A strategic screening approach to identify transformation products of organic micropollutants formed in natural waters†
Received
25th November 2016
, Accepted 14th February 2017
First published on 14th February 2017
Abstract
Many transformation products (TPs) from organic micropollutants are not included in routine environmental monitoring programs due to limited knowledge of their occurrence and fate. An efficient method to identify and prioritize critical compounds in terms of environmental relevance is needed. In this study, we applied a strategic screening approach based on a case–control concept to identify TPs formed along wastewater-impacted rivers. Time-integrated samples were collected over one week at both ends of a river stretch downstream of a wastewater treatment plant (WWTP) outfall and were analyzed by ultrahigh performance liquid chromatography interfaced with quadrupole time-of-flight mass spectrometry (UHPLC-QToF-MS/MS). The screening procedure of the high-resolution MS (HRMS) datasets consisted of three major steps: (i) screening for parent compounds (PCs) attenuated along the stretch; (ii) prediction of potential TPs from these PCs; and (iii) screening for TPs from this list with an increasing trend along the stretch. In total, 32 PCs decreased along the investigated river stretches. From these PCs, eight TPs had increasing concentrations along the studied stretches and could be tentatively identified. The identification of one TP (benzamide) was confirmed by its corresponding reference standard, while no standards were available for the remaining TPs.
Environmental impact
Organic micropollutants are continuously discharged from wastewater treatment plants into receiving aquatic systems where they undergo further transformation. The number of unknown transformation products is large; hence prioritizations for analysis and risk assessment are needed. We established a strategic screening approach to identify micropollutant transformation products formed in wastewater-impacted rivers. The essential case–control concept effectively allocates attenuating parent micropollutants and transformation products generated in situ. Our screening concept can be applied in process-oriented studies at both laboratory and field scale to identify unknown transformation products prioritized for further investigation. EU chemical legislation calls for hazard assessments of formed transformation products, when motivated. The concept presented in this study can help to identify such transformation products, and thereby contribute to a more comprehensive risk assessment for parent chemicals.
|
Introduction
Surface water in urban and agriculturally influenced catchments contains a large number of organic micropollutants such as pesticides, pharmaceuticals and personal care products (PPCPs) as well as industrial chemicals.1–5 This is because the removal of micropollutants during conventional chemical and/or biological wastewater treatment processes is often incomplete.4,6 As a consequence, organic micropollutants and their transformation products (TPs) are emitted from wastewater treatment plants (WWTPs)7 into receiving aquatic systems where they can undergo further transformation.5,8–10 Previous research has demonstrated that certain TPs can be as toxic, or even more toxic than their parent compounds (PCs).11–15 Yet, the identities of many TPs are still unknown, resulting in a limited understanding of their environmental fate and ecotoxicity.
In the past few years, a rapidly growing number of studies have focused on suspect and/or non-target screening to identify currently overlooked pollutants in water samples.16–21 These techniques are enabled by high-resolution mass spectrometry (HRMS). Most studies are initially based on laboratory experiments at elevated concentrations to facilitate the identification of TPs,22–24 which are then searched for in environmental samples.16,25,26 Strategies have also been developed to assist and simplify the detection of TPs directly in natural waters and wastewater, based on the use of characteristic fragmentation27,28 or mass defect filtering.29 Such applications mostly contribute with information on the occurrence of TPs18,25 likely formed under the conditions during conventional wastewater treatment processes and emitted directly from the WWTPs.7 However, there is less information available on TPs that are formed in natural waters after discharge of their PCs.
In the present work we applied a screening approach based on a case–control concept30 to identify the TPs that are formed from organic micropollutants along four typical wastewater-impacted rivers. By comparing the full-scan datasets obtained from the samples collected from a downstream site to those from an upstream site (i.e., the control samples), we were able to rapidly allocate those PCs that showed a decreasing trend and their corresponding TPs that were formed along the river stretch.
Experimental methods
Chemicals and reagents
High-purity standards for PCs and – if available – TPs were obtained from Sigma-Aldrich (Steinheim, Germany), Wellington Labs (Toronto, Canada) and Riedel-de Haën (Seelze, Germany) and were stored under the recommended conditions until use. Ultra-gradient HPLC grade acetonitrile and methanol were obtained from Merck (Darmstadt, Germany). A Milli-Q-Plus ultra-pure water system from Millipore (Milford, MA, USA) was used. High purity formic acid was obtained from Sigma-Aldrich. Stock solutions of the standards were prepared in methanol and were stored in amber screw-capped glass vials in the dark at −20 °C. A standard working solution containing 65 analytes was prepared in methanol at 1 mg L−1. These analytes are part of an already validated target analysis method that were used to confirm the presence of PCs in this study (see Table S1 in ESI† for a complete list). A recovery standard mixture containing the isotope-substituted compounds 2,4-dichlorophenoxyacetic acid–13C, caffeine–13C, codeine-d3, gabapentin-d10, venlafaxine-d6, carbamazepine-d10, fluoxetine-d10, temazepam-d5, simazine-d10, acesulfame-d4, diuron-d6, atrazine-d5 and atenolol-d7 was prepared in methanol. Acetylsulfamethoxazole-d4 and 2,4-dichlorophenylacetic acid were prepared in methanol and used as injection standard.
Sampling
Sampling was carried out in four small- to medium-sized European wastewater-impacted rivers, i.e., Gründlach (GR) in Germany, Fyris (FY), Rönne (RO), and Viskan (VI) in Sweden. The selection criteria and characteristics of the studied rivers are discussed in detail in Li et al. (2016), with maps for the sampling area provided.7 Water was concurrently sampled over a period of one week in June–August 2014 using automatic samplers (3700 compact, equipped with Teflon suction line and stainless steel inlet filter; Teledyne ISCO, Lincoln, NE) at both ends of a stretch downstream of a WWTP outfall. Site A in each river system was located after the complete mixing of the WWTP effluent with the river water, while site B was located 6–12 km further downstream but before the next main source of organic micropollutants into the river (e.g. a major confluence or effluent from other WWTPs). Every hour 50 mL of water was sampled at a depth of approximately 20 cm below the water surface, and 24 consecutive samples were combined to get one daily composite sample. At the end of the sampling period, aliquots of these daily samples were combined to give a weekly composite sample for each site. During the sampling period, all samples were kept in darkness in the sample container filled with ice to immediately refrigerate the samples. Samples were stored in 1 L HDPE containers and transported frozen to the laboratory.
Analytical method
Extraction.
