DOI:
10.1039/C5RA24911B
(Paper)
RSC Adv., 2016,
6, 13626-13635
Computational insights into different inhibition modes of the κ-opioid receptor with antagonists LY2456302 and JDTic†
Received
24th November 2015
, Accepted 19th January 2016
First published on 22nd January 2016
Abstract
In drug design and discovery, ligand binding kinetics combines pharmacokinetics and pharmacodynamics and more and more attention is paid to it. The κ-opioid G-protein coupled receptor (κ-OR) has been determined to be a promising drug target for the treatment of depression- and anxiety-related diseases. Among the κ-OR selective antagonists, JDTic is a failed antidepressant with a short drug-target residence time (RT), whereas LY2456302 exhibits better effects with a longer RT than JDTic. To investigate the inhibition mechanism of the κ-OR induced by the two ligands, unbiased molecular dynamics and well-tempered metadynamics simulations were performed on JDTic–κ-OR and LY2456302–κ-OR complexes. Through detailed analyses of the simulations, a strong but single interaction mode was found to be responsible for the adverse effects and short RT of JDTic, which could be considered as an alert for other chemotypes, whereas LY2456302 was more advanced, mainly due to its multiple metastable states. Based on Eyring’s equation, the relative RT of LY2456302/JDTic, determined from the activation free energy of dissociation ΔG≠off, was efficiently calculated and was in good agreement with experimental data. Thus, these simulations might be helpful for the further design of antidepressants targeting κ-OR with reasonable RT.
Introduction
The gold standard of higher binding affinity was strongly questioned for long time in drug design and discovery.1,2 Some binding agents with a lower binding affinity to the target but suitable drug-target residence time (RT) have actually exhibited better efficiency.3–5 For instance, maraviroc, an inverse agonist of CCR5, was successfully released into the market essentially due to its long residence time, which took researchers seven years to learn after its approval.6 Based on the formation of a binary complex (RL) through ligand (L)–receptor (R) interaction, the dissociation rate (koff) and association rate (kon) are defined as in eqn (1),3 |
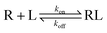 | (1) |
Too short a RT for drug candidates means a high koff and low concentration of RL, which then causes poor oral bioavailability of the ligands, while extremely long receptor occupancy could bring long-term on-target or mechanism-based toxicity,5 which means compound accumulation in tissues and further adverse side effects and outweighs the therapeutic advantages. Therefore, to some extent an appropriate residence time, or ligand binding kinetics, is more important than binding affinity, which has caused drug developers to be interested in adequately obtaining the RTs of a series of compounds in the preclinical stages of drug discovery.7
The ligands targeting G protein-coupled receptors (GPCRs), which are the largest superfamily of drug targets,8 have naturally been taken seriously in terms of their related properties, especially their RTs.9 Besides the above-mentioned CCR5, the ligand RTs of other class A GPCRs were also paid considerable attention to, such as muscarinic receptors, tachykinin receptors and dopamine receptors.5 Furthermore, binding kinetics studies of corticotropin-releasing factor type-1 receptor (CRF1), which belongs to class B GPCRs, were performed by experimental methods.10 In addition, instead of the efficiency induced by the long interaction time of maraviroc, long-time on-target or mechanism-based toxicity has been observed in many GPCR inhibitors, like some dopamine receptor antagonists.11
The κ-opioid receptor, one subtype of opioid receptors and the class A (rhodopsin-like) subfamily of GPCRs, is a very interesting target, because κ-OR selective antagonists have been proven to have therapeutic effects on depression- and anxiety-related diseases.12–15 Notably, κ-OR selective JDTic (Fig. 1 and Table S1†) has a pM binding affinity and entered into phase I clinical trials for cocaine abuse, but terminated due to adverse effects.15 JDTic and other early κ-OR selective antagonists all displayed an abnormal time course characterized by a delay in the onset of action of hours or days and antagonistic effects lasting up to several weeks at minimally-effective doses,16,17 which was identified to be due to activation of the enzyme c-Jun N-terminal kinase 1 (JNK1) with consequent κ-OR functional disruption.18
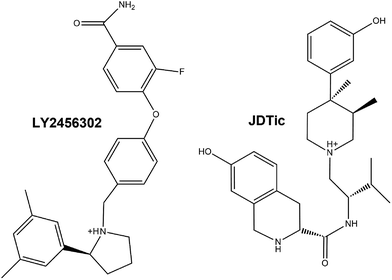 |
| Fig. 1 Two-dimensional structure formulae of the antagonists LY2456302 and JDTic. | |
After efficient modification by Mitch et al., κ-OR selective LY2456302 (ref. 19) successfully passed phase I clinical trials and was advanced to the phase II stage for the augmentation of antidepressant therapy.15,20 Pharmacokinetics data showed that the half-life period (t1/2) of LY2456302 was improved to 3.8 h in PO (peros) (Table S1†),21 which meant that the RT of LY2456302 is 2.53 fold than that of JDTic. Additionally, in Takeuchi’s previous work, the amide group was validated to be detrimental for the potency or selectivity of ligands like LY2456302.22
Binding free energy landscapes (BFELs) were effectively developed to compute the binding free energy profile and free energy barriers for biomolecular dynamics and dissociation.23–26 In order to accurately predict drug-target RT (t = 1/koff), Buch et al. obtained an accurate binding free energy of zamidine to trypsin with an error of ∼1 kcal mol−1 using AceMD (accelerating molecular dynamics).27 When the transmission factors were calculated, kon and koff values could thus be accurately derived according to the Eyring equation by Bai et al.28 Meanwhile, metadynamics (MT) simulations were successfully used for complex sampling. The simulation achieved by MT usually consumed less computational resources,29 probably due to the theoretical30 and empirical31 equations which were proposed for the calculation of statistical errors.
Here, in order to elucidate the inhibition mechanism of κ-OR selective LY2456302/JDTic in detail, unbiased MD and well-tempered32,33 MT simulations were performed on JDTic–κ-OR and LY2456302–κ-OR systems based on the crystal structure of inactive JDTic-bound κ-OR.34 The possible dissociation pathways of the κ-OR selective antagonists JDTic and LY2456302 were obtained in the well-tempered MT simulations. Combined with the experimental binding affinity under the same test conditions,21 a transmission factor was introduced to guarantee the accuracy of the ΔG0binding of LY2456302 and JDTic. Finally, the relative RT value of LY2456302/JDTic, which was proved to be determined from the activation free energy of dissociation (ΔG≠off), was calculated for comparison with the reported results. All this information might be helpful for the design of novel antidepressants targeting κ-OR.
