DOI:
10.1039/C6SM00768F
(Review Article)
Soft Matter, 2016,
12, 5245-5256
Interactions of complex polymers with nanoporous substrate
Received
30th March 2016
, Accepted 27th May 2016
First published on 6th June 2016
Abstract
With the advance of polymer synthesis, polymers that possess unique architectures such as stars or cyclic chains, and unique chemical composition distributions such as block copolymers or statistical copolymers have become frequently encountered. Characterization of these complex polymer systems drives the development of interactive chromatography where the adsorption of polymers on the porous substrate in chromatography columns is finely tuned. Liquid Chromatography at the Critical Condition (LCCC) in particular makes use of the existence of the Critical Adsorption Point (CAP) of polymers on solid surfaces and has been successfully applied to characterization of complex polymer systems. Interpretation and understanding of chromatography behaviour of complex polymers in interactive chromatography motivates theoretical/computational studies on the CAP of polymers and partitioning of these complex polymers near the CAP. This review article covers the theoretical questions encountered in chromatographic studies of complex polymers.
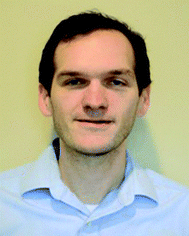
Jesse D. Ziebarth
| Jesse Ziebarth is currently a post-doctoral fellow in the Chemistry Department of the University of Memphis. He received his PhD in Chemistry from the University of Memphis in 2010 and, then, worked as a post-doctoral fellow in bioinformatics at the University of Tennessee Health Science Center. His current research is focused on multiscale modeling of macromolecules, with particular interest in polyelectrolytes and polymers with complex architectures. |
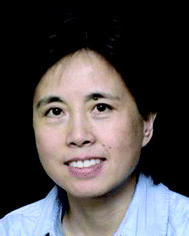
Yongmei Wang
| Yongmei Wang received her BS degree from University of Science and Technology of China in 1985 and PhD in Chemistry from University of Notre Dame in 1990. She then worked as post-doctoral researcher at University of Akron under the supervision of Prof. Wayne L. Mattice from 1990–1993, and at University of Houston under the supervision of Prof. Raj Rajagopalan from 1994–1996. She began her own academic career in 1996 as an Assistant Professor at North Carolina Agricultural and Technical State University and moved to the University of Memphis in 2003. She now is a tenured full professor at Chemistry Department of the University of Memphis. Her research interests are theoretical/computational studies for polymer separation and characterization, polymer based gene delivery and nanomedicines. |
Introduction
The term “complex polymer” is a vague term that can refer to any polymeric system other than simple linear homopolymers. Here we will be concerned with complexity arising from chain architecture (i.e., star, ring, graft or hyperbranched polymers) and/or chemical composition (i.e., copolymers or end-functionalized polymers). Recent advances in polymer synthesis have enabled the creation of a large number of novel complex polymers, such as block, star, dendritic, cyclic and graft polymers that exhibit unique properties and have important applications.1–3 Although synthesis of these complex polymers is performed with good control and one might hope that the final product will have a narrow dispersity in the desired architecture or chemical composition, the reality however is that the final product contains mixtures of products with different structures that may also be mixed with initial macromonomers. A detailed characterization of complex polymer system is therefore needed. Polymer liquid chromatography in different elution modes has been shown to be very useful in characterizing these complex polymers.4–7 To understand the elution of polymers in polymer liquid chromatography, one needs to consider the interaction of polymers with the nanoporous materials used in the column substrate. It is in this context that we discuss the interaction of complex polymers with nanoporous materials.
We will begin with a brief introduction to different elution modes encountered in polymer liquid chromatography and how they relate to the interaction of polymers with the porous substrate. Several excellent reviews, mostly focusing on experimental results and procedures, have been written on this subject.4–10 Here we will focus on the microscopic picture of how a complex polymer interacts with nanoporous materials in these elution modes. It will become clear that there is an interesting fundamental question encountered in understanding the elution of complex polymers in polymer liquid chromatography, namely, the existence of a Critical Adsorption Point (CAP) of a polymer on the column substrate. The existence and properties of the CAP has been actively studied theoretically and computationally in the past,11–17 mostly independent of liquid chromatography developments. We will try to bridge these areas of studies to bring a better understanding of how complex polymers interact with porous media in the context of liquid chromatography characterization of these complex polymers.
Theory for polymer elution in liquid chromatography
The elution time (or elution volume VR) of a polymer sample in liquid chromatography can be related to a thermodynamic quantity, the partition coefficient of a polymer chain, K, between the mobile phase and the stationary phase, K = Cs/Cm, where Cs and Cm are the polymer concentrations in the two phases respectively. Most polymer liquid chromatography is performed with columns packed with porous beads containing pores ranging from 100 Å to 500 Å. The mobile phase is taken as the eluent moving through the interstitial packing space between the porous beads, and the stationary phase is taken as the pore space inside the porous beads. Thus, theoretical study of the partition coefficient K of a polymer solution between a pore (modelled as either a slit pore or a cylindrical pore) and a bulk unconfined solution will provide a basic understanding of polymer elution in liquid chromatography. Since chromatography is performed at dilute concentration, one only needs to consider the partition coefficient K of a single polymer chain. The partition coefficient is related to the difference in standard free energy ΔG0 of transferring a single chain from a bulk solution to the pore through the equation RT
ln
K = −ΔG0. Several different theories and computational approaches can be used to compute the free energy difference and these will be discussed.
Elution modes in polymer liquid chromatography
Polymer chains in synthetic polymer sample do not have the same molecular weight, instead there is a distribution of molecular weights. Size exclusion chromatography (SEC) has long been used to characterize the molecular weight distribution in polymer samples. In SEC, polymer chains do not “absorb” on the porous substrate in a chromatography column and they elute through the column based on their sizes. The elution time decreases with polymer molecular weight in SEC. If polymer chains adsorb on the column substrate in what is often called Liquid Adsorption Chromatography (LAC), then the elution time usually increases with the molecular weight. In transitioning from SEC to LAC modes, one can achieve a “Critical Condition” where the elution time becomes independent or nearly independent of the molecular weight of the polymer (see Fig. 1). This mode is now called Liquid Chromatography at the Critical Condition (LCCC), although other names such as liquid chromatography at the Critical Adsorption Point (LCCAP) have been used in the literature.18–20 More and more groups are now using LCCC to characterize complex polymer systems.
