−1 added) pentachlorophenol (PCP) as a surrogate chlorinated phenolic DBP. After processing 85% of the manufacturer's recommended water volume, effluent heterotrophic plate counts plateaued at levels up to 100 times greater than influent counts. The upstream filter fabric developed a significant biofilm that lightened with increasing PCP concentration; however, the overall biofilm mass was not different between treatments. Influent, effluent, and fabric samples had distinct bacterial community structures, and the structure of effluent communities was more similar to that of fabric communities than that of influent communities. The bacterial community structure changed across the filter primarily due to the filter environment itself, while PCP effects were subtle yet significant. The relative abundance of Mycobacterium increased within fabric biofilms when PCP was added, and the absolute abundance was estimated to increase in the effluent relative to the influent. In conclusion, ACB PoU filters significantly alter the bacterial abundance and composition of drinking water.
Water impact
This research provides evidence of changes in microbial communities across activated carbon block-based point-of-use (PoU) drinking water filters, in response to the presence and absence of trace chlorinated phenol-based disinfection by-products. These findings are important for assessing the biological stability of PoU filter-treated water and for justifying a proper drinking water point-of-use treatment strategy.
|
Introduction
Point-of-use (PoU) drinking water filtration devices are increasingly deployed by consumers as a stopgap approach to deal with tap water quality concerns, including inorganic and organic chemicals as well as taste and odor issues. Only limited research has been undertaken, however, to determine the effect these all-in-one treatment devices have on bacteria from the distribution system that pass into filters when the faucet is turned on. Given that the structure and abundance of bacterial communities in drinking water distribution systems, including opportunistic pathogens, are known to shift in response to the concentration of disinfectants, halogenated and non-halogenated organic compounds, metals, and nutrients,1–3 it is reasonable to expect that PoU filter performance will also influence the fate of bacteria within filters and, consequently, in filtered drinking water. Given that 2 and 19% annual market growth in PoU devices is expected over the next decade until 2030 in developed countries and developing countries with fast growing economies,4 respectively, more research is needed to examine the microbial ecology of these devices under the conditions they are used so that risks that come from employing PoU filters can be understood.
Commercial PoU filters in the United States most commonly employ activated carbon as the primary filter and adsorbing material, although materials and designs in other countries are more varied. Faucet-mounted PoU filters, the type considered in our study, consist of a fabric pre-filter and a low-porosity activated carbon block (ACB). Their carbon block design requires line pressures of at least 210 kPa (30 psi) for water throughput as a result of their very low porosity, and thus these filters are unlikely to allow influent microorganisms to traverse the entire filter media depth as long as the ACB is not cracked or otherwise compromised during use. ACB PoU filters are certified for water quality through NSF/ANSI Standards 53 and 42, which target removal of health-based (heavy metals, fluoride, nitrate, and a range of volatile organic chemicals) and aesthetic (chlorine for taste-and-odor concerns, chloramine, iron, manganese, hydrogen sulfide, zinc) contaminants.5,6 Notably, the certification does not include removal of bacteria. Indeed, the World Health Organization has long held that any PoU filter with an activated carbon base is inappropriate to use in poorly disinfected waters because it will support the excessive growth of bacteria and increase the risk of enhancing pathogen levels.7
Only a few published studies have evaluated changes in microbial community abundance or composition across ACB PoU filters. These studies used culture-based methods and consistently showed that bacterial counts are elevated in filtered water relative to the influent tap water.8–14 In addition, when changes in microbial diversity were tracked across PoU filters in one study, diversity decreased even as total cell counts increased.14 Culture-based studies have implicated the enrichment of opportunistic pathogens like Pseudomonas aeruginosa13 and Stenotrophomonas maltophilia14 across the filter. Given that PoU filters are user-maintained, wide variations in usage patterns that may impact bacterial colonization and filtered water microbial quality are possible. These studies indicate that ACB PoU filters change the microbial community counts and composition that consumers are exposed to relative to tap water; however, the impact of this shift on public health is not well characterized. Importantly, past studies are constrained by the use of culture-based methods; culture-independent techniques coupled with culture-based methods can provide a more comprehensive understanding of how PoU filters influence drinking water microbial ecology.
Since PoU filters are designed to remove halogenated DBPs, it is logical to ask whether DBP accumulation within filters has a significant impact on the microbial communities that colonize them. We are particularly interested in chlorophenol-based DBPs, which are unregulated DBPs that are formed from phenolic moiety precursors in natural organic matter at low levels in response to both chlorine and monochloramine.15,16 Our past work showed that chlorinated phenols upregulate the MexAB-OprM multi-drug resistance efflux pump in P. aeruginosa,17 which may make bacteria with this or similar efflux pumps vulnerable to enhanced biofilm formation because quorum sensing molecules are also released by these systems.18,19 We hypothesize that chlorophenol-based DBPs may enhance the microbial colonization of PoU filter surfaces. Indeed, our preliminary data suggest that chlorophenols induce biofilm formation by P. aeruginosa (data in ESI† section 1).
In this study, we evaluated how bacteria colonized PoU filters with and without a supplemental chlorophenol-based DBP. We selected pentachlorophenol (PCP) as our model DBP, which has the strongest effect among the chlorophenols we have studied on the MexAB-OprM system,17 and applied it at 100 ng L−1 and below to mimic the concentrations of chlorinated phenols detected in the few drinking water studies where these chemicals have been monitored.20–22 By conducting experiments with and without PCP, we were able to evaluate the relative impact of PoU filter architecture (or physical design) versus the role of trace DBP concentrations on microbial ecology.
Materials and methods
Bench-scale PoU manifold system
A manifold system (Fig. S1†) with three pairs of commercial PoU filters (faucet-mounted type) was fed with municipal tap water (Ann Arbor, Michigan) and the influent to each pair was spiked with 0, 50, or 100 ng L−1 of PCP (Fig. 1). Each PoU filter consisted of an annular activated carbon block (ACB) surrounded by a synthetic fabric mesh, which serves as an ACB prefilter. The ACB is held together with a proprietary, low melting point polymer binder. Surface areas, the average pore size, and porosities of the carbon block material were determined for three unused filters with a pressure sorption analyzer (Micromeritics, ASAP 2050) (Table S2†). The fabric mesh was separated based on visually apparent layers and consists of at least three distinct layers of synthetic silica or polymer-coated silicic fibers, as determined by scanning electron microscopy and X-ray microanalysis (Fig. S2†). Water radially flowed from the fabric face, through the ACB, and exited through the inner channel of the filter directly into an effluent reservoir (without connection tubing). Effluent reservoirs were autoclaved on days when samples were collected to perform microbiological analysis. Sterile foil was applied loosely over the PoU filter–effluent reservoir opening to protect the effluent reservoir from contamination not associated with the PoU filter flow.
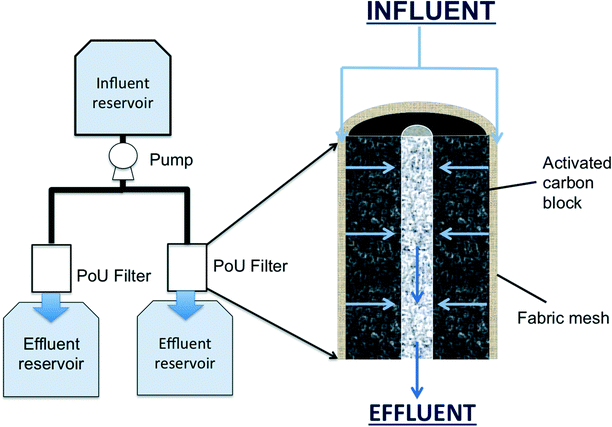 |
| Fig. 1 Schematic of the PoU manifold system (left) and the cross sectional profile of the filter architecture (right). The influent flow is indicated by blue arrows. | |
Influent reservoirs were filled at the beginning of each daily cycle with tap water, which was spiked with a known amount of base-solubilized PCP stock solution to meet the influent target concentrations. To avoid photodegradation of PCP, the influent reservoirs were covered with fitted black felt jackets. Before filling the influent containers, the tap water was allowed to run at least 15 minutes until a steady-state water temperature was achieved to purge stagnant water from the plumbing. The source of Ann Arbor municipal water supply is primarily Huron River water that is treated using lime softening and coagulation, settling, ozonation, filtration, and chloramination.
