Online quantitative mass spectrometry for the rapid adaptive optimisation of automated flow reactors†
Received
20th October 2015
, Accepted 25th November 2015
First published on 1st December 2015
Abstract
An automated continuous reactor for the synthesis of organic compounds, which uses online mass spectrometry (MS) for reaction monitoring and product quantification, is presented. Quantitative and rapid MS monitoring was developed and calibrated using HPLC. The amidation of methyl nicotinate with aqueous MeNH2 was optimised using design of experiments and a self-optimisation algorithm approach to produce >93% yield.
Introduction
Flow reactors are increasing in popularity for the synthesis of organic compounds. Their advantages over batch reactors include higher reproducibility; safer operating conditions (particularly at increased temperatures and pressures); ease of automation; and facile integration of analysis. Therefore great success has been achieved for the optimisation of chemical systems using continuous reactors.1 Automated flow reactors typically combine online analysis with a feedback loop or PC interface to carry out reactions without any further human interference.2 This technology has been used for the optimisation of reactions using evolutionary algorithms (self-optimisation),3–8 design of experiments (DoE)9,10 and kinetic parameters.10,11
Process analytical technologies (PAT) for automated flow reactors include UV-vis,3 IR,6,8,11,12 Raman13 and NMR spectroscopy;7 gas chromatography5 and high performance liquid chromatography (HPLC).4 Spectroscopy benefits from rapid analytical method times, which can be used as real-time feedback to assess the steady state of a continuous reactor.6 However, vibrational spectroscopy generates complex spectra, which may require extensive deconvolution, and can be difficult to calibrate for multi-component systems. NMR spectroscopy is typically easier to analyse and provides more structural information than IR. The resolution and sensitivity of miniaturized low-field bench-top NMR spectrometers, which due to their small size can be used for inline analysis, means that subtle chemical transformations may not be detected and accurate quantification of low level impurities may prove difficult.7 Chromatography generates data that is easy to analyse and can provide structural information if combined with mass spectrometry (MS) detection. However the long method times significantly decrease throughput.
To overcome the issues in analysis duration, demanding calibration and sensitivity in these PAT techniques, in this communication we explore the use of online MS to enable rapid quantification (<1 min analysis duration). Online MS has been used to qualitatively monitor continuous reactors for the identification of compounds and intermediates14 or analysis of relative composition.15 MS can provide structural information and product composition, all in real-time due to its short method times. Therefore it could be the ideal analytical technique for optimising an automated flow reactor as it can determine steady state and then calculate a product yield with minimal data manipulation.
This hypothesis was tested by carrying out a self-optimisation and DoE, to optimise the synthesis of N′-methyl nicotinamide 2 by reacting methyl nicotinate 1 with aqueous methylamine in methanol (Scheme 1). 1 can also hydrolyse to form niacin 3. This reaction was selected due to the presence of an easily ionisable pyridine nitrogen, loss of selectivity due to the presence of water in the aqueous methylamine and the requirement of high loadings of methylamine which may cause suppression effects. Overcoming such suppression effects is an important factor if direct MS is used for quantitative analysis.
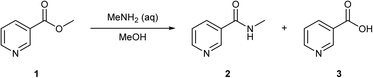 |
| Scheme 1 The reaction of methyl nicotinate 1 with aqueous methylamine to form the desired N′-methyl nicotinamide 2 and the impurity niacin 3. | |
Results and discussion
Reactor set-up
Methyl nicotinate 1, and methylamine solutions were pumped using dual piston LC pumps, with an additional pump of solvent to clean the reactor between experiments and prevent accumulation of analyte in the mass spectrometer. Reagent feeds were mixed in tee-pieces before entering a tubular reactor (Cambridge Reactor Design, Polar Bear Plus Flow Synthesizer) with active heating and cooling, significantly reducing the time required to perform subsequent experiments at different temperatures. Upon exiting the reactor, aliquots of reaction mixture were introduced to the mobile phase of the MS using a 4 port microvolume (0.06 μL) sample valve. The reactor was maintained under fixed pressure using a back pressure regulator. Pump flow rates; reactor temperature and sample intervals were controlled by a custom written MatLab program, see Fig. 1.
