Enhancing undergraduate chemistry learning by helping students make connections among multiple graphical representations
Received
20th April 2015
, Accepted 11th June 2015
First published on 11th June 2015
Abstract
Multiple representations are ubiquitous in chemistry education. To benefit from multiple representations, students have to make connections between them. However, connection making is a difficult task for students. Prior research shows that supporting connection making enhances students' learning in math and science domains. Most prior research has focused on supporting one type of connection-making process: conceptually making sense of connections among representations. Yet, recent research suggests that a second type of connection-making process plays a role in students' learning: perceptual fluency in translating among representations. I hypothesized that combining support for both conceptual sense making of connections and for perceptual fluency in connection making leads to higher learning gains in general chemistry among undergraduate students. I tested this hypothesis in two experiments with altogether N = 158 undergraduate students using an intelligent tutoring system for chemistry atomic structure and bonding. Results suggest that the combination of conceptual sense-making support and perceptual fluency-building support for connection making is effective for students with low prior knowledge, whereas students with high prior knowledge benefit most from receiving perceptual fluency-building support alone. This finding suggests that students' learning in chemistry can be enhanced if instruction provides support for connection making among multiple representations in a way that tailors to their specific learning needs.
Introduction
In chemistry, students have to learn concepts that are inherently visuo-spatial. To make these concepts accessible to students, chemistry instruction tends to heavily rely on the use of graphical representations (Bodner and Domin, 2000; Taber, 2009; De Jong and Taber, 2014). Graphical representations are instructional materials that use visuo-spatial elements to depict domain-relevant concepts (as opposed to text or symbols). Furthermore, graphical representations are important tools that chemists use to think, to solve problems, and to communicate–in other words, graphical representations are an integral part of discourse within the chemistry discipline (Kozma et al., 2000; Schönborn and Anderson, 2006; Airey and Linder, 2009). Therefore, representational competences are key to students' learning in chemistry (Wu et al., 2001; Kozma and Russell, 2005a, 2005b; Justi et al., 2009; Linenberger and Bretz, 2012).
In particular, students' learning success depends on their ability to make connections among graphical representations. Fig. 1 shows examples of graphical representations typically used to depict atoms; Fig. 2 shows examples of representations used to illustrate bonding in molecules (Bowen, 1990; Kozma et al., 2000; Höst et al., 2012; Hinze et al., 2013). For example, a student who is learning about bonding may be asked to use the representations in Fig. 2 to predict how ethyne will react with other molecules. To do so, the student needs to connect the triple bond shown in the Lewis structure and the ball-and-stick figure to the red region in the electrostatic potential map (EPM), and infer that this molecule is unstable. Airey and Linder (2009) refer to this ability to make connections as “apresentation”–as the ability to “spontaneously infer the presence of further facets of a disciplinary way of knowing over and above those made available through the mode a student has been presented with” (p. 10). Hence, the ability to make connections among multiple graphical representations enables students to participate in discourse and practices that are common in scientific and professional practices that chemistry students aspire to join. Because graphical representations are the visual language through which instruction conveys chemistry concepts, students learn domain knowledge by making connections among graphical representations (Schönborn and Anderson, 2006; Airey and Linder, 2009).
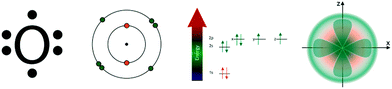 |
| Fig. 1 Representations of an oxygen atom: Lewis structure, Bohr model, energy diagram, orbital diagram. | |
 |
| Fig. 2 Representations of an ethyne molecule: Lewis structure, ball-and-stick figure, space-filling model, electrostatic potential map (EPM). | |
Yet, making such connections is a difficult and cognitively demanding task for students (Johnstone, 1991; Taber, 2013). Indeed, undergraduate students' frequent failure to make connections among multiple representations has been found to jeopardize their learning of important chemistry concepts (Dori and Barak, 2001; Talanquer, 2013). Even PhD students in chemistry lack crucial representational competences and focus on surface-level features of representations when asked to explain reactions (Strickland et al., 2010). Unfortunately, there is also evidence that instructors tend to overestimate students' ability to make connections among graphical representations (Schönborn and Anderson, 2006; Uttal and O’Doherty, 2008; Airey and Linder, 2009). Hence, supporting students in learning to make connections among multiple graphical representations is a major goal in chemistry education (Cheng and Gilbert, 2009; Talanquer, 2013). A number of studies in chemistry education indicate that students' learning can be enhanced by providing students with connection-making activities (Davidowitz and Chittleborough, 2009; Luxford et al., 2011; Linenberger and Bretz, 2012).
Most prior research on connection making has focused on supporting only one type of connection-making ability; namely, conceptual sense making of connections (Kozma and Russell, 2005a, 2005b; Stieff, 2005; Bodemer and Faust, 2006; van der Meij and de Jong, 2006; Michalchik et al., 2008). Conceptual sense-making support targets explicit connection-making processes by helping students reason about how different representations show the same concepts and how the information shown about the given concept differs between representations (e.g., in Fig. 1, relating the local negative charge that results from the triple bond shown in the Lewis structure to the region of high electron density shown in red in the EPM).
However, chemistry expertise also involves a second type of connection-making ability: perceptual fluency in translating between representations (Kozma and Russell, 1997; Kozma and Russell, 2005a, 2005b; Airey and Linder, 2009) (e.g., in Fig. 1, rapidly “seeing” that the four representations likely show the same molecule because they all have the same linear geometry). Recent research in educational psychology draws attention to novel ways to support students in acquiring perceptual fluency in translating between representations (Kellman and Massey, 2013). Research on perceptual fluency in connection making builds on research on expertise, which shows that experts can quickly and effortlessly make connections by “just seeing” connections between representations, without cognitive effort (Chi et al., 1981; Kellman and Garrigan, 2009; Gegenfurtner et al., 2011). For example, Airey and Linder (2009) argue that this type of fluency is characterized by connection making becoming “unproblematic, almost second nature” (p. 10). This type of perceptual fluency is an important aspect of domain expertise because it frees “cognitive head room” to engage in higher-order conceptual thinking about domain-relevant concepts (Goldstone and Barsalou, 1998; Gibson, 2000; Kellman and Garrigan, 2009). Cognitive theories of learning suggest that perceptual fluency is the result of non-verbal inductive learning processes (Richman et al., 1996; Koedinger et al., 2012). Inductive processes are learning processes that students engage in when they learn to discriminate, classify, categorize, and become more accurate and efficient in doing so (Koedinger et al., 2012). Perceptual learning processes are considered to be non-verbal because they do not rely on explicit reasoning (Kellman and Garrigan, 2009; Kellman and Massey, 2013). They are implicit because they happen unintentionally and unconsciously (Shanks, 2005), through experience with many examples (Gibson, 1969, 2000; Richman et al., 1996; Airey and Linder, 2009; Kellman and Garrigan, 2009). Recent research in educational psychology shows that providing perceptual trainings that support students' acquisition of perceptual fluency in connection making enhances their learning in math and science (Kellman et al., 2009; Massey et al., 2011).
