DOI:
10.1039/C6RA11292G
(Paper)
RSC Adv., 2016,
6, 54149-54161
Modeling and optimization of Hg2+ ion biosorption by live yeast Yarrowia lipolytica 70562 from aqueous solutions under artificial neural network-genetic algorithm and response surface methodology: kinetic and equilibrium study
Received
1st May 2016
, Accepted 17th May 2016
First published on 19th May 2016
Abstract
This study was devoted to the investigation of Hg2+ ion biosorption by a live yeast organism, namely, Yarrowia lipolytica 70562 (supplied commercially), which was sufficiently identified and studied by SEM-EDS and FT-IR methods. Dependency and relation among experimental parameters and conventional experimental conditions such as pH, concentration of Hg2+ ion, contact time and temperature were examined. Subsequently, these parameters were modeled and the optimum conditions were determined accordingly. It was found that the real experimental data with high efficiency can be represented and explained by the ANN-GA model and response surface methodology. Furthermore, kinetic and isotherm models under the optimum conditions obtained from ANN-GA were studied. Lateral studies reveal that a pseudo-second order kinetic model, an intraparticle diffusion model and a Langmuir model are good and efficient models for representation and explanation for the experimental result trends.
1. Introduction
Toxic dyes and heavy metals are major pollutants that extensively affect the quality and safety of industrial and urban waste water. Such seriously polluted water adversely affects mass transfer in a system by attacking the cell wall of living organisms. Therefore, their accumulation from the food chain is associated with the arrival of relatively low concentrations of heavy metals that may generate serious problems for most living things.1,2 Among all the existing heavy metals, considerable attention has been devoted to the presence of Hg2+ ions because as a soft ion, it can bind to thiols and nitrogen centers, which will lead to local distraction of the cells and finally cause organism death. Mercury metal ions can be present as free inorganic ions or methylated mercury that can arrive into the system via effluents from oil refining, pulp and paper, chlor-alkali, pharmaceutical processing, electrical processing, rubber processing and fertilizer industries. The toxicity of the Hg+2 ion depends on its concentration and chemical form. This has significant concern in most industries due to economic and environmental factors.3,4
Membrane process, chemical precipitation, and ion exchange are conventional techniques having their own advantages and disadvantages for the cleanup procedure and lowering of metal ion contents to a value lower than the threshold limit. One of the most safe and efficient procedures for this propose is biosorption, which is superior to most conventional techniques in terms of operational simplicity and providing lower costs for reducing5–7 heavy metal concentrations using inexpensive biosorbent materials even in more diluted wastewater.8–10 Among microorganisms, including bacteria, algae, fungi and yeast, the most attention was devoted to yeast applications that offer advantages such as a high percentage of cell wall material that shows excellent metal binding properties. Literature corresponding to biosorption of Hg2+ ions using yeast Y. lipolytica 70562 in aqueous systems is quite scarce, whereas previously we supplied this yeast from the same media to remove metal ions with acceptable efficiency for malachite green or some other metal ions.7,11 Due to the labor intensive time and tedious stage of supplying and purification of yeast, their lower efficiency for mercury removal, and the great demand for reducing Hg2+ ions, we were encouraged to apply commercially available conventional Y. lipolytica 70562 to improve and investigate the applicability of this low cost, and even accessible, yeast for removal of pollutants. This non-conventional yeast is strictly an aerobic microorganism that laterally produces important metabolites while it also has intense secretory activity that justifies the subsequent effort for its usage in industry (as a biocatalyst), molecular biology and genetics studies. It is considered as nonpathogenic and several processes based on this organism have been classified as generally recognized safe (GRAS) by the Food and Drug Administration (FDA, USA).12,13
The extensive application of the biosorption process for wastewater treatment on the industrial scale encourages researchers to improve process efficiency and reduce operational costs. In a short time, this purpose can simply be achieved following the use of simultaneous optimization techniques such as response surface methodology (RSM) and artificial neural network-genetic algorithms (ANN-GA).14,15 RSM is based on proper selection of factors and levels that lead to the least number of experiments to give useful idea about the main interaction of variables and can be used to guide researchers to find the best real optimum point despite the existence of localized imaginary points.16 A mathematical model supplied by RSM in a linear and/or nonlinear relationships, lateral response surface graphs and contours plots help to visualize the shape of a response surface.17,18
Artificial neural network (ANN) is a powerful modeling technique based on no assumptions concerning the nature of the phenomenological mechanisms and understanding the mathematical background of the problem underlying the process. Moreover, it has the ability to learn linear and nonlinear relationships between variables that can represent the relationship between input and output variables.19,20
Genetic algorithms (GA), according to evolutionary algorithms via parameters space coding, can be applied in complex spaces of various scientific disciplines following admittance of a maximize or minimize objective function. The GA can obtain acceptable results using operators (namely, reproduction, crossover and mutation).21,22
The present study describes the efficiency of Y. lipolytica 70562 for biosorption of Hg2+ ions from an aqueous solution following optimization of influencing parameters, including pH, concentration of Hg2+ ions, contact time and temperature. The aims of this study are (i) to obtain a predictive model based on ANN-GA and RSM techniques for prediction of the biosorption percentage of Hg2+ ions, (ii) to maximize the biosorption percentage of Hg2+ ion using RSM and ANN-GA models, and (iii) to develop a simple and fast method for biosorption of Hg2+ ions.
2. Experimental
2.1. Instruments and reagents
All chemicals, including Hg(NO3)2, NaOH and HCl, with the highest available purity were purchased from Merck (Dermasdat, Germany). A stock solution (1000 mg L−1) of Hg2+ ions was prepared and laterally their different working solutions were prepared by proper dilution. A HERMLE bench centrifuge (2206 A, Germany) was used to accelerate the phase separation. The samples were agitated in an incubator shaker (Labcon, FSIM-SPO16, USA) at 160 rpm, whereas the pH was adjusted using a pH/Ion meter model-686. The concentration of Hg2+ ions at all stages was determination by inductively coupled plasma mass spectroscopy (ICP, PerkinElmer Company, Optima 8300 model, USA). The morphology of the yeast Y. lipolytica 70562 was observed by field emission scanning electron microscopy (FE-SEM, Hitachi S-4160) under an acceleration voltage of 18 kV. Fourier transform infrared (FT-IR) spectra were obtained using a Perkin Elmer-Spectrum RX-IFTIR spectrometer in the range of 400–4000 cm−1. The STATISTICA 10 software was used for the regression and graphical analysis of the obtained data.