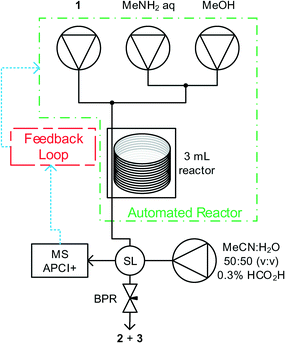 |
| Fig. 1 Reactor set-up. Reagents were pumped using Jasco PU980 pumps and were mixed in Swagelok tee-pieces. A Polar Bear Plus Flow Synthesizer was used for heating and cooling of the tubular reactor. Aliquots of reaction mixture were delivered to the MS mobile phase using a VICI Valco 4 port sample loop (SL). The reaction was maintained under fixed back pressure using an Upchurch Scientific back pressure regulator (BPR). PTFE tubing (1/16′′ OD, 1/32′′ ID) provided by Polyflon was used throughout the reactor. Swagelok unions and fittings were used throughout apart from the sample loop (VICI) and BPR (Upchurch). An Agilent 1100 G1311A quaternary pump provided the mobile phase to the Advion Expression CMS. The automated reactor was controlled by a custom written MatLab program. | |
Existing quantitative techniques have used specialist spectrometers.16 This work uses a bench-top spectrometer, which is cheaper, more flexible and easier to operate for a non-specialist. The spectrometer used was an Advion Expression CMS operating in positive atmospheric pressure chemical ionisation mode (APCI). APCI was selected over electrospray ionisation (ESI) due to a reduction in baseline noise and being able to handle a larger mobile phase flow rate.
The yield of each component was calculated by internal normalization of the [M + H]+ adducts. The internally normalized areas were corrected for the isotope abundance as the [M + 1 + H]+ isotope of 2 could be confused with the [M + H]+ adduct of 1. Calibration curves for 1 to 3 were calculated for HPLC and it was possible to quantify accurately the MS to the calibrated HPLC using experiments in a central composite face centred (CCF) plot, with very good fit (R2 0.997 – see ESI† for full details of calibration).
Self-optimisation
Fully automated optimisations were carried out using the SNOBFIT algorithm and a DoE statistical design (see ESI† for full details) using the reactor in Fig. 1 and the boundary limits shown in Table 1. SNOBFIT is a branch and fit algorithm that fits polynomials to experimental points and can find multiple optima.17 For each experiment, the reactor is set to the desired temperature and methanol is pumped at 0.5 mL min−1 and the other pumps at 0.02 mL min−1 to minimise reagent usage during temperature changes. When the reactor reaches the set temperature the reagent pumps are set to their desired flow rates and allowed to pump for 1.1 residence times. During this time, the MS is directly sampled at 40 s intervals using a 5
:
2 flow splitter to further reduce sample concentration. We believe that the nanolitre injection volumes, combined with the flow splitter and APCI ionisation technique reduce the sample concentration within the MS detector to the linear range allowing accurate quantification. After 1.1 reactor volumes of fluid are pumped, a steady state function monitors the last three samples and when variation of the % yield of 2 was less than a deviation of ±0.75% the system is deemed to be at steady state. The composition of the fluid is then recorded and the next experiment conditions are set and the process above repeated. Detection of steady state with near real-time monitoring reduces material usage and more accurate quantification than single data point analysis.
Table 1 Optimisation limits for the self-optimisation and DoE. Ester 1 concentration 1.46 mol L−1, MeNH2 concentration 5.77 mol L−1
Limit |
Ester 1 flow rate (mL min−1) |
MeNH2 molar eq. |
Temperature (°C) |
Lower |
0.100 |
1.0 |
0.0 |
Upper |
0.400 |
10 |
130.0 |
The change in the responses of 1–3 for the first 4 experiments in the self-optimisation is shown in Fig. 2. Optimum conditions were reached in 21 experiments, which corresponded to less than 12 hours of experiment time. The optimum conditions generate 2 in 93% yield (ester 1 flow rate 0.1 mL min−1, MeNH2 10 eq., 10.6 °C, Fig. 3).