Composite river samples (100 mL; including one upstream and one downstream sample from each of the four rivers) were spiked with the recovery standard mixture (final concentration 50 μg L−1) before solid phase extraction (SPE) with Oasis® HLB cartridges (6 cc, 200 mg). SPE cartridges were preconditioned with 4 mL methanol followed by 4 mL Milli-Q water prior to sample loading. Following sample loading, the SPE cartridges were left to dry under low vacuum for one hour and then were extracted twice with 2 mL methanol. The eluents were evaporated to approximately 1 mL using a 40 °C heating block and a gentle stream of nitrogen. The extracts were filtered through a syringe fitted with a 0.22 μm PTFE filter, and transferred to 1.5 mL glass vials. Sample volumes were further reduced under a gentle stream of nitrogen to 200 μL and made up to their final volume of 500 μL with 20
:
80 methanol
:
Milli-Q water. All samples were spiked with the injection standard (final concentration: 10 μg L−1) prior to analysis.
UHPLC-QToF-MS/MS analysis.
Chromatographic separation of the analytes was achieved using a Shimadzu Nexera X2 UHPLC system equipped with a binary pump. A reverse-phase XDB-C18 analytical column (4.6 mm × 50 mm and 1.8 μm particle size; Agilent Technologies, Santa Clara, CA) was used for negative mode and a XDB-C18 column (2.1 mm × 100 mm and 1.8 μm particle size, Agilent Technologies) for positive mode. For compounds analyzed using the negative ionization mode, 1% methanol in Milli-Q water was used as mobile phase A and 10% Milli-Q water in methanol as mobile phase B, with 5 mmol L−1 ammonium acetate in both phases. The initial gradient (10% B) was held for 0.2 min followed by a gradient ramp to 100% B within 6.5 min and maintained for 3.0 min, then the gradient returned to the initial composition within 0.1 min, followed by equilibration for 2.2 min. The injection volume was 10 μL. The column unit was held at 45 °C with a flow rate of 0.6 mL min−1. For analysis in positive ionization mode mobile phases A and B were Milli-Q water and methanol, respectively, both containing 0.1% formic acid. A linear gradient ramped from 5% B to 100% B within 10 min, and then was kept for 4.5 min at 100% B before returning to the initial conditions within 0.1 min, followed by equilibration for 2.0 min. The flow rate was 0.4 mL min−1; the column oven was held at 50 °C. The injection volume was 5 μL. The analytical columns and mobile phases differed between positive and negative ionization modes to optimize detection and retention of the compounds of interest.
The UHPLC was coupled to a hybrid QToF mass spectrometer (TripleTOF 5600 System, AB Sciex, Framingham, MA, USA) with an electrospray ionization (ESI) interface working in negative and positive ionization modes. The ion source parameters were optimized to the following final conditions: ion spray voltage floating (ISVF) of −4500 V and 5500 V for negative and positive ionization, respectively; temperature (TEM) of 600 °C; curtain gas (CUR) of 35 L min−1 and ion source gas (GS1 and GS2) of 70 psi. High purity nitrogen was used as the nebulizer gas, curtain gas, and collision gas. The declustering potential (DP) and collision energy (CE) were (−)80 V and (−)10 eV. The MS was operated under full-scan ToF-MS (m/z 100–950) and MS/MS mode (m/z 30–950) through Information Dependent Acquisition (IDA) in single run analysis. The criteria for the IDA experiment were: (i) an intensity threshold of 500 counts per second; (ii) a mass range of 30 to 950 Da and; and (iii) a mass tolerance of 10 ppm.
Mass calibration and resolution adjustments were performed automatically using a 10−5 mol L−1 solution of polypropylene glycol introduced via a syringe pump connected to the interface. The instrument was calibrated in both full-scan ToF-MS and MS/MS modes. The mass spectrometer was operated with a resolution power (RP) of approx. 30
000 FWHM (full width at half maxima). This QToF uses an automated external calibration system for mass accuracy with an injector system (CDS, calibrant Delivery System Status) and an internal auto calibration by means of an interactive recalibration tool based on the common background ions found.
Post-acquisition data processing.
Post-acquisition data processing was carried out for the identification of target compounds and the structural characterization of non-target and unknown polar compounds. Data obtained with LC-ESI-QToF-MS were processed with the PeakView software (Version 2.2, AB Sciex) and MasterView software (Version 1.1, AB Sciex), which incorporate tools to display, filter, and process data-dependent MSn acquisition (IDA), along with XIC Manager and MS Library tools. XIC Manager and the MS library were used for rapid screening and identification of target compounds and for other compounds not included a priori in the analytical method (considered as non-target compounds in this study). The library of accurate mass information and MS/MS spectra from AB Sciex was utilized, which includes approx. 3000 compounds (mainly pesticides and pharmaceuticals). In addition to this, a new library database containing both ToF MS and MS/MS spectra of the 65 target compounds selected in this study at CE (−)35 ± 15 eV was made.
Calculations
A case–control concept was applied, with water samples collected from sites A (downstream of the WWTP outlet) and B (∼10 km further downstream) were considered as control and case samples, respectively. For each river Attx (unitless) of a substance along the stretch was calculated by first dividing the peak area at case site B by that at control site A, then normalizing this ratio to the peak area ratio of acesulfame at both sites. The artificial sweetener acesulfame – a persistent compound frequently detected in aquatic systems31,32 as well as in the studied rivers at high concentrations (μg L−1 level)7 – was used as reference compound to account for dilution along the stretch. Positive values of Attx indicate a decreasing trend from site A to B, while negative values indicate an increasing trend. Attx for a substance x along the studied stretch is thus given by: | 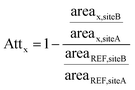 | (1) |
where areax,siteA and areax,siteB are the peak areas of substance x at site A and B, respectively and areaREF,siteA and areaREF,siteB are the peak areas of the reference compound acesulfame at sites A and B. Attx is set to −∞ in cases where a substance was not detected at site A.
Case–control screening strategy
A three-step screening strategy was established to identify TPs increasing along the river stretches: (i) screening for PCs; (ii) prediction of TPs; and (iii) screening for TPs. This approach is detailed below (more parameter settings can be found in ESI†) and schematically illustrated in a flowchart combining the screening results as Fig. 1. In both steps I and III, blank subtraction was performed by first comparing the results of a river sample and a reagent blank (i.e. extracted blank) against each other, then subtracting all the compounds detected in both samples at the same peak areas (within a difference range of 20%).
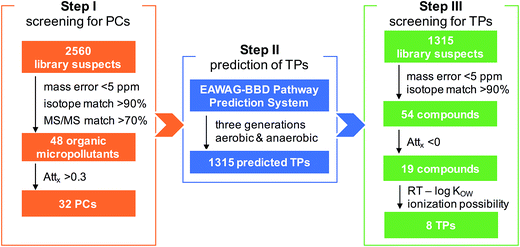 |
| Fig. 1 Overview and procedural performance of the case–control screening strategy for the identification of transformation products formed along the four wastewater-impacted rivers. The 1315 TPs in the suspect library in step III were obtained from TP prediction in step II. Numbers are based on a sum of all four studied rivers. | |
Step I – screening for parent compounds.
