DOI:
10.1039/C5FD00157A
(Paper)
Faraday Discuss., 2016,
187, 105-118
Label-free classification of colon cancer grading using infrared spectral histopathology
Received
10th November 2015
, Accepted 11th December 2015
First published on 11th December 2015
Abstract
In recent years spectral histopathology (SHP) has been established as a label-free method to identify cancer within tissue. Herein, this approach is extended. It is not only used to identify tumour tissue with a sensitivity of 94% and a specificity of 100%, but in addition the tumour grading is determined. Grading is a measure of how much the tumour cells differ from the healthy cells. The grading ranges from G1 (well-differentiated), to G2 (moderately differentiated), G3 (poorly differentiated) and in rare cases to G4 (anaplastic). The grading is prognostic and is needed for the therapeutic decision of the clinician. The presented results show good agreement between the annotation by SHP and by pathologists. A correlation matrix is presented, and the results show that SHP provides prognostic values in colon cancer, which are obtained in a label-free and automated manner. It might become an important automated diagnostic tool at the bedside in precision medicine.
Introduction
Colorectal cancer
Colorectal cancer is a major cause of morbidity and mortality throughout the world, and the third most common cancer worldwide. It affects men and women almost equally, with just over 1 million new cases every year.1 In the majority of cases the colorectal carcinoma originates in polyps, referred to as benign adenomas. About 80% of colorectal carcinomas are sporadic with no hereditary deposition. The two most common hereditary risk factors are “familial adenomatous polyposis” (FAP)2,3 and “hereditary non polyposis colon cancer” (HNPCC or Lynch Syndrome).3 Patients with FAP suffer from hundreds or thousands of polyps in the colorectal mucosa, with a manifested near 100% risk of malignancy. Lynch syndrome shows only a small number of polyps. Non-hereditary risk factors are aging, high fat nourishment in combination with a lack of physical exercise, and smoking.
The first level of detecting and characterizing colon cancer is visual inspection during colonoscopy. A diagnosis is performed on a biopsy by pathologists via histopathological examination using haematoxylin and eosin (H&E) stained tissue thin sections.4 The current guidelines of the UICC for classification of colorectal carcinoma follow the TNM (tumour, lymph nodes, metastasis) system.5 The staging is a measure of how the cancer has spread through the organism. The TNM system characterizes the local infiltration of the primary tumour, the lymph node status, and potential distant metastasis in other organs. In contrast to staging, the WHO GRADING system addresses the differentiation of tumour cells.6 The grade score reaches from G1 (well-differentiated), to G2 (moderately differentiated), G3 (poorly differentiated) and in rare cases to G4 (undifferentiated). Well and moderately differentiated tumours are summarised as “low grade”, and poorly and undifferentiated tumours as “high grade” carcinomas. The grading of cancer tissue samples is important for the prognosis of cancer patients.7–9Fig. 1 shows exemplary images of H&E stained colorectal cancer tissue at different differentiation states, and for comparison a sample of normal colon mucosa. Here we illustrate that spectral histopathology not only distinguishes between tumour and healthy tissue, but in addition provides the grading of the tumour.
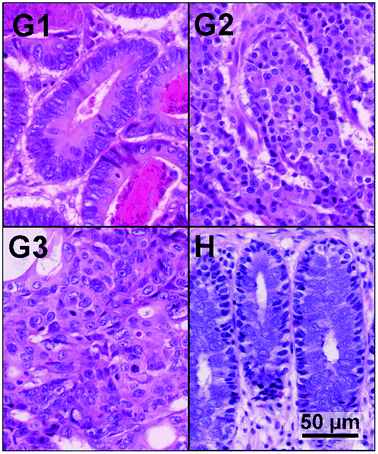 |
| Fig. 1 Different dedifferentiation states of colon carcinoma. G1, G2 and G3 show the grading states during tumour genesis and H represents a H&E stained sample of healthy colonic tissue with normal cells. | |
Spectral histopathology via infrared imaging
In the last decade, many studies have shown that spectral histopathology is capable of classifying tissue10,11 and especially diseased tissue.12–20 The IR spectra measured per pixel represent mostly an integral signal of the proteome and genome. Each spectrum is assigned a specific colour. This results in an index colour image, by which the tissue is classified and tumour is identified. In particular, colorectal carcinoma is identified in this way by IR imaging.21,22 Even though these previous studies have shown that SHP can differentiate between healthy and cancer tissue label-free, it is of limited value for clinical diagnosis, because this question is easily and very quickly answered by histopathology already. Therefore here the approach is significantly extended, and in addition the grading of the tumour is determined. The grading is much more difficult to classify and depends critically on the expertise of the respective pathologist and the time taken for the diagnosis. Biomax tissue micro arrays (TMA) were measured with an Agilent Cary 620 FTIR microscope and subsequently H&E stained. The resulting index colour images were analysed and compared with the morphological characteristics provided by H&E staining. These results exhibited good correlation between the annotation by SHP and the annotation by pathologists, as shown in a correlation matrix. This shows that the method is a useful tool for label-free automated and precise colon cancer tissue grading.