To simulate the daily on–off flow and stagnation periods of normal household use, influent water was pumped (Brass Rotary Vane Pump, Procon) intermittently from the covered influent reservoirs to the three manifold systems. The pump program was set to run once hourly for 16 h, followed by a night time stagnation period of 8 h. A design daily process volume of about 8 L was targeted and the filters were operated for 67 days. Due to decreases in hydraulic permeability in the filters over the course of the experiment, filter cycles were gradually lengthened in an attempt to maintain a constant daily process volume; however, the daily process volume gradually decreased toward the end of the run to around 7 L per day. Exact process volumes were determined by weighing the effluent reservoir. The total processed volume for each filter averaged 137% of the manufacturer's rated design process volume for the filter cartridge (100 gallons or 379 L) (Table S3†).
At the end of the run period, each PoU filter was cut open and photographed (Fig. S3†). The fabric mesh surrounding the PoU filter media was removed using an aseptic technique from the carbon block, photographed (Fig. S4†) and stored at either −20 °C for extracellular polymeric substance (EPS) analysis or −80 °C for nucleic acid-based analyses; the methods for both are given below.
Water quality analyses
Samples were collected for water quality analysis from the influent and effluent according to the schedule in Table S4.† The frequency (daily, weekly or bi-weekly) and type (grab or two-week composite) of samples collected varied for different sample locations and water quality parameters are also described in Table S4.† All analyses were in accordance with Standard Methods.23 Briefly, total alkalinity was analysed using the titration method according to Standard Method 2320. The free chlorine and total chlorine were measured by the N,N-diethyl-p-phenylenediamine (DPD) colorimetric method (Standard Method 4500-Cl G) with a pocket colorimeter (Hach, Loveland, CO). Heterotrophic plate counts (HPCs) were performed by the R2A spread plate method (Standard Method 9215C) or the membrane filter method for counts ≤102 CFU mL−1 (Standard Method 9215D). Dissolved (DOC) and total organic carbon (TOC) were determined using a Shimadzu ASI-V TOC analyzer equipped with an infrared detector (Standard Method 5310B). Specific UV absorbance was determined by Standard Method 5910 B. The analysis of chlorophenols (di-, tri- and pentachlorophenol) is described in section 7 of the ESI.† During the operation of the PoU manifold system, no chlorophenols were detected in the control (0 ng L−1 PCP) influent. Finally, pH was monitored using an Orion 3-Star Benchtop pH meter (Thermo Fisher Scientific, Beverly, MA). The probe calibration was confirmed at least once daily when in use.
Total protein and extracellular polymeric substance (EPS) measurements of the fabric biomass
Biomass from each of the six PoU filter fabric samples was extracted at the end of the experiment and the concentrations of total protein, EPS protein, and EPS carbohydrate were determined. Fabric samples were cut into 1.5 × 1.5 cm2 sections, which were then cut into small pieces and placed in glass vials with 8 mL of deionized water. The EPS was isolated from the fabric via sonication. The samples were sonicated in an ice bath for 30 s at 25% amplitude (150 J mL−1) with a ¼′′ diameter microtip sonicator (Model VCX500, 750 watts, Cole Parmer, Vernon Hills, IL). The sonication setting was optimized to yield maximum carbohydrate and protein while limiting cell lysis.24 Following sonication, the liquid phase was filtered through a 0.2 μm PVDF syringe filter to remove cells and fabric debris from solution. Carbohydrate was measured by the phenol-sulfuric acid method.25 EPS protein was measured from the filtered liquid phase using the micro-bicinchoninic acid (microBCA) method.26 To measure total protein, cell lysis of fabric samples was accomplished by heating at 95 °C in a 0.1 M sodium hydroxide solution for one hour. The cooled solution was sonicated using the same settings as those used for EPS extraction to reduce biomolecule clumping. The liquid phase was filtered through a 0.2 μm PVDF syringe filter and analyzed using the microBCA method. We confirmed that clean fabric from an unused PoU filter did not interfere with the total protein method. Cellular protein was calculated as the difference between total protein and EPS protein.
DNA extraction from fabric and water samples
DNA was extracted from influent and effluent water samples on two to four dates for each treatment over the last three weeks of operation. Two liter water samples were filtered through sterile 0.22 μm polycarbonate membrane filters (Millipore GTTP04700, Billerica, MA). DNA was also extracted from the six PoU fabric pieces that had been handled in a sterile manner and stored at −80 °C. Each PoU fabric was cut into four equal strips with an area of 2.25 cm2 serving as sample replicates. Both polycarbonate membrane filters and fabrics were cut further into small pieces for DNA extraction. A three-step bead beating method with lysis buffer was evaluated and chosen (based on DNA recovery) as the best method to extract DNA. Polycarbonate membrane filter and PoU fabric pieces were added to 300 μL lysis buffer provided with the DNA IQTM Reference Sample Kit for MaxWell® 16 (Promega, Madison, WI, USA) with 0.5 g zirconium beads (previously baked at 400 °C for 4 hours) and beaten for 2 minutes using a Bead Beater (BioSpec, Bartlesville, OK, USA). After centrifuging at 12
500×g for 10 min to pellet the beads and particulate matter, the aqueous phase was collected. The process was repeated two more times as described except that only 150 μL lysis buffer was added at the start. The aqueous phase was collected after each process and composited into a single vial for DNA purification. Sixty microliters of Proteinase K (20 mg mL−1) was added to the cumulative liquid phase volume and incubated at 56 °C for 20 minutes to remove nucleases. DNA was purified with a Maxwell 16 automated DNA extraction instrument (Promega, Madison, WI, USA) and eluted in 50 μL elution buffer provided in the DNA IQTM Reference Sample Kit for MaxWell® 16 (Promega, Madison, WI, USA). The amount of DNA extracted was quantified using PicoGreen reagent (Invitrogen, Carlsbad, CA, USA) and ranged from 0.16 to 64 ng μL−1. Using the same extraction and DNA quantification protocol, a blank extraction conducted with nuclease-free water yielded a DNA concentration of 0.01 ng μL−1. The final concentrations (based on the amount extracted) of DNA in water samples or on fabric mesh are given in Table S5.†
16S rRNA gene PCR and sequence analysis
DNA extracts were submitted for 16S rRNA gene amplicon sequencing at the University of Michigan Medical School using the protocol outlined by Kozich et al.27 This protocol uses dual index-labeled primers that target the V4 region of bacterial and archaeal 16S rRNA genes (515F/806R).28 Pooled and purified libraries were sequenced on an Illumina MiSeq sequencer, using v2 chemistry 2 × 250 (500 cycles) paired-end reads. A total of 1
862
282 sequences were obtained from the 54 samples sequenced for this study to compare the similarity of bacterial community composition and structure between treatments. The raw data are available at the NCBI Sequence Read Archive database under access No. SRP072518. All data processing was conducted using Mothur (v 1.32.1)29 based on the MiSeq standard operating protocol accessed on April 2013 using SILVA Release 102 April 2012 for alignment. After data quality filtering (including chimera removal), a total of 1
521
189 sequences were retained in the final data library (Table S6†). The sequences were assigned a taxonomy using a naïve Bayesian classifier30 with a confidence threshold of 80%. The sequences were clustered using the average neighbor approach to form operational taxonomic units (OTUs) with a sequence similarity cut-off of 97% (3% sequence divergence). Consensus taxonomy for each OTU was determined using classify.otu with a consensus taxonomy cut-off = 51%.