Thus, the educational psychology literature suggests that both conceptual sense making of connections and perceptual fluency in connection making play a role in students' learning. This is in line with the chemistry education literature, which also suggest that both conceptual sense making and perceptual fluency are important aspects of students' learning (Wu et al., 2001; Kozma and Russell, 2005a, 2005b; Justi et al., 2009). Empirical evidence for the notion that conceptual sense making and perceptual fluency in connection making are distinguishable representational competences comes from a factor analysis on students' responses to a test of connection making with multiple graphical representations of atoms and molecules (Rau et al., 2015). This study also showed that conceptual sense making and perceptual fluency in connection making are predictors of students' knowledge about chemistry concepts. However, little empirical research has investigated whether instruction is most effective if it combines support for both conceptual sense-making of connections and perceptual fluency in connection making among multiple representations. This paper presents two experiments that test this hypothesis in the context of undergraduate students learning about atomic structure and bonding.
The experiments were carried out in the context of an educational technology designed to support students' learning of chemistry concepts through problem solving with multiple graphical representations: Chem Tutor. The use of an educational technology to support connection making in chemistry is appropriate for the following reasons. First, educational technologies can support interactive problem solving, which aligns with research on chemistry education that indicates that problem-solving activities can enhance conceptual learning (Bodner and Domin, 2000), especially when they include graphical representations (Bowen, 1990) and instructional support (Wu and Shah, 2004). Second, educational technologies can ask students to manipulate dynamic, interactive representations. This choice aligns with research showing that interactive representations lead to better learning in chemistry than static representations (Williamson, 2014). Finally, educational technologies play an increasingly important role in undergraduate education (Eichler and Peeples, 2013), thanks to the availability of course management systems such as Moodle. The push towards the use of educational technologies is also due to increasing enrollments in undergraduate courses while financial resources are decreasing. Therefore, situating this research in the context of educational technologies is both appropriate and timely.
In the following, I first describe Chem Tutor. I then present results from two experiments that test the effects of conceptual sense-making support and perceptual fluency-building support for connection making on students' learning of chemistry knowledge. I conclude the paper by discussing implications from this research for our understanding of how students learn with multiple graphical representations in chemistry, as well as practical recommendations for chemistry education practice.
Educational technology for undergraduate chemistry: chem tutor
Chem Tutor is an intelligent tutoring system for undergraduate chemistry learning (Rau et al., 2015). Intelligent tutoring systems are a type of educational technology that is grounded in cognitive theories of learning and artificial intelligence. They pose complex problem-solving activities and provide individualized step-by-step guidance at any point during the problem-solving process (VanLehn, 2011). At the heart of intelligent tutoring systems lies a cognitive model of the students' problem-solving steps. This model allows to detect multiple strategies a student might use to solve a problem (Aleven et al., 2009), and to provide detailed feedback and hints on how to solve the next step (Corbett et al., 2001). Traditional intelligent tutoring systems use a rule-based cognitive model that is based on production-rule theories of learning (Anderson et al., 1995; Corbett and Anderson, 2001; Ritter et al., 2007). Chem Tutor is a newer type of intelligent tutoring systems, called example-tracing tutors (Aleven et al., 2009). Example-tracing tutors use a cognitive model that is not rule based, but instead relies on generalized examples of correct and incorrect solution paths. Chem Tutor was created using Cognitive Tutor Authoring Tools (CTAT; Aleven et al., 2009), which allows for rapid iterations of prototyping and pilot-testing. The design of Chem Tutor followed a learner-centered approach, which involved surveys of undergraduate chemistry students, interviews and eye-tracking studies with undergraduate and graduate students, and extensive pilot testing (Rau et al., 2015; Rau, under review). As part of this learner-centered approach, experienced chemistry instructors provided feedback on the design of Chem Tutor's activities. Findings from these learner-centered studies were used to design hints and error feedback messages so that they address misconceptions students might have about the content covered in the tutor problems. For example, if a student makes a mistake that indicates he/she does not understand VSEPR theory, an error feedback message would provide an explanation of the concept. This design ensures that Chem Tutor provides detailed content-level instruction only to students who need it.
Chem Tutor covers two units: one focuses on atomic structure, another focuses on bonding. Each unit uses a variety of graphical representations that are commonly used in instructional materials on these topics (Shusterman and Shusterman, 1997; Furio et al., 2000; Matta and Gillespie, 2002; Cooper et al., 2012; Höst et al., 2012; Bergqvist et al., 2013; Chung, 2013; Luxford, 2013). Specifically, the atomic structure unit incorporates Lewis structures, Bohr models, energy diagrams, and orbital diagrams (see Fig. 1). The bonding unit incorporates Lewis structures, ball-and-stick figures, space-filling models, and EPMs (see Fig. 2). These representations were chosen based on a review of the chemistry education literature and based on a review of a number of curricula used at the high school and undergraduate levels (Rau, under review). Even though Chem Tutor is designed for introductory undergraduate courses, high-school curricula were included in this review of curricula because they likely yield insights into freshman students' prior knowledge about representations and about chemistry. Chem Tutor is available for free for anyone who would like to sign up at http://https://chem.tutorshop.web.cmu.edu/. It features a variety of problem types, described in the following.
Introductory units
Each unit of Chem Tutor provides a brief introduction into the topic covered and into the graphical representations used in the unit. To introduce students to the given topic, the key concepts that students need to know about in order to solve the tutor problems are reviewed (e.g., electronegativity in the introduction to the bonding unit). The introduction provides a review of these concepts with the expectation that students have encountered these concepts in prior chemistry instruction, because Chem Tutor is designed to align with curricula commonly used in introductory undergraduate courses (Rau et al., 2015). To introduce students to the graphical representations, Chem Tutor reviews how each of them depicts information about atoms and molecules. Specifically, the instruction for the atomic structure unit explains what information each of the graphical representations (see Fig. 1) show about atomic structure; in particular, which visual features denote relevant information, and what inferences they allow about properties of the atom. The introduction for the bonding unit explains what information each representation (see Fig. 2) shows about bonds, and what representational conventions they use (e.g., CPK coloring in ball-and-stick figures and space-filling models). The introduction also briefly discusses how the information that each representation provides about bonds relate to macroscopic phenomena that students are likely familiar with (e.g., ionic bonds result in crystalline structures that we know from table salt). The choice of topics covered in the introduction was informed by the learner-centered studies already mentioned: the introduction covered topics that students often struggle with and focused more on representations that proved to be difficult for students (e.g., understanding orbital diagrams and electron density concepts) (Rau, under review).
Individual-representation problems
In individual-representations problems, students work with one graphical representation at a time to solve a given problem. Fig. 3 shows an example of an individual-representation problem from Chem Tutor's atomic structure unit, in which students construct a Bohr model for oxygen. First, students are asked to reflect on properties of the atom. They can use the periodic table to look up information about the atom (e.g., oxygen has 8 electrons). Second, they plan how to construct the Bohr model (e.g., the Bohr model should show 2 electrons on the inner shell and 6 on the outer shell). Third, they use an interactive tool to construct the representation. They receive error-specific feedback on their interactions (e.g., the Bohr model shows all electrons, not only the valence electrons). They have to construct a correct representation before they can move on. Allowing students to construct graphical representations has been shown to be effective in chemistry education (Wu et al., 2001; Wu and Shah, 2004). Fourth, students are prompted to make inferences about the atom from use the representation. Hints, error feedback messages, and the wording of the prompts to help students make inferences are designed based on findings of interviews with PhD students and undergraduate students that were conducted as part of the learner-centered design approach (Rau et al., 2015; Rau, under review). The individual-representation problems were designed to correspond to typical problems in chemistry textbooks (Wibraham, 2005; Loudon, 2009; Moore et al., 2010; Brown et al., 2011), where students typically use one graphical representation at a time to solve a problem. In addition, the individual-representation problems were designed to help students gain some basic understanding of each graphical representation, which is considered to be a prerequisite to making connections between different representations (Ainsworth, 2006; Eilam, 2013).
