DOI:
10.1039/C6AN02516A
(Paper)
Analyst, 2017,
142, 808-814
Detection of glycosylation and iron-binding protein modifications using Raman spectroscopy
Received
22nd November 2016
, Accepted 1st February 2017
First published on 1st February 2017
Abstract
In this study we demonstrate the use of Raman spectroscopy to determine protein modifications as a result of glycosylation and iron binding. Most proteins undergo some modifications after translation which can directly affect protein function. Identifying these modifications is particularly important in the production of biotherapeutic agents as they can affect stability, immunogenicity and pharmacokinetics. However, post-translational modifications can often be difficult to detect with regard to the subtle structural changes they induce in proteins. From their Raman spectra apo-and holo-forms of iron-binding proteins, transferrin and ferritin, could be readily distinguished and variations in spectral features as a result of structural changes could also be determined. In particular, differences in solvent exposure of aromatic amino acids residues could be identified between the open and closed forms of the iron-binding proteins. Protein modifications as a result of glycosylation can be even more difficult to identify. Through the application of the chemometric techniques of principal component analysis and partial least squares regression variations in Raman spectral features as a result of glycosylation induced structural modifications could be identified. These were then used to distinguish between glycosylated and non-glycosylated transferrin and to measure the relative concentrations of the glycoprotein within a mixture of the native non-glycosylated protein.
Introduction
It is well established that the large majority of proteins undergo one or more modifications following translation which will ultimately affect both structure and function. Even the most subtle of changes can affect function, including activation and regulation. For example, during glycosylation of protein based biopharmaceuticals it is essential that the correct number and sequence of glycans are attached to the correct amino-acid for stability, pharmacokinetics and appropriate immunogenicity of the drug.1–3 Furthermore, a protein's function may specifically revolve around the binding of ligands, as is the case for iron-transporting and iron storage proteins such as transferrin and ferritin.
Whilst iron is an essential micronutrient and associated with many important biological functions, including respiration and cell division,4–7 it is also extremely toxic to cells in its free form (Fe0) and therefore requires well regulated uptake, transport and storage. Misregulation of iron at any stage can result in severe consequences including the accumulation of iron in the brain and this has been linked to neurogenerative diseases including Halleroerden-Spatz syndrome, Parkinsons and Alzheimer's diseases.8–10 Two iron-binding proteins which have essential roles in iron metabolism are the iron storage protein, ferritin and the iron transporting protein, transferrin. The majority of previous Raman spectroscopy studies of metal binding proteins, such as transferrin, have used resonance Raman to enhance the Raman signal arising from the presence of metal with wavenumbers below 900 cm−1 strongly influenced by the specific metal ligand.11,12 More recently, iron saturation in transferrin13 and differences between ferritin and magnetoferritin have been determined using the non-resonance Raman spectroscopy14 and although both studies focused on Raman peaks arising from the presence of iron they also observed intense protein structure and polysaccharide associated peaks in the spectra.
The role of transferrin as a transporter protein has also made it an important biopharmaceutical fusion protein. Transferrin's biopharmaceutical role is to bind to other therapeutics which have short half-lives and improve the pharmacokinetics of these drugs by extending their activity. However, transferrin also undergoes glycosylation with the addition of mannose and it is also important for this protein to have correct glycosylation. Previous studies have demonstrated how Raman spectroscopy combined with multivariate analysis can be used to detect the glycosylation state of a protein quantifying mixture of the model glycosylated and non-glycosylated proteins.15 Here we extend this work to determine glycosylation status of transferrin, identify iron-binding in transferrin and ferritin and identify structural modifications as a result of the ligand-binding, using Raman spectroscopy.
Materials and methods
Samples
Holoferritin (holoF) and apoferritin (apoF), both from equine spleen, were purchased from Sigma-Aldrich (Dorset, UK) in a 0.15 M NaCl buffer and used without any further purification at a concentration of 10 mg mL−1. D-Mannose was also purchased from Sigma-Aldrich and used without further purification. Variants of transferrin proteins, non-glycosylated apotransferrin (apoTf), glycosylated apotransferrin (apoTfG), non-glycosylated holotransferrin (holoTf) and glycosylated holotransferrin (holoTfG) were kindly supplied by Albumedix® in a standard PBS buffer at a concentration of 1 mg mL−1. Mixtures of apoTf and apoTfG were made, with increasing concentrations of apoTf at 5% intervals, keeping the total protein concentration the same for each sample (1 mg mL−1).