Suspect screening was performed using a reference library containing 2560 common aquatic organic micropollutants that was assembled from a commercial database from AB Sciex and represent structurally diverse chemical classes. Confirmation of target analytes was based on the retention time, the accurate mass measurement of the molecular ion (mass error), the isotopic pattern and by automatic MS/MS library search. A compound was discarded if the monoisotopic peak intensity in the extracted chromatogram was <1000 counts or if the signal to noise ratio was <200. The cut-off values were chosen due to two reasons: (i) characteristic fragments for structural elucidation can only be achieved at high intensity of the precursor ion, and (ii) TPs are more likely to be detected at a response sufficiently high for identification. The exact masses of all compounds were extracted with a mass error window of 5 ppm. The isotope pattern of the precursor ion was automatically compared with the theoretical cluster predicted from the molecular formula and a compound was retained in case of a match >90%, calculated by a combination of the exact masses and intensity ratios. Thereafter the MS/MS data of all retained compounds were compared to those documented in the reference library and the compounds for which >70% of the fragmentation (both the number of fragments and their intensity ratios) can be explained by the MS/MS library were defined as tentatively identified organic micropollutants.
Step II – prediction of transformation products.
The tentatively identified organic micropollutants which had a concentration decrease >30% (i.e. Attx > 0.30) between sites A and B were considered as PCs. Potential TPs from each PC due to microbial degradation were predicted using the EAWAG-BBD pathway prediction system. The transformation rules of this system have been derived from an extensive collection of known biodegradation pathways for over 1400 chemicals (database last updated on June 30, 2014).33 For each PC, we predicted three generations of TPs, allowing for both aerobic and anaerobic transformations.
Step III – screening for transformation products.
A suspect list containing all predicted TPs was established, using their formulas, molecular structures and exact masses (see Table S2 in ESI† for a complete list). The isotope patterns of the compounds were automatically calculated by MasterView based on their molecular formulas. A compound was discarded if the monoisotopic peak intensity in the extracted chromatogram was <500 counts or if the signal to noise ratio was <50. The exact masses were then extracted from the sample chromatograms and detected peaks were filtered using the same criteria for mass error and isotope pattern as described in step I for PCs. For compounds fulfilling these criteria, the Attx was calculated and only those with a negative value (Attx < 0 or Attx of −∞) were retained and further checked for ionization plausibility under the detected ESI mode. For instance, compounds containing acidic functional groups were considered detectable in negative ionization mode, while compounds containing amino functional groups were expected to be detectable in positive mode. Furthermore, the octanol–water partition coefficient (log
KOW) is known to represent a reasonable indicator of the retention of organic substances on a C18 column.25 We therefore compared log
KOW (predicted on the website http://chemicalize.org) and measured RT of the proposed TPs to those of their corresponding PCs. The TPs for which the RT relative to the RT of the PCs was consistent with the difference in log
KOW between PC and TP (e.g., a TP with a lower log
KOW than that of its PC has an earlier RT and vice versa) were considered as tentatively identified TPs. To further confirm the tentatively identified TPs in the samples, their molecular structures proposed by the EAWAG-BBD pathway prediction system were searched within the ChemSpider database. In case of a match, we further compared their recorded MS/MS information with the theoretical spectra of the associated compounds using the in silico fragmentation program MetFrag.34
For the tentatively identified TPs that were retained from the screening procedure, commercial reference standards were purchased if available (only benzamide at the time this study was carried out). The RT and mass spectra of the reference standard was recorded by injection of a standard solution (concentration: 10 μg L−1 in methanol) on the UHPLC-QToF-MS/MS.
Results and discussion
Performance of the case–control screening approach
Overall, the case–control screening approach was efficient and accurate in reducing the complexity of processing HRMS data to identify polar organic micropollutants with a desired mass trend along the river stretches (Fig. 1). In total, 48 organic micropollutants were tentatively identified in the four studied rivers receiving treated wastewater. To evaluate the detection efficiency of organic micropollutants and the reliability of the screening procedure, the screening results from the present study were cross checked with the results obtained from a target analysis on the same samples.7 Overall, the Attx determined for 17 different micropollutants common in the two studies were well correlated (R2 = 0.89). Of the 17 compounds detected using target analysis by Li et al. (2016), 14 were also identified during step I in this study, of which three compounds (acesulfame, carbamazepine, and fluconazole) were persistent while the others had a decreasing trend, albeit to different extents (Fig. 2). The remaining three compounds (chlorthalidone, glimepiride, and ketoprofen) that were not detected by the case–control screening procedure still agree with the results from the target analysis as these compounds were only detected occasionally in the rivers and in general had relatively low concentrations compared to the other target compounds.
 |
| Fig. 2 Comparison of the Attx values in the four rivers for all compounds detected in both the present study and Li et al. (2016). Values were derived from quantitative results from Li et al. (2016), calculated from absolute concentrations, and semi-quantitative results from this study, calculated from peak areas. The 1:1-line (dashed line) indicates a 100% match between the results from both measurements. The solid line illustrates the linear regression of all data. | |
For both the PC and TP screening steps, the two criteria peak intensity and signal to noise ratio led to a reduction by >90% of the features, and the criteria exact mass, isotope pattern and MS/MS information further narrowed down the datasets. From the PC screening step, 48 organic micropollutants were tentatively identified in the studied rivers with a match in the reference library (Table 1): two flame retardants (hydroxylphenyl diphenyl phosphate and triphenyl phosphate), two fungicides (fludioxonil and pyroquilon), one herbicide (dinoseb), one insecticide (DEET), one antiseptic (8-hydroxyquinoline) and 41 PPCPs. Out of these 48 organic micropollutants, 32 had a >30% decrease between sites A and B and were therefore treated as PCs in step II in which TPs were predicted. As shown in Table 1, the Attx values for the organic micropollutants detected in the four rivers varied notably, i.e., from −1.6 for metamfepramone (indicating a higher concentration at the downstream site) and gabapentin in river FY to 1.0 for losartan in GR (indicating an elimination). This is also qualitatively in good agreement with the results obtained from the target analysis on the same samples,7 showing that Attx values of the target analytes varied between the rivers. For instance, the attenuation of one pharmaceutical hydrochlorothiazide was similar in the three Swedish rivers (i.e., FY, RO, and VI) but lower than in GR by a factor of up to 2. We hypothesized the different attenuation rates between the rivers to mainly be attributed to complex biogeochemical processes in combination with the hydraulic conditions of the rivers. The confirmed persistence of carbamazepine along the investigated river stretches (Attx of 0; Table 1) further supports that our screening method and the Attx calculation strategy (by normalizing the peak areas to those of a reference compound) are valid.
