DOI:
10.1039/C5SM02844B
(Tutorial Review)
Soft Matter, 2016,
12, 4287-4294
Challenges in tissue engineering – towards cell control inside artificial scaffolds
Received
19th November 2015
, Accepted 15th April 2016
First published on 18th April 2016
Abstract
Control of living cells is vital for the survival of organisms. Each cell inside an organism is exposed to diverse external mechano-chemical cues, all coordinated in a spatio-temporal pattern triggering individual cell functions. This complex interplay between external chemical cues and mechanical 3D environments is translated into intracellular signaling loops. Here, we describe how external mechano-chemical cues control cell functions, especially cell migration, and influence intracellular information transport. In particular, this work focuses on the quantitative analysis of (1) intracellular vesicle transport to understand intracellular state changes in response to external cues, (2) cellular sensing of external chemotactic cues, and (3) the cells' ability to migrate in 3D structured environments, artificially fabricated to mimic the 3D environment of tissue in the human body.
1. Introduction
To ensure the viability of living cells, a great variety of biochemical and physical processes have to be coordinated.1 This sophisticated out-of-equilibrium system is orchestrated by complex interdependent processes within the extremely crowded and active cell interior. Chemical and physical signals in and outside of cells determine biological processes, such as cell migration and morphogenesis.2 Mechanisms of cell functions are based on highly organized intracellular structures and systems, ranging in size from the nano- to the micron-scale. These structures are intrinsically dynamic, exhibiting active and passive transport phenomena to pass on information between different regions inside the cell.3
While the genome provides the blueprint for all vital processes, the interaction of living cells with their environment determines cell functions. Not only molecular concentration gradients4 but also mechanical interactions with 3D scaffolds of the extracellular matrix determine functionality of living cells, such as adhesion, migration, proliferation and differentiation.5
The ability of cells to migrate in 3D environments under defined external cues (i.e. in the form of chemical or topographic gradients) in the host organism is essential for life. Malfunction of these processes often results in diseases in living organisms.
A new insight into disease mechanisms is of utmost importance to develop novel strategies for curing diseases like neuronal degeneracy,6,7 cancer,8 and inflammation9 or to identify the mechanistic origins of rare diseases. Additionally, this understanding will promote the development of innovative cell guiding strategies, e.g. for scaffold design in tissue engineering and innovative cell sorting techniques for diagnostic purposes.
This review summarizes studies that advance the abilities to control and analyse cellular functions using physical methods (Fig. 1), with a focus on amoeboid cells. Central questions are:
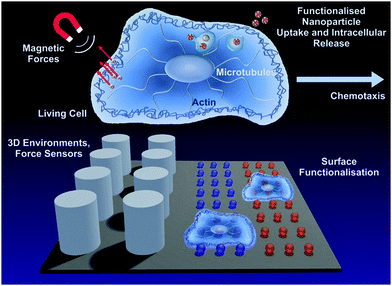 |
| Fig. 1 Schematic overview of experimental approaches to elucidate cell behaviour, signal processing and cell-substrate interaction. Intracellular information and material transport are probed by uptake and intracellular release of functionalised nanoparticles, in addition to magnetic force application. Cell-substrate interactions are controlled by cell type specific surface functionalisation. 3D environments are investigated and specifically adapted to match individual cell requirements for scaffold design, in addition to force sensors for the analysis of cellular pulling and pushing forces. | |
(1) How does the seemingly random noise pattern of intracellular transport reflect the plasticity of the cell interior? And how can this be analysed to extract relevant motion states?
(2) How is a living cell capable of precisely sensing chemotactic cues from the environment?
(3) How are active dynamic control mechanisms changing the global shape of the cell by microtubule-actin crosstalk to adapt to the 3D environment? And how do cells perform directed migration in 3D environments?
To answer these questions, it is essential to exert boundary conditions to living cells by applying external cues which keep key cell functions in a defined state. A controlled change of these predefined conditions induces state changes in living cells which can be quantified by extracting the relevant motion data. The following sections will display the importance of a mechanistic understanding of intracellular transport states (Section 2), chemotaxis (Section 3) and topographic cell guidance (Section 4) for the development of novel solutions for medical diagnosis and therapy. This systematic approach will facilitate the design of cell type specific 3D environments that allow for cell guidance and provide culture conditions similar to the cells' physiological environment.
2. Intracellular information and material transport
A living organism is a highly dynamic system that depends on the accurate interaction and performance of every subunit. In a densely crowded cell interior under non-equilibrium conditions, the transport of proteins, vesicles, and cellular building blocks is crucial for fast adaptation and for response mechanisms in reaction to extracellular cues. These cellular state changes are characterised by distinct transitions in intracellular transport processes. Extracting these state transitions from intracellular motion data will serve as a readout of cell response to mechanical or chemical cues and as a novel tool for the analysis of cell–material interactions.
