Using cluster analysis to characterize meaningful learning in a first-year university chemistry laboratory course
Received
2nd May 2015
, Accepted 31st July 2015
First published on 31st July 2015
Abstract
The Meaningful Learning in the Laboratory Instrument (MLLI) was designed to measure students' cognitive and affective learning in the university chemistry laboratory. The MLLI was administered at the beginning and the end of the first semester to first-year university chemistry students to measure their expectations and experiences for learning in their laboratory course. To better understand what students' expectations for learning were fulfilled, and what expectations went unmet, cluster analysis was used to examine students' expectations and experiences as measured by their average scores on the MLLI at both the beginning and the end of the semester. The cluster solution yielded four clusters. Three of the four clusters consisted of parallel responses, i.e., the first cluster had both low expectations and low experiences, the third cluster had both high expectations and high experiences, and the second cluster was in the middle of the first and third. The fourth cluster had expectations similar to those of the high cluster, but surprisingly had experiences similar to those of the middle and low clusters. Descriptions of each cluster of students, including distinguishing characteristics of their learning in the laboratory, and the implications of this research are discussed.
Introduction
While the majority of chemists would agree that chemistry is a laboratory science, the value of the university chemistry laboratory has been called into question due to the large amount of time, money, and resources that it requires in the absence of evidence that the laboratory helps students learn chemistry (Hofstein and Lunetta, 1982, 2004; Hilosky et al., 1998; Nakhleh et al., 2002; Hawkes, 2004; Reid and Shah, 2007; Elliot et al., 2008; Sevian and Fulmer, 2012). Evidence for how students learn and their perceptions of learning in their laboratory courses is needed to distinguish learning in the laboratory and demonstrate its necessity to learning chemistry (National Research Council, 2012).
Hofstein and Lunetta's (1982) seminal review of research on learning in the laboratory led the call for “comprehensively examining the effect of laboratory instruction on student learning” due to the fact that “there is insufficient data to confirm or reject convincingly many of the statements that have been made about the importance and effects of laboratory teaching” (p. 212). In their 2003 re-examination of their 1982 review, Hofstein and Lunetta stand by their claim that “the assumption that laboratory experiences help students understand materials, phenomena, concepts, models, and relationships, almost independent of the nature of the laboratory experience, continues to be widespread in spite of the sparse data from carefully designed and conducted studies” (Hofstein and Lunetta, 2004, p. 46). No longer can the assumption be made that laboratory experiences are indeed enhancing students' learning experiences; robust research studies are needed to provide evidence for such conclusions. Since these reports, studies have been published on reformed laboratory curricula (Cooper and Kerns, 2006; Sandi-Urena et al., 2011a, 2011b, 2012), the role of the graduate teaching assistant (Herrington and Nakhleh, 2003; Sandi-Urena et al., 2011a; Sandi-Urena and Gatlin, 2013), and of faculty goals for student learning (Bruck et al., 2010; Bretz et al., 2013; Bruck and Towns, 2013). The results from the studies on the role of the graduate student teaching assistants and the faculty goals for laboratory learning suggest ways to create a learning environment where explicit instructional objectives can be met. Despite these recent reports, there has yet to be research on what and how students are learning in the laboratory environment (National Research Council, 2012). Such data would be exceptionally useful when making evidence-based decisions on innovations in university laboratory pedagogy and/or curriculum.
In order to measure students' perceptions of their learning in the university chemistry laboratory, we developed the Meaningful Learning in the Laboratory Instrument (MLLI) (Galloway and Bretz, 2015) using Joseph Novak's Theory of Meaningful Learning and Human Constructivism (Novak, 1993, 2010; Bretz, 2001). A meaningful learning experience “underlies the constructive integration of thinking, feeling, and acting leading to human empowerment for commitment and responsibility” (Novak, 2010, p. 18). The opposite of meaningful learning is rote learning in which the student chooses to memorize new knowledge rather than integrate it with prior knowledge (Ausubel, 1968; Novak, 2010). In order for meaningful learning to take place, the learner must have relevant prior knowledge, the new knowledge must be presented to the learner in a meaningful way, and the learner must choose to relate the new knowledge to the prior knowledge (Ausubel, 1968; Novak, 1993, 2010; Bretz, 2001). Underlying these criteria are the distinct, but connected and interactive, systems humans have for acquiring new knowledge, meaning making, and storing information. These distinct human learning systems are cognitive learning, affective learning, and psychomotor learning. As the major areas of the brain involved in long-term memory are also involved in storage of emotion, there is an inherent integration of feeling with thinking and acting when a human is learning new knowledge (Neidenthal, 2007). Thus, students' prior experiences and expectations can greatly influence how students may choose to act in their chemistry laboratory course.
To better understand the cognitive, affective, and psychomotor learning domains, consider the example of a chemistry student who conducts an experiment to explore the activity series of metals. The student fills a well plate up with different salt solutions, drops different metals into each one, and records any observations as to whether or not a reaction took place. In this example, the student is engaging is psychomotor learning through the tactile movements of filling up the well plate, adding metals, and writing observations. Cognitive learning takes place as the student decides whether a reaction takes place, how to interpret the results, and making sense of why some metals reacted and some didn't. While less obvious, the affective domain is activated as the student attempts to retrieve prior knowledge about oxidation–reduction reactions, laboratory equipment, how to fill out a laboratory notebook, etc. She may be excited to see bubbles, color changes, formation of precipitates. She may feel concern if her results differ from those of her peers. The student could be concerned by the lack of visible evidence for a reaction – did she just not see the reaction? That is to say, a students' ability to carry out the experiment and write down observations does not directly indicate that the student understands why certain reactions took place and why some metals did not react at all. The student's prior knowledge and experiences, both cognitive and affective, will influence how the student attributed meaning to the laboratory experiment.
There has been little research into the integration of the cognitive, affective, and psychomotor learning domains in students' learning experiences. Some studies have looked at the relationship between either thinking and doing or thinking and feeling, but few examine the necessary integration of all three learning domains. One study used Novak's theory of meaningful learning as a lens to analyze student descriptions of their learning in an analytical laboratory course by classifying their activated prior knowledge as cognitive, psychomotor, and/or affective (Emenike et al., 2011). Results from this study concluded that different types of laboratory experiments influenced how students viewed their learning, their prior knowledge, and their subsequent choice to learn (Emenike et al., 2011). Another study used structural equation modelling (SEM) to examine students' thinking, feeling, and doing measured through short surveys and national standardized chemistry exams (Brandriet et al., 2013). Through testing one theoretical and seven alternative SEM models, the strongest fitting model was the theoretical model with relationships between students' thinking, feeling, and doing in their learning of chemistry. Such studies are just beginning to explore the meaning-making experiences students have while learning chemistry. Despite the value of studies to inform the creation of evidence-based laboratory curricula, there have yet to be studies investigating the integration of the cognitive, affective, and psychomotor domains learning university chemistry laboratory learning.
While the “doing” of laboratory work (psychomotor) is obvious and visible to both students and instructors in an undergraduate chemistry laboratory course, the necessary thinking and feeling needed to achieve meaningful learning are less obvious and visible. Therefore, MLLI asks about students' cognitive and affective learning in the chemistry laboratory while performing experiments (psychomotor). MLLI is administered both before and after a semester of chemistry laboratory instruction as the MLLI assesses students' expectations for learning and their corresponding experiences. Just as students bring prior knowledge of content to the lecture or laboratory, students also bring with them to the laboratory a variety of ideas about how they will learn including their feelings and beliefs about learning and the skills they will need to use to succeed (Reddish et al., 1998; Grove and Bretz, 2007). Measuring students' expectations for learning in the laboratory, and whether or not these expectations were met, will generate new insights into the current function of the university chemistry laboratory.
Research questions
The primary question guiding this research was what does cluster analysis reveal about students' expectations and experiences, as learners in the chemistry laboratory, as measured by their MLLI responses?