Table 1 The organic micropollutants tentatively identified (sorted alphabetically) in the studied rivers using the case–control screening approach. Shown are their molecular formula, ESI mode, theoretical exact mass, mass error, retention time (RT) and shift, log
KOW and the screening scores for isotope match and MS/MS match during step I
Organic micropollutant |
Molecular formula |
ESI mode |
Theoretical mass (Da) (mass error in ppm) |
RT (min) (shift in min)a |
log KOWb |
Isotope match (%) |
MS/MS match (%) |
Attx |
Detected in river(s) |
RT shifts only apply to the detected compounds on the list of 65 target analytes for which RT and MS/MS information were available (Table S1 and Fig. S1–S9).
log KOW was predicted on the website http://chemicalize.com.
|
8-Chlorotheophylline |
C7H7ClN4O2 |
ESI− |
214.0258 (4.4) |
2.5 (—) |
0.1 |
97 |
86 |
−0.34 |
GR |
8-Hydroxyquinoline |
C9H7NO |
ESI+ |
145.0528 (0.5) |
3.0 (—) |
1.8 |
96 |
93 |
0.35 |
RO |
Acetaminophen |
C8H9NO2 |
ESI+ |
151.0633 (−1.0) |
2.3 (0.3) |
0.9 |
99 |
78 |
0.38 |
FY |
Adenosine |
C10H13N5O4 |
ESI+ |
267.0968 (1.4) |
1.3 (—) |
−2.1 |
99 |
88 |
−0.12 |
FY |
−0.13 |
RO |
−0.54 |
VI |
Amantadine |
C10H17N |
ESI+ |
151.1361 (0.5) |
4.0 (—) |
1.5 |
98 |
97 |
0.20 |
GR |
Bezafibrate |
C19H20ClNO4 |
ESI− |
361.1081 (0.2) |
5.3 (—) |
4.0 |
99 |
98 |
0.82 |
GR |
0.74 |
FY |
Bicalutamide |
C18H14F4N2O4S |
ESI− |
430.0610 (0.3) |
6.0 (—) |
2.7 |
99 |
91 |
0.36 |
GR |
0.25 |
FY |
0.13 |
RO |
0.17 |
VI |
Bisoprolol |
C18H31NO4 |
ESI+ |
325.2253 (0.8) |
5.4 (—) |
2.2 |
98 |
86 |
0.88 |
GR |
Candesartan |
C24H20N6O3 |
ESI+ |
440.1597 (0.9) |
7.5 (—) |
5.2 |
99 |
96 |
0.14 |
GR |
Carbamazepine |
C15H12N2O |
ESI+ |
236.0950 (0.6) |
6.8 (0.2) |
2.8 |
99 |
92 |
0.03 |
GR |
0.04 |
FY |
Celiprolol |
C20H33N3O4 |
ESI+ |
379.2471 (0.8) |
4.9 (—) |
1.3 |
96 |
89 |
0.27 |
GR |
Cetirizine |
C21H25ClN2O3 |
ESI+ |
388.1554 (1.4) |
7.2 (—) |
0.9 |
98 |
85 |
0.34 |
GR |
0.23 |
FY |
Cycloheximide |
C15H23NO4 |
ESI+ |
281.1627 (0.6) |
3.1 (—) |
0.9 |
93 |
81 |
0.55 |
FY |
DEET |
C12H17NO |
ESI+ |
191.1310 (3.2) |
7.4 (0.3) |
2.5 |
99 |
94 |
−1.20 |
GR |
−0.54 |
FY |
−0.45 |
VI |
Demoxepam |
C15H11N2O2Cl |
ESI+ |
286.0509 (1.2) |
7.2 (—) |
3.6 |
94 |
88 |
0.18 |
FY |
Diclofenac |
C14H11Cl2NO2 |
ESI+ |
295.0167 (3.4) |
8.8 (0.3) |
4.3 |
94 |
93 |
0.76 |
GR |
Dinoseb |
C10H12N2O5 |
ESI+ |
240.0746 (1.7) |
5.5 (—) |
3.2 |
90 |
90 |
−0.26 |
GR |
Fexofenadine |
C32H39NO4 |
ESI+ |
501.2879 (0.1) |
6.7 (—) |
2.9 |
99 |
95 |
0.45 |
GR |
0.36 |
FY |
Fludioxonil |
C12H6F2N2O2 |
ESI+ |
248.0397 (0.9) |
6.3 (—) |
3.6 |
93 |
89 |
0.23 |
FY |
Furosemide |
C12H11ClN2O5S |
ESI− |
330.0077 (2.2) |
3.9 (0.1) |
3.6 |
96 |
79 |
0.42 |
FY |
Gabapentin |
C9H17NO2 |
ESI+ |
171.1259 (−2.0) |
2.9 (0.2) |
−1.3 |
95 |
84 |
0.45 |
GR |
1.06 |
FY |
Gemfibrozil |
C15H22O3 |
ESI− |
250.1569 (3.0) |
6.6 (—) |
4.4 |
94 |
80 |
0.37 |
VI |
Heptaminol |
C8H19NO |
ESI+ |
145.1467 (0.1) |
1.8 (—) |
0.8 |
99 |
91 |
0.65 |
FY |
Hydrochlorothiazide |
C7H8ClN3O4S2 |
ESI− |
296.9645 (−1.2) |
2.2 (0.0) |
−0.6 |
94 |
86 |
0.43 |
GR |
0.43 |
FY |
Hydroxyphenyl diphenyl phosphate |
C18H15O5P |
ESI+ |
342.0657 (2.1) |
8.5 (—) |
4.8 |
98 |
89 |
0.64 |
RO |
Irbesartan |
C25H28N6O |
ESI+ |
428.2325 (1.1) |
7.7 (—) |
5.5 |
99 |
88 |
0.26 |
GR |
Lamotrigine |
C9H7Cl2N5 |
ESI+ |
255.0078 (0.6) |
4.3 (—) |
1.9 |
99 |
80 |
0.46 |
FY |
Losartan |
C22H23ClN6O |
ESI+ |
422.1622 (4.3) |
7.4 (—) |
5.1 |
95 |
91 |
1.00 |
GR |
Metamfepramone |
C11H15NO |
ESI+ |
177.1154 (1.0) |
6.5 (—) |
2.0 |
97 |
79 |
−1.64 |
FY |
−0.24 |
RO |
Metoprolol |
C15H25NO3 |
ESI+ |
267.1834 (−0.3) |
4.4 (—) |
1.8 |
97 |
90 |
0.65 |
GR |
0.37 |
FY |