Bioinformatics workflow
We established a workflow that integrates FTIR microscopy, bioinformatics and histopathology (Fig. 2). Primarily, the tissue thin sections were measured with FTIR imaging using a focal plane array detector (FPA) with 128 × 128 MCT elements. The measured spectral map is clustered by an unsupervised algorithm in the training stage (hierarchical clustering, HCA, k-means). The resulting index colour image represents the spectral distribution over the examined tissue section. In parallel the tissue is still accessible for H&E and/or immunohistochemical staining due to the marker-free character of the SHP. In collaboration with pathologists, the index colour image based on the spectral map is correlated with the classically stained image of the sample. A database of spectral “fingerprints” is generated for different tissue and disease types from this expert annotation.
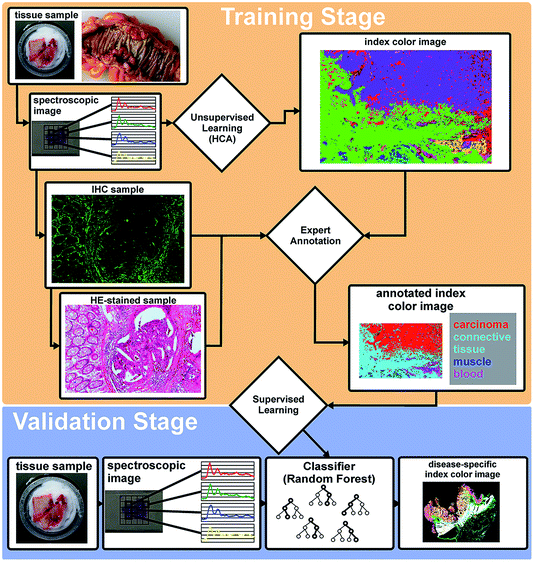 |
| Fig. 2 Workflow of the training and validation stage. In the training stage the spectral maps were correlated to classical histopathological annotation by an expert. The resulting spectral database is used for the training of a supervised classification algorithm that is validated on independent samples in the validation stage. | |
The spectral database enables us to train supervised classification algorithms like the artificial neural networks (ANN), support vector machines (SVM), or random forests (RF).23–25 As previously shown in our approach, we are using Random Forest (RF) classifiers, which have proven to be accurate, easy to use and robust. The workflow was established in our lab previously for colon and lung cancer.22,31 Unknown spectral maps of tissue thin sections can be automatically annotated with the trained RF (validation stage). The accuracy of the trained supervised classifier is determined on an independent pool of patients to ensure that no over fitting is occurring.
Experimental
Sample preparation
Tissue micro arrays (TMA, Table 1) displaying samples of colorectal carcinomas with different gradings were purchased from US Biomax Inc. (Rockville, MD, USA). The samples were 5 μm thick and were placed on LowE slides [Kevley Technologies, Chesterland, OH, USA]. Before the spectral measurements, they were deparaffinised using standard protocols.26 Afterwards the samples were stored and measured under dry air.
