DOI:
10.1039/C5RA27378A
(Paper)
RSC Adv., 2016,
6, 39963-39971
Monodisperse magnetic mesoporous silica microspheres facilitate the studies of gastric cancer-specific peptides in sera†
Received
21st December 2015
, Accepted 14th April 2016
First published on 18th April 2016
Abstract
Background: The prognosis of gastric cancer remains poor despite the recent improvements in therapies. The aim of this study was to develop a novel strategy to discover gastric cancer-specific peptides in sera. Methods: Here, Fe3O4@mSiO2 microspheres were prepared to separate and enrich serum peptides in patients with gastric cancer. Stable isotope labeling coupled with LC-MALDI then quantified those differentially expressed peptides. In contrast with the commonly used strategy, this novel strategy was more efficient and sensitive, which also enabled the accurate identification and quantification of serum peptides. Results: Based on this strategy, the results showed that serum CK18, apoE, C3 precursor, Clusterin, C4a, HMW-kininogen, apoA-4 and ghrelin peptides were differentially expressed significantly in patients, especially when there had been no available information concerning the serum level of active ghrelin in predicting gastric cancer. In addition, our data demonstrated that serum active ghrelin expression was closely correlated with shorter survival in patients which would show biological activity for promotion of lymph node metastasis. Conclusions: Active ghrelin might be a novel circulating biomarker in the prognosis of gastric cancer.
1 Introduction
Biomarkers are molecules that are objectively measured and evaluated as indicators of biological and pathological processes or pharmacological responses to a therapeutic treatment. Biomarkers possess great promise for the detection, diagnosis, and prognosis of cancer due to their ability in identifying unique molecular signatures detrimental to certain patho-physiological states.1 An ideal biomarker has high sensitivity and specificity for diagnosis, its expression level should correlate with the disease status and response to treatment, and it should be easily and reproducibly measured. However, most candidate biomarkers currently available for the prognosis of solid tumors rarely fulfill all the above criteria and, therefore, are not presently recommended for screening of the general population.2
Gastric cancer is one of the leading causes of cancer related death worldwide. The prognosis of gastric cancer remains poor despite the recent improvements in bioanalytic chemistry.3 The level of biomarkers is expected to be measured using less invasive methods and monitored easily, for example, by a simple blood test. Several serum biomarkers have been used in gastric cancer. Carcino-embryonic antigen (CEA) and carbohydrate antigen (CA) 19-9 are established as biomarkers for advanced gastric cancer. However, these biomarkers were unable to detect early stage gastric cancer; some patients with advanced gastric cancer even had normal serum levels of these biomarkers. Thus, the development of novel biomarkers for gastric cancer that can be routinely used in clinical practice is required.
Circulating macromolecules of cell death have been proposed as useful indicators for patients with cancer and the other critical illness.4,5 The levels of nucleosomal DNA, Fasligand, cytochrome c, and a variety of cytoskeletal components have been measured upon cancer cell death.6–8 Intracellular proteins are released from dying tumor cells, and the free-floating form of these proteins (cleaved or non-cleaved) could be detectable in the patient blood as a potential biomarker of cancer happening.9
The emergence of mass spectrometry (MS)-based signatures as biomarkers has generated considerable enthusiasm among oncologists. MS techniques permit the analysis of hundreds of small- to medium-sized peptides from only microliters of sera.10 Several reports have highlighted MS-based determinations of specific peptide patterns that indicate the presence of tumors with very high sensitivity and specificity. These studies have been performed on high resolution MS instruments, when MALDI-TOF permits the MS/MS sequence of a larger number of peptides due to its high performance of precursor ion selection.11 However, MS-based solutions can't real-time quantify differentially expressed peptides without adequate internal standards. The commonly used strategy mainly adopts stable isotopes to label serum peptides, then identify and quantify those differentially expressed peptides by MS-based solutions.12 Unfortunately, it still can't avoid the loss of low abundant peptides without effective enrichment.
Recently, ordered mesoporous silica materials have been successfully applied for the separation of peptides from complex biological matrices based on the combination of size-exclusion mechanism and the hydrophobic interaction with siloxane bridge group mainly on the surface of inside pores.13 Meanwhile, the combination of mesoporous morphology and magnetic property can be applied to the enrichment of low abundant endogenous peptides, making the separation of trapped peptides from sample solutions convenient and effective.14 Today, the concept of pseudomorphic transformation (P-T) has been applied to various particle sizes of amorphous silica to produce ordered mesoporous silica, because pseudomorphic synthesis can independently optimize the properties of particle shaping and mesophase self-assembly.15,16 Briefly, Fe3O4 particles were coated with silica shell by a sol–gel method, followed by pseudomorphic synthesis to transform nonporous silica shell into ordered mesoporous silica shell.17
Considering the advantages of magnetic mesoporous silica particles, herein, we reported a novel application of monodisperse Fe3O4@mSiO2 microspheres in the studies of circulating gastric cancer-specific peptides. Meanwhile, we compared our novel strategy with the current strategy.