Statistical analysis
Nonparametric Shannon diversity31 and Shannon evenness indices32 were estimated using Mothur (v 1.35.0).29 The nonparametric Shannon index ranges between 0 and unbounded values, with higher values indicating both higher richness and evenness. Shannon evenness ranges between 0 and 1, with 0 indicating not even and 1 indicating completely even composition. The sequences were subsampled to the depth of the sample with the lowest sequence count (8906 sequences). A neighbor-joining tree of the sequences constructed with Clearcut33 was analysed by unweighted and weighted UniFrac metrics as implemented by Mothur.34 The significance of UniFrac reported dissimilarities between samples (i.e. p values) were determined by conducting 1000 random permutation tests. The unweighted Unifrac accounts for the presence and absence of observed taxa between samples to estimate the dissimilarity between them, while weighted Unifrac incorporates the differences in relative abundance of observed taxa between samples to estimate dissimilarity.35 Jaccard and abundance-based Jaccard36 are OTU-based dissimilarity indices that are used to assess the overlap between different sample locations or PCP treatments. Distance indices between location or PCP treatment groups were compared by the nonparametric Kruskal–Wallis rank sum test. The UniFrac-based distance matrices were imported into R (http://www.r-project.org) for permutational multivariate analysis of variance (PERMANOVA) using the “vegan” package. Two-dimensional Principle Coordinate Analysis (PCoA) plots were generated to visualize the similarity between bacterial communities based on the UniFrac distance metrics. Permutational univariate analysis of variances (PERANOVA) was performed to compare the Unifrac distances within each replicate to the average of pooled samples, thus further confirming whether the two dimensional clustering patterns seen in the PCoA plots were statistically supported by differences in the distance matrices.37 We used corr.axis to determine the OTUs driving the clustering pattern observed using the distance matrices constructed from the UniFrac metrics (Pearson correlation, p < 0.05). The Kruskal–Wallis test was conducted to compare the diversity indices and relative abundance of OTUs between sample types or PCP treatments.
qPCR
Selected influent and effluent DNA samples from different collection dates and fabric DNA samples from different extraction replicates were composited to determine the total bacterial 16S rRNA genes by quantitative PCR (qPCR). qPCR was performed using an Eppendorf® Mastercycler (Eppendorf, Germany). The PCR mixture (10 μL) consisted of Fast-Plus EvaGreen Master Mix (Biotium, CA, USA), primer sets (0.5 μM each, Eub338: 5′-ACT-CCT-ACG-GGA-GGC-AGC-AG-3′, Eub518: 5′-ATT-ACC-GCG-GCT-GCT-GG-3′),38 0.625 mg mL−1 BSA, and 1 μL template DNA. Nuclease free water and genomic DNA of P. aeruginosa were included in all qPCR assays as negative and positive controls, respectively. All analyses were performed in triplicate. A standard curve was generated by amplifying 8-fold serially diluted PCR amplicons with a known amount of gene copies for each targeted gene. The thermal cycling program was set at 95 °C for 2 min, followed by 35 cycles of 5 s at 95 °C, 5 s at 58 °C, and 25 s at 72 °C. A melting curve was used to ensure specificity of amplification.
Results
Effluent heterotrophic bacteria concentrations exceed influent levels
Influent and effluent HPC profiles are presented in Fig. 2 over the 67 day operating period of the PoU manifold system. Influent HPC concentrations were relatively stable across all treatments (with and without PCP) and ranged between 101 and 103 CFU mL−1 with a mean concentration of 1.6 × 102 ± 2.5 × 102 CFU mL−1. In contrast, effluent HPC values increased for all treatments over the first 30 days of operation when counts increased rapidly (some counts could not be recorded because dilutions were insufficient to allow reliable counts). Effluent samples eventually stabilized at an average of 2.3 × 104 ± 1.0 × 104 CFU mL−1 after 40 days or when the filters were at approximately 85% of the manufacturer's recommended maximum throughput volume. The average DNA concentrations extracted from the influent and effluent samples were 10 ± 4 and 390 ± 320 ng L−1, respectively (Table S5†), and corroborate the large increase in cell abundance in the effluent. Culture-based methods are vulnerable to underestimation due to their inability to cultivate a wide range of bacteria on a single growth medium and due to cell's entry into a “viable but not culturable” growth state,39 and extracted DNA from environmental samples is vulnerable to overestimation due to extracellular DNA or DNA associated with non-viable cells;40 nevertheless, considered together, these data indicate a substantial and statistically significant increase in bacterial cell abundance between influent and effluent.
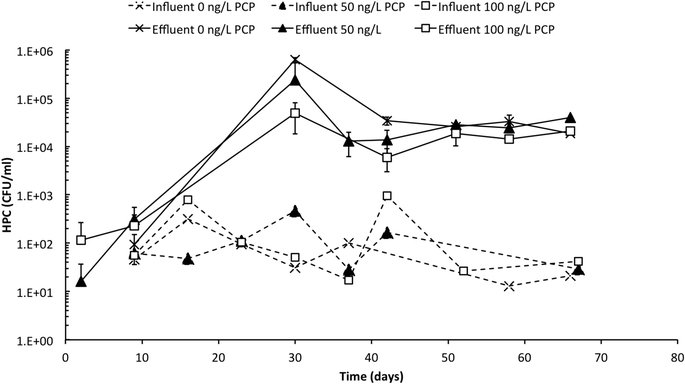 |
| Fig. 2 Concentrations of heterotrophic plate counts in influent (dashed line) and effluent (solid line) during operation. The PoU filters were fed with 0 ng PCP L−1 (cross), 50 ng PCP L−1 (triangle), or 100 ng PCP L−1 (square). The standard deviations of effluent samples are indicated by the error bars. | |
PCP exposure impacted the fabric biofilm in a way that is only partly explained by biofilm biochemical composition
The fabric surrounding the influent side of the ACB serves as a “pre-filter” and we expected that it would develop a significant biofilm. After opening the filters at the end of the experiment (Fig. S3†), we observed significant biofilm growth with distinct coloration differences between the control and PCP-fed filters (Fig. S4†). The control fabric biofilms were dark grey (Fig. S4(b)†) while the fabric biofilms from all the filters exposed to PCP were medium (50 ng L−1, Fig. S4(c)†) to light tan (100 ng L−1, Fig. S4(d)†); in contrast, the unused fabric mesh was bright white (Fig. S4(a)†). To determine if the biofilm color variations between treatments were due to differences in either biomass amounts or extracellular polymer (EPS) composition across PCP treatments, protein and carbohydrate analyses were conducted on the biofilm. As demonstrated in Fig. 3, the averages of total protein were 40 ± 9.0, 41 ± 5.2, and 36 ± 2.1 μg BSA cm−2 for 0, 50, and 100 ng L−1 PCP treatment, respectively. The cell protein averages were 21 ± 10, 21 ± 8.6, and 25 ± 2.9 μg BSA cm−2 for 0, 50, and 100 ng L−1 PCP treatment, respectively. There was no significant impact of PCP treatment on either total biomass (via total protein, p = 0.45) or cellular protein (total protein – EPS protein, p = 0.71), indicating that exposure to 100 ng L−1 PCP over the lifetime of a PoU filter did not influence the extent of overall biofilm growth and that differences in total cell mass do not explain the shift in biofilm color across PCP treatments. The EPS carbohydrate concentrations averaged 7.9 ± 2.7, 6.9 ± 2.2, and 7.7 ± 1.1 mg glucose per cm2 for 0, 50, and 100 ng L−1 PCP treatment, respectively, and were also not significantly different across treatments (p = 0.83). The EPS protein for the 100 ng L−1 PCP treatment (11 ± 1.5 μg BSA cm−2) was significantly lower than the control (19 ± 2.4 μg BSA cm−2) and 50 ng L−1 PCP treatments (18 ± 1.6 μg BSA cm−2) based on Tukey's pairwise analysis (p = 0.49 for 0 vs. 50 ng L−1, p = 0.002 for 0 vs. 100 ng L−1, and p = 0.001 for 50 vs. 100 ng L−1 PCP treatment). In turn, the averages of the EPS carbohydrate
:
protein ratio were 0.4 ± 0.5, 0.3 ± 0.4, and 0.6 ± 0.2 for 0, 50, and 100 ng L−1 PCP treatment, respectively; the ratio for the 100 ng L−1 PCP treatment was significantly higher than that for the 50 ng L−1 PCP treatment (p < 0.05) based on Tukey's pairwise analysis (p = 0.79 for 0 vs. 50 ng L−1, p = 0.13 for 0 vs. 100 ng L−1, and p = 0.03 for 50 vs. 100 ng L−1 PCP treatment). These results indicate that the extent of cell formation on the PoU filter fabric was similar across all three treatments. This result, however, does not exclude the possibility that changes in microbial community composition and function could have caused the color shade change in the biofilms.