2.2. Preparation of live yeast Y. lipolytica 70562
Yeast Y. lipolytica 70562 was cultivated at 30 °C in a 250 mL Erlenmeyer flask containing 50 mL of production medium composed of 20 g L−1 glucose, 10 g L−1 yeast extract, 10 mg L−1 MnSO4·4H2O and 20 mg L−1 CuSO4·5H2O. Cells of yeast Y. lipolytica 70562 were inoculated into 100 mL peptone water in 500 mL flasks and incubated on a shaker at 150 rpm for 24 h at 30 °C. The cells were grown to the late exponential phase and the strains were grown in nutrient broth agar for 24 h at 30 °C. A colony of each strain was incubated in 3 mL of broth solution (brain heart infusion) for 24 h at 30 °C. 100 mL of each strain was transferred to fresh broth solution and incubated for 24 h at 30 °C. They were transferred to SPC agar (Standard Plate Count) and incubated for 24 h at 30 °C for storage.
2.3. Batch biosorption studies
The growth medium (10 g of peptone, 10 g of glucose and 5 g of yeast extract dissolved in 500 mL deionization water) was sterilized by autoclaving at a pressure of 15 pounds per square inch and a temperature of 121 °C for 15 min. A loop of the yeast Y. lipolytica 70562 was added to this growth medium by shaking at 160 rpm at 25 °C. After 12 h, a known concentration of Hg2+ ion (20–60 mg L−1) was added to the Erlenmeyer flask with different pH levels (4.0–8.0) at various temperatures (15–35 °C) for known contact times (6–18 h) on incubator shakers. In the batch biosorption experiments, samples were taken at given time intervals and were centrifuged at 3500 rpm for 15 min and loose Hg2+ ion concentrations were determined using ICP. Biosorption efficiency, expressed as percent biosorption of Hg2+ ion, was determined using the following equation:23 |
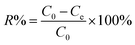 | (1) |
where C0 and Ce are the initial and final concentrations of Hg2+ ion (mg L−1), respectively. Equilibrium biosorption studies were conducted to develop the isotherm model that may describe the media performance and correlates metal distribution between biosorbent (yeast Y. lipolytica 70562) and aqueous solution. The extent of biosorption at equilibrium (qe, mg g−1) was calculated from the initial and equilibrium Hg2+ concentrations in a solution based on the mass balance eqn (2): |
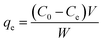 | (2) |
where V is the volume (L) of solution and W is the amount (g) of biosorbent.
2.4. Response surface methodology (RSM) definition
RSM following CCD (Table 1) based on a desirability function was used to optimize the relationship among response and experimental variables such as pH (X1), concentration of Hg2+ ion (X2), temperature (X3) and contact time (X4) on the biosorption of Hg2+ ion via subsequent calculation of each term coefficient. The design matrix was generated and results were statistically analyzed using STATISTICA 10 software.24 The regression analysis and constructed model were used to collaborate the experimental response over a pre-specified space that also shows information about the contribution of each term on the response. This guides researchers to conduct experimental runs to improve characteristic performances of the method while STATISTICA 10 software was used for the analysis of experimental results and also to judge the ability of the method for fitting experimental data.25 Experiments were conducted randomly in order to avoid questionable variability that affects the outcome of the responses due to extraneous factors. The results and the second-order polynomial coefficients were completely analyzed using the analysis of variance (ANOVA) by the statistical software. Multiple regressions were applied in analyzing experimental data to predict the coefficients of the fitted second-order polynomial model.26
Table 1 Experimental factors and levels in the central composite design
Factors |
Unit |
Symbol |
Levels |
Star point (α = 2.0) |
−α |
Low (−1) |
Central (0) |
High (+1) |
+α |
pH |
|
X1 |
4.0 |
5.0 |
6.0 |
7.0 |
8.0 |
Hg2+ concentration |
mg L−1 |
X2 |
20 |
30 |
40 |
50 |
60 |
Temperature |
°C |
X3 |
15 |
20 |
25 |
30 |
35 |
Contact time |
h |
X4 |
6 |
9 |
12 |
15 |
18 |
Run |
Factors |
Response (R% Hg2+) |
X1 |
X2 |
X3 |
X4 |
Experimental |
Predicted |
Residual |
1 |
7.0 |
30 |
20 |
9 |
91.130 |
87.499 |
0.291 |
2 |
6.0 |
40 |
25 |
12 |
94.740 |
95.013 |
0.227 |
3 |
5.0 |
50 |
30 |
9 |
79.670 |
94.716 |
0.165 |
4 |
7.0 |
50 |
20 |
15 |
93.890 |
94.716 |
1.095 |
5 |
6.0 |
40 |
15 |
12 |
94.170 |
94.716 |
−0.185 |
6 |
5.0 |
50 |
30 |
15 |
75.300 |
90.368 |
0.762 |
7 |
7.0 |
30 |
20 |
15 |
97.520 |
94.716 |
0.025 |
8 |
6.0 |
20 |
25 |
12 |
93.660 |
78.765 |
0.905 |
9 |
8.0 |
40 |
25 |
12 |
89.090 |
93.826 |
0.064 |
10 |
4.0 |
40 |
25 |
12 |
69.410 |
94.127 |
0.043 |
11 |
6.0 |
40 |
25 |
6 |
88.090 |
75.811 |
−0.511 |
12 |
6.0 |
40 |
25 |
12 |
95.020 |
98.357 |
−0.837 |
13 |
5.0 |
30 |
30 |
12 |
80.480 |
93.803 |
−0.143 |
14 |
7.0 |
30 |
30 |
9 |
92.120 |
89.412 |
−0.322 |
15 |
5.0 |
50 |
20 |
15 |
84.740 |
69.406 |
0.004 |
16 |
5.0 |
30 |
20 |
9 |
87.360 |
88.473 |
−0.383 |
17 |
5.0 |
50 |
20 |
9 |
83.180 |
94.716 |
0.305 |
18 |
6.0 |
40 |
25 |
12 |
94.180 |
80.926 |
−0.446 |
19 |
6.0 |
40 |
25 |
12 |
93.960 |
91.807 |
0.313 |
20 |
5.0 |
30 |
30 |
9 |
81.050 |
84.986 |
−0.246 |
21 |
6.0 |
40 |
35 |
12 |
86.030 |
88.152 |
−0.792 |
22 |
7.0 |
50 |
30 |
15 |
94.460 |
83.157 |
0.023 |
23 |
6.0 |
60 |
25 |
12 |
87.220 |
94.716 |
−0.535 |
24 |
5.0 |
30 |
20 |
15 |
92.610 |
94.716 |
−0.755 |
25 |
7.0 |
50 |
30 |
9 |
91.340 |
80.641 |
0.409 |
26 |
6.0 |
40 |
25 |
18 |
93.460 |
86.391 |
−0.361 |
27 |
5.0 |
30 |
30 |
12 |
80.480 |
93.601 |
0.859 |
28 |
4.0 |
40 |
25 |
12 |
69.410 |
87.396 |
−0.176 |
29 |
6.0 |
40 |
15 |
12 |
94.170 |
91.643 |
0.967 |
30 |
6.0 |
40 |
25 |
12 |
94.880 |
92.057 |
−0.717 |
31 |
5.0 |
50 |
20 |
9 |
83.180 |
93.507 |
−0.047 |
2.5. Artificial neural network definition
In this study, the Neural Network Toolbox of MATLAB 7.12.0.635 (R2011a) mathematical software was used to predict biosorption efficiency. Input variables for feed-forward neural network were pH, Hg2+ ion concentration, contact time and temperature, and their influence on the Hg2+ ion biosorption efficiency was examined following conduction of 31 experimental data points for the ANN ratio of 70%, 15% and 15% for train, test and validation set, respectively. A three-layer ANN with log-sigmoid transfer functions with a back-propagation algorithm was designed for this study. All the data (input and output) for ANN models were normalized between 0.1 and 0.8 to avoid numerical overflows due to very large or small weights. The normalization equation applied was as follows:27 |
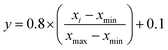 | (3) |
where y is the normalized value of xi and xmax and xmin are the maximum and minimum value of xi, respectively. The criterion for selection of the optimum ANN structure is the MSE of the test data as well as the correlation coefficient (R2), which can be defined as follows:28 |
 | (4) |
|
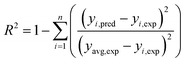 | (5) |
where yi,pred was the predicted value by ANN model, yi,exp was the experimental value and N was the number of data points.