2 Materials and methods
2.1 Preparation of Fe3O4@mSiO2 microspheres
Magnetic microspheres coated with nonporous silica (Fe3O4@nSiO2) were synthesized through Stöber method with minor modification.18,19 Briefly, Fe3O4 (120 mg) was homogeneously dispersed in a mixture of ethanol (467 mL), deionized water (139 mL) and NH3·H2O solution (15 mL). Then this dispersion was homogenized by vigorously stirring for 30 min. Finally, under continuous mechanical stirring, tetraethyl orthosilicate (TEOS, 6 mL) was slowly added to this dispersion, and after stirring 8 h at room temperature, silica was formed on the surface of Fe3O4 through hydrolysis and condensation of TEOS. The Fe3O4@nSiO2 particles were washed by ethanol and deionized water several times, and then dried in vacuum at 60 °C for 8 h. The mass fraction of silica in Fe3O4@nSiO2 can be estimated via the mass difference between Fe3O4 and Fe3O4@nSiO2. Fe3O4@nSiO2 spheres were converted into Fe3O4@mSiO2 particles according to pseudomorphic transformations as previously reported (Fig. 1).17 The P-T procedure was directly adapted from the synthesis of mobil composition of matter no. 41 (MCM-41), by using silica shell of Fe3O4@nSiO2 particles as the source of silica.15 In the P-T step, silica was dissolved by the alkaline solution, and the resulting silicate species interacted with the surfactant cetyltrimethylammonium bromide (CTAB) and condensed into ordered mesophase. When the molar ratio of all components was 1 SiO2
:
0.2 NaOH
:
0.1 CTAB
:
80H2O, Fe3O4@nSiO2 spheres (0.4 g of SiO2) were added to a mixture of CTAB (0.243 g), water (9.6 mL), and NaOH (0.054 g) and stirred 30 min at room temperature. The hydrothermal reaction was carried out in a Teflon-lined autoclave at 130 °C for 24 h. The products were recovered with the help of magnet and purified by multiple cycles of washing with ethanol and deionized water. Therefore, magnetic nonporous silica microspheres were transformed into magnetic CTAB silica microspheres. Finally, the purified microspheres were redispersed in 50 mL of NH4NO3 ethanol solution (0.2 wt%) and heated 0.5 h at 60 °C to remove the template CTAB. This step was repeated 6 times and CTAB was successfully removed. After the microspheres were washed twice with deionized water, Fe3O4@mSiO2 microspheres were finally obtained.
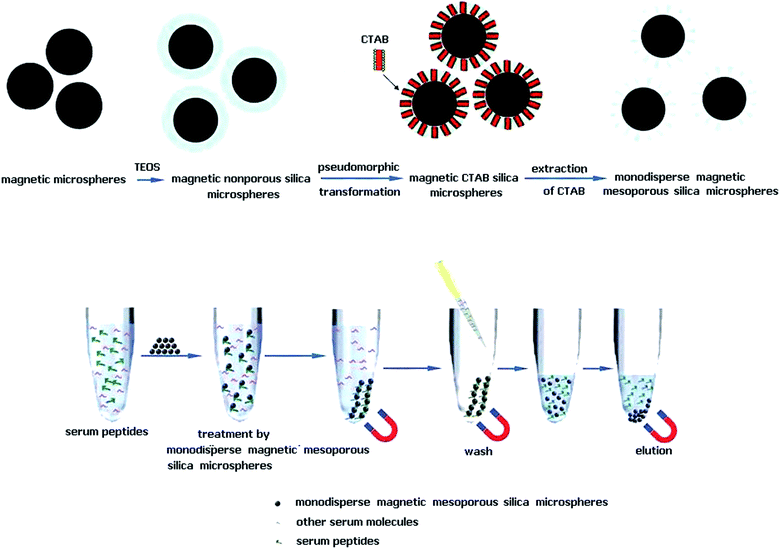 |
| Fig. 1 Synthesis and usage of monodisperse magnetic mesoporous silica microspheres. | |
2.2 Characterization of Fe3O4@mSiO2 microspheres
Scanning electron microscopy image was taken using JSM-6700F field emission scanning electron microscope (FESEM, JEOL, Japan). Nitrogen sorption measurement was performed at 77 K using a JW-BK surface area and pore size analyzer (JWGB Sci. & Tech., Beijing, China). The composites were activated by evacuating in vacuum and heating to 423 K for 6 h to remove any physically adsorbed substances before analysis. The specific surface area value was calculated according to the BET (Brunauer–Emmett–Teller) equation at P/P0 between 0.05 and 0.3. The pore parameters (pore volume and pore diameter) were evaluated from the desorption branch of isotherm based on BJH (Barrett–Joyner–Halenda) model.