Raman spectroscopy
Raman spectra of all the transferrin variants were collected using a Renishaw 2000 Raman microscope and ferritin spectra were collected using a Renishaw inVia Raman Spectrometer (Renishaw Plc. Old Town, Wotton-under edge, Gloucestershire, UK), both with an excitation wavelength of 785 nm. For the transferrin samples the laser power was ∼27 mW at source and 2–4 mW at the sample, whereas for the ferritin samples the laser power was ∼100 mW at source and ∼50 mW at the sample. The higher laser power was necessary for the ferritin samples in order to overcome fluorescent background interference due to the brown colouring of the samples. At lower laser power only weak Raman peaks were observed but these became stronger as laser power was increased with a reduction in fluorescence most likely due to photo-bleaching during spectral acquisition although no damage to the dried sample was observed through the light microscope after data collection.
All samples were prepared on Tienta Spectra RIM™ slides (Tienta Sciences Inc., Indianapolis, IN, USA) as previously reported.15 All spectra were single accumulation collected for 60 s. For the pure samples six repeat spectra were collected. For the mixed samples three repeat measurements were recorded from each of the 21 samples and collection order of all spectra was randomized.
Data preprocessing
In order to compare the spectra directly cosmic ray removal, baseline correction, smoothing and normalization was carried out on all spectra using MATLAB software version R2012a (The MathWorks, Natwick, MA, USA). Smoothing was applied using a triangular sliding average and baseline correction was applied using an asymmetric least squares algorithm. For the pure transferrin variants and the ferritin samples spectra were normalized to the peak at ∼1445 cm−1 (arising from CH2 groups) since this band has been shown not to fluctuate in signal strength in proteins.16–19 Principal component analysis (PCA) was also applied using MATLAB software version R2012a (The MathWorks, Natwick, MA, USA).
Partial least squares regression
Partial least squares regression (PLSR) is a supervised learning method that relates a set of independent variables X (e.g., the Raman intensities) to a set of dependent variables Y (the apoTf concentration). PLSR projects the X and Y variables into sets of orthogonal latent variables, scores of X and scores of Y, so that the covariance between these two sets of latent variables is maximized.20 The purpose of PLSR is to build a linear model Y = XB + E, where B is a matrix of regression coefficients and E represents the difference (error) between observed and predicted Y values.21 The size of the absolute value of the coefficient for each independent variable (Raman shifts) represents the influence of that variable on the prediction or dependent variable (apoTf concentration). The higher the absolute value of the coefficient is, the higher the influence of the variable. Once the model has been built, it can then be used to predict, or estimate, the values of the dependent variables (apoTf concentrations) of new samples. In addition to these predictions, loadings plots from B regression coefficients can be generated and used to ascertain which are the important variables (Raman shifts) that are used in model construction, and hence related to the concentration of apoTf.
Results and discussion
Detection of iron-binding modifications
Transferrin is a relatively small molecule (molecular mass ca. 79 kDa) with two evolutionary related lobes each consisting of two domains with a central β-sheet backbone that interacts to form the iron binding site.22–24 Ferritin however is composed of 24 subunits, each adopting a 4-helix bundle fold, which together form a spherical molecule (molecular mass ca. 480 kDa) with a hollow central cavity where iron is stored.25–27 Channels link the interior and exterior of the shell to control the release of iron. Fig. 1A and B compare the averaged Raman spectra of holotransferrin (holoTf), apotransferrin (apoTf), holoferritin (holoF) and apoferritin (apoF). Although the majority of spectral variations in Fig. 1 arise from differences in secondary structure and amino-acid residues (see Table 1) two distinctive peaks can be observed in the proteins with iron at ∼425 cm−1 (Fig. 1A) and ∼455 cm−1 (Fig. 1B) not observed in the apo spectra. Peaks in the region of ∼405–550 cm−1 have been reported as arising from both Fe–O and Fe–NO complexes.28–31 Consequently, even though the holoF spectral peak at ∼455 cm−1 is weaker and less noticeable than the holoTf peak at ∼425 cm−1, the apo and holo forms are easily distinguishable in both the ferritin and transferrin Raman spectra. The weaker holoF peak may be due to the fact that the binding site for iron is buried inside the core of the ferritin molecule and may suppress the intensity of the peaks arising from the Fe–O complexes compared to the smaller transferrin protein where the iron binding site is less compact.22 Alternatively, the ferritin samples were commercially bought as opposed to the transferrin samples provided by Albumedix and so these may not be as pure or all protein molecules may not completely bound to the iron.