Methods
Data collection & sample description
Prior to data collection, approval from the Institutional Review Board was obtained for human subjects research. The MLLI is a 31-item assessment tool designed to measure students' cognitive and affective expectations and experiences in regards to learning in their chemistry laboratory courses. Of the 31 items, 16 items are worded to reflect experiences that contribute to meaningful learning, and 14 statements are worded to reflect experiences that hinder meaningful learning. There is one indicator item used as a check to see if students are reading the survey questions. The context for the students was set as the “doing” of laboratory experiments. The stem for each item was “When I perform experiments in my chemistry laboratory course, I expect…” (the verb tense was changed from future to past tense for the post-test). Each item on the MLLI was coded by multiple raters (with an initial agreement of 83% and final agreement of 100%) as cognitive, affective, or cognitive/affective using the meaningful learning framework, resulting in 16 cognitive items, 8 affective items, and 6 cognitive/affective items. Cognitive items ask about experiences where students ought to engage their minds during the experiments (e.g., Q3: to make decisions about what data to collect or Q11: to think about what the molecules are doing). Affective items ask about experiences where students' feelings may impact their laboratory work (e.g., Q8: to be excited to do chemistry or Q9: to be nervous about making mistakes). By extension, a cognitive/affective item inquires about an experience that is both cognitive and affective in nature (e.g. Q20: to worry (affective part) about the quality of my data (cognitive part)). Students were asked to indicate their percent agreement with each statement. The MLLI was administered online via Qualtrics Survey software where the answer format was a slider bar from 0% (completely disagree) to 100% (completely agree). At the beginning of the semester, the MLLI asked students about their expectations for learning chemistry in their laboratory course. At the end of the semester, the statements were modified from future to past tense to ask students about their learning experiences. Composite scores were calculated for each cognitive, affective, and cognitive/affective scale by first reverse coding the negatively worded items and then averaging the item responses for the items on each scale. A detailed report on the development of the MLLI has been reported elsewhere (Galloway and Bretz, 2015).
The MLLI was administered to university students at the beginning and the end of the first semester of a first-year chemistry laboratory course at a midsize liberal arts university in the midwestern United States. Both chemistry majors and non-chemistry science majors participated in the study. For the pre-test, the Qualtrics link was sent to the students during the first week of classes, before they had completed any laboratory work. This timing was crucial to measuring students' expectations for learning in the laboratory. The post-test survey link was sent to the students near the end of the semester (within the last two weeks). Students were permitted two weeks to complete the survey for both the pre and post-test. For the first-year chemistry laboratory course for non-chemistry majors, the survey link was sent to the students as part of their pre-lab assignments. For the chemistry-majors first-year chemistry laboratory course, the survey link was sent to students through the online course management software. The students in the chemistry-majors course also were offered the opportunity to receive nominal extra points if they completed the assessment both at the beginning and the end of the semester. During the fall 2013 semester, 839 first-year chemistry students completed the MLLI at least once, at either the pre or post-test administrations. Of those students, 436 students completed both the pre and post-test such that their MLLI responses could be matched and any changes in their responses could be explored. Of the 839 who responded at least once, 218 students took the MLLI only at the beginning of the semester, and 185 took the MLLI only at the end of the semester. Descriptive statistics were calculated and visualizations were compared for the students with matched data with the students who took the MLLI only once. This examination showed the averages for the composite variables were within three percentage points for the matched students compared with those who participated only once. Of the students who completed the MLLI both at the beginning and end of the semester, 60% were female. This sample has slightly more females than the university which is 52% female. This sample consisted of 87% white students which is representative of the university.
In these laboratory courses, students performed 11 experiments in a 14 week semester. The majority of the experiments that the students conducted were structured inquiry with some guided inquiry experiments throughout (Bruck et al., 2008). The topics covered included stoichiometry, acid/base titrations, oxidation–reduction reactions and the activity series, thermochemistry, UV-VIS spectrophotometry, and properties of gases. The students worked both individually and in small groups throughout the course, and they were expected to complete the experiments in the allotted 3 hour lab time. Students completed individual lab reports due the week following the experiment. The format of the lab reports was a summary data sheet with one formal lab report during the semester. Each lab room held a maximum of 42 students with 2 graduate teaching assistants per lab room.
Cluster analysis
The goal of this analysis was to explore students' MLLI responses in order to identify groups of students who reported similar cognitive and affective expectations and experiences with regard to their learning in a first-year undergraduate chemistry laboratory course. Grouping or classifying objects in chemistry is common. We classify elements, intermolecular interactions, and types of reactions, to just name a few. The advantage of classifying large sets of objects into a smaller number of groups is that these smaller groups can offer a concise description of the patterns and similarities of the objects in the group with convenient and efficient retrieval of information (Everitt et al., 2011). In addition to summarizing the characteristics of each group, the classification of objects allows for predictions and inferences about the objects. While several classifications may be possible, meaningful classifications are ultimately those that provide the most useful information. For instance, organic chemists could choose to classify compounds by whether or not they contain oxygen or nitrogen. While those classifications would be accurate, a far more useful classification is based upon functional groups (alkane, ether, ester, ketone, aldehyde, carboxylic acid, amine, amide, etc.) that sort compounds into groups based on structural similarities that can be used to predict function. Thus, the statistical technique of cluster analysis was chosen for this study, which sorts respondents into groups (‘clusters’) based on patterns in their response choices.
Cluster analysis has been previously reported as a valuable analytical technique in chemistry education research (Drechsler and Van Driel, 2009; Lewis et al., 2009; Brandriet and Bretz, 2014; Chan and Bauer, 2014; Linenberger and Holme, 2014; Raker and Holme, 2014). Recent studies using cluster analysis have examined students' misconceptions about redox reactions (Brandriet and Bretz, 2014), affective characteristics influencing at-risk students in chemistry (Chan and Bauer, 2014), biochemistry instructors' use of representations in instruction and assessment (Linenberger and Holme, 2014), and teachers' familiarity with assessment terminology (Raker and Holme, 2014). The knowledge of particular groupings of students or teachers yields valuable information for researchers and practitioners for developing evidence-based interventions, curricula, and programs. For example, results from the Brandriet and Bretz (2014) study about students' conceptual understanding of redox chemistry, and confidence regarding that understanding, revealed three groups of students: one with high conceptual understanding and high confidence, and two groups with poor conceptual understanding. However, the two groups with poorer understanding differed in an important way as revealed by cluster analysis – one of the two had low confidence while the other had confidence equivalent to that of the high conceptual understanding group. Clearly, the latter group with poor understanding and misplaced confidence would require a different kind of instructional intervention than students who are more aware of the gaps in their knowledge frameworks.
As with other statistical techniques, performing cluster analysis requires the researcher to make multiple methodological decisions based on the type of data gathered and the questions to be answered. Everitt et al. (2011) details Milligan's (1996) list of cluster analysis steps with accompanying descriptions of these considerations. The methodological decisions regarding cluster analysis in this study are described below.
The objects to be clustered were the first-year chemistry students who responded to the MLLI both at the beginning and end of the fall semester of a first-year chemistry I laboratory course. Aldenderfer and Blashfield (1984) emphasize that “the choice of variables to be used with cluster analysis is one of the most critical steps” and that “the importance of using theory to guide the choice of variables should not be underestimated” (p. 20). In total, six variables were selected as the clustering variables using the meaningful learning framework as a guide to make this choice. To explore how students' MLLI responses changed from the beginning to the end of the semester, the clustering variables were chosen to be the composite averages of the cognitive, affective, and cognitive/affective variables for the pre and post-tests. The choice was made not to standardize the variables. Even though the variables were a priori on the same unit scale, there was some variation in the standard deviations of the clustering variables. Standardizing the variables would have assigned each the same weight with a “hope of achieving objectivity” to place the students in clusters based on these variables (Kauffman and Rousseeuw, p. 11). By purposefully choosing to forego standardization, our cluster solution considered the intrinsic, relative weights of the variables such that the resulting interpretation of the clusters would be based upon the students' responses, weighted by their expectations and experiences – not artificially deemed of equal weight.