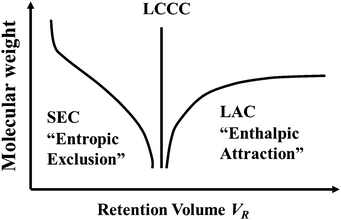 |
| Fig. 1 Illustration of the three elution modes in polymer liquid chromatography: Size Exclusion Chromatography (SEC), Liquid Adsorption Chromatography (LAC), and Liquid Chromatography at the Critical condition (LCCC). | |
The link between LCCC and the Critical Adsorption Point
The critical condition underlying LCCC is related to the Critical Adsorption Point (CAP) of a polymer chain adsorbing on a solid surface. The relationship was recognized by Skvortsov and Gorbunov21 during the initial development of LCCC. They drew their understanding from earlier studies of adsorption of a long flexible polymer chain near a solid surface. These earlier studies of polymer adsorption revealed that there exists a critical polymer segment/surface interaction energy, εcapw. If the polymer segment/surface interaction is less attractive than εcapw, then the long polymer chain will form a depletion layer near the surface since the solid surface restricts the number of conformations of the polymer chain. On the other hand, if the segment/surface attraction is more attractive than εcapw, then the polymer chain will be adsorbed on the surface and form an adsorption layer. The specific value of εcapw depends on specific polymer models used in theoretical discussion, very much like a specific type of polymer would achieve LCCC condition at a specific temperature and solvent condition. If a polymer chain is modelled as a random walk (RW) on a lattice, then the CAP for the RW chain is given by εcapw = ln[(Z − λ1)/Z], where Z is the coordination number and λ1 is the number of steps forbidden by the presence of the surface (εcapw is reduced by thermal energy kBT). The rationale behind this is that the partition function of a RW chain with N monomers in free solution is simply given by qfree = ZN−1. The partition function of a chain above the surface can be written as qsurf = Z(N−M−1)(Z − λ1)M
exp(−εwM) where M is the number of monomers in contact with the surface. Therefore, when |εw| = |εcapw| = ln[(Z − λ1)/Z], the entropic loss is compensated by the enthalpic gain. The chemical potential of the chain in free solution becomes the same as when absorbed on the surface, signifying a Critical Adsorption Point.
Alternatively, the polymer chain can be represented by a Gaussian chain consisting of N segments each of length b, the conformation of the chain is accounted for by PN(r), the probability of a chain with end-to-end vector r that satisfies the diffusion equation:
| 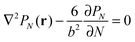 | (1) |
In the unbounded free solution, this gives a Gaussian distribution function with average radius
R = (
Nb2/6)
1/2. Near the surface, the solution to the above equation needs to satisfy the boundary condition which can be written as:
|  | (2) |
The physical significance of the above boundary condition was discussed by de Gennes.
22 The parameter
c is related to the surface potential energy (reduced by
kBT) and the above boundary condition can be interpreted as how the polymer chain free energy changes per segment near the surface. The CAP corresponds to the condition that
c → 0, whereas a repulsive wall corresponds to
c > 0, while an adsorptive wall corresponds to
c < 0. The physical interpretation of the CAP under the context of the Gaussian chain model is that the free energy change per monomer near the surface is exactly zero, not positive (repulsive wall case), not negative (attractive wall case).
The above discussions focus on how a long flexible chain interacts with a single solid surface. More relevant to polymer elution in chromatography would involve the consideration of polymer chains partitioning into pores with surfaces that are adsorptive or repulsive. The pore is often approximated by a slit pore consisting of two parallel walls separated by a given distance. Consideration of how other pore geometries would impact the partitioning of polymer chains is also relevant and will be discussed briefly later. The elution behaviour of the polymer in SEC mode was studied by Casassa using the Gaussian chain model. The boundary condition near surface in SEC was simply given by PN(r) = 0, which correspond to c → +∞. Skvortsov and Gorbunov21,23,24 extended Casassa's theory and considered the partitioning of a polymer chain into a pore with adsorptive surfaces. They theoretically computed the partition coefficient K for a single polymer chain into a slit pore under the conditions where the pore surface is non-adsorptive, at the CAP, and adsorptive. They clearly show that the calculated K versus chain length N exhibits the kind of pattern depicted in Fig. 1. Guttmann et al.25 considered the same problem but used a lattice based random walk chain model using a matrix generation method. Both theoretical approaches clearly showed that the LCCC corresponds to the condition that the polymer surface interaction energy is at the CAP. Moreover, these theories showed that, at the CAP, the partition coefficient K becomes independent of chain length N and KCAP = 1 at the CAP (for the lattice based random walk model, K becomes one at long chain limit).
Excluded volume effect on the Critical Adsorption Point
Both the random walk and Gaussian chain models fail to take into account the excluded volume interaction, which can be significant for polymer chains confined in nanopores. An unphysical consequence of using RW and Gaussian chain models to study polymer chains confined in the pore is discussed in de Gennes's textbook.26 For a RW chain or a Gaussian chain confined in a pore, the physical dimension of the chain along the unconfined direction will remain the same as in the free unconfined bulk solution regardless of how strongly the chain is confined. The chain will not be stretched in the unconfined directions. A real polymer chain confined in a pore will be stretched in the unconfined dimensions because of the excluded volume interaction. Another misconception is that the excluded volume interaction will be absent in a theta solvent. In theta solvent, the polymer chain radius of gyration Rg no longer scales with N as Rg ∼ Nν (ν is the Flory exponent) as in the case of the SAW chain model, but scales as Rg ∼ N0.5 as in the case of the RW model. This change in scaling behaviour is a result of balance between “attractive interaction between repeating units” due to a bad solvent condition and the excluded volume interaction between the repeating units. However, when the chain is confined in the pore at a theta solvent condition, the chain will still be stretched in the unconfined dimension. Therefore, there are some fundamental differences between a real polymer chain and a Gaussian chain or RW chain model regardless of the solvent condition. Fortunately, most chromatography separations are done using porous media that only provide moderate confinement. Hence theoretical studies using RW and Gaussian chain models in most cases are relevant to experimental conditions, but these theoretical predictions need to be understood in light of understanding gained from consideration of the excluded volume effect.
A more realistic model to represent a polymer chain is the self-avoiding walk (SAW) on the lattice. For studying SAW chain, analytical approaches often fail and one needs to resort to computer simulations. For self-avoiding walks on the lattice, Hammersely et al.15 have proved mathematically that a SAW chain near an impenetrable surface has a phase transition dictated by the CAP. The exact value of the CAP is not precisely known and is model dependent, but the definition of the CAP can be understood as the point where the monomeric chemical potential of an infinite long chain near the surface (defined as
, where ZN is the canonical partition function of a chain with length N) equals the monomeric chemical potential of the chain in the bulk solution. Many computational studies14,27–33 have tried to quantify the behaviour of the chain crossing the CAP. For example, when the chain goes from a nonadsorbed state to an adsorbed state, the energy of the chain E changes from an intensive variable independent of chain length N to an extensive variable dependent on N. At the CAP, E is expected to scale with Nϕ where ϕ is the crossover exponent. The cross over exponent ϕ, which is believed to be a universal constant, has been the subject of debate. For chains with excluded volume interaction, the CAP is defined in the limit of long chain, hence these computational studies have tried to determine the CAP by extrapolating quantities such as the number of surface contacts per chain or peak position in the heat capacity of the adsorbed chain, to infinite chain length. These studies usually focus on a single chain interacting with a single solid surface.27–31,34,35
In order to understand how the excluded volume interaction impacts the elution behaviour of chains in LCCC, Gong and Wang36 examined the partitioning of SAW chains into a slit pore with interactive surfaces via computer simulations. The simulation method relied on the determination of the chemical potential of a polymer chain via the biased chain insertion method. From the chemical potential one obtains the partition coefficient K using −RT
ln
K = μconf − μbulk, where μconf is the chemical potential of the chain in the confined situation and μbulk is the chemical potential in bulk solution. A challenge first encountered is the unknown value of εcapw in the SAW model. Hence the authors simultaneously employed the RW model as a reference since the εcapw for the RW is known precisely. One thing that became apparent in that study is that the LCCC condition cannot be defined at the point where K(N) = 1. K(N) crosses over from K < 1 to K > 1 at different surface interaction energies for different chain length. This complication does not exist for the RW chain model. Nevertheless, for the SAW chain, one can still define a co-elution point where K(N) exhibits the least dependence on chain length N, similar to the LCCC condition identified in experiments. This point can be determined by plotting the variation in K for a given range of chain length, σ(ln
K), as function of surface interaction εw, which yields a well-defined minimum (see Fig. 2). This minimum, which we ascribe as the pseudo-CAP, was the apparent co-elution point and occurs at surface interaction energy εcapw = −0.276 for a SAW in a simple cubic lattice with coordination number z = 6. If we identify εcw as the point where K(N) = 1, then in the limit of N → ∞, εcw approaches the εcapw by assuming that the critical point scales37 with N0.5. All these results point to the fact that the co-elution point in LCCC condition likely occurs at the CAP of a polymer sample. Although the CAP of a polymer chain with excluded volume interaction is traditionally defined in the limit of infinite chain length, in the context of understanding LCCC chromatographic behaviour, we will no longer use that definition, but instead define it as the apparent co-elution point of polymer chains with a finite molecular weight range. Whether such a pseudo-CAP corresponds to the traditional definition of CAP in the infinite long chain limit is not known precisely, but this will not impact how one can understand the chromatographic behaviour of polymers in LCCC.
