DOI:
10.1039/C6AY00111D
(Paper)
Anal. Methods, 2016,
8, 2013-2019
Validating heteronuclear 2D quantitative NMR
Received
13th January 2016
, Accepted 5th February 2016
First published on 10th February 2016
Abstract
NMR is used extensively for accurate quantitation of simple analytes and can, with careful application, generate SI traceable measurements. Its application for more complex analytes is limited due to spectrum complexity and signal overlap. Due to its higher resolving power, 2D qNMR is starting to emerge as a viable quantitative technique for such complex analytes but brings with it a variety of sources of experimental bias not present in the simple 1D qNMR method. In order to claim SI traceability of 2D NMR techniques, these biases and additional sources of measurement uncertainty need to be quantified. This work evaluates the application of HSQC methods to perform quantitative analysis with minimal bias and using an internally standardised glucose assay as an example, to assess the variation between experimental and theoretical biases and discuss approaches to determine the measurement uncertainty with a minimum of method validation.
Introduction
Quantitative NMR (qNMR) has predominantly been utilised in the simple one-dimensional state. It is a routine technique advantageous over traditional chromatographic techniques due to its non-destructive nature, relatively fast experiment times, simpler and faster method development with the potential to give direct SI (Systeme Internationale) traceability to its measurements.1 A major drawback is poor resolution of peaks in complex spectra, giving poor integration ranges and hindering any quantification. Large or complex molecules such as peptides, macrolides and forensic matrix samples are often not suited to quantitative measurements by 1D NMR as the signals of interest they generate lack the necessary resolution from adjacent peaks to allow accurate integration. Dispersing signals into the second dimension and exploiting the large carbon chemical shift range can increase resolution and peak specificity thus allowing for a more precise quantitation method for complex spectra. Two-dimensional NMR has traditionally been used as a qualitative tool but also gives opportunity for quantitative measurements as sufficient discrimination of peaks arise from the correlation of chemical shifts of the two nuclei.2 The complex spectra generated by 1D qNMR for large molecules, highlights the need to develop 2D qNMR approaches which can provide accurate quantitative measurements. Conventional 2D experiments such as COSY, TOCSY, J-resolved NMR, HSQC can be used but have generally been limited to qualitative measurements due to high sensitivity to pulse imperfections and instrumental parameters.3,4 Although major contributors of bias have been well established and minimised for in 1D qNMR, this has not, until now, been extended to 2D qNMR. In 1D qNMR the peak area is directly proportional to analyte concentration (provided correct sample parameters are used) allowing for accurate and precise quantitation, but the intensities of cross peaks in standard 2D NMR experiments are not directly proportional to analyte concentration due to resonance specific signal attenuation during the pulse sequence. HSQC experiments rely on the polarization transfer through J coupling and is typically one bond CH coupling, therefore signal intensity is not only dependent on the delay value of the CH coupling constant for which the experiment is optimised but additional factors such as peak multiplicity, homonuclear coupling constants and T1 and T2 relaxation during the pulse sequence also have an impact that needs to be addressed if precise quantitative measurements are to be obtained in such 2D experiments. Another major drawback to 2D qNMR is the impact of adding long relaxation delays to already long experimental times due to the low natural abundance of 13C and need for multiple FIDs to achieve adequate resolution in the 2nd time domain.5 This inhibits the use of long relaxation delays as required for 1D qNMR experiments (typically 5 × T1 (ref. 6)). The impact of the effects seen from J coupling, T1, T2 relaxation times and resolution need to be accounted for to derive a complete uncertainty budget for the application in 2D qNMR.