Table 1 Summary of the measured samples by their grading, the used TMA slides and patient number used overall and for training
Biomax ID |
Chosen patients (cases) |
G1 |
G2 |
G3 |
CO1002b |
32 (32) |
6 (6) |
18 (18) |
8 (8) |
BCO51111 |
70 (136) |
6 (9) |
55 (110) |
9 (17) |
CO722 |
23 (23) |
4 (4) |
17 (17) |
2 (2) |
|
No. independent patients – validation |
14
|
87
|
17
|
No. patients – training |
2
|
3
|
2
|
Data acquisition
Infrared hyperspectral data acquisition was performed in transflection mode using an Agilent system (Santa Clara, California, USA), consisting of a Cary 620 infrared microscope in combination with a Cary 670 FTIR spectrometer. Spectral data were collected by a mounted liquid nitrogen cooled focal plane array (FPA) MCT detector with 128 × 128 elements, providing a field of view (FOV) of approximately 715 μm × 715 μm. The Fourier transformation was performed with the Agilent Resolution Pro Software with Mertz phase correction, a Blackman-Harris-4-term apodization and a zero filling of 2. The spectra were saved between 3700–950 cm−1 with a spectral resolution of 4 cm−1. For the transflection (reflection–absorption) measurements, the tissue sections had been prepared on LowE slides. An inherent problem of the occurrence of a standing wave electric field in the transflection mode was described for infrared microscopy, which leads to shifts of and variances in the ratio of absorption bands, especially between the amid I and amid II bands.32,33 However, simulations have shown that the resulting intensity artefact is minimized when objectives with high numeric apertures are used.34 Therefore, here a high numeric aperture of 0.62 was used. In addition we tested the second derivative, which minimizes the effects of the standing wave artefact. This resulted in the same supervised classification of the colon cancer grading. Therefore, we used the vector normalized spectra.
The resulting raw spectral maps were pre-processed using the previously described workflow.22 Strong artefacts possibly arising from cracks or folds in the tissue were eliminated by quality control based on the signal-to-noise ratio and the intensity of the amid I band. The remaining spectra were subjected to a Mie and resonance-Mie correction based on EMSC27–29 in the wavenumber range from 2300 to 950 cm−1. The correction was performed with only one iteration step, but a higher number of iteration steps (up to 20) were tested due to low scattering effects, as this does not alter the final classification. During the last step the spectra were smoothed by a 9 point Savitzky Golay filter,30 providing second derivative spectra for the unsupervised multivariate methods like hierarchical and k-means clustering. For the RF classification, the spectra were only corrected for resonance Mie scattering as previously described. For both methods the analysis was performed on the fingerprint region from 1800–950 cm−1.
Data selection and training of a supervised classifier
As we have shown before, the RF classifier is capable of distinguishing tissue types and identifying cancerous regions in colorectal tissue sections.22 Here we established a hierarchical application of two consecutive RFs (Fig. 3). Spectra identified as cancerous were isolated and furthermore analysed by a second RF trained for recognizing the grade of differentiation of colorectal carcinomas. Training data were acquired from two spots of G1 and three spots of G2 and G3. Compared to the first RF using 100 data points in the fingerprint region, the second RF is supplied with 385 data points on an equidistant wavenumber scale from the wavenumber interval of 1800–950 cm−1. All computations were performed in MATLAB (TheMathWorks Inc., Natick, MA, USA).
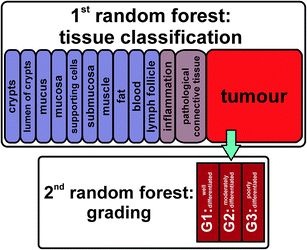 |
| Fig. 3 The first RF detects different tissue types and pathological regions. The spectra of tumorous regions were transferred to the second RF, which determines the grading of the cancer cells. | |
For this study 191 sample spots collected from 125 patients, covering different carcinoma grades (1, 2 and 3), were analysed. 19 samples from 16 patients were identified as G1, 145 samples from 90 patients were identified as G2, and G3 was analysed from 27 samples from 19 patients (Table 1). The TMAs provided by US Biomax were standardised and annotated by two clinical pathologists. The microarrays were H&E stained after IR data collection. This allowed us to compare the morphological characteristics of the tissue spots with the index colour images provided by IR-SHP, leading to good correlation not only of tissue types but even regarding the grading of colon carcinomas. This study was performed in two phases. During the training stage, samples of each grade were selected randomly, spectra were analysed and training spectra representative of each grade were identified by visual inspection supported by the expertise of a Biomax independent clinical pathologist. The distribution of the measured patients over the three TMAs is shown in Table 1. The number of patients in the training set is balanced (2 for G1, 3 for G2 and 2 for G3). From these we established a training dataset of 987 representative spectra – 355 spectra for G1, 285 spectra for G2, and 347 spectra for G3. The training data set is well balanced among the three dedifferentiation grades.