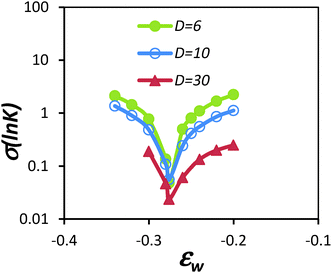 |
| Fig. 2 Plot of standard deviation in the natural log of partition coefficient, σ(ln(K(N))), for a self-avoiding walk with chain length varied from N = 25, 50, 100 and 200, partitioning into a slit with two parallel walls separated by a distance D. εw is the segment/surface interaction energy. The minimum identified in the plot corresponds to the CAP (figure reproduced from ref. 38). | |
The use of the SAW model also revealed some features absent when using the RW chain model. Notably, when the pore size decreases, the partition coefficient K showed a non-linear variation with the chain length, as shown in Fig. 3. These data suggest that entropic loss is not exactly balanced by enthalpic gain per monomer. At the CAP, the entropic loss is slightly over compensated by the enthalpic gain. For short chain, as the pore size decreases, the over compensation seems to increase. But for long chains, K decreases with N as the pore size decreases. In comparison, the same calculation for the RW chain model showed that K is independent of chain length at the CAP, although K was slightly greater than one.
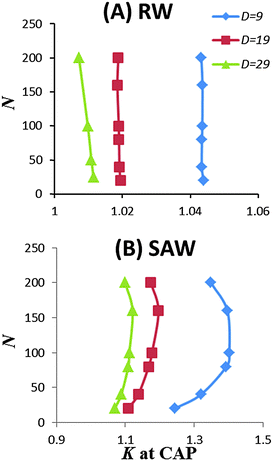 |
| Fig. 3 Dependence of partition coefficient K of a linear homopolymer with chain length N at the predetermined CAP condition at three different pore widths D. (A) RW chain model; (B) SAW chain model on a simple cubic lattice with coordination z = 6. | |
Recently Neimark and coworkers39,40 looked into critical conditions of polymer chains adsorbed on porous substrate with off-lattice chain models. They determined the incremental chemical potential of adsorbed and free chains and defined the CAP as the point μadincr = μfreeincr. This definition of the CAP would naturally yield the condition that ∂K/∂N = 0, which coincides with the LCCC condition in experiment. Neimark and coworkers considered a chain completely adsorbed in a spherical cavity or partially translocated into spherical cavity and they compared the behaviour of both ideal chains and chains with excluded volume interaction. For chains with excluded volume interaction, they showed that K was never completely independent of chain length N, similar to results obtained by Gong and Wang.
Impact of pore size and geometry on the CAP
Changes in pore size and pore geometry can impact polymer adsorption in pores as was mentioned in the previous section. Let's more carefully consider whether the change in pore size may cause a shift in the CAP of a polymer chain inside the pore. The answer to this question depends on both the pore geometry and the chain model used. If one uses the RW or Gaussian chain model, then the CAP accordingly will not shift if the pore geometry is slit like or cylindrical. However, for a cylindrical pore, one needs to be careful to take into account a trivial geometry effect: upon decreasing the pore size, the monomeric surface interaction strength changes.16 If a cylindrical pore is modelled by a square lattice, then the “corner” sites experience a different geometrical confinement. As pore size decreases, the fraction of these corner sites increase. This can lead to an apparent shift in the CAP even for RW chains. However, with proper handling of this corner effect, one can show that the CAP for the RW does not shift in a cylindrical pore as pore size decrease.41 In contrast, when chains are confined in spherical geometries, the CAP will shift even for RW and Gaussian chain models, as demonstrated in the work by Yang and Neimark.39
Consideration of the excluded volume effect leads to different conclusions. Whittington and coworkers42,43 considered long self-avoiding walks chains in a slit with attractive walls using Monte Carlo simulations and a directed walk approach. For the slab situation, if both the top and bottom walls are the same, they have shown that the critical point remain the same as for chains adsorbed on one single solid surface. This implies that the CAP for SAW chain absorption in a slit will not shift. This conclusion is consistent with what we observe as shown in Fig. 2. However, Monte Carlo simulations of SAW chains in the cylindrical pore showed that the CAP shifts to lower temperature (or stronger adsorption) as pore size decreases. Experimental studies have reported shifts in the LCCC condition when the pore size of the chromatography column beads changed; the shift in most cases is toward a more adsorptive condition as the pore size decreases.44
LCCC characterization of complex polymers
Complex polymers introduce heterogeneity in properties in addition to the molecular weight distribution found in all synthetic polymers—a star polymer sample may contain chains with different numbers of arms and a copolymer sample may contain chains with different monomer fractions or chain sequences. We will not go into details to explain how LCCC or other forms of interactive chromatography are useful to characterize complex polymer systems. One may refer to other reviews for successful examples. Here we want to briefly point out some special features of LCCC characterization of complex polymers. First, by performing chromatography separation at the LCCC condition, one suppresses the sample separation according to the molecular weight distribution, enabling separation according to heterogeneity in other chemical identifiers. For example, polymer chains with different end groups can be separated according to the number and types of end groups without interference from molecular weight distributions at the LCCC condition of the backbone.45 Another advantage is that establishing LCCC or another mode of interactive chromatography allows for two-dimensional separation that provides better characterization of samples.
It is important to recognize that different chemical components in a complex polymer sample interact with pore surfaces differently. In practice, the LCCC condition is typically established at one of the chemical components. The other components may be more adsorptive (LAC mode) or less adsorptive (SEC mode). In addition, the relationship between the molecular weight and physical size of architecturally complex polymers differs from that of linear polymers, and the dependence of K on N for complex polymers partitioning into pores will not be the same as linear polymers. The remainder of this review will focus on recent experimental and theoretical efforts to understand liquid chromatography characterization of complex polymers in light of these and other complications.