Many 2D quantitative advances have already been made in the last decade largely in metabolomics and more recently methods for overcoming the limitation set out by the non-uniform magnetisation transfer due to variation in JCH values. Heikkinen et al. discusses the importance of using non-optimal polarization transfer delays for quantitative measurements, highlighting that if the range of heteronuclear coupling constants is large between the two chosen signals, the corresponding peak volumes will be significantly reduced.7 Rai et al. propose an approach for quantification of metabolites by correlating measured cross peak volumes with the concentration of metabolites in urine samples using internal standards with known concentrations.8 Alternatively Gronwald et al. use calibration curves to determine the concentration of metabolites with the need to calibrate each individual signal for quantitation.9 Hu et al. present a method of extrapolating peak intensities from a series of constant time gradient selective HSQC experiments to obtain a gradient selective HSQC0 spectrum, from which concentrations of metabolites can be determined.10 All of the above methods have shown precision of around 3% or higher but have not been optimised for experimental parameters such as relaxation delays and variation in JCH coupling constants. The lack of reporting on how the parameters affect the uncertainty of the measurements, mean that the above reported methods are not applicable to the metrological community as they have no SI traceability. Metrological traceability is achieved through the International System of Units (SI), through recognized National Measurement Institutes (NMI) who aim to keep precision and uncertainty of measurement to a minimum. Traceability plays a key role in many fundamental industries for example the food and pharmaceutical industries.11,12 There is also a pressing need in proteomics and metabolomics to be able to quantify large molecules (conventionally analysed by amino acid analysis) with a great deal of accuracy and realistic, cost-effective experimental times. Hence accurate, reliable analysis using heteronuclear 2D NMR experiments, require an evaluation of additional sources of error and represent a fundamental step in producing more efficient, traceable quantitative methodologies by 2D qNMR, that have broad application to a variety of key industry sectors.
This study validates a typical 2D qHSQC experiment in order to provide an accurate and standardised calibration protocol to harmonise 2D qNMR measurements within the NMR community. This standard approach provides optimal experimental conditions and achieves SI traceable qNMR measurements with smaller uncertainties for large complex compounds, exploring the major uncertainty components attributable to different qHSQC methods. After initially assessing the suitability of the standard Bruker HSQC pulse program in implementing quantitative analysis compared to 1D protocols, the various sources of bias were investigated to compose a comprehensive uncertainty budget for qHSQC experiments. Sample dependant parameters such as 1H T1, T2 and coupling constant effects were investigated to achieve accurate quantitative measurements. Fig. 1 shows a summary of the experiments carried out to determine the different sources of uncertainty.
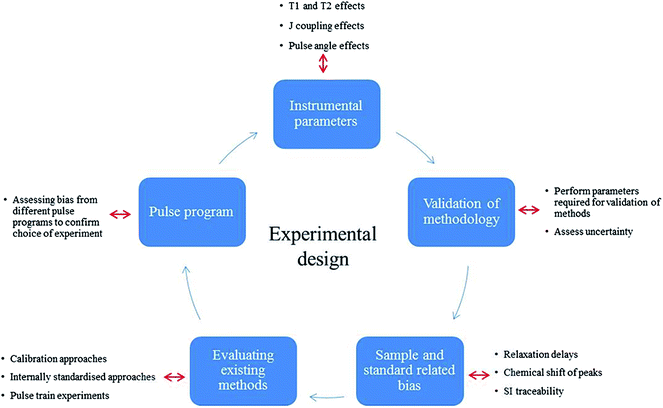 |
| Fig. 1 Experimental design for validating quantitation by HSQC. | |
Experimental
Chemicals and reagents
D-Glucose (Sigma Aldrich) spiked with an internal standard of Adenosine monophosphate (AMP) (Sigma Aldrich) at concentrations of 11, 28, 67 and 167 mM was used as a model system to determine the linearity and precision working range of the different approaches for qNMR. The AMP was found to be a suitable standard for glucose as both have anomeric protons in similar chemical environments. All samples were dissolved in deuterated D2O (Sigma Aldrich). The structures of glucose and AMP are shown in Fig. 2 along with their corresponding 1H NMR spectra.