To advance our understanding of the impact of cellular architecture on intracellular dynamics, several methods have been developed for automated, reliable and time-resolved identification of motility signatures of cytoplasmic tracers.1,10–15 Such approaches are both experimentally challenging and of fundamental importance. As an example, investigations on intracellular trajectories of beads moved by optical tweezers revealed anomalous diffusion behaviour depending on the particle diameter.11
In living cells, motile vesicles undergo two types of motion (Fig. 2): directed transport, driven by molecular motors on filaments, and thermal diffusion in the crowded, active medium.13,16–21 The combination of random and directed motion phases in the cytoplasm exhibit great advantages. Directed motion by bio-motors along microtubules (MT) rapidly transports vesicles between the periphery and the cell center.14,15 In contrast, random walks are very slow considering the high viscosity of the cytoplasm. This motion alone would explore the whole space but it would be too slow to ensure viability. The combination of these two motions facilitates very effective intracellular transport with fast-directed motion phases and diffusion, to ensure efficient search modes for chemical reactions to occur in the cell. This efficiency of combined directed and random motions in cells has also been predicted by theoretical studies.1,22
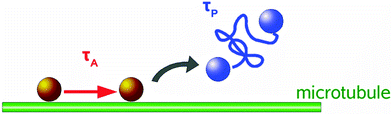 |
| Fig. 2 Two state distribution of tracer bead motion: State 1 – molecular motor associated motion along microtubules with attachment duration τA. State 2 – detachment from microtubule and diffusive motion of duration τP. | |
2.1. Two state analysis of intracellular transport
A basic and general analysis method to investigate intracellular transport motions is the mean squared displacement (MSD) method applied to trajectories of intracellular tracers.3 A more detailed local MSD method with directional persistence analysis of the tracer path distinguishes diffusive motion from directed transport along MTs, by reliably separating diffusive and directed motion phenomena of particles.13 To extract these two motion modes, particle motion is analysed in terms of a two-state motility model, yielding distributions of state durations as well as state parameters, i.e. velocities during directed motion phases and diffusion coefficients.
Experiments with colloidal probes engulfed by Dictyostelium discoideum cells exhibit the described two-state motion in the cytoplasmic space: random walks and directed motions with high velocities. Thus, transport of intracellular cargoes proceeds by successive phases of diffusion and directed motion. The calculated local velocities are best characterised in terms of very broad log-normal distributions comprising velocities over 3 orders of magnitude up to a few microns per second.13,23
The velocity distributions reveal subtle changes in the intracellular transport behaviour caused by modifications of the cytoskeleton composition due to mutations, hormones, or drugs.13,16,19 To identify the precise effect of different components of the cellular micro- and nanoarchitecture on vesicle transport, cytoskeleton parts were selectively disturbed by using depolymerising drugs. Examples are the decomposition of the MT network by benomyl and of the actin cortex by latrunculin A. In both cases, the fast motion states vanish. The removal of myosin II motors results in a decrease of the overall velocities corresponding to an apparent increase in the cytoplasm viscosity. Determined active state durations obey a decaying exponential distribution, whereas the durations of the random states follow log-normal distributions.24 The diffusion coefficients exhibit a broad log-normal distribution revealing that the random walks are not pure Brownian motions.20 Such behaviour is typical for diffusion in complex fluids with fractal dimensionality.23
2.2. Langevin model of intracellular subdiffusion
The subdiffusive regime is particularly interesting for inferring information about the dynamics of the cytoskeleton morphology and intracellular transport. To investigate the more complex intracellular transport processes leading to subdiffusion, all directed motion was excluded.16 Experimental results are compared to simulations of a data-driven Langevin model with finite correlations, capturing essential statistical features of the local MSD analysis.13,16,17 The statistics of this local MSD algorithm were calculated and a stochastic model of intracellular subdiffusion was developed for random motion with Gaussian velocity fluctuations, with a given correlation function.17 Thus, subdiffusive transport in D. discoideum cells can be described by Brownian motion with correlated Gaussian velocity fluctuations. In the experimental data, increments are Gaussian only to a certain approximation and no higher order or nonlinear correlations were incorporated in the model.16
Both microtubules and actin filaments act as the cause of intracellular subdiffusion.16 In general, microtubule sweeping motions liquefy the cytoplasm on all time scales, whereas actin-microtubule cross-talk generates a liquefying effect only at time scales longer than 0.2 s. The F-actin induced decrease in effective diffusion coefficients was revealed at all investigated time scales.16 Furthermore, intracellular motion in the sub-diffusive regime is qualitatively distinct from overdamped Brownian motion. Negative correlations between increments of motion generate this sub-diffusive behaviour. This suggests another class of Langevin-type models for the velocity, generating Gaussian fluctuations with correlations characteristic of an anti-persistent motion.17
2.3. Dimensionality in intracellular transport
To reduce the complexity of the 3D cell interior, a quasi-1D cell system was realised by special surface treatment, confining cell adhesion to pre-patterned quasi-1D surface lines.18,25 On these quasi-1D surface structures of 1 μm width, straight cellular outgrowth of up to several 100 μm was observed for PC12 cells.18 Quasi-1D intracellular transport phases of inserted fluorescent nanoparticles (NPs) were analysed by the two-state local MSD analysis as described in Section 2.1. Upon direct insertion of non-functionalised NPs into the cells, which could not bind to bio-motors for directed transport, sub-diffusive intracellular transport was predominant. However, this transport behaviour reverses completely towards directed transport when attachment to bio-motors is possible. Thus, tuning the attachment rate to intracellular bio-motors is a useful tool to control the efficiency of intracellular transport processes.18