Materials and methods
Protein preparation
The crystal structure of human JDTic-bound κ-OR was obtained from the Protein Data Bank (PDB, entry code: 4DJH), which was an engineered protein with part of the intracellular loop between transmembrane helices TM5 and TM6 replaced by T4 lysozyme (T4L).34 In order to do MD simulations on the wild type receptor, T4L and other unnecessary parts were removed from the crystal structure, and the loop was reconstructed by adding the missing residues S262 and T302–S303–H304–S305–T306 with the loop refinement protocol in Discovery Studio 3.5. 10 loops were generated and the most reasonable one was chosen for receptor construction. According to the latest crystal structure of inactive NTI-complexed δ-OR, the Na+ ion was exactly located at the allosteric pocket position.35
The above integrated three-dimensional structure of κ-OR was then imported into the Schrödinger software package. The protein structure was prepared with the Protein Preparation Tool (ProPrep) in the Schrödinger 2012 software suite. Residues Asn, Gln, and His were checked for protonated state automatically in ProPrep. The hydrogen atoms were added into the κ-OR crystal structure in a physiological pH environment by the PROPKA tool in Maestro with an optimized hydrogen bond network. No non-standard protonation states of amino acids were found.
Molecular docking
Referring to the conformation of JDTic in the crystal structure with κ-OR, the antagonist LY2456302 was sketched in Maestro and subjected to a Monte Carlo Μultiple Minimum conformational search using the OPLS_2005 force field and water as an implicit solvent (Surface Generalized Born (SGB) model). The output conformations were used as the starting point for the docking experiments.
The pocket Grid file of κ-OR was generated on the centroid of residue D1383.32 with the Receptor Grid Generation module, and docked with the Ligand Docking module within length 20 Å around D3.32.36,37 In the initial Glide docking (Glide 5.8), the van der Waals (vdW) scaling was set to 0.8 for the nonpolar atoms of the receptor and ligand. During docking, the number of docking outputs was set as 10
000 poses per docking run at most. The most reasonable conformation was picked out for dynamic simulation.
MD preparations
Two systems, JDTic–κ-OR and LY2456302–κ-OR complexes, were built for the simulations. A POPC (1-palmitoyl-2-oleoyl-sn-glycero-3-phosphocholine) bilayer with a surface area of 75 × 75 Å2 on the X–Y plane was generated under the Charmm36 force field by the VMD program (Version 1.9.1). For each system, the receptor was first embedded into the POPC bilayer using our in-house program pre-aligned in the OPM (Orientations of Proteins in Membranes) database.38–41 Thereafter, 103 POPC lipids coupled with about 11
000 TIP3P water molecules in a box ∼75 × 75 × 100 Å3 were pre-equilibrated and used to solvate the protein. Lipid molecules within 0.85 Å of the heavy atoms in the protein structure and water molecules in the bilayer were removed. 51 Na+ and 58 Cl− ions were used to produce neutral systems with 0.15 M NaCl in the water phase. We described the protein using the CHARMM36 force field with cmap correction.
The ligand force field was generated by the Paramchem webserver (http://cgenff.paramchem.org/), a program coupled with the CHARMM Force field.42–44 Small molecules with a correct configuration were imported into the Paramchem webserver and force field parameters of these compounds were then obtained through a script CHARMM General Force Field (CGenFF).
Molecular dynamics simulations
All the simulations were carried at the Supercomputer Centre at the State Key Laboratory of Bioreactor Engineering with 608 computing cores using Gromacs V.4.6.5 (ref. 45) and the CHARMM36 parameters for all compositions. In the first simulation step, the system was subjected to a 10
000-step energy minimization with 1000.0 kJ mol−1 nm−1 as the force threshold. Then, the systems were gradually heated from 0 K to 310 K followed by 50 ps initial equilibration at constant volume and temperature at 310 K (NVT). An additional 1 ns equilibration was performed at constant pressure and temperature (NPT ensemble; 310 K, 1 atm) with two thermostats (stabilizing the temperature independently for the protein–ligand system, and the lipids–water-ions system) at 2 ps time steps. VdW and short-range electrostatic interactions were cut off at 12 Å. Long-range electrostatic interactions were computed using the Particle Mesh Ewald (PME) summation scheme. The MD simulations of the LY2456302/JDTic-κ-OR systems were performed for 300 ns under NPT conditions with Parrinello–Rahman pressure coupler methods and a Nose–Hoover thermostat for temperature coupling. The time step for MD simulation was 2 fs and the integrator leap-frog algorithm was employed.
Metadynamics simulations
Theory. In a MT simulation, an additional history-dependent biased potential VG(S,t) was introduced into the system, |
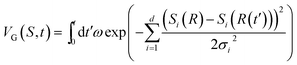 | (2) |
where t represents time, S represents the collective variables, ω is the energy rate and σi controls the width of the Gaussian for the ith collective variable. With the evolution of the system, the wells in the FES of the collective variables are filled up with the biased potential VG. The underlying free energy −F(S) is assumed to be estimated from the biased potential once all the wells have been filled after a sufficiently long time: |
limt→∞ VG(S, t) ∼ −F(S)
| (3) |
The correctness of the relationship as shown in eqn (2) has proven to be empirical by extensive tests under the assumption that the stochastic dynamics in the collective variable space is memoryless in the absence of the bias. Under this assumption, the error in FES construction has proven to be:
|
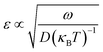 | (4) |
where
D is the intrinsic system diffusion coefficient in the collective variable space,
κB is the Boltzmann constant, and
T is the temperature of the system.
46
In fact, Barducci et al. introduced a “well-tempered” and “smoothly converging” algorithm to improve the biased potential.32 In the well-tempered MT, the deposition rate for the biased potential decreases by rescaling the Gaussian height (W) over the simulation time,
|
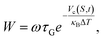 | (5) |
where
VG(
S,
t) is the biased potential at the current position and current time,
τG is the deposition stride, and Δ
T is a temperature-like parameter. The underlying free energy is a scaled approximation to the
VG(
S,
t), with
|
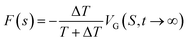 | (6) |
With respect to the standard MT, the biased potential decreases as 1/t when the simulation proceeds, which allows it to smoothly converge to an approximation of F(s).