0.38 |
VI |
Norethisterone |
C20H26O2 |
ESI+ |
298.1933 (−4.6) |
10.4 (—) |
3.2 |
93 |
86 |
−0.90 |
FY |
Phenazone |
C11H12N2O |
ESI+ |
188.0950 (−4.2) |
4.4 (—) |
1.2 |
91 |
83 |
0.50 |
GR |
Phenprobamate |
C10H13NO2 |
ESI+ |
179.0946 (3.2) |
2.2 (—) |
0.2 |
90 |
85 |
0.56 |
FY |
Pyroquilon |
C11H11NO |
ESI+ |
173.0841 (0.9) |
2.0 (—) |
1.4 |
90 |
76 |
0.86 |
GR |
Sotalol |
C12H20N2O3S |
ESI+ |
272.1195 (2.5) |
1.9 (—) |
−0.4 |
99 |
89 |
0.34 |
GR |
0.43 |
FY |
0.15 |
VI |
Sulfamethoxazole |
C10H11N3O3S |
ESI+ |
253.0521 (0.1) |
4.0 (0.3) |
0.8 |
96 |
90 |
0.36 |
GR |
Sulfapyridine |
C11H11N3O2S |
ESI+ |
249.0572 (2.9) |
2.8 (—) |
1.0 |
95 |
88 |
0.33 |
FY |
Sulfasalazine |
C18H14N4O5S |
ESI+ |
398.0685 (−0.4) |
7.0 (—) |
3.9 |
95 |
84 |
0.28 |
FY |
Sulpiride |
C15H23N3O4S |
ESI+ |
341.1409 (2.0) |
2.1 (—) |
0.2 |
90 |
86 |
0.46 |
GR |
Telmisartan |
C33H30N4O2 |
ESI+ |
514.2369 (1.2) |
7.5 (—) |
6.0 |
94 |
91 |
0.48 |
GR |
Torasemide |
C16H25NO2 |
ESI+ |
348.1256 (0.2) |
4.0 (—) |
1.9 |
97 |
91 |
0.39 |
GR |
Tramadol |
C10H11N3O3S |
ESI+ |
263.1885 (0.1) |
5.0 (—) |
2.4 |
99 |
90 |
0.50 |
GR |
0.46 |
FY |
0.15 |
RO |
Trenbolone |
C18H22O2 |
ESI+ |
270.1620 (−2.0) |
10.0 (—) |
2.2 |
99 |
79 |
0.33 |
GR |
−0.14 |
RO |
−0.35 |
VI |
Trihexyphenidyl |
C20H31NO |
ESI+ |
301.2406 (−3.2) |
10.5 (—) |
4.2 |
97 |
81 |
−0.37 |
RO |
−0.26 |
VI |
Triphenyl phosphate |
C18H15O4P |
ESI+ |
326.0708 (0.7) |
9.1 (—) |
5.1 |
99 |
95 |
−0.53 |
FY |
Valsartan |
C24H29N5O3 |
ESI+ |
435.2270 (3.4) |
4.9 (—) |
5.3 |
99 |
93 |
0.84 |
GR |
Venlafaxine |
C17H27NO2 |
ESI+ |
277.2042 (1.9) |
5.4 (0.2) |
2.7 |
98 |
91 |
0.54 |
GR |
0.13 |
FY |
Xipamide |
C15H15ClN2O4S |
ESI− |
354.0441 (−2.7) |
4.2 (—) |
3.0 |
99 |
92 |
0.72 |
GR |
Screening for transformation products
In total, for the 32 PCs that were attenuated with Attx >0.30 between the control and case sites, 1315 potential TPs (three generations for each PC) were predicted by the EAWAG-BBD pathway prediction system, yielding another suspect list for TP screening in step III. In the end, this procedure led to eight tentatively identified TPs (Table 2), all in rivers GR and FY – the two rivers that had the highest proportion of wastewater among the four investigated rivers (i.e., up to 80% for GR and 20% for FY as compared to 1% for RO and 7% for VI). The abundance of these TPs at sampling site B compared to site A (close to WWTP outlet) was estimated using the instrument response ratio normalized to the reference compound in each river. The abundance increased by a factor of 1.6 for MET-265 in FY (Attx of −0.6) to 11 for BEZ-178 in GR (Attx of −10.1). Furthermore, one of the eight tentatively identified TPs, benzamide (BEZ-121), was confirmed by its corresponding reference standard, while no reference standard was available for the remaining compounds. For these seven tentative candidates, molecular structures were therefore proposed directly based on the suggestion of the EAWAG-BBD pathway prediction system and elucidated based on the MS/MS data.
Table 2 Summary of the (tentatively) identified transformation products (TPs), named after the abbreviations of their parent compounds (PCs) and their nominal mass
TP (ChemSpider ID) |
Elemental composition |
Theoretical mass (Da) (mass error in ppm) |
RT (min) |
log KOWa |
Isotope match (%) |
Attx |
Detected in river |
Proposed molecular structureb |
Identification confidence levelc |
log KOW was predicted on the website http://chemicalize.org.
Molecular structures were proposed by the EAWAG-BBD pathway prediction system.
Identification confidence level was introduced by Schymanski et al. (2014)35 and explained in the text.