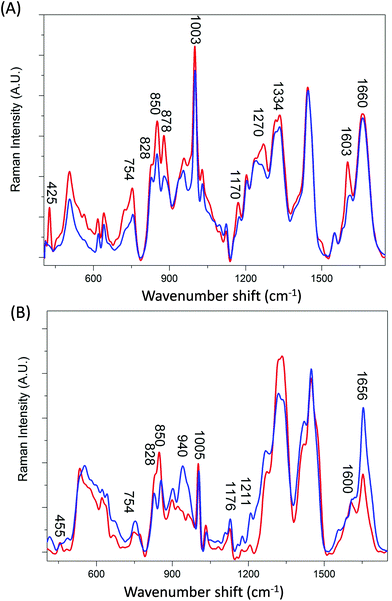 |
| Fig. 1 Averaged Raman spectra of (A) transferrin and (B) ferritin, averaged from 6 preprocessed spectra. Iron bound proteins (holotransferrin and holoferritin) are shown in red. Iron free proteins (apotransferrin and apoferritin) are shown in blue. | |
Table 1 Proposed Raman assignments for ferritin (F) and transferrin (Tf) with (holo) and without (apo) iron as well as glycosylated (TfG) forms of transferrin
Assignment |
holoF |
apoF |
holoTf |
apoTf |
holoTfG |
apoTfG |
Fe–O, Fe–NO complexes29–31 |
455 cm−1 |
|
425 cm−1 |
|
|
|
Tryptophan, indole ring45,46 |
748 cm−1 |
754 cm−1 |
754 cm−1 |
754 cm−1 |
752 cm−1 |
752 cm−1 |
Tyrosine, H-bonding32,33 |
|
828 cm−1 |
|
828 cm−1 |
|
|
Tyrosine H-bonding32,33 |
850 cm−1 |
850 cm−1 |
850 cm−1 |
850 cm−1 |
850 cm−1 |
850 cm−1 |
Tryptophan, H-bonding47 |
|
|
878 cm−1 |
880 cm−1 |
880 cm−1 |
880 cm−1 |
α-Helix, C–C, C–N stretch37,48 |
|
940 cm−1 |
|
|
|
|
Phenylalanine48 |
1005 cm−1 |
1005 cm−1 |
1003 cm−1 |
1003 cm−1 |
1000 cm−1 |
1000 cm−1 |
Tyrosine, CH345 |
1176 cm−1 |
1176 cm−1 |
1170 cm−1 |
1174 cm−1 |
|
|
Tyrosine48 |
1208 cm−1 |
1211 cm−1 |
|
|
|
|
Tyrosine45/α-helix33,48 |
|
|
1270 cm−1 |
1264 cm−1 |
1272 cm−1 |
1270 cm−1 |
α-Helix41 |
|
|
|
|
1314 cm−1 |
1314 cm−1 |
α-Helix41,49 |
|
|
1334 cm−1 |
1334 cm−1 |
1334 cm−1 |
1334 cm−1 |
Phenylalanine, ring modes45,48 |
1600 cm−1 |
|
1603 cm−1 |
|
1600 cm−1 |
|
Disordered, C O stretch33,39 |
|
1665 cm−1 |
1660 cm−1 |
1660 cm−1 |
1660 cm−1 |
1660 cm−1 |
The distinction between Raman spectra of holo and apo protein forms is further confirmed in the PCA scores plots of PC1 and PC2 (Fig. 2A) where good separation between the four samples can be observed. The two different proteins, transferrin and ferritin can be observed to separate along PC1 with a total explained variance (TEV) of 67.1% while the holo and apo proteins are separated along PC2 with TEV 21.1%. As expected the loading plot for PC1 reveals variance is due to differences in secondary structure and amino acid residues (Table 1) However, the loadings plot for PC2 is not dominated by the peaks at ∼425 and 455 cm−1 but also indicates that the main variations between the apo and holo spectra arise from conformational differences.