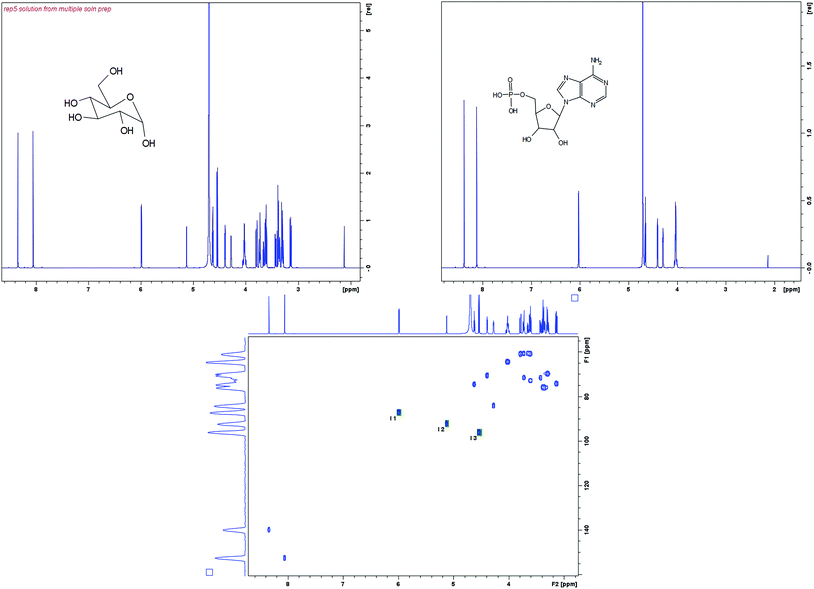 |
| Fig. 2 (Top left) Structure of glucose, with a 1H NMR spectrum of glucose spiked with AMP. (Top right) AMP structure and 1H NMR spectrum. (Bottom left) A HSQC spectrum of glucose and AMP showing the three signals integrated. | |
NMR spectroscopy experiments
A Bruker Avance III 600 MHz NMR spectrometer with 5 mm TXI Probe was used for all experiments. All experiments were carried out at 298 K. Mass measurements were carried out on either a Mettler Toledo X or a Mettler Toledo XP6 balance. 5 mm Bruker Single Use NMR tubes (product code Z117777) were used. All spectra were acquired using Icon NMR (Bruker, Germany).
The pulse programs used were all standard Bruker experiments (1H, 1H water suppression, and HSQC) (Fig. 3).13 A modified constant-time HSQC experiment as developed by John Markley et al.10 was used for the HSQC0 experiments, which is based on a standard Bruker HSQC constant-time experiment but with repeated elements to allow compensation for signal attenuation. Although the HSQC0 method is intended to be run over three increments, only two increments were used for all analyses in this case as earlier data showed that the third increment added little effect to the result outcome and two increments were sufficient to achieve the required accuracy, when extrapolating back to time zero.
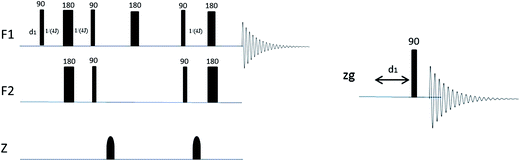 |
| Fig. 3 Left: A simplified Bruker HSQC pulse sequence consisting of shaped gradient pulses (z) which are iterated three times to create the HSQC0 experiment. F1 channel = 1H, F2 channel = 13C Right: a 1H Bruker proton pulse sequence. | |
Variations of parameters such as relaxation delay, coupling constants and pulse angles were investigated to assess their impact to HSQC0 experiments and their contribution to uncertainty. The acquisition parameters which were investigated are shown in Table 1 with the variables used as outlined.
Table 1 Parameters investigated and instrumental settings
Instrumental parameters/settings |
Bruker prefix |
Variables investigated |
Relaxation delay |
D
1
|
2, 5, 10, 20 s |
Coupling constant |
Constant 2 |
140, 145, 150, 155, 160, 165, 170 |
Pulse angle |
P
1, P2, P3, P4 |
±0.5 from optimal |
Number of scans |
NS |
4 |
Sweep width |
SW |
180 ppm (F1), 10 ppm (F2) |
Acquisition time |
AQ |
0.0047 s (F1), 0.0852 s (F2) |
Data points |
TD |
256 (F1), 1024 (F2) |
Since the HSQC0 experiments are a simple adaptation of the standard Bruker HSQC experiment, comparisons between 1D and 2D HSQC experiments were run to assess the impact of parameters which were then subsequently used to optimise HSQC0 experiments.