Simulation details. We carried out about 100 ns well-tempered MT simulations for the systems of JDTic–κ-OR and LY2456302–κ-OR complexes. The crystal structure of inactive JDTic-bound κ-OR was directly used as a system for MT simulation, while the conformation of the LY2456302–κ-OR complex used for metadynamics analysis was the frame at the final 300 ns of the unbiased MD simulation. For each system, the MT simulations were carried out one time using plumed 2.02 (ref. 47 and 48) implemented in Gromacs 4.6.5. The RMSD evolutions of the heavy atoms in each diffusing ligand were used as the first collective variable. The second collective variables were selected based on the binding experiments and our unbiased MD simulation. The residue D1383.32 was proved to be indispensable for JDTic binding,34 while the amide group was validated to be detrimental for potency or selectivity.22 The Z-component of the vector connecting the protonated nitrogen of JDTic and the two carboxyl oxygen atoms of D1383.32 was thus selected as the second collective variable for JDTic egress, whereas those atoms connecting the amide of LY2456302 and C210ECL2 were utilized as the second collective variable for the LY2456302 system. Given the electrostatic interaction was preserved between the protonated nitrogen and D1383.32 in the unbiased MD simulation of the LY2456302 system, we then built an additional system for LY2456302 egress, in which the Z-component of the vector connecting the protonated nitrogen of LY2456302 and the two carboxyl oxygen atoms of D1383.32 was selected as the second collective variable. For the MT simulations, the biasing potential was added every 250 steps, with the width and height of the Gaussian hills set to 0.05 and 0.3 kJ mol−1, respectively, and ΔT = 2700.
Analysis of metadynamics simulation
To determine the sub-state conformations that result in the ligands being bound to κ-OR in MT simulations, structural clustering was performed using the g_cluster tool in Gromacs with the linkage algorithm based on the RMSD of each ligand molecule after alignment of the Cα atoms in the transmembrane helices in each frame to the starting structure.49 A 0.5 Å RMSD cut-off was chosen because it best captured spatially distinct clusters and allowed the top clusters to be representative of the predominant binding sites explored. The clustering was performed on the simulation frames with 20 ps interval time. The populations of each cluster are given in Table S1.† We examined the several most populated clusters and calculated the RMSDs for each ligand found in these clusters.
The binding free energy of metastable poses was collected through the sum_hills command, the theory of which was introduced in the above-mentioned eqn (2)–(6).
Another program, prime_mmgbsa, was used for scanning the binding free energy of each MT system through a rigid pattern with 10 ps interval time. Prime_mmgbsa, a hybrid MM-GBSA50,51 method combining pair-wise-based and grid-based methods, includes the following energy terms of MM-GBSA to calculate the binding free energy:
|
ΔG0binding-cal = ΔGMM + ΔGSOL + TΔS = ΔEvdw + ΔEes + ΔGGB + ΔGSA
| (7) |
where Δ
GMM, Δ
GSOL, and
TΔ
S respectively mean the gas-phase molecular mechanical energy, the solvation energy and the entropy contribution upon ligand binding at temperature
T, while Δ
Evdw, Δ
Ees, Δ
GGB, and Δ
GSA are the Lennard-Jones 6–12 potential, coulombic potential, and polarization and nonpolarization components of the solvation free energy, respectively.
Residence time calculations
First of all, we need to gain the transition factor ω in the case of ΔG0binding (predicted binding affinity) equal to ΔGexpbinding (experimental binding affinity) (Table S1†): |
ΔG0binding = ΔGexpbinding = ΔGminbinding − ΔGmin0binding = ω(ΔGminbinding-cal – ΔGmin0binding-cal)
| (8) |
where ΔGminbinding-cal and ΔGmin0binding-cal are obtained at the min and min0 states by prime_gbsa in the MT simulation, respectively. Then, the activation free energies for association (ΔG≠on) and dissociation (ΔG≠off) can be computed through |
ΔG≠off = ΔG0binding + ΔG≠on = ΔGminbinding − ΔGmin0binding = ω(ΔGminbinding-cal − ΔGmaxbinding-cal)
| (9) |
in which ΔGmaxbinding-cal is the binding free energy at the max state in the MT simulation.
According to the residence time formula (t = 1/koff) and Eyring’s equation,
|
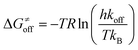 | (10) |
where
h is the Planck constant and
kB is the Boltzmann constant, we derived one relative method for computing the residence time ratio of two ligands towards the same target:
|
 | (11) |
After ΔG≠off is replaced by the values gained from eqn (9), we can capture the relative residence time of LY2456302 and JDTic towards κ-OR.
Results
Initial interaction modes of both ligands with the receptor
The crystal structure of JDTic-bound with κ-OR34 was directly used as a system for unbiased MD simulation, while it was utilized for docking LY2456302 into the orthostatic site of κ-OR. The docking pose of LY2456302 on the receptor was very similar to that of JDTic (Fig. 2). Electrostatic interactions were formed between the protonated nitrogens of both ligands and with D1383.32 (Ballesteros/Weinstein numbering52) of κ-OR. Both ligands were stabilized in a V-shaped conformation. In addition, the two ligands formed hydrophobic interactions with W2876.48, which could be observed in other opioid receptor structures.35,53,54
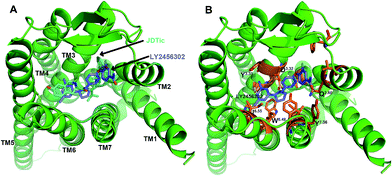 |
| Fig. 2 (A) Alignment of docking pose with the crystal structure of pure antagonist JDTic-bound κ-OR. (B) Key interactive residues in the LY2456302–κ-OR complex. The backbones of the ligands were colored with cyan-blue (JDTic) and slate-blue (LY2456302). Hydrogen bond interactions are shown as yellow dotted lines. | |
However, there was still a little difference between the two complexes. Similar to other opioid compounds, the phenolic hydroxyl group of JDTic interacted with H2916.52 through two water molecules, whereas the carbonyl group of LY2456302 formed a hydrogen bond (HB) interaction with Y3137.36. With the structure of LY2456302 in the binding pocket of κ-OR, we built the LY2456302–κ-OR system used for the unbiased MD simulation.