To further investigate the aspects of dimensionality in intracellular transport, 3D trajectories of fluorescent NPs inside living cells were analysed by local MSD calculation and compared to their 2D projections.21 This way, the impact of each dimension for directed transport and diffusion was identified. Two cell types were compared: D. discoideum cells exhibiting a rather 3D morphology during adhesion and migration and HUH7 cells that are rather flat (2D-like) when adhered to a flat substrate. It was found that directed transport along microtubules is performed isotropically in D. discoideum cells. Here, the projection of the transport trajectories into 2D showed significant deviations from 3D directed transport behaviour. In contrast, the 2D projection of the directed phase trajectories did not result in a significant change in motion characteristics in the more 2D-organised HUH7 cells, where directed transport along MTs occurs predominantly in the 2D plane of adhesion. Diffusion coefficients obtained from 2D projections of the 3D tracks were underestimated in both cell types, proving that intracellular diffusion is a complex, anisotropic process in living cells with distinct 3D features.21
2.4. Force application inside living cells by magnetic tweezers
The micro-viscoelasticity of the intracellular space of D. discoideum cells was studied by evaluating the intracellular transport of magnetic force probes and their viscoelastic responses to force pulses in the pN to nN regime.24,26 Magnetic tweezer technology was applied by pulling super-paramagnetic micro- and nano-scaled particles inside cells via an inhomogeneous, external magnetic field.19,24,26
The robustness of intracellular transport processes can be correlated with the resistance against external force application. In general, it was found that nN forces exerted on magnetic beads attached to microtubules (MTs) are balanced by traction forces. Those arise at the MT ends coupled to the actin cortex and the microtubule organising centre (MTOC), respectively. Mechanical coupling between the MTs and the viscoelastic actin cortex provides cells with high mechanical stability despite the softness of the cytoplasm. Stronger external forces have to be balanced by the interplay of the traction forces in the MT network and the shear stress in the viscoelastic actin cortex.19
Therefore, the cytoplasmic space of cells is an active body that is mechanically stabilised by interactive crosstalk between the viscoelastic shell and the aster-like array of microtubules embedded in the viscoplastic cytoplasmatic space.26 External cues strongly influence these mechanisms and recent investigations showed that mechano-transduction also occurs in the nucleus and is therefore not only restricted to adhesion sites and cell surface receptors.27
3. Control of cell motion by spatio-temporally controlled external chemical stimuli
The prime example of complex intracellular feedback on fast time scales is chemotaxis, the capability of cells to sense,28,29 polarise30 and migrate towards an external chemical stimulus.4 In this orchestrated process, chemoattractants bind to cell surface receptors and induce cell polarisation and the subsequent generation of protrusions in the direction of the gradient (Fig. 3).31,32 Chemotaxis is essential for embryogenesis,33 neuron guidance,34 and inflammatory response,9 but also in pathologic aberrations like metastasis.8,35 Understanding the key mechanisms of chemotaxis will allow for active cell guidance. This will greatly facilitate artificial tissue formation in tissue engineering and novel approaches for regenerative medicine, e.g. by directing the migration of specific cell types towards defined regions inside 3D scaffolds. Additionally, the elucidation of chemotactic processes in metastasis might lead to the discovery of potential targets for tumour therapy.
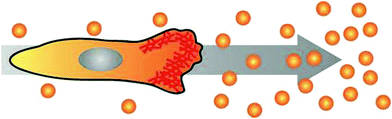 |
| Fig. 3 Cell polarising its actin cortex (red) towards the source of a chemical gradient and initiating directed migration. | |
Therefore, many recent developments in microfluidics aim at a better experimental setup to stimulate cells in highly controlled, spatio-temporally varying gradient fields. Micropipette36,37 and diffusion chamber38–40 assays are easily applicable methods to create chemical gradients, while for the generation of temporally stable gradients, chemical mixing in cascade microfluidic structures is applied experimentally.41–43 Y-chambers even add the advantage of temporal on/off-switching.44,45 For rapid chemotactic stimulation, photo-induced release of chemotactic agents was implemented.46–48 However, this technique leads to temporal and local inhomogeneities.
3.1. Cell motion analysis in alternating gradient fields
The creation of realistic artificial cell environments needs both aspects: stable gradients and fast changes in the gradient direction. This was first achieved by a complex valve-based approach examining migratory responses of neutrophils to fast gradient variations.42 An even higher stability accompanied by faster switching frequencies and a less complex setup is gained using a 3-inlet microfluidic gradient generator. This technique allows for the generation of highly stable, homogeneous gradients on large scales to address many cells in parallel.49 The cells sense chemotactic fields arising from two opposite directions, which can be switched on alternatingly at any frequency, to adapt to the exposure times the cells experience in the currently applied chemotactic field. Two fundamentally different cell repolarisation and migration types were identified during reorientation of D. discoideum cells towards the new gradient directions: repolarisation and U-turn behaviour. Even dynamical cell trapping was performed at high gradient switching frequencies. Here, the cells could not follow the changing stimulus and therefore were trapped.49 This can be used to probe the mechanistic time scales of the sensing abilities of cells.