2.6. Genetic algorithm definition
The GA begins with a population of represented random solutions in some series of structures. In fact, GA is based on global optimization without any dependency to the initial value to achieve convergence, although the process is very time consuming. The GA is composed of various stages of fitness computation based on the objective function. Selection of the best chromosomes and genetic propagation of each chosen parent chromosome is accomplished by genetic operators such as crossover and mutation. Crossover and mutation are implemented to produce new and better populations of chromosomes.
3. Result and discussion
3.1. FTIR and SEM analyses
The identification and existence of functional groups that belong and appear in the biomass of yeast Y. lipolytica 70562 and metal-loaded biosorbent were studied by FT-IR analysis at room temperature and the respective FT-IR spectra are shown in Fig. 1 and Table 2. In these spectra, a peak in the broad band range of 3200–3400 cm−1 corresponds to the existence of hydroxyl groups on Y. lipolytica 70562. The stretching peak at 2900–2960 cm−1 is probably due to the asymmetric stretching vibration of C–H bonds from alkyl groups in the proteins and carbohydrates of the cell wall, whereas the absorption peak around 1652 cm−1 (COOH stretching) was significantly increased. Each spectra demonstrate a sharp, intense peak at 1656 cm−1 corresponding to aromatic C
O. The methyl asymmetric C–H bands at 1050 cm−1 are due to the C–O stretching of alcohol, sulfoxide, carbohydrates or polysaccharide-like substances. The peak at 1450–1420 cm−1 indicates the presence of carboxyl, whereas the one at 1200–1300 cm−1 was assigned to the asymmetric stretching of –C–O. The final trough at 532 cm−1 could correspond to O–C–O scissoring and C–O bending vibrations. The abovementioned evidence (see Table 2) suggests that the mechanism of Hg2+ ion biosorption involves hydroxyl (–O–H), amine (–N–H) and carboxylate ions (–COO–) groups of the polysaccharides on the peptidoglycan layer, and following loading the metal ion do not change significantly. The FE-SEM and EDS spectra of Y. lipolytica 70562 before Hg2+ biosorption indicated the presence of C, O, K and Cu as natural species on the biosorbent (Fig. 2a), whereas after Hg2+ biosorption (Fig. 2b), the presence of signal corresponding to this element shows high efficiency of this biosorption process.
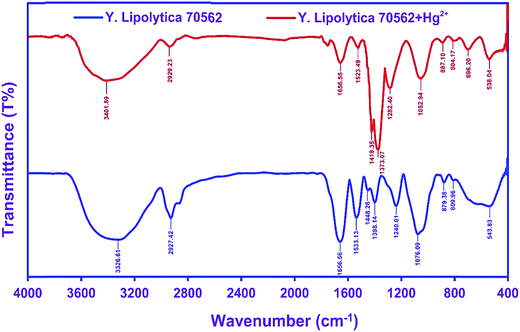 |
| Fig. 1 FTIR spectra of yeast Y. lipolytica 70562 and yeast Y. lipolytica 70562 – Hg2+. | |
Table 2 FT-IR peaks for yeast Y. lipolytica 70562 and yeast Y. lipolytica 70562 – Hg2+
Biosorbent (cm−1) |
Metal loaded biosorbent (cm−1) |
Change in peak behavior after Hg2+ loaded |
IR peak range (cm−1) |
Functional group reported corresponding to the observed peak behavior7,11,36 |
3326.61 |
3401.89 |
Shift |
3600–3200 |
–O–H stretching vibration |
2927.42 |
2929.23 |
Shift |
2850–2956 |
Alkanes –CH stretching vibration |
1656.56 |
1656.55 |
No change |
1730–1625 |
–C O stretching vibration |
1533.13 |
1523.49 |
Shift |
1600–1500 |
–N O stretching vibration |
1448.28 |
1419.35 |
Shift |
1550–1450 |
–N–H bending vibration |
1398.14 |
1373.07 |
Shift |
1400–1300 |
–N O stretching vibration |
1240.01 |
1282.40 |
Shift |
1300–1000 |
–C–O stretching vibration |
1076.09 |
1052.94 |
Shift |
1260–1000 |
–CO stretching vibration, carboxylic acid |
879.38 |
887.10 |
Shift |
750–1750 |
Phenyl ring substitution band |
C–H aromatic |
809.96 |
804.17 |
Shift |
C–H aromatic |
— |
696.20 |
Additional peak |
— |
C–CO–C bend in ketones |
543.83 |
538.04 |
Shift |
— |
C=O bend in amides |
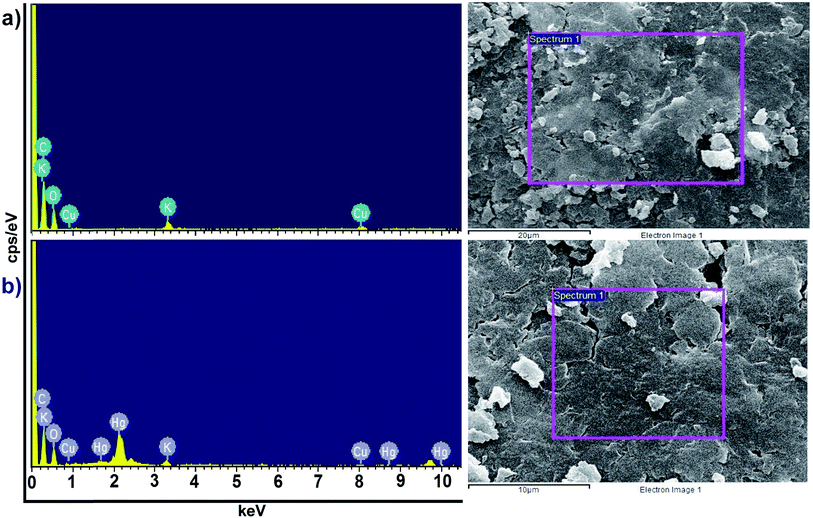 |
| Fig. 2 SEM-EDS spectra of yeast Y. lipolytica 70562: (a) in the absence of Hg2+ and (b) after Hg2+ biosorption (C0 = 20 mg L−1). | |
3.2. Response surface methodology
Optimization of Hg2+ ion biosorption under RSM based on a 4-factor and 5-level CCD and their subsequent analysis by ANOVA gives useful and suggestive information about the contributions of pH, Hg2+ ion concentration, temperature and contact time on the efficiency the system under study. According to the RSM results and subsequent ANOVA analysis, the following constructed model was used to express dependency of response to significant terms: |
Y(R% Hg2+) = −29.36 + 33.12X1 + 0.12X2 − 0.51X3 + 3.20X4 + 0.45X1X3 + 0.37X1X4 + 0.016X2X3 − 0.08X3X4 − 3.83X12 − 0.01X22 − 0.05X32 − 0.10X42
| (6) |
where X1, X2, X3 and X4 are the coded independent variables of pH, concentration of Hg2+ ion, temperature and contact time, respectively.
