Highly time resolved chemical characterization of submicron organic aerosols at a polluted urban location†
Received
1st July 2016
, Accepted 1st September 2016
First published on 19th September 2016
Abstract
Non-refractory submicron (NR-PM1) aerosols were measured during the late winter period (February–March) via an Aerodyne High Resolution Time of Flight Aerosols Mass Spectrometer (HR-ToF-AMS) along with Black Carbon (BC) and trace gasses in an industrial city, Kanpur, situated in the Gangetic Plain (GP) of India. The composition of NR-PM1 aerosols was dominated by organics (54%), followed by inorganics (36%), and BC (10%). Source apportionment via Positive Matrix Factorization (PMF) of AMS measured organic aerosols (OAs) revealed 6 factors. Factors are identified as 2 types of oxidized organic aerosols (OOAs), 2 types of biomass burning organic aerosols (BBOAs), freshly emitted hydrocarbon-like organic aerosols (HOAs) and oxygenated primary organic aerosols (OPOAs). A substantial increase in relative OOA contributions (200%) and O/C ratio (26%) has been observed from the high loading events (HLE) to the low loading events (LLE). Back trajectory analysis indicated that the study location received contributions from regional and long-range transported aerosols. OA composition and evolution during this study period were also very different from those observed during a fog influenced peak winter period of December–January. This is the first study to present detailed aerosol composition during the late winter period in India highlighting the changes in OA composition and chemistry within the same winter season.
Environmental impact
In this manuscript, we report the real time chemical characterization of late winter (February–March) non-refractory submicron (NR-PM1) aerosols in a polluted urban location (Kanpur) of India, using a HR-ToF-AMS (High resolution-Time of Flight-Aerosol Mass Spectrometer). Some insightful results from this study are: (1) first ever real time characterization of late winter time (February–March) NR-PM1 characterization from India revealed complete dominance of organic aerosols (OAs). (2) OA chemical characteristics and compositions differ vastly from high pollution (high aerosol loading) to low pollution events, showing a loading dependent OA evolution and oxidation. (3) OAs during the late winter period evolve very differently with lower O/C ratios from fog dominated peak winter (December–January) indicating changes in OA evolution chemistry within the same season.
|
1. Introduction
Atmospheric aerosols play a significant role in influencing radiative climate forcing directly or indirectly,1 and cause adverse health effects resulting in an increase in mortality rates.2 Especially in developing countries like India and China, the PM (particulate matter) concentrations are reaching unprecedented levels due to rapid industrialization, population explosion and lack of mitigation technologies and control strategies. Atmospheric aerosols are emitted from both natural and anthropogenic activities. Among the different size fractions of aerosols, submicron aerosols contribute most mass and number concentrations to the total ambient aerosols.3,4 Organic aerosols (OAs) generally dominate the composition of submicron aerosols.4,5 Depending upon whether OAs are directly emitted or formed via atmospheric processing, they are segregated into primary organic aerosols (POAs) and secondary organic aerosols (SOAs), respectively. Different sources of primary organic aerosols are fossil fuel combustion, biomass burning, forest fires, sea salts, and volcanic emissions.4,6 OA sources and composition are poorly characterized due to multiple origins, complex formation and transformation processes like aging, mixing, volatilization and cloud processing. Many of the processes occur at a much shorter time scale than routinely deployed 8–12 hours long offline-filter sampling, so they often fail to capture the dynamic processes and underlying mechanisms of OA transformation in the ambient environment.
In the last decade, real-time aerosol mass spectrometer measurements coupled with receptor-only based factor analysis have revolutionized source apportionment studies.7-9 Zhang et al. (2007)10 used Multiple Component Analysis (MCA) to resolve further the OOA (oxidized OA, a surrogate of the traditional SOA) into OOA-I (highly oxidized) and OOA-II (less oxidized). Lanz et al. (2007)11 first used Positive Matrix Factorization (PMF)12 to resolve the Q-AMS data into six components: OOA-I, OOA-II, charbroiling, wood burning, HOAs (hydrocarbon-like OAs) and minor sources. Other types of OAs like biomass burning OAs (BBOAs),13,14 cooking related OAs (COAs)15 and nitrate rich OAs (NOAs)16 were also identified based on characteristic fragments at m/z 60 (C2H4O2+), 55 (C3H3O+), and 58 (C3H8N+) signals, respectively. Currently, PMF is the most widely used source apportionment tool throughout the world.11,17,18
This paper presents high resolution time-of-flight AMS (HR-ToF-AMS, hereafter abbreviated as AMS) data collected in an urban background in Kanpur located in the Indo-Gangetic plain (IGP). The IGP accommodates nearly 40% of the total Indian population and has undergone large scale urbanization and land use changes. Numerous offline filter based studies have been carried out at various locations in the IGP, especially in Kanpur.19–23 However, only a handful of efforts have been made to explore the sources, variability and composition of the OA24,25 in real time, mostly during peak winter/pre-winter time. To devise an effective control strategy for pollution control and air quality improvement, seasonal variations of the sources, their chemical composition, and formation mechanisms are required. Therefore, this late winter study will provide valuable insights into those important parameters for the first time at this polluted location. Comparisons of the same parameters with peak wintertime aerosols are made to provide some novel information on how and why aerosol characteristics change from fog dominated peak winter to late winter periods.
2. Sampling and methodology
2.1 Site description
The measurement of non-refractory submicron ambient aerosols (NR-PM1, part of the submicron aerosols that evaporates within few seconds at 600 °C (ref. 26)) was carried out at the Centre for Environmental Science and Engineering (CESE) in the Indian Institute of Technology (IIT), Kanpur (26.46°N, 80.32°E, 130 amsl). The sampling was carried out from 12th February to 22nd March of 2013. It is one of the most densely populated (1452 persons per sq km as per the 2011 census) and highly polluted areas in the IGP region.21,24 It is one of the major industrial hubs, and many coal fired power plants are clustered in this region. Panki power plant is the nearest one, located nearly 3 km downwind of the IIT Kanpur campus. Grand Trunk (G-T) road is nearly 1.5 km from the sampling location which has moderate traffic in the daytime with heavy duty vehicles plying in the city from 21:00 h to 07:00 h. Several sources like automobile and industrial emissions, biomass burning, cooking and residential cooking and agricultural activities27 contribute to aerosol loadings in this area. Along with the above mentioned sources, trash burning is a major concern in the city which includes paper, wood, plastics and other garbage for heating in the winter season.
2.2 Instrumentation
The real-time measurement of ambient NR-PM1 composition (organics, sulfate, nitrate, ammonium and chloride) was carried out with a HR-ToF-AMS (High Resolution Time of Flight-Aerosol Mass Spectrometer).7 Here, only a brief description of the AMS working principle is given. It has three regions namely, an inlet system, particle sizing region and particle detection region. In the inlet region, there is an aerodynamic lens system28,29 which focuses the aerosols into a narrow beam. At the end of the inlet system, there is a chopper which modulates the particle beam through ‘Open’ and ‘Closed’ modes. Due to supersonic expansion, the particles attain size dependent velocities and move in the sizing chamber. The known length of the chamber and time taken to reach the detection region give the particle velocity through which sizing of the particles is done.30 Further, the particles have an impact on a vaporizer at 600 °C, so the non-refractory portion of those aerosols are vaporized and then ionized via standard 70 eV electron impact. Finally, the ionized fragments go to the mass spectrometer region for detection and mass quantification. Additional details on the HR-ToF-AMS can be obtained from the study of Canagaratna et al. (2007),8 DeCarlo et al. (2006),7 Drewnick et al. (2005).31 The HR-ToF-AMS is capable of measuring the high resolution mass spectra of the species, which can provide the elemental composition of organic aerosols.32
Regular ionization efficiency (IE) calibrations were done before, during and at the end of the campaign. IE/AB (air beam, signal intensity of m/z 28) values were found to be very stable with only 5–10% variation throughout the campaign. The calculated average relative ionization efficiency (RIE) value used for NH4+ is 5.6 while for organics it was assumed to be 1.4. The HR-ToF-AMS was operated in V-mode and W-mode alternatively with a duration of 1 minute each. In this study, only V mode data were used to generate mass spectra for PMF analysis as they have a high signal to noise (S/N) ratio. HEPA (high efficiency particulate arrestance; Whatman) measurements were also carried out every other day and during the IE calibration to remove gaseous interference from the AMS spectra.