Intracellular repolymerisation dynamics visualized by the GFP fusion protein LimEΔcc in D. discoideum cells has been quantified by a fluorescence dipole moment correlating the direction of the actin front to the gradient direction (Fig. 4). Repolymerisation behaviour involves reorganisation of the actin cortex towards the new migration direction and the establishment of a new migration front at the opposite end of the cell.49 The temporal evolution of this process is shown in Fig. 4.
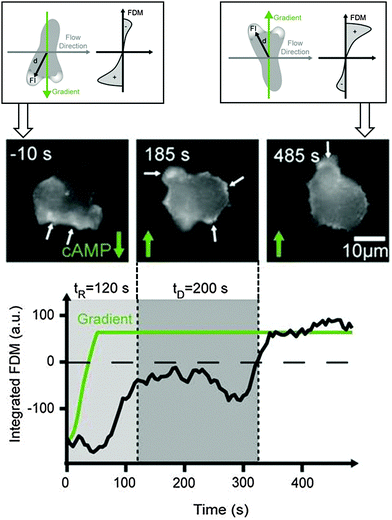 |
| Fig. 4 Schematic illustration of a cell polarising towards alternating gradient directions (top). Actin polymerisation is quantified by LimEΔcc GFP and the fluorescence dipole moment (FDM) quantifies the amount of fluorescence in the upper versus the lower hemisphere of the cell during this reorientation process (bottom). Cell polarisation (mid image gallery) and fluorescence dipole moment (black line in plot) following the gradient direction (green line in plot) [according to data from ref. 49]. | |
Future experiments need to combine these highly stable but temporarily variable gradient fields with further external stimuli and internal cell readouts to clarify the still remaining questions regarding chemotactically induced movement.
3.2. Influcence of cell shape on chemotaxis
Amoeboid cell motion in general occurs by cyclic formation of protrusions (pseudopods) at the cell front, followed by active retraction of the cell rear.50 Cell shape changes have been investigated in more detail to extract optimal combinations of cell shape changes and migration behaviour for the sensing of chemotactic fields at the sensing limit.51,52 Results show that D. discoideum cells are capable of chemotaxis at the fundamental limit of gradient sensing, as predicted by a static absorber model.53 This model shows that an amoeboid cell exhibits the same average sensing precision as a spherical object binding the chemoattractant permanently to its surface. Additional insight was gained about a windshield effect produced by the flow of a chemotactic medium across a cell. This effect interferes with accurate chemotaxis, but cells are capable of compensating for this effect, if it is caused by their own motion.53 The highly correlated shapes and motion behaviours of amoeba are strategies for coping with such motion induced gradients at a low signal-to-noise-ratio in chemotaxis.54 It was found that sideway sensing strategies fit with experimentally observed pseudopod splitting and zig-zag movement up the gradient.51 Furthermore, a stopping and sensing strategy was revealed, exhibiting reduced speed accompanied by cell shape oscillations.55 A third strategy would be the compensation by chemoattractant secretion at the rear of the cell, which D. discoideum is known to do. Since similar cell behaviour is observed in a diverse range of cell types,51 these could be conserved traits in evolution and could be used for medical applications, e.g. for guiding the migration of cell ensembles or immune cells by utilizing cell–cell signalling.
4. Cellular adhesion and motion control in predefined quasi-3D environments
In the absence of external cues, motile amoeboid cells migrate by the formation of stochastically generated pseudopods. The current model assumes two alternating motility modes: a random probing mode and a directed, fast migration mode.56 This efficient “search strategy” is highly dynamic and can be characterised by distinct differences in the protrusion frequency and angle distribution of successive turns in the migration direction.55 Cell velocity and shape analysis during these random migration phases led to simple models of amoeboid random walk.57 In the last decade, a refined view emerged considering correlations between the cellular orientation and the migration direction.58–61 In the presence of mechanical or chemical cues, the protrusions can be stabilized and trigger directed migration by cell polarisation.62 This specific type of cell migration is observed in several eukaryotic cell types, e.g. stem cells, specific immune cells or metastatic tumour cells,63 which can travel long distances inside the complex tissues of the human body to reach their point of destination.64 Thus, a profound understanding of the mechanisms governing amoeboid migration in 3D environments will facilitate novel approaches in diagnostics and therapy. It will give rise to the development of novel drugs in cancer therapy, targeting specific cytoskeletal parts or intracellular proteins that have been shown to promote tumour metastasis. Furthermore, the abilities for cell guidance by topographic cues will be of utmost importance for the 3D distribution of cells inside artificial scaffolds in tissue engineering.