2.3 Patients and serum collection
We enrolled 48 patients diagnosed with gastric cancer confirmed by histological examination at Jiangsu Academy of TCM; blood samples of the patients were collected (Table 1). The disease status of patients was assessed according to the American Joint Commission on Cancer (AJCC)/International Union against Cancer (UICC) TNM classification. Patients with a history of malignancies, diabetes mellitus, or uncontrolled infection were excluded from the study. Control blood samples were collected from 12 healthy volunteers [age: 35–65 (median: 55); 8 males and 4 females]. Blood samples of patients were collected before any cancer therapy. Blood samples collected from patients and controls were stored into dry tubes. After clotting, the blood samples were centrifuged at 3000g for 10 min to separate the sera. The sera were stored at −80 °C until analysis. Written informed consent was obtained before starting the study from all patients and healthy volunteers for using data obtained from hematological and clinical examinations to be used for research purpose. This study was approved by the Medical Ethics Committee of Jiangsu Academy of TCM, and was performed in compliance with the Declaration of Helsinki.
Table 1 Patient characteristics
Patients |
Number (N = 48) |
According to the AJCC Cancer Staging Handbook 6th edition. |
Gender |
Female |
16 |
Male |
32 |
![[thin space (1/6-em)]](https://www.rsc.org/images/entities/char_2009.gif) |
Age (year) |
Median |
63 |
Range |
40–72 |
![[thin space (1/6-em)]](https://www.rsc.org/images/entities/char_2009.gif) |
Invasiona |
T1 |
22 |
T2 |
14 |
T3 |
8 |
T4 |
4 |
![[thin space (1/6-em)]](https://www.rsc.org/images/entities/char_2009.gif) |
Lymph node metastasisa |
N0 |
19 |
N1 |
7 |
N2 |
9 |
N3 |
13 |
![[thin space (1/6-em)]](https://www.rsc.org/images/entities/char_2009.gif) |
Clinical stagea |
Stage I |
20 |
Stage II |
8 |
Stage III |
6 |
Stage IV |
14 |
2.4 Serum sample pretreatments prior to MALDI MS analysis
Human serum (50 μL) was diluted with 350 μL H2O, and then was processed by Pierce Albumin and IgG removal kit to decrease the abundant albumin and antibody components of human serum samples. On the other hand, human serum (50 μL) was diluted with 350 μL H2O, and then 10 μL suspension of Fe3O4@mSiO2 microspheres (5 mg mL−1) was added. Then, the supernatant was decanted and the microspheres were collected and rinsed. After that, the adsorbed peptides were eluted with 10 μL 0.1% TFA-50% ACN. Both of them were in preparation for LC-MALDI analysis.
2.5 Stable isotope-labeled peptidomic quantification
4,6-Dimethoxypyrimidine-2-isothiocyanate (DMPITC) tagging was performed on pretreated serum samples according to the method described previously.20 Briefly, protein extracts of each sample was labeled with isotopically [d0]-DMPITC (healthy volunteers) or [d6]-DMPITC (patients with gastric cancer) reagent. DMPITC-tagged proteins were then mixed and desalted by MonoTip C18 (Shimadzu Inc, Kyoto). Here, a synthetic peptide corresponding to the fragment between residues 14 and 38 (“TQCPDDSTCCELPTGK”) of mouse Granulin3 was used an internal standard. Desalted peptides were separated by a Shimadzu prominence nano-liquid chromatography system with a flow rate ranging from 1 nL min−1 to 5 μL min−1. The sample was processed by Prominence nano-HPLC system (Shimadzu Inc, Kyoto), which was firstly loaded onto the trap column (L-column 2 ODS), then the trapped samples were separated via RP column (MonoCap C-18 column). Eluates were automatically deposited onto MALDI target plates by the LC spotting system (AccuSpot, Shimadzu Inc, Kyoto). These spotted samples were automatically analyzed by MALDI-TOF/TOF (Shimadzu Kratos, Manchester). Data acquisition and processing were performed using Shimadzu Biotech MALDI-MS Launchpad v 2.9 software. For MS data, only the [d0]/[d6]-DMPITC-labeled peptides with a signal-to-noise (S/N) ratio of more than 10 were treated as positive signals used for data analysis. Relative ratios between [d0]-DMPITC- and [d6]-DMPITC-tagged peptides were submitted to independent sample T-test (SPSS 17.0) to calculate the differentially expressed peptides. A p value of less than 0.05 was regarded as significant. The peptides were identified by searching the MS/MS spectra against the Swissprot database using a local Mascot search engine with the following parameters: Homo sapiens, no cleavage, fixed modification [d0]-/[d6]-DMPITC at any N-terminus, MS tolerance of 0.1 Da, MS/MS tolerance of 0.3 Da, and peptide charge of +1.
2.6 ELISA quantification of ghrelin (active or total)
Serum levels of total ghrelin were assayed by an ELISA kit (Bioworld Inc, Minnesota), which is a one-step in vitro immuno-assay for the quantitative determination of total soluble ghrelin in the serum or plasma. Serum levels of active ghrelin (ghrelin peptide 24-51, octanoyl-modified) were also assayed by an ELISA kit (Bioworld Inc, Minnesota), which is a one-step in vitro immunoassay for the quantitative determination of the octanoyl-modified ghrelin Ser26 neoepitope in serum and plasma.