For the black carbon measurement, an Aethalometer (AE 42, Magee Scientific) was operated at a flow rate of 2 L min−1 at seven different wavelengths (370, 470, 520, 590, 660, 880 and 950 nm) with a time resolution of 15 minutes. Mass concentrations at 880 nm represent black carbon which is considered for this study. 1 h averaged meteorological data including temperature, rainfall and relative humidity (RH) were measured through an Automatic Weather Station (AWS). A scanning mobility particle size analyzer (SMPS) was also deployed during the study period. A Thermo Fisher NOx (nitrogen oxide) analyzer (model 42i), SO2 (sulfur di-oxide) analyzer (model 43i), O3 (ozone) analyzer (model 49i) and CO (carbon mono-oxide) analyzer (model 48i) were deployed to measure NOx, SO2, O3 and CO respectively with 15 min time resolution. However, apart from CO, other gas analyzers were very rarely used for ambient sampling as they were used in another chamber experiment, so only CO data are reported in this study.
2.3 Data analysis
The scientific data analysis package Igor (ver. 6.3 A, Wavemetrics) was used for the AMS data analysis. A collection efficiency (CE) value of 0.5 was used to calculate mass concentrations of different aerosol species in AMS. CE is a factor used to compensate for the aerosol particles lost during transmission through aerodynamic lenses and/or bounces off vaporizer surface without getting ionized.33 This value of CE (=0.5) is calculated using Middlebrook et al. (2006)34 formulation and found to be satisfactory for this location from previous studies.24,25 In this study also, CE = 0.5 yielded an almost 1
:
1 correlation (Fig. S1 in the ESI†) with SMPS mass (converted from the volume concentration assuming a particle density of 1.4 g cm−3), so the choice of this CE value is justified. RIE values of 1.1, 1.2, 1.4, 1.3 and 5.6 were used for the HR fragments of nitrate, sulfate, organics, chloride, and ammonium, respectively. Elemental analysis of HR data is carried out using A–A (Aiken–Ambient method).
In the present study, PMF was applied on the organic spectra by using a PMF evaluation tool (PET v2.04)18 which is a graphical interface developed by Colorado University, Boulder based on the PMF2 model.35 Details of these can be found in the previously published literature.12,18 A total of 407 HR species and 22
773 data points were used. For the whole dataset, a minimum error was introduced for single ion values.18 The bad m/z values (fragments with a signal to noise ratio (S/N) < 0.2) were completely removed while the species with 0.2 < S/N < 2 were considered as weak and down-weighted by a factor of 2. The model was run from p = 1 to 8 in robust mode. The PMF's f peak values were considered from −5 to 5 with an incremental step of 0.5. A six factor solution was chosen as OA components, depending on the correlation with both internal and external factors. A detailed explanation of the factors is given in Section 3.
The effects of regional and long-range transport on NR-PM1 aerosol loading and composition are determined by performing a back trajectory (BT) analysis using the Hybrid Single-Particle Lagrangian Integrated Trajectory (HYSPLIT4) model developed by NOAA/Air Resources Laboratory (ARL).36 The meteorological data used in the computation of the trajectories come from the Global Data Assimilation System archive maintained by ARL (available online at http://www.ready.arl.noaa.gov/archives.php). First, 48 h back trajectories starting at 500 m above the ground in Kanpur (26.46°N, 80.33°E) were calculated for every 6 hours throughout the study period. Next, the trajectories were clustered according to their similarities in terms of spatial distributions using HYSPLIT4 software. The clustering principles and processes are described in the software user guide.37 The six-cluster (denoted as C1 to C6) solution was considered optimum for clustering according to the change in the total spatial variance. After clustering, the PM1 chemical compositions corresponding to the BTs in each cluster were averaged and plotted.
3. Results and discussion
3.1 General characteristics of PM1 and BC
Fig. 1b and c show the time series of non-refractory species namely organics, ammonium, sulfate, nitrate and chloride along with black carbon measured by the AMS and Aethalometer, respectively. All the values reported in this manuscript are of 15 min time resolution; 1 min AMS data are also averaged to 15 min to match other collocated instruments. The average PM1 loading was found to be 51 (±30) μg m−3 varying from 8 μg m−3 to 210 μg m−3. Among the major species, organics ranged from 4 μg m−3 to 133 μg m−3 with an average loading of 32 (±19) μg m−3 while sulfate and nitrate varied from 1 μg m−3 to 28 μg m−3 and 0.1 μg m−3 to 33 μg m−3 with average loadings of 6 (±4) μg m−3 and 7 (±3) μg m−3, respectively. BC concentrations varied from 0.2 to 38 μg m−3 with an average of 6 (±4) μg m−3. The average meteorological parameters, i.e., temperature and relative humidity (RH), are shown in Fig. 1a. The average temperature and RH values were found to be 21.9 °C (±5 °C) and 69.4% (±17.3%) for the overall campaign.
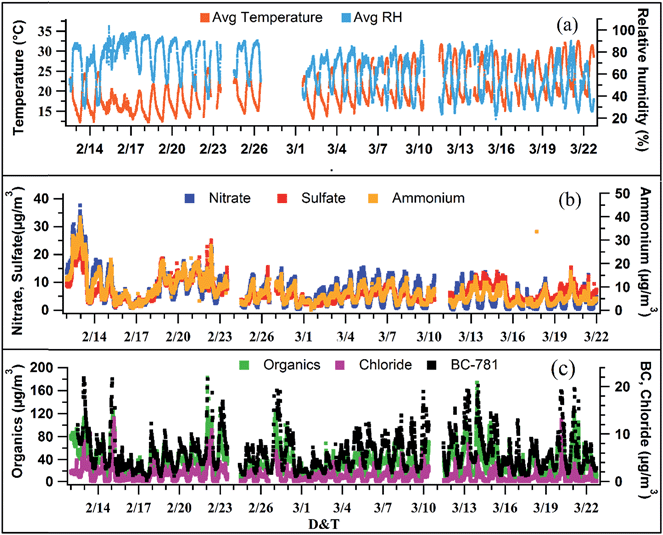 |
| Fig. 1 (a) Time series (15 min resolution) of temperature (T, °C) and relative humidity (RH, %). (b) Nitrate, sulfate and ammonium mass concentrations (μg m−3). (c) Organics, chloride, and BC concentrations (μg m−3). | |
Overall, non-refractory species account for >80% of PM1, with organics contributing nearly 50% of the total PM1 concentration (Fig. 2a). A strong diurnal variation has been observed for all the species except sulfate (Fig. 2b). The sharp decrease in all the species concentrations from late morning (11:00 h) to evening (18:00 h) is mainly due to temperature driven boundary layer expansion (Fig. 2b) which dilutes the concentration.