4.1. Cell-substrate interactions in amoeboid migration
Spontaneous generation of pseudopods at random sites is a basic activity of vital cells and can be found in homogeneous cell migration environments lacking topographic and chemotactic stimuli. D. discoideum cells perform a special kind of random motion consisting of zig-zag-like motions over distances of about 20 μm and a subsequent change in the direction.23,55,65 This motion behaviour is correlated with the dynamics of pseudopod spreading. Pseudopods protrude constantly for several microns and stop abruptly. Then, the cell rear is retracted by unbinding from the substrate, decreasing the contact area. Subsequently, migration in a new direction is induced. Hence, cell motion is characterised by a concomitant cyclic variation of the contact area.23
The influence of surface structure and chemistry on this type of cell dynamics in D. discoideum cells during adhesion has been investigated quantitatively for different actin manipulating proteins by tracking the cell adhesion area and the protein distribution during the adhesion process.61–66 The analysis of the gain and loss of contact area revealed fluctuations in forces of protrusion and retraction that prevent D. discoideum cells from approaching a steady-state of interaction with a substrate. In conclusion, non-monotonic cell spreading is induced by spatio-temporal patterns resulting from the interplay of motor proteins and regulatory proteins, either promoting or terminating the polymerisation of actin.61
The importance of substrate adhesion and interaction was stressed by investigations on the size and number of actin foci in D. discoideum, where a negative correlation between the actin foci number and the cell velocity was found.67 Thus, amoeboid cell motility strongly depends on the interaction with the substrate. This is a very important principle for future studies and potential applications, as similar correlations between focal adhesion dynamics and cell motility have been found for other types of cell migration.68
4.2. Influence of quasi-3D structures on amoeboid cell motility
3D structures influence adhesion and migration behaviour of living cells by inducing local and global changes in cellular morphology and protein expression.69–71
To analyse differences in cell migration in 3D structured environments versus on flat substrates (2D), the motion behaviour of D. discoideum cells has been investigated in micro-pillar arrays with defined geometry and density.56,72 Results elucidate that microstructures on surfaces are not sensed as simple obstacles, leading to a deflection of the cell path, but can trap cells in contact with these structures and stop cell motion. This dynamic cell trapping effect depends on the cell's initial motility mode, which is enhanced with increasing number of surface structures the cell is in contact with at a given time (Fig. 5).56,72
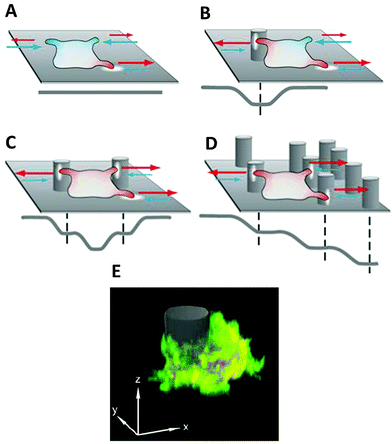 |
| Fig. 5 Schematic illustration of cell behaviour on differently structured substrates, characterised by protrusion spreading (red) and retraction (blue), where extracellular obstacles can be described as potential wells mediating cellular motion processes; the resulting potential level is displayed in grey: (A) random protrusion formation on flat substrates, (B) protrusion stabilisation and subsequent adhesion to the additional surface contact with 3D structures, (C) dynamic cell trapping effect by adhesion to multiple 3D structures in the absence of other structures in the cell vicinity, (D) topographic guidance effect by a gradual increase of the adhesion surface, (E) fluorescent microscopy image of a D. discoideum cell expressing LimEΔcc RFP and Arp2/3-GFP while adhering to a pillar structure. | |
Additionally, switching from a randomly formed pseudopod (random motility mode) into a stabilised pseudopod (directed migration) is enhanced by surface contact, meaning that cells migrate by maximising contact with available surface structures. However, cells lacking microtubules do not show pronounced attraction to surface pillars, leading to the conclusion that microtubules mediate cellular interaction with surface structures.56 The general dependence of focal adhesion build-up and disassembly on microtubules has also been observed in fibroblasts73,74 and proves the relevance of the principles derived from investigations on amoeboid cells.
Further investigations revealed that the spatial density of the quasi-3D environment has a distinct effect on cell migration, e.g. the number of directed pillar-to-pillar runs is increased by higher pillar densities, triggering cell polarisation.56,72 A long-term shift of cell migration towards regions with pillar distances in the range of the cell diameter was observed. This effect is based on directed migration from low density regions and a contrary passive drift from high density regions where cells are spatially confined.60 This “topophoresis” effect opens the possibility of guiding or sorting different cell types according to the spatial geometry and density of the 3D environment. Additionally, in D. discoideum cells exposed to traction forces by 3D spatial confinement, a transition from actin-driven pseudopod formation towards bleb-driven motility can be observed.37 Interestingly, the migration of mesenchymal-like cells shows a plasticity depending on the adhesion to the substrate and spatial confinement. They exhibited a tendency to perform amoeboid-like migration for low substrate adhesion and spatial confinement inside artificial environments.75 This finding underlines the importance of amoeboid migration for the development of novel materials for cell guidance in tissue engineering.