Unbiased MD simulations on the ligand-receptor systems
In our simulations, the LY2456302 system reached equilibrium at about 100 ns as indicated from the root mean square deviation (RMSD) values (Fig. S1A†). The RMSD values of κ-OR with respect to its crystal structure ranged from 2.3 to 3.2 Å in both the JDTic and LY2456302 systems. In addition, we calculated the root mean square fluctuations (RMSFs) of the systems to analyze the fluctuations of the receptor (Fig. S1B†). The loop section of the receptor exhibited a much larger fluctuation compared to the conserved TM section. IL (intracellular loop) 2, EL (extracellular loop) 2 and IL3 showed significant fluctuations, which were related to the fact that there are many residues on these three loops. The large fluctuation of the loop section reflected the significant conformational changes of the residues adjacent to these loops.
From the ligand RMSD analysis, JDTic exhibited much smaller conformational changes, mainly stabilizing at about 0.4 Å on average, whereas the range was from 1.0 to 1.8 Å among the LY2456302 frames. Meanwhile, the binding mode of JDTic at the final 300 ns was basically identical with that of the initial crystal through the alignment shown in Fig. S1C†. Given the significance34 of residue D3.32 and the number changes in the hydrogen bonds between JDTic and D3.32 (Table 1), we think the slight RMSD variations in ligand JDTic were mainly due to the tiny difference in HB formation modes.
Table 1 Occupancies of the hydrogen bonds in the two 300 ns JDTic/LY2456302-κ-OR systems
HB no. |
JDTic system |
LY2456302 system |
1 (%) |
2 (%) |
3 (%) |
1 (%) |
2 (%) |
D3.32-ligand |
3.8 |
35.6 |
60.6 |
66.4 |
32.5 |
C210-amide |
— |
— |
— |
83.8 |
— |
Protein-amide |
— |
— |
— |
83.8 |
— |
In the LY2456302 system, the ligand RMSD was stabilized at about 1.5 Å from about 150 ns. The final 300 ns frame with a 1.6 Å ligand RMSD was extracted for further analysis (Fig. 3B). In the snapshot, ligand LY2456302 was surrounded by the residues D1383.32, Q1152.60, C210ECL2, Y3127.35 and Y3207.43. The electrostatic interaction was preserved between the protonated nitrogen and D1383.32. The position of the two benzene rings changed a little in comparison with the initial docking LY2456302–κ-OR pose. The amide group of LY2456302 formed a hydrogen bond with C210ECL2. Detailed analysis showed that the HB existed for 83.8% of the simulation time and was the sole bond formed for the amide group of LY2456302 (Table 1).
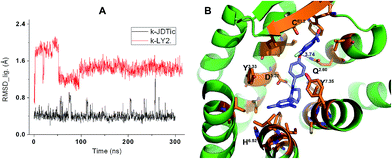 |
| Fig. 3 (A) RMSD calculations of ligands in unbiased JDTic/LY2456302–κ-OR systems. (B) The key interactions of the LY2456302–κ-OR complex at the final 300 ns. | |
According to the unbiased MD simulations of the κ-OR and ligand, the final 300 ns LY2456302-κ-OR complex and the crystal structure of the inactive JDTic-bound κ-OR were utilized as starting points for MT simulation.
Unbinding pathways of JDTic and LY2456302
In our well-tempered MT simulations, JDTic and LY2456302 left the antagonist binding pocket in κ-OR, and explored the binding pathways to exit the receptor through a series of conformational changes. Ligand cluster analysis was performed on the two MT systems, and two state conformations were obtained under a 0.5 Å RMSD cut-off. Meanwhile, several sub-state conformations were obtained according to the evolutions of ligand position and key interactions, which could clearly show us the whole unbinding pathway of each of the two ligands (Fig. 4 and Table S2†).
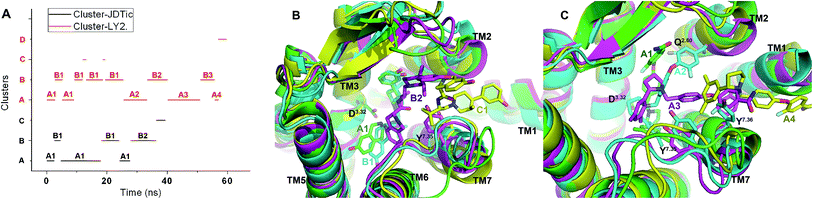 |
| Fig. 4 Cluster analysis of the metadynamics simulation. (A) Trajectory of JDTic/LY2456302 between the several main clusters during metadynamics simulation. (B) Alignment of representative poses of JDTic–κ-OR for cluster A1 (green), cluster B1 (blue), cluster B2 (pink) and cluster C (yellow). (C) Structural features of LY2456302–κ-OR complexes for cluster A1 (green), cluster A2 (blue), cluster A3 (pink) and cluster A4 (yellow). | |
The population of the top two state conformations (A/B) ran up to 90% in the JDTic MT system, whereas that only amounted to 72% in the LY2456302 MT system (Table S2†). However, cluster A in the LY2456302 MT system could be classified into 4 groups by the evolutions of ligand position and key interactions, while that in the JDTic MT system could only be split into 2 types (Fig. 4A). The different performances could also be observed in cluster B of the two MT systems.
From the representative pose of each cluster, we could see that JDTic first changed its conformation along TM3 and TM2 and got to the position of cluster B2 (Fig. 4B), which could deflect the nitrogen ion of JDTic far away from D3.32. Secondly, JDTic overcame the block of Y7.35 and reached the extracellular vestibule of κ-OR (cluster C). Similarly, LY2456302 first varied its conformation along TM3 and TM2 and moved to the position of cluster A2, where the amide group could form a HB with Q2.60 (Fig. 4C). Secondly, LY2456302 moved along TM7 and reached the position of cluster A3 with the help of Y3137.36. Lastly, LY2456302 moved through the space between TM1 and TM7 (cluster A4) and escaped completely. Interestingly, detailed analysis on the side chain torsion of Y7.36 showed that this residue played important roles not only among the cluster changes but also in the egress of the ligand in the LY2456302 system (Fig. 2C). However, Y7.36 exhibited little involvement in JDTic dissociation.
Interestingly, the dissociation pathways we obtained in both systems were very similar to recent work on muscarinic receptor ligands through AceMD,55 but different from that of β2-adrenergic receptor captured by conventional MD for a long time period.56 In addition, from the unbinding process of the two κ-OR selective antagonists, we could find that the distance evolution exhibited near synchrony with the ligand cluster variations (Fig. 4 and S2B†).