Partitioning of star polymers
Partitioning of a star polymer with f number of arms each consisting of N segments under SEC condition was studied by Casassa and Tagami46 and later reinvestigated by Teraoka.47 The partition coefficient K was found to be dependent on the number of arms in addition to the radius of gyration. Teraoka showed that the hydrodynamic radius Rh provides a better measurement on the partition coefficient K and can provide a better universal calibration curve for star polymers prepared with different numbers of arms.
An interesting question arises when one considers the partitioning of star polymers at the LCCC condition. What would the partition coefficient K of a star be if the surface interaction of the polymer with the substrate is at the CAP of the corresponding arm? Using the Gaussian chain model, the continuum theory predicts that K(stars) = 1, just like that of the linear homopolymer. However, using the lattice based random walk (RW) model, K for stars with finite arm length is actually slightly greater than one and increases with the number of arms.25 Nevertheless, the above physical picture of stars partitioning in LCCC is not complete because of the use of the RW model and the Gaussian chain model. As one might expect, a star with many long arms will experience greater entropic exclusion from the pore even at the LCCC condition. Experimentally Chang's group prepared multi-arm polystyrene stars using macromonomers and investigated the elution behaviour of these stars at the LCCC condition of the polystyrene homopolymers. They found that stars made with short arms eluted after the LCCC retention time. However, for stars made with long arms they clearly observed that the stars eluted before the LCCC retention time and in the exclusion region.48 Monte Carlo simulations of stars modelled using the SAW chains were able to reproduce this behaviour nicely. However, simulations using RW chains would not predict the exclusion of stars with long arms.48 A rigorous comparison with experimental data needs careful consideration of end-group effects.
A different question may be asked here: what is the CAP for the star? Is it same as the linear polymer? To answer this question, one needs be careful with how the CAP for the stars is defined. If one defines the CAP as the point where K(N) = 1, which we will call the compensation point, then, for the same total molecular weight, stars reach the compensation point at a less adsorptive condition than linear polymers as long as the pores are wide enough, as demonstrated in computational studies by Chen and Escobedo.49 The situation may change if the pores are small and polymers in pores encounter strong confinement.
Partitioning of ring polymers
The conformational properties of ring polymers have attracted many studies due to complexities arising from topological constraints.50–54 Rings can be either knotted or unknotted and they cannot interconvert between different topologies without bond breaking and reformation. As a result, theoretical studies of rings using the Gaussian chain model suffer from the inability to account for these topological constraints. It was shown that, even in the absence of excluded volume interactions, the size of rings do not scale with chain length Nν with ν = 0.5 as predicted by the Gaussian chain model, instead the exponent ν > 0.5 for rings.50,53,54 Interestingly, Li et al.55 recently showed that while the conformational properties of unknotted rings differ from Gaussian chain rings, the confinement free energy of unknotted rings by a non-adsorptive slit follows the same scaling law as that of Gaussian chain rings.
Despite the limitation of Gaussian chain model to treat ring polymers, Gaussian chain theory has been used to study the partition of ring polymers under both SEC and LAC conditions. The partition coefficient K for a ring polymer is predicted to be greater than that of a linear polymer with identical chemical repeating units and the same molecular weight in the SEC condition. A ring polymer would have a smaller size than the corresponding linear polymer due to the connectivity of the two ends. Hence it is easy to envision that Kring will be larger than Klinear in SEC (Kring > Klinear). In the LAC mode, the partition coefficient K for the ring polymer is also greater than that of the linear chain. This may not appear obvious, but is predicted by the Gaussian chain theory. At the LCCC of the linear polymer, the ring elutes in LAC mode and Kring increases with an increase in molecular weight. Chang's group reported the successful fractionation of ring polymers from the impurity of the linear precursors.56 The elution of the ring at the LCCC condition of the linear polymers was shown to be in the LAC mode and elution time increased with an increase in the molecular weight.57 The impact of topological constraints and excluded volume interactions on the partitioning of ring polymers at the LCCC condition has not been investigated computationally.
Partitioning of block copolymers or grafted copolymers
In the case of block copolymers, such as AB diblock or ABA triblock copolymers, one can define a CAP for individual A or B blocks, which is the same as the CAP for the homopolymer A or homopolymer B. When one of the blocks is at the CAP (say the B block), it is very unlikely that the A block will be at the CAP as well. Most likely, the A block will be either repelled from the porous surface (SEC condition) or more adsorbed on the column (LAC condition). Having one of the blocks at the LCCC condition enables better characterization of the other block in several ways. For example, Chang's group characterized poly(ethylene oxide)-b-poly(L-lactide) (PEO–PLLA) while the PEO block eluted in LCCC mode and the PLLA block eluted in LAC mode.58 The chromatogram of the block copolymer sample obtained under this condition achieved baseline separation of PLLA repeating units. Away from the LCCC condition of the PEO block, the influence of the PEO block on the elution of the copolymer spoiled the baseline separation of PLLA repeating units. The explanation for these results is that although the PEO block has a polydispersity, at the LCCC condition of PEO, the PEO block does not perturb the retention of the block copolymer because the PEO block is in LCCC mode. Hence separation of the block copolymer is determined by the PLLA length at the LAC condition, which could enable the baseline separation according to PLLA repeating units. However, away from the LCCC condition of PEO, the PEO block perturbs the retention of the copolymer sample and the polydispersity of the PEO block spoils the baseline separation of PLLA repeating units.
Another possible separation scheme involves eluting the copolymer at the LCCC condition of one of the blocks, say the B block, while the A block elutes in SEC condition or LAC condition. It was hypothesized that the B block will not influence the retention of the copolymer sample and hence the retention time of the block copolymer can be compared against the calibration curve of the A homopolymer, allowing for the determination of the A block molecular weight based on the calibration curve. This approach has been used to characterize the polystyrene-b-poly methylmethacrylate (PS-b-PMMA) by Pasch et al.59,60 According to the Gaussian chain theory, the invisible block would have K = 1 and therefore does not impact the elution of the copolymer sample. However, Chang's group examined this hypothesis critically by preparing a series of polystyrene-b-polyisoprene (PS-b-PI) samples where either the PS block (SI series) and/or the PI block (IS series) were kept at the same length.61 They then subjected the copolymer samples to a chromatography condition where PS (the invisible block) was at the LCCC condition, and the other block PI eluted at the SEC condition. The elution time of the SI series copolymer, which had constant visible PS block length and varying PI block length, increased with increase in the invisible PI block length. This shows that the invisible PI block could still impact the elution time of the copolymer, although the influence was weak (the peak position shifted very slightly). Their experimental observation therefore raised questions about the validity of the theoretical conclusion regarding block copolymer elution when one of the blocks is at the LCCC condition.
Later, the same group62 characterized block copolymers composed of a hydrogenated PS block and a deuterated PS block (hPS-b-dPS). The strength of interaction of hPS and dPS with the column strength is similar but sufficiently different to allow for different chromatographic behaviour of the two blocks. With the same mixed solvent CH2Cl2/CH3CN (57/43, v/v), and the same column substrate (C18 Lunasil), the LCCC condition for a hPS homopolymer was found to be 40 °C while the LCCC condition of a dPS homopolymer was 33.6 °C. Thus the hPS block interacts with the column substrate more favourably than dPS at the same condition. When hPS homopolymer is at the LCCC condition, dPS is eluted in SEC mode whereas, when dPS is at the LCCC condition, hPS is in LAC mode. They then examined the elution of block copolymer samples by setting either the hPS at LCCC or the dPS at LCCC condition respectively. This study further revealed that when the visible block hPS is eluted in the LAC mode, the “invisible” dPS block decreases the elution time of the block copolymer. Previously, during characterization of graft copolymers with an hPS backbone and dPS side chains,63 they also found that increase in the length of dPS side chains decreases the elution of the graft copolymer even though the overall graft copolymer is eluted after the solvent peak (in LAC mode).