2.7 Statistical analysis
The statistical data were analyzed using SPSS 17.0 software (SPSS Inc). Differences were considered statistically significant when P values were <0.05. In order to evaluate the performance of serum active ghrelin antigen levels on the prediction of clinical response, Receiver Operator Curve (ROC) analysis was performed. The sensitivities, specificities, positive/negative predictive values and area under curves (AUC) of serum active ghrelin values were calculated using ROC analysis for death. AUC (>0.5) means good diagnostic performance of the test. The cut-off values were determined using the MedCalc demo program. The overall survival (OS) is defined as the time from initial diagnosis to death due to any cause or the date of last follow up. Survival curves were calculated using the Kaplan–Meier method and compared using the log-rank test. The exact χ2 test was used to evaluate the association of serum active ghrelin expression with lymph node metastasis in patients with gastric cancer.
3 Results
3.1 Characterization of Fe3O4@mSiO2 microspheres
Fig. 2A showed that prepared Fe3O4@mSiO2 microspheres were discrete and uniform in size and shape, so the P-T transformation didn't destroy the macroscopic morphology of these microspheres. The N2 sorption–desorption isotherms of Fe3O4@mSiO2 microspheres were measured (Fig. 2B). The Brunauer–Emmett–Teller (BET) surface area of Fe3O4@mSiO2 microspheres was found to be 450 mm2 g−1 and the pore volume of was 0.61 cm3 g−1. In the P-T step, the function of the alkaline solution was to dissolve silica, so increasing the amount of NaOH could promote more proportion of the silica shell dissolved and then converted into surfactant-silica mesophase, which resulted in larger surface area and pore size for the adsorption and separation of peptides from biological matrices. As mentioned previously, when the ratio of NaOH/SiO2 was raised to 0.25, the structure of silica shell would be gradually destroyed.17 Thus, the recommended value of NaOH/SiO2 in the pseudomorphic synthesis should be inferior to 0.25. Typically, we selected those Fe3O4@mSiO2 microspheres prepared under the condition that the molar ratio of NaOH/SiO2 was 0.2, for future characterizations and applications. Moreover, the nonporous pore structure was converted into ordered mesostructure after P-T reaction, and this could contribute to the high efficiency enrichment of peptides by microspheres. Typically, N2 sorption–desorption isotherms indeed revealed IV-type curve for prepared Fe3O4@mSiO2 microspheres (Fig. 2B), indicating the presence of mesostructure. The narrow and sharp pore size distribution curve (Fig. 2C), which centered at the mean value of 2.9 nm, suggested that the mesopores had very uniform sizes.
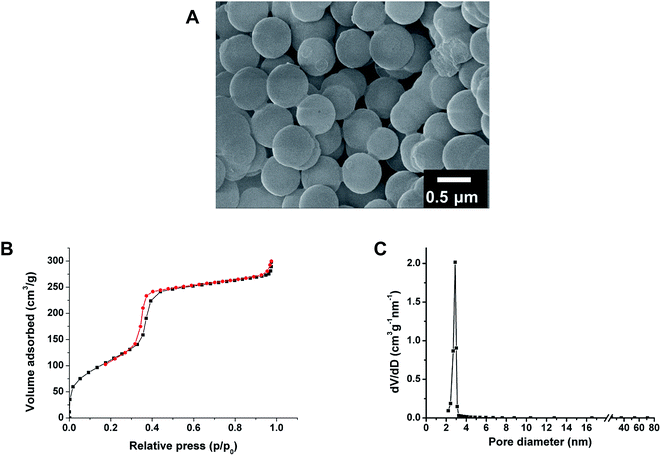 |
| Fig. 2 Characterization of monodisperse magnetic mesoporous silica microspheres, SEM image (A); N2 adsorption–desorption isotherms (B); and pore size distribution (C) of monodisperse magnetic mesoporous silica microspheres. | |
3.2 Enrichment of serum peptides by Fe3O4@mSiO2 microspheres
To further confirm their superior efficiency for peptide enrichment, Fe3O4@mSiO2 microspheres were used to extract endogenous peptides from human serum. Direct identification of peptides in human serum by MALDI-TOF MS analysis was difficult (Fig. 3A and B), due to three factors including high-concentration salts, high-abundant proteins and other organic molecules. However, after the enrichments with Fe3O4@mSiO2, many peptides were clearly observed in the spectra (Fig. 3C and D). More importantly, the difference of mass spectra from human serum peptides between healthy volunteers and patients with gastric cancer could be clearly observed after the enrichments by Fe3O4@mSiO2, indicating its potential use in biomarker discovery. As shown in methods, the serum samples were directly diluted for subsequent enrichments without any other processes to remove high-concentration proteins or salts, because the high-MW molecules were excluded with the microspheres and the salts or other organic contaminants could be eliminated during the washing step. The data markedly suggested that these Fe3O4@mSiO2 microspheres were capable of the rapid and effective separation of serum peptides.