In the validation stage the trained grading RF classifier was validated on 14 patients with a well-differentiated (G1), 87 patients with a moderately differentiated (G2), and 17 patients with a poorly differentiated (G3) tumour. This distribution represents the clinical occurrence of colorectal adenocarcinomas with around 10% well (G1), 70% moderately (G2) and 20% poorly differentiated carcinomas.35 In the validation the data set does not need to be balanced between the three grades. The prediction of the grading RF was performed on the tumour spectra identified by the previously published first RF.22 The grading RF was trained with 5000 trees and 16 features randomly chosen from the spectral data points per decision in the trees. We present the H&E stained images of the core samples, combined with corresponding spectral images that are the basis of the spectral grading of the tumour.
Results and discussion
Classification of colon tissue and colon carcinoma by infrared spectral histopathology
In the past we reported on the automated label-free classification of colon cancer tissue sections.22 Such classification by the first RF is shown in Fig. 4 in comparison with the H&E stained sample image. We reached an accuracy of 96% combined with a high sensitivity of 94% and a specificity of 100%. This analysis was performed on 46 randomly chosen independent samples. The spectra that were classified as tumorous (see Fig. 4D) were further analysed in the second RF, which determined the grading of the cancer cells.
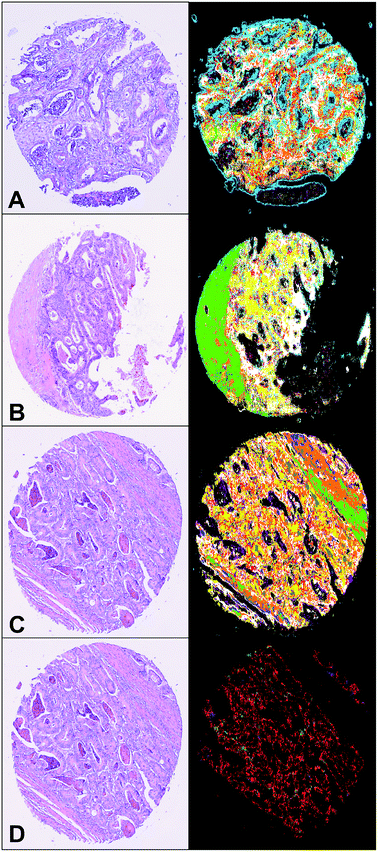 |
| Fig. 4 From A to C exemplary index colour images of the first RF are presented. The high correlation between the H&E and the SHP can be seen. The colour code is as follows: green and yellow hues denote connective tissue, white indicates musculature, cyan is connective tissue with supporting cells, pink is the lumen of the crypts, olive indicates blood, light blue is mucus (this occurs in whole tissue and is highly influenced by scattering effects, as seen in A, but it does not affect the tumour detection), blue is pathological connective tissue, orange is inflammatory tissue, and red is the tumour region. (D) The same spot as C but only the pathological regions are presented. | |
SHP yields reliable classification of well-differentiated colonic carcinomas
For each grade one microarray tissue sample is shown as an example in Fig. 5. In total 191 tissue spots were measured and analysed. The validation samples are presented, while the training data originates from independent samples. Fig. 5 shows the H&E stained sample of a colorectal well-differentiated cancer overlaid with tumour class spectra of the RF-based IR image. The cancerous regions via IR-SHP are clearly identified. The grading is given by the colour codes: G1 – well-differentiated – in red, G2 – moderately differentiated – in green, and G3 – poorly differentiated – in blue pixels.