Processing parameters
Data was processed on TopSpin 3.2 and automatic baseline optimisation was applied after automatic phasing on all 2D data. All 1D data were phased manually with a 0.3 Hz exponential apodization applied to the FID. A polynomial baseline correction was manually applied to the peaks of interest before manual integrations were applied. Signal integration for 1D peaks and 2D cross peaks were performed on the three anomeric signals.
Results and discussion
HSQC and HSQC0 comparison with 1H NMR on a model system – trueness and linearity
2D HSQC qNMR experiments were initially compared to 1D qNMR with and without water suppression (NOESY presat) to assess the uncertainty and inaccuracy in the different experiments. Four different concentrations of gravimetrically prepared solutions (11, 28, 67 and 167 mM) of glucose spiked with AMP were prepared and run using standard Bruker pulse programs. The alpha (α) and beta (β) anomeric protons from the glucose were integrated (around 5.75 and 5.07 ppm respectively) and normalised against the anomeric proton from the AMP (around 5.99 ppm). The values obtained from the different pulse programs were then compared to the gravimetric values to demonstrate the trueness of the methods. Table 2 shows the total glucose concentration compared with the gravimetric value for the three experiment types. The water presaturation transfer experiment showed significantly lower concentrations of glucose which is expected since the glucose peaks used for integration are close to the water signal.
Table 2 The three experiments run compared against the gravimetric ‘true’ value
|
Gravimetric value |
1H |
1H sat trans |
HSQC |
11 mM |
0.28 |
0.28 |
0.22 |
0.28 |
28 mM |
0.60 |
0.60 |
0.44 |
0.61 |
67 mM |
1.70 |
1.73 |
1.33 |
1.71 |
167 mM |
3.97 |
3.99 |
3.09 |
4.03 |
Linearity on the 1H, 1H with solvent suppression and HSQC experiments were run using 7 gravimetrically prepared solutions of glucose and AMP with glucose concentrations between 1.3 and 167 mM.
The HSQC and 1D with solvent suppression experiments both show acceptable linearity over this concentration range with R2 values of 0.9980 and 0.9975 respectively.
The signal to noise (S/N) was then assessed for each of these experiments. It was found the lowest concentration solution of 1.3 mM was not within the limit of quantification (10 times the signal to noise S/N) but was still within the acceptable range for limit of detection (3 times S/N). Concentrations above 3.2 mM were all found to have S/N values acceptable for quantitation (Fig. 4).
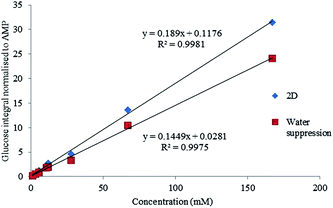 |
| Fig. 4 Linearity for a 1D water suppression and HSQC experiment shown across the range of solutions from 1.33–166.5 mM. | |
Although the linearity was acceptable for both approaches confirmed by the high R2, the water suppression experiment showed to have a bias of approximately 3% due to the proximity of the beta anomeric hydrogen to the water peak.
It has been established that the quality of saturation in water suppression experiments are impacted by low concentration solutions,14 so concentrations below 11 mM may not achieve usable data with a presaturation experiment. The linearity experiments confirmed that the HSQC based method was potentially quantitative over the range 3.2 to 167 mM providing that the biases can be quantified.
Repeatability and precision
tlsbThe 2D repeatability of the HSQC and the reproducibility of the HSQC0 experiments were evaluated. A solution of glucose (222 mM) spiked with the reference material AMP was prepared and analysed 5 times and a second experiment where 5 independent solutions of glucose (67 mM) and AMP at the same concentration were prepared and run to assess reproducibility of sample preparation. For the repeatability, only increment 1 from the HSQC0 suite of experiments were run and compared against the gravimetric values. For the reproducibility, both increments 1 and 2 were run and back extrapolation of data gave the assay value which was compared to the gravimetric assay values. The gravimetric assay values for glucose were determined with the use of a reference material (AMP) of known purity.