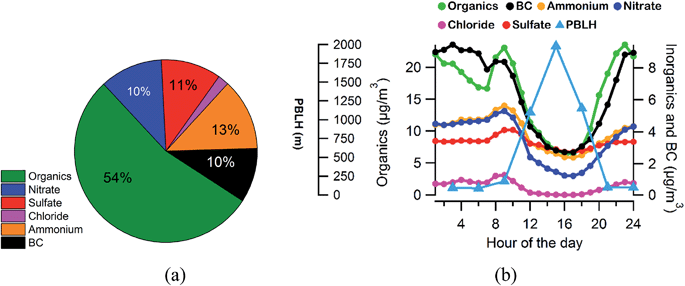 |
| Fig. 2 (a) Average NR-PM1 chemical composition based on the AMS data. (b) Diurnal profiles of NR-PM1 species, PBLH (planetary boundary layer height, 3 h resolution data) and BC. | |
The organics concentration showed an increasing trend from 06:00 h and peaked around 09:00 h, mainly due to rush hours and also due to the local biomass and trash burning for domestic purposes. The evening rush hour from 17:00 h to 21:00 h, decreasing boundary layer heights, along with trash and biomass burning activities increased the OA concentration. OA concentration reached a peak around 23:00 h mainly due to the combination of lowest PBLH and additional contributions from heavy duty vehicles plying in the city. Nitrate and sulfate also increase during evening hours with a combination of vehicular emissions and lower boundary layer heights. BC contributes around 10% of total PM1 (NR-PM1 + BC) mass, and its diurnal pattern is very much similar to that of OA but with less variation. This suggests that BC is also getting released with the organics from sources like traffic emissions, biomass burning, etc., and since it is less prone to atmospheric aging, its value was relatively stable than that of OAs. The O/C ratio increased during the afternoon due to photochemistry and decreased at night time due to more primary emissions and less efficient nocturnal chemistry (Fig. 3a).
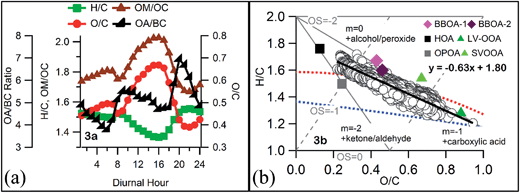 |
| Fig. 3 (a) Diurnal variations of elemental and OA/BC ratios (b) Van Krevelen diagram showing the evolution of organic aerosols along with H/C and O/C of the identified OA factors from this study. | |
The overall O/C ratio of OA during the campaign period was 0.51 with daytime (6–18 hours) O/C of 0.58 being much higher than the night time (18–6 hours) O/C of 0.45. The OA/BC ratio follows an opposite trend to the O/C ratio, which indicates that higher OA loadings are mostly associated with primary organics. The highest OA/BC ratio was observed during evening traffic rush hours when biomass burning activities also take place. This indicates that BB activities emit more OAs compared to BC, in the late night emissions from heavy duty vehicles contribute more BC leading to a decrease in the OA/BC ratio. The Van Krevelen diagram (H/C vs. O/C plot, Fig. 3b) indicates that OAs evolved with a slope of −0.63, this relatively shallow slope indicates that either functionalization reactions are happening with –OH/–COOH groups being added to the organic molecules or a –COOH group is being added with the fragmentation of the carbon backbone.38,39 A strong anti-correlation is also observed (Fig. S2†) between f60 (biomass burning marker) and f44 (a marker for carboxylic acids), this is very similar to what is reported by Cubison et al.40 from Mexico city during a study of a biomass burning plume. This strong anti-correlation indicates that the moderately oxidized biomass burning OA is being continuously oxidized to a more oxidized carboxylic acid fraction via ambient processing.40
Inorganics have contributed nearly 34% by mass to PM1, with ammonium dominating (12%), followed by a 10% contribution for both nitrate and sulfate and 2% for chloride. Among all of them, sulfate shows a weaker diurnal profile suggesting its regional transport and/or photochemical production. The continuous emission of SO2 from a nearby coal fire power plant and its subsequent atmospheric processing may have contributed to the additional production of secondary sulfate as reported in previous studies from this location.19,41 The diurnal profile of nitrate is driven by high RH and lower temperatures that result in gas to particle phase transformation of HNO3.3 The NOx coming from vehicular emissions reacts with ammonia to form NH4NO3.21,42 The diurnal profiles of nitrate, sulfate, and chloride matched with that of ammonium particularly in the morning time from 06:00 h to 11:00 h indicating possible neutralization of inorganic anions by ammonium leading to the formation of NH4NO3 (ammonium nitrate), (NH4)2SO4 (ammonium sulfate) and NH4Cl (ammonium chloride). The relative contribution of chloride is much higher (2%) compared to that reported in other global studies (0.2%, New York, Sun et al., 2011 (ref. 16) and 1%, Mexico, Aiken et al., 2010 (ref. 13)), and is expected to be coming from biomass/trash burning in the winter period.44,45 To know the extent of neutralization of the aerosols, the ratio of NH4+measured/NH4+expected [=(NH4+/18)/(2 × SO42−/96 + NO3−/62 + Chl−/35.5)] was calculated. The value turned out to be 1.08 which shows that the aerosols were completely neutralized during the study period.
3.2 Organic aerosol source apportionment via PMF (positive matrix factorization)
Fig. 4 shows the various factors, their time series, diurnal profiles and percentage contribution. A six factor solution was chosen based on the diurnal profiles, O/C ratios, correlations with the tracers and residual errors. PMF diagnostics and correlations with the tracers are shown in Fig. S3 and S4.† Table S1 in the ESI section† describes the rationale behind choosing the number of PMF factors. Fig. S5 and S6† show the factor profiles, diurnal pattern, and residues for 5 and 7 factor PMF solutions.
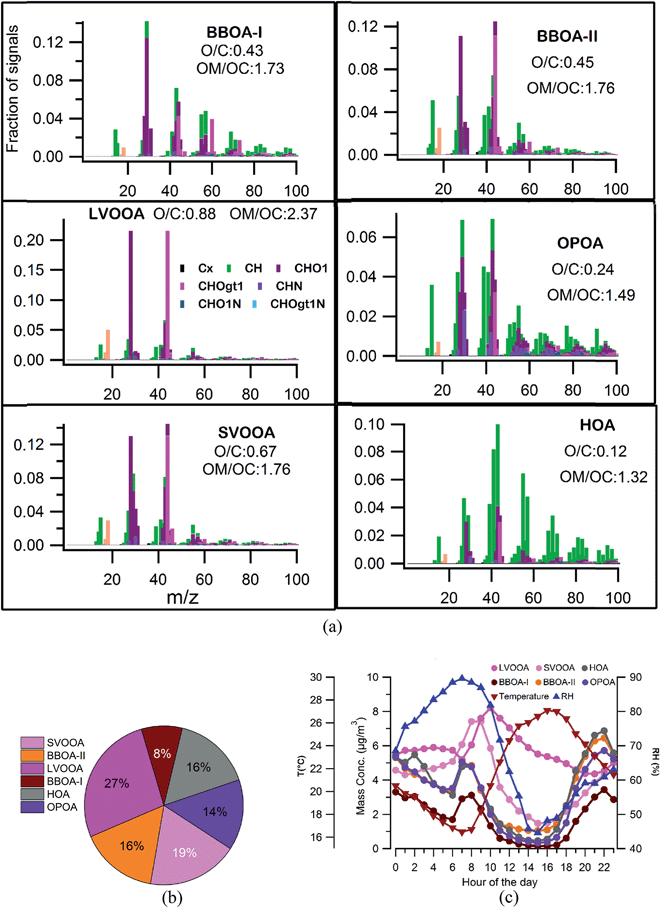 |
| Fig. 4 AMS-PMF factors for OAs: (a) the m/z spectra for the 6 factor solution, (b) percent contribution of the different factors resolved in PMF, and (c) the diurnal profiles of the different OA factors and meteorological parameters (RH & T). | |
3.2.1. HOA and OPOA.
The mass spectrum of HOAs found here is quite similar to the one found in many previous studies11,16,18,46 and is characterized by the large peaks at m/z 55 and 57 (Fig. 4a). The mass spectrum is dominated by the saturated CnH2n+1 (29, 43, 57, and 71) and unsaturated hydrocarbons CnH2n−1 (27, 41, 55, 69, and 83).43,47 This factor contributes nearly 16% to the overall OA (Fig. 4b)and the O/C ratio of this factor is 0.12 which is similar to the O/C ratios reported in urban environments such as Beijing and Mexico43 for HOAs. This factor shows a clear diurnal profile with peaks between 09:00–11:00 and 21:00–23:00 (Fig. 4c). Both peaks were mostly due to freshly emitted vehicular exhausts. HOAs have a similar trend to CO and BC (R2 = 0.59 and 0.41 vs. BC & CO, respectively, Fig. S4†) suggesting that the aerosols were mainly emitted from primary combustion activities.