Initially we thought that the above phenomenon was due to the excluded volume effect that was absent in the ideal chain model (either the RW lattice model or the Gaussian chain model).64 However, Monte Carlo simulations with the RW chain model were able to see the impact of the invisible block on the elution of block copolymer in exactly the same pattern as observed in Chang's experimental studies. Using the RW lattice model, we showed that K(A-b-B)/K(A) deviates from one when the B block is added (Fig. 4). Here the A block is the chromatographically visible block and the B block is set at the LCCC condition. When the A block is in the SEC mode, K(A-b-B) > K(A) (Fig. 4a for |εw(A)| < 0.18). The presence of the invisible B block lengthens the elution of the block copolymer. When the A block is LAC mode, the presence of the invisible B block reduces the elution of the block copolymer and K(A-b-B) < K(A). For graft copolymers, when the side chain is set in the LCCC condition, the side chain impacts the elution of graft copolymer similarly, but to an even greater extent because of a higher number of grafts attached to the backbone.65 Introducing the excluded volume effect in the SAW chain makes the overall observed phenomena more significant. We attributed the impact of the invisible block on the elution of block and graft copolymers to chain connectivity. A more detailed explanation can be found in the original article.65
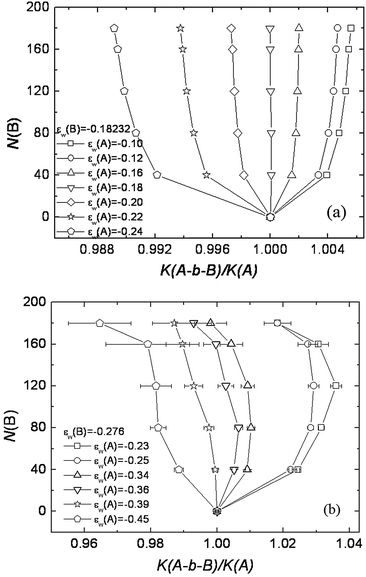 |
| Fig. 4 Plot of B block length N(B) vs. K(A-b-B)/K(A) at the critical condition of B block. (a) εw(B) = −0.18232 and (b) εw(B) = −0.276. The length of visible A block is fixed as 40. The interaction of A block with the surface εw(A) is varied from SEC mode to LAC mode. (a) RW chain model: εw(A) = −0.10, −0.12, −0.16 correspond to the SEC mode and εw(A) = −0.20, −0.22 and −0.24 correspond to the LAC mode. (b) SAW chain model: εw(A) = −0.23, −0.25 correspond to the SEC mode and εw(A) = −0.34, −0.36, −0.39 and −0.45 correspond to the LAC mode. (figure reproduced with permission from Fig. 5 of ref. 62). | |
CAP of statistical random copolymers
For copolymer samples, the distribution in chemical composition becomes an important characteristic in addition to the molecular weight distribution. Copolymers are typically prepared by copolymerization of two or more monomers. The chemical composition and sequence distribution of copolymers depends on the overall feed ratio of the monomers during polymerization, the reactivity of the monomers and other factors. Here the chemical composition refers to the fraction of each type of monomer found in a given copolymer chain, and the sequence distribution refers to the connectivity of the monomers along the chain. Taking copolymers made of A and B monomers, the sequence distribution refers to how the A and B monomers are arranged along the chain. One can have two extreme cases: block copolymers like A-b-B, and alternating copolymers (AB)n, as well as many intermediate sequence distributions. The sequence distributions can be further characterized by defining P(A|B), the conditional probability of finding a B monomer immediately after an A monomer. For a copolymer consisting of A and B monomers, there are four such conditional probabilities: P(A|B), P(A|A), P(B|A) and P(B|B). These four conditional probabilities satisfy the rule P(A|B) + P(B|B) = fB, P(A|A) + P(B|A) = fA where fA and fB are the fractions of A and B monomers present on the chain, and fA + fB = 1. Using these conditional probabilities, one can now define a sequence order parameter χ = 1 − P(A|B) − P(B|A). The sequence order parameter χ can range from −1 to +1, with −1 for an alternating copolymer, and +1 for a block copolymer. A statistical random copolymer refers to an AB copolymer where the probability of finding a monomer A is independent of previous monomers. This would imply that P(A|A) = P(B|A) = fA, and P(A|B) = P(B|B) = fB, thus χ = 0.
As discussed above, the interaction between the surface and the two monomers likely will not be the same. Let's assume that only type B is attractive, εBw < 0, and the other type A is non-interactive, εAw = 0. The question is then whether this type of copolymer will have a CAP point and, if it exists, how it compares to the CAP for the homopolymer B. The answer to this question is not straightforward. In the case of a block copolymer AB, each individual block would have a CAP like homopolymer A or B. In the case of alternating copolymer, one may treat (AB) as a new monomer, and naturally this would behave just like a homopolymer. Nevertheless this question was studied using different theoretical approaches66–71 and the conclusion is that statistical AB copolymers have a CAP just like a homopolymer. A few Monte Carlo simulations have also determined the CAP for a statistical copolymer with different compositions of A and B, but the general equation that gives the dependence of CAP of statistical random copolymers on the chemical compositions were only discussed in Brun's paper72,73 and in our paper.37 For statistical random copolymers, one can define an effective surface interaction, εeff, that is related to εAw and εBw through:
| exp(εeff) = fA exp(εAw) + fB exp(εBw) | (3) |
The adsorption of a statistical random copolymer is then reduced to the adsorption of a homopolymer with the effective surface interaction
εeff and the CAP of the statistical random copolymer is determined when
εeff = −0.276, the CAP for a homopolymer in the lattice model. As a result, the CAP of a statistical random copolymer CAP is not linearly dependent on
fA or
fB.
Fig. 5 illustrates the dependence of the CAP for a statistical random copolymer on the B composition assuming that
εAw = 0 and
εBw is attractive. If
εAw = 0 and
fB = 1, the statistical copolymer chain becomes a B homopolymer. The strength of
εBw needed to make
εeff equals the CAP is just the same as the CAP for the homopolymer B, which was
εw(CAP) = −0.276. As
fB decreases, a stronger surface interaction
εBw is needed to reach the CAP for the statistical copolymer. The dependence of
εBw at the CAP on
fA and
fB predicted by
eqn (3) was confirmed by Monte Carlo simulations, as shown in the
Fig. 5.
37 Here the CAP for the statistical random copolymer was determined following the method we used to determine the co-elution point, namely we determined the chemical potential of a grafted chain for a given range of chain length
N above a solid surface and identified the CAP as the point where −
RT![[thin space (1/6-em)]](https://www.rsc.org/images/entities/char_2009.gif)
ln
K =
μsurf −
μbulk varied least with chain length. It is worth mentioning that the above derivation uses the “annealed approximation” which is also met in Monte Carlo simulations. For a given fraction
fB, there are still many possible different sequence arrangements for a statistical random copolymer. The annealed approximation implies that calculated chemical potentials are averaged over all possible sequences. In our Monte Carlo simulations, the chemical potential of a copolymer chain above a solid surface was averaged over many different sequences of a statistical random copolymer for a given
fB, so the simulation mimics the annealed approximation.