From Table 3 it is evident that the HSQC and HSQC0 experiments achieve quantitative results comparable to the gravimetric value.
Table 3 (Left) A solution of AMP and glucose at a 222 mM concentration run 5 times, the HSQC experiment gave a RSD of 0.89% compared to the gravimetric value. (Right) 5 independent solutions prepared at an approximate concentration of 67 mM, each run once
Repeatability |
Gravimetric |
HSQC |
Reproducibility |
Gravimetric |
HSQC |
HSQC0 |
1 |
4.50 |
4.64 |
Solution 1 |
1.54 |
1.53 |
1.54 |
2 |
4.50 |
4.53 |
Solution 2 |
1.70 |
1.75 |
1.71 |
3 |
4.50 |
4.58 |
Solution 3 |
1.83 |
1.89 |
1.88 |
4 |
4.50 |
4.61 |
Solution 4 |
1.90 |
1.90 |
1.89 |
5 |
4.50 |
4.61 |
Solution 5 |
1.91 |
1.93 |
1.93 |
|
Average |
4.60 |
|
|
|
|
|
Std dev |
0.04 |
|
|
|
|
|
% rsd |
0.89 |
|
|
|
|
Robustness
Robustness is the ability to reproduce results from an analytical method under different circumstances and variations without any significant deviation in the obtained result. In addition to repeatability the following variations were considered for the robustness of the HSQC0 method and investigated;
• Stability of analytical solution (day to day stability).
Day to day stability of the analytical process was investigated on three days over a period of 6 weeks using one of the glucose and AMP solutions. A maximum variation (% RSD) of 1% was observed in the HSQC0 experiments which showed good precision and stability of the analytical process.
• T1 relaxation delays.
A T1 experiment on three glucose and AMP solutions showed that the longest T1 was attributable to the AMP CH giving a T1 of 2.3 s; therefore a relaxation delay (D1) of 11.5 seconds (5 times T1) would be optimal for quantitation. Concentration effects on T1 were assessed by running T1 experiments on 5.5, 11 and 167 mM concentration solutions of glucose and AMP. The T1 values in seconds for the anomeric protons of glucose and AMP show negligible variation between solutions across the concentration range tested.
Given the lengthy 2D experiment times it is impractical in many laboratories to run such experiments with a D1 of 11.5 seconds (experiment time of approximately 5 hours) therefore a D1 of 5 seconds was used for all experiments. An experiment was run to assess the variability in integration originating from using the non-optimal D1 value. A set of HSQC experiments were run with a D1 of 2, 5, 10 and 20 seconds to assess the error coming from the non-optimal T1 being used. The results showed a significant bias from gravimetric values at a D1 of 2 seconds but no statistical difference when using D1 from 5–20 seconds.
• Effects of different coupling constants (homonuclear and heteronuclear).
In 2D NMR, the relationship between 2D peak area and concentration of solution is highly signal specific and depends on parameters such as T1, T2 and coupling constants. Therefore, having non-optimal values of the 1/4J tau delay will affect the accuracy of results significantly. A series of experiments were run to determine the impact of having values of the 1/4J tau delay incorrectly matched to the JCH coupling constants. Experiments with a 1/4J corresponding to a range of JCH coupling constants ranging from 145–170 Hz were run to see the implications this had on resultant peak volumes. Fig. 5 below shows the theoretical and experimental impact of having a non-optimal coupling constant value assigned for experiments with varying JC–H and JH–H values. The 1JCH values for three signals were experimentally determined by both analysis of the 13C satellites of a 1H NMR spectrum and via a heteronuclear J-resolved experiment; α glucose – 169 Hz, β glucose – 165 Hz and AMP – 166 Hz.