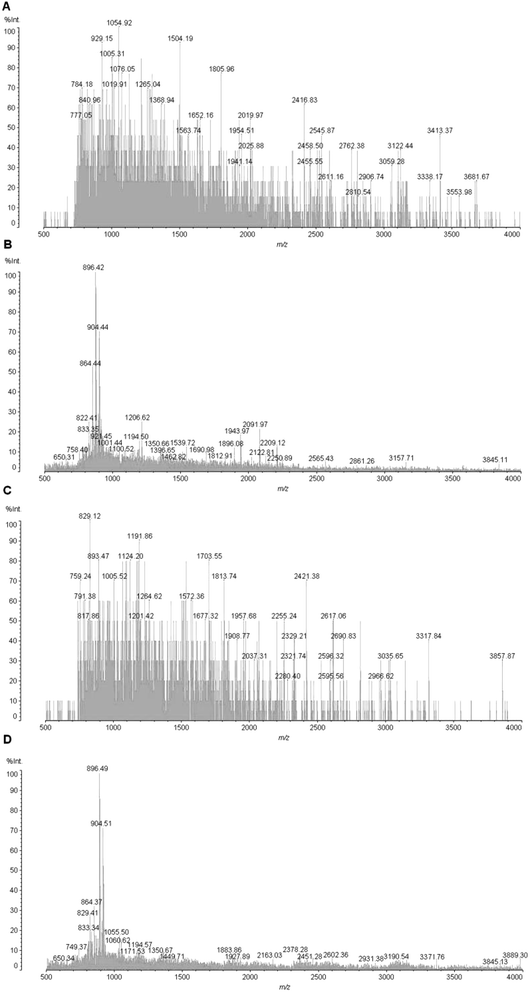 |
| Fig. 3 Microsphere enrichments greatly enhanced the efficiency and sensitivity of MS-based peptide identification. MALDI-TOF merged spectra of human serum from healthy volunteers treated without (A), and with magnetic mesoporous silica microspheres (B); MALDI-TOF merged spectra of human serum from patients with gastric cancer treated without (C), and with magnetic mesoporous silica microspheres (D). | |
3.3 Differentially expressed peptidomic quantification by the current strategy
Here, DMPITC-labeled peptidomic measurement was performed. After the ablation of the abundant albumin and antibody components of human serum samples, the serum protein extracts were subjected to desalting, isotope labeling and offline nanoLC-MALDI quantification, in which N-terminus of serum peptides were labeled with [d0]-DMPITC- or [d6]-DMPITC-tag specifically (Fig. 4). Then, the relative ratios of serum protein expressions were estimated from the intensity of paired peaks with mass difference of 6 Da derived from [d0]-DMPITC- and [d6]-DMPITC-tagged peptides in mass spectra. As a result, a total of 44 peptides (15 proteins) were identified accurately and sequenced (Table S1†). Finally, the differentially expressed peptides of four proteins were detected with statistical significance (p < 0.05). Clusterin, C4a, and apoA-4 peptides were highly expressed, while C3 precursor peptide was lowly expressed in patients with gastric cancer.
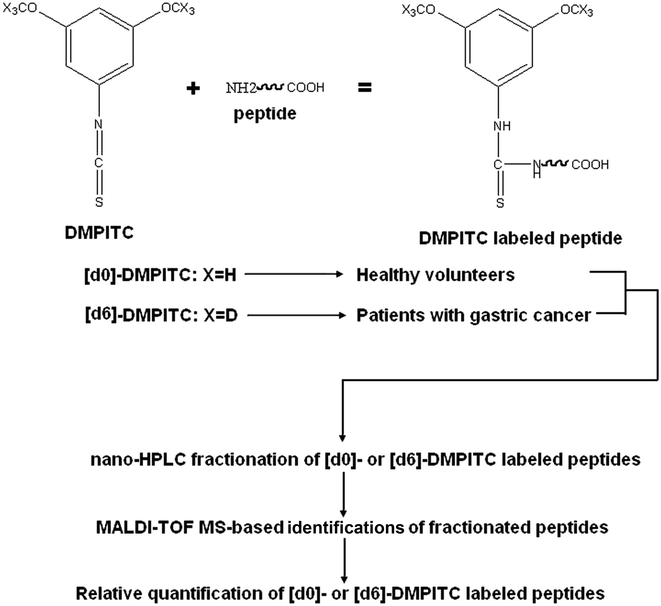 |
| Fig. 4 Workflow of DMPITC-labeled peptidomic quantification. | |
3.4 Differentially expressed peptidomic quantification by our novel strategy
Here, DMPITC-labeled peptidomic measurement was also performed by comparison with the commonly used strategy stated above. After the enrichment of serum peptides by Fe3O4@mSiO2 microspheres, the serum peptides were subjected to isotope labeling and offline nanoLC-MALDI quantification, in which N-terminus of serum peptides were labeled with [d0]-DMPITC- or [d6]-DMPITC-tag specifically. Then, the relative ratios of serum protein expressions were estimated from the intensity of paired peaks with mass difference of 6 Da derived from [d0]-DMPITC- and [d6]-DMPITC-tagged peptides in mass spectra. As a result, a total of 96 peptides (24 proteins) were identified accurately and sequenced (Table S2†). Finally, the differentially expressed peptides of eight proteins were selected with statistical significance (p < 0.05). CK18, Clusterin, C4a, HMW-kininogen, apoA-4, apoE, and ghrelin peptides were highly expressed, while C3 precursor peptide was lowly expressed in patients with gastric cancer. These changes were consistent with previous quantitative results. However, this novel strategy yielded more data, which was more sensitive and efficient than the current strategy. More interestingly, the ghrelin peptide detected here was just active ghrelin (ghrelin fragment 24-51, octanoyl-modified).