Oxygenated primary organic aerosols (OPOAs) are characterized by the relative abundance of f44 (0.038) and f43 (0.069) fragments (Fig. 4a) which are very much similar to the OPOA produced in the laboratory by the oxidation of atmospherically relevant anthropogenic and biogenic precursors.48 Similar characteristics were also reported for the OPOA at the same location25 during winter. The relative contribution of the OPOA is nearly 14% (Fig. 4b). The O/C value is observed to be 0.24 which is in the range of 0.18–0.4 mostly reported for oxygenated POAs.14,25,48,49
Good correlations with C3H7 (m/z 43) and a CxHyO+ fragment (C6H10O+)16 (Fig. S4†) and a high f55/f57 (ratio of f55 and f57)25 of 2.3 suggest contributions from COAs to this factor. COAs generally have a higher m/z 55 signal and f55/f57 ratio as compared to HOAs for which m/z 57 is considered to be the marker species.25 This suggests that the OPOA is a complex mixture of aerosols coming from multiple sources like biomass burning, and cooking which cannot be resolved by PMF entirely due to similar temporal trends of those OAs as reported in previous studies.14,49 A similar type of factor has already been identified from a lab study48 and also identified in this location from a previous study.25
3.2.2. LVOOA.
The LVOOA found in this study is characterized by the highest f44 (0.24) and O/C ratio (0.88). The mass spectrum obtained in this study is quite similar to that of the OOA found in Pittsburgh18 and Langley.50 The mass spectrum (Fig. 4a) is dominated by m/z 44 (CO2+) and m/z 28 (CO+) which together contributed 46% to the factor. The LVOOA showed a good correlation with secondary sulfate (R2 = 0.56) (Fig. S4†) and the CO2+ ion (R2 = 0.68), indicating its low volatile nature. Similar values have been observed for other highly polluted cities, e.g., Beijing in China.51 An O/C ratio of 0.88 suggests its most oxidized nature among all the factors. The LVOOA mass concentration is steadily increasing from morning 06:00 h as the sun rose and reached maxima around noon (Fig. 4c). Later although it started to decrease gradually due to boundary layer expansion, it remained much higher than any other factor. This indicates that it is strongly driven by photochemical oxidation of primary and semi-volatile organic aerosols.
3.2.3. SVOOA.
The mass spectrum of the SVOOA (Fig. 4a) has a relatively lower O/C (0.67) and higher f43 (0.0807) compared to that of the LVOOA (21% higher than LVOOA's f43). The mass spectrum is similar to those found in Beijing52 and Pittsburgh.18 The time series strongly correlates with secondary nitrate (R2 = 0.86), indicating its semi volatile nature17,18 (Fig. S4†). The diurnal pattern (Fig. 4c) showed a peak around 09:00 hours just after HOA and BBOA peaked although SVOOA concentration started increasing from early morning, most likely due to the fresh formation of the OOA due to photochemistry. Afterward, this factor continuously decreased, possibly due to boundary layer expansion and photochemical conversion to the LVOOA. However, it remained significantly higher than other primary emissions which suggests that the SVOOA probably forms from the oxidation of primary emissions like HOAs and BBOAs17 and/or continued conversion of less oxidized gas phase products into the particle phase. During the FM period, the contribution from LV and SV-OOA increased slightly to 43% during this study from 34% of DJ period.24
3.2.4. BBOA.
The m/z tracers for BBOA identification are m/z 60 and 73 which represent the fragments (C2H4O2+ and C3H5O2+) of levoglucosan (C6H10O5).40,43 Two BBOAs are found in this study via PMF analysis (Fig. 4a) with very little O/C difference: BBOA-I (0.43) and BBOA-II (0.46). The mass spectrum of BBOA-I is very much similar to the trash53 and pine burning OA54 spectrum. Both the BBOA factors correlate strongly with C2H4O2+ (R2 = 0.96 and 0.76, respectively). Both the BBOAs have similar diurnal profiles (Fig. 4c) with two peaks around 08:00 h and 22:00 h. It could be due to local wood burning generally practiced for heating purposes. However, a closer look reveals some of the salient differences in their mass spectrum. BBOA-II has a higher f44/f43 ratio (=2.06) than BBOA-1 (=1.12), which indicates that organic (carboxylic) acid moieties are dominant over carbonyl moieties in BBOA-II. BBOA-I has relatively more contributions from higher m/z fragments (% of total f signal at >m/z 100 = 11%) than BBOA-II (% of total signal at >m/z 100 = 3%), indicating different types of biomass or burning conditions. Many previously filed studies have already reported various types of BBOA factors25,55 and laboratory studies showed that depending upon type of biomass and burning condition their mass spectra can differ greatly.56,57 In Kanpur apart from wood, people burn different types of biomasses like cow dung, plastics, tires, etc,58 so identification of different types of BBOAs is not surprising and has already been reported in previous studies from the same location.24,25 The average mass contribution of BBOA-I (8%) is smaller than that of BBOA-II (16%) (Fig. 4b).
It is also interesting to note that the HOA and OPOA seemed to be aligned with a slope of −2 in the Van Krevelen diagram (Fig. 3b) while BBOAs and other oxidized OAs aligned along a −1 or even shallower line. This is in line with the understanding that less oxidized OAs are first oxidized by addition of less oxidized carbonyl groups while already oxidized OAs are further oxidized by addition of highly oxidized carboxylic and/or peroxide groups.38 Similar diurnal trends of OPOAs, BBOAs and HOAs can be explained by the fact that they are all primary OAs and have similar temporal emission profiles. Biomass burning activities generally occurred in late evening to night and early morning periods during winter, overlapping with traffic rush hours. The OPOA factor identified in this study has a similar diurnal profile to that reported earlier from this location.25 Several previous AMS-PMF based studies14,43,49 reported that sources with similar temporal emission profiles (like HOAs, COAs, BBOAs, etc.) are not always clearly separated by PMF and residues of the BBOA factor may influence the HOA or COA factor. So, similar temporal trends of the sources of different types of OAs thus may have resulted in their similar diurnal trends.
3.3 Back trajectory analysis
Different back trajectories (BTs) and corresponding PM1 chemical compositions are shown in Fig. 5 (and S7†).