Metastable poses of JDTic/LY2456302 obtained by MT
For JDTic in κ-OR, we observed only one energy minimum (basin B0) as displayed in the free energy surfaces (FES) (Fig. 5A). The deepest free energy minimum in the FES was depicted as basin B0 in Fig. 5B and corresponded to the conformation that JDTic adopted in the X-ray crystallography structure. In the complex, ligand JDTic was stabilized in a V-shaped conformation and was surrounded by the residues D1383.32, Y1393.33, W2876.48, H2916.52 and Y3207.43.
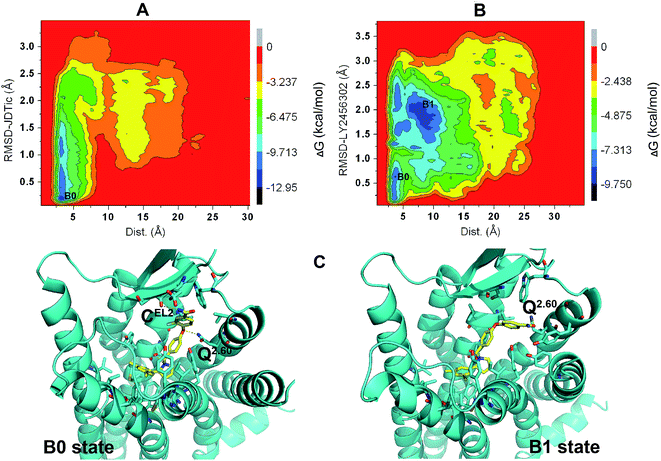 |
| Fig. 5 Metastable states in the dissociation of JDTic/LY2456302 from κ-OR. (A) The binding free energy surface for the dissociation of JDTic from κ-OR as a function of the Z-component of the vector connecting the nitrogen ion on the piperidine group of the ligand and residue D1383.32 and the RMSDs of JDTic. (B) The binding free energy surface for the dissociation of LY2456302 from κ-OR as a function of the Z-component of the vector connecting the amide group of the ligand and residue C210ECL2 and the RMSDs of LY2456302. (C) Structural characterization of the two main energy basins B0–B1 in the LY2456302 metadynamics system. | |
We observed two energy minima (basin B0 and basin B1) during the dissociation of LY2456302 from κ-OR as displayed in the FES (Fig. 5B). The deepest free energy minimum in the FES was depicted as basin B0 (Fig. 5C), where the benzene ring connecting with the amide group exhibited some rotation in comparison with the conformation that LY2456302 adopted in the final unbiased simulation (Fig. 3B). In the complex, ligand LY2456302 still formed a HB interaction with C210ECL2.
However, for basin B1, the scaffold of LY2456302 was twisted by a large amount. The amide group moved and formed a HB interaction with Q1152.60 (Fig. 5C). In the basin, the RMSDs of LY2456302 reached about 1.5–2.2 Å, and the average distance between C210ECL2 and the amide group of LY2456302 was up to about 6–10 Å (Fig. 5B). Such differences indicated that rotameric changes of the ligand were required for LY2456302 to move from the site corresponding to the basin B0.
Once all the metastable states in the helix bundle were filled, the antagonists JDTic/LY2456302 crossed the helix bundle to reach the extracellular vestibule of κ-OR to form a metastable vestibule-bound state, which was the first step for JDTic to enter the antagonist binding site. In this stage, the antagonists JDTic/LY2456302 were located among TM1, TM2 and TM7 (Fig. 4). Finally, the antagonist LY2456302 exited the receptor with the help of the residue Y3137.36 (Fig. S2C†).
Comparison of key interactions in both ligand unbinding pathways
From the free energy profile of JDTic–κ-OR along the evolution of the distance reaction coordinate (Fig. 6A), we could see that the electrostatic interaction between the nitrogen ion and D3.32 played an indispensable role in JDTic binding. The lowest energy was observed only at about 2.9 Å between the nitrogen ion and D3.32. A slight increase in the distance would greatly decrease the binding affinity of JDTic. Meanwhile, we could capture another extremely low-energy basin in the additional LY2456302–κ-OR system when the distance between the nitrogen ion and D3.32 ran up to about 5–6 Å (Fig. S3A†). Therefore, the significance of the nitrogen ion was much different between the JDTic and LY2456302 systems.
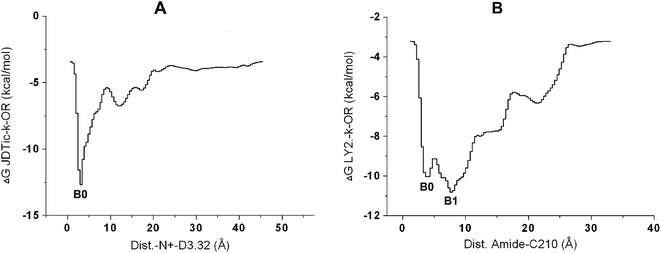 |
| Fig. 6 (A/B) Free energy profiles of the systems with respect to the distance evolution between the nitrogen ion of JDTic and residue D1383.32 (A) and between the amide group of LY2456302 and residue C210ECL2 (B). | |
For the LY2456302 system, several low-energy basins could be found not only in the 60 ns MT simulation (Fig. 6B) but also in the additional system (Fig. S3A†), which further reflected the binding diversity of LY2456302 resulting from some efficient modifications of JDTic. In the 60 ns LY2456302 system, a barrier of 1 kcal mol−1 needed to be crossed from basin0 to basin1. We could observe that this evolutionary process was coupled with the HB breaking between the ligand and C210, as well as new HB formation between the amide group and Q2.60 (Fig. 5C).
In addition, we could determine the predicted binding free energy from these metastable poses, −12.8 kcal mol−1 for JDTic and −10.9 kcal mol−1 for LY2456302 (Fig. 6), both of which showed about 2 kcal mol−1 deviation from the experimental binding affinities (Table S1†). However, in the LY2456302–κ-OR complex, the fact that the free energy was 1.9 kcal mol−1 weaker than the predicted value in the JDTIc system was in basic agreement with the experimental results.
Binding free energy scanning and residence time calculations of both systems
The binding free energy of each MT system was calculated using the prime_mmgbsa program with 10 ps interval time. The min/min0 low-energy wells and max transition state were extracted along the time evolution (Fig. 7 and S4†). The min low-energy well was obtained from the lowest-energy complex through prime_mmgbsa, while the max state corresponded to the highest-energy pose during the ligand-bound situation. The min0 low-energy well was the last lowest energy frame after the ligand crossed the max state. The poses at the min state belonged to cluster A1 and the basin B0 state in both the LY2456302 and JDTic systems (Table S3†). The frames at the min0/max transition states could also be found in the ligand clusters that they were associated with.