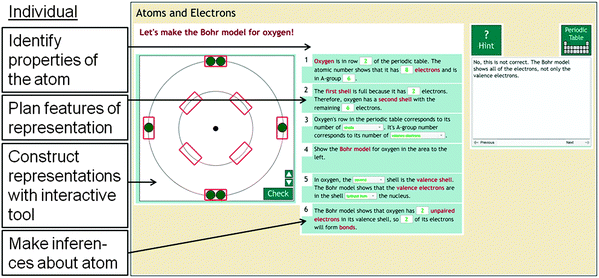 |
| Fig. 3 Example of an individual-representations problem from Chem Tutor's atomic structure unit. Students are first asked to identify properties of the atom, using the periodic table if they need to. Second, they plan the graphical representation. In the third step, they construct the graphical representation using an interactive tool. Finally, they are prompted to make inferences about the atom based on the information depicted in the graphical representation. | |
Conceptual sense-making problems for connection making
To support students in conceptually making sense of connections, Chem Tutor provides problems in which students have reason about differences and similarities among graphical representations by relating visual features to domain-relevant concepts. Fig. 4 shows an example from Chem Tutor's bonding unit in which students have to reason about similarities between the Lewis structure and the EPM in terms of what information they show about polarity. Students are prompted to self-explain how the two representations show where the electrons are likely to be and how they depict local charges. To this end, students are prompted to connect the dipole moment vectors in the Lewis structure to the blue-to-red color gradient in the EPM because that is how the two representations depict the polar nature of the molecule that results from the electronegativity difference between carbon and oxygen.
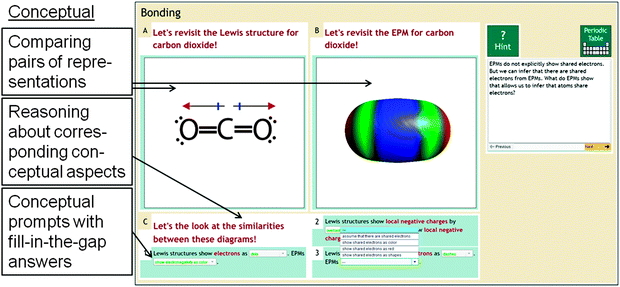 |
| Fig. 4 Example of a conceptual sense-making problem from Chem Tutor's bonding unit. Students are comparing pairs of graphical representations that show the same molecule. They are prompted to conceptually reason about similarities or differences between these graphical representations. | |
The design of conceptual sense-making problems aligns with findings from the educational psychology literature: they ask students to actively make connections based on visual features that depict corresponding conceptual aspects of the chemistry content, and they provide prompts and assistance for students to make these connections (Seufert, 2003; Bodemer and Faust, 2006; van der Meij and de Jong, 2006). The conceptual prompts in these problems use a fill-in-the-gap format with menu-based selection to support self-explanation. Menu-based selection prompts have been shown to support self-explanation in several empirical studies with intelligent tutoring systems (Aleven and Koedinger, 2002; Atkinson et al., 2003), and have been shown to be more effective in enhancing learning outcomes than open-ended prompts (Johnson and Mayer, 2010; Van der Meij and de Jong, 2011; Gadgil et al., 2012).
The content of the self-explanation prompts was designed based on findings from learner-centered studies (Rau et al., 2015; Rau, under review) in which PhD students in chemistry and undergraduate students enrolled in chemistry courses were interviewed about conceptual connections. The learner-centered studies showed that focusing solely on visual features was associated with shallow reasoning about chemistry concepts overall, whereas attending to information content was associated with inferences about chemistry concepts that extended beyond what was explicitly depicted in the visual representations. This finding suggests that students should learn to pay attention to the information the different representations show about conceptual aspects of bonding, rather than to surface features of the representations. Therefore, the conceptual sense-making problems were designed to draw students' attention to information content of the graphical representations, rather than to the visual features.
The content of the self-explanation prompts was also informed by the learner-centered studies of PhD students and undergraduate students. These studies identified concepts about atomic structure and bonding that were mentioned significantly more frequently by PhD students than by undergraduate students. The self-explanation prompts focus on these concepts because they are important (PhD students mention them frequently) but difficult for undergraduate students to notice (undergraduate students often fail to mention them). Finally, the wording of the prompts was based on actual student statements obtained from the learner-centered studies when students were interviewed about conceptual connections.
Perceptual fluency-building problems for connection making
To support students in becoming perceptually fluent in translating among representations, Chem Tutor provides students with many short classification problems. Consider the two example problems shown in Fig. 5. The design of these problems aligns with Kellman and colleagues' perceptual learning paradigm (Kellman and Garrigan, 2009; Massey et al., 2011; Kellman and Massey, 2013) and encourage perceptual processes (i.e., non-verbal inductive learning processes) rather than conceptual processes in the following ways. First, they provide repeated experience with a large variety of examples. In Chem Tutor's perceptual problems students are given one representation (e.g., a Lewis structure) and have to identify which of four alternative representations (e.g., an EPM) likely shows the same molecule. Each problem is short (i.e., it involves only one step). Students receive several of these problems in a row, and they receive immediate correctness feedback.
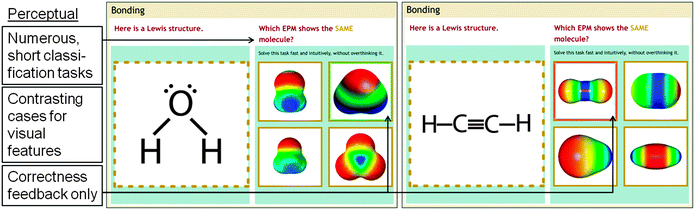 |
| Fig. 5 Example of a perceptual fluency-building problem from Chem Tutor's bonding unit. Students receive many rapid classification tasks. They are prompted to solve these tasks fast, based on perceptual strategies. The choice options use contrasting cases to emphasize relevant visual features. Students receive only correctness feedback. | |
Second, perceptual problems encourage students to rely on perceptual problem-solving strategies, so as to foster implicit, inductive learning. To this end, Chem Tutor provides a prompt to “solve this problem fast, without overthinking it”, before each problem starts in order to encourage students to rely on visual cues to solve the problem.
Third, students receive immediate feedback. In Chem Tutor's perceptual problems, immediate feedback is given through color highlighting: if the student gets the problem wrong, the choice is highlighted in red, if the student gets the problem right, the choice is highlighted in green.
Fourth, the choice options use contrasting cases. In Chem Tutor, the four alternative representations vary irrelevant visual features of the representations and contrast visual features that provide relevant information (e.g., geometry). Contrasting cases were designed based on findings from the learner-centered studies already mentioned (Rau et al., 2015; Rau, under review): the alternative representations varied visual features that students tend to confuse, fail to attend to, or are often distracted by. Thus, the four alternative representations emphasize visual features that students should learn to pay attention to.
In sum, the perceptual fluency-building problems are designed to help students become faster and more efficient at extracting relevant information from graphical representations. In contrast to the conceptual problems, the perceptual problems draw students' attention to visual features, such as the geometry of the molecule. Note that the goal of the perceptual fluency-building problems is to get students to the level at which connection making no longer requires cognitive effort. However, during the learning experience, before students achieve this desired level of perceptual fluency, the perceptual fluency-building problems may require cognitive effort.