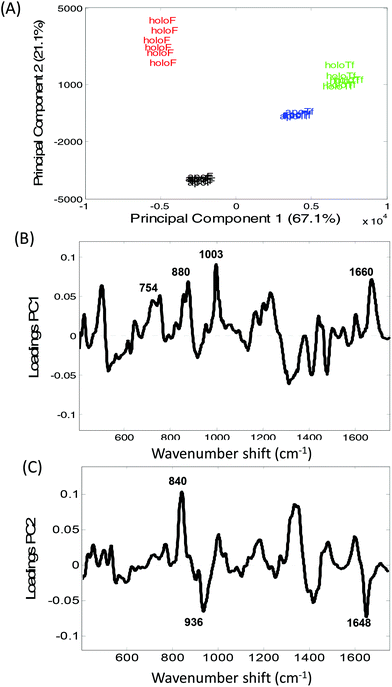 |
| Fig. 2 Principal component analysis of transferrin and ferritin spectra. (A) Scores plot of PC1 versus PC2 of holoferritin (red), holotransferrin (green), apotransferrin (blue) and apoferritin (black), along with the associated (B) PC1 loadings plot and (C) PC2 loadings plots. | |
The largest variance in PC2 loadings plot can be observed for the peak occurring at ∼840 cm−1. When the holo and apo spectra are compared (Fig. 1) a change in the ratio of the peaks at ∼830 and 850 cm−1 can be observed which has been extensively assigned to a change in tyrosine exposure32–34 suggesting that with iron binding tyrosine residues in both proteins become less exposed with a reduction in H-bonding. Structurally, transferrin consists of two lobes, designated the N-lobe and C-lobe linked by a short spacer sequence with each lobe containing two domains interacting to form the metal binding site.22 Iron binding or release has been shown to be associated with a large scale domain movement that results in the closing or opening of the binding cleft of each lobe, mediated by a hinge in the two antiparallel β-strands that run behind the iron-binding site in each lobe.24,35,36 Without iron apoTf is in the more open conformation possibly increasing the distance between β-sheets. Consequently, the majority of intensity variations observed in Fig. 1A may be associated with the change in solvent exposure of the side chain residues and the change in orientation of structural elements between the open and more closed forms of transferrin.
In Fig. 1B a large increase in intensity can be observed for the peaks at ∼940 and 1656 cm−1 for apoF compared to holoF. Peaks in the regions of ∼930–950 cm−1 and ∼1645–1665 cm−1 have been assigned to both α-helical33,37,38 and disordered structure.37,39 The loadings for PC2 (Fig. 2C) also indicate significant variation in these regions. The ferritin molecule is composed of 24 subunits, each adopting a 4-helix bundle fold, which together form a spherical molecule (molecular mass ca. 480 kDa) with a hollow central cavity where iron is stored.5,40 Channels link the interior and exterior of the shell to control the release of iron and the spectra may be monitoring either a decrease in helical structure and/or less ordered structure when iron is bound, possibly due to a closing of the channel. Consequently, for both ferritin and transferrin, it is possible to determine protein modifications from their Raman spectra that occur due to iron-binding.
Detection of glycosylation modifications
Transferrin's role as a transporter protein has resulted in its extensive use as a biopharmaceutical fusion protein but this also requires the protein to be correctly glycosylated, specifically with mannose, to maintain protein stability and immunogenicity.1–3Fig. 3A and B display the averaged Raman spectra of glycosylated and non-glycosylated transferrin, with and without iron. Despite the distinctive Raman spectra of mannose (Fig. 3C) visual inspection of the spectra reveals that although there is some variation in peak intensity no additional mannose peaks are observed. However, when the PCA scores are plotted (PC1 versus PC2, Fig. 4A) clear separation between the four samples can be determined. Again, the loading plots (Fig. 4B and C) reveal that the majority of variance is due to differences in peaks assigned to secondary structure and amino acid residues (Table 1). Consequently, although the variation in PTM could be determined using Raman spectroscopy it is most likely due to subtle changes in secondary structure rather than the presence or absence of mannose.