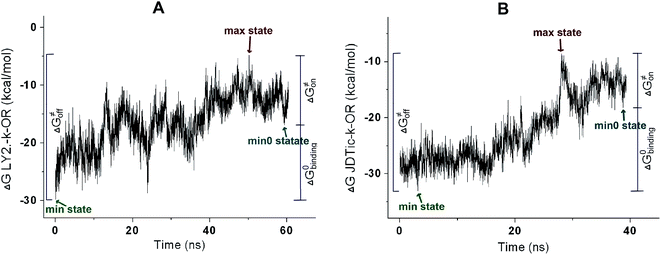 |
| Fig. 7 (A/B) Binding free energy profiles corresponding to the possible unbinding processes in the metadynamics simulations of the LY2456302 (A) and JDTic (B) systems. The min low-energy well was obtained from the lowest-energy complex in the simulation, while the max state corresponded to the highest-energy pose in κ-OR. The min0 low-energy well was the last lowest-energy frame after the ligand crossed the max state. | |
According to Bai’s report,28 the activation free energy of association (ΔG≠on) and dissociation (ΔG≠off) was calculated based on the free energies of the above-mentioned three states. This meant that ΔG≠off, ΔG0binding, and ΔG≠on all needed to be computed based on the whole dissociation pathway. Consequently, we could obtain a reasonable ΔG≠off value that determined the ligand RT in the case of the predicted ΔG0binding being identical to the corresponding experimental data. Thus, based on the MT simulation, we introduced a transition factor ω (eqn (8)) to guarantee that the ΔG0binding of LY2456302/JDTic was equal to the experimental binding affinity obtained under the same test conditions (Table 2 and Table S1†). Then we calculated the separate ΔG≠off values of the two ligands, which were −25.00 and −24.47 kcal mol−1 for LY2456302 and JDTic, respectively. Finally, using eqn (11) derived from Eyring’s equation, we determined 2.35 to be the residence time ratio of LY2456302/JDTic (ratioRT(LY2456302/JDTic)), which was only 7.5% deviated from the experimental data.16,17,21
Table 2 Binding free energies in kcal mol−1 and residence time ratio of LY2456302/JDTic during the metadynamics simulation
|
ΔGminbinding |
ΔGmin0binding |
ΔGmaxbinding |
ΔG0binding |
ΔG≠off |
rRT(LY2./JDTic) |
LY2456302-κ-OR |
−29.78 |
−16.88 |
−4.78 |
−12.90 |
−25.00 |
2.35 |
JDTic-κ-OR |
−33.07 |
−18.17 |
−8.60 |
−14.90 |
−24.47 |
In comparison with JDTic, LY2456302 had a higher ΔGminbinding (Table 2) that was consistent with the above-mentioned sections. In addition, LY2456302 exhibited a much higher ΔGmaxbinding, which was the critical reason for the longer RT of the ligand. At the max state of the JDTic complex (Fig. S4†), Y7.35 was surrounded by two nitrogen atoms and one oxygen atom, which would form electrostatic interactions with the oxygen atom of Y7.35. However, the key interaction between Y7.36 and LY2456302 was found to be through two aromatic rings at the max state.
Discussion
In this study, the key interactions of κ-OR with selective ligands JDTic and LY2456302 and their possible dissociation pathways were investigated using the unbiased MD and well-tempered MT simulations. For antagonist JDTic, the significance of the nitrogen ion was demonstrated repeatedly in our simulations. Mutation of D1383.32 was performed to evaluate the specific effect of D1383.32 on the binding of JDTic.34 The D138A mutation led to a 4-fold lower binding affinity of JDTic towards κ-OR, whereas other residue mutations did not cause such a decrease. In addition, increasing the electrostatic interactions between a charged ligand and a charged receptor was proven to hardly affect ligand dissociation rates.57
In contrast, LY2456302 exhibited multiple low-energy states in our well-tempered MT simulations. Such a difference possibly came from two aspects. One was the introduction of the amide group that could form a HB interaction either with C210 on ECL2 or with Q2.60 on TM2. The other one was the deletion of the two nitrogen atoms around the nitrogen ion, which caused an obvious decrease in the electrostatic interaction between the nitrogen ion and D3.32 compared with JDTic, while the left aromatic ring could play an important role in the improvement of ΔG≠off.
Instead of a sole strong interaction, such multiple metastable states were also observed in the dissociation of CCR5-selective maraviroc through AceMD.58 Meanwhile, given that many ligands exhibited high binding affinity coupled with largely adverse effects,5 it was supposed that the above-mentioned strong but single interaction mode might be responsible for the adverse effects and short RT of JDTic.
Furthermore, the relative RT value of LY2456302 and JDTic towards κ-OR was predicted with a little deviation based on their binding pathways. In comparison with Bai’s28 and Buch’s27 methods, a transmission factor was introduced to guarantee that the ΔG0binding of LY2456302/JDTic was equal to the experimental binding affinity obtained under the same test conditions. Our method need not predict the specific RT value of one ligand directly, which would save a large amount of experimental and computational resources.
Most generally, our calculations still need to be supported by much key interaction information, such as the crystal structure of the target κ-OR and the efficiency of the binding of the amide group in LY2456302, as well as the binding affinity at the same level. Obviously, related experimental binding data will be necessary for ligands with new scaffolds or different targets.
Conclusions
In this work, we investigated the inhibition mechanisms of LY2456302–κ-OR and JDTic–κ-OR complexes using unbiased MD and well-tempered MT simulations. The possible dissociation patterns of the κ-OR selective antagonists LY2456302 and JDTic were obtained in the simulations. We found that a strong but single interaction mode might be responsible for the adverse effects and short RT of JDTic, which could be considered as important information for new ligand design, while there might be better prospects for those chemicals with multiple metastable states. Finally, the relative RT value of LY2456302/JDTic, which was proved to be determined from the activation free energy of dissociation ΔG≠off, was calculated and was in good agreement with the reported results. Thus, our studies provided detailed analyses of the different inhibition modes caused by the separate chemotypes of LY2456302/JDTic and an efficient method to predict the relative RT values of the ligands.