Experiment 1
The goal of Experiment 1 was to test the hypothesis that instruction is most effective if it combines support for both conceptual sense making of connections and perceptual fluency in making connections among multiple graphical representations. To test this hypothesis, Experiment 1 contrasted the effects of providing students with conceptual sense-making problems (compared to not providing conceptual sense-making problems), and of providing students with perceptual fluency-building problems (compared to not providing perceptual fluency-building problems) on students' learning of chemistry knowledge. In addition, Experiment 1 explored whether students' prior knowledge about chemistry and their prior knowledge about connections affects whether they benefit from conceptual sense-making support and from perceptual fluency-building support. Experiment 1 was conducted in the context of Chem Tutor's bonding unit.
Methods
Participants.
Students were recruited from an introductory general chemistry course for science majors at a large Midwestern university. Sixty-six students participated in the experiment. The institution's internal review board (IRB) approved of the study. To protect students' confidentiality, they were assigned an anonymous code for the duration of the study. The experimenters used this code to store the collected data. Informed consent was obtained from students after they were given the option to ask questions about the experiment, and after they read over an IRB-approved document detailing in laymen's terms that participation in the study is voluntary, that the student may withdraw participation at any time without consequences, and that participation in the study is not associated with foreseeable risks (they only worked with a computer, not with chemicals). To compensate students for their participation, they received extra credit towards their course grade. The experiment was conducted in the middle of the semester. According to the course instructor, the content and the graphical representations in Chem Tutor's bonding unit were aligned with the content covered in the chemistry course.
Experimental design.
Students were randomly assigned to one of four conditions that varied two experimental factors: (1) whether or not students received conceptual sense-making support for connection making, and (2) whether or not they received perceptual fluency-building support for connection making. All students worked through the introduction to Chem Tutor's bonding unit, and all students encountered all representations (see Fig. 2). Students in the control condition (no-sense-making/no-fluency-building) worked on individual-representation problems only. Students in the sense-making/no-fluency-building condition received individual-representation problems and problems with conceptual sense-making support for connection making. Students in the no-sense-making/fluency-building condition received individual-representation problems and problems with perceptual fluency-building support for connection making. Finally, students in the sense-making/fluency-building condition received individual-representation problems, problems with conceptual sense-making support for connection making, and problems with perceptual fluency-building support for connection making.
To ensure that the amount of instruction students received was the same in all conditions, the number of problems per condition was adjusted such that the number of steps was the same across conditions. Equating the number of steps (rather than the number of problems) was necessary because the problems had different number of steps: individual problems had between seven and thirteen steps per problem, conceptual sense-making problems had between four and six steps per problem, and perceptual fluency-building problems had only one step per problem. Hence, students in the experimental conditions received fewer individual-representation problems than students in the control condition. Likewise, students in the sense-making/fluency-building condition received fewer sense-making problems than students in the sense-making/no-fluency-building condition and fewer fluency-building problems than students in the no-sense-making/fluency-building condition. This adjustment yielded interventions that were equivalent in time.
The sequence of individual-representation problems, conceptual sense-making problems and perceptual fluency-building problems was organized as follows. For each pair of representations, students first received individual-representation problems (e.g., one problem with a Lewis structure, and one problem with a ball-and-stick model). Next, if they were in one of the sense-making conditions, they received conceptual sense-making problems for this pair of representations. Then, if they were in one of the fluency-building conditions, they received perceptual fluency-building problems for this pair of representations. This sequence proved to be more effective than other sequences in prior research (Rau et al., 2014).
Measures.
The tests used in this experiment were developed as part of the learner-centered studies already mentioned (Rau et al., 2015; Rau, under review). Students took two tests, each described in turn.
Chemistry knowledge tests.
To investigate the effects of connection-making support on students' learning of chemistry knowledge, students took a pretest, an intermediate test, and a final posttest that assessed their understanding of the bonding concepts covered in Chem Tutor's bonding unit. There were three equivalent versions of the chemistry knowledge tests, such that the items on the test asked similar but not identical questions (e.g., they contained different molecules). Students were randomly assigned to receive the three different versions of the chemistry knowledge test in different orders for the pretest, the intermediate posttest, and the final posttest. The chemistry knowledge tests included reproduction items with and without graphical representations that were similar to the tutor problems, and transfer items that were different from the problems in Chem Tutor. For example, test items that were similar to the problems in Chem Tutor asked students to specify the local negative charge in a polar covalent bond, or to provide a definition for covalent bonds. Test items that were dissimilar from the problems in Chem Tutor asked students to select a schematic drawing that best describes the relationship between electronegativity differences and bond polarity, or to select a novel visualization of ionic bonding that best describes their mental model of ionic bonding, and to explain their reasoning. Thus, the chemistry knowledge test assessed both reproduction and transfer of student's knowledge about bonding.
Prior connections knowledge tests.
To investigate whether students' prior knowledge about connections affects their benefit from connection-making support, students were given prior knowledge tests that assessed their prior conceptual understanding of connections (prior conceptual-connections test), and their prior perceptual fluency in making connections (prior perceptual-fluency test). The tests included the graphical representations shown in Fig. 2. For example, the prior conceptual-connections test asked students to answer a number of true/false questions, such as “The EPM of formaldehyde uses red to show a local negative charge, but the space-filling model of formaldehyde uses red to denote the oxygen atom” (true), or “The Lewis structure shows that the Nitrogen atom in ammonia has an empty 2sp3 orbital, but the orbital diagram does not” (false). In the prior perceptual-fluency test students were given one graphical representation (e.g., a space-filling model showing water), and a selection of six other graphical representations (e.g., Lewis structure showing water, ball-and-stick figure showing carbon dioxide, EPM showing water, Lewis structure showing ammonia, ball-and-stick figure showing nitrogen dioxide, EPM showing carbon disulfide). Their task was to select all graphical representations that show the same molecule. So as to not force students to select any visual representations, they had the option to select “none of the above.”
Grading.
Students' performance on the tests was graded based on a rubric that assigned one point to each test item if the student had answered the item correctly. Partial credit was given for items that involved more than one step. To compute the final test scores, the sum of points was divided by the number of items on the test, so that the range of final scores was between 0 and 1.
Procedure
The experiment took place in a laboratory and involved two sessions of 90 minutes each. Sessions were scheduled no more than three days apart from one another. At the beginning of the first session, informed consent was obtained. Then, students received the chemistry knowledge pretest, the prior conceptual-connections test, and the prior perceptual-connections test. They then received an introduction into using the tutoring system. Next, students worked with the version of Chem Tutor's bonding unit that corresponded to their experimental condition. In the first session, students worked through about half of the tutor problems. At the end of the first session, they took the intermediate chemistry knowledge posttest. When they returned for the second session, students worked through the remainder of the tutor problems. Then, they took a final chemistry knowledge posttest.
Results
The final sample contained N = 66 students, n = 21 in the no-sense-making/no-fluency-building condition, n = 13 in the sense-making/no-fluency-building condition, n = 15 in the no-sense-making/fluency-building condition, and n = 17 in the sense-making/fluency-building condition. There were no differences between conditions at the pretest (F < 1). Table 1 shows means and standard deviations for students' scores on the chemistry knowledge tests, the prior conceptual-connections test, and the prior perceptual-fluency test, organized by test time and condition. In the analyses reported in the following, all p-values for post-hoc comparisons were adjusted using the Bonferroni correction. I report partial η2 for effect sizes on effects including more than two conditions, and Cohen's d for effect sizes of pairwise comparisons. According to (Cohen, 1988), an effect size partial η2 of 0.01 corresponds to a small effect, 0.06 to a medium effect, and 0.14 to a large effect. An effect size d of 0.20 corresponds to a small effect, 0.50 to a medium effect, and 0.80 to a large effect.