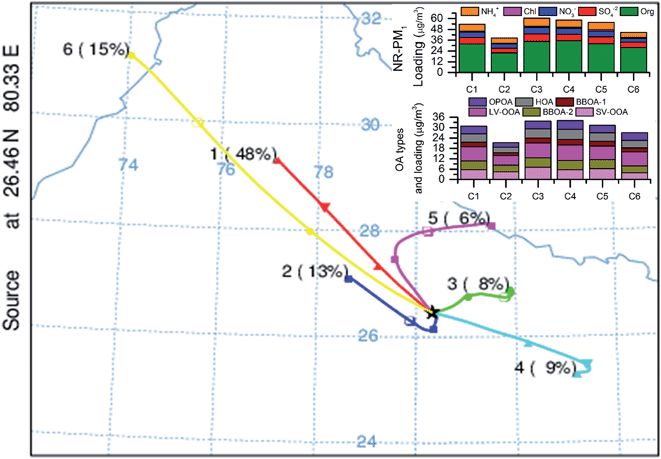 |
| Fig. 5 Air masses arriving at Kanpur (latitude and longitudes are mentioned of Kanpur as the source) as identified from back trajectory analysis. Bar charts show average composition and mass concentrations of NR-PM1 and different types of OAs in upper and lower panels, respectively. C1⋯C6 refer to clusters 1 to 6. | |
It can be seen from Fig. 5 that the site was influenced both by local and long-range transported aerosols and majority of the times air masses were arriving from the NW direction. Arrival timings of back trajectories are evenly distributed between day and night with % of daytime (06:00–18:00 hours) trajectories varied within a narrow range of 42–54%. In terms of NR-PM1 concentrations, clusters 2 and 6 (C2 & C6, Fig. 5) are the least polluted ones. These two trajectories are coming from locations where fewer industries are present (as per industrial maps of India and Pakistan), so that might be the reason for them being less polluted, while the other trajectories are coming mostly from relatively more industry populated areas. In terms of NR-PM1 composition, there is not much difference among the various trajectories. However, in terms of OA composition, the airmass associated with cluster 6, which traveled the longest path, contains slightly higher relative contributions from the most oxidized LVOOA (Fig. S7,† the difference is statistically significant, p < 0.002), indicating more processing time for OAs due to the longest travel path.
3.4 High and low pollution events
To understand the impact of high aerosol loading on the OA aerosol composition and chemistry, the whole campaign is further divided into two segments of high and low pollution events. High pollution events (HPEs) are marked when total PM1 loading is greater than the 75th percentile of the entire campaign PM1 loading value, which in this case is 76 μg m−3. Low pollution events (LPEs) are marked when PM1 loading is less than the 25th percentile of its entire campaign PM1 loading value, in this case, 30 μg m−3.59 As a whole, average aerosol loading during the HPE (100 μg m−3) was 4 times more compared to that during the LPE (22 μg m−3), Fig. 6. Emissions were higher during the HPE as average BC values during this period (8 μg m−3) were almost thrice as high compared to that during the LPE (3 μg m−3). Also the average CO value was almost 20–30% higher during the HPE compared to that during the LPE. Meteorological parameters during the HPE were also more suitable for pollution accumulation than the LPE, with lower wind speeds (1.2 m s−1vs. 1.9 m s−1), solar radiation (430 W m−2vs. 510 W m−2), temperature (18 °C vs. 26 °C), boundary layer height (500 m vs. 850 m) and higher RH (76% vs. 49%). Most of the HPEs occurred during night (70% of all HPE data points) while LPEs were more evenly distributed between day and night (58% & 42% of all LPE data points, respectively) periods. In terms of overall PM1 composition these events are not very different (Fig. 6), only the sulfate and BC contributions were enhanced slightly during the LPE compared to the HPE while relative contributions of OA remained very similar during both types of events. However, drastic changes can be seen in the OA composition (Fig. 6) with complete dominance of the most oxidized LVOOA during the LPE, with 3 times higher contributions to the OA (52%) compared to the HPE (19%) period.
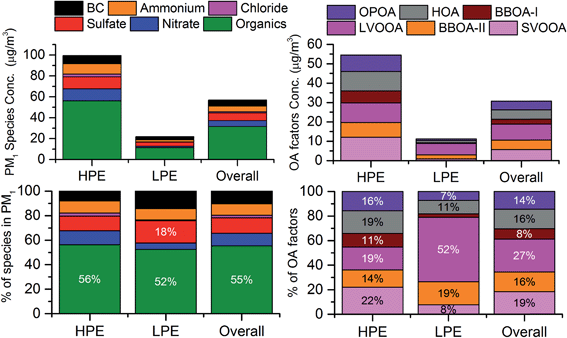 |
| Fig. 6 PM1 (left panels) and OA (right panels) concentrations and compositions during various events of the entire campaign. HPEs = high pollution events and LPEs = low pollution events. | |
In contrast, the relative contribution of the SVOOA to the total OA decreased by 3 times from the HPE (22%) to LPE (8%). In terms of mass, the SVOOA decreased 10 times from the HPE to LPE, 8.51 μg m−3 to 0.81 μg m−3. However, the LVOOA decreased only by 50% from the HPE (10.25 μg m−3) to LPE (5.87 μg m−3), this has led to the complete dominance of the LVOOA in OA composition during the LPE. Apart from these differences, Fig. 6 clearly shows that the LPE is dominated more by the oxidized OA with 60% contributions from SV + LVOOA while the HPE only has 41% contributions from SV + LVOOA. This dominance of the oxidized OA during the LPE is also quite evident from the much higher O/C ratio of the ambient OA (0.58) compared to that of the HPE (0.46). Higher OA loading favors gas to particle conversion of less oxidized gas phase oxidation products, thus bringing down the overall O/C ratio. In contrast, low OA loading favors partitioning of more oxidized gas phase products leading to an enhancement in the overall O/C ratio.39,60 Apart from this, the more primary OA contribution during the HPE may have also contributed to its observed lower O/C ratio. Slopes of the Van Krevelen diagram (H/C vs. O/C plot, Fig. S8†) also differ during the two types of events, the LPE has a shallower slope of −0.54 compared to a steep slope of −0.68 during the HPE. This indicates that for every unit of O/C increase, the H/C ratio will go down much more quickly during the HPE. Usually, a shallower slope means a different mechanism and/or addition of various functional groups to organic molecules, but the mixing of various types of OAs in different proportions can also result in different slopes in a VK diagram.24
3.5 Comparison with wintertime aerosol characteristics
To better understand how late wintertime aerosol composition, chemistry, and evolution change from the peak winter period, a comparison is made. During the December–January (DJ) period of 2012–13, the total NR-PM1 and organic aerosols loading were much higher (139 and 94 μg m−3, respectively)24 compared to what is observed in this study (51 and 32 μg m−3, respectively) during the February–March (FM) period. Several factors like meteorology and changes in emissions can result in such a massive decrease from the peak winter period. RH and temperature during DJ were very different from those in FM. On average, DJ RH (82%) was much higher than that in FM (RH = 64%) while the opposite is true for average temperature (15 °C and 22 °C, respectively). During the DJ period several fog events were observed while none occurred in the FM period, stagnant conditions during the foggy periods made the accumulation of pollutants more feasible. The average boundary layer (BL) height during the DJ period was 279 m while during the FM period it was 626 m [obtained from National Oceanic and Atmospheric Administration (NOAA) Air Resources Laboratory (ARL)], a factor of two times higher than that in the DJ period. However, average night time BL heights of the two periods (70 m vs. 108 m, for DJ & FM periods, respectively) are relatively closer compared to average daytime BL heights (458 m vs. 1149 m, for DJ & FM periods, respectively). Wind speed also increased significantly from 1.08 m s−1 to 1.65 m s−1 during the transition from the DJ to FM period. Higher BL height and WS during this study compared to peak winter time of the DJ period indicate that pollutants were more efficiently dispersed and diluted leading to lower concentrations.