Prior to clustering, the collinearity of the clustering variables was examined because highly correlated variables can be overrepresented in the cluster solution (Mooi and Marko, 2011). Table 1 shows the Spearman's Rho correlations for the six clustering variables. The majority of the correlations are less than 0.5 except for the correlations between affective and cognitive/affective, both pre and post. This correlation could show through in how the clusters are formed, but again, the cluster solution would represent the intrinsic, relative weights of the variables as voiced by the students through their responses on the MLLI. Multicollinearity was further examined by calculating the variance inflation factor (VIF). A high VIF can indicate redundancy or that one variable is highly influenced by one or more of the other variables (Cohen, 2008). VIF values were calculated using SPSS where one variable was specified as the dependent variable and the other five as the independent variables and repeated for all clustering variables. All VIF values were less than 2.6 confirming the lack of multicollinearity among the clustering variables.
Table 1 Spearman's Rho correlation matrix for all six clustering variables
MLLI scales |
Pre cognitive |
Pre affective |
Pre cognitive/affective |
Post cognitive |
Post affective |
* Significant at the 0.05 level. |
Pre affective |
0.48* |
|
|
|
|
Pre cogn./aff. |
0.49* |
0.71* |
|
|
|
Post cognitive |
0.52* |
0.24* |
0.22* |
|
|
Post affective |
0.22* |
0.42* |
0.26* |
0.48* |
|
Post cogn./aff. |
0.18* |
0.34* |
0.35* |
0.48* |
0.71* |
The final methodological decisions to be made concerned the clustering procedure itself. Hierarchical agglomerative clustering was selected using Ward's method as the linkage method and squared Euclidian distance for the dissimilarity measure. Hierarchical clustering was chosen because no hypothetical cluster solution could be identified based upon prior research. Hierarchical agglomerative starts with all the objects clustered as separate individual clusters and combines objects together. How and when the objects are fused together depends on the proximity measure and clustering method. Ward's method was chosen as the linkage method because it combines clusters by minimizing the sum of squared deviations within a group; thus, the clusters produced by Ward's method should have greater similarities within clusters and greater differences between clusters based on the clustering variables (Ward, 1963). In hierarchical agglomerative clustering, each step is irrevocable, so having a linkage method that fuses clusters that result in the smallest increase in the overall within cluster variance is favorable (Norusis, 2011). Squared Euclidian distance was chosen as the proximity measure (or measure of dissimilarity) for this analysis because the clustering variables can be interpreted as continuous in nature and the Squared Euclidian distance can be interpreted as a physical distance between points in Euclidian space (Everitt et al., 2011). The clustering procedure was carried out using SPSS 21; the output was analysed for distinctiveness and meaningful interpretation of the clusters.
Results & discussion
Descriptive statistics
Analysis of the changes in students' responses from the pre-test to the post-test has been reported (Galloway and Bretz, 2015). Summary statistics are listed in Table 2 for the cognitive items, affective items, and cognitive/affective items. While the sample showed significant decreases on all three scales as a whole, scatterplots (Fig. 1) comparing pre and post scores indicate that the difference between the mean affective responses may not be representative of the entire sample. The solid line at y = x is included on each plot to aid in visual interpretation of changes in scores from pre to post. Data points above the line indicate an increase in score from pre to post while data points below the line indicate a decrease in score from pre to post.
Table 2 Descriptive statistics for MLLI pre and post-test administrations
|
Pre |
Post |
Cognitive |
Affective |
Cognitive/affective |
Cognitive |
Affective |
Cognitive/affective |
Mean (SD) |
69.9 (10.8) |
54.7 (17.0) |
55.1 (14.7) |
57.9 (12.9) |
50.3 (18.1) |
44.8 (14.6) |
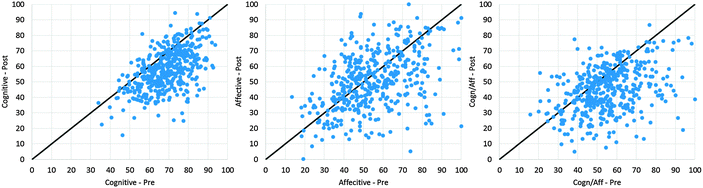 |
| Fig. 1 Scatterplots of composite subscales pre vs. post. Reprinted with permission from Galloway K. R. and Bretz S. L., (2015), Development of an Assessment Tool to Measure Students' Meaningful Learning in the Undergraduate Chemistry Laboratory, J. Chem. Educ., 92(7), 1149–1158. ©2015 American Chemical Society. | |
Upon noticing the large variation in affective changes from pre to post, the relationship between students' cognitive and affective responses were examined. Scatterplots of affective vs. cognitive averages were plotted for pre and post-test. As shown in Fig. 2, the sample appears to “shift left” as the cognitive averages decreased overall while the affective averages remained dispersed over the entire range of scores.
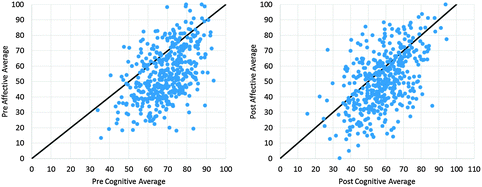 |
| Fig. 2 Scatterplots of affective vs. cognitive averages for pre and post-tests. Reprinted with permission from Galloway, K. R. and Bretz, S. L., (2015), Development of an Assessment Tool to Measure Students' Meaningful Learning in the Undergraduate Chemistry Laboratory, J. Chem. Educ., 92(7), 1149–1158. ©2015 American Chemical Society. | |
To explore how an individual student's cognitive and affective responses change together, students with extreme responses were selected to create vector plots. In these plots (Fig. 3), a small arrow, or vector, was drawn to connect a student's pre affective vs. cognitive score to their post score. While the vectors are not exact in terms of magnitude, they do show the direction and general extent to which a student changes from pre to post in a qualitative sense. As shown in Fig. 3, the students who had the highest cognitive responses for the post-test also had rather high cognitive responses for the pre-test. Interestingly, though, these students' affective responses were still quite diverse for the pre and post-test. By contrast, the students with the lowest cognitive scores at the end of the semester appear to decrease in their cognitive responses from pre to post. As with the high cognitive scorers, their affective responses were diverse. Some students' responses appear unchanged for affective, yet others appear to decrease. For the highest affective scoring students on the post test, these students' affective responses appear to increase from pre to post while their cognitive responses seem to either remain unchanged or decrease. The lowest affective scoring students on the post-test had an opposite direction and magnitude. These students' affective responses appear to either decrease or remain about the same from pre to post, but their cognitive responses all decrease.
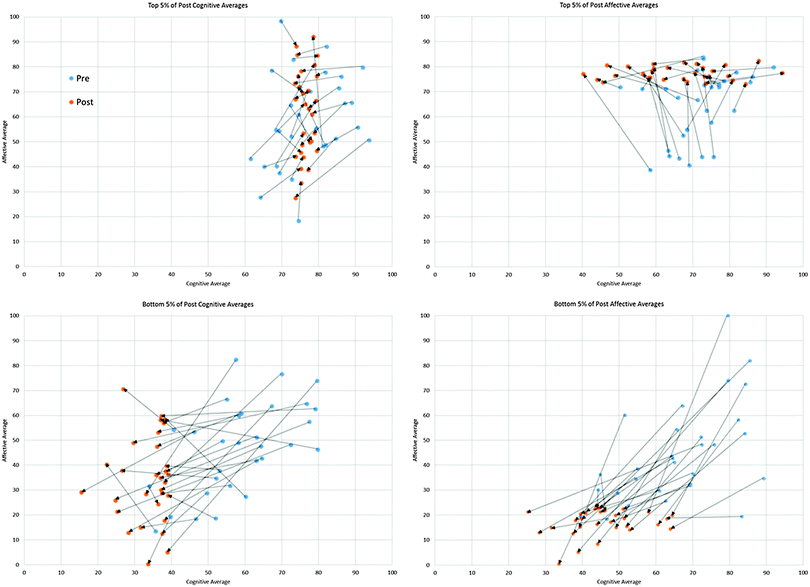 |
| Fig. 3 Vector plots for the highest and lowest scoring 5% for cognitive and affective averages on the MLLI post-test for first year chemistry students. | |
These plots suggest that students' cognitive and affective perceptions about learning were changing in different ways. From the beginning to the end of the semester, while the students are enrolled in the first-year chemistry laboratory course, their experiences as learners differ from their expectations. Because only students with extreme responses were used to generate these vector plots, the question remained as to how the rest of the sample changed from pre to post. Were there similar patterns? How might examining changes in response to cognitive, affective, and cognitive/affective MLLI items inform our understanding of students' ideas about learning in the first-year chemistry laboratory?