3.5 Serum ghrelin assessment as a potential biomarker
Hitherto, serum ghrelin expression was rarely investigated in previous studies of gastric cancer. The serum levels of total ghrelin or active ghrelin in surviving patients (n = 15) were compared to those obtained from dead patients (n = 33) by ELISA quantification. It was demonstrated that the serum levels of active ghrelin in dead patients were significantly higher than those of surviving patients (p < 0.05, Fig. 5). These changes were also consistent with our quantitative peptidomic results. The mean active ghrelin levels of dead and surviving patients were 0.095 nM and 0.057 nM, respectively. The relationship between serum active ghrelin levels and patient survival was analyzed. For this purpose, the serum active ghrelin levels of 48 patients (15 surviving, 33 dead) were evaluated by ROC analyses (Fig. 6). The best cut-off value for prediction of death was 0.045 nM where AUC = 0.69 (95% CI = 0.52–0.83). The sensitivities, specificities, positive predictive values, positive likelihood ratios of baseline serum active ghrelin values calculated using ROC analysis for death were 82.1, 60.5, 82.1% and 2.15, respectively. Mean survival time for 48 patients was 20 months and probability for 24 month survival was 33.3%. Next, the relationship between serum active ghrelin levels and survival was analyzed by the Kaplan–Meier survival curve (Fig. 7). When the cut-off value taken 0.045 nM, the patients with lower active ghrelin levels (n = 14) survived for 32 months, while those with higher active ghrelin levels (n = 34) survived for 19 months. In the groups, probabilities for 24 month survival were 63.4 and 23.2%, respectively. It revealed that the mean survival of patients with lower active ghrelin levels was statistically significantly higher than those with higher active ghrelin levels (p = 0.014, log-rank test). In previous investigations, ghrelin activation was able to trigger multiple downstream signaling events in cells, including calcium mobilization, protein kinase C (PKC) activation, and matrix metalloproteinase-2 (MMP2) expression, where MMP2 expression has been often linked with tumor invasion, angiogenesis, and metastasis.21 Thus, we further analyzed the correlation between serum active ghrelin expression and lymph node metastasis. It was shown that serum active ghrelin expression was significantly associated with lymph node metastasis (p = 0.021), indicating that it might be involved in the lymph node metastasis of magliant gastric tumors (Table 2).
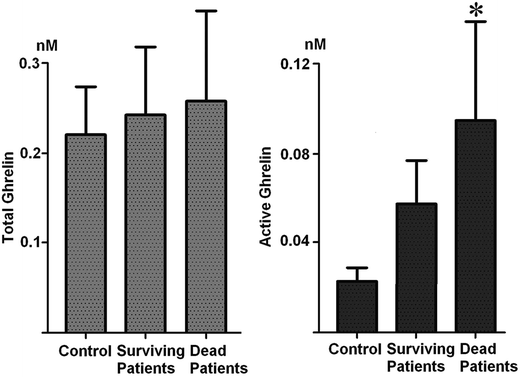 |
| Fig. 5 Serum levels of total ghrelin and active ghrelin in healthy volunteers and patients with gastric cancer (*p < 0.05). | |
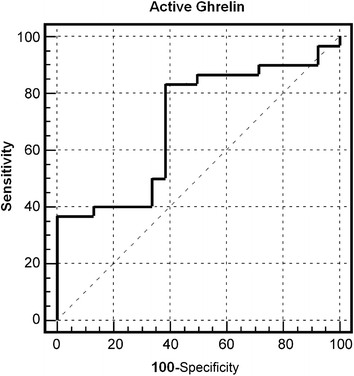 |
| Fig. 6 Prediction of death by ROC analysis: the best cut-off value for prediction of death was 0.045 nM where AUC = 0.69 (95% CI = 0.52–0.83). | |
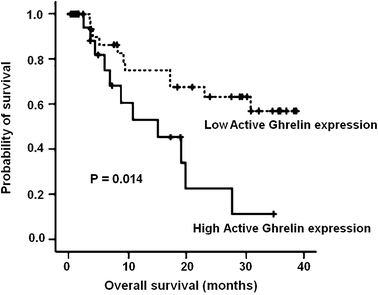 |
| Fig. 7 Kaplan–Meier survival curves were plotted based on serum active ghrelin level. Patients with high active ghrelin expression showed significantly shorter survival than those with low active ghrelin expression tumor (p = 0.014; log-rank test). | |
Table 2 The relationship between serum active ghrelin expression and lymph node metastasis
Lymph node metastasis |
High expression group (n = 34) |
Low expression group (n = 14) |
P value a |
Exact χ2 test. |
Absent |
11 (32.4%) |
8 (57.1%) |
0.021 |
Present |
23 (67.6%) |
6 (42.9%) |
4 Discussions
The commonly used strategy usually obtains limited information on serum peptidome due to the low abundant peptides and interference from serum matrix. A promising alternative step to the current strategy is effective enrichment of serum peptides.