The overall compositions of PM1 aerosols are very similar for DJ and FM and are with complete dominance of the OA, followed by nitrate and sulfate. However, composition, chemistry and characteristics of OAs are very different in the two periods in question. During the DJ period, OA composition was completely dominated by biomass burning OAs, both of primary and secondary (highly oxidized, O/C = 0.69) nature.24 BBOAs contributed 57% during the DJ period while BBOA contribution to OAs is found to be only 24% with the absence of a secondary (oxidized) BBOA during the FM period. Local burning activities are usually more active during the DJ period, due to much lower temperatures and frequent foggy conditions, and these activities slow down during the FM period. Contributions from HOAs have been doubled to 16% during this study period from the DJ period (7%). However, actual HOA mass concentration increased very marginally from the DJ period (4.70 μg m−3) to the FM period (5.10 μg m−3), indicating that observed enhancement in HOA relative contribution is mainly caused by a reduction in other types of OAs. Apart from composition, the oxidation levels of OAs also decreased from the DJ (0.55) to FM period (0.50) (the difference is statistically significant with p < 0.001). The lower O/C ratio during the FM period than the DJ period is a bit surprising as solar radiation (483 W m−2) was higher during FM compared to DJ (405 W m−2). Apart from that, the OA loading is much lower during the present study (32 μg m−3) compared to that of the DJ period (94 μg m−3), and a lower OA loading favors higher O/C ratio via gas to particle condensation of highly oxidized organics.39,60 So, in spite of favorable conditions for higher oxidation ratios, the overall O/C ratio during the FM period is lower compared to the DJ period. This apparent ambiguity can be explained via stagnant conditions and aqueous phase oxidation during the DJ period. Stagnant conditions have allowed more processing time of OAs while due to high RH and presence of fog, aqueous phase OA oxidation is enhanced.24,61 Also, the overall difference in the O/C ratio between these two periods is driven by night time differences (Fig. 7), while daytime O/C ratios are almost the same, which points towards night time fog (aqueous) oxidation and stagnant conditions. The role of enhanced aqueous oxidation during the DJ period is further supported by better correlation between night time RH and O/C ratio during that period compared to the FM one (Fig. S9†). The efficiency of aqueous phase oxidation is independent of the OA loading,62 so a combination of stagnant conditions and aqueous phase oxidation had enhanced the OA oxidation level during winter despite lower solar radiations and higher OA loading. Apart from differences in OA oxidation levels, the Van Krevelen diagram (H/C vs. O/C plot) revealed that some differences also exist in the evolution of OAs. Slopes of the VK diagram become much steeper from the DJ period (slope = −0.42, Fig. 7) to the FM period (slope = −0.63, Fig. 7).
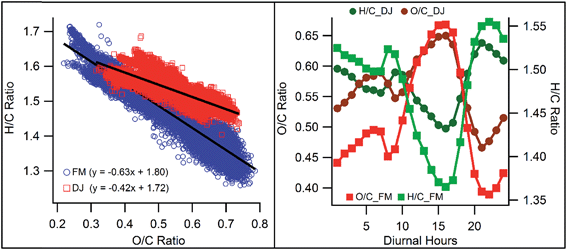 |
| Fig. 7 Van Krevelen diagram (H/C vs. O/C plot) and diurnal elemental ratios showing difference in slopes of OA evolution from the DJ (December–January) to FM (February–March) period. Differences in O/C ratios between DJ and FM periods are more pronounced during evening-night time frames compared to daytime ones. | |
Usually, shallower slopes indicate addition of –COOH and –OH groups to the carbon backbone (functionalization) and/or addition of –COOH functional groups with the fragmentation of the carbon backbone. So, the differences among these slopes indicate that the mechanism of OA oxidation is possibly different from the DJ to FM period.
4. Conclusions
A high resolution PM1 mass spectrum is obtained from a HR-ToF-AMS at an urban location (Kanpur city) in the Indo Gangetic plain. Organic aerosols dominate the PM1 mass with 54% followed by inorganics (36%) and BC (10%). PMF is applied to AMS organic mass spectra to know the different sources and types of OAs contributing to the ambient OA. PMF revealed 6 factors including one highly oxidized and aged LVOOA, moderately oxidized SVOOA, two very similar types of BBOAs with very little O/C variation, one freshly generated primary organic aerosol, HOA, and one oxygenated POA, OPOA. Overall, the OA composition is almost equally divided into primary and secondary OA factors, with LV + SV-OOA contributing 46% of total OA while the rest is primary OA factors (BBOA-I, II + HOA + OPOA). However, OA composition and O/C ratios differ significantly from high to low pollution periods as observed during the study. The HPE has 25% lower O/C ratio and 50% lesser contributions from OOAs compared to the LPE, clearly demonstrating the impact of pollution and higher OA loading on OA oxidation. Overall, the OA composition and chemistry observed in this study also differ significantly from those of the DJ (peak winter) period. During the DJ period, OAs were more oxidized in spite of lower solar radiation and completely dominated by primary and secondary BBOA factors. Also, the evolution of OAs took place differently along a much shallower slope in H/C vs. O/C space than that observed in this present study. These findings indicate that chemistry of the ambient OA was very different in the DJ period compared to this study period. Stagnant conditions, high RH and frequent fog events may have allowed more processing time and enhanced aqueous phase oxidation during the DJ period compared to this study, causing such differences. Back trajectory analysis indicated that the study location was influenced both by regional and long-range transported aerosols; however, major differences in composition and chemistry were more impacted by local sources, meteorology and processings. This information provides valuable insights into late winter chemistry of the organic aerosols at the study location and highlights the significant changes that occur in OA composition and evolution from the peak winter to late winter period.
Acknowledgements
We are grateful to IIT Kanpur for providing us a HR-ToF-AMS for research purposes.
References
- M. Kanakidou, J. H. Seinfeld, S. N. Pandis, I. Barnes, F. J. Dentener, M. C. Facchini, R. Van Dingenen, B. Ervens, A. Nenes, C. J. Nielsen, E. Swietlicki, J. P. Putaud, Y. Balkanski, S. Fuzzi, J. Horth, G. K. Moortgat, R. Winterhalter, C. E. L. Myhre, K. Tsigaridis, E. Vignati, E. G. Stephanou and J. Wilson, Atmos. Chem. Phys., 2005, 5, 1053–1123 CrossRef CAS
.
- D. W. Dockery and C. A. Pope, Annu. Rev. Public Health, 1994, 15, 107–132 CrossRef CAS PubMed
.
-
J. H. Seinfeld and S. N. Pandis, Atmospheric Chemistry and Physics: From Air Pollution to Climate Change, Wiley, Sussex, 2nd edn, 2006, vol. 2 Search PubMed
.
- M. Hallquist, J. C. Wenger, U. Baltensperger, Y. Rudich, D. Simpson, M. Claeys, J. Dommen, N. M. Donahue, C. George, A. H. Goldstein, J. F. Hamilton, H. Herrmann, T. Hoffmann, Y. Iinuma, M. Jang, M. E. Jenkin, J. L. Jimenez, A. Kiendler-Scharr, W. Maenhaut, G. McFiggans, T. F. Mentel, A. Monod, A. S. H. Prévôt, J. H. Seinfeld, J. D. Surratt, R. Szmigielski and J. Wildt, Atmos. Chem. Phys., 2009, 9, 5155–5236 CrossRef CAS
.
- Q. Zhang, J. L. Jimenez, M. R. Canagaratna, I. M. Ulbrich, N. L. Ng, D. R. Worsnop and Y. Sun, Anal. Bioanal. Chem., 2011, 401, 3045–3067 CrossRef CAS PubMed
.
- M. C. Jacobson, H. C. Hansson, K. J. Noone and R. J. Charlson, Rev. Geophys., 2000, 38, 267–294 CrossRef CAS
.
- P. F. DeCarlo, J. R. Kimmel, A. Trimborn, M. J. Northway, J. T. Jayne, A. C. Aiken, M. Gonin, K. Fuhrer, T. Horvath, K. S. Docherty, D. R. Worsnop and J. L. Jimenez, Anal. Chem., 2006, 78, 8281–8289 CrossRef CAS PubMed
.
- M. R. Canagaratna, J. T. Jayne, J. L. Jimenez, J. D. Allan, M. R. Alfarra, Q. Zhang, T. B. Onasch, F. Drewnick, H. Coe, A. Middlebrook, A. Delia, L. R. Williams, A. M. Trimborn, M. J. Northway, P. F. DeCarlo, C. E. Kolb, P. Davidovits and D. R. Worsnop, Mass Spectrom. Rev., 2007, 26, 185–222 CrossRef CAS PubMed
.
- Q. Zhang, J. L. Jimenez, D. R. Worsnop and M. Canagaratna, Environ. Sci. Technol., 2007, 41, 3213–3219 CrossRef CAS PubMed
.