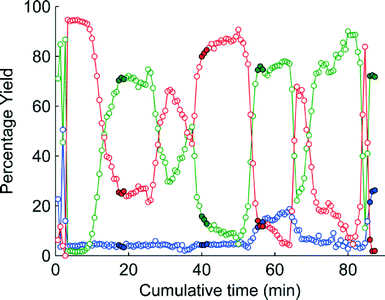 |
| Fig. 2 MS plot for the first 4 experiments in the self-optimisation where red is 1, green is 2 and blue is 3. The filled points show the last three points where steady state was reached. | |
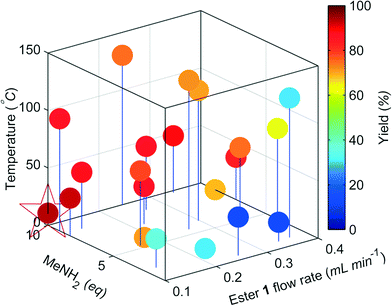 |
| Fig. 3 Optimisation plot for the SNOBFIT self-optimisation of amide 2. Optimum point highlighted by the star, ester 1 flow rate 0.1 mL min−1, MeNH2 10 eq., 10.6 °C. | |
Design of experiments
A DoE statistical design was constructed using a CCF design, which enables curvature of the response surface to be modelled statistically. The reaction conditions were ranked into blocks of ascending temperature and then randomised within these blocks. Traditionally, statistical experiments require full randomisation to eliminate systematic errors that can create bias in the results.18 However, we have found that waiting for heating and cooling of the reactor is the biggest contributor to the total optimisation time, and that randomisation did not lead to any difference in experimental results. Therefore it was decided that a higher intensification of experiments could be achieved with ascending ordering of temperature.
Models for the composition of compounds 1–3 were generated by creating a saturated model including all square and interaction terms and then manually removing any non-significant terms.19 The yield of 2 for each data point is shown in Fig. 4, and further model information can be found in the ESI.† These models were generated using experiments conducted over a period of 5.5 hours with excellent fit and predictability (R2 = 0.999 and Q2 = 0.977). An optimum for 2 was predicted by minimizing 1 and 3 and maximizing 2, which predicted conditions to generate 2 in 96% yield (ester 1 flow 0.1 mL min−1, MeNH2 9.7 eq., 7 °C, Fig. 5).
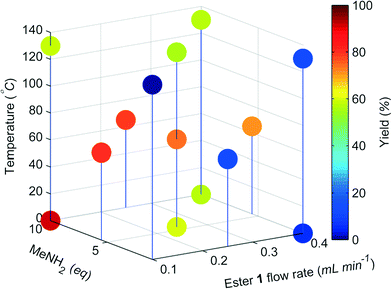 |
| Fig. 4 3-D plot showing the yield of 2 for each experimental data point in the CCF DoE. | |
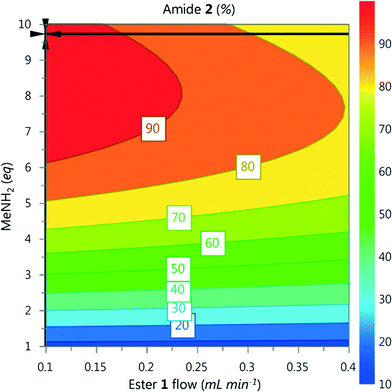 |
| Fig. 5 Contour plot for the optimum conditions derived from the CCF model, generated in MODDE. Temperature fixed at 7 °C, optimum point highlighted by the crosshair. | |
Conclusions
Online MS has been shown to optimise a model reaction using an automated continuous reactor. It was possible to calibrate the MS signal to HPLC using linear relative response values, with minimal effort in data manipulation. It was also possible to distinguish between product adducts and isotope patterns. The MS was subsequently used to determine steady state and calculate the yield in two separate optimisations. The optimum conditions achieved match very well showing the high reproducibility using this approach (Table 1) and either approach could be used to optimise the reaction system. It is important to consider that SNOBFIT experimentally verifies the optimum as part of the algorithm process giving higher confidence but took significantly longer (12 hours vs. 5.5 hours) than the structured DoE optimisation as these experiments were ordered to minimize reactor temperature changes. However it should be noted that a fully randomised statistical design would take considerably longer.
In addition, statistical modelling of the SNOBFIT data could also be performed to generate similar response surface models to the DoE model due to good coverage of the reaction space. It is also possible to verify model performance by inputting the SNOBFIT dataset into the DoE model. For example the optimal SNOBFIT data point from Table 2 was predicted to have a yield of 96% by the DoE model.