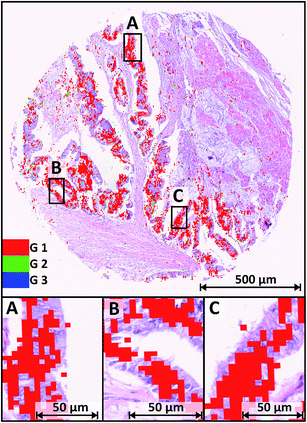 |
| Fig. 5 H&E stained image overlaid with spectral false colour image representing the annotation of tumours. The three grades are shown with different colours. G1 is marked red, green was used for G2 and blue pixels represent G3. | |
We have chosen a threshold value of 5% of all tumour spectra for the highest grade to be taken into account for the final classification, in order to prevent false classification. The occasionally visible pixels annotating higher grades than G1 are isolated and mostly located at the edge of tumour tissue. They represent between 3.5% (G3) and 4.2% (G2) of all spectra that were annotated as “tumour” by the first level RF. The majority of 92.3% of tumour spectra were classified as G1, matching the annotation given by two clinical pathologists at US Biomax. Thus, the lowest grade with cells showing the least dedifferentiation of cancerous cells is reliably recognized by the algorithm. The pixels of the SHP index colour image match with the tumour cells quite well even at higher magnification of the H&E stained image. Small parts of the samples' cancerous regions are shown at a higher magnification in Fig. 5A–C. Tumour cells with barely visible changes in the morphology of the nuclei were assigned by the classifier as G1 cancer. The RF-based spectral image derived from a marker-free method leads to a precise classification of G1 colonic cancer tissue. This is promising, because tumour grading provides information on treatment and prognosis of colon cancer patients. Patients suffering from an early detected well-differentiated cancer have a better prognosis and may be treated with a less aggressive medication, providing better and also individual treatment with a positive outcome and the consequence of a better health-related quality of life. For 19 of 191 samples, these were characterized as well-differentiated (G1). For 18 of these 19, the annotation by our method was correct, leading to a sensitivity of 94%. The lack of false positive predictions results in a specificity of 100% regarding G1. Note that the indicators of sensitivity and specificity for the evaluation of classifiers can only be specified for binary classifiers. Thus we present these indicators (Fig. 8A) for each individual grade against the remaining other grades, rather than providing comprehensive indicators for the overall prediction of all three grades. As a comprehensive indicator for all three classes, we present a confusion matrix in Fig. 8B.
A significant fraction of cancerous regions in G2 samples is classified as G1
Fig. 7 shows one exemplary tissue core sample annotated as G2 carcinoma by the pathologists' annotation. The IR image is again enlarged to the same resolution as the H&E stained image. The overlay shows a compliance of the tumour identified with SHP and cancerous regions visibly highlighted through the H&E staining. The majority of spectra in this case have been classified as G1 or G2, represented by red and green pixels. Again a small amount of isolated G3 (blue) pixels (approximately 1%) are scattered throughout the index colour image. They have not been taken into account for the analysis. In particular, 60% of the tumour class spectra were annotated G1, while 39% were classified as moderately differentiated (G2). Thus the sample is annotated as a G2 tumour, even though the large amount of over 60% were annotated G1. This is reasonable due to the fact that a tumour is never a homogenous mass of cells in the exact same state of dedifferentiation. In routine histopathological work up, the grading was performed according to the less differentiated part of the tumour. There are always cells or whole regions present, still barely differing from their tissue of origin. In the enlarged areas shown in Fig. 6, tumour cells with enlarged nuclei are visible. The changes in shape and morphology during the progress of dedifferentiation are more pronounced as compared to the G1 sample. In total, 145 core samples, annotated as moderately differentiated (G2) carcinoma by clinical pathologists, were in the dataset. 119 out of these 145 samples were correctly predicted by SHP. The remaining 26 samples were annotated as G3, but none was spectrally graded lower than the grading by a pathologist.