Cluster solution
In order to more closely examine the changes in students' responses that were clearly evident in the vector plots, a hierarchical agglomerative cluster analysis was conducted using Squared Euclidian distance with Ward's linkage method. There were 6 clustering variables – the mean responses for cognitive, affective, and cognitive/affective variables for both the pre-test and the post-test. This method of cluster analysis starts by considering each object to be clustered (in this case, each of the 436 students) as its own individual cluster. Objects (students) are then combined based on the dissimilarity measure and linkage method. Ward's method combines objects and groups by minimizing their sum of squared Euclidean distances between objects (Ward, 1963; Kaufman and Rousseeuw, 1990).
Clustering with these methods yielded a solution that suggested either four or two clusters as determined by examining the dendogram generated from the SPSS output and the agglomeration table. The dendogram displayed each fusion of clusters made during the agglomeration. To decide on the number of clusters, a “best cut” was made on the dendogram where clusters below that cut were distant from each other by the least amount (Everitt et al., 2011). Thus, the cut was made at the first substantial distance between the clusters. The agglomeration schedule lists the distance (or sum of squared distances in the case of Ward's method) at which each fusion was made (Burns and Burns, 2009; Mooi and Marko, 2011; Norusis, 2011). By locating the point at which the change in distances (or coefficients in this case) changes, the number of clusters can be determined. A number of different criteria have been developed to decide what should the optimal cluster solution (Milligan and Cooper, 1985). Using Caliński and Harabasz's procedures (1974), a variance ratio criterion (VRC) was calculated for 2, 3, 4, and 5 cluster solutions (Mooi and Marko, 2011). An optimal number of clusters is suggested when the VRC is maximized, and in this case, the VRC was maximized for both the two and four cluster solutions (Caliński and Harabasz, 1974; Milligan and Cooper, 1985; Mooi and Marko, 2011). The combination of these analyses suggested a four or two cluster solution. Choosing between whether to use the two-cluster or four-cluster solution could not be done empirically. Rather, choosing between two possible cluster solutions required interpreting the responses of students when in two clusters vs. when in four clusters in order to decide which cluster solution led to a more meaningful understanding of students' learning experiences in the chemistry laboratory. Clusters were identified using whole number integers until given meaningful names.
Cluster descriptions.
The four cluster solution was initially explored by looking at not only the summary statistics, but also the scatterplots of pre vs. post for each cluster. Table 3 displays the summary statistics grouped by MLLI scale instead of by pre and post-test (as in Table 2) in order to more easily visualize how the means for each cluster changed over time.
Table 3 Descriptive statistics for the four cluster solution
|
Scale mean (Stdev) |
Cognitive |
Affective |
Cognitive/affective |
Pre |
Post |
Pre |
Post |
Pre |
Post |
Cluster 1 N = 89 |
61.4 (10.6) |
46.4 (10.9) |
37.2 (11.0) |
28.7 (9.1) |
43.4 (10.9) |
28.4 (9.5) |
Cluster 2 N = 184 |
68.7 (9.6) |
61.3 (9.8) |
48.4 (8.9) |
52.3 (10.7) |
49.7 (9.6) |
46.1 (9.6) |
Cluster 3 N = 64 |
76.2 (7.7) |
71.6 (11.1) |
72.0 (7.7) |
77.9 (8.1) |
66.3 (13.1) |
63.4 (10.1) |
Cluster 4 N = 99 |
71.6 (10.9) |
53.2 (9.1) |
70.8 (10.3) |
48.2 (13.8) |
68.2 (12.1) |
45.2 (12.4) |
To visualize how each cluster changed over time for each of the three scales, boxplots were constructed (Fig. 4). These plots allowed for side by side visual comparison of each cluster's distribution of responses on one variable at two points in time (pre vs. post). The cognitive boxplot shows that all four clusters appear to decrease in score from pre to post, but some clusters decrease to a greater extent than others. For the affective plot, the clusters appear to behave differently. The medians for Cluster 2 and 3 appear to increase slightly, while Cluster 1 and 4 appear to have large decreases. The cognitive/affective plot also shows discriminant behaviour for each cluster. Examining Table 2 and Fig. 4 together shows three clusters that are sequential in their scale averages. Cluster 3 is consistently higher than Cluster 2 which is consistently higher than Cluster 1. Cluster 4, however, appears to behave differently with a larger change from pre to post. Visually exploring the data prior to applying statistics allowed for a more thorough understanding and interpretation of the data.
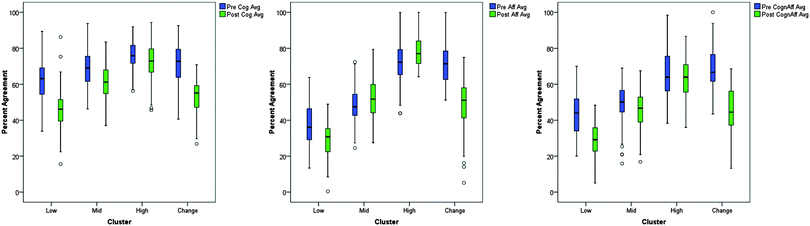 |
| Fig. 4 Boxplots of composite scale averages from pre to post by cluster. | |
By examining the summary statistics and plots for the two cluster solution, clusters 2, 3, & 4 would be combined, resulting in only a lower responding group and higher responding group. With the four cluster solution, however, smaller, more homogeneous groups could be described and analysed. These smaller groups, i.e., the four cluster solution, offered greater insight into students' perceptions of learning in the laboratory than the two cluster solution. Therefore, the subsequent analyses focused on the four cluster solution.
The initial scatterplots from Fig. 1 were remade with color coding for each of the four clusters. These plots are shown in Fig. 5. Like Table 2 and Fig. 4, Fig. 5 shows the responses of clusters 1, 2, and 3 are sequential from low to high in their responses; cluster 4 overlaps the other clusters. The differences in cluster separation should be interpreted while remembering the choice to not standardize the clustering variables. It is not surprising that the affective average scatterplot demonstrates both the separation and cohesion that are expected for clear, distinct clusters because the affective averages had the larger variations in the students' responses. The cognitive/affective plot depicts less separation than the affective plot, but more than the cognitive plot. The greater variation in the affective domain could indicate that students have a greater intrinsic importance of the affective domain than the cognitive domain to laboratory learning.
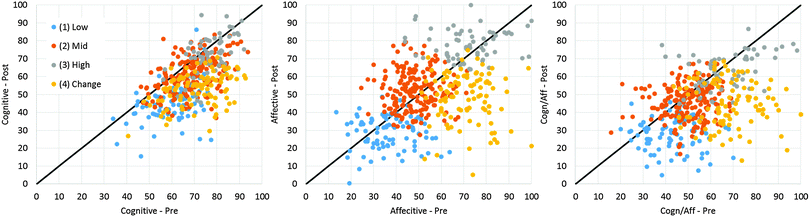 |
| Fig. 5 Scatterplots of composite scale average pre vs. post, color-coded by cluster. | |
These analyses led to re-naming the clusters to represent their characteristics: Low (cluster 1), Mid (cluster 2), High (cluster 3), and Change (cluster 4). This cluster naming is introduced in Fig. 5 and used throughout the rest of this paper.
Cluster distinctness
The interpretability of the cluster solution is supported by how distinct the clusters are from each other as well as the pedagogical usefulness of these distinctions. A well supported cluster solution has clusters that are well separated from other clusters (distinct) and sufficiently similar within a cluster (cohesive) (Kaufman and Rousseeuw, 1990). The distinctiveness of each cluster was measured both quantitatively using inferential statistics and qualitatively by examining how the clusters responded to specific items on the MLLI.