|
BEZ-178 (n.a.) |
[M − H]− |
178.0994 |
5.1 |
2.1 |
90 |
−10.1 |
GR |
|
3 |
PC: bezafibrate |
[C11H14O2–H]− |
(1.0) |
BEZ-121 (2241) confirmed TP: benzamide |
[M + H]+ |
121.0528 |
3.1 |
0.8 |
91 |
−1.6 |
GR |
|
1 |
PC: bezafibrate |
[C7H7NO + H]+ |
(2.6) |
FEX-222 (15549364) |
[M − H]− |
222.1256 |
4.8 |
2.5 |
92 |
−0.8 |
FY |
|
2 |
PC: fexofenadine |
[C13H18O3–H]− |
(0.5) |
HEP-144 (71101) |
[M − H]− |
144.1150 |
5.2 |
1.0 |
90 |
−1.6 |
FY |
|
3 |
PC: heptaminol |
[C8H16O2–H]− |
(3.3) |
MET-265 (32675184) |
[M + H]+ |
265.1678 |
7.6 |
2.1 |
90 |
−0.7 |
GR |
|
3 |
PC: metoprolol |
[C15H23NO3+H]+ |
(−4.8) |
−0.6 |
FY |
SUL-167 (n.a.) |
[M − H]− |
167.0582 |
5.6 |
0.4 |
93 |
−0.8 |
GR |
|
3 |
PC: sulpiride |
[C8H9NO3–H]− |
(0.6) |
TOR-378 (n.a.) |
[M − H]− |
378.0998 |
2.3 |
0.7 |
90 |
−1.4 |
GR |
|
3 |
PC: torasemide |
[C16H18N4O5S–H]− |
(−0.8) |
VAL-157 (460722) |
[M + H]+ |
157.1467 |
7.7 |
2.0 |
94 |
−1.0 |
GR |
|
3 |
PC: valsartan |
[C9H19NO + H]+ |
(1.9) |
Here we use one TP (FEX-222) of fexofenadine (an antihistamine) as an illustrative example to describe the identification procedure (Fig. 3). FEX-222 was proposed as a third-generation microbial degradation product of fexofenadine by the EAWAG-BBD pathway prediction system. The extraction of the m/z (C13H17O3, [M − H]− = 221.1184) of this proposed TP revealed a distinct peak at a RT of 4.8 min (Fig. 3A) in both the control and case site samples. The isotope pattern with a 13C signal of 20% abundance compared to the monoisotopic peak fitted well with the molecular structure (Fig. 3B). The Attx value was <0 and thus the peak was retained as a TP candidate. The detection of the compound under negative ionization mode agreed with the presence of an acid moiety on the molecule. Furthermore, the predicted log
KOW of the proposed TP (2.5) is lower than the log
KOW of its PC fexofenadine (2.9), supporting the shorter RT of the TP relative to fexofenadine. Based on the fulfillment of all criteria, the peak was processed further and the MS/MS spectra for the precursor ion were extracted. As the specific candidate TP is contained in the ChemSpider database, the fragmentation elucidation with the assistance of MetFrag was possible, and a plausible structure was assigned to all the four dominant fragments (Fig. 3C).
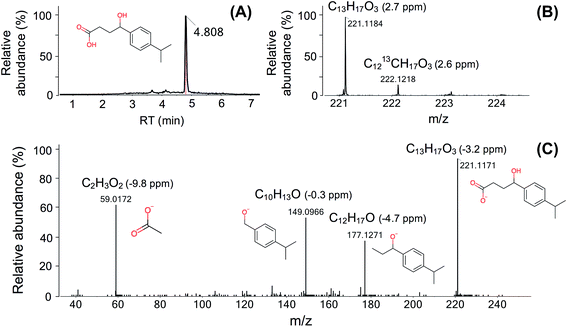 |
| Fig. 3 Structure of the TP FEX-222 with the corresponding extracted ion chromatogram (A), HR-MS spectra of the molecular ion with its isotope pattern (B) and the HR-MS/MS spectra of [M − H]− = 221.1184 (C). Numbers shown in parenthesis indicate the absolute error of the measured exact mass from the theoretical value. The fragment structures are those proposed by MetFrag. All spectra are from the sample at sampling site B of river FY where FEX-222 was detected. | |
Evaluation of the screening strategy
Non-target and suspect screening studies enabled by HRMS techniques have opened new opportunities in environmental science and environmental monitoring. One important application is the identification of known and/or unknown compounds such as TPs in environmental and biological samples. To reduce the common communication difficulties regarding identification confidence and reliability, which are inevitable in such HRMS-based studies due to varying identification pathways and substances, a comprehensive identification confidence level system was proposed by Schymanski et al. (2014),35 introducing five confidence levels of the identification of a specific (unknown) compound. The so-called identification confidence reflects the occurrence probability of false positives generated from the developed/applied screening approach. A higher identification confidence indicates that it is highly unlikely that the identified compounds are false positives, and vice versa. Reference standards are always essential for an identified compound to be fully excluded from a false positive, as also described in the requirement for level 1. In this study, the identification of benzamide reaches level 1 – confirmed structure – using the reference standard (see Fig. S10 in ESI† for structural elucidation); while the identification of FEX-222 falls into level 2, which indicates that the probable structure can be supported by different evidences such as library spectra data and/or diagnostic MS/MS fragments (as shown above in Fig. 3). However, the identification of the other six TPs (see Table 2) can at this stage only be categorized into level 3, which describes tentative candidates but with insufficient information on the exact structures. The lack of detectable fragments of these six TPs is highly likely attributed to the low abundance in the rivers and a possible sensitivity limitation of the instrument. To increase the identification confidence level for these TPs, a higher injection volume in combination with additional optimization of the MS tuning parameters (e.g., increasing the collision energy) and/or reference standards are necessary.
The false negatives linked to this screening approach are mostly the TPs overlooked during individual screening steps. In this study, the three main causes for false negatives are: (i) TPs absent on the suspect list; (ii) TPs being outside the analytical method and screening approach domain; and (iii) unidentified PCs with Attx >0.30. A major reason for case (i) is that the EAWAG-BBD pathway prediction system predicts TPs formed exclusively from biotic transformation processes. False negatives may therefore be TPs originating from the abiotic transformation processes such as photolysis and hydrolysis. In this study, we focused on biotransformation products mainly due to a lack of equally good prediction tools for abiotic transformation processes. Nevertheless, even though we consciously limited our scope to biotransformation products, the presented approach can be potentially applied to screen for TPs from any other transformation pathway given such a prediction tool. The second cause for false negatives (ii) directly depends on the sensitivity of the instrument and the concentrations of PCs as peaks with too low intensity are immediately discarded during step I (PC screening). Outside the method domain would also be the case for all compounds that cannot be ionized in ESI for instance. Additionally, there is one type of false negatives inherent to our method, namely compounds that are discriminated during the sample extraction step. This limitation can potentially be overcome if other extraction methods (e.g., complementary SPE cartridges, different pH conditions or different extraction techniques) are used to retain PCs and TPs with considerably different physical–chemical properties. The third cause for false negatives (iii); i.e., that attenuating PCs are not identified, can be due to varying reasons such as exclusion of the PC in the database or poor PC detection during step I which again directly results from analytical problems, e.g., sensitivity and selectively. It is also possible that a PC does not disappear, but is still transformed. This would be possible in the case of a PC that is transformed, but to some extent is also back-transformed from some co-emitted TP(s). An exclusion of potential PCs could also be due to the arbitrary criterion of Attx >0.30. In total, there are six detected PCs having the Attx value >0 but ≤0.30 (Table 1). A main reason for choosing this threshold value (apart from considering the uncertainty) was to increase the chance that the increased TP concentration can be captured. One additional limitation of the screening strategy is related to the intermediate TPs that are formed before the downstream sampling site and further degraded or back-transformed into their PCs at sampling. This limitation can potentially be addressed by shortening the distance between the control and case sampling sites or by adding more sampling sites in future studies. Nevertheless, even though we can assume that our screening concept produces a substantial number of false negatives, the inevitable false negative issue is inherent to any type of suspect and non-target screening analysis using HRMS. Effort has been dedicated to tackle the challenge of balancing false positive and false negative rates in a suspect screening study using multivariate statistics,36 but such approaches cannot be easily extrapolated to the non-target analysis, mostly due to the unlimited number of unknowns. Therefore, a rational prioritization of critical unknowns would be essential for the process-oriented studies combining non-target screening approaches.