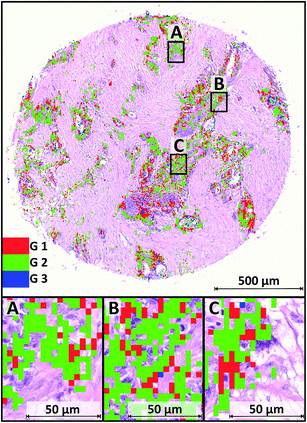 |
| Fig. 6 H&E stained sample of grade 2 tumour overlaid with IR spectral image. The three colours red, green and blue cover again the three grades in ascending order. | |
G3 colorectal carcinoma shows the whole range of the dedifferentiation progress
Fig. 7 shows a Biomax TMA sample annotated by Biomax pathologists as a G3 carcinoma, exhibiting diffuse regions of poorly differentiated cells. The enlarged areas show selected regions with a variety of cancer cells. The nuclei are big in relation to the cell bodies and their shape differs distinctly from the healthy cells in their tissue of origin. The morphology of tumour cells is prominently illustrated in the H&E stained sample, due to the massively upregulated transcription activity necessary for a high proliferation rate. About one third (33.9%) of all spectra annotated as tumour in this sample were classified as poorly differentiated (G3) carcinoma. The rest is annotated as moderately differentiated (G2 – 44.4%) and 21.6% of tumour spectra were classified as well-differentiated (G1). With this outcome of the prediction the sample is annotated as a G3 carcinoma, matching the pathologists' diagnosis. In the dataset there were 27 core samples that were given a diagnosis of G3 adenocarcinoma. The classifier predicted 25 out of 27 correctly. A further 24 samples were annotated as G3 but didn’t actually match the pathologists’ vote (false positive). These 24 samples are exactly predicted as false negatives regarding G2. This might be due to the fact that the intermediate G2 and G3 are broadly similar in the biochemical status of the tissue. Tumour cells from G1 carcinomas, which are still well-differentiated, are better distinguished from the higher grades than moderately (G2) and poorly (G3) differentiated carcinomas are from each other. None of the microarrays includes a sample of a G4 carcinoma, therefore no analysis was possible of tissue and cells showing anaplasia.
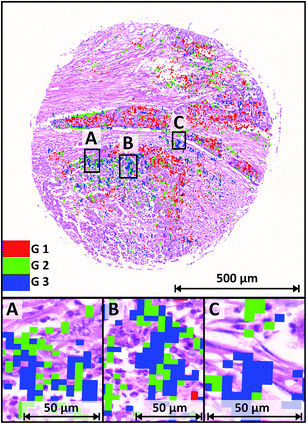 |
| Fig. 7 H&E stained sample of a G3 colorectal carcinoma. The colour code is used as before. All three grades are determined in this sample, with a high amount of G3 (approx. 34%), leading to the annotation matching the diagnosis given by clinical pathologists. | |
SHP analysis leads to a reproducible annotation of colorectal carcinoma grading
Overall SHP predicted the given diagnosis of cancer grading in 85% of 191 cases of colorectal cancer tissue samples. For each grade the sensitivity, specificity and accuracy was determined using standards for evaluating a binary classifier. The prediction was assigned to true positives (TP), true negatives (TN), false positives (FP) and false negatives (FN). These four basic values, corresponding to the actual diagnosis and classification outcome, built the basis of the evaluation of the classifier. In Fig. 8B the sensitivity, specificity and accuracy for each grade is demonstrated in a diagram. The sensitivity reaches from 83% to 94%, giving the peak ratio of the automated annotation. The specificity is determined with values from 87% up to 100% for well-differentiated (G1) carcinomas. Both values combined lead to the accuracy of the classifier, which reaches from 86% to 99%, as shown in Fig. 8A. Again this shows the improved capabilities of our SHP approach in detecting G1 tumours. Summing up these evaluations in a confusion matrix, the emphasis on the identification of well-differentiated (G1) is even more visible. Fig. 8B shows the confusion matrix of our classifier, with columns corresponding to the actual values (diagnosis by pathologists), and the rows correspond to the classification value (predicted grade via SHP). The matrix has a colour scheme for better understanding, beginning with blue for low values and ending in red for the high values. The desirable intrinsic diagonal pattern can be seen in the three red fields, referring to the fact that the classifier achieves high agreement rates. It illustrates also that well-differentiated (G1) has the best identification rates. In summary, the presented work demonstrates a workflow for fast, accurate and reproducible annotation of colorectal carcinoma. Earlier we presented that SHP is capable of distinguishing different tissue types and disease patterns like cancerous tissue regions in colorectal tissue sections, and now we advance our work for analysing the dedifferentiation state of tumour cells. This paves the way to precise and individual care for patients suffering from colorectal carcinoma.