Analysis of variance was carried out to justify the significance and adequacy of the regression model and the results are summarized in Table 3. The model F-value for Hg2+ ion biosorption efficiency was 182.15, indicating that the model was highly adequate and significant. Moreover, the determination coefficient (R2) of the model was 0.9938 for biosorption efficiency and indicated that the prediction of Hg2+ ion biosorption efficiency by the polynomial model was excellent.29 The Radj2 value (0.9883) indicated the high degree of correlation between the observed and predicted values and the Rpred2 value (0.9702) was in agreement with R2 and Radj2. Moreover, the p-values of lack of fit of 0.2646 for biosorption efficiency of Hg2+ ion implied that the lack of fit was not significant.30
Table 3 Analysis of variance (ANOVA) for biosorption efficiency of Hg2+ ion
Factor |
Sums of squares |
Degrees of freedom |
Mean squares |
F-Value |
p-Value |
Model |
1347.91 |
14 |
96.28 |
182.15 |
<0.0001 |
X1 |
590.65 |
1 |
590.65 |
1117.41 |
<0.0001 |
X2 |
60.63 |
1 |
60.63 |
114.70 |
<0.0001 |
X3 |
88.38 |
1 |
88.38 |
167.20 |
<0.0001 |
X4 |
35.18 |
1 |
35.18 |
66.55 |
<0.0001 |
X1X2 |
4.43 |
1 |
4.43 |
8.37 |
0.0106 |
X1X3 |
78.19 |
1 |
78.19 |
147.92 |
<0.0001 |
X1X4 |
18.01 |
1 |
18.01 |
34.07 |
<0.0001 |
X2X3 |
9.52 |
1 |
9.52 |
18.00 |
0.0006 |
X2X4 |
2.47 |
1 |
2.47 |
4.67 |
0.0463 |
X3X4 |
20.35 |
1 |
20.35 |
38.50 |
<0.0001 |
X12 |
417.55 |
1 |
417.55 |
789.93 |
<0.0001 |
X22 |
30.23 |
1 |
30.23 |
57.19 |
<0.0001 |
X32 |
35.35 |
1 |
35.35 |
66.89 |
<0.0001 |
X42 |
24.73 |
1 |
24.73 |
46.78 |
<0.0001 |
Residual |
8.46 |
16 |
0.53 |
|
|
Lack of fit |
6.26 |
10 |
0.63 |
1.71 |
0.2646 |
Pure error |
2.20 |
6 |
0.37 |
|
|
Cor total |
1356.37 |
30 |
|
|
|
R2 |
Adj-R2 |
Pred-R2 |
Std. dev. |
AP |
CV% |
0.9938 |
0.9883 |
0.9702 |
0.728 |
57.251 |
0.81 |
Furthermore, relatively low values of coefficient of variation (CV%) in Table 3 showed that the variation was acceptable and satisfactory. In addition, adequate precision could measure the signal to noise ratio. The desirable ratios of 57.251 implied an adequate signal.31 Therefore, the models could be applied to navigate the design space. Table 3 also demonstrated that the linear coefficients (X1, X2, X3 and X4), cross product coefficients (X1X2, X1X3, X1X4, X2X3, X2X4 and X3X4) and quadratic terms (X12, X22, X32 and X42) significantly affected the Hg2+ ion biosorption efficiency by yeast Y. lipolytica 70562 (p < 0.05).
3.3. Tridimensional response surface plot
Fig. 3 displays the 3D surface plots and planar contour plots between every two independent variables on the basis of eqn (6). Fig. 3a shows the simultaneous effect of initial solution pH and initial Hg2+ ion concentration on the Hg2+ ion biosorption efficiency in an aqueous solution. The Hg2+ ion biosorption efficiency increased with an increase in the initial solution pH ranging from 4 to 7 as well as with initial Hg2+ ion concentration ranging from 20 to 60 mg L−1. Nevertheless, pH values higher than 7 reduced the biosorption efficiency of Hg2+ ion. On the other hand, Hg2+ ions biosorption efficiency decreased with increasing of the initial Hg2+ ions concentration ranging from 20 to 60 mg L−1. More contributions from pH on the method efficiency can be related to dependency on change in the chemistry of both Hg2+ ion and the biosorbent. It seems that hydrolysis of Hg2+ ions hinders their efficient accumulation on the biosorbent surface and simultaneously leads to protonation of biosorbent functional groups that strongly affect the biosorption efficiency. On the other hand, increasing the pH to the more alkaline region is associated with the formation of non-soluble Hg(OH)2 and/or Hg(OH)+ species, both of which strongly led to the reduction of metal ion biosorption. The effect of initial temperature and contact time on the Hg2+ ions biosorption (Fig. 3b) shows a positive relationship among biosorption efficiency and temperature until 23 °C, as well as contact time until 18 h, whereas a temperature higher than 23 °C lead to a decrease in biosorption efficiency. On the other hand, metal uptake was increased at higher until 16 h and subsequently contact time above this value was associated with a reduction in the biosorption efficiency of Hg2+ ion.