Inferential statistics.
A one-way repeated measures ANOVA was conducted for each cluster to analyze how each cluster's responses changed from pre to post. The results from these tests can be found in Table 4. Both the Low and Change clusters had significant decreases for all three scales with large effect sizes (Cohen, 1973; Levine and Hullett, 2002) indicating a lack of alignment between their expectations and experiences. The Mid cluster had a large significant decrease for cognitive, a small increase for affective, and a small decrease for cognitive/affective. While the High cluster also had a significant decrease for cognitive (with a medium effect), conversely, High had a significant increase for affective (with a medium effect), and no significant change for cognitive/affective. These results demonstrate the clusters' unique characteristics when their experiences were compared to their expectations for cognitive and affective learning.
Table 4 Results from repeated measures ANOVA for each cluster separately
Cluster |
RM ANOVA results comparing pre to post on 3 scales |
Pairwise comparisons (p, ηp2) |
Cognitive |
Affective |
Cognitive/affective |
Low N = 89 |
Wilks's Λ = 0.303, F(3,86) = 66.08, p ≤ 0.0001, ηp2 = 0.70 |
<0.0001, 0.65 |
<0.0001, 0.26 |
<0.0001, 0.53 |
Mid N = 184 |
Wilks's Λ = 0.551, F(3,181) = 49.13, p ≤ 0.0001, ηp2 = 0.45 |
<0.0001, 0.35 |
<0.001, 0.07 |
<0.0001, 0.07 |
High N = 64 |
Wilks's Λ = 0.601, F(3,61) = 13.51, p ≤ 0.0001, ηp2 = 0.40 |
<0.0001, 0.21 |
0.002, 0.14 |
0.077, 0.05 |
Change N = 99 |
Wilks's Λ = 0.303, F(3,86) = 66.08, p ≤ 0.0001, ηp2 = 0.70 |
<0.0001, 0.65 |
<0.0001, 0.26 |
<0.0001, 0.53 |
Cluster profiles
In order to further understand each cluster's ideas about learning chemistry in the laboratory, the MLLI item responses for each cluster were explored. Both boxplots and scatterplots were constructed for each item to explore how the clusters responded. This analysis revealed individual items that demonstrated clear distinctions between clusters, as well as items with similar responses across all clusters. Examples of these items will be discussed in the following sections.
Discriminant items.
Certain items, and combinations of items, gave insight to the clusters' ideas about learning that aren't easily extracted from the boxplots or scatterplots of the composite variables. For example, one item that generated distinctive responses among the clusters is MLLI item 29. On the pre-test, item 29 says “to be confused about what my data mean” and changed to “was confused about what my data meant” for the post-test. This is a cognitive item where a high percent agreement indicates a hindrance to meaningful learning. Fig. 6 shows the scatterplot and boxplot for MLLI item 29. The scatterplot shows the Low responses clustered at the top right with high percent agreement for both the pre and post-tests. By contrast, the High responses are clustered at the bottom left with low percent agreement for both the pre- and post-tests. The students in Change tend to be more towards the top left with high percent agreement for the post-test, despite their pre-test response. Mid is scattered in the middle of the plot, not suggesting any particular consensus. The boxplot displays the same information, but in a different format. The spread of Mid's responses is seen more clearly in the boxplot, along with how High converges towards disagreement for the post-test.
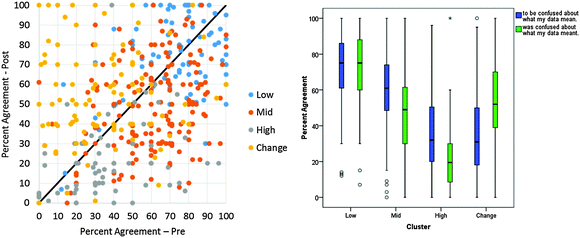 |
| Fig. 6 Scatterplot and boxplot of MLLI item 29. Scatterplot shows post vs. pre. Boxplot shows. | |
These plots offered deeper insight into the experiences of the students in the different clusters. Low expected to be confused about what their data meant, and this expectation was fulfilled by their experiences. On the other hand, High, for the most part, did not expect to be confused about what their data meant and their post-test responses demonstrate that, overall, they were in fact not confused about what their data meant. Change also did not expect to be confused, yet their responses on the post-test show that this expectation was not met. One explanation could be that Low was more aware of their cognitive limitations and that Change was unable to gauge how their learning would take place. Alternatively, it could be that the pedagogy did not support Change in making connections between that they already knew and what they needed to learn, i.e., the instruction that Change received was unable to help them meet their learning expectations. (Characterizing the pedagogy and curriculum in relation to MLLI scores was beyond the scope of this particular study, but is currently being investigated in a larger study with multiple institutions.)
Comparing responses on groups of MLLI items also yielded additional insight. For example, item 4 asks students about feeling unsure about the purpose of procedures, while items 14 and 20 both ask students if they worry (ied) about getting good data and the quality of the data, respectively. All three of these items are cognitive/affective items and would hinder meaningful learning with a high score. (The similarity of these two items acts as an additional reliability check to see if students were responding consistently on the MLLI.) The boxplots for these items are shown in Fig. 7. For item 4, each cluster uniquely responded to the item. Low showed convergence on the post-test. At the time of the pre-test, the cluster was not sure whether to expect to be unsure about the purpose of the procedures, but they responded that they did feel unsure on the post-test. High indicated that they did not expect to feel unsure and that that was their experience as well. Change also expected to not feel unsure, but their expectation was not met; their responses show great diversity in how they understood the purpose of the procedures. Overall, Low, Mid, and Change all indicated they felt unsure about the purpose of the procedures during their chemistry laboratory course. Only High responded feeling somewhat sure about the purpose of the procedures.
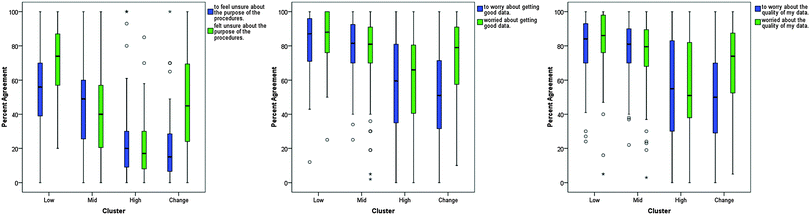 |
| Fig. 7 Boxplots of MLLI items 4, 14, and 20. | |
Items 14 and 20 told a slightly different story. Consistent with their previous descriptions, Low indicated that they expected to be worried about their data and that expectation was met. Like Low, Mid also indicated they expected to, and did experience, worrying about their data. Change had diverse expectations as to whether they would worry about their data, but moved toward more agreement that they indeed did worry about their data for the post-test. Unlike their previous responses, High shows diverse responses for the pre and post-tests. While High reported that they did not feel unsure about the procedures, their confidence in understanding the procedure did not preclude worrying about the outcome of the procedure. Many of the students in High remained concerned with getting good quality data, despite indicating little feeling of being unsure about the purpose of procedures.
Similar response patterns.
The combination of other items showed a similar response pattern across all clusters for both the pre and post-tests. Like the pattern seen with the composite variables, Low, Mid, and High have sequential responses and Change initially responds similar to High but ends closer to Mid and Low. Three cognitive items that typify this response pattern are MLLI items 11 (think about what the molecules are doing), 22 (interpret my data beyond only doing calculations), and 25 (use my observations to understand the behaviour of atoms and molecules). These items, whose boxplots are shown in Fig. 8, were written so that a higher response indicated a contribution to meaningful learning. A common idea among these items is that they ask for higher order thinking and take the “doing” of laboratory work to a more abstract level when thinking about the procedures and data collection. Each of these items showed a similar response pattern across the clusters for both the pre-test and post-test. Each cluster decreased from pre to post. While each cluster did not decrease the same amount on all three items, the fact that there was an overall decrease across each cluster is noteworthy. The students indicated that they expected to think about what they were doing in lab, only to have these expectations go unfulfilled.