5. Outlook
This review summarizes recent research on the influence of chemical and mechanical stimuli on cell behaviour and the underlying processes, with a focus on amoeboid migration. The survival of cells in organisms depends on their ability to respond to external cues with changes in their intracellular signalling pathways. The extraction of these state changes from intracellular motion data will serve as a novel readout tool for the precise analysis of cell interactions with materials and active agents, e.g. for more efficient screening assays in drug development.
Future research should aim at advancing the mechanistic understanding of the complex interplay of extracellular cues and intracellular states in setups combining mechanical and chemical stimuli in 3D, successively approaching physiological conditions. The systematic analysis and elucidation of these interactions will greatly facilitate the buildup of a toolbox for the design of cell-type specific surface structures and 3D scaffolds and initiate novel approaches in biomedical research. The crucial step here is to optimize the combination of stimuli for manipulating cell functions with bioactive materials, ranging in size from the micron to the nanoscale. Mechanical stimuli will be provided by 3D scaffolds to promote or hinder directed cell migration, guiding cells to desired destinations and providing ideal conditions for specific cell types. To enhance these effects, local chemotactic gradients will be generated inside the scaffolds by incorporated nanocarriers, altering the cytoskeleton or triggering local changes in cell functions.
The presented approach mimics the amount of information a cell has to compute in vivo and will elucidate the decision making process in living cells. This will be greatly facilitated by computational studies, helping to find consistent biophysical models for cellular behaviour.76,77 In a greater scheme, this will allow for the design of more realistic drug screening assays, organs-on-a-chip, bioactive scaffolds and open up new prospects for applications in tissue engineering and regenerative medicine.
Acknowledgements
Funding by DFG grant HE 5958/2-1 and Volkswagen Foundation grant 85/100 is acknowledged for this work.
References
- C. Loverdo, O. Benichou, M. Moreau and R. Voituriez, Nat. Phys., 2008, 4, 134–137 CrossRef CAS.
- T. Pollard and G. Borisy, Cell, 2003, 112, 453–465 CrossRef CAS PubMed.
- A. Caspi, R. Granek and M. Elbaum, Phys. Rev. Lett., 2000, 85, 5655–5658 CrossRef CAS PubMed.
- C. A. Parent and P. N. Devreotes, Science, 1999, 284, 765–770 CrossRef CAS PubMed.
- D. A. Fletcher and R. D. Mullins, Nature, 2010, 463, 485–492 CrossRef CAS PubMed.
- T. L. Williamson and D. W. Cleveland, Nat. Neurosci., 1999, 2, 50–56 CrossRef CAS PubMed.
- M. Hafezparast,
et al.
, Science, 2003, 300, 808–812 CrossRef CAS PubMed.
- L. Coultas, K. Chawengsaksophak and J. Rossant, Nature, 2005, 438, 937–945 CrossRef CAS PubMed.
- P. Martin and S. J. Leibovich, Trends Cell Biol., 2005, 15, 599–607 CrossRef CAS PubMed.
- C. Brangwynne, G. Koenderink, F. MacKintosh and D. A. Weitz, Trends Cell Biol., 2009, 19(9), 423–427 CrossRef CAS PubMed.
- A. Caspi, R. Granek and M. Elbaum, Phys. Rev. E: Stat., Nonlinear, Soft Matter Phys., 2002, 66(1), 011916 CrossRef PubMed.
- V. Levi and E. Gratton, Cell Biochem. Biophys., 2007, 48(1), 1–15 CrossRef CAS PubMed.
- D. Arcizet, B. Meier, E. Sackmann, J. O. Rädler and D. Heinrich, Phys. Rev. Lett., 2008, 101, 248103 CrossRef PubMed.
- M. A. Welte, Curr. Biol., 2004, 14(13), R525–R537 CrossRef CAS PubMed.
- H. Salman, Y. Gil, R. Granek and M. Elbaum, Chem. Phys., 2002, 284(1–2), 389–397 CrossRef CAS.
- M. Otten, A. Nandi, D. Arcizet, M. Gorelashvili, B. Lindner and D. Heinrich, Biophys. J., 2012, 102, 758–767 CrossRef CAS PubMed.
- A. Nandi, D. Heinrich and B. Lindner, Phys. Rev. E: Stat., Nonlinear, Soft Matter Phys., 2012, 86, 021926 CrossRef PubMed.
- C. Pelzl, D. Arcizet, G. Piontek, J. Schlegel and D. Heinrich, ChemPhysChem, 2009, 10, 2884 CrossRef CAS PubMed.
- J. Mahowald, D. Arcizet and D. Heinrich, ChemPhysChem, 2009, 10, 1559 CrossRef CAS PubMed.
- M. Götz, K.-F. Hodeck, P. Witzel, A. Nandi, B. Lindner and D. Heinrich, Eur. Phys. J.: Spec. Top., 2015, 224, 1169–1183 CrossRef.
- A. Dupont, M. Gorelashvili, V. Schüller, F. Wehnekamp, D. Arcizet, Y. Katayama, D. C. Lamb and D. Heinrich, New J. Phys., 2013, 15, 7 CrossRef.