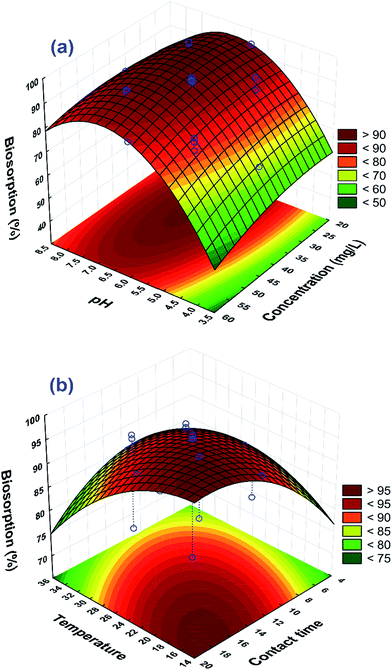 |
| Fig. 3 Tridimensional response surface plot for the biosorption efficiency of Hg2+ ion. | |
3.4. Optimization of biosorption efficiency by RSM
The profile for predicted values and desirability option in the STATISTICA 7.0 software was used to optimize the process (Fig. 4). The desirability of responses for each dependent variable (biosorption efficiency of Hg2+ ion) to any variable was carried out by assigning predicted values. The critical value for the presentation of an undesirable value to a very desirable value (0.0 and 1.0, respectively) will help the researcher find the best operational condition.
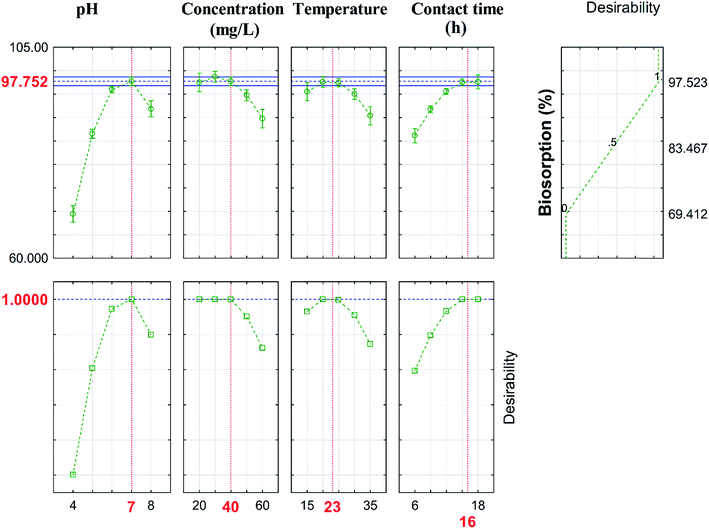 |
| Fig. 4 Profiles for predicated values and desirability function for biosorption efficiency of Hg2+ ion. Dashed line indicated current values after optimization. | |
The CCD design matrix results (Table 1) show that the maximum (97.52%) and minimum (69.41%) biosorption efficiency of Hg2+ ion correspond to the critical value.
The overall response obtained from these plots with the current level of each variable in the model is depicted at the top (left) of Fig. 4. On the basis of these calculations and the desirability score of 1.0, the maximum biosorption efficiency of Hg2+ ion (97.75%) was obtained at optimum conditions set as pH of 7, 40 mg L−1 of Hg2+ ion, 23 °C and 16 h, and replication of five experiments at these specific conditions was associated with the achievement of 95.69 ± 2.58. Statistical analysis by the t-test does not show a significant difference among the expected and experimental results. This confirms the high ability of this method for prediction of behavior of the system under study.
3.5. Modeling and optimization by ANN-GA
Based on preliminary experiments, the four variables, including pH, initial concentration of Hg2+ ion, temperature and contact time, were selected (Table 1). In this study, MLP (three layers) with a tansig transfer function at the hidden layer and a purelin transfer function at the output layer was used. The training cycles were carried out for various numbers of neurons in the hidden layer. Network architecture has important influences on the predicted values. The number of input and output nodes was equal to the number of input and output data, respectively (4 and 1 in this study). However, the number of neurons in the hidden layer was recognized by training several feed-forward neural networks of different architectures and selection of the optimal one was based on minimization of MSE and improving the generalization ability of the topology. In this study, the optimal topology of the ANN model developed involves four inputs, one hidden layer with 7 neurons and one output layer (4
:
7
:
1). The MSE and R2 for biosorption process are shown in Table 4. The MSE for the training set, testing set, validation set and all data were 0.087, 0.029838, 0.03893 and 0.071158, respectively. Moreover, ANN offered the weights for the coefficients between the artificial neurons and was considered analogous to synapse strengths between the axons and dendrites in real biological neurons. The respective weights that correspond to each term are shown in Table 5.
Table 4 Comparison of 10 neurons in the hidden layer for biosorption efficiency of Hg2+ ion by ANN model development with the Levenberg–Marquardt algorithm
Neuron |
MSEtrain |
MSEtest |
MSEvalidation |
MSE |
Rtrain2 |
Rtest2 |
Rvalidation2 |
R2 |
1 |
6.748698 |
7.488629 |
7.746596 |
7.014978 |
0.94653 |
0.927681 |
0.908499 |
0.924784 |
2 |
1.182237 |
3.87869 |
4.897789 |
2.164722 |
0.968947 |
0.98669 |
0.932759 |
0.975848 |
3 |
0.422384 |
0.60704 |
1.510644 |
0.617428 |
0.994743 |
0.99428 |
0.990259 |
0.99304 |
4 |
3.960392 |
2.649906 |
5.454408 |
3.988514 |
0.958376 |
0.954285 |
0.953262 |
0.953384 |
5 |
0.112222 |
0.143761 |
0.158311 |
0.124117 |
0.998208 |
0.998592 |
0.998756 |
0.998581 |
6 |
0.429581 |
0.410193 |
0.21464 |
0.393675 |
0.996113 |
0.995227 |
0.997089 |
0.995496 |
7 |
0.087 |
0.029838 |
0.03893 |
0.071158 |
0.999585 |
0.999072 |
0.999492 |
0.999187 |
8 |
0.808569 |
0.692291 |
0.764442 |
0.783991 |
0.98757 |
0.9919 |
0.988441 |
0.991025 |
9 |
0.155289 |
0.789754 |
0.802219 |
0.351632 |
0.988759 |
0.998727 |
0.981143 |
0.99607 |
10 |
0.14093 |
0.189818 |
0.131366 |
0.146956 |
0.998551 |
0.998965 |
0.998329 |
0.998728 |
Table 5 The weight and bias of trained ANN for predicting the biosorption efficiency of Hg2+ ion
w1 |
w2 |
b1 |
b2 |
−3.5974 |
3.8986 |
0.3608 |
1.7173 |
0.5231 |
1.5075 |
−0.0711 |
−4.0753 |
−4.3703 |
0.7949 |
−1.9124 |
0.1416 |
5.6578 |
|
−1.8286 |
0.6816 |
1.6296 |
−5.0658 |
−0.1595 |
3.9773 |
|
−5.7366 |
−1.2064 |
2.4372 |
−0.6970 |
−0.1320 |
0.4460 |
|
4.7448 |
−2.1442 |
2.6615 |
5.1964 |
0.1980 |
−3.5264 |
|
4.5236 |
1.3803 |
−2.3733 |
−1.8782 |
0.4560 |
0.3282 |
|
−3.5019 |
−2.4645 |
0.8401 |
0.7908 |
0.2905 |
0.7122 |
|
The MSE versus the number of epochs for optimal ANN models show that the training was stopped after 8 epochs (Fig. 5a). The good simulation and correlation of experimental and predicted results (test data) for the optimum number of hidden layer neurons confirm the accuracy of the neural network for modeling and prediction of the outlet composition of biosorption efficiency. Fig. 5b, error histogram for biosorption efficiency of Hg2+ ion by Y. lipolytica 70562, indicates very low errors for prediction of biosorption efficiency.