Table 1 Means and standard deviations (in parentheses) on the prior conceptual-connections test, the prior perceptual-fluency test, and the chemistry knowledge tests, organized by test time and condition for Experiment 1
|
Pretest |
Intermediate posttest |
Final posttest |
Conceptual-connections test |
Perceptual-fluency test |
Chemistry knowledge test |
Chemistry knowledge test |
Chemistry knowledge test |
No-sense-making/no-fluency-building |
0.68 (0.09) |
0.66 (0.26) |
0.44 (0.15) |
0.56 (0.15) |
0.61 (0.13) |
Sense-making/no-fluency-building |
0.69 (0.10) |
0.73 (0.26) |
0.39 (0.12) |
0.56 (0.11) |
0.53 (0.13) |
No-sense-making/fluency-building |
0.69 (0.14) |
0.68 (0.29) |
0.42 (0.10) |
0.67 (0.16) |
0.64 (0.15) |
Sense-making/fluency-building |
0.64 (0.11) |
0.71 (0.28) |
0.41 (0.11) |
0.58 (0.19) |
0.56 (0.14) |
Overall
|
0.67 (0.11)
|
0.72 (0.27)
|
0.42 (0.12)
|
0.58 (0.14)
|
0.59 (0.14)
|
Learning gains.
First, I investigated whether working with Chem Tutor resulted in learning of chemistry knowledge. To do so, I used a repeated-measures ANOVA with test time (i.e., pretest, intermediate, and final posttest) as the repeated within-subjects factor, and performance on the chemistry knowledge tests as dependent measures. There was a significant effect of test time, F(2,130) = 57.20, p < 0.01, partial η2 = 0.47. Post-hoc comparisons showed that students performed significantly better at the intermediate posttest than at the pretest, t(65) = 8.56, p < 0.01, d = 1.30, and that they performed significantly better at the final posttest than at the pretest, t(65) = 9.05, p < 0.01, d = 1.32. These results show that working with Chem Tutor's bonding unit resulted in significant learning gains on a test that assessed reproduction and transfer of students' conceptual understanding of bonding.
Effects of connection-making support.
Next, I investigated whether providing conceptual sense-making support and perceptual fluency-building support for connection making is effective in enhancing students' learning of chemistry knowledge, and whether students' prior knowledge affects the effectiveness of these two types of connection-making support. To investigate main effects of the experimental conditions on students' learning outcomes, I used a repeated-measures ANCOVA with posttest time (i.e., intermediate, and final posttest) as the repeated within-subjects factor, and conceptual sense-making support and perceptual fluency-building support as between-subjects factors, and performance on the chemistry knowledge pretest as the covariate. The ANCOVA model also tested for interactions of sense-making support and of perceptual fluency-building support with students' performance on the chemistry knowledge pretest, on the prior conceptual-connections test, and on the prior perceptual-connections test. Performance on the intermediate and final chemistry knowledge posttests was used as the dependent measure. Further, there was a marginally significant negative main effect of conceptual sense-making support, F(1,61) = 3.32, p < 0.10, partial η2 = 0.05. There was a marginally significant positive main effect of perceptual fluency-building support, F(1,61) = 3.10, p < 0.10, partial η2 = 0.05. There was no significant interaction between conceptual sense-making support and perceptual fluency-building support, F(1,61) = 1.02, p > 0.10. Fig. 6 provides a summary of these results.
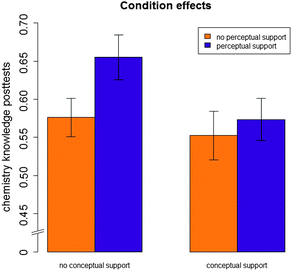 |
| Fig. 6 Effects of conceptual sense-making support and perceptual fluency-building support on students' learning outcomes on the intermediate and final posttests in Experiment 1. Bars show estimated marginal means from the ANCOVA model. Error bars show standard errors of the mean. | |
There were no significant interactions of perceptual fluency-building support with students' performance on the chemistry knowledge pretest, F(1,61) = 1.52, p > 0.10, with students' performance on the prior conceptual-connections test (F < 1), or on the prior perceptual-connections test (F < 1). There were no significant interactions of conceptual sense-making support with students' performance on the prior conceptual-connections test (F < 1), or on the prior perceptual-connections test (F < 1). However, there was a marginally significant interaction of conceptual sense-making support with students' performance on the chemistry knowledge pretest, F(1,61) = 3.40, p < 0.10, partial η2 = 0.06, such that conceptual sense-making support had a stronger negative effect on students with high prior chemistry knowledge than for students with low prior chemistry knowledge.
Discussion
Experiment 1 tested the hypothesis that a combination of conceptual sense-making support for connection making and perceptual fluency-building support for connection making would enhance students' learning of chemistry knowledge. With respect to conceptual sense-making support, the results do not support this hypothesis. To the contrary: conceptual sense-making support had a small, marginally significant negative effect on students' learning outcomes. This finding is surprising because it stands in contrast to prior research on learning with multiple representations in educational psychology (Seufert, 2003; Bodemer and Faust, 2006) and chemistry education (Stieff, 2005; Luxford et al., 2011; Linenberger and Bretz, 2012), which showed that interventions that help students in conceptually making sense of connections among multiple representations can enhance their learning outcomes. It is possible that the students who participated in Experiment 1 already had considerable experience in making sense of among between the graphical representations that Chem Tutor provided. The fact that the negative effect of conceptual sense-making support was more pronounced for students with high prior chemistry knowledge lends credibility to this interpretation. Indeed, the instructor for the chemistry course from which these students were recruited confirmed that they had encountered the graphical representations in their course prior to the experiment. Table 1 (column 2, labeled “conceptual-connections test”; M = 0.67, SD = 0.11) indicates that the students in Experiment 1 had some prior conceptual understanding of connections, although the average score of 0.67 is certainly not perfect. The results from Experiment 1 indicate that students with at least a moderate level of prior conceptual understanding of connections do not benefit from conceptual sense-making support, but instead should practice to solve problems with the graphical representations, as they do in Chem Tutor's individual-representations problems. Given that the individual-representations problems were designed to resemble regular textbook problems, this finding is promising because it indicates that regular educational practice is effective for students who have a good conceptual grasp of how different graphical representations depict similar and different aspects of chemistry concepts.
With respect to perceptual fluency-building support, the results provide qualified support for the hypothesis: perceptual fluency-building support had a marginally significant positive effect on students' learning outcomes. This finding suggests that students' learning of chemistry knowledge can be enhanced by supporting them in becoming perceptually fluent in making these connections. However, in light of the marginally significant effects, further research is warranted to support this claim.
A limitation of this experiment is that the majority of the students who participated in Experiment 1 were science majors who had a considerable amount of prior knowledge about the content covered in Chem Tutor's bonding unit, and (perhaps more importantly) who had encountered the graphical representations used in Chem Tutor in their course. This raises the question whether choosing a different population of students, who have less prior knowledge about chemistry and less experience with the graphical representations, would yield similar results. Experiment 2 was conducted to address this question.
Experiment 2
The goal of Experiment 2 was to investigate whether the findings from Experiment 1 generalize to students who encounter representations they are unfamiliar with. To this end, students were recruited from a chemistry course for non-science majors. Experiment 2 was conducted in the context of Chem Tutor's atomic structure unit, because the course instructor felt that this unit was appropriate for the course.