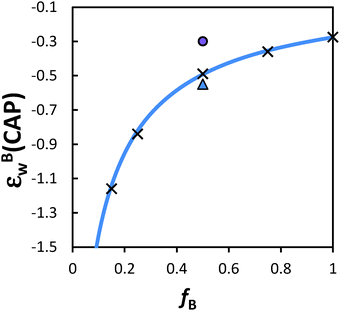 |
| Fig. 5 Dependence of the interaction strength of B monomers needed to make a statistical AB copolymer reach the CAP on composition of monomer B. Solid blue line is the plot according to eqn (3); (×) are the CAP determined from Monte Carlo simulations of statistical random copolymers; (▲) is the CAP determined for alternating AB copolymers; (●) is the CAP determined for AB block copolymers (reproduced with permission from ref. 37). | |
The above discussion on a statistical random copolymer adsorbing on a solid surface can also be extended to the case of a homopolymer chain adsorbing over a statistical random surface composing of two surface sites, a and b. Again under the “annealed approximation”, a similar effective interaction can be used to describe the adsorption of a homopolymer over a statistical random surface. Here the annealed approximation implies that the chain samples different regions of the random surface. This condition was met in our Monte Carlo simulations since the chain was grown at random locations on the surface.
The above theoretical results have the following implications on chromatographic separation of statistical copolymers. Experimentally it is not convenient to determine LCCC condition of a statistical copolymer since samples of statistical copolymer with different molecular weight but with the same chemical composition are not easily available. However, there is another way to reach the Critical Adsorption Point, namely gradient adsorption chromatography. In such an experiment, polymer samples are initially adsorbed on the column substrate. Then the eluent composition is changed during gradient elution such that eluent promotes desorption of polymers from the column substrate. One might expect that short polymer chains detach from the substrate more easily. However, at a proper eluent composition, high molecular weight species of the same polymer type will detach from the surface almost independent of the molecular weight. This eluent composition is close to the LCCC condition for that type of polymer. Brun discussed and demonstrated this experimentally.74 By applying gradient elution to statistical copolymers, Brun was able to separate polyethylene samples with similar molecular weights but different amount of chlorination.72 On the other hand, one could intuitively expect a dependence of adsorption on the fraction of adsorptive monomers without such rigorous theoretical consideration. Back in the 1980's, Mori and coworkers applied gradient elution in LAC and separated copolymers of styrene-co-methyl methacrylate according to the chemical composition.75,76 Sato et al. studied the same system but went a step further and elucidated that the separation of styrene-co-methyl methacrylate on polar column substrate was an adsorption based, and not a precipitation based mechanism.77
CAP of statistical non-random copolymers
The final class of copolymers we will discuss is statistical non-random copolymers, that is, the sequence order parameter χ is non-zero. In the extreme cases, χ = +1 reduces to a block copolymer and χ = −1 reduces to an alternating copolymer. How does the CAP of such non-random copolymers depend on the composition and sequence order parameter? Brun also discussed this situation. The conclusion is that one can still define an effective interaction parameter for such non-random statistical copolymers and the effective interaction parameter depends on the sequence order parameter χ in addition to the other four variables, εAw, εBw, fA and fB. The equation is rather complex and we refer the interested reader to the original references.73,78 However, one can gain a simple physical understanding by considering a statistical copolymer with a given fB but different sequence order. We again assume that only the B monomer interacts with the wall and that this interaction is attractive and can be tuned. The theory predicts that the more blocky the chain is, the smaller the εBw (less attractive) is needed to reach the CAP. In other words, a block AB copolymer would have the smallest εBw and an alternating AB copolymer would have the largest εBw at the CAP (see data shown in Fig. 5). The reason for this result is that an absorbed polymer chain forms trains on the surface. If the interactive B monomers are connected to each other, the polymer chain can more easily maximize enthalpic attraction to the surface.
Adsorption of statistical copolymers onto heterogeneous surfaces
The final situation we will discuss is the adsorption of statistical copolymers on heterogeneous surfaces. The pursuit of knowledge in this situation is no longer driven by chromatography separation, but rather is driven by curiosity of understanding molecular recognition or other innovative technology applications. Given a surface composed of two different sites arranged in a given surface pattern, can a heteropolymer consisting of A monomers that prefer one type of surface site and B monomers that prefer the other type of surface site recognize the surface pattern? There are a number of ways to investigate this problem. For example, one may design surfaces with regular patterns such as stripes or checker boards and find sequence of copolymers that are selectively adsorbed.30,79–82 One may also consider adsorption of statistical copolymers on surfaces that contains statistically correlated sites.83–87 These studies all suggest that there is a commensurability between heteropolymer sequence and distribution of surface sites. There are many interesting phenomena in this area of research and we will not go into details but refer interested readers to review articles.
By making use of our experience in determining the CAP, we considered and determined the CAP of statistical AB copolymers adsorbed on statistical heterogeneous surfaces.88,89 The surface contains two sites, a and b; A interacts with a with the same strength as B interacts with b, and all other interactions were zero. The statistical correlation of a and b sites on the surface can be controlled by a two-dimensional Ising model and one may use a surface order parameter Cs to describe the correlation, with Cs = −1 representing an alternating surface pattern and with Cs = +1 representing a patchy surface. One interesting thing that emerged from this study is that different types of AB copolymers with sequences ranging from blocky to statistically random to alternating have identical CAP above a random heterogeneous surface. However, when the heterogeneous surface becomes patchy, then statistically blocky AB copolymer will have the lowest CAP. Similarly, statistical alternating AB copolymer will have the lowest CAP on a statistically alternating surface. Later, Poloksy68 considered the adsorption of AB random copolymers onto heterogeneous surfaces using a two-dimensional partially directed walk and considered the impact of the surface order parameter Cs and the sequence order parameter Cp on the transition temperature. He showed that in the case considered by Ziebarth et al.88 using Monte Carlo simulation, the CAP (expressed as the inverse transition temperature) of a statistical copolymer is independent of sequence order parameter Cp if the surface is random (Cs = 0) (see Fig. 6), but the inverse transition temperature increases with Cp when Cs < 0 and decreases with Cp when Cs > 0, in agreement with Monte Carlo simulation data.
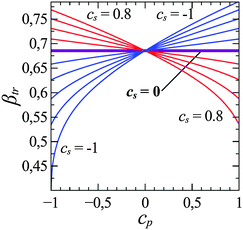 |
| Fig. 6 Inverse transition temperature of a statistical AB copolymer absorption above a statistical ab surface in the annealed approximation. Here eAa = eBb = −1; and eAb = eBa = 0, Cp and Cs are the order parameters for the polymer and surface respectively (reproduced from Fig. 3a from ref. 68). | |
Conclusions
The content of this review article is motivated by the experimental development of polymer liquid chromatography, especially the development of Liquid Chromatography at the Critical Condition (LCCC) and the application of LCCC to the separation and characterization of complex polymers. By making use of and fine tuning the surface interaction of polymers with the column substrate, experimentalists have been able to discern and analyse polymer samples that have chemical composition distribution or architectural distribution using LCCC. In these experiments, the knowledge of how complex polymers interact with porous substrates at or near the Critical Adsorption Point (CAP) of polymers are important to understand and interpret the experimental chromatography data.