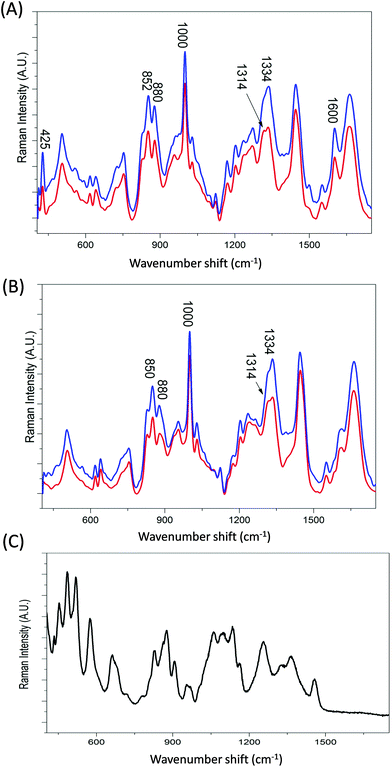 |
| Fig. 3 Averaged Raman spectra of (A) holo- and (B) apo-transferrin with and without glycosylation and (c) D-mannose, averaged from 6 spectra. Glycosylated transferrin (HoloTfG and ApoTfG) are shown in blue and non-glycosylated transferrin (HoloTf and ApoTf) are shown in red. | |
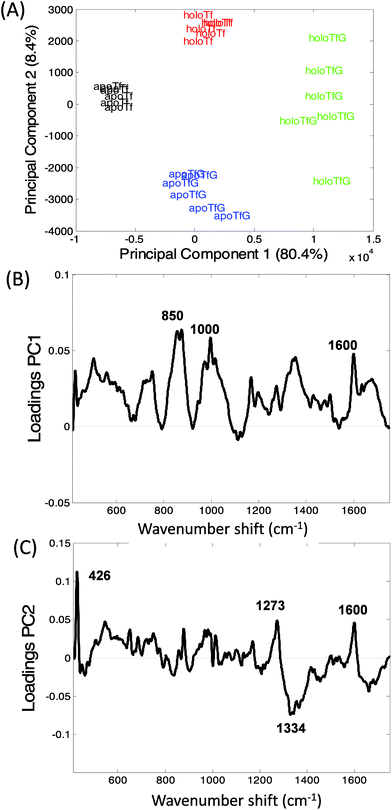 |
| Fig. 4 Principal component analysis of glycosylated and non-glycosylated transferrin. (A) PCA scores plot, PC1 versus PC2, holoTfG (green), holoTf (red) apoTfG (blue), apoTf (black). (B) PC1 loadings plot and (C) PC2 loadings plots. | |
The variation within holoTfG along PC2 may be due to differences in intensity of the iron peak at 425 cm−1 which was observed to vary slightly depending on the location on the slide where the spectra is taken from. A similar affect can be observed for the holo samples in Fig. 2 which are spread further apart than the apo samples. The intensity of the iron peak may be affected by the orientation of the protein when drying out on the slide. This is further supported by the intense peak at 425 cm−1 observed in the loading plot of PC2 (Fig. 3C).