Given the countless number of unknown potential organic micropollutants in the environment, and a major focus on monitoring the known pollutants so far,37 society urgently needs a strategic screening concept to prioritize the critical pollutants with respect to their environmental relevance and significance. The process-oriented screening approach proposed in this study based on the case–control concept can have a broad environmental application range. By treating samples collected from two sampling locations as case and control samples, the high-throughput screening method provides an overall trend of pollutants from varying classes, which is valuable information when deciding on compounds for further investigations. More tailored studies with better resolution of sampling sites and/or sampling time can then be designed depending on the specific target compounds. The scope of this study has been limited to the TPs formed along a river stretch, but the approach may also be used to investigate the trend of PCs alone. For instance, in our study nine organic micropollutants on the suspect list were detected in at least one river with an increased concentration along the stretch (Attx < 0, see Table 1), including one flame retardant (triphenyl phosphate), one insecticide (DEET), one herbicide (dinoseb) and six PPCPs (8-chlorotheophylline, adenosine, metamfepramone, norethisterone, trenbolone, and trihexyphenidyl). These compounds were identified in step I but excluded from further screening as we specifically looked for those PCs with an evident attenuation. As we consciously limited the sampling location to a river stretch where there was no known major emission source of organic micropollutants other than the WWTP upstream the control site, an increased concentration of these pollutants after normalizing to a reference tracer would imply a hidden emission source, or groundwater leachate, or back-transformation from their TPs. This can be illustrated by the insecticide DEET detected in this study with increased concentrations at downstream of three out of four studied rivers. The occurrence of DEET in the aquatic environment has been demonstrated to involve multiple routes.38 Although a primary source is WWTP effluent discharge, additional nonpoint diffuse sources from contaminated soil and/or contamination routes via direct transfer to water bodies used for recreational activities such as swimming should also be taken into consideration.
Conclusions
Generally, the lack of knowledge of dominant TPs formed in surface water hampers comprehensive research on environmental distribution, persistence, and (eco)toxicological effects of both PCs and TPs. This was addressed in the present study, providing a rapid and reliable process-oriented screening approach to prioritize and identify TPs of high environmental relevance with respect to a formation along rivers. The case–control concept retaining only PCs and TPs with their attenuation (for PCs) and formation (for TPs) rates meeting defined criteria (i.e., a spatial trend in the field) was essential in reducing data processing time. The accuracy of the overall approach was shown by the confirmation of one identified TP for which a reference standard was available. TPs were mostly identified in the two rivers (GR and FY) receiving the highest proportions of wastewater. The formation and detection of TPs highlights the need of TPs to be included when investigating the environmental fate of organic micropollutants. Unlike many TPs that have been previously identified from well-defined lab incubation experiments, the eight TPs identified in this study were specifically generated in natural waters. One such example is the tentatively identified TP BEZ-178, which had an Attx value of approx. −10, indicating that its concentration was 10 times higher at the downstream site compared to the site close to the WWTP outlet. Detection of certain TPs at higher concentrations even 10 km downstream of the WWTP outlet points to a need for (eco)toxicological risk assessments. In addition, improved transformation prediction tools (especially for abiotic transformation processes) are necessary to extend the suspect libraries and to reduce the number of overlooked dominant TPs.
Acknowledgements
The authors gratefully acknowledge Chunjie Luo for his assistance during sampling and Jochen Müller for his insightful discussions. This study was financially supported by a FORMAS strategic grant and by the BEAM research program at Stockholm University. Anna Sobek was funded by the FORMAS grant 2012-1211.
References
- R. P. Schwarzenbach, B. I. Escher, K. Fenner, T. B. Hofstetter, C. A. Johnson, U. von Gunten and B. Wehrli, Science, 2006, 313, 1072–1077 CrossRef CAS PubMed.
- P. H. Howard and D. C. G. Muir, Environ. Sci. Technol., 2010, 44, 2277–2285 CrossRef CAS PubMed.
- P. H. Howard and D. C. G. Muir, Environ. Sci. Technol., 2011, 45, 6938–6946 CrossRef CAS PubMed.
- B. Petrie, R. Barden and B. Kasprzyk-Hordern, Water Res., 2015, 72, 3–27 CrossRef CAS PubMed.
- A. B. A. Boxall, M. A. Rudd, B. W. Brooks, D. J. Caldwell, K. Choi, S. Hickmann, E. Innes, K. Ostapyk, J. P. Staveley, T. Verslycke, G. T. Ankley, K. F. Beazley, S. E. Belanger, J. P. Berninger, P. Carriquiriborde, A. Coors, P. C. DeLeo, S. D. Dyer, J. F. Ericson, F. Gagné, J. P. Giesy, T. Gouin, L. Hallstrom, M. V. Karlsson, D. G. J. Larsson, J. M. Lazorchak, F. Mastrocco, A. McLaughlin, M. E. McMaster, R. D. Meyerhoff, R. Moore, J. L. Parrott, J. R. Snape, R. Murray-Smith, M. R. Servos, P. K. Sibley, J. O. Straub, N. D. Szabo, E. Topp, G. R. Tetreault, V. L. Trudeau and G. Van Der Kraak, Environ. Health Perspect., 2012, 120, 1221–1229 CrossRef PubMed.
- Y. Luo, W. Guo, H. H. Ngo, L. D. Nghiem, F. I. Hai, J. Zhang, S. Liang and X. C. Wang, Sci. Total Environ., 2014, 473–474, 619–641 CrossRef CAS PubMed.
- Z. Li, A. Sobek and M. Radke, Environ. Sci. Technol., 2016, 50, 5614–5621 CrossRef CAS PubMed.
- U. Hass, U. Duennbier and G. Massmann, Water Res., 2012, 46, 6013–6022 CrossRef CAS PubMed.
- L. J. Fono, E. P. Kolodziej and D. L. Sedlak, Environ. Sci. Technol., 2006, 40, 7257–7262 CrossRef CAS PubMed.