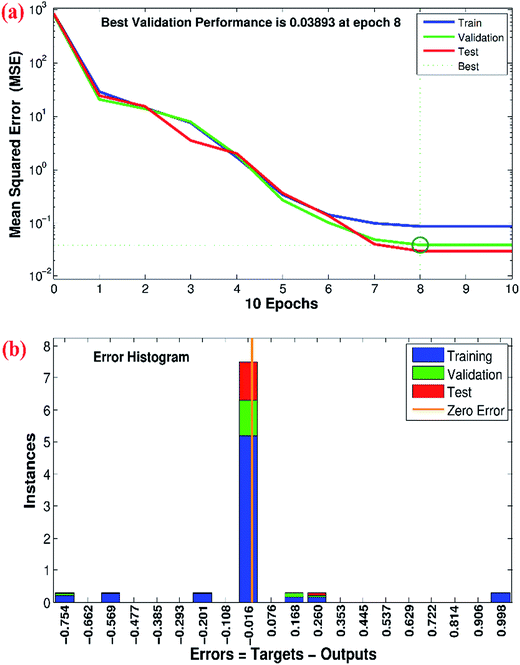 |
| Fig. 5 (a) MSE versus the number of epochs and (b) plot of the error histogram for biosorption efficiency of Hg2+ ion. | |
In the present study, as a continuation of other recent studies, the combination of ANN and GA was successfully applied for optimization. The method starts with a population of random regimes using pH, concentration of Hg2+ ion (mg L−1), temperature (°C) and contact time (h) as the optimization parameters. The objective function was the equation achieved from the ANN model that correlates the inputs with output and can be defined as follows:
|
y = −purelin(w2 × tansig(w1 × [x(1); x(2); x(3); x(4)] + b1) + b2)
| (7) |
where
x(1),
x(2),
x(3) and
x(4) represent the inputs,
w1 and
b1 are the weight and bias of the hidden layer and
w2 and
b2 are the weight and bias of the output layers (
Table 5). The Matlab R2011a genetic algorithm toolbox was applied for the GA process with 68 generations (
Fig. 6a), population size of 20 with a scattered crossover function of 0.8, rank scaling function and stochastic uniform option. For biosorption efficiency of Hg
2+ ion, the ANN-GA method predicts maximum extraction percentage (99.86%) at pH 6.9, 43.1 mg L
−1 of Hg
2+ ion, 25.1 °C and 15.4 h mixing time (
Fig. 6b).
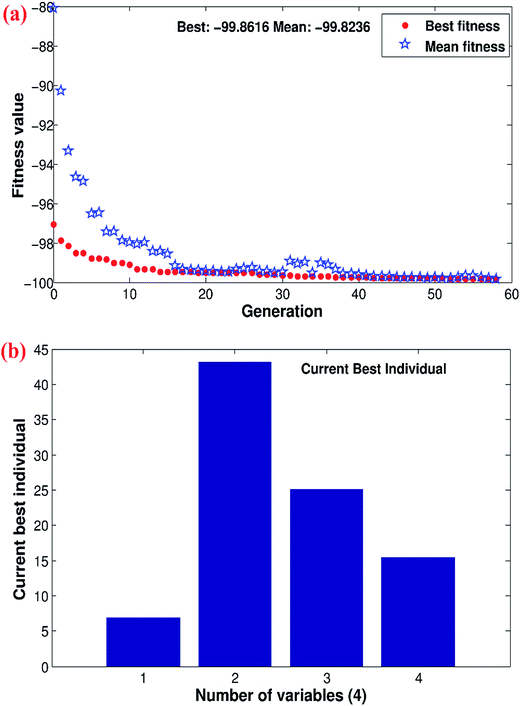 |
| Fig. 6 Plots of best fitness and best individual for biosorption efficiency of Hg2+ ion by the ANN-GA method. | |
3.6. Comparison of CCD and ANN-GA
CCD and ANN-GA with a high capability for solving linear and non-linear multivariate problems in the system under study were used for optimization and modeling Hg2+ ion biosorption efficiency by yeast Y. lipolytica 70562. The CCD approach has a regression equation for forecasting and achieving optimum conditions of variables, unlike ANN-GA. While CCD was able to solve only quadratic non-linear correlations, this limitation can be efficiently overcome by GA. The predicted optimum conditions by CCD and ANN-GA (Table 6) reveal an average Hg2+ ion biosorption efficiency of 99.67% ± 1.36% (n = 5). Existence of the high degree of agreement between the experimental and predicted optimum result indicates the high applicability of ANN-GA as the best evaluation and optimization of the effects of biosorption efficiency.
Table 6 The comparison between optimum conditions of CCD and ANN-GA modelsa
Approach |
Variables |
Biosorption (R%) |
pH |
Hg2+ concentration |
Temperature (°C) |
Contact time (h) |
Experimentalb |
Predicted |
CCD, central composite design. ANN-GA, artificial neural networks based on genetic algorithm. Average of five analyses. |
CCD |
7.0 |
40.0 |
23.0 |
16.0 |
95.69 ± 2.58 |
97.75 |
ANN-GA |
6.6 |
43.1 |
25.1 |
15.4 |
99.67 ± 1.36 |
99.86 |
3.7. Kinetic models
Different kinetic models such as pseudo-first-order, pseudo-second-order, Weber–Morris intraparticle diffusion and Elovich models are able to predict the rate controlling steps (mass transport, chemical reaction processes and biosorption).32,33 The linearized form of the pseudo-first order, pseudo-second order, intra-particle diffusion and Elovich models are shown in Table 7. The pseudo-second-order kinetic model correlation coefficients were close to unity for all the initial concentrations, suggesting the applicability of this model for best fitting and expression of Hg2+ ion biosorption efficiency by yeast Y. lipolytica 70562. Furthermore, the calculated biosorption capacity (194.28 mg g−1) corresponds to the pseudo-second-order kinetic reaction and has better agreement with the qe,exp values (173.94 mg g−1). Therefore, the second-order model could be used to describe the biosorption efficiency of Hg2+ ion by yeast Y. lipolytica 70562 (Table 7). To investigate the mechanism involved in biosorption of Hg2+ ion onto yeast Y. lipolytica 70562, experimental kinetic data were tested with the intraparticle diffusion and Elovich models. Table 7 shows that the regression lines for the intraparticle diffusion model had a good coefficient of determination (R2 = 0.99), which shows the suitability of the model for this process.