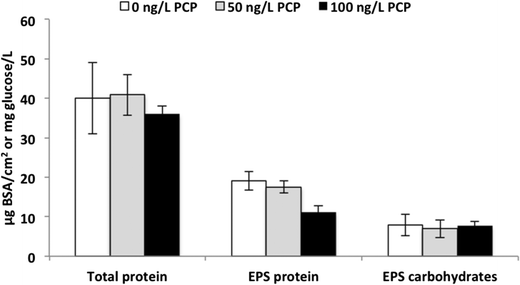 |
| Fig. 3 Biofilm compositions on fabrics treated with 0, 50, 100 ng L−1 PCP treatments. | |
Bacterial community structure changes across the PoU filter
The community diversity in the control (0 ng L−1 PCP) influent, fabric, and effluent samples changed significantly across the PoU filters. The number of dominant OTUs (defined here as taxa having a relative abundance equal to or greater than 1%) in the influent, fabric and effluent samples were 34, 22 and 15, and represented 75, 87 and 94% of the total characterized communities, respectively. The nonparametric Shannon index and Shannon evenness index were computed for influent (4.17 ± 0.24 and 0.66 ± 0.02), fabric (3.15 ± 0.03 and 0.54 ± 0.01) and effluent (2.40 ± 0.04 and 0.49 ± 0.04) communities, respectively; both indexes are statistically different between the three sample locations based on the Kruskal–Wallis test (p < 0.05). This suggests that influent communities had the highest diversity and a slightly more even structure because influent samples had more minor taxa with a relative abundance smaller than 1% compared to other sample types. Conversely, effluent communities were dominated by only a handful of OTUs and were the least diverse even among the three sample locations.
A relative abundance analysis exemplified these community differences across sample locations (Tables S7†). The most “highly dominant” (defined as ≥5% relative abundance) OTUs are mostly distinct (Table 1) and show informative patterns. Influent samples were dominated by Hydrogenophaga sp., which was also the second most abundant in effluent communities but of modest abundance in fabric communities. Three other OTUs (two unclassified Bacteroidetes and Brevundimonas) were “highly dominant” in the influent and present in decreasing relative abundance along the water flow path, suggesting that the filter imposed selective pressure against these taxa in contrast to others that became more abundant. These four OTUs plus other lower abundance OTUs that became less abundant across the flow path are designated as “filtered OTUs” (blue shaded bars) in Fig. 4. “Highly dominant” fabric OTUs (including Sphingopyxis sp., Mycobacterium sp., Aquabacterium sp., Acidovorax sp. and an unclassified Sphingomonadaceae) are classified as “attached OTUs” (red shaded bars) in Fig. 4. Finally, the most “highly dominant” OTUs found in the effluent (an unclassified Proteobacteria, an unclassified Betaproteobacteria, an unclassified bacteria, and Nitrospira) were of low relative abundance in the influent and are classified as “unfiltered OTUs” (green shaded bars) in Fig. 4. Indeed, even across all treatments, the most dominant OTUs in the effluent community were significantly different from those in the influent (Table S8,†p < 0.001). The sample location had a more significant influence on microbial composition than PCP treatment (Table S9†). Across all locations, these results show that the PoU filter significantly impacted where and to what degree different OTUs proliferated across the control PoU filters. In conclusion, the most highly dominant taxa across the three locations are generally different, implying selective pressures influencing community structure changes across the filter flow path.
Table 1 Highly dominant OTU (>5%) in PoU filter influent, fabric, and effluent samples in the absence of PCPa
Highly dominant taxa (OTU#) |
Influent |
Fabric |
Effluent |
Values shown in bold are the highly dominant OTUs present in each environment.
|
Hydrogenophaga (4) |
14.8%
|
1.4% |
20.1%
|
Unclassified Bacteroidetes (19) |
7.5%
|
2.4% |
0.0% |
Unclassified Bacteroidetes (12) |
7.2%
|
1.7% |
0.0% |
Brevundimonas (30) |
5.2%
|
0.7% |
0.3% |
Sphingopyxis (1) |
0.4% |
21.9%
|
2.0% |
Mycobacterium (3) |
1.0% |
18.0%
|
0.4% |
Aquabacterium (5) |
2.9% |
7.2%
|
3.6% |
Acidovorax (2) |
1.9% |
6.5%
|
3.8% |
Unclassified Sphingomonadaceae (14) |
0.1% |
5.9%
|
1.3% |
Unclassified Proteobacteria (7) |
0.1% |
0.0% |
30.4%
|
Unclassified Betaproteobacteria (15) |
0.2% |
0.0% |
8.6%
|
Unclassified bacteria (9) |
0.7% |
3.8% |
8.4%
|
Nitrospira (20) |
0.5% |
0.3% |
6.6%
|
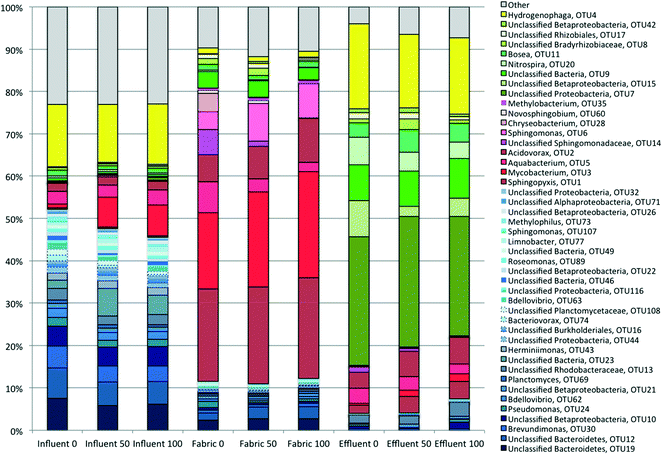 |
| Fig. 4 Average relative abundance of dominant taxa across all sample types. The number beside the sample name indicates the level of PCP treatment. Taxa names are labeled on the right. Taxa in blue colors are “filtered OTUs” that are mainly present in influent and fabric. Taxa in red colors are “attached OTUs” that flourish in fabric samples. Taxa in green colors are “unfiltered” OTUs that have a relative abundance greater in effluent than in influent. Taxa in yellow color have similar abundance in the influent and effluent. Note that not all OTUs mentioned above were included; this figure only shows dominant OTUs with relative abundance >1% and correlated with PCP treatment. | |
Trace PCP has a subtle but significant effect on community structure in the filter fabric and effluent
Influent, effluent, and fabric communities were significantly different independent of treatment when the dominant OTUs are considered (p < 0.001, UniFrac analysis), as shown in Fig. 5. The influent community structure does not vary with the presence of PCP (p > 0.05, UniFrac analysis, data not shown), despite the variation among samples with time (see ESI† section 10). In contrast, fabric and effluent communities were affected by PCP (Table S9†). For the fabric samples, the PCP concentration was relevant (Fig. 6, p < 0.001 from PERANOVA), as demonstrated by eight dominant OTUs that changed with PCP concentration (Fig. S5†). For the effluent samples, only three OTUs showed a significant shift in relative abundance in the presence of PCP (Fig. S6†), but the PCP concentration was not relevant (Fig. 7, p > 0.017 from PERANOVA with Bonferroni correction). Four OTUs proliferated considerably in either the fabric or effluent community with PCP treatment. Among them are two “attached OTUs” (shaded red in Fig. 4), Mycobacterium (OTU 3) and Sphingomonas (OTU 6), which had significantly higher relative abundance in PCP-exposed fabric samples. Furthermore, Mycobacterium (OTU 3) and Sphingopyxis (OTU 1) are “attached OTUs” that had significantly higher relative abundance in the effluent of PCP-exposed filters (p < 0.05, Kruskal–Wallis test). Interestingly, Mycobacterium (OTU 3) was the only OTU that showed an increase in relative abundance with PCP treatment in both the fabric and effluent (p < 0.05, Kruskal–Wallis test). The majority of “unfiltered OTUs” (shaded green in Fig. 4) and Hydrogenphaga (OTU 4) (shaded yellow in Fig. 4) in the effluent all had similar relative abundances between PCP treatments. Note that the relative abundance of one “filtered OTU”, Pseudomonas (OTU 24), decreased significantly in fabric samples exposed to PCP (p < 0.05, Kruskal–Wallis test). Collectively, these results show that attached OTUs on the fabric were more responsive to PCP treatments than when they were in the effluent, and few of them carried this effect to the downstream side of the filter.