Author contributions
J. C. carried out the molecular dynamics simulations. J. C. and Y. T. designed the study and analyzed the data. Y. T. was responsible for the project. J. C., W. L., G. L., W. Z. and Y. T. contributed to writing and commenting on the manuscript.
ConfliGood plct of interest
The authors declare no competing financial interest.
Acknowledgements
All the simulations were performed on the high-performance cluster kindly provided by Prof. Bangce Ye of the State Key Laboratory of Bioreactor Engineering, East China University of Science and Technology. This work was supported by the National Natural Science Foundation of China (Grant 81273438).
References
- D. C. Swinney, Nat. Rev. Drug Discovery, 2004, 3, 801–808 CrossRef CAS PubMed.
- R. Zhang and F. Monsma, Expert Opin. Drug Discovery, 2010, 5, 1023–1029 CrossRef CAS PubMed.
- R. A. Copeland, D. L. Pompliano and T. D. Meek, Nat. Rev. Drug Discovery, 2006, 5, 730–739 CrossRef CAS PubMed.
- M. R. Dowling and S. J. Charlton, Br. J. Pharmacol., 2006, 148, 927–937 CrossRef CAS PubMed.
- D. Guo, J. M. Hillger, A. P. IJzerman and L. H. Heitman, Med. Res. Rev., 2014, 34, 856–892 CrossRef CAS PubMed.
- D. C. Swinney, P. Beavis, K. T. Chuang, Y. Zheng, I. Lee, P. Gee, J. Deval, D. M. Rotstein, M. Dioszegi and P. Ravendran, et al., Br. J. Pharmacol., 2014, 171, 3364–3375 CrossRef CAS PubMed.
- W. Yan, Nat. Med., 2015, 21, 545 CrossRef CAS PubMed.
- M. C. Lagerstrom and H. B. Schioth, Nat. Rev. Drug Discovery, 2008, 7, 339–357 CrossRef PubMed.
- X. Zhang, R. C. Stevens and F. Xu, Trends Biochem. Sci., 2015, 40, 79–87 CrossRef CAS PubMed.
- B. A. Fleck, S. R. Hoare, R. R. Pick, M. J. Bradbury and D. E. Grigoriadis, J. Pharmacol. Exp. Ther., 2012, 341, 518–531 CrossRef CAS PubMed.
- S. Kapur and P. Seeman, J. Psychiatr. Neurosci., 2000, 25, 161–166 CAS.
- M. D. Metcalf and A. Coop, AAPS J., 2005, 7, E704–E722 CrossRef CAS PubMed.
- S. P. Runyon, L. E. Brieaddy, S. W. Mascarella, J. B. Thomas, H. A. Navarro, J. L. Howard, G. T. Pollard and F. I. Carroll, J. Med. Chem., 2010, 53, 5290–5301 CrossRef CAS PubMed.
- F. I. Carroll and W. A. Carlezon Jr, J. Med. Chem., 2013, 56, 2178–2195 CrossRef CAS PubMed.
- M. Urbano, M. Guerrero, H. Rosen and E. Roberts, Bioorg. Med. Chem. Lett., 2014, 24, 2021–2032 CrossRef CAS PubMed.
- I. Carroll, J. B. Thomas, L. A. Dykstra, A. L. Granger, R. M. Allen, J. L. Howard, G. T. Pollard, M. D. Aceto and L. S. Harris, Eur. J. Pharmacol., 2004, 501, 111–119 CrossRef CAS PubMed.
- T. A. Munro, L. M. Berry, A. Van’t Veer, C. Beguin, F. I. Carroll, Z. Zhao, W. A. Carlezon Jr and B. M. Cohen, BMC Pharmacol., 2012, 12, 1–18 Search PubMed.
- M. R. Bruchas, T. Yang, S. Schreiber, M. Defino, S. C. Kwan, S. Li and C. Chavkin, J. Biol. Chem., 2007, 282, 29803–29811 CrossRef CAS PubMed.
- C. H. Mitch, S. J. Quimby, N. Diaz, C. Pedregal, M. G. de la Torre, A. Jimenez, Q. Shi, E. J. Canada, S. D. Kahl and M. A. Statnick, et al., J. Med. Chem., 2011, 54, 8000–8012 CrossRef CAS PubMed.
- L. M. Rorick-Kehn, J. W. Witcher, S. L. Lowe, C. R. Gonzales, M. A. Weller, R. L. Bell, J. C. Hart, A. B. Need, J. H. McKinzie and M. A. Statnick, et al., Int. J. Neuropsychopharmacol., 2014, 18, 1–11 Search PubMed.
- L. M. Rorick-Kehn, J. M. Witkin, M. A. Statnick, E. L. Eberle, J. H. McKinzie, S. D. Kahl, B. M. Forster, C. J. Wong, X. Li and R. S. Crile, et al., Neuropharmacology, 2014, 77, 131–144 CrossRef CAS PubMed.
- K. Takeuchi, W. G. Holloway, J. H. McKinzie, T. M. Suter, M. A. Statnick, P. L. Surface, P. J. Emmerson, E. M. Thomas, M. G. Siegel and J. E. Matt, et al., Bioorg. Med. Chem. Lett., 2007, 17, 5349–5352 CrossRef CAS PubMed.
- R. Zhou, B. J. Berne and R. Germain, Proc. Natl. Acad. Sci. U. S. A., 2001, 98, 14931–14936 CrossRef CAS PubMed.
- R. R. Johnson, A. Kohlmeyer, A. T. Johnson and M. L. Klein, Nano Lett., 2009, 9, 537–541 CrossRef CAS PubMed.
- J. Higo, Y. Nishimura and H. Nakamura, J. Am. Chem. Soc., 2011, 133, 10448–10458 CrossRef CAS PubMed.
- Y. Miao, S. E. Nichols and J. A. McCammon, Phys. Chem. Chem. Phys., 2014, 16, 6398–6406 RSC.
- I. Buch, T. Giorgino and G. de Fabritiis, Proc. Natl. Acad. Sci. U. S. A., 2011, 108, 10184–10189 CrossRef CAS PubMed.
- F. Bai, Y. Xu, J. Chen, Q. Liu, J. Gu, X. Wang, J. Ma, H. Li, J. N. Onuchic and H. Jiang, Proc. Natl. Acad. Sci. U. S. A., 2013, 110, 4273–4278 CrossRef CAS PubMed.