- S. Klumpp and R. Lipowsky, Phys. Rev. Lett., 2005, 95, 268102 CrossRef PubMed.
- E. Sackmann, F. Keber and D. Heinrich, Annu. Rev. Condens. Matter Phys., 2010, 1, 257–276 CrossRef CAS.
- D. Heinrich and E. Sackmann, Acta Biomater., 2006, 2, 619–631 CrossRef PubMed.
- S. Gunawardena, L. S. Her, R. G. Brusch, R. A. Laymon, I. R. Niesman, B. Gordesky- Gold, L. Sintasath, N. M. Bonini and L. S. B. Goldstein, Neuron, 2003, 40, 25–40 CrossRef CAS PubMed.
-
E. Sackmann, A. Reuther and D. Heinrich, Micromechanics of Cells: Viscoelastic Microscopy of Cells, Mechanics of the 21st Century, ed. W. Gutkovski and T. Kowalevski, Springer Verlag, Dordrecht, Netherlands, 2005, pp. 313–328, ISBN-10 1-4020-3456-3 Search PubMed.
- C. Guilluy, L. D. Osborne, L. Van Landeghem, L. Sharek, R. Superfine, R. Garcia-Mata and K. Burridge, Nat. Cell Biol., 2014, 16(4), 376–381 CrossRef CAS PubMed.
- C. A. Parent and P. N. Devreotes, Science, 1999, 284, 765–770 CrossRef CAS PubMed.
- C. Janetopoulos and R. A. Firtel, FEBS Lett., 2008, 582, 2075–2085 CrossRef CAS PubMed.
- O. D. Weiner, Curr. Opin. Cell Biol., 2002, 14, 196–202 CrossRef CAS PubMed.
- L. Boosgraf and P. J. N. van Haastert, PLoS One, 2009, 4(8), e6842 Search PubMed.
- D. R. Soll, D. Wessels, P. J. Heid and H. Zhang, J. Muscle Res. Cell Motil., 2002, 23, 659–672 CrossRef CAS PubMed.
- G. Reig, E. Pulgar and M. L. Concha, Development, 2014, 141(10), 1999–2013 CrossRef CAS PubMed.
- H. Song and M. Poo, Nat. Cell Biol., 2001, 3, E81–E88 CrossRef CAS PubMed.
- A. Müller, B. Homey, H. Soto, N. Ge, D. Catron, M. E. Buchanan, T. McClanahan, E. Murphy, W. Yuan, S. N. Wagner, J. L. Barrera, A. Mohar, E. Verástegui and A. Zlotnik, Nature, 2001, 410, 50–56 CrossRef PubMed.
- R. P. Futrelle, J. Cell. Biochem., 1982, 18, 197–212 CrossRef CAS PubMed.
- E. Zatulovsky, R. Tyson, T. Bretschneider and R. R. Kay, J. Cell Biol., 2014, 204(6), 1027–1044 Search PubMed.
- D. Zicha, G. A. Dunn and A. F. Brown, J. Cell Sci., 1991, 99, 769–775 Search PubMed.
- S. Boyden, J. Exp. Med., 1962, 115, 453–466 CrossRef CAS PubMed.
- S. García, R. Sunyer, A. Olivares, J. Noailly, J. Atencia and X. Trepat, Lab Chip, 2015, 15, 2606–2614 RSC.
- S. K. W. Dertinger, D. T. Chiu, N. L. Jeon and G. M. Whitesides, Anal. Chem., 2001, 73, 1240–1246 CrossRef CAS.
- D. Irimia, S. Y. Liu, W. G. Tharp, A. Samandani, M. Toner and M. C. Poznansky, Lab Chip, 2006, 6(2), 191–198 RSC.
- L. Song, H. U. Bödeker, C. Beta, A. Bae, C. Franck, W.-J. Rappel, W. F. Loomis and E. Bodenschatz, Eur. J. Cell Biol., 2006, 85, 981–989 CrossRef CAS PubMed.
- F. Lin and E. C. Butcher, Lab Chip, 2006, 6, 1462–1469 RSC.
- F. Lin, C. M.-C. Nguyen, S.-J. Wang, W. Saadi, S. P. Gross and N. L. Jeon, Ann. Biomed. Eng., 2005, 33(4), 475–482 CrossRef PubMed.
- C. Beta, D. Wyatt, W. Rappel and E. Bodenschatz, Anal. Chem., 2007, 79, 3940–3944 CrossRef CAS PubMed.
- B. Kuczenski, W. C. Ruder, W. C. Messner and P. R. LeDuc, PLoS One, 2009, 4(3), e4847 Search PubMed.
- H. Kress,
et al.
, Nat. Methods, 2009, 6, 905–909 CrossRef CAS PubMed.
- B. Meier, A. Zielinski, C. Weber, D. Arcizet, S. Youssef, T. Franosch, J. O. Rädler and D. Heinrich, Proc. Natl. Acad. Sci. U. S. A., 2011, 108(28), 11417–11422 CrossRef CAS PubMed.