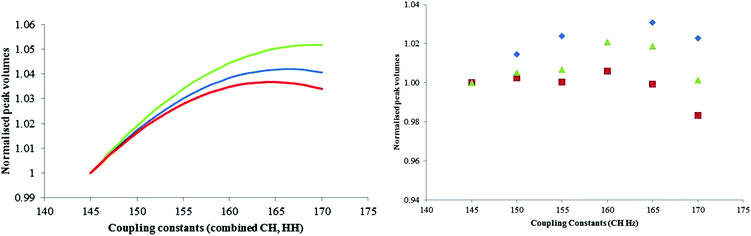 |
| Fig. 5 (Left) Theoretical implications to peak volumes on the glucose signal using different JC–H and JH–H values (green: αGlu, red: βGlu, blue: AMP). (Right) 2D experimental implications to peak volumes when varying JCH (blue: αGlu, green: AMP and red: βGlu). | |
The theoretical variation in assay values over the range 145 to 170 Hz was shown to be 0.7% which is smaller than the standard deviation from the repeatability studies carried out earlier (Section 3.2). It was shown that experimentally over the coupling constant range of 145–170 Hz the error is still not greater than the standard deviation of replicates and this concludes that the experiment is in this case insensitive to the value in coupling constant selected over the range 145 to 170 Hz.
• Impact of 90° pulse.
Incorrectly calibrated 90° pulses clearly do not impact the assay results in internally standardised 1H experiments but the impact on 2D assays is less obvious. The impact on non-optimal pulses was investigated to determine the bias or uncertainty attributable to incorrectly set pulse angles (Fig. 6). HSQC experiments were run with purposely off setting the 1H hard pulse (P1 and P2 instrumental parameters) and 13C hard pulse (P3 and P4) away from the optimal values of; P1 – 7.25 μs, P2 – 14.5 μs, P3 – 12 μs and P4 – 24 μs.
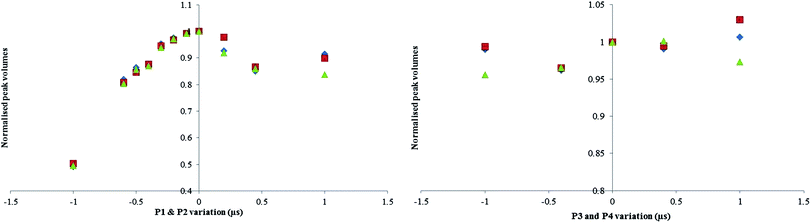 |
| Fig. 6 (Left) Impact of varying the 13C and 1H pulse widths on the peak volumes, 0 corresponds to a P1 of 7.25 μs and a P2 of 14.50 μs. (Right) Impact on 13C when varying P3 and P4, 0 corresponds to a P3 of 12 μs (for both; blue: αGlu, red: βGlu, green: AMP). | |
The P1 and P2 graph confirms that the maximum signal intensity peaked at about 7.25 μs, a larger affect is seen for a badly offset P1 and P2 experiment (50% error at −1 μs) compared to the P3 and P4 offset experiment (5% error at −1 μs).
For incorrectly calibrated 90° pulse experiments, significant bias is only seen where the error is substantial i.e.> ±0.5. It has been observed that over the range of ±0.2 μs which is where the error in 90° pulse is most likely to be, there was no statistically significant difference seen, and is covered by the standard deviation of results. For that reason an uncertainty contribution associated to pulse angle has not been included in Table 3.
Uncertainty of measurement
The major sources of uncertainty and contributors to bias for 2D analyses have been established with correction factors quantified and calculated using multiple solutions and determinations (Table 4).
Table 4 Uncertainty contribution to the major sources of bias, the total uncertainty is calculated using the following equation,
(ref. 15)
Parameter |
Maximum error on determination |
Maximum impact on individual signal intensity |
Uncertainty contributuion |
CH coupling |
0.5 Hz |
0.2 |
0.12 |
HH coupling |
0.2 Hz |
0.03 |
0.02 |
T
1 value |
0.2 s |
0.3 |
0.17 |
T
2 value |
0.1 s |
0.2 |
0.12 |
Total uncertainty |
|
|
0.24% |
Conclusion
Both 1D and 2D qNMR measurements were carried out using internally standardised qNMR assays on a model system of glucose and adenosine monophosphate. The standard approach to handling attenuation issues would be through construction of a calibration curve but many of the applications of interest lack sufficient reference materials to adopt such an approach.