The CAP of a linear polymer itself is a heavily investigated theoretical topic because it is an interesting tri-critical point in polymer adsorption. The link between the CAP understood in the context of polymer adsorption on a solid surface with the LCCC condition encountered in polymer liquid chromatography has some subtlety. Careful consideration of the excluded volume effect in polymer chains leads to the conclusion that true independence of partition coefficient on chain length does not exist. However, this does not preclude the experimental identification of the Critical Condition in actual polymer chromatography system. It is safe to say that LCCC operates at the CAP point of a homopolymer sample. Under this context, we discussed the partitioning of star, block and graft polymers at the CAP of one of the blocks. We have further discussed the CAP of adsorption of statistical copolymers on solid homogeneous and heterogeneous surfaces. We hope that these discussions motivate more theoretical and computational studies of complex polymers in porous media and also provide experimentalists better understanding of issues encountered when they characterize polymer samples using LCCC or other forms of interactive chromatography.
Acknowledgements
We thank financial support from ACS/PRF (PRF# 46933-AC7) and from National Science Foundation under Grant Number CHE-0724117 (co-funded by MPS/CHE and OISE).
Notes and references
- N. Hadjichristidis, M. Pitsikalis, S. Pispas and H. Iatrou, Chem. Rev., 2001, 101, 3747–3792 CrossRef CAS PubMed.
- R. K. Iha, K. L. Wooley, A. M. Nyström, D. J. Burke, M. J. Kade and C. J. Hawker, Chem. Rev., 2009, 109, 5620–5686 CrossRef CAS PubMed.
- H. Mori and A. H. Müller, Prog. Polym. Sci., 2003, 28, 1403–1439 CrossRef CAS.
- H. Pasch, Adv. Polym. Sci., 1997, 128, 1–45 CrossRef CAS.
- D. Berek, Prog. Polym. Sci., 2000, 25, 873–908 CrossRef CAS.
- T. Chang, Adv. Polym. Sci., 2003, 163, 1–60 CrossRef CAS.
- B. Trathnigg, Prog. Polym. Sci., 1995, 20, 615–650 CrossRef CAS.
- T. Macko, R. Brüll, Y. Zhu and Y. Wang, J. Sep. Sci., 2010, 33, 3446–3454 CrossRef CAS PubMed.
- W. Radke, J. Chromatogr. A, 2014, 1335, 62–79 CrossRef CAS PubMed.
- T. Chang, J. Polym. Sci., Part B: Polym. Phys., 2005, 43, 1591–1607 CrossRef CAS.
-
G. J. Fleer, M. A. Cohen Stuart, J. M. H. M. Scheutjens, T. Cosgrove and B. Vincent, Polymers at interfaces, Chapman & Hall, 1993 Search PubMed.
- T. M. Birshtein, Macromolecules, 1979, 12, 715–721 CrossRef CAS.
- P. G. de Gennes, Macromolecules, 1981, 14, 1637–1644 CrossRef CAS.
- E. Eisenriegler, K. Kremer and K. Binder, J. Chem. Phys., 1982, 77, 6296–6320 CrossRef CAS.
- J. M. Hammersley, G. M. Torrie and S. G. Whittington, J. Phys. A: Math. Gen., 1982, 15, 539–571 CrossRef CAS.
- A. A. Gorbunov, E. B. Zhulina and A. M. Skvortsov, Polymer, 1982, 23, 1133–1142 CrossRef CAS.
- Z.-G. Wang, A. M. Nemirovsky and K. F. Freed, J. Chem. Phys., 1987, 86, 4266 CrossRef CAS.
- A. V. Gorshkov, H. Much, H. Becker, H. Pasch, V. V. Evreinov and S. G. Entelis, J. Chromatogr. A, 1990, 523, 91–102 CrossRef CAS.
- H. Pasch, Polymer, 1993, 34, 4095–4099 CrossRef CAS.
- D. Berek, M. Janco and G. R. Meira, J. Polym. Sci., Part A: Polym. Chem., 1998, 36, 1363–1371 CrossRef CAS.
- A. M. Skvortsov and A. A. Gorbunov, J. Chromatogr., 1986, 358, 77–83 CrossRef CAS.
- P. G. De Gennes, Rep. Prog. Phys., 1969, 32, 187–205 Search PubMed.
- A. A. Gorbunov and A. M. Skvortsov, Adv. Colloid Interface Sci., 1995, 62, 31–108 CrossRef CAS.
- A. A. Gorbunov and A. V Vakhrushev, Polymer, 2009, 50, 2727–2735 CrossRef CAS.
- C. M. Guttman, E. A. Di Marzio and J. F. Douglas, Macromolecules, 1996, 29, 5723–5733 CrossRef CAS.
-
P.-G. de Gennes, Scaling Concepts in Polymer Physics, Cornell Univ. Press, 1979 Search PubMed.
- S. Metzger, M. Müller, K. Binder and J. Baschnagel, Macromol. Theory Simul., 2002, 11, 985–995 CrossRef CAS.
- P. Grassberger, J. Phys. A: Math. Gen., 2005, 38, 323–331 CrossRef CAS.
- A. Milchev, J. Phys.: Condens. Matter, 2011, 23, 103101 CrossRef PubMed.
- S. Bhattacharya, H.-P. Hsu, A. Milchev, V. G. Rostiashvili and T. A. Vilgis, Macromolecules, 2008, 41, 2920–2930 CrossRef CAS.
- A. Milchev and K. Binder, Macromolecules, 1996, 29, 343–354 CrossRef CAS.
- H. Meirovitch and S. Livne, J. Chem. Phys., 1988, 88, 4507 CrossRef CAS.
- S. Livne and H. Meirovitch, J. Chem. Phys., 1988, 88, 4498–4506 CrossRef CAS.
- R. Descas, J.-U. Sommer and A. Blumen, J. Chem. Phys., 2004, 120, 8831 CrossRef CAS PubMed.
- M.-B. Luo, J. Chem. Phys., 2008, 128, 044912 CrossRef PubMed.
- Y. Gong and Y. Wang, Macromolecules, 2002, 35, 7492–7498 CrossRef CAS.
- J. D. Ziebarth, Y. Wang, A. Polotsky and M. Luo, Macromolecules, 2007, 40, 3498–3504 CrossRef CAS.
- S. Orelli, W. Jiang and Y. Wang, Macromolecules, 2004, 37, 10073–10078 CrossRef CAS.
- S. Yang and A. V. Neimark, J. Chem. Phys., 2013, 138, 244903 CrossRef PubMed.
- R. Cimino, C. J. Rasmussen and A. V. Neimark, J. Chem. Phys., 2013, 139, 201101 CrossRef CAS PubMed.
- Y. Zhu, J. D. Ziebarth and Y. Wang, Polymer, 2011, 52, 3219–3225 CrossRef CAS.
- R. Brak, A. L. Owczarek, A. Rechnitzer and S. G. Whittington, J. Phys. A: Math. Gen., 2005, 38, 4309–4325 CrossRef.