From the loadings plots of PC1 (Fig. 4B) the main peaks of variance are observed to occur at ∼850, 1000 and 1600 cm−1. Intense peaks in these regions are also frequently assigned to tyrosine, tryptophan and phenylalanine vibrations indicating possible changes in orientation/exposure of these side chain residues with the addition of glycans in the transferrin molecule (Table 1). Peaks observed at ∼830, 880 and 1000 cm−1 have also been previously assigned to glycosidic ring and C–O–C stretches in sugars14 however for these samples spectral variations in this region are most likely from changes in secondary structure. A further spectral region of importance identified in the loadings plot of PC2 (Fig. 3C) occurs at ∼1270–1350 cm−1 frequently assigned to α-helical structure33,41 again indicating that the glycosylated and non-glycosylated forms of transferrin are distinguishable from their Raman spectra but most likely due to subtle protein modifications as a result of glycosylation rather than from the determination of the peaks arising from the sugars. This was also reported for our previous study of RNaseA and RNAseB.15
Quantifying glycosylation in transferrin
In order to develop the application of Raman spectroscopy for the analysis of glycosylated transferrin further, spectra were recorded from mixtures of glycosylated and non-glycosylated transferrin in order to attempt to quantify glycosylation from the Raman spectra. PLSR was applied to the data with bootstrap cross validations and Fig. 5A shows the liner regression results of a single bootstrap cross validation selected as a typical model based on the average R-squared value computed over all cross validations.
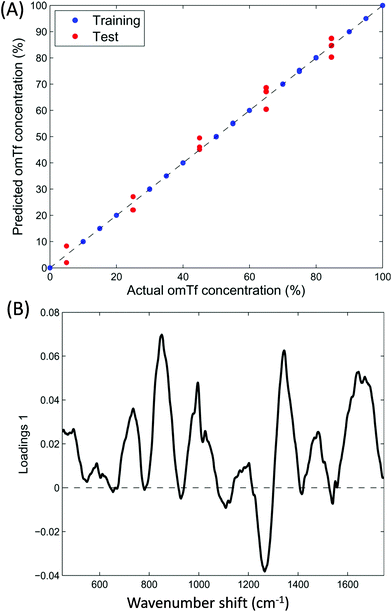 |
| Fig. 5 Partial least squares regression (PLSR) modelling from Raman data of the mixed samples of glycosylated and none-glycosylated apotransferrin. (A) PLSR predictions and (B) PLSR loadings plot. | |
Bootstrap is a re-sampling technique that can be applied as cross-validation to estimate the prediction performance of a model.42 The basic idea of this method is to select randomly, with replacement, N samples from a set containing exactly N samples. All selected samples, including the repetitions, are then used as training set and the non-selected samples are used as test set.43,44 One can think of this as having all N samples analysed in a bag (N = 21 in our case where the 3 sample replicates are kept together, either in training or testing, during sample picking). A single sample is taken out randomly and its number noted, this sample now forms part of the training data, and the sample is placed back into the bag. This random sample picking process is repeated until 21 samples are in the training set; this will include the some sample multiple times, and on average 63.2% of all of the samples will have been selected for training. The remainders (36.8%) are used as the test data. As can be observed in Fig. 5A displaying the liner regression results of a typical bootstrap cross validation (based on the average R-squared value computed over all cross validations) there is a good correlation between the predicted and actual concentrations of apoTfG. Furthermore the loadings plot from the PLSR model (Fig. 5B) also indicates very similar regions of importance as the loadings for the PCA (Fig. 4). Consequently, Raman spectroscopic data can be used to accurately measure the relative concentrations of the glycoprotein within a mixture of the native non-glycosylated protein.
Conclusion
Transport fusion proteins such as transferrin are being increasing utilized in pharmaceutical processes. However, the successful application of such proteins greatly depends on a good understanding of how ligand binding and glycosylation affect protein structure which in turn affects stability, immunogenicity and pharmacokinetics. Here we have demonstrated the unique sensitivity of Raman spectroscopy for determining iron binding, as well as glycosylation, and identifying the subtle structural modifications.
Acknowledgements
L. A., V. L. B and R. G. would like to thank the U.K. EPSRC and BBSRC and the industrial members of the Bioprocessing Research Industry Club (BRIC) for funding (BB/G010250/1; BB/K011170/1). We are also very grateful to Albumedix for providing us with transferrin protein variants.
References
- P. W. Berman and L. A. Lasky, Trends Biotechnol., 1985, 3, 51–53 CrossRef CAS.
- A. S. De Groot, Drug Discovery Today, 2006, 11, 203–209 CrossRef CAS PubMed.
- X. Zhong and J. F. Wright, Int. J. Cell Biol., 2013, 2013, 1–19 CrossRef PubMed.