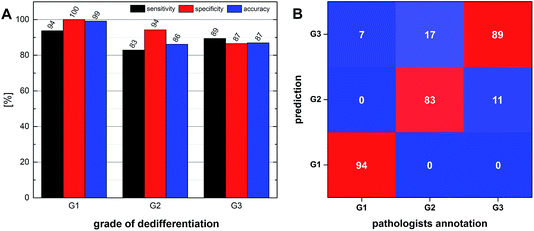 |
| Fig. 8 (A) Sensitivity, specificity and accuracy determined for each grade. Note that these statistical values refer only to a binary classifier. (B) Confusion matrix comparing the prediction of the classifier with the annotation given by the pathologist (all values in %). Red indicates a high value of congruence. The red diagonal pattern is the desirable intrinsic pattern in this presentation. The blue squares represent fields of mismatch and show low values. | |
Conclusions
This follow-up study presents a new level in SHP for classification of colon carcinoma. We demonstrate that FTIR imaging may not just classify tissue morphology and identify tumour, as has been demonstrated in numerous previous studies, but is also able to distinguish cell differentiation and thereby tumour grading. The grading, in addition to the detection of the tumour, paves the way to a better and more precise characterization of colon carcinoma. The approach of using a hierarchy of two (or potentially even more) spectral classifiers seems promising and should also lead to good results in annotation and characterization of other diseases.31 Furthermore, our study utilizes higher order features – namely relative proportions of areas associated with different grades – for characterizing the samples. In our case, a label-free, robust, reliable, operator-independent and reproducible method for the identification and characterization of colon cancer and its grading is presented.
Acknowledgements
This research was supported by the Protein Research Unit Ruhr within Europe (PURE) – project 233-1.08.03.03-031.68079 – Ministry of Innovation, Science and Research of North-Rhine Westphalia, Germany. Furthermore we want to thank Prof. Dr M. Heise for English corrections.
Notes and references
- American Cancer Society. Colorecatal Cancer, http://www.cancer.org/cancer/colonandrectumcancer/detailedguide/colorectal-cancer-key-statistics, Accessed 09/2015.
- A. E. de Jong, M. van Puijenbroek, Y. Hendriks, C. Tops, J. Wijnen, M. G. Ausems, H. Meijers-Heijboer, A. Wagner, T. van Os, A. H. Brocker-Vriends, H. F. Vasen and H. Morreau, Clin. Cancer Res., 2004, 10, 972–980 CrossRef CAS PubMed.
- A. de la Chapelle, N. Engl. J. Med., 2003, 349, 209–210 CrossRef PubMed.
- G. Avwioro, Int. J. Pharmacol. Clin. Sci., 2011, 1, 24–34 Search PubMed.
-
Ch. Wittekind and H.-J. Meyer, TNM Klassifikation maligner Tumoren. 7, Auflage, Wiley-VCH, Weinheim, 2010 Search PubMed.
- National Cancer Institute of the US National Institutes of Health, http://www.cancer.gov/cancertopics/factsheet/Detection/tumor-grade, Accessed 09/2015.
- C. Hassan, A. Zullo, M. Risio, F. P. Rossini and S. Morini, Dis. Colon Rectum, 2005, 48, 1588–1596 CrossRef PubMed.
- H. Ueno, H. Mochizuki, Y. Hashiguchi, H. Shimazaki, S. Aida, K. Hase, S. Matsukuma, T. Kanai, H. Kurihara, K. Ozawa, K. Yoshimura and S. Bekku, Gastroenterology, 2004, 127, 385–394 CrossRef.
- W. Schmiegel, A. Reinacher-Schick, D. Arnold, U. Graeven, V. Heinemann, R. Porschen, J. Riemann, C. Rödel, R. Sauer, M. Wieser, W. Schmitt, H. J. Schmoll, T. Seufferlein, I. Kopp and C. Pox, Z. Gastroenterol., 2008, 46, 799–840 CrossRef CAS PubMed.
- M. Diem, M. Miljkovic, B. Bird, T. Chernenko, J. Schubert, E. Marcsisin, A. Mazur, E. Kingston, E. Zuser, K. Papamarkakis and N. Laver, Spectroscopy, 2012, 27, 463–496 CrossRef CAS.
- L. Chiriboga, P. Xie, H. Yee, V. Vigorita, D. Zarou, D. Zakim and M. Diem, Biospectroscopy, 1998, 4, 47–53 CrossRef CAS PubMed.