- Q. Zhang, J. L. Jimenez, M. R. Canagaratna, J. D. Allan, H. Coe, I. Ulbrich, M. R. Alfarra, A. Takami, A. M. Middlebrook, Y. L. Sun, K. Dzepina, E. Dunlea, K. Docherty, P. F. DeCarlo, D. Salcedo, T. Onasch, J. T. Jayne, T. Miyoshi, A. Shimono, S. Hatakeyama, N. Takegawa, Y. Kondo, J. Schneider, F. Drewnick, S. Borrmann, S. Weimer, K. Demerjian, P. Williams, K. Bower, R. Bahreini, L. Cottrell, R. J. Griffin, J. Rautiainen, J. Y. Sun, Y. M. Zhang and D. R. Worsnop, Geophys. Res. Lett., 2007, 34, L13801 Search PubMed
.
- V. A. Lanz, M. R. Alfarra, U. Baltensperger, B. Buchmann, C. Hueglin and A. S. H. Prévôt, Atmos. Chem. Phys., 2007, 7, 1503–1522 CrossRef CAS
.
- P. Paatero and U. Tapper, Environmetrics, 1994, 5, 111–126 CrossRef
.
- A. C. Aiken, B. de Foy, C. Wiedinmyer, P. F. DeCarlo, I. M. Ulbrich, M. N. Wehrli, S. Szidat, A. S. H. Prévôt, J. Noda, L. Wacker, R. Volkamer, E. Fortner, J. Wang, A. Laskin, V. Shutthanandan, J. Zheng, R. Zhang, G. Paredes-Miranda, W. P. Arnott, L. T. Molina, G. Sosa, X. Querol and J. L. Jimenez, Atmos. Chem. Phys., 2010, 10, 5315–5341 CAS
.
- L. Y. He, Y. Lin, X. F. Huang, S. Guo, L. Xue, Q. Su, M. Hu, S. J. Luan and Y. H. Zhang, Atmos. Chem. Phys., 2010, 10, 11535–11543 CAS
.
- C. Mohr, P. F. DeCarlo, M. F. Heringa, R. Chirico, J. G. Slowik, R. Richter, C. Reche, A. Alastuey, X. Querol, R. Seco, J. Peñuelas, J. L. Jimenez, M. Crippa, R. Zimmermann, U. Baltensperger and A. S. H. Prévôt, Atmos. Chem. Phys., 2012, 12, 1649–1665 CAS
.
- Y. L. Sun, Q. Zhang, J. J. Schwab, W. N. Chen, M. S. Bae, Y. C. Lin, H. M. Hung and K. L. Demerjian, Atmos. Chem. Phys., 2011, 11, 12737–12750 CAS
.
- J. L. Jimenez, M. R. Canagaratna, N. M. Donahue, A. S. H. Prévôt, Q. Zhang, J. H. Kroll, P. F. DeCarlo, J. D. Allan, H. Coe, N. L. Ng, A. C. Aiken, K. S. Docherty, I. M. Ulbrich, A. P. Grieshop, A. L. Robinson, J. Duplissy, J. D. Smith, K. R. Wilson, V. A. Lanz, C. Hueglin, Y. L. Sun, J. Tian, A. Laaksonen, T. Raatikainen, J. Rautiainen, P. Vaattovaara, M. Ehn, M. Kulmala, J. M. Tomlinson, D. R. Collins, M. J. Cubison, E. J. Dunlea, J. A. Huffman, T. B. Onasch, M. R. Alfarra, P. I. Williams, K. Bower, Y. Kondo, J. Schneider, F. Drewnick, S. Borrmann, S. Weimer, K. Demerjian, D. Salcedo, L. Cottrell, R. Griffin, A. Takami, T. Miyoshi, S. Hatakeyama, A. Shimono, J. Y. Sun, Y. M. Zhang, K. Dzepina, J. R. Kimmel, D. Sueper, J. T. Jayne, S. C. Herndon, A. M. Trimborn, L. R. Williams, E. C. Wood, A. M. Middlebrook, C. E. Kolb, U. Baltensperger and D. R. Worsnop, Science, 2009, 326, 1525–1529 CrossRef CAS PubMed
.
- I. M. Ulbrich, M. R. Canagaratna, Q. Zhang, D. R. Worsnop and J. L. Jimenez, Atmos. Chem. Phys., 2009, 9, 2891–2918 CAS
.
- T. Gupta and A. Mandariya, Environ. Sci. Pollut. Res., 2013, 20, 5615–5629 CrossRef CAS PubMed
.
- D. K. Singh, Lakshay and T. Gupta, Environ. Sci. Pollut. Res., 2014, 21, 4551–4564 CrossRef CAS PubMed
.
- D. S. Kaul, T. Gupta, S. N. Tripathi, V. Tare and J. L. Collett Jr, Environ. Sci. Technol., 2011, 45, 7307–7313 CrossRef CAS PubMed
.
- K. Ram, S. N. Tripathi, M. M. Sarin and D. Bhattu, Atmos. Environ., 2014, 89, 655–663 CrossRef CAS
.
- V. Tare, S. N. Tripathi, N. Chinnam, A. K. Srivastava, S. Dey, M. Manar, V. P. Kanawade, A. Agarwal, S. Kishore, R. B. Lal and M. Sharma, J. Geophys. Res., 2006, 111, D23210 CrossRef
.
- A. Chakraborty, D. Bhattu, T. Gupta, S. N. Tripathi and M. R. Canagaratna, J. Geophys. Res.: Atmos., 2015, 120, 9006–9019 CAS
.
- D. Bhattu and S. N. Tripathi, J. Geophys. Res.: Atmos., 2015, 120, 766–783 CAS
.
- Q. Zhang, M. R. Canagaratna, J. T. Jayne, D. R. Worsnop and J. L. Jimenez, J. Geophys. Res., 2005, 110, D07S09 Search PubMed
.
- S. N. Tripathi, V. Tare, N. Chinnam, A. K. Srivastava, S. Dey, A. Agarwal, S. Kishore, R. B. Lal, M. Manar, V. P. Kanwade, S. S. S. Chauhan, M. Sharma, R. R. Reddy, K. R. Gopal, K. Narasimhulu, L. S. S. Reddy, S. Gupta and S. Lal, J. Geophys. Res., D: Atmos., 2006, 111, D23209 Search PubMed
.
- P. Liu, P. J. Ziemann, D. B. Kittelson and P. H. McMurry, Aerosol Sci. Technol., 1995, 22, 293–313 CrossRef CAS
.
- P. Liu, P. J. Ziemann, D. B. Kittelson and P. H. McMurry, Aerosol Sci. Technol., 1995, 22, 314–324 CrossRef CAS
.
- J. D. Allan, J. Geophys. Res., 2003, 108, 4091 CrossRef
.
- F. Drewnick, S. S. Hings, P. DeCarlo, J. T. Jayne, M. Gonin, K. Fuhrer, S. Weimer, J. L. Jimenez, K. L. Demerjian, S. Borrmann and D. R. Worsnop, Aerosol Sci. Technol., 2005, 39, 637–658 CrossRef CAS
.
- A. C. Aiken, P. F. DeCarlo and J. L. Jimenez, Anal. Chem., 2007, 79, 8350–8358 CrossRef CAS PubMed
.
- K. S. Docherty, M. Jaoui, E. Corse, J. L. Jimenez, J. H. Offenberg, M. Lewandowski and T. E. Kleindienst, Aerosol Sci. Technol., 2013, 47, 294–309 CrossRef CAS
.
- A. M. Middlebrook, R. Bahreini, J. L. Jimenez and M. R. Canagaratna, Aerosol Sci. Technol., 2012, 46, 258–271 CrossRef CAS
.
- P. Paatero, P. K. Hopke, B. A. Begum and S. K. Biswas, Atmos. Environ., 2005, 39, 193–201 CrossRef CAS
.