By choosing a simple example of an HSQC assay of glucose, we have shown how biases and uncertainties can be generated and quantified through a mixture of theoretical and practical measurement which can be applied for more complex matrix materials where calibration curve approaches are not viable. The HSQC0 experiment was shown to be a useful tool to help validate such methods but did not, in this case improve the experimental accuracy.
This paper has given comprehensive insight to the importance of the biases and errors associated with 2D quantification for the first time. Through understanding the effects of homonuclear and heteronuclear coupling, T1/T2 relaxation along with the additional sources of uncertainty generated through 2D NMR, a simple, realistic uncertainty budget can be derived that allows measurements to be made with full S.I traceability. A total uncertainty of 0.24% was attributable to variations in coupling constant and T1/T2 relaxation; however a full uncertainty budget would encompass the standard deviation from repeatability studies and sample preparation uncertainty. This would allow appropriate compensation for complex mixtures where time or sample availability may not permit for a calibration type experimental design.
The choice of internal standard is critical to avoid significant experimental bias that would require the use of response factors. This validation study showed that in this case there was no significant bias between the analytes, but for cases where the NMR properties of the internal standard are less well matched with the analyte, a bias will be evident and a correction factor can be derived from the data generated.
Through the provision of a straightforward method for the determination of uncertainty, the components of complex mixtures for a variety of applications in metabolomics and proteomics can be quantified with the requisite traceability to SI units. This represents a useful tool, with broad appeal to analysts in the field of quantitative NMR.
Acknowledgements
The authors would like to thank LGC Ltd for the funding of Fahmina Fardus-Reid. Dr Jean-Marie Peron, Kingston University is thanked for excellent technical support.
References
- F. Malze and H. Janke, J. Pharm. Biomed. Anal., 2005, 38, 813–823 CrossRef PubMed.
- R. A. Chylla, K. Hu, J. J. Ellinger and J. L. Markley, Anal. Chem., 2011, 83, 4871–4880 CrossRef CAS PubMed.
- E. Martineau, P. Giraudeau, I. Tea and S. Akoka, J. Pharm. Biomed. Anal., 2011, 54, 252–257 CrossRef CAS PubMed.
- P. Giraudeau, G. S. Remaud and S. Akoka, Anal. Chem., 2009, 81, 479–484 CrossRef CAS PubMed.
- P. Giraudeau, Magn. Reson. Chem., 2014, 52, 259–272 CrossRef CAS PubMed.
- S. K. Bharti and R. Roy, Trends Anal. Chem., 2012, 35, 5–26 CrossRef CAS.
- S. Heikkinen, M. M. Toikka, P. T. Karhunen and I. A. Kilpelainen, J. Am. Chem. Soc., 2003, 125, 4362–4367 CrossRef CAS PubMed.
- R. K. Rai, P. Tripathi and N. Sinha, Anal. Chem., 2009, 81, 10232–10238 CrossRef CAS PubMed.
- W. Gronwald, S. M. Klein, H. Kaspar, R. S. Fagerer, N. Nurnberger and K. Dettmer, Anal. Chem., 2008, 80, 9288–9297 CrossRef CAS PubMed.
- K. Hu, J. J. Ellinger, R. A. Chylla and J. L. Markley, Anal. Chem., 2011, 83(24), 9352–9360 CrossRef CAS PubMed.
- F. Schwagelle, Meat Sci., 2005, 71, 164–173 CrossRef PubMed.
- U. Holzgrabe, Prog. Nucl. Magn. Reson. Spectrosc., 2010, 57, 229–240 CrossRef CAS PubMed.
-
http://www.rit.edu/cos/scms/pdf/300MHZ-NMR/pulse-prog-catalogue.pdf, accessed 04 September 2015.
- G. Zheng and W. S. Price, Prog. Nucl. Magn. Reson. Spectrosc., 2010, 56, 267–288 CrossRef CAS PubMed.
-
http://www.npl.co.uk/acoustics/underwater-acoustics/research/uncertainties-in-free-field-hydrophone-calibration/*/viewPage/2, accessed 01 February 2016.
|
This journal is © The Royal Society of Chemistry 2016 |