- J. V. Neves, J. M. Wilson and P. N. S. Rodrigues, Dev. Comp. Immunol., 2009, 33, 848–857 CrossRef CAS PubMed.
- D. Wang, B. Y. Kim, K. S. Lee, H. J. Yoon, Z. Cui, W. Lu, J. M. Jia, D. H. Kim, H. D. Sohn and B. R. Jin, Comp. Biochem. Physiol., Part B: Biochem. Mol. Biol., 2009, 152, 20–27 CrossRef PubMed.
- H. Takagi, D. Shi, Y. Ha, N. M. Allewell and E. C. Theil, J. Biol. Chem., 1998, 272, 18685–18688 CrossRef.
- D. B. Kell, BMC Med. Genomics, 2009, 2 CrossRef PubMed.
- D. B. Kell, Arch. Toxicol., 2010, 577, 825–889 CrossRef PubMed.
- Y. Ke and Z. M. Qian, Prog. Neurobiol., 2007, 83, 149–173 CrossRef CAS PubMed.
- C. M. Cahill, D. K. Lahiri, X. Huang and J. T. Rogers, Biochim. Biophys. Acta, 2009, 1790, 615–628 CrossRef CAS PubMed.
- B. P. Gaber, V. Miskowski and T. G. Spiro, J. Am. Chem. Soc., 1974, 6868–6873 CrossRef CAS.
- S. L. Mecklenburg, A. B. Mason, R. C. Woodworth and R. J. Donohoe, Biospectroscopy, 1997, 3, 435–444 CrossRef CAS.
- C. Frank, O. Rienitz, R. Jahrling, D. Schiel and S. Zakel, Metallomics, 2012, 4, 1239–1244 RSC.
- M. Szybowicz, M. Koralewski, J. Karon and L. Melnikova, Acta Phys. Pol., A, 2015, 127, 534–536 CrossRef CAS.
- V. L. Brewster, L. Ashton and R. Goodacre, Anal. Chem., 2011, 83, 6074–6081 CrossRef CAS PubMed.
- J. M. Benevides, P. Bondre, R. L. Duda, R. W. Hendrix and J. G. J. Thomas, Biochemistry, 2004, 43, 5428–5436 CrossRef CAS PubMed.
- S. M. Kurtz, O. K. Muratoglue, F. J. Buchanan, B. Currier, R. Gsell, F. W. Shen and S. S. Yau, Biomaterials, 2001, 22, 2875–2881 CrossRef CAS.
- M. Shimoyama, H. Maeda, K. Matsukawa, H. Inoue and T. Ninomiya, Vib. Spectrosc., 1997, 253–259 CrossRef CAS.
- J. B. Cooper, Chemom. Intell. Lab. Syst., 1999, 46, 231–247 CrossRef CAS.
-
H. Martens and T. Næs, Multivariate Calibration, Wiley Press, 1992 Search PubMed.
-
E. V. Vinzi, W. W. Chin, J. Henseler and H. Wang, Handbook of Partial Least Squares, Springer, 2010 Search PubMed.
- P. T. Gomme and K. B. McCann, Drug Discovery Today, 2005, 10, 267–273 CrossRef CAS PubMed.
- S. L. Byrne, N. D. Chasteen, A. N. Steere and A. B. Mason, J. Mol. Biol., 2010, 396, 130–140 CrossRef CAS PubMed.
- J. Clarkson and D. A. Smith, FEBS Lett., 2001, 503, 30–34 CrossRef CAS PubMed.
- D. Wang, B. Y. Kim, K. S. Lee, H. J. Yoon, Z. Cui, W. Lu, J. M. Jia, D. H. Kim, H. D. Sohn and B. R. Jin, Comp. Biochem. Physiol., Part B: Biochem. Mol. Biol., 2009, 152, 20–27 CrossRef PubMed.
- C. Krebs, D. E. Edmondson and B. H. Huynh, Methods Enzymol., 2002, 354, 436–454 CAS.
- L. S. Vedula, G. Brannigan, N. J. Economou, J. Xi, M. A. Hall, R. Liu, M. J. Rossi, W. P. Dailey, K. C. Grasty, M. L. Klein and R. G. Eckenhoff, J. Biol. Chem., 2009, 284, 24176–24184 CrossRef CAS PubMed.