-
G. D. Draxler and R. Rolph, HYSPLIT (Hybrid Single-Particle Lagrangian Integrated Trajectory) Model, NOAA, 2003 Search PubMed.
-
R. Draxler, B. Stunder, G. Rolph, A. Stein and A. Taylor, HYSPLIT4 user’s guide, NOAA, 2014 Search PubMed.
- C. L. Heald, J. H. Kroll, J. L. Jimenez, K. S. Docherty, P. F. Decarlo, A. C. Aiken, Q. Chen, S. T. Martin, D. K. Farmer and P. Artaxo, Geophys. Res. Lett., 2010, 37, L08803 CrossRef
.
- N. L. Ng, M. R. Canagaratna, J. L. Jimenez, P. S. Chhabra, J. H. Seinfeld and D. R. Worsnop, Atmos. Chem. Phys., 2011, 11, 6465–6474 CAS
.
- M. J. Cubison, A. M. Ortega, P. L. Hayes, D. K. Farmer, D. Day, M. J. Lechner, W. H. Brune, E. Apel, G. S. Diskin, J. A. Fisher, H. E. Fuelberg, A. Hecobian, D. J. Knapp, T. Mikoviny, D. Riemer, G. W. Sachse, W. Sessions, R. J. Weber, A. J. Weinheimer, A. Wisthaler and J. L. Jimenez, Atmos. Chem. Phys., 2011, 11, 12049–12064 Search PubMed
.
- S. Ghosh, T. Gupta, N. Rastogi, A. Gaur, A. Misra, S. N. Tripathi, D. Paul, V. Tare, O. Prakash, D. Bhattu, A. K. Dwivedi, D. S. Kaul, R. Dalai and S. K. Mishra, Aerosol Air Qual. Res., 2014, 14, 879–891 CAS
.
- S. N. Behera and M. Sharma, Sci. Total Environ., 2010, 408, 3569–3575 CrossRef CAS PubMed
.
- A. C. Aiken, D. Salcedo, M. J. Cubison, J. A. Huffman, P. F. DeCarlo, I. M. Ulbrich, K. S. Docherty, D. Sueper, J. R. Kimmel, D. R. Worsnop, A. Trimborn, M. Northway, E. A. Stone, J. J. Schauer, R. M. Volkamer, E. Fortner, B. de Foy, J. Wang, A. Laskin, V. Shutthanandan, J. Zheng, R. Zhang, J. Gaffney, N. A. Marley, G. Paredes-Miranda, W. P. Arnott, L. T. Molina, G. Sosa and J. L. Jimenez, Atmos. Chem. Phys., 2009, 9, 6633–6653 CAS
.
- J. Lobert, W. Keene, J. Logan and R. Yevich, J. Geophys. Res., 1999, 104, 8373–8389 CrossRef CAS
.
- G. Li, W. Lei, N. Bei and L. T. Molina, Atmos. Chem. Phys., 2012, 12, 8751–8761 CrossRef CAS
.
- Q. Zhang, D. R. Worsnop, M. R. Canagaratna and J. L. Jimenez, Atmos. Chem. Phys., 2005, 5, 3289–3311 CrossRef CAS
.
- M. R. Canagaratna, J. T. Jayne, D. A. Ghertner, S. Herndon, Q. Shi, J. L. Jimenez, P. J. Silva, P. Williams, T. Lanni, F. Drewnick, K. L. Demerjian, C. E. Kolb and D. R. Worsnop, Aerosol Sci. Technol., 2004, 38, 555–573 CrossRef CAS
.
- A. T. Lambe, T. B. Onasch, P. Massoli, D. R. Croasdale, J. P. Wright, A. T. Ahern, L. R. Williams, D. R. Worsnop, W. H. Brune and P. Davidovits, Atmos. Chem. Phys., 2011, 11, 8913–8928 CAS
.
- L.-Y. He, X.-F. Huang, L. Xue, M. Hu, Y. Lin, J. Zheng, R. Zhang and Y.-H. Zhang, J. Geophys. Res., 2011, 116, D12304 CrossRef
.
- M. R. Alfarra, H. Coe, J. D. Allan, K. N. Bower, H. Boudries, M. R. Canagaratna, J. L. Jimenez, J. T. Jayne, A. A. Garforth, S. M. Li and D. R. Worsnop, Atmos. Environ., 2004, 38, 5745–5758 CrossRef CAS
.
- Y. L. Sun, Q. Zhang, J. J. Schwab, T. Yang, N. L. Ng and K. L. Demerjian, Atmos. Chem. Phys., 2012, 12, 8537–8551 CAS
.
- X. F. Huang, L. Y. He, M. Hu, M. R. Canagaratna, Y. Sun, Q. Zhang, T. Zhu, L. Xue, L. W. Zeng, X. G. Liu, Y. H. Zhang, J. T. Jayne, N. L. Ng and D. R. Worsnop, Atmos. Chem. Phys., 2010, 10, 8933–8945 CAS
.
- C. Mohr, J. A. Huffman, M. J. Cubison, A. C. Aiken, K. S. Docherty, J. R. Kimmel, I. M. Ulbrich, M. Hannigan and J. L. Jimenez, Environ. Sci. Technol., 2009, 43, 2443–2449 CrossRef CAS PubMed
.
- A. C. Aiken, P. F. Decarlo, J. H. Kroll, D. R. Worsnop, J. A. Huffman, K. S. Docherty, I. M. Ulbrich, C. Mohr, J. R. Kimmel, D. Sueper, Y. Sun, Q. Zhang, A. Trimborn, M. Northway, P. J. Ziemann, M. R. Canagaratna, T. B. Onasch, M. R. Alfarra, A. S. H. Prévôt, J. Dommen, J. Duplissy, A. Metzger, U. Baltensperger and J. L. Jimenez, Environ. Sci. Technol., 2008, 42, 4478–4485 CrossRef CAS PubMed
.
- H. Timonen, S. Carbone, M. Aurela, K. Saarnio, S. Saarikoski, N. L. Ng, M. R. Canagaratna, M. Kulmala, V.-M. Kerminen, D. R. Worsnop and R. Hillamo, J. Aerosol Sci., 2013, 56, 61–77 CrossRef CAS
.
- S. K. Saarikoski, M. K. Sillanpaeae, K. M. Saarnio, R. E. Hillamo, A. S. Pennanen and R. O. Salonen, Water, Air, Soil Pollut., 2008, 191, 265–277 CrossRef CAS
.
- A. M. Ortega, D. A. Day, M. J. Cubison, W. H. Brune, D. Bon, J. A. de Gouw and J. L. Jimenez, Atmos. Chem. Phys., 2013, 13, 11551–11571 CAS
.
- S. N. Behera and M. Sharma, Aerosol Sci. Technol., 2010, 44, 983–992 CrossRef CAS
.
- A. Misra, A. Gaur, D. Bhattu, S. Ghosh, A. K. Dwivedi, R. Dalai, D. Paul, T. Gupta, V. Tare, S. K. Mishra, S. Singh and S. N. Tripathi, Atmos. Environ., 2014, 97, 386–396 CrossRef CAS
.
- E. Kang, D. W. Toohey and W. H. Brune, Atmos. Chem. Phys., 2011, 11, 1837–1852 CrossRef CAS
.
- X. Ge, Q. Zhang, Y. Sun, C. R. Ruehl and A. Setyan, Environ. Chem., 2012, 9, 221–235 CrossRef CAS
.
- B. Ervens, B. J. Turpin and R. J. Weber, Atmos. Chem. Phys., 2011, 11, 11069–11102 CrossRef CAS
.
Footnote |
† Electronic supplementary information (ESI) available. See DOI: 10.1039/c6em00392c |
|
This journal is © The Royal Society of Chemistry 2016 |
Click here to see how this site uses Cookies. View our privacy policy here.