A further change in Experiment 2 was that it investigated the role of an additional prior competence: mental rotation ability. Mental rotation ability has been found to significantly predict students' learning outcomes in chemistry (Stieff, 2007, 2013; Stieff et al., 2011) and in other STEM domains that heavily rely on the use of graphical representations (Wai et al., 2009). Mental rotation ability has also been associated with students' pursuit of careers in STEM domains (Uttal et al., 2013). Hence, including a test of mental rotation ability may yield additional insights into how non-science majors learn with graphical representations.
Methods
Participants.
A total of ninety-two students from an introductory general chemistry course for non-science majors participated in the experiment. For most of the students enrolled in this course, this course is the only required chemistry course for their undergraduate degree. The same IRB process was used as in Experiment 1. Students received course credit for participating in the study. According to the instructor, students had not encountered the representations used in this unit, except for the Lewis structure.
Experimental design.
The experimental design was the same as in Experiment 1: Students were randomly assigned to one of four conditions (i.e., the no-sense-making/no-fluency-building condition, the sense-making/no-fluency-building condition, the no-sense-making/fluency-building condition, or the sense-making/fluency-building condition). The difference to Experiment 1 was that students worked through the atomic structure unit of Chem Tutor (instead of the bonding unit), and that the tests were adjusted accordingly.
Procedure
The experiment took place in a laboratory and involved two sessions of 90 minutes each. Sessions were scheduled no more than three days apart. At the beginning of the first session, informed consent was obtained. Next, students first received the chemistry knowledge pretest, the prior conceptual-connections test, the prior perceptual-connections test, and the mental rotation ability test. They then received an introduction into atomic structure. Next, students worked with the version of Chem Tutor's atomic structure unit that corresponded to their experimental condition. In the first session, students worked through about half of the tutor problems. At the end of the first session, they took the intermediate chemistry knowledge posttest. When they returned for the second session, students worked through the remainder of the tutor problems. Then, they took the final chemistry knowledge posttest.
Measures.
The measures were identical to those used in Experiment 1, with the following two exceptions. First, the chemistry knowledge tests focused on concepts of atomic structure (to align with the Chem Tutor unit on atomic structure). Second, students received an additional test that assessed their mental rotation ability. Specifically, they were given the Vandenberg and Kuse mental rotation ability test (Peters et al., 1995). This test presents students with a drawing of an object and asks them to identify which of four other drawings show the same object. This task requires students to mentally rotate the given object to align it with the comparison objects. I chose this test for the experiment because it has been used in prior research on the impact of students' mental rotation ability on STEM learning (Stieff, 2007, 2013; Wai et al., 2009; Stieff et al., 2011; Uttal et al., 2013).
Results
The final sample contained N = 92 students, n = 18 in the no-sense-making/no-fluency-building condition, n = 24 in the sense-making/no-fluency-building condition, n = 23 in the no-sense-making/fluency-building condition, and n = 27 in the sense-making/fluency-building condition. There were no differences between conditions at the pretest, F(1,94) = 1.22, p > 0.10. Table 2 shows means and standard deviations for students' scores on the chemistry knowledge test, the prior conceptual-connections test, the prior perceptual-fluency test, and the mental rotation ability test organized by test time and condition.
Table 2 Means and standard deviations (in parentheses) on the prior conceptual-connections test, the prior perceptual-fluency test, the mental rotation ability test, and the chemistry knowledge tests, organized by test time and condition for Experiment 2
|
Pretest |
Intermediate posttest |
Final posttest |
Conceptual-connections test |
Perceptual-fluency test |
Mental rotation ability test |
Chemistry knowledge test |
Chemistry knowledge test |
Chemistry knowledge test |
No-sense-making/no-fluency-building |
0.45 (0.21) |
0.29 (0.16) |
0.50 (0.20) |
0.42 (0.17) |
0.52 (0.21) |
0.56 (0.16) |
Sense-making/no-fluency-building |
0.32 (0.22) |
0.25 (0.16) |
0.48 (0.20) |
0.33 (0.16) |
0.49 (0.18) |
0.51 (0.15) |
No-sense-making/fluency-building |
0.31 (0.14) |
0.34 (0.21) |
0.60 (0.17) |
0.40 (0.16) |
0.50 (0.21) |
0.57 (0.18) |
Sense-making/fluency-building |
0.43 (0.24) |
0.34 (0.16) |
0.53 (0.20) |
0.40 (0.18) |
0.56 (0.18) |
0.59 (0.18) |
Overall
|
0.37 (0.21)
|
0.31 (0.17)
|
0.51 (0.20)
|
0.38 (0.17)
|
0.52 (0.19)
|
0.56 (0.17)
|
Learning gains.
First, I investigated whether working with Chem Tutor resulted in learning of chemistry knowledge. Like in Experiment 1, I used a repeated-measures ANOVA with test time (i.e., pretest, intermediate, and final posttest) as the repeated within-subjects factor, and performance on the chemistry knowledge tests as dependent measures. There was a significant effect of test time, F(2,182) = 32.28, p < 0.01, partial η2 = 0.26. Post-hoc comparisons showed that students performed significantly better at the intermediate posttest than at the pretest, t(91) = 6.14, p < 0.01, d = 0.73, and that they performed significantly better at the final posttest than at the pretest, t(91) = 7.41, p < 0.01, d = 1.02. These results show that working with Chem Tutor's atomic structure unit resulted in significant learning gains on tests that assess reproduction and transfer of students' conceptual understanding of atomic structure.
Effects of connection-making support.
Like in Experiment 1, I investigated the effects of connection-making support using a repeated-measures ANCOVA with posttest time (i.e., intermediate, and final posttest) as the repeated within-subjects factor, and conceptual sense-making support and perceptual fluency-building support as between-subjects factors, and performance on the chemistry knowledge pretest as the covariate. The ANCOVA model tested for interactions of conceptual sense-making support with pretest performance, with students' performance on the mental rotation ability test, on the prior conceptual-connections test, and on the prior perceptual-connections test, as well as of perceptual fluency-building support with pretest performance, with students' performance on the mental rotation ability test, on the prior conceptual-connections test, and on the prior perceptual-connections test. Performance on the chemistry knowledge posttests was used as the dependent measure.
There was no significant main effect of conceptual sense-making support, F(1,84) = 1.50, p > 0.10. There was a significant positive main effect of perceptual fluency-building support, F(1,84) = 4.27, p < 0.05, partial η2 = 0.05. There was a marginally significant interaction between conceptual sense-making support and perceptual fluency-building support, F(1,84) = 2.89, p < 0.10, partial η2 = 0.03. Fig. 7 illustrates the nature of this effect: perceptual fluency-building support was effective only if it was provided in combination with conceptual sense-making support. For students who did not receive conceptual sense-making support, perceptual fluency-building support was not effective; these students learned better with individual-representations problems only.
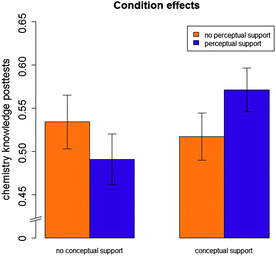 |
| Fig. 7 Effects of conceptual sense-making support and perceptual fluency-building support on students' learning outcomes on the intermediate and final posttests in Experiment 2. Bars show estimated marginal means from the ANCOVA model. Error bars show standard errors of the mean. | |
There were no significant interactions of conceptual sense-making support or perceptual fluency-building support with students' performance on the chemistry knowledge pretest, on the prior conceptual-connections test, or on the prior perceptual-connections test (p > 0.10). There was no significant interaction of conceptual sense-making support with students' mental rotation ability (F < 1), but there was a significant interaction of perceptual fluency-building support with students' mental rotation ability, F(1,84) = 5.21, p < 0.05, partial η2 = 0.06, such that students with high mental rotation ability benefited more from perceptual fluency-building support than students with low mental rotation ability.