- E. J. J. van Rensburg, E. Orlandini, A. L. Owczarek, A. Rechnitzer and S. G. Whittington, J. Phys. A: Math. Gen., 2005, 38, L823–L828 CrossRef.
- A. I. Abdulahad and C. Y. Ryu, J. Polym. Sci., Part B: Polym. Phys., 2009, 47, 2533–2540 CrossRef CAS.
- K. Baran, S. Laugier and H. Cramail, J. Chromatogr. B: Anal. Technol. Biomed. Life Sci., 2001, 753, 139–149 CrossRef CAS.
- F. Casassa and Y. Tagami, Macromolecules, 1969, 2, 14–26 CrossRef.
- I. Teraoka, Macromolecules, 2004, 37, 6632–6639 CrossRef CAS.
- K. Im, H.-W. W. Park, Y. Kim, S. Ahn, T. Chang, K. Lee, H.-J. J. Lee, J. Ziebarth and Y. Wang, Macromolecules, 2008, 41, 3375–3383 CrossRef CAS.
- Z. Chen and F. A. Escobedo, Phys. Rev. E: Stat., Nonlinear, Soft Matter Phys., 2004, 69, 021802 CrossRef PubMed.
- A. Y. Grosberg, Phys. Rev. Lett., 2000, 85, 3858–3861 CrossRef CAS PubMed.
- C. Micheletti and E. Orlandini, Macromolecules, 2012, 45, 2113–2121 CrossRef CAS.
- A. Narros, A. J. Moreno and C. N. Likos, Macromolecules, 2013, 46, 3654–3668 CrossRef CAS PubMed.
- J. Suzuki, A. Takano and Y. Matsushita, J. Chem. Phys., 2013, 139, 184904 CrossRef PubMed.
- A. Takano, Y. Ohta, K. Masuoka, K. Matsubara, T. Nakano, A. Hieno, M. Itakura, K. Takahashi, S. Kinugasa, D. Kawaguchi, Y. Takahashi and Y. Matsushita, Macromolecules, 2012, 45, 369–373 CrossRef CAS.
- B. Li, Z.-Y. Sun, L.-J. An and Z.-G. Wang, Macromolecules, 2015, 48, 8675–8680 CrossRef CAS.
- H. C. Lee, H. Lee, W. Lee, T. H. Chang and J. Roovers, Macromolecules, 2000, 33, 8119–8121 CrossRef CAS.
- W. Lee, H. H. C. Lee, D. Cho, T. Chang, A. A. Gorbunov and J. Roovers, Macromolecules, 2002, 35, 529–538 CrossRef CAS.
- H. Lee, W. Lee, T. Chang, S. Choi, D. Lee, H. Ji, W. K. Nonidez and J. W. Mays, Macromolecules, 1999, 32, 4143–4146 CrossRef CAS.
- H. Pasch, C. Brinkmann and Y. Gallot, Polymer, 1993, 34, 4100–4104 CrossRef CAS.
- H. Pasch, M. Augenstein and B. Trathnigg, Macromol. Chem. Phys., 1994, 195, 743–750 CrossRef CAS.
- W. Lee, D. Cho, T. Chang, K. J. Hanley and T. P. Lodge, Macromolecules, 2001, 34, 2353–2358 CrossRef CAS.
- S. Lee, H. Lee, L. Thieu, Y. Jeong, T. Chang, C. Fu, Y. Zhu and Y. Wang, Macromolecules, 2013, 46, 9114–9121 CrossRef CAS.
- S. Ahn, K. Im, T. Chang, P. Chambon and C. M. Fernyhough, Anal. Chem., 2011, 83, 4237–4242 CrossRef CAS PubMed.
- W. Jiang, S. Khan and Y. Wang, Macromolecules, 2005, 38, 7514–7520 CrossRef CAS.
- Y. Zhu, J. Ziebarth, C. Fu and Y. Wang, Polymer, 2015, 67, 47–54 CrossRef CAS.
- M. S. Moghaddam and S. G. Whittington, J. Phys. A: Math. Gen., 2002, 35, 33–42 CrossRef CAS.
- E. A. Di Marzio and C. M. Guttman, Macromolecules, 1995, 28, 2930–2937 CrossRef CAS.
- A. A. Polotsky, J. Phys. A: Math. Theor., 2012, 45, 425004 CrossRef CAS.
- A. Polotsky, A. Degenhard and F. Schmid, J. Chem. Phys., 2009, 131, 054903 CrossRef PubMed.
- K. Sumithra and A. Baumgaertner, J. Chem. Phys., 1999, 110, 2727–2731 CrossRef CAS.
- L. Gutman and A. K. Chakraborty, J. Chem. Phys., 1995, 103, 10733 CrossRef CAS.
- Y. Brun, J. Liq. Chromatogr. Relat. Technol., 1999, 22, 3067–3090 CrossRef CAS.
- Y. Brun, J. Liq. Chromatogr. Relat. Technol., 1999, 22, 3027–3065 CrossRef CAS.
- Y. Brun and P. Alden, J. Chromatogr. A, 2002, 966, 25–40 CrossRef CAS PubMed.
- S. Mori, Y. Uno and M. Suzuki, Anal. Chem., 1986, 58, 303–307 CrossRef CAS.
- S. Mori, Anal. Chem., 1988, 60, 1125–1128 CrossRef CAS.
- H. Sato, H. Takeuchi and Y. Tanaka, Macromolecules, 1986, 19, 2613–2617 CrossRef CAS.
- Y. Zhu, J. Ziebarth, T. Macko and Y. Wang, Macromolecules, 2010, 43, 5888–5895 CrossRef CAS.
- K. Sumithra and E. Straube, J. Chem. Phys., 2006, 125, 154701 CrossRef CAS PubMed.
- K. Sumithra, M. Brandau and E. Straube, J. Chem. Phys., 2009, 130, 234901 CrossRef CAS PubMed.
- A. Jayaraman, C. K. Hall and J. Genzer, Phys. Rev. Lett., 2005, 94, 078103 CrossRef PubMed.
- J. Genzer, Phys. Rev. E: Stat., Nonlinear, Soft Matter Phys., 2001, 63, 022601 CrossRef CAS PubMed.
- Y. A. Kriksin, P. G. Khalatur and A. R. Khokhlov, J. Chem. Phys., 2006, 124, 174904 CrossRef PubMed.
- S. Srebnik, A. K. Chakraborty and D. Bratko, J. Chem. Phys., 1998, 109, 6415 CrossRef CAS.
- D. Bratko, A. K. Chakraborty and E. I. Shakhnovich, Comput. Theor. Polym. Sci., 1998, 8, 113–126 CrossRef CAS.
- D. Bratko, A. K. Chakraborty and E. I. Shakhnovich, Chem. Phys. Lett., 1997, 280, 46–52 CrossRef CAS.
- G. K. Iliev, E. Orlandini and S. G. Whittington, J. Phys. A: Math. Theor., 2011, 44, 405004 CrossRef.
- J. D. Ziebarth, J. Williams and Y. Wang, Macromolecules, 2008, 41, 4929–4936 CrossRef CAS.
- B. Patel, J. D. Ziebarth and Y. Wang, Macromolecules, 2010, 43, 2069–2075 CrossRef CAS.
|
This journal is © The Royal Society of Chemistry 2016 |
Click here to see how this site uses Cookies. View our privacy policy here.