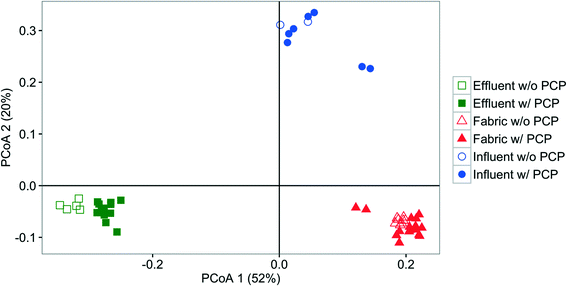 |
| Fig. 5 PCoA plot based on weighted UniFrac comparing the bacterial community structure of the fabric (collected at the end of operation, triangle), influent (circle) and effluent (square) from different time points without (blank) and with (solid) PCP treatment. | |
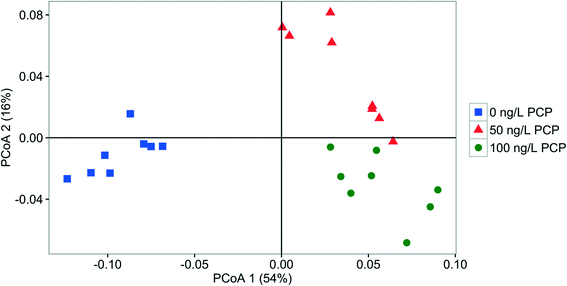 |
| Fig. 6 PCoA plot based on weighted UniFrac comparing the fabric bacterial community structure fed with 0 ng PCP L−1 (square), 50 ng PCP L−1 (triangle), or 100 ng PCP L−1 (circle). | |
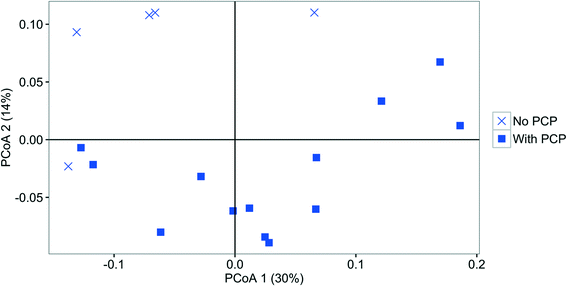 |
| Fig. 7 PCoA plot based on weighted UniFrac comparing the effluent bacterial community structure fed without PCP (cross) and with PCP (square). PCP exposed samples are combined because there was no significant difference between 50 and 100 ng L−1 treatments. | |
The water chemistry differed between the filter influent and effluent
Influent and effluent microbial communities were exposed to distinctly different water chemistry due to concentration changes in PCP, total chlorine residual, pH (Fig. S7†) and total organic carbon (Fig. S8†) across the filters (Tables S10 and S11†). We confirmed using multi-day composited samples analyzed by gas chromatography that the PCP-spiked influent jars contained PCP concentrations close to the targets (see Table S10†). No PCP was detected in the PoU filter effluent over the course of the experiment, and calculations show that PCP only penetrated ≤25 μm into the ACB due to sorption (see ESI† section 13.4). Influent total chlorine residuals averaged 2.5 ± 0.46 mg L−1 as Cl2 and effluent concentrations were below the detection limit of the test kit (0.2 mg L−1 as Cl2) until day 49 when the PCP-fed system effluents had concentrations around the detection limit. Monochloramine, the form of chloramine expected at the influent pH 8.9, is known to be converted by reactions with activated carbon to chloride, ammonia and N2.41 The likely presence of ammonia (which was, unfortunately, not measured) would be available to support bacterial growth on the filter and should be compared to the performance of PoU filters in free chlorine-treated systems where ammonia would, presumably, be much less. The increase in Nitrospira relative abundance across all effluent samples also suggests the presence of sufficient ammonia to support the strain's growth.
There was no significant difference between average TOC and DOC concentrations in the influent samples (p = 0.45), indicating that the measurable organic carbon introduced to the filters was dissolved (Table S10†). Influent TOC concentrations ranged between 1.8 and 3.7 mg L−1 with a mean concentration of 2.4 mg L−1 (with and without PCP). Effluent TOC values ranged between less than 1.0 and 6.0 mg L−1 with a mean concentration of 2.1 mg L−1 (with and without PCP) (Table S11†). Occasional sloughing of cell biomass or periodic generation of soluble microbial products may account for the effluent TOC variability. TOC removals were not statistically different between treatments (Fig. S8†). The net average organic carbon mass removed by the PoU filters was 343 ± 208 mg, whereas the total average mass of organic carbon fed to the filters was 1136 ± 99 mg for the duration of the experiment. TOC was detected in all effluent samples analysed, starting approximately two weeks into the experiment, suggesting rapid breakthrough and would have been available to support bacterial growth in the effluent.
Changes in chemical species across the filter, coupled with the correlation between dominant taxa in the fabric and effluent communities, led us to estimate the absolute abundances of highly dominant OTUs across sample locations between PCP treatments by multiplying the total 16S rRNA gene copies by relative abundance. Although this method is just an estimate, it allows us to observe trends in how highly dominant OTUs change between the influent and effluent of the PoU systems. Sphingopyxis (OTU1), Aquabacterium (OTU5), Acidovorax (OTU2), and Nitrospira (OTU20) had higher absolute abundance in the effluent than in the influent for all PCP treatments (Table 2). Brevundimonas (OTU30), Mycobacterium (OTU3), and an unclassified betaproteobacteria (OTU10) had higher absolute abundance in the effluent relative to the influent of systems treated with 50 and 100 ng L−1 PCP. Among the genera that are known to contain opportunistic pathogens found in drinking water, the most significant among the dominant taxa found in this study is Mycobacterium (OTU 3); however, the sequencing amplicon size does not allow us to identify if pathogenic forms were present. Of equal importance is the absence of taxa linked to opportunistic pathogens in our study but that have been historically observed in drinking water. Four Legionella OTUs were detected but at trace abundance (total no. of reads ≤36) and there was no consistent pattern of change in abundance across the PoU filters. Likewise, three Pseudomonas sp. were detected in very low abundance (total no. of reads ≤39). Only one Pseudomonas taxon (OTU24) was higher than 1% in the influent samples, and its abundance decreased across the filter (Fig. 4). The partial 16S rRNA sequence (253 bp) of all Pseudomonas OTUs was evaluated via a BLAST search and none included P. aeruginosa as an associated taxon. The Mycobacterium data show that PoU filters may be able to increase the abundance of selected opportunistic pathogens across the flow path of activated carbon block PoU filters.
Table 2 Estimated absolute abundances of highly dominant OTUs in PoU filter influent, fabric and effluent samples across all PCP treatmentsa
Highly dominant taxa (OTU#) |
0 ng L−1 PCP |
50 ng L−1 PCP |
100 ng L−1 PCP |
Influent |
Effluent |
Influent |
Effluent |
Influent |
Effluent |
Estimated absolute abundance = Relative abundance (%) × total 16S rRNA gene (copy no./L)/4.2 copies/genome. The average copy no. of 16S rRNA genes is 4.2 copy no. per cell.70 The total number of 16S rRNA genes were [influent]: 2 × 106 in 0 ng L−1, 2 × 104 in 50 ng L−1; 4 × 104 in 100 ng L−1 PCP treatment; [effluent]: 3 × 106 in 0 ng L−1, 5 × 106 in 50 ng L−1; 4 × 106 in 100 ng L−1 PCP treatment. Values in bold are the OTUs that have higher estimated abundance in effluent than in influent.