Discussion
Experiment 2 tested the hypothesis that a combination of conceptual sense-making support for connection making and perceptual fluency-building support for connection making would enhance students' learning of chemistry knowledge. The results are somewhat surprising: only the combination of conceptual sense-making support and perceptual fluency-building support for connection making were effective. Providing only perceptual fluency-building support (i.e., without conceptual sense-making support) resulted in lower learning gains on a chemistry knowledge test than providing no connection-making support at all. Likewise, conceptual sense-making support was effective only when combined with perceptual connection-making support. This finding provides support for the overall hypothesis that both aspects of connection making, the ability to conceptually make sense of connections and the ability to fluently translate among them based on perceptual cues, are important aspects of students' learning. By supporting students in making connections, we can enhance their learning of chemistry knowledge.
In contrast to Experiment 1, Experiment 2 shows that students benefit from conceptual sense-making support (when combined with perceptual fluency-building support). It is possible that the fact that students in Experiment 2 had lower prior knowledge and less experience with the graphical representations accounts for this difference between Experiments 1 and 2. According to the instructor of the chemistry course from which students were recruited, they only had experience with the Lewis structure, not with the other representations. Comparing Tables 1 and 2 (column 2, labeled “conceptual-connections test”) confirms that students in Experiment 2 had lower average scores on the prior conceptual-connections test (M = 0.37, SD = 0.23) than students in Experiment 1 (M = 0.67, SD = 0.11). It is important to note that a comparison between Experiments 1 and 2 is difficult because the participating students differed on many factors (e.g., the experiments took place in different semesters, the students were enrolled in different courses with different instructors, and they worked on different units of Chem Tutor). Yet, the conclusion seems reasonable that students who already have a relatively good conceptual understanding of how to make sense of connections (Experiment 1) do not benefit from conceptual sense-making support, whereas students who do not know how to make sense of connections (Experiment 2) benefit from conceptual sense-making support.
This difference between Experiments 1 and 2 may also explain why Experiment 2 revealed a significant interaction between conceptual sense-making support and perceptual fluency-building support, whereas Experiment 1 did not. It seems that, in order to benefit from perceptual fluency-building support, students need to have some level of conceptual understanding of the connections among graphical representations. Because students in Experiment 1 had this conceptual understanding prior to the experiment, they benefited from perceptual fluency-building support. Because students in Experiment 2 did not have this prior conceptual understanding of connections, they needed conceptual sense-making support in order to benefit from perceptual fluency-building support. This result suggests that learning perceptual mappings based on visual features is only beneficial if students understand what concepts the mappings between visual features stand for. If students only learn mappings based on visual features (i.e., if they do not have prior knowledge about conceptual connections and receive only perceptual fluency-building problems), students learn meaningless mappings, and these do not help them learn chemistry. Put differently, it seems that acquiring conceptual understanding of connections is an intermediate step that students have to engage in to become perceptually fluent in making connections, if the goal is to enhance their learning of chemistry knowledge. Thus, the results from Experiments 1 and 2 provide some support for the notion that the ability to conceptually make sense of connections is a prerequisite for students' benefit from support that helps them to become perceptually fluent in translating between multiple graphical representations.
Another interesting finding is that the positive effect of perceptual fluency-building support is more pronounced for students with high mental rotation ability than for students with low mental rotation ability. The perceptual fluency-building problems ask students to map visual features that are not always spatially aligned. Hence, students may have to mentally rotate the graphical representations when solving the perceptual fluency-building problems. This task is arguably harder for students with low mental rotation ability than for students with high mental rotation ability. As a consequence, students with low mental rotation ability may fail at this task, which might jeopardize their benefit from perceptual fluency-building support. It is possible that students with low mental rotation ability may benefit more from a version of perceptual fluency-building support that provides spatially aligned examples. Future research should investigate how best to tailor perceptual fluency-building support to the needs of students with low mental rotation ability.
It would also be interesting to investigate how students' familiarity with the different representations may have affected their benefit from particular connection-making problems. For example, it is possible that students benefit more from conceptual sense-making problems and perceptual fluency-building problems in which they connect a familiar representation (e.g., the Lewis structure) to an unfamiliar representation (e.g., the orbital diagram), than from problems in which they connect two unfamiliar representations (e.g., energy diagram and orbital diagram). Experiment 2 was not set up to investigate this question, but it would be interesting to explore this question in future research.
Conclusions
This paper presented two experiments that investigated how best to enhance students' learning of chemistry by helping them acquire critical representational competences: the ability to make connections among multiple graphical representations. The hypothesis tested in this paper was that two aspects of connection making are important: (1) students' ability to conceptually make sense of connections and (2) perceptual fluency in making connections. By testing this hypothesis, this paper extends prior research on connection making that has focused on conceptual sense making of connections (Stieff, 2005; Bodemer and Faust, 2006; van der Meij and de Jong, 2006; Michalchik et al., 2008) and integrates this research with positions arguing that perceptual fluency plays a critical role in students' becoming part of community practices because it allows them to participate in discipline discourse (Kozma and Russell, 2005a, 2005b; Airey and Linder, 2009).
This paper provides a somewhat surprising result: support for conceptual sense-making of connections may not always be beneficial. For students who already have a relatively good conceptual understanding of connections, this type of support is not effective. These students benefited more from individual-representation problems alone. Recall that the individual-representation problems in Chem Tutor were designed to resemble typical textbook problems, in which students solve chemistry problems using only one graphical representation at a time. Hence, the results presented in this paper suggest that regular practice is effective for students who have a good conceptual understanding of connections. For chemistry educators, this finding suggests that they might want to test their students' conceptual-connections knowledge before they decide whether to provide instructional support that aims at helping them conceptually make sense of connections among multiple graphical representations, or to have them work on regular chemistry problems. Specifically, chemistry educators could test whether their students have understood how different graphical representations (e.g., a Lewis structure, an EPM) provide complementary information about the given topic (e.g., bonding). For example, a chemistry educator could ask students to explain that the lines in Lewis structures that denote bonds do not indicate the polarity of the bond (i.e., how the electrons are likely to be distributed across the molecule), whereas EPMs use color to indicate electron density do not show which atoms form the bonds. If students have not yet understood these conceptual connections, they should work on conceptual sense-making problems. If they already do understand how the different representations about the given topic, they should rather work on problem-solving tasks without conceptual sense-making support.
The results from both experiments reported provide empirical evidence for the hypothesis that helping students become perceptually fluent (i.e., by helping them become more efficient at attending to visual features that depict relevant information) promotes their learning of chemistry knowledge. However, the results from the two experiments also cautions that perceptual fluency-building support is only effective if students either already have at least a moderate level of prior conceptual understanding of connections (Experiment 1) or if they also receive conceptual sense-making support for connection making (Experiment 2). This finding extends our theoretical understanding of how students learn with multiple graphical representations. It seems that perceptual fluency in connection making builds on a prerequisite ability to conceptually make sense of connections. This finding also extends prior observational studies showing that fluency can facilitate students' learning of domain knowledge (Airey and Linder, 2009): the present experiments indicate that fluency enhances learning of domain knowledge only if it is built on a solid foundation of students' ability to conceptually make sense of connections. This finding also provides practical suggestions for chemistry educators: they should be aware of their students' conceptual-connections knowledge when deciding whether and when to provide perceptual fluency-building support designed to help students become efficient at translating between multiple graphical representations based on perceptual cues. Specifically, educators should make sure that their students have a sound conceptual understanding of what the relations between different graphical representations mean in terms of conceptual aspects they show about the topic, before they provide students with perceptual fluency-building problems.