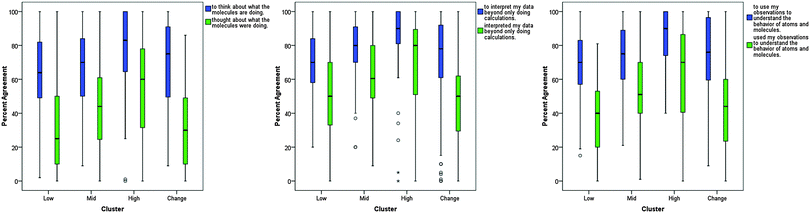 |
| Fig. 8 Boxplots of MLLI items 11, 22, and 25. | |
Non-discriminant items.
While most items indicated some distinctions between two or more clusters, there were a few items that yielded little to no discriminant information. One such item was item 27 (to be intrigued by the instruments). This item is a cognitive/affective item, and a higher response indicates a contribution toward meaningful learning. As shown in Fig. 9, the whiskers for each boxplot overlapped across each cluster and spread out over almost the entire range of possible responses. The medians did vary for each cluster, but with such a large spread, this item offered no new information about how the clusters differed. In previously reported interviews with students about this item (Galloway and Bretz, 2015), students used both cognitive and affective words to describe this item. When asked whether they felt “intrigued by the instruments” during laboratory, some students indicated they would be more likely to say ‘curious’ as opposed to ‘intrigued’. This item was intentionally written using the word ‘intrigued’ rather than ‘curious’ due to previous reports of faculty goals for the undergraduate laboratory (Bruck et al., 2010; Bretz et al., 2013). The results from this item suggest that experience with laboratory equipment and instruments did not differentiate students' ideas about learning in the laboratory that were seen in the clusters. While one may question the utility of item 27 if it didn't appear to discriminate among the clusters, the information from this item is still valuable to researchers and instructors. If students across all the clusters have similar expectations and experiences, then any modifications to improve the experience would benefit students across the each cluster.
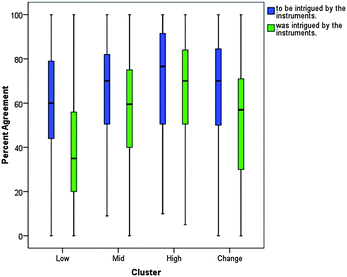 |
| Fig. 9 Boxplot of MLLI item 27. | |
Summary of cluster descriptions
Cluster 1: Low.
This cluster had 89 students with the lowest composite averages of the 4 clusters and significant decreases from pre to post with large effects for cognitive, affective, and cognitive/affective scales. As whole, Low tended to have negative affective expectations for their laboratory experiences. Consequently, these negative expectations were met and for some, the experiences were more negative than expected. In a study of students in the Netherlands on agreement between students' expectations, experiences, and the actual objectives of the practicals, Kirschner et al. (1993) stated that “experiences do not give rise to expectation, if anything expectations determine experience” (p. 178). Konings et al. (2008) had similar findings in their study of students' expectations and perceptions of learning in new environments. They concluded that students “selectively pay attention to information consistent with their expectations and also interpret this information in such a way that their expectations are confirmed” (p. 546). Because these students expected to not have a positive experience, they only paid attention to the negative experiences, and thus, their experiences were even worse than expected. For Low's cognitive expectations, there was a greater diversity than in their indicated experiences. One possible explanation is that these students weren't sure what kind of cognitive experiences to expect; therefore, they weren't able to anticipate what to look for and pay attention to. Kirschner et al. (1993) stated that “if a student does not expect to encounter certain things in a piece of instruction, but comes across them anyways, the student will try to avoid this discrepancy (and any possible unpleasant feeling) by resisting the notion that those things were actually present in the instruction” (p. 179). The absence of what to anticipate in terms of cognitive learning experiences could also be influencing Low's affect towards their learning.
Cluster 2: Mid.
This cluster had 184 students and was the largest cluster (two to three times larger than the other clusters). On composite scores, this cluster tended to hover in the middle. Mid had a significant decrease for cognitive with a large effect and a significant decrease for cognitive/affective with a small effect. There was significant increase for affective with a small effect which can be supported by the scatterplot showing a greater number of students above the y = x line (Fig. 5). Perhaps this increase may be due to Mid being indecisive or indifferent about their experiences in chemistry laboratory as Mid's responses were often just more spread out than Low's. The fact that the Mid cluster tends to have quite diverse responses in between Low and High could possibly indicate Mid as a “catch-all” group for the students without extreme values in either the positive or negative direction or those without a large decrease from pre to post like the Change cluster. Since Mid constitutes almost half of the sample, it is still useful to know how the bulk of the sample perceived their learning. Similar to Low, the fact that Mid remained in the middle of the subscale for both the pre-test and post-test continues to support the findings from Kirschner et al. (1993) and Konings et al. (2008) that students only encounter the things that they anticipate to happen. Conclusions about Mid's perceptions of learning in the laboratory can only be speculated upon and could form the basis of a future research study.
Cluster 3: High.
This cluster was the smallest cluster with only 64 students. This cluster also exhibited some of the most extreme behaviour, mostly in the positive direction. High had a significant decrease for cognitive with a medium effect size, a significant increase for affective with a medium effect, and no significant change for cognitive/affective. The composite scatter plots were interesting for High because there were more students to the left of the y = x line, demonstrating the significant increase for the affective items (Fig. 5). High can be characterized by their exceeded expectation for developing confidence in laboratory (Q13 and Q30) and for not being nervous handling chemicals (Q18), but they showed little consensus on worrying about their data (Q14 and Q20) and making mistakes (Q9). Furthermore, High had cognitive expectations that were fulfilled including experiencing moments of insight (Q5), considering if data makes sense (Q10), and thinking about the chemistry they already know (Q19). High also exhibited little consensus on the cognitive/affective items which is illustrative of how students can have isolated positive affective and cognitive experiences, but unless there is integration and overlap in these areas, meaningful learning can be hindered (Novak, 2010). The students in this cluster could have had some robust prior experience before enrolling in university chemistry as demonstrated by their lack of confusion about how instruments work (Q6) or about the underlying concepts (Q16); they thought the procedures were simpler than expected (Q15); and they thought about chemistry they already knew (Q19). Yet, interestingly, High did not indicate experiencing making decisions about what data to collect (Q3), learning critical thinking and problem solving skills (Q7 and Q31), thinking about the chemistry on a particulate level (Q11 and Q25), and interpreting their data beyond calculations (Q22). A robust knowledge of procedural skills for High did not necessarily lead to the cognitive processing essential for conceptual understanding.
Cluster 4: Change.
This cluster had 99 students and was second largest. Change began the semester not different from High on any subscale and ended the semester not different from Mid. Change had significant decreases with large effect sizes on all three scales. The majority (>90%) of students were below the y = x line of the scatterplots (Fig. 5). For each subscale, Change had high expectations that were not met by the end of the semester. For the affective items, Change indicated their expectations for being excited to do chemistry (Q8) and for not being nervous about making mistakes (Q9) or not being frustrated (Q21). None of these expectations were met. Despite these clear shifts as a whole, Change did not have a consensus on being nervous about finishing on time (Q2). For the cognitive subscale, Change expected to experience moments of insight (Q5), learn critical thinking and problem solving skills (Q7 and Q31), think about the chemistry on a particulate level (Q11 and Q25), and interpret the data beyond calculations (Q22), but these expectations were not met. The students in Change seemed to struggle on many levels. They had high expectations for what they would do to learn in their chemistry laboratory course, but their experiences didn't live up to their expectations. Additional research studies are needed to explore why these students were not able to meet their expectations.