Table 7 Kinetic parameters for the biosorption of Hg2+ ion onto yeast Y. lipolytica 70562
Model |
Equation |
Parameters |
Value |
Pseudo-first-order kinetic |
log(qe − qt) = log(qe) − k1/2.303t |
Plot the values of log(qe − qt) versus t to give a linear relationship from which k1 and qe can be determined from the slope and intercept, respectively |
k1 (min−1) |
15.85 |
qe(calc) (mg g−1) |
35.70 |
R2 |
0.869 |
Pseudo-second-order kinetic |
(t/qt) = 1/(k2qe2) + 1/qe(t) |
Plot the values of (t/qt) versus t to give a linear relationship from which k1 and qe can be determined from the slope and intercept, respectively |
k2 (min−1) |
4.38 |
qe(calc) (mg g−1) |
194.28 |
R2 |
0.999 |
Intraparticle diffusion |
qt = Kdift1/2 + C |
The values of Kdif and C were calculated from the slope and intercept of the plot of qt versus t1/2, respectively |
Kdiff (mg g−1 min−1/2) |
8.62 |
C (mg g−1) |
44.81 |
R2 |
0.995 |
Elovich |
qt = 1/β ln(αβ) + 1/β ln(t) |
Plot the values of (qt) versus ln(t) to give a linear relationship from which α and β can be determined from the slope and intercept, respectively |
β (g mg−1) |
0.794 |
α (mg g−1 min−1) |
37 418 |
R2 |
0.924 |
Experimental data |
qe(exp) (mg g−1) |
173.94 |
3.8. Biosorption isotherms
Isotherm models such as the Langmuir and Freundlich biosorption isotherms were used to interpret the experimental data. Judgment on their suitability for the prediction of experimental data was performed according to the correlation coefficients of the models that were used to establish the best fitting model.34,35
The recorded values of coefficient of determination (R2) for biosorption efficiency of Hg2+ ion by yeast Y. lipolytica 70562 for the Langmuir isotherm and the Freundlich isotherm were 0.999 and 0.903, respectively (Table 8). Overall, the Langmuir isotherm fitted the isotherm data best and hence, it could be inferred that the biosorption process was better modelled by a monolayer (Qm (mg g−1)) compared to multilayer adsorption. For comparison, the Hg2+ biosorption capacity (Qm) obtained by the Y. lipolytica 70562 was also higher than those of some other biosorbent materials reported in the literature (Table 9), although this direct comparison is difficult due to the varying experimental conditions used in these studies. Differences of metal uptake are due to the properties of each biosorbent material such as structure, functional groups and surface area.
Table 8 Isotherm constant parameters and correlation coefficients calculated for the biosorption of Hg2+ ions onto yeast Y. lipolytica 70562
Model |
Equation |
Parameters |
Value |
Langmuir |
1/qe = 1/(KaQmCe) + 1/Qm |
A plot Ce/qe versus Ce should indicate a straight line of slope 1/Qm and an intercept of 1/(KaQm) |
Qm (mg g−1) |
214.19 |
Ka (L mg−1) |
28.57 |
R2 |
0.999 |
Freundlich |
ln qe = ln KF + (1/n)ln Ce |
The values of KF and 1/n were determined from the intercept and slope of linear plot of ln qe versus ln Ce, respectively |
1/n |
0.285 |
KF (L mg−1) |
8.620 |
R2 |
0.903 |
Table 9 Comparison of biosorption performance of yeast Y. lipolytica 70562 for removal of Hg2+ with some other biosorbents
Adsorbent or biosorbent |
Concentration ranges (mg L−1) |
Qmax (mg g−1) |
Ref. |
Phoenix dactylifera biomass |
50–200 |
46.73 |
37 |
Alginate |
30–500 |
29.00 |
38 |
Sugarcane Bagasse |
20–100 |
35.71 |
39 |
Ulva lactuca biomass |
50–250 |
149.25 |
40 |
Sargassum glaucescens |
10–400 |
147.50 |
41 |
Leaves of castor tree [Ricinus communis L.] |
50–500 |
32.70 |
42 |
Thiourea-modified magnetic chitosan microspheres |
50–1500 |
625.2 |
43 |
Alginate |
30–500 |
31.7 |
38 |
Alginate immobilized live |
30–500 |
84.4 |
38 |
Heat inactivated Phanerochaete chrysosporium |
30–500 |
172.1 |
38 |
Copper oxide nanoparticles |
10–100 |
825.21 |
44 |
Microalgae Chlamydomonas reinhardtii |
20–400 |
123.42 |
45 |
Carica papaya |
40–400 |
183.64 |
46 |
Buckwheat hulls |
200–1000 |
110.07 |
47 |
Y. lipolytica 70562 |
20–60 |
214.19 |
This work |
4. Conclusion
In this study, the live yeast Y. lipolytica 70562 was presented as an efficient biosorbent for the biosorption of Hg2+ from aqueous solutions. The influence of parameters, including pH, concentration of Hg2+ ion, contact time and temperature, were investigated and optimized with CCD and ANN-GA methods, and the results were compared. The results showed that the ANN-GA model was in good agreement with the experimental data in comparison with RSM. The equilibrium and kinetic models were studied for investigation of the biosorption process. The Langmuir equilibrium model and pseudo-second-order kinetic model gave the best fitness with experimental data.
Acknowledgements
The authors express their appreciation to the Graduate School and Research Council of the University of Yasouj for financial support of this study.
References
- W. M. Ibrahim, J. Hazard. Mater., 2011, 192, 1827–1835 CrossRef CAS PubMed.
- Z. Hajahmadi, H. Younesi, N. Bahramifar, H. Khakpour and K. Pirzadeh, Water Resources and Industry, 2015, 11, 71–80 CrossRef.
- N. Salamun, S. Triwahyono, A. Jalil, T. Matsuura and N. Salleh, RSC Adv., 2015, 5, 14129–14137 RSC.
- B. Henriques, L. S. Rocha, C. B. Lopes, P. Figueira, R. J. Monteiro, A. Duarte, M. Pardal and E. Pereira, Chem. Eng. J., 2015, 281, 759–770 CrossRef CAS.
- K. Zhou, Z. Yang, Y. Liu and X. Kong, J. Environ. Chem. Eng., 2015, 3, 2219–2228 CrossRef CAS.
- Z. Chen, W. Ma and M. Han, J. Hazard. Mater., 2008, 155, 327–333 CrossRef CAS PubMed.
- A. Asfaram, M. Ghaedi, G. R. Ghezelbash, E. A. Dil, I. Tyagi, S. Agarwal and V. K. Gupta, J. Mol. Liq., 2016, 214, 249–258 CrossRef CAS.
- H. Benaïssa and M. Elouchdi, J. Hazard. Mater., 2011, 194, 69–78 CrossRef PubMed.
- L. Shi, D. Wei, H. H. Ngo, W. Guo, B. Du and Q. Wei, Bioresour. Technol., 2015, 194, 297–304 CrossRef CAS PubMed.