Since monodisperse Fe3O4@mSiO2 owned the optimized properties of monodispersion, magnetization and mesostructure, we applied it into the rapid and selective enrichment of peptides in the sera of patients with gastric cancer here. Contrasted with the commonly used strategy, our novel strategy possessed three advantages in this study: (1) it avoided the treatment with albumin/IgG removal kit and desalting. Instead, it only required the enrichment by microspheres. Generally, it cost approximately 5 min per sample on sample pretreatment, while the current strategy cost 30 min per sample at least; (2) it yielded more data than the current strategy, probably because some sample pretreatments would bring in the loss of some low-abundant peptides, including ablation of albumin/IgG from sera and desalting of isotope-labeled peptides; (3) it improved the S/N ratios of low abundant peptides which were hardly detected due to the ion suppression resulting from high-abundant peptides, because the effective enrichments greatly enhanced the sensitivity of MS-based detections.
Based on our novel strategy, the results showed that C3 precursor, CK18, Clusterin, C4a, HMW-kininogen, apoA-4, apoE and ghrelin peptides were differentially expressed in the sera of patients with gastric cancer significantly. CK18 is usually expressed during oncogenic transformation, and its expression is commonly correlated with lymph node metastases, tumor differentiation, and tumor invasion of gastric cancer.22 ApoE was predominantly expressed in gastric cancer. Tumors with high apoE mRNA expression showed deeper tumor invasion into the muscle layer, the serosal layer, or more positive lymph node metastasis.23 Liu W. T., et al. previously adopted 2-dimensional difference gel electrophoresis (2D-DIGE) to characterize protein biomarkers with tandem mass spectrometry in human sera.24 They also confirmed that Clusterin, C4a, HMW-kininogen, and apoA-4 proteins were highly expressed, while C3 precursor protein was lowly expressed in patients with gastric cancer obviously.
Active ghrelin, a 28 amino acid peptide hormone with diverse physiological roles, is produced and secreted by gastrointestinal tract, which is the endogenous ligand for the GH secretagogue receptor (GHS-R). Only active ghrelin can activate GHS-Rs and release growth hormone to regulate cell growth and proliferation.25 GHS-Rs can trigger several downstream signaling events in cells, including calcium mobilization, protein kinase C (PKC) activation, and matrix metalloproteinase-2 (MMP2) expression.21 ghrelin and the GHS-Rs are expressed in a variety of normal and tumor tissues. While the roles of ghrelin and the GHS-Rs in normal tissues are well studied, their roles in cancer are elusive. Recent reports reveal that ghrelin is overexpressed in multiple malignant carcinoma cells, and plays important roles in tumorigenesis.26 Chuang T. et al. demonstrated that ghrelin signaling promoted the oncogene CDK6 gene expression and repressed the tumor suppressor gene p53 protein expression in gastric cancer. Meanwhile, ghrelin could up-regulate the metastasis factor MMP2 expression via GHS-R/NF-κB signaling pathway in gastric cancer cells and promote tumor cells migration and invasion, indicating that ghrelin signaling was a critical pathway in cancer metastasis.27 Here, the results also indicated that highly expressed active ghrelin would show biological activity for promotion of lymph node metastasis in gastric cancer.
5 Conclusions
In conclusion, our data showed that Fe3O4@mSiO2 microspheres-based enrichments of serum peptides coupled with stable isotope labeled peptidomic quantification could contribute to the studies of gastric cancer-specific peptides in sera. Further analyses using this novel strategy demonstrated that serum active ghrelin was highly expressed and closely correlated with shorter survival in patients, which might be a novel circulating biomarker in the prognosis of gastric cancer.
Acknowledgements
This work was supported by National Nature Science Foundation of China (Grant No. 81001299) and sponsored by Nanjing Newtop Biotech Co., Ltd.
References
- S. Srivastava and R. G. Srivastava, J. Proteome Res., 2005, 13, 1098–1103 CrossRef PubMed.
- L. C. Scott, T. R. Evans, J. Cassidy, S. Harden, J. Paul, R. Ullah, V. O'Brien and R. Brown, Br. J. Cancer, 2009, 101, 410–417 CrossRef CAS PubMed.
- A. Jemal, R. Siegel, E. Ward, Y. Hao, J. Xu, T. Murray and M. J. Thun, CA-Cancer J. Clin., 2008, 58, 71–96 CrossRef PubMed.
- S. Holdenrieder, P. Stieber, H. Bodenmüller, M. Busch, G. Fertig, H. Fürst, A. Schalhorn, N. Schmeller, M. Untch and D. Seidel, Int. J. Cancer, 2001, 95, 114–120 CrossRef CAS PubMed.