|
Hydrogenophaga (4) |
6E + 04 |
1E + 05
|
5E + 02 |
2E + 05
|
1E + 03 |
2E + 05
|
Unclassified Bacteroidetes (19) |
3E + 04 |
0E + 00 |
2E + 02 |
0E + 00 |
6E + 02 |
2E + 01 |
Unclassified Bacteriodetes (12) |
3E + 04 |
1E + 02 |
2E + 02 |
1E + 02 |
5E + 02 |
1E + 02 |
Brevundimonas (30) |
2E + 04 |
2E + 03 |
1E + 02 |
4E + 03
|
4E + 02 |
3E + 03
|
Unclassified Betaproteobacteria (10) |
2E + 04 |
5E + 03 |
2E + 02 |
3E + 03
|
5E + 02 |
1E + 04
|
Sphingopyxis (1) |
2E + 03 |
1E + 04
|
1E + 01 |
4E + 04
|
4E + 01 |
4E + 04
|
Mycobacterium (3) |
4E + 03 |
2E + 03 |
3E + 02 |
2E + 04
|
7E + 02 |
2E + 04
|
Aquabacterium (5) |
1E + 04 |
2E + 04 |
1E + 02 |
4E + 04
|
4E + 02 |
2E + 04
|
Acidovorax (2) |
8E + 03 |
3E + 04
|
8E + 01 |
6E + 04
|
2E + 02 |
6E + 04
|
Unclassified Sphingomonadaceae (14) |
6E + 02 |
8E + 03 |
0E + 00 |
8E + 03
|
6E + 00 |
5E + 02
|
Unclassified Proteobacteria (7) |
3E + 02 |
2E + 05
|
2E + 00 |
3E + 05
|
1E + 01 |
3E + 05
|
Unclassified Betaproteobacteria (15) |
7E + 02 |
6E + 04
|
7E + 00 |
3E + 04
|
2E + 01 |
4E + 04
|
Unclassified bacteria (9) |
3E + 03 |
6E + 04
|
2E + 01 |
9E + 04
|
5E + 01 |
9E + 04
|
Nitrospira (20) |
2E + 03 |
4E + 04
|
2E + 01 |
5E + 04
|
3E + 01 |
4E + 04
|
Discussion
PoU filters supported biofilm growth upstream of the activated carbon block
Calculations show that most of the biofilm found on the fabric was due to growth that occurred during the two month PoU study. We calculated the protein mass that would exist on the fabric if all cells from the influent over the course of the study were retained, assuming our plate counts only captured 10% of the total bacterial population (ESI† section 14), and estimated that fabrics would have 4 μg cell protein per cm2. We also calculated the amount of biomass that would grow on the total mass of PCP applied to the PCP-supplemented filter systems, assuming at least one bacterial strain was present that could mineralize all the PCP applied (ESI† section 15). For this scenario, we calculated that the 100 ng L−1 PCP fed fabric would support growth of 2.5 × 10−2 μg cell protein per cm2. Both estimates are six to 2000 times smaller than the average fabric cell protein measured, which was 23 μg cm−2. Therefore, we conclude that substantial cell growth occurred on the fabric over the course of the study, presumably on sorbed TOC, soluble TOC and microbial decay products; however, PCP did not significantly support growth.
PoU filtration alters bacterial abundance and community composition in filtered drinking water
Our results show that ACB PoU filters increase the abundance of bacteria in filtered drinking water relative to what is present in the premise plumbing that supplies the PoU filter, and are consistent with the results of past PoU filter studies that used culture-based methods.8–14 Despite this increase, there is a decrease in bacterial community diversity (richness and evenness) across the filters, which could be linked to changes in BDOC (biodegradable organic carbon) across the filter. The historical average BDOC concentration in the source water was 1.3–1.6 mg L−1,42 which is 60% of the TOC measured during our study, suggesting that most of the TOC was available to support microbial growth. This growth would be focused in the biofilm, upstream of the activated carbon block within range of the carbon surface where the TOC would adsorb. Although we did not measure the composition of TOC in effluent versus influent samples, we expect that the nature of effluent organic carbon was different and could have been more recalcitrant in the effluent as is often seen during conventional drinking water treatment that invokes biologically active GAC.43 A reduction in BDOC across filters could explain the dominance of the community by only a handful of OTUs with an ability to grow well on the few biodegradable organic constituents present, thus resulting in decreased microbial richness in the effluent.
It is noteworthy that the influent bacteria are likely to be predominantly planktonic1,44 while the fabric bacteria survive by growing in biofilms; therefore, planktonic bacteria that can transition to biofilm growth are likely favoured for growth on the fabric wrap of ACB PoU filters. Other studies of community structure in drinking water distribution systems have shown that the planktonic bacteria present in bulk water are different from sessile bacteria that grow in biofilms on the walls and scale of the same pipe systems,45 suggesting that the predominant planktonic bacteria do not favour biofilm growth but are not necessarily precluded from it. The fate of planktonic bacteria derived from the distribution system and applied to an activated carbon block PoU filter, like that used in our study, is unclear. It is possible that bacteria that grow preferentially in a planktonic state are caught by the biofilm through sieving and either transition to a biofilm growth state or eventually succumb to death and lysis, which provides nutrients that support the growth of biofilm-thriving strains.
We hypothesize that the community composition differences observed in the fabric biofilm are a complex outcome of the mode of operation and the structure of the filter. The PoU filter fabric is wrapped around and in close contact with the surface of the activated carbon block; bacteria can grow on the activated carbon block surface (not measured by us in this study) and also on the fabric wrap (measured during this study), and constitute upstream biomass. These microorganisms would be exposed to hourly (<1 min) pulses of residual chloramine disinfectant as it flows to the block from the influent for 16 hours; then no new flow for an 8 hour stagnation period where substantial growth could occur. The pulsed growth environment in the filter housing is, admittedly, quite different from what is common in distribution systems. The biofilm bacteria would also be exposed to BDOC that sorbed to the activated carbon block and is in equilibrium with the liquid zone immediately adjacent to the ACB surface. Furthermore, the ACB and fabric biofilms would have also been exposed to equilibrium concentrations of PCP and disinfection by-products that also sorbed to the ACB surface, and could have resulted in the selection of taxa that are able to resist the stress effects of these chemicals and grow on them. Indeed, the fabric-abundant OTUs including Sphingopyxis, Sphingomonas, Mycobacterium, and Acidovorax are of genera known to include species capable of degrading chlorinated compounds.46–49 Our study did not evaluate growth kinetic differences among different OTUs found on the filters; however, it appears that these multiple factors affected growth in a way that reduced the community diversity across the flow path.
The increase in effluent HPC with time and the structurally distinct composition of effluent bacterial communities suggest that bacteria colonized and grew inside the filter annular space in a way that inoculated the effluent water flow. Theoretically, bacteria should not pass through an intact activated carbon block since its average pore size (22 Å measured during this study) is much smaller than a bacterial cell. The observed passage of bacteria through the filter, however, indicates the likely presence of a few preferential flow paths of larger than average diameter. Another possible source of inoculum for the effluent community is residual bacteria from the manufacturing process itself. Further study of the transport pathway in ACB filters is needed to test these two hypotheses and is currently underway in our laboratory. Although the inoculum source(s) for the effluent channel remain(s) unknown, the significant increase in effluent cell counts relative to the influent suggests that bacteria colonized and grew on the activated carbon face of the effluent chamber or passed through the block from the fabric biofilm and influent. Since our effluent samples were composited over 24 hours, it is possible that the discharge of bacteria via the effluent was sporadic and occurred when biofilm detachment or sloughing events occurred.
It is interesting that Nitrospira became abundant in the effluent relative to levels in the influent across all filters, although we have not confirmed which species were present. The likely presence of ammonia as a product of the reaction of chloramine with the ACB may have supported the growth of the nitrifier and should be compared to the performance of PoU filters in free chlorine-treated systems where ammonia concentrations would, presumably, be much less. Pinto et al.50 found comammox in Ann Arbor's distribution system; a metabolism performed by some Nitrospira, which suggests complete nitrification to nitrate, is possible. Perhaps more importantly, these results imply that there is a path for bacteria from the distribution system through the filter to the effluent. In this way, Nitrospira is serving as a non-pathogenic bacterial tracer that is unlikely to have been inoculated by the manufacturing process.
Trace concentrations of PCP shifted the bacterial community structure and abundance in the fabric and effluent
As hypothesized, PCP influenced the composition of bacterial biofilm in PoU filters, though the shifts were subtle. The total biomass amount did not change with PCP exposure, however. Three dominant OTUs in the fabric and effluent shifted with PCP exposure: Mycobacterium in fabric and effluent, Sphingomonas in fabric, and Sphingopyxis in effluent. Pseudomonas aeruginosa, the strain we based our hypothesis on, was not present at a detectable abundance during this study. Although none of the affected taxa contain the resistance–nodulation–division (RND) MexAB-OprM multidrug efflux pump associated with our initial hypothesis, Mycobacteria contain RND homologs called Mycobacterial membrane protein large transporters (MmpLs) that are associated with exporting multiple antibiotics and may play a role in detoxification-mediated efflux of other chemicals.51,52 Furthermore, Sphingopyxis and Sphingomonas also have RND homologs53,54 and belong to the family of Sphingomonadaceae that are known to be chlorine-resistant.55,56 Some strains of Sphingomonas found in drinking water systems have also demonstrated antibiotic resistance.56,57 The mechanisms that lead to the selection of these taxa in the presence of PCP are unclear. The impact on the biofilm communities was apparent phenotypically as well, as the biofilm color changed in response to PCP. We cannot determine whether this was due to the subtle shifts in composition or physiological changes in response to PCP. The bacterial communities in the effluent of filters where PCP was added to the influent were different from those in the non-PCP control effluent, even though we did not detect PCP in any effluent sample (detection limit, 10 ng L−1). It is not clear what factors affected the effluent communities. Collectively, and although mechanisms have not been elucidated, these data show that chemicals present at trace (ng L−1) concentrations were able to influence the community structure in a PoU filter. This suggests that disinfection by-products can influence the microbial community found in PoU filters. Although our experiments only focused on PCP, these results provide motivation to evaluate the impact of other DBPs on microbial communities in ACB PoU filters to determine if the phenomena observed in this study are typical.