- A. J. Ridley, M. A. Schwartz, K. Burridge, R. A. Firtel, M. H. Ginsberg, G. Borisy, J. T. Parsons and A. R. Horwitz, Science, 2003, 302, 1704–1709 CrossRef CAS PubMed.
- L. Tweedy, B. Meier, J. Stephan, D. Heinrich and R. G. Endres, Sci. Rep., 2013, 3, 2606 Search PubMed.
- P. J. M. van Haastert and M. Postma, Biophys. J., 2007, 93, 1787–1796 CrossRef CAS PubMed.
- R. G. Endres and N. S. Wingreen, Proc. Natl. Acad. Sci. U. S. A., 2008, 105, 15749–15754 CrossRef CAS PubMed.
- Y. Maeda, J. Inose, M. Matsuo, S. Iwaya and M. Sano, PLoS One, 2008, 3, e3734 Search PubMed.
- L. Li, S. F. Nørrelykke and E. C. Cox, PLoS One, 2008, 3, e2093 Search PubMed.
- D. Arcizet, S. Capito, S. Youssef, C. Leonhardt and D. Heinrich, Soft Matter, 2012, 8(5), 1473–1481 RSC.
- P. J. M. van Haastert, PLoS Comput. Biol., 2010, 6(8), e1000874 Search PubMed.
- R. Kemkemer, S. Jungbauer, D. Kaufmann and H. Gruler, Biophys. J., 2006, 90, 4701–4711 CrossRef CAS PubMed.
- J. Kaiser, A. Reinmann and A. Bruinink, Biomaterials, 2006, 27, 5230–5241 CrossRef CAS PubMed.
- J. C. Del Alamo, R. Meili, B. Alonso-Latorre, J. Rodriguez-Rodriguez, A. Aliseda, R. A. Firtel and J. C. Lasheras, Proc. Natl. Acad. Sci. U. S. A., 2007, 104, 13343–13348 CrossRef CAS PubMed.
- D. Heinrich, S. Youssef, B. Schroth-Diez, U. Engel, D. Aydin, J. Blümmel, J. Spatz and G. Gerisch, Cell Adhes. Migr., 2008, 2(2), 58–68 CrossRef.
- I. R. Nabi, J. Cell Sci., 1999, 112, 1803–1811 CAS.
- P. Friedl, Curr. Opin. Cell Biol., 2004, 16, 14–23 CrossRef CAS PubMed.
- G. de la Rosa, N. Longo, J. L. Rodríguez-Fernández, a. Puig-Kroger, A. Pineda, Á. L. Corbí and P. Sánchez-Mateos, J. Leukocyte Biol., 2003, 73, 639–649 CrossRef CAS PubMed.
- M. Schindl, B. Wallraff, B. Deubzer, W. Witke, G. Gerisch and E. Sackmann, Biophys. J., 1995, 68, 1177–1190 CrossRef CAS PubMed.
- I. Weber, E. Wallraff and R. Albrecht. G. Gerisch, J. Cell Sci., 1995, 108, 1519–1530 CAS.
- K. S. K. Uchida and S. Yumura, J. Cell Sci., 2003, 117, 1443–1455 CrossRef PubMed.
- D. H. Kim and D. Wirtz, FASEB J., 2013, 27(4), 1351–1361 CrossRef CAS PubMed.
- S. Grebhi, D. W. Hamilton, J. D. Waterfield and D. M. Brunette, J. Biomed. Mater. Res., Part A, 2013, 101(7), 2118–2128 Search PubMed.
- G. Charras and E. Sahai, Nat. Rev. Mol. Cell Biol., 2014, 15, 813–824 CrossRef CAS PubMed.
- E. Cukierman, R. Pankov, D. R. Stevens and K. M. Yamada, Science, 2001, 294, 1708–1712 CrossRef CAS PubMed.
- M. Gorelashvili, M. Emmert, K.-F. Hodeck and D. Heinrich, New J. Phys., 2004, 16, 075012 CrossRef.
- E. J. Ezratty, M. A. Partridge and G. G. Gundersen, Nat. Cell Biol., 2005, 7(6), 581–590 CrossRef CAS PubMed.
- O. Krylyshkina, K. I. Anderson, I. Kaverina, I. Upmann, D. J. Manstein, J. V. Small and D. K. Toomre, J. Cell Biol., 2003, 161(5), 853–859 CrossRef CAS PubMed.
- Y.-J. Liu, M. Le Berre, F. Lautenschlaeger, P. Maiuri, A. Callan-Jones, M. Heuzé, T. Takaki, R. Voituriez and M. Piel, Cell, 2015, 160, 659–672 CrossRef CAS PubMed.
- I. Hecht, H. Levine, W.-J. Rappel and E. Ben-Jacob, PLoS One, 2011, 6(8), e21955 CAS.
- I. Niculescu, J. Textor and R. J. de Boer, PLoS Comput. Biol., 2015, 11(10), e1004280 Search PubMed.
|
This journal is © The Royal Society of Chemistry 2016 |
Click here to see how this site uses Cookies. View our privacy policy here.