- S. E. J. Villar, H. G. M. Edwards and L. G. Benning, Icarus, 2006, 184, 158–169 CrossRef.
- J. Wang, D. L. Rousseau, H. M. Abu-Soud and D. J. Stuehr, Proc. Natl. Acad. Sci. U. S. A., 1994, 91, 10512–10516 CrossRef CAS.
- R. M. Solbrig, L. L. Duff, D. F. Shriver and I. M. Klotz, J. Inorg. Biochem., 1982, 17, 69–74 CrossRef CAS PubMed.
- J. E. Plowman, T. M. Loehr, C. K. Schauer and O. P. Anderson, Inorg. Chem., 1984, 23, 3553–3559 CrossRef CAS.
- M. N. Siamwiza, R. C. Lord, M. C. Chen, T. Takamatsu, I. Harada, H. Matsuua and T. Shimanouchi, Biochemistry, 1975, 14, 4870–4876 CrossRef CAS PubMed.
- S. W. Ellepola, S.-Z. Choi, D. L. Phillips and C.-Y. Ma, J. Cereal Sci., 2006, 43, 85–93 CrossRef CAS.
- C. N. Maiti, M. M. Apetri, M. G. Zargorski, P. R. Carey and V. E. Anderson, J. Am. Chem. Soc., 2004, 126, 2399–2408 CrossRef PubMed.
- B. F. Anderson, H. M. Baker, G. E. Norris, S. V. Rumball and E. N. Baker, Nature, 1990, 344, 784–787 CrossRef CAS PubMed.
- R. T. A. MacGillivray, S. A. Moore, J. Chen, B. F. Anderson, H. Baker, Y. Luo, M. Bewley, C. A. Smith, M. E. P. Murphy, Y. Wang, A. B. Mason, R. C. Woodworth, G. D. Brayer and E. N. Baker, Biochemistry, 1998, 37, 7919–7928 CrossRef CAS PubMed.
- N. Howell and E. Li-Chan, Int. J. Food Sci. Technol., 1996, 31, 439–451 CAS.
- T. Takekiyo, N. Takeda, Y. Isogai, M. Kato and Y. Taniguchi, Biopolymers, 2006, 85, 185–188 CrossRef PubMed.
-
T. Miura and G. J. Thomas, in Subcellular Biochemistry, Proteins: Structure, Function and Engineering, ed. B. B. Biswas and S. Roy, Plenum Press, New York, 1995, vol. 24, pp. 55–99 Search PubMed.
- C. Krebs, D. E. Edmondson and B. H. Huynh, Methods Enzymol., 2002, 354, 436–454 CAS.
- M. Tsuboi, M. Suzuki, S. A. Overman and G. J. Thomas, Biochemistry, 2000, 39, 2677–2684 CrossRef CAS PubMed.
-
K. Varmuza and P. Filzmoser, Introduction to Multivariate Statistical Analysis in Chemometrics, CRC Press, 2009 Search PubMed.
-
B. Efron and R. J. Tibshirani, An introduction to the bootstrap, Chapman and Hall/CRC, 1994 Search PubMed.
- B. Efron, Biometrika, 1981, 68, 589–599 CrossRef.
- S. Vohnik, C. Hanson, R. Tuma, J. A. Fuchs, C. Woodward and J. G. J. Thomas, Protein Sci., 1998, 7, 193–200 CrossRef CAS PubMed.
- M. Liang, V. Y. T. Chen, H.-L. Chen and W. Chen, Talanta, 2006, 69, 1269–1277 CrossRef CAS PubMed.
- T. Miura, H. Takeuchi and I. Harada, J. Raman Spectrosc., 1989, 20, 667–671 CrossRef CAS.
- J. P. E. Prevelige, D. Thomas, K. L. Aubrey, S. A. Towse and J. G. J. Thomas, Biochemistry, 1993, 32, 537–543 CrossRef PubMed.
- A. V. Mikhonin and S. A. Asher, J. Am. Chem. Soc., 2006, 128, 13789–13795 CrossRef CAS PubMed.
|
This journal is © The Royal Society of Chemistry 2017 |