- Y. Picó and D. Barceló, Anal. Bioanal. Chem., 2015, 407, 6257–6273 CrossRef PubMed.
- X.-H. Wang and A. Y.-C. Lin, Environ. Sci. Technol., 2012, 46, 12417–12426 CrossRef CAS PubMed.
- M. Herrmann, J. Menz, O. Olsson and K. Kümmerer, Water Res., 2015, 85, 11–21 CrossRef CAS PubMed.
- B. I. Escher and K. Fenner, Environ. Sci. Technol., 2011, 45, 3835–3847 CrossRef CAS PubMed.
- S. Kern, H. Singer, J. Hollender, R. P. Schwarzenbach and K. Fenner, Environ. Sci. Technol., 2011, 45, 2833–2841 CrossRef CAS PubMed.
- M. D. Celiz, J. Tso and D. S. Aga, Environ. Toxicol. Chem., 2009, 28, 2473–2484 CrossRef CAS PubMed.
- P. Gago-Ferrero, E. L. Schymanski, A. A. Bletsou, R. Aalizadeh, J. Hollender and N. S. Thomaidis, Environ. Sci. Technol., 2015, 49, 12333–12341 CrossRef CAS PubMed.
- C. Moschet, I. Wittmer, J. Simovic, M. Junghans, A. Piazzoli, H. Singer, C. Stamm, C. Leu and J. Hollender, Environ. Sci. Technol., 2014, 48, 5423–5432 CrossRef CAS PubMed.
- M. Ruff, M. S. Mueller, M. Loos and H. P. Singer, Water Res., 2015, 87, 145–154 CrossRef CAS PubMed.
- B. Zonja, A. Delgado, S. Pérez and D. Barceló, Environ. Sci. Technol., 2015, 49, 3464–3472 CrossRef CAS PubMed.
- E. L. Schymanski, H. P. Singer, J. Slobodnik, I. M. Ipolyi, P. Oswald, M. Krauss, T. Schulze, P. Haglund, T. Letzel, S. Grosse, N. S. Thomaidis, A. Bletsou, C. Zwiener, M. Ibáñez, T. Portolés, R. de Boer, M. J. Reid, M. Onghena, U. Kunkel, W. Schulz, A. Guillon, N. Noyon, G. Leroy, P. Bados, S. Bogialli, D. Stipaničev, P. Rostkowski and J. Hollender, Anal. Bioanal. Chem., 2015, 407, 6237–6255 CrossRef CAS PubMed.
- C. Hug, N. Ulrich, T. Schulze, W. Brack and M. Krauss, Environ. Pollut., 2014, 184, 25–32 CrossRef CAS PubMed.
- Z. Li, M. P. Maier and M. Radke, Anal. Chim. Acta, 2014, 810, 61–70 CrossRef CAS PubMed.
- D. E. Helbling, J. Hollender, H.-P. E. Kohler, H. Singer and K. Fenner, Environ. Sci. Technol., 2010, 44, 6621–6627 CrossRef CAS PubMed.
- S. Kern, R. Baumgartner, D. E. Helbling, J. Hollender, H. Singer, M. J. Loos, R. P. Schwarzenbach and K. Fenner, J. Environ. Monit., 2010, 12, 2100–2111 RSC.
- S. Kern, K. Fenner, H. P. Singer, R. P. Schwarzenbach and J. Hollender, Environ. Sci. Technol., 2009, 43, 7039–7046 CrossRef CAS PubMed.
- A. A. Bletsou, J. Jeon, J. Hollender, E. Archontaki and N. S. Thomaidis, TrAC, Trends Anal. Chem., 2015, 66, 32–44 CrossRef CAS.
- M. J. Gómez, M. M. Gómez-Ramos, O. Malato, M. Mezcua and A. R. Férnandez-Alba, J. Chromatogr. A, 2010, 1217, 7038–7054 CrossRef PubMed.
- M. D. M. Gómez-Ramos, A. Pérez-Parada, J. F. García-Reyes, A. R. Fernández-Alba and A. Agüera, J. Chromatogr. A, 2011, 1218, 8002–8012 CrossRef PubMed.
- S. H. López, M. M. Ulaszewska, M. D. Hernando, M. J. Martínez Bueno, M. J. Gómez and A. R. Férnandez-Alba, Environ. Sci. Pollut. Res., 2014, 21, 12583–12604 CrossRef PubMed.
- A. Rotander, A. Kärrman, L.-M. L. Toms, M. Kay, J. F. Mueller and M. J. Gómez Ramos, Environ. Sci. Technol., 2015, 49, 2434–2442 CrossRef CAS PubMed.
- H. Zou, M. Radke, A. Kierkegaard, M. MacLeod and M. S. McLachlan, Environ. Sci. Technol., 2015, 49, 1646–1653 CrossRef CAS PubMed.
- I. J. Buerge, H.-R. Buser, M. Kahle, M. D. Müller and T. Poiger, Environ. Sci. Technol., 2009, 43, 4381–4385 CrossRef CAS PubMed.
- J. Gao, L. B. M. Ellis and L. P. Wackett, Nucleic Acids Res., 2010, 38, D488–D491 CrossRef CAS PubMed.
- S. Wolf, S. Schmidt, M. Müller-Hannemann and S. Neumann, BMC Bioinf., 2010, 11, 148 CrossRef PubMed.
- E. L. Schymanski, J. Jeon, R. Gulde, K. Fenner, M. Ruff, H. P. Singer and J. Hollender, Environ. Sci. Technol., 2014, 48, 2097–2098 CrossRef CAS PubMed.
- L. Vergeynst, H. Van Langenhove and K. Demeestere, Anal. Chem., 2015, 87, 2170–2177 CrossRef CAS PubMed.
- A. Sobek, S. Bejgarn, C. Rudén and M. Breitholtz, Environ. Sci.: Processes Impacts, 2016, 18, 1042–1049 Search PubMed.
- S. Merel and S. A. Snyder, Environ. Int., 2016, 96, 98–117 CrossRef CAS PubMed.
Footnotes |
† Electronic supplementary information (ESI) available: Parameter settings of the post-acquisition data processing workflow, a complete list of the 65 target analytes used for confirming PCs detected in the studied rivers, comparison of chromatographic and spectral information of the detected PCs with the library, structural elucidation of the confirmed TP benzamide, a complete list of the 1315 TPs predicted from the EAWAG-BBD pathway prediction system. See DOI: 10.1039/c6em00635c |
‡ Present address: Institute for Hygiene and Environment, Marckmannstraße 129b, 20539 Hamburg, Germany. |
|
This journal is © The Royal Society of Chemistry 2017 |
Click here to see how this site uses Cookies. View our privacy policy here.