- D. Bochicchio, E. Panizon, R. Ferrando, L. Monticelli and G. Rossi, J. Chem. Phys., 2015, 143, 1–7 CrossRef PubMed.
- A. Laio, A. Rodriguez-Fortea, F. L. Gervasio, M. Ceccarelli and M. Parrinello, J. Phys. Chem. B, 2005, 109, 6714–6721 CrossRef CAS PubMed.
- G. Bussi, A. Laio and M. Parrinello, Phys. Rev. Lett., 2006, 96, 1–4 CrossRef PubMed.
- A. Barducci, G. Bussi and M. Parrinello, Phys. Rev. Lett., 2008, 100, 1–4 CrossRef PubMed.
- M. Deighan, M. Bonomi and J. Pfaendtner, J. Chem. Theory Comput., 2012, 8, 2189–2192 CrossRef CAS PubMed.
- H. Wu, D. Wacker, M. Mileni, V. Katritch, G. W. Han, E. Vardy, W. Liu, A. A. Thompson, X. P. Huang and F. I. Carroll, et al., Nature, 2012, 485, 327–332 CrossRef CAS PubMed.
- G. Fenalti, P. M. Giguere, V. Katritch, X. P. Huang, A. A. Thompson, V. Cherezov, B. L. Roth and R. C. Stevens, Nature, 2014, 506, 191–196 CrossRef CAS PubMed.
- R. A. Friesner, J. L. Banks, R. B. Murphy, T. A. Halgren, J. J. Klicic, D. T. Mainz, M. P. Repasky, E. H. Knoll, M. Shelley and J. K. Perry, et al., J. Med. Chem., 2004, 47, 1739–1749 CrossRef CAS PubMed.
- T. A. Halgren, R. B. Murphy, R. A. Friesner, H. S. Beard, L. L. Frye, W. T. Pollard and J. L. Banks, J. Med. Chem., 2004, 47, 1750–1759 CrossRef CAS PubMed.
- A. L. Lomize, I. D. Pogozheva and H. I. Mosberg, J. Chem. Inf. Model., 2011, 51, 930–946 CrossRef CAS PubMed.
- M. A. Lomize, I. D. Pogozheva, H. Joo, H. I. Mosberg and A. L. Lomize, Nucleic Acids Res., 2012, 40, D370–D376 CrossRef CAS PubMed.
- J. B. Klauda, R. M. Venable, J. A. Freites, J. W. O’Connor, D. J. Tobias, C. Mondragon-Ramirez, I. Vorobyov, A. D. MacKerell Jr and R. W. Pastor, J. Phys. Chem. B, 2010, 114, 7830–7843 CrossRef CAS PubMed.
- W. Humphrey, A. Dalke and K. Schulten, J. Mol. Graphics, 1996, 14, 33–38 CrossRef CAS PubMed.
- K. Vanommeslaeghe, E. Hatcher, C. Acharya, S. Kundu, S. Zhong, J. Shim, E. Darian, O. Guvench, P. Lopes and I. Vorobyov, et al., J. Comput. Chem., 2010, 31, 671–690 CAS.
- K. Vanommeslaeghe and A. D. MacKerell Jr, J. Chem. Inf. Model., 2012, 52, 3144–3154 CrossRef CAS PubMed.
- K. Vanommeslaeghe, E. P. Raman and A. D. MacKerell Jr, J. Chem. Inf. Model., 2012, 52, 3155–3168 CrossRef CAS PubMed.
- B. Hess, C. Kutzner, D. van der Spoel and E. Lindahl, J. Chem. Theory Comput., 2008, 4, 435–447 CrossRef CAS PubMed.
- A. Barducci, M. Bonomi and M. Parrinello, Wiley Interdiscip. Rev.: Comput. Mol. Sci., 2011, 1, 826–843 CrossRef CAS.
- A. Laio and M. Parrinello, Proc. Natl. Acad. Sci. U. S. A., 2002, 99, 12562–12566 CrossRef CAS PubMed.
- G. A. Tribello, M. Bonomi, D. Branduardi, C. Camilloni and G. Bussi, Comput. Phys. Commun., 2014, 185, 604–613 CrossRef CAS.
- S. Pronk, S. Pall, R. Schulz, P. Larsson, P. Bjelkmar, R. Apostolov, M. R. Shirts, J. C. Smith, P. M. Kasson and D. van der Spoel, et al., Bioinformatics, 2013, 29, 845–854 CrossRef CAS PubMed.
- P. A. Kollman, I. Massova, C. Reyes, B. Kuhn, S. Huo, L. Chong, M. Lee, T. Lee, Y. Duan and W. Wang, et al., Acc. Chem. Res., 2000, 33, 889–897 CrossRef CAS PubMed.
- B. Kuhn and P. A. Kollman, J. Med. Chem., 2000, 43, 3786–3791 CrossRef CAS PubMed.
- J. A. Ballesteros and H. Weinstein, Methods Neurosci., 1995, 25, 366–428 CAS.
- A. Manglik, A. C. Kruse, T. S. Kobilka, F. S. Thian, J. M. Mathiesen, R. K. Sunahara, L. Pardo, W. I. Weis, B. K. Kobilka and S. Granier, Nature, 2012, 485, 321–326 CrossRef CAS PubMed.
- S. Granier, A. Manglik, A. C. Kruse, T. S. Kobilka, F. S. Thian, W. I. Weis and B. K. Kobilka, Nature, 2012, 485, 400–404 CrossRef CAS PubMed.
- K. Kappel, Y. Miao and J. A. McCammon, Q. Rev. Biophys., 2015, 48, 479–487 CrossRef CAS PubMed.
- R. O. Dror, A. C. Pan, D. H. Arlow, D. W. Borhani, P. Maragakis, Y. Shan, H. Xu and D. E. Shaw, Proc. Natl. Acad. Sci. U. S. A., 2011, 108, 13118–13123 CrossRef CAS PubMed.
- A. C. Pan, D. W. Borhani, R. O. Dror and D. E. Shaw, Drug Discovery Today, 2013, 18, 667–673 CrossRef CAS PubMed.
- Q. Bai, Y. Zhang, X. Li, W. Chen, H. Liu and X. Yao, Phys. Chem. Chem. Phys., 2014, 16, 24332–24338 RSC.
Footnote |
† Electronic supplementary information (ESI) available. See DOI: 10.1039/c5ra24911b |
|
This journal is © The Royal Society of Chemistry 2016 |
Click here to see how this site uses Cookies. View our privacy policy here.