Conclusions
This study used cluster analysis to explore data from a novel assessment tool that measured students' ideas about learning in the undergraduate chemistry laboratory, specifically by comparing the students' expectations for learning with their experiences in a first year university chemistry laboratory course. The results from this cluster analysis revealed ideas about how students perceive learning in the undergraduate chemistry laboratory that can inform the design of laboratory curricula. The combination of cognitive, affective, and cognitive/affective composite variables for the pre and post-test as clustering variables yielded a clustering solution with four distinct groups of students. Three of the four groups demonstrated sequential characteristics where the first group scored lowest on all 6 variables, the third group scored the highest, and the second group was in the middle. Thus, for three-fourths of the sample, the students' learning experiences in their laboratory course were framed by their expectations. What students expected to think and feel in their laboratory course framed what they actually experienced. The results from these three groups support previous studies that found that students' expectations determine their experiences (Kirschner et al., 1993; Roese and Sherman, 2007; Könings et al., 2008). The fourth cluster of students demonstrated considerable change from pre-test expectations to post-test experiences; these students reported expectations similar to students in the High group, but reported experiences similar to students in the Mid or even Low group. The fourth group of students suggested a misalignment of some students' expectations for laboratory learning with what they actually experienced.
Limitations
Cluster analysis is an inherently exploratory technique. The analysis is executed based upon the methodological decisions made by the researchers regarding the objects to cluster, the clustering variables, and the clustering algorithm (including the distance and linkage method). The results of this particular cluster analysis depend on the decision to cluster only first-year chemistry students. A different solution might be expected if data collected from organic chemistry students had been included in the analysis. The specific combination of clustering variables also led to this particular solution, and clustering on only expectations or only on experiences would probably also lead to different solutions. The intention of cluster analysis is not to identify the one and only perfect solution, but rather to identify naturally occurring groups of students based on certain variables for which meaningful interpretations can be proposed and supported. Thus with this cluster analysis, four meaningful groups emerged that lead to better understanding of how students perceived their learning in a first-year university chemistry laboratory course.
This cluster analysis was conducted with student responses from one midsize midwestern liberal arts university. The results show the profile for the students who voluntarily responded to the survey during one semester of first-year university chemistry laboratory. This analysis is currently being applied to a second, larger, national data collection from students with a variety of laboratory experiences (differing pedagogies and curricula). While the results from the cluster analysis reported herein are not generalizable to all first-year university chemistry laboratory courses, they do describe the experiences of one set of students. The information gained from understanding these students' ideas about learning in their laboratory course may be applicable to other first-year university chemistry laboratory courses.
Implications for research
Following recent cluster analysis reports in chemistry education research (Brandriet and Bretz, 2014; Chan and Bauer, 2014; Linenberger and Holme, 2014; Raker and Holme, 2014), this article reports a model for making decisions regarding data analysis. The choice of variables for cluster analysis is one of the most important decisions for the researcher and should be thoughtfully considered. In this research, our choices were guided by learning theory. The cluster analysis of the MLLI data examined students' pre and post scores as a means to classify them into groups. In this way, students were uniquely analysed as to how their responses on MLLI items changed from pre to post, or more precisely, how their expectations for learning compared with their actual experiences in the first-year undergraduate chemistry laboratory course. The cluster solution was analysed using a variety of graphs both of composite variables and at the item level. These visualizations allow for a more complete picture of the cluster characteristics as well as for making appropriate claims about each cluster.
By analysing MLLI results through the lens of the Meaningful Learning framework, interpretations can be made about how students are learning chemistry that can directly transfer to evidence-based decisions for laboratory curriculum design. Thoughtful analysis of these findings generates a thought provoking question – what is it about these students' experiences in laboratory that creates a disconnect between what they think they “do” in the laboratory and how they “think” and “feel” about their results? Additional research studies are needed to explore the role of the affective domain in learning chemistry, particularly in the laboratory setting. Also further research is needed to explore the gap in the Change cluster's expectations and experiences. The question remains as to whether the students are miscalibrated about laboratory learning or whether the curriculum or pedagogy is unable to effectively support those students in learning.
Implications for teaching
If students' behaviours are governed by their expectations, then the results from this study reinforce the importance for laboratory instructors to make clear their expectations for their students at the start of the course (Hofstein and Lunetta, 2004; Reid and Shah, 2007). Even so, the results described above regarding the Change cluster indicate that anticipating certain experiences is not enough to ensure they will occur and contribute to meaningful learning. There needs to be guidance and scaffolding for how to accomplish tasks in order to help students realize on their own how to accomplish and meet their expectations for their learning. As Strike & Posner have written, “Seeing is something we do with ideas as well as sense. We cannot see what we cannot conceive. Moreover, people who approach the world with different conceptions will see it differently.” (Strike and Posner, 1985, p. 215). When students are made aware of what learning experiences they should expect in their laboratory courses, then instructors might have reason to begin to expect the desired effects of innovative and reformed curriculum to appear.
Many students' experiences in their chemistry laboratory course did not offer them the expected opportunities to think more deeply about their laboratory work. While the argument could be made that the students did not know how to think about their lab work even if they had been given the opportunity, Ausubel was explicit in his educational theory that students need to have relevant prior knowledge and consciously choose to incorporate meaningful material. Teachers need to offer students opportunities to learn that are relatable to the prior knowledge that students have (Ausubel, 1968). The responsibility lies with both the student and the teacher. Thus, if students' responses indicate that they are not thinking about their lab work, then change needs to be made both with students and instructors.
While the cognitive domain is more obvious to both students and instructors, the affective domain is rarely attended to by the laboratory instructors (Bretz et al., 2013). The neglect of the affective domain creates a hindrance for meaningful learning to occur in the laboratory. When designing curricula for the laboratory, chemists tend to focus predominately on what the students will do, with little attention to how students will engage affectively and mentally process their actions (Sevian and Fulmer, 2012; Bretz et al., 2013). The extent to which the affective domain is attended to in laboratory curricula design lies only in attention to group work/collaboration and in making connections to the real world (Bretz et al., 2013). In order to facilitate meaningful learning, these cannot be the only affective characteristics attended to. The clusters' affective profiles point to the need for attention to the affective dimension in the undergraduate chemistry laboratory. Learning is not purely a cognitive exercise. Learning requires integration of the doing (psychomotor) and the feeling (affective) with the thinking (cognitive) in order for meaningful learning to occur (Novak, 1993, 2010; Bretz, 2001). While laboratory instructors often voice their goals for laboratory learning to focus upon mastery of technique, the data presented here emphasize the importance of students understanding the mechanism, purpose, and applicability of the techniques and thereby lead to more integrated, meaningful learning experiences.
Acknowledgements
This work was supported by and the Volwiler Family Endowment to the Miami University Department of Chemistry & Biochemistry and grant No. from the National Science Foundation Any opinions, findings, and conclusions or recommendations expressed in this material are those of the authors and do not necessarily reflect the views of the National Science Foundation. The authors thank Ellen Yezierski for her invaluable feedback on the research and statistics.
References
- Aldenderfer M. S. and Blashfield R. K., (1984), Cluster analysis, Beverly Hills, CA: Sage Publications.
- Ausubel D. P., (1968), Educational psychology: a cognitive view, New York, NY: Holt, Rinehart, and Winston Inc.
- Brandriet A. R. and Bretz S. L., (2014), Measuring meta-ignorance through the lens of confidence: Examining students' redox misconceptions about oxidation numbers, charge, and electron transfer, Chem. Educ. Res. Pract., 15, 729–746.
- Brandriet A. R., Ward R. M. and Bretz S. L., (2013), Modeling meaningful learning in chemistry using structural equation modeling, Chem. Educ. Res. Pract., 14, 421–430.
- Bretz S. L., (2001), Novak's theory of education: human constructivism and meaningful learning, J. Chem. Educ., 78(8), 1107.
- Bretz S. L., Fay M., Bruck L. B. and Towns M. H., (2013), What faculty interviews reveal about meaningful learning in the undergraduate chemistry laboratory, J. Chem. Educ., 90(3), 281–288.
- Bruck A. D. and Towns M. H., (2013), Development, implementation, and analysis of a national survey of faculty goals for undergraduate chemistry laboratory, J. Chem. Educ., 90(6), 685–693.
- Bruck L. B., Bretz S. L. and Towns M. H., (2008), Characterizing the level of inquiry in the undergraduate chemistry laboratory, J. Coll. Sci. Teach., 87(12), 1416–1424.