Table 2 Comparison of the optimum conditions obtained through the CCF (predicted) and self-optimisation (experimental)
Optimisation |
Ester 1 flow rate (mL min−1) |
MeNH2 molar eq. |
Temperature (°C) |
Amide 2 yield (%) |
DoE predicted |
0.100 |
9.7 |
7 |
96 (predicted) |
Experimental SNOBFIT |
0.100 |
10 |
10.6 |
93 (experimental) |
MS has the potential to be a powerful process analytical technology. Discrete separation and product quantification can be achieved with minimal method development, and significantly reduced method times when compared to chromatography. Therefore rapid analysis with detailed molecular characterization information can be obtained. This has been exploited to enable rapid optimisation using both a black-box algorithm and statistical optimisation of an automated flow reactor and we aim to extend the scope to more complex chemistries using compounds that are difficult to analyse using other techniques.
Acknowledgements
We would like to thank Tony Bristow and Andy Ray from AZ for MS experimental set-up advice and Andy Baker, Mark Allen and Clive Aldcroft from Advion. We would also like to thank Matthew Broadbent for technical support as well as Katherine Jolley, William Reynolds and Mary Gunn. Financial support was provided by AstraZeneca, EPSRC DTG funding and the University of Leeds.
Notes and references
-
N. Holmes and R. A. Bourne, in Chemical Process Technology for a Sustainable Future, ed. T. M. Letcher, J. L. Scott and D. A. Paterson, RSC Publishing, 1st edn, 2014, ch. 3, pp. 28–45 CrossRef CAS PubMed; C. Henry, D. Bolien, B. Ibanescu, S. Bloodworth, D. C. Harrowven, X. L. Zhang, A. Craven, H. F. Sneddon and R. J. Whitby, Eur. J. Org. Chem., 2015, 1491–1499 CrossRef CAS PubMed; B. Gutmann, P. Elsner, A. O'Kearney-McMullan, W. Goundry, D. M. Roberge and C. O. Kappe, Org. Process Res. Dev., 2015, 19, 1062–1067 CrossRef; S. C. Stouten, T. Noel, Q. Wang and V. Hessel, Chem. Eng. J., 2015, 279, 143–148 CrossRef; C. J. Smith, N. Nikbin, S. V. Ley, H. Lange and I. R. Baxendale, Org. Biomol. Chem., 2011, 9, 1938–1947 Search PubMed.
- R. A. Skilton, R. A. Bourne, Z. Amara, R. Horvath, J. Jin, M. J. Scully, E. Streng, S. L. Y. Tang, P. A. Summers, J. Wang, E. Perez, N. Asfaw, G. L. P. Aydos, J. Dupont, G. Comak, M. W. George and M. Poliakoff, Nat. Chem., 2015, 7, 1–5 CrossRef CAS PubMed; M. Rasheed and T. Wirth, Angew. Chem., Int. Ed., 2011, 50, 357–358 CrossRef PubMed; S. V. Ley, D. E. Fitzpatrick, R. J. Ingham and R. M. Myers, Angew. Chem., Int. Ed., 2015, 54, 3449–3464 CrossRef PubMed; S. V. Ley, D. E. Fitzpatrick, R. M. Myers, C. Battilocchio and R. J. Ingham, Angew. Chem., Int. Ed., 2015, 54, 10122–10136 CrossRef PubMed.
- S. Krishnadasan, R. J. C. Brown, A. J. de Mello and J. C. de Mello, Lab Chip, 2007, 7, 1434–1441 RSC.
- J. P. McMullen and K. F. Jensen, Org. Process Res. Dev., 2010, 14, 1169–1176 CrossRef CAS.
- A. J. Parrott, R. A. Bourne, G. R. Akien, D. J. Irvine and M. Poliakoff, Angew. Chem., Int. Ed., 2011, 50, 3788–3792 CrossRef CAS PubMed; R. A. Bourne, R. A. Skilton, A. J. Parrott, D. J. Irvine and M. Poliakoff, Org. Process Res. Dev., 2011, 15, 932–938 CrossRef; D. N. Jumbam, R. A. Skilton, A. J. Parrott, R. A. Bourne and M. Poliakoff, J. Flow Chem., 2012, 2, 24–27 CrossRef.