- G. A. Roth, C. Krenn, M. Brunner, B. Moser, M. Ploder, A. Spittler, L. Pelinka, T. Sautner, E. Wolner, G. Boltz-Nitulescu and H. J. Ankersmit, Shock, 2004, 22, 218–220 CrossRef CAS PubMed.
- S. Holdenrieder, P. Stieber, J. Von Pawel, H. Raith, D. Nagel, K. Feldmann and D. Seidel, Ann. N. Y. Acad. Sci., 2006, 1075, 244–257 CrossRef CAS PubMed.
- M. F. Pichon, M. Labroquere, K. Rezai and F. Lokiec, Anticancer Res., 2006, 26, 2387–2392 CAS.
- K. Barczyk, M. Kreuter, J. Pryjma, E. P. Booy, S. Maddika and S. Ghavami, Int. J. Cancer, 2005, 116, 167–173 CrossRef CAS PubMed.
- S. Linder, A. M. Havelka, T. Ueno and M. C. Shoshan, Cancer Lett., 2004, 214, 1–9 CrossRef CAS PubMed.
- E. F. Petricoin, C. Belluco, R. P. Araujo and L. A. Liotta, Nature, 2006, 6, 961–967 CAS.
- K. He, X. Y. Wen, A. L. Li, T. Li, J. Wang, H. X. Wang and N. Wang, PLoS One, 2013, 8, e63724 CAS.
- J. Leng, H. Wang, L. Zhang, J. Zhang, H. Wang, T. Cai, J. Yao and Y. Guo, J. Am. Soc. Mass Spectrom., 2011, 22, 1204–1213 CrossRef CAS PubMed.
- R. Tian, H. Zhang, M. Ye, X. Jiang, L. Hu, X. Li, X. Bao and H. Zou, Angew. Chem., Int. Ed. Engl., 2007, 46, 962–965 CrossRef CAS PubMed.
- S. Liu, H. Chen, X. Lu, C. Deng, X. Zhang and P. Yang, Angew. Chem., Int. Ed. Engl., 2010, 49, 7557–7561 CrossRef CAS PubMed.
- T. Martin, A. Galarneau, F. Di Renzo, F. Fajula and D. Plee, Angew. Chem., Int. Ed. Engl., 2002, 41, 2590–2592 CrossRef CAS.
- B. Lefevre, A. Galarneau, J. Iapichella, C. Petitto, F. Di Renzo and F. Fajula, Chem. Mater., 2005, 17, 601–607 CrossRef CAS.
- G. T. Zhu, X. S. Li, Q. Gao, N. W. Zhao, B. F. Yuan and Y. Q. Feng, J. Chromatogr. A, 2012, 1224, 11–18 CrossRef CAS PubMed.
- L. N. Nagy, N. Abraham, O. Sepsi, E. Hild, D. Cot, A. Ayral and Z. Horvolgyi, Langmuir, 2008, 24, 12575–12580 CrossRef CAS PubMed.
- Y. B. Luo, Z. G. Shi, Q. Gao and Y. Q. Feng, J. Chromatogr. A, 2011, 1218, 1353–1358 CrossRef CAS PubMed.
- J. Leng, D. Zhu, D. Wu, T. Zhu, N. Zhao and Y. Guo, Rapid Commun. Mass Spectrom., 2012, 26, 2555–2562 CrossRef CAS PubMed.
- M. R. Alipour, M. R. Aliparasti, R. Keyhanmanesh, S. Almasi, M. Halimi, K. Ansarin and H. Feizi, J. Endocrinol. Invest., 2011, 34, e369–e373 CAS.
- W. Xu, M. W. Zhang, J. Huang, X. Wang, S. F. Xu, Y. Li and S. J. Wang, World J. Gastroenterol., 2005, 11, 6530–6534 CrossRef CAS PubMed.
- K. Sakashita, F. Tanaka, X. Zhang, K. Mimori, Y. Kamohara, H. Inoue, T. Sawada, K. Hirakawa and M. Mori, Oncol. Rep., 2008, 20, 1313–1319 CAS.
- W. Liu, B. Liu, Q. Cai, J. Li, X. Chen and Z. Zhu, Clin. Chim. Acta, 2012, 413, 1098–1106 CrossRef CAS PubMed.
- M. K. K. Kojima, Physiol. Rev., 2005, 85, 495–522 CrossRef CAS PubMed.
- L. K. Chopin, I. Seim, C. M. Walpole and A. C. Herington, Endocr. Rev., 2012, 33, 849–891 CrossRef CAS PubMed.
- C. Tian, L. Zhang, D. Hu and J. Ji, Mol. Cell. Biochem., 2013, 382, 163–172 CrossRef CAS PubMed.
Footnotes |
† Electronic supplementary information (ESI) available. See DOI: 10.1039/c5ra27378a |
‡ Cao Meng and Zhou Zhen-Xian are co-first authors. |
|
This journal is © The Royal Society of Chemistry 2016 |