- Bruck L. B., Bretz S. L. and Towns M. H., (2010), Faculty perspectives of undergraduate chemistry laboratory: goals and obstacles to success, J. Chem. Educ., 37(7), 52–58.
- Burns R. P. and Burns R., (2009), Cluster analysis, Business research methods and statistics using SPSS, London: Sage Publication, pp. 552–567.
- Caliński T. and Harabasz J., (1974), A dendrite method for cluster analysis, Commun. Statist., 3(1), 1–27.
- Chan J. Y. K. and Bauer C. F., (2014), Identifying at-risk students in general chemistry via cluster analysis of affective characteristics, J. Chem. Educ., 91(9), 1417–1425.
- Cohen J., (1973), Eta-squared and partial eta-squared in fixed factor ANOVA designs, Educ. Psychol. Meas., 33, 107–112.
- Cohen B., (2008), Multiple regression, Explaining psychological statistics, 3rd edn, New York, NY: Wiley, pp. 5298–5299.
- Cooper M. M. and Kerns T. S., (2006), Changing the laboratory: effects of a laboratory on students' attitudes and perceptions, J. Chem. Educ., 83(9), 1356–1361.
- Drechsler M. and van Driel J., (2009), Teacher's perceptions of the teaching of acids and bases in Swedish upper secondary schools, Chem. Educ. Res. Pract., 10, 96–96.
- Elliot M. J., Stewart K. K. and Lagowski J. J., (2008), The role of the laboratory in chemistry instruction, J. Chem. Educ., 85(1), 145–149.
- Emenike M. E., Daneilson N. D. and Bretz S. L., (2011), Meaningful learning in a first-year chemistry laboratory course: differences across classical, discovery, and instrumental experiments, J. Coll. Sci. Teach., 41, 84–92.
- Everitt B. S., Landau S., Leese M. and Stahl D., (2011), Cluster analysis, 5th edn, Chichester, UK: Wiley.
- Galloway K. R. and Bretz S. L., (2015), Development of an assessment tool to measure students' meaningful learning in the undergraduate chemistry laboratory, J. Chem. Educ., 92(7), 1149–1158.
- Grove N. and Bretz S. L., (2007), CHEMX: An instrument to assess student's cognitive expectations for learning chemistry, J. Chem. Educ., 84(9), 1524–1529.
- Hawkes S. J., (2004), Chemistry is not a laboratory science, J. Chem. Educ., 81(9), 1257.
- Herrington D. G. and Nakhleh M. B., (2003), What defines effective chemistry laboratory instruction? Teaching assistant and student perspectives, J. Chem. Educ., 80(10), 1197–1205.
- Hilosky A., Sutman F. and Schmuckler J., (1998), Is laboratory based instruction in beginning college-level chemistry worth the effort and expense? J. Chem. Educ., 75, 100–104.
- Hofstein A. and Lunetta V. N., (1982), The role of the laboratory in science teaching: neglected aspects of research, Rev. Educ. Res., 52, 201–217.
- Hofstein A. and Lunetta V. N., (2004), The laboratory in science education: foundations for the twenty-first century, Sci. Educ., 88, 28–54.
- Kaufman L. and Rousseeuw P. J., (1990), Finding groups in data: an introduction to cluster analysis, New York, NY: Wiley.
- Kirschner P., Meester M., Middelbeek E. and Hermans H., (1993), Agreement between student expectations, experiences, and actual objectives of practicals in the natural sciences at the Open University of The Netherlands, Int. J. Sci. Educ., 15(2), 175–197.
- Könings K. D., Brand-Gruwel S., van Merriënboer J. J. G. and Broers N. J., (2008), Does a new learning environment come up to students' expectations? A longitudinal study, J. Educ. Psychol., 100(3), 535–548.
- Levine T. R. and Hullett C. R., (2002), Eta squared, partial eta squared, and misreporting of effect size in communication research, Human Comm. Res., 28, 612–625.
- Lewis S. E., Shaw J. L., Heitz J. O. and Webster G. H., (2009), Attitude counts: self-concept and success in general chemistry, J. Chem. Educ., 86(6), 744–749.
- Linenberger K. J. and Holme T. A., (2014), Results of a national survey of biochemistry instructors to determine the prevalence and types of representations used during instruction and assessment, J. Chem. Educ., 91(6), 800–806.
- Milligan G. W., (1996), Clustering validation: results and implications for applied analyses, in Arabie P. and Desoete G. (ed.), Clustering and classification, Singapore: World Scientific.
- Milligan G. W. and Cooper M. C., (1985), An examination of procedures for determining the number of clusters in a data set, Psychometrika, 50(2), 159–179.
- Mooi E. and Marko S., (2011), Cluster analysis, A concise guide to market research, Berlin: Springer, pp. 237–284.
- Nakhleh M. B., Polles J. and Malina E., (2002), Learning chemistry in a laboratory environment, in Gilbert J. K., de Jong R., Justi D. F., Treagust J. H. and Van Driel, J. H. (ed.), Chemical education: towards research-based practice, Dordrecht, Netherlands: Kluwer, pp. 69–94.
- National Research Council, (2012), Discipline-based education research: understanding and improving learning in undergraduate science and engineering, in Singer S. R., Nielsen N. R. and Schweingruber H. A. (ed.), Committee on the Status, Contributions, and Future Direction of Discipline-Based Education Research, Board on Science Education, Division of Behavioral and Social Sciences and Education, Washington, DC: The National Academies Press.
- Neidenthal P. M., (2007), Embodying emotion, Science, 316, 1002–1005.
- Norusis M. J., (2011), Cluster analysis, in Statistical procedures companion, Upper Saddle River, NJ: Prentice Hall, pp. 375–404.
- Novak J. D., (1993), Human constructivism: a unification of psychological and
epistemological phenomena in meaning making, Inter. J. Pers. Const. Psych., 6, 167–193.
- Novak J. D., (2010), Learning, creating, and using Knowledge, New York, NY: Taylor & Francis Group.
- Raker J. R. and Holme T. A., (2014), Investigating faculty familiarity with assessment terminology by applying cluster analysis to interpret survey data, J. Chem. Educ., 91(8), 1145–1151.
- Reddish E. F., Saul J. M. and Steinberg R. M., (1998), Student expectations in introductory physics, Am. J. Phys., 66(3), 212–224.
- Reid N. and Shah I., (2007), The role of laboratory work in university chemistry, Chem. Educ. Res. Pract., 8, 172–185.
- Roese N. L. and Sherman J. W., (2007), Expectancies, in A. W. Kruglanski and E. T. Higgins (ed.), Social psychology: handbook of basic principles, 2nd edn, New York, NY: Guilford Press, pp. 91–115.
- Sandi-Urena S. and Gatlin T. A., (2013), Factors contributing to the development of graduate teaching assistant self-image, J. Chem. Educ., 90(10), 1303–1309.
- Sandi-Urena S., Cooper M. M. and Gatlin T. A., (2011a), Graduate teaching assistants' epistemological and metacognitive development, Chem. Educ. Res. Pract., 12, 92–100.
- Sandi-Urena S., Cooper M. M., Gatlin T. A. and Bhattacharyya G., (2011b), Students' experience in a general chemistry cooperative problem based laboratory, Chem. Educ. Res. Pract., 12, 434–442.
- Sandi-Urena S., Cooper M. M. and Stevens R., (2012), Effect of cooperative problem-based lab instruction on metacognition and problem solving skills, J. Chem. Educ., 89(6), 700–706.
- Sevian H. and Fulmer G. W., (2012), Student outcomes from innovations in undergraduate chemistry laboratory learning: a review of projects funded by the US National Science Foundation between 2000–2008, Educ. Quim., 23, 149–161.
- Strike K. A. and Posner G. K. (1985), A conceptual change view of learning and understanding, in West L. H. T. and Pines A. L. (ed.), Cognitive Structure and Conceptual Change, Orlando, FL: Academic Press.
- Ward J. H., (1963), Hierarchical grouping to optimize an objective function, J. Am. Stat. Assoc., 58, 236–244.
|
This journal is © The Royal Society of Chemistry 2015 |
Click here to see how this site uses Cookies. View our privacy policy here.