- R. A. Skilton, A. J. Parrott, M. W. George, M. Poliakoff and R. A. Bourne, Appl. Spectrosc., 2013, 67, 1127–1131 CrossRef CAS PubMed.
- V. Sans, L. Porwol, V. Dragone and L. Cronin, Chem. Sci., 2015, 6, 1258–1264 RSC.
- J. S. Moore and K. F. Jensen, Org. Process Res. Dev., 2012, 16, 1409–1415 CrossRef CAS.
- J. P. McMullen and K. F. Jensen, Org. Process Res. Dev., 2011, 15, 398–407 CrossRef CAS.
- B. J. Reizman and K. F. Jensen, Org. Process Res. Dev., 2012, 16, 1770–1782 CrossRef CAS.
- J. S. Moore and K. F. Jensen, Angew. Chem., Int. Ed., 2014, 53, 470–473 CrossRef CAS PubMed.
- C. J. Smith, N. Nikbin, S. V. Ley, H. Lange and I. R. Baxendale, Org. Biomol. Chem., 2011, 9, 1938–1947 Search PubMed; C. F. Carter, H. Lange, S. V. Ley, I. R. Baxendale, B. Wittkamp, J. G. Goode and N. L. Gaunt, Org. Process Res. Dev., 2010, 14, 393–404 CrossRef CAS.
- S. A. Leung, R. F. Winkle, R. C. R. Wootton and A. J. deMello, Analyst, 2005, 130, 46–51 RSC; M. F. Roberto, T. I. Dearing, S. Martin and B. J. Marquardt, J. Pharm. Innov., 2012, 7, 69–75 CrossRef CAS; O. Bleie, M. F. Roberto, T. I. Dearing, C. W. Branham, O. M. Kvalheim and B. J. Marquardt, J. Flow Chem., 2015, 5, 183–189 CrossRef.
- D. L. Browne, S. Wright, B. J. Deadman, S. Dunnage, I. R. Baxendale, R. M. Turner and S. V. Ley, Rapid Commun. Mass Spectrom., 2012, 26, 1999–2010 CrossRef CAS PubMed; T. W. T. Bristow, A. D. Ray, A. O'Kearney-McMullan, L. Lim, B. McCullough and A. Zammataro, J. Am. Soc. Mass Spectrom., 2014, 25, 1794–1802 CrossRef PubMed; K. M. Roscioli, X. Zhang, S. X. Li, G. H. Goetz, G. Cheng, Z. Zhang, W. F. Siems and H. H. Hill Jr., Int. J. Mass Spectrom., 2013, 336, 27–36 CrossRef.
- J. S. Mathieson, M. H. Rosnes, V. Sans, P. J. Kitson and L. Cronin, Beilstein J. Nanotechnol., 2013, 4, 285–291 CrossRef PubMed; D. E. Fitzpatrick, C. Battilocchio and S. V. Ley, Org. Process Res. Dev., 2015 DOI:10.1021/acs.oprd.5b00313.
- W. Zhao, Z. Jusys and R. J. Behm, Anal. Chem., 2010, 82, 2472–2479 CrossRef CAS PubMed; Z. Zhu, J. E. Bartmess, M. E. McNally, R. M. Hoffman, K. D. Cook and L. Song, Anal. Chem., 2012, 84, 7547–7554 CrossRef PubMed; A. W. Owen, E. A. J. McAulay, A. Nordon, D. Littlejohn, T. P. Lynch, J. S. Lancaster and R. G. Wright, Anal. Chim. Acta, 2014, 849, 12–18 CrossRef PubMed.
- W. Huyer and A. Neumaier, ACM Trans. Math. Softw., 2008, 35, 1–25 CrossRef.
- M. R. Owen, C. Luscombe, Lai, S. Godbert, D. L. Crookes and D. Emiabata-Smith, Org. Process Res. Dev., 2001, 5, 308–323 CrossRef CAS.
- DoE models were analysed and optimised using MODDE v10.1.1 (Umetrics).
Footnote |
† Electronic supplementary information (ESI) available. See DOI: 10.1039/c5re00083a |
|
This journal is © The Royal Society of Chemistry 2016 |