- S. Muthusamy and S. Venkatachalam, RSC Adv., 2015, 5, 45817–45826 RSC.
- A. Asfaram, M. Ghaedi and G. R. Ghezelbash, RSC Adv., 2016, 6, 23599–23610 RSC.
- S. Zinjarde, M. Apte, P. Mohite and A. R. Kumar, Biotechnol. Adv., 2014, 32, 920–933 CrossRef CAS PubMed.
- R. Lanciotti, A. Gianotti, D. Baldi, R. Angrisani, G. Suzzi, D. Mastrocola and M. Guerzoni, Bioresour. Technol., 2005, 96, 317–322 CrossRef CAS PubMed.
- A. Esmaeili, E. Hejazi and Y. Vasseghian, RSC Adv., 2015, 5, 91776–91784 RSC.
- D. Podstawczyk, A. Witek-Krowiak, A. Dawiec and A. Bhatnagar, Ecol. Eng., 2015, 83, 364–379 CrossRef.
- A. Asfaram, M. Ghaedi, S. Hajati, A. Goudarzi and E. A. Dil, Ultrason. Sonochem., 2017, 34, 1–12 CrossRef CAS.
- F. Ghorbani, H. Younesi, S. M. Ghasempouri, A. A. Zinatizadeh, M. Amini and A. Daneshi, Chem. Eng. J., 2008, 145, 267–275 CrossRef CAS.
- C. Han, H. Pu, H. Li, L. Deng, S. Huang, S. He and Y. Luo, J. Hazard. Mater., 2013, 254, 301–309 CrossRef PubMed.
- A. Witek-Krowiak, K. Chojnacka, D. Podstawczyk, A. Dawiec and K. Pokomeda, Bioresour. Technol., 2014, 160, 150–160 CrossRef CAS PubMed.
- N. Prakash, S. Manikandan, L. Govindarajan and V. Vijayagopal, J. Hazard. Mater., 2008, 152, 1268–1275 CrossRef CAS PubMed.
- M. Ghaedi, S. Hajati, B. Barazesh, F. Karimi and G. Ghezelbash, J. Ind. Eng. Chem., 2013, 19, 227–233 CrossRef CAS.
- M. Ghaedi, N. Zeinali, A. Ghaedi, M. Teimuori and J. Tashkhourian, Spectrochim. Acta, Part A, 2014, 125, 264–277 CrossRef CAS PubMed.
- T. Mitra, B. Singha, N. Bar and S. K. Das, J. Hazard. Mater., 2014, 273, 94–103 CrossRef CAS PubMed.
- E. A. Dil, M. Ghaedi, A. Ghaedi, A. Asfaram, M. Jamshidi and M. K. Purkait, J. Taiwan Inst. Chem. Eng., 2016, 59, 210–220 CrossRef CAS.
- A. Asfaram, M. Ghaedi, F. Yousefi and M. Dastkhoon, Ultrason. Sonochem., 2016, 33, 77–89 CrossRef CAS PubMed.
- A. Asfaram, M. Ghaedi, S. Hajati and A. Goudarzi, Ultrason. Sonochem., 2016, 32, 418–431 CrossRef CAS PubMed.
- A. Asfaram, M. Ghaedi, S. Hajati and A. Goudarzi, RSC Adv., 2015, 5, 72300–72320 RSC.
- E. A. Dil, M. Ghaedi, A. Ghaedi, A. Asfaram, A. Goudarzi, S. Hajati, M. Soylak, S. Agarwal and V. K. Gupta, J. Ind. Eng. Chem., 2016, 34, 186–197 CrossRef CAS.
- A. Asfaram, M. Ghaedi, M. H. A. Azqhandi, A. Goudarzi and M. Dastkhoon, RSC Adv., 2016, 6, 40502–40516 RSC.
- A. Asfaram, M. Ghaedi, A. Goudarzi and M. Rajabi, Dalton Trans., 2015, 44, 14707–14723 RSC.
- A. Asfaram, M. Ghaedi, S. Hajati, M. Rezaeinejad, A. Goudarzi and M. K. Purkait, J. Taiwan Inst. Chem. Eng., 2015, 53, 80–91 CrossRef CAS.
- M. Ghaedi, A. Ansari and R. Sahraei, Spectrochim. Acta, Part A, 2013, 114, 687–694 CrossRef CAS PubMed.
- A. S. Saini and J. S. Melo, Bioresour. Technol., 2013, 149, 155–162 CrossRef CAS PubMed.
- İ. A. Şengil, M. Özacar and H. Türkmenler, J. Hazard. Mater., 2009, 162, 1046–1052 CrossRef PubMed.
- P. Thilagavathy and T. Santhi, Chin. J. Chem. Eng., 2014, 22, 1193–1198 CrossRef CAS.
- M. Fereidouni, A. Daneshi and H. Younesi, J. Hazard. Mater., 2009, 168, 1437–1448 CrossRef CAS PubMed.
- N. Rajamohan, M. Rajasimman and M. Dilipkumar, J. Taiwan Inst. Chem. Eng., 2014, 45, 2622–2627 CrossRef CAS.
- Y. Kacar, Ç. Arpa, S. Tan, A. Denizli, Ö. Genç and M. Y. Arıca, Process Biochem., 2002, 37, 601–610 CrossRef CAS.
- E. Khoramzadeh, B. Nasernejad and R. Halladj, J. Taiwan Inst. Chem. Eng., 2013, 44, 266–269 CrossRef CAS.
- Y. Zeroual, A. Moutaouakkil, F. Z. Dzairi, M. Talbi, P. U. Chung, K. Lee and M. Blaghen, Bioresour. Technol., 2003, 90, 349–351 CrossRef CAS PubMed.
- M. Tuzen, A. Sari, D. Mendil and M. Soylak, J. Hazard. Mater., 2009, 169, 263–270 CrossRef CAS PubMed.
- S. W. Al Rmalli, A. A. Dahmani, M. M. Abuein and A. A. Gleza, J. Hazard. Mater., 2008, 152, 955–959 CrossRef CAS PubMed.
- L. Zhou, Y. Wang, Z. Liu and Q. Huang, J. Hazard. Mater., 2009, 161, 995–1002 CrossRef CAS PubMed.
- A. Fakhri, Process Saf. Environ. Prot., 2015, 93, 1–8 CrossRef CAS.
- I. Tüzün, G. Bayramoğlu, E. Yalçın, G. Başaran, G. Celik and M. Y. Arıca, J. Environ. Manage., 2005, 77, 85–92 CrossRef PubMed.
- S. Basha, Z. Murthy and B. Jha, Biorem. J., 2011, 15, 26–34 CrossRef CAS.
- M. Xu, P. Yin, X. Liu, X. Dong, Y. Yang, Z. Wang and R. Qu, Desalin. Water Treat., 2013, 51, 4546–4555 CrossRef CAS.
|
This journal is © The Royal Society of Chemistry 2016 |