The abundance of Mycobacteria is affected by the activated carbon block PoU filter
The Mycobacterium genus was among the five dominant OTUs that were enriched on all fabric samples under all treatment conditions, and Mycobacterium was also enriched in the effluent relative to the influent treated with PCP. Numerous species of nontuberculosis Mycobacteria (NTM) have been found in drinking water,58–60 including in Ann Arbor drinking water.61 Genetic and epidemiological methods have been employed to show that NTM may cause infection to immuno-compromised humans.62,63 Due to their hydrophobic cell wall component, Mycobacteria have strong resistance to disinfectants, especially chloramine,64 and easily adhere to form biofilms in drinking water systems.65–68 Adding to their tolerance of disinfectants is their ability to co-associate with amoeba in drinking water distribution systems, which provides protection against disinfectants.69 Amoeba would be readily retained by the PoU filters given their size, consistent with testing done during certification of the filters.5 In summary, the selection of Mycobacterium across the activated carbon block PoU filters in this study shows that filters may increase one's exposure to opportunistic pathogens that are otherwise present at lower numbers in distributed drinking water.
Deployment of faucet-mounted, activated carbon block PoU filters is on the rise, especially in the wake of very public incidents of public drinking water contamination. These filters are primarily and justifiably used to remove non-biological contaminants that the filters are certified to remove. An unintended consequence of using ACB PoU filters, however, is that they change the structure and abundance of the microbial community that consumers are exposed to. This community shift is influenced by both the architecture of the filter and, to a lesser degree, trace chemicals present in the water. Indeed, the World Health Organization has warned against the use of activated carbon block filters as a sole point-of-use treatment method for over two decades.7 The microbiological changes experienced across these filters may be particularly problematic for immune-compromised individuals who are vulnerable to infection, which justifies considering the use of an additional protective barrier to provide disinfection; however, further study is needed to determine whether and under what conditions this is needed. Our results provide an initial assessment of the consequence of deploying point-of-use treatment on distributed drinking water systems and suggest a need to better understand the public health consequences of this choice.
Acknowledgements
The authors are grateful to Angelica Perez De La Rosa and Gwendolyn Ryskamp for their contributions through laboratory work associated with this study. This material is based upon work supported by the National Science Foundation under Grant No. CBET-1067450. Any opinions, findings, and conclusions or recommendations expressed in this material are those of the author(s) and do not necessarily reflect the views of the National Science Foundation.
References
- S. Gillespie, P. Lipphaus, J. Green, S. Parsons, P. Weir, K. Juskowiak, B. Jefferson, P. Jarvis and A. Nocker, Water Res., 2014, 65, 224–234 CrossRef CAS PubMed.
- H. Wang, S. Masters, M. A. Edwards, J. O. Falkinham and A. Pruden, Environ. Sci. Technol., 2014, 48, 1426–1435 CrossRef CAS PubMed.
- L. F. Stanish, N. M. Hull, C. E. Robertson, J. K. Harris, M. J. Stevens, J. R. Spear and N. R. Pace, PLoS One, 2016, 11, e0157966 Search PubMed.
-
Freedonia, Consumer Water & Air Treatment Systems to 2017 - Industry Market Research, Market Share, Market Size, Sales, Demand Forecast, Market Leaders, Company Profiles, Industry Trends, 2013 Search PubMed.
-
NSF International/ANSI, Standard 53 2015a: Drinking Water Treatment Units—Health Effects, Ann Arbor, 2015 Search PubMed.
-
NSF International/ANSI, Standard 42 2015b : Drinking Water Treatment Units—Aesthetic Effects, Ann Arbor, 2015 Search PubMed.
-
M. D. Sobsey, Managing Water in the Home : Accelerated Health Gains from Improved Water Supply, Geneva, 2002 Search PubMed.
- R. S. Tobin, D. K. Smith and J. A. Lindsay, Appl. Environ. Microbiol., 1981, 41, 646–651 CAS.
- C. Wallis, C. H. Stagg and J. L. Melnick, Water Res., 1974, 8, 111–113 CrossRef.
- E. E. Geldreich, R. H. Taylor, J. C. Blannon and D. J. Reasonrer, J. - Am. Water Works Assoc., 1985, 77, 72–80 Search PubMed.
- S. L. Molloy, R. Ives, A. Hoyt, R. Taylor and J. B. Rose, J. Appl. Microbiol., 2008, 104, 998–1007 CrossRef CAS PubMed.
- D. J. Reasoner, J. C. Blannon and E. E. Geldreich, J. - Am. Water Works Assoc., 1987, 60–66 CAS.
- C. Chaidez and C. Gerba, Int. J. Environ. Health Res., 2004, 14, 253–260 CrossRef PubMed.
- F. Su, M. Luo, F. Zhang, P. Li, K. Lou and X. Xing, J. Environ. Sci., 2009, 21, 1237–1246 CrossRef CAS.
- L. M. Rebenne, A. C. Gonzalez and T. M. Olson, Environ. Sci. Technol., 1996, 30, 2235–2242 CrossRef CAS.
- V. L. Heasley, A. M. Fisher, E. E. Herman, F. E. Jacobsen, E. W. Miller, A. M. Ramirez, N. R. Royer, J. M. Whisenand, D. L. Zoetewey and D. F. Shellhamer, Environ. Sci. Technol., 2004, 38, 5022–5029 CrossRef CAS PubMed.
- S. Ghosh, C. M. Cremers, U. Jakob and N. G. Love, Mol. Microbiol., 2011, 79, 1547–1556 CrossRef CAS PubMed.
- J. L. Martinez, M. B. Sanchez, L. Martinez-Solano, A. Hernandez, L. Garmendia, A. Fajardo and C. Alvarez-Ortega, FEMS Microbiol. Rev., 2009, 33, 430–449 CrossRef CAS PubMed.
- H. Maseda, I. Sawada, K. Saito, H. Uchiyama, T. Nakae and N. Nomura, Antimicrob. Agents Chemother., 2004, 48, 1320–1328 CrossRef CAS PubMed.
- B. B. Sithole and D. T. Williams, J. Assoc. Off. Agric. Chem., 1986, 69, 807–810 CAS.
- J. Michałowicz, Pol. J. Environ. Stud., 2005, 14, 327–333 Search PubMed.
- Y. Zhao, J. Anichina, X. Lu, R. J. Bull, S. W. Krasner, S. E. Hrudey and X.-F. Li, Water Res., 2012, 46, 4351–4360 CrossRef CAS PubMed.
- APHA, AWWA and WEF, Standard Methods
for the Examination of Water and Wastewater, American Public Health Association, Washinton DC., 21st edn, 2005 Search PubMed.
- C. Pellicer-Nàcher, C. Domingo-Félez, A. G. Mutlu and B. F. Smets, Water Res., 2013, 47, 5564–5574 CrossRef PubMed.
- T. Masuko, A. Minami, N. Iwasaki, T. Majima, S.-I. Nishimura and Y. C. Lee, Anal. Biochem., 2005, 339, 69–72 CrossRef CAS PubMed.
- P. K. Smith, R. I. Krohn, G. T. Hermanson, A. K. Mallia, F. H. Gartner, M. D. Provenzano, E. K. Fujimoto, N. M. Goeke, B. J. Olson and D. C. Klenk, Anal. Biochem., 1985, 150, 76–85 CrossRef CAS PubMed.
- J. J. Kozich, S. L. Westcott, N. T. Baxter, S. K. Highlander and P. D. Schloss, Appl. Environ. Microbiol., 2013, 79, 5112–5120 CrossRef CAS PubMed.