- M. Diem, A. Mazur, K. Lenau, J. Schubert, B. Bird, M. Miljković, C. Krafft and J. Popp, J. Biophotonics, 2013, 6, 855–886 CrossRef CAS PubMed.
- B. R. Wood, L. Chiriboga, H. Yee, M. A. Quinn, D. McNaughton and M. Diem, Gynecol. Oncol., 2004, 93, 59–68 CrossRef CAS PubMed.
-
R. Bhargava, D. C. Fernandez, M. D. Schaeberle and I. W. Levin, PittCon, Paper 211, 2002 Search PubMed.
- W. Steller, J. Einenkel, L.-C. Horn, U.-D. Braumann, H. Binder, R. Salzer and C. Krafft, Anal. Bioanal. Chem., 2006, 384, 145–154 CrossRef CAS PubMed.
-
C. Krafft and R. Salzer, Handbook vibrational spectroscopy, 2008 Search PubMed.
-
M. Diem, P. R. Griffiths and J. M. Chalmers, Vibrational Spectroscopy for Medical Diagnosis, John Wiley & Sons, Chichester, UK, 2008 Search PubMed.
- N. Amharref, A. Beljebbar, S. Dukic, L. Venteo, L. Schneider, M. Pluot, R. Vistelle and M. Manfaita, Biochim. Biophys. Acta, Biomembr., 2006, 1758(7), 892–899 CrossRef CAS PubMed.
- M. J. Romeo and M. Diem, Vib. Spectrosc., 2005, 38, 115–119 CrossRef CAS PubMed.
- B. Bird, M. Miljkoviæ, S. Remiszewski, A. Akalin, M. Kon and M. Diem, Lab. Invest., 2012, 92, 1358–1373 CrossRef PubMed.
- P. Lasch, M. Diem, W. Hänsch and D. Naumann, J. Chemom., 2006, 20, 209–220 CrossRef CAS PubMed.
- A. Kallenbach-Thieltges, F. Großerüschkamp, A. Mosig, M. Diem, A. Tannapfel and K. Gerwert, J. Biophotonics, 2013, 6, 88–100 CrossRef PubMed.
- R. Diaz-Uriarte and S. A. de Andres, BMC Bioinformatics, 2006, 7(1), 3 CrossRef PubMed.
- A. Statnikov, L. Wang and C. F. Aliferis, BMC Bioinformatics, 2008, 9(1), 319 CrossRef PubMed.
- L. Breiman, Mach. Learn., 2001, 45(1), 5–32 CrossRef.
- DCS-Innovative Diagnostik, http://www.dcs-diagnostics.de/data/IHC_Entparaffinierung_web.pdf, Accessed 06/2015.
- P. Bassan, A. Kohler, H. Martens, J. Lee, H. J. Byrne, P. Dumas, E. Gazi, M. Brown, N. Clarke and P. Gardner, Analyst, 2010, 135, 268–277 RSC.
- P. Bassan, H. J. Byrne and F. Bonnier, Analyst, 2009, 134, 1586–1593 RSC.
- P. Bassan, A. Sachdeva, A. Kohler, J. Lee, P. Dumas and P. Gardner, Analyst, 2012, 137(6), 1370–1377 RSC.
- A. Savitzky and M. J. E. Golay, Anal. Chem., 1964, 36(8), 1627–1639 CrossRef CAS.
- F. Großerüschkamp, A. Kallenbach-Thieltges, T. Behrens, T. Brüning, M. Altmayer, G. Stamatis, D. Theegarten and K. Gerwert, Analyst, 2015, 140, 2114–2120 RSC.
- P. Bassan, J. Lee, A. Sachdeva, J. Pissardini, K. M. Dorling, J. S. Fletcher, A. Henderson and P. Gardner, Analyst, 2013, 138, 144 RSC.
- M. Miljkovic, B. Bird and M. Diem, Analyst, 2012, 137, 3954–3960 RSC.
- T. P. Wrobel, B. Wajnchold, H. J. Byrne and M. Baranska, Vib. Spectrosc., 2013, 69, 84–92 CrossRef CAS.
- M. Fleming, S. Ravula, S. F. Tatishchev and H. L. Wang, J. Gastrointest. Oncol., 2012, 3(3), 153–173 Search PubMed.
|
This journal is © The Royal Society of Chemistry 2016 |