- J. G. Caporaso, C. L. Lauber, W. A. Walters, D. Berg-Lyons, J. Huntley, N. Fierer, S. M. Owens, J. Betley, L. Fraser, M. Bauer, N. Gormley, J. A. Gilbert, G. Smith and R. Knight, ISME J., 2012, 6, 1621–1624 CrossRef CAS PubMed.
- P. D. Schloss, S. L. Westcott, T. Ryabin, J. R. Hall, M. Hartmann, E. B. Hollister, R. A. Lesniewski, B. B. Oakley, D. H. Parks, C. J. Robinson, J. W. Sahl, B. Stres, G. G. Thallinger, D. J. Van Horn and C. F. Weber, Appl. Environ. Microbiol., 2009, 75, 7537–7541 CrossRef CAS PubMed.
- Q. Wang, G. M. Garrity, J. M. Tiedje and J. R. Cole, Appl. Environ. Microbiol., 2007, 73, 5261–5267 CrossRef CAS PubMed.
- A. Chao and T.-J. Shen, Environ. Ecol. Stat., 2003, 10, 429–443 CrossRef.
- T. C. J. Hill, K. A. Walsh, J. A. Harris and B. F. Moffett, FEMS Microbiol. Ecol., 2003, 43, 1–11 CrossRef CAS PubMed.
- J. Evans, L. Sheneman and J. Foster, J. Mol. Evol., 2006, 62, 785–792 CrossRef CAS PubMed.
- C. Lozupone and R. Knight, Appl. Environ. Microbiol., 2005, 71, 8228–8235 CrossRef CAS PubMed.
- C. A. Lozupone, M. Hamady, S. T. Kelley and R. Knight, Appl. Environ. Microbiol., 2007, 73, 1576–1585 CrossRef CAS PubMed.
- A. Chao, R. L. Chazdon, R. K. Colwell and T.-J. Shen, Ecol. Lett., 2005, 8, 148–159 CrossRef.
- P. D. Schloss, ISME J., 2008, 2, 265–275 CrossRef PubMed.
- N. Fierer, J. A. Jackson, R. Vilgalys and R. B. Jackson, Appl. Environ. Microbiol., 2005, 71, 4117–4120 CrossRef CAS PubMed.
- J. T. Trevors, J. Microbiol. Methods, 2011, 86, 266–273 CrossRef CAS PubMed.
- Å. Frostegård, S. Courtois, V. Ramisse, S. Clerc, D. Bernillon, F. Le Gall, P. Jeannin, X. Nesme and P. Simonet, Appl. Environ. Microbiol., 1999, 65, 5409–5420 Search PubMed.
- J. D. Komorita and V. L. Snoeyink, J. - Am. Water Works Assoc., 1985, 62–64 CAS.
- J. Lee and R. A. Deininger, Ozone: Sci. Eng., 2003, 25, 473–484 CrossRef CAS.
- C. J. Volk and M. W. Lechevallier, J. - Am. Water Works Assoc., 2002, 94, 112 CAS.
- F. Hammes, M. Berney, Y. Wang, M. Vital, O. Köster and T. Egli, Water Res., 2008, 42, 269–277 CrossRef CAS PubMed.
- G. Liu, G. L. Bakker, S. Li, J. H. G. Vreeburg, J. Q. J. C. Verberk, G. J. Medema, W. T. Liu and J. C. Van Dijk, Environ. Sci. Technol., 2014, 48, 5467–5476 CrossRef CAS PubMed.
- F. Godoy, M. Vancanneyt, M. Martinez, A. Steinbuchel, J. Swings and B. H. A. Rehm, Int. J. Syst. Evol. Microbiol., 2003, 53, 473–477 CrossRef CAS PubMed.
- C.-F. Yang, C.-M. Lee and C.-C. Wang, Chemosphere, 2006, 62, 709–714 CrossRef CAS PubMed.
- M. M. Häggblom, L. J. Nohynek and M. S. Salkinoja-Salonen, Appl. Environ. Microbiol., 1988, 54, 3043–3052 Search PubMed.
- M. V. Monferra, R. J. Echenique and D. A. Wunderlin, Chemosphere, 2005, 61, 98–106 CrossRef PubMed.
- A. J. Pinto, D. N. Marcus, Z. Ijaz, Q. M. Bautista-de los Santos, G. J. Dick and L. Raskin, mSphere, 2015, 1, e00054 Search PubMed.
- M. R. Pasca, P. Guglierame, E. De Rossi, F. Zara and G. Riccardi, Antimicrob. Agents Chemother., 2005, 49, 4775–4777 CrossRef CAS PubMed.
- C. Chalut, Tuberculosis, 2016, 100, 32–45 CrossRef CAS PubMed.
- M. Shintani, M. Urata, K. Inoue, K. Eto, H. Habe, T. Omori, H. Yamane and H. Nojiri, J. Bacteriol., 2007, 189, 2007–2020 CrossRef CAS PubMed.
- Universal Protein Resource, Heavy metal efflux pump CzcA in Shpingopyxis alaskensis, June 27, 2006, http://www.uniprot.org/uniprot/Q1GQB6, accessed April 27 2017.
- K. Furuhata, Y. Kato, K. Goto, K. Saitou, J.-I. Sugiyama, M. Hara and M. Fukuyama, Biocontrol Sci., 2007, 12, 39–46 CrossRef CAS PubMed.
- S. Khan, T. K. Beattie and C. W. Knapp, Chemosphere, 2016, 152, 132–141 CrossRef CAS PubMed.
- I. Vaz-Moreira, O. C. Nunes and C. M. Manaia, Appl. Environ. Microbiol., 2011, 77, 5697–5706 CrossRef CAS PubMed.
- M. J. M. Vaerewijck, G. Huys, J. C. Palomino, J. Swings and F. Portaels, FEMS Microbiol. Rev., 2005, 29, 911–934 CrossRef CAS PubMed.
- H. Wang, M. Edwards, J. O. Falkinham and A. Pruden, Appl. Environ. Microbiol., 2012, 78, 6285–6294 CrossRef CAS PubMed.
- E. D. Hilborn, T. C. Covert, M. A. Yakrus, S. I. Harris, S. F. Donnelly, E. W. Rice, S. Toney, S. A. Bailey and G. N. J. Stelma, Appl. Environ. Microbiol., 2006, 72, 5864–5869 CrossRef CAS PubMed.
- T.-H. Chiao, T. M. Clancy, A. Pinto, C. Xi and L. Raskin, Environ. Sci. Technol., 2014, 48, 4038–4047 CrossRef CAS PubMed.
- J. O. Falkinham, Emerging Infect. Dis., 2011, 17, 419–424 CrossRef PubMed.
- J. O. Falkinham, M. D. Iseman, P. de Hass and D. van Soolingen, J. Water Health, 2008, 6, 209–213 Search PubMed.
- J. L. Baron, A. Vikram, S. Duda, J. E. Stout and K. Bibby, PLoS One, 2014, 9, e102679 Search PubMed.
- M. M. Williams, M. A. Yakrus, M. J. Arduino, R. C. Cooksey, C. B. Crane, S. N. Banerjee, E. D. Hilborn and R. M. Donlan, Appl. Environ. Microbiol., 2009, 75, 2091–2098 CrossRef CAS PubMed.
- R. P. Revetta, V. Gomez-Alvarez, T. L. Gerke, C. Curioso, J. W. S. Domingo and N. J. Ashbolt, FEMS Microbiol. Ecol., 2013, 86, 404–414 CrossRef CAS PubMed.
- S. N. Mullis and J. O. Falkinham, J. Appl. Microbiol., 2013, 115, 908–914 CrossRef CAS PubMed.
- C. K. Gomez-Smith, T. M. LaPara and R. M. Hozalski, Environ. Sci. Technol., 2015, 49, 8432–8440 CrossRef CAS PubMed.
- V. Delafont, F. Mougari, E. Cambau, M. Joyeux, D. Bouchon, Y. Hechard and L. Moulin, Environ. Sci. Technol., 2014, 48, 11872–11882 CrossRef CAS PubMed.
- T. Vetrovsky and P. Baldrian, PLoS One, 2013, 8, e57920 Search PubMed.
Footnotes |
† Electronic supplementary information (ESI) available. See DOI: 10.1039/c7ew00134g |
‡ Associated with the University of Michigan during the project period. |
|
This journal is © The Royal Society of Chemistry 2017 |
Click here to see how this site uses Cookies. View our privacy policy here.