The finding that perceptual fluency-building support is effective is also interesting against the background of recent developments in chemistry education to incorporate connection-making games that ask students to rapidly translate among graphical representations (Eastwood, 2013; Moreira, 2013). These connection-making games are similar to the perceptual fluency-building problems tested in this paper with respect to some aspects: they ask students to rapidly translate among graphical representations, in order to promote efficiency in connection making. Thus, these connection-making games provide experience with many examples and they provide immediate feedback. However, these games do not incorporate other aspects of perceptual fluency-building problems: the examples are not designed based on considerations of visual features that depict relevant information (i.e., contrasting cases), and they do not explicitly ask students to employ perceptual problem-solving strategies, as opposed to conceptual strategies. Although it remains an open question whether these games indeed foster perceptual fluency in connection making, it might be advisable to consider students' levels of conceptual-connections knowledge before providing them with connection-making games.
The finding that mental rotation ability affects students' benefit from perceptual fluency-building support is also interesting in the light of the discussion about connection-making games. It is possible that students with low mental rotation ability benefit less from playing connection-making games, or that they may need additional support when playing these games. Future research should investigate this question to ensure that novel interventions that target representational competences do not put students with low mental rotation ability at a disadvantage.
Several limitations of the two experiments need to be discussed. First, both experiments were conducted in a lab setting. Chem Tutor is designed to be used as a homework system within undergraduate chemistry courses. In future research, I will investigate whether a more natural setting will yield similar results as the experiments reported in this paper. A second limitation is that Experiment 1 did not include a mental rotation ability test. Therefore, it is unclear whether the mental rotation ability of the students who were enrolled in a course for chemistry majors affected their benefit from perceptual fluency-building support. Thus, more research is needed to explore how mental rotation ability affects students' benefit from different types of support for connection making among multiple graphical representations.
Another limitation is that, as already mentioned, comparing students from two different experiments based on their prior conceptual understanding of connections is difficult because many other factors may have contributed to the differences between Experiments 1 and 2. Future research should therefore investigate whether, indeed, students' prior conceptual understanding of connections as it develops over the course of a longer learning experience (e.g., during a chemistry course) affects their benefit from conceptual and perceptual support for connection making. The experiments reported here cannot test this hypothesis because they were cross-sectional (i.e., they investigated the effects of students' prior competences as they happened to be at the beginning of the intervention). A longer experiment with a longer intervention would be needed to investigate how students' benefit from conceptual and perceptual support differs, depending on how much conceptual understanding of connections they have at any point in time. In other words, the experiments reported in this paper yield a new hypothesis that can be evaluated empirically; namely, that students' learning of chemistry knowledge can be enhanced by providing different types of connection-making support as they learn to conceptually make sense of connections throughout a longer learning experience.
A third limitation of this research is that it was situated in a highly interactive educational technology. As mentioned, educational technologies are becoming more prevalent in undergraduate education in chemistry, because they offer several features that provide advantages over more traditional learning environments, such as textbooks and lectures: they can support problem solving with interactive representations, and they can provide detailed, individualized feedback in real time. While it seems reasonable to assume that findings from the experiments reported in this paper may generalize to other educational technologies, it is possible that the interactive nature of Chem Tutor restricts the generalizability of the findings to educational technologies. Therefore, further research would be needed to address the question whether the combination of conceptual and perceptual support for connection making is most effective when these types of support are implemented in more traditional, less interactive types of materials, such as textbooks and lectures.
Finally, a potential limitation of the experiments reported here is that they did not investigate whether the specific pairing of graphical representations that were presented in the connection-making problems had an impact on students' benefit from the connection-making problems. The representation pairs (e.g., Bohr model and Lewis structure vs. Bohr model and energy diagram) differed with respect to their “information overlap”: that is, to what extent they provide the same information about the given concept. The information overlap may affect how cognitively demanding the connection-making problems were, which may in turn affect how much students benefited from these problems. Further, it is possible that the effect of information overlap on students' benefit from connection-making problems depends on the individual student's prior experience with the given graphical representations. Future research should investigate these questions, so as to provide further insights into how best to design conceptual sense-making problems and perceptual fluency-building problems with the optimal representation pairs.
To conclude, the experiments presented in this paper extend our understanding of how students learn with multiple graphical representations. Specifically, both studies provide empirical evidence that we can enhance undergraduate students' learning of chemistry knowledge by supporting them in making connections among multiple graphical representations. They also provide evidence that both conceptual sense-making of connections and perceptual fluency in connection making are important aspects of students' learning of chemistry content. The overall finding across Experiments 1 and 2 seems to be that perceptual fluency-building builds on conceptual sense-making of connections. These findings yield practical recommendations for how best to combine conceptual and perceptual support for connection making. If students have low prior conceptual understanding of connections, they should receive both conceptual sense-making support and perceptual fluency-building support. If they have high prior conceptual understanding of connections, they should receive only perceptual fluency-building support for connection making. Given the pervasiveness of multiple graphical representations in chemistry education, these findings have the potential to generalize to other topics than atomic structure and bonding.
Acknowledgements
The University of Wisconsin—Madison Graduate School and the Wisconsin Center for Education Research supported this research. We thank John Moore for his advice. I thank John Moore, Oana Martin, Teri Larson, Ned Siebert, and Stephen Block for their help in recruiting students. I thank Amanda Evenstone, Joe Michaelis, Sally Wu, Greyson Bahr, Youn Ku Choi, Brady Cleveland, Natalie Fay, Taryn Gordon, Ashley Hong, Will Keesler, Amber Kim, Ashley Lee, Marguerite Lee, Aditi Renganathan, Jamie Schuberth, Mike Schwanke, Peter Van Sandt, Theresa Shim, and Philip Zimring for their help in conducting the experiments.
References
- Ainsworth S., (2006), DeFT: a conceptual framework for considering learning with multiple representations, Learn. Instr., 16, 183–198.
- Airey J. and Linder C., (2009), A disciplinary discourse perspective on university science learning: achieving fluency in a critical constellation of modes, J. Res. Sci. Teach., 46, 27–49.
- Aleven V. A. W. M. M. and Koedinger K. R., (2002), An effective metacognitive strategy: learning by doing and explaining with a computer-based cognitive tutor, Cognitive Sci., 26, 147–179.
- Aleven V., McLaren B. M., Sewall J. and Koedinger K. R., (2009), A new paradigm for intelligent tutoring systems: example-tracing tutors, Int. J. Artif. Intelligence in Educ., 19, 105–154.
- Anderson J. R., Corbett A. T., Koedinger K. R. and Pelletier R., (1995), Cognitive tutors: lessons learned, J. Learn. Sci., 4, 167–207.
- Atkinson R. K., Renkl A. and Merrill M. M., (2003), Transitioning From Studying Examples to Solving Problems: Effects of Self-Explanation Prompts and Fading Worked-Out Steps., Journal of Educational Psychology, 95, 774–783.
- Bergqvist A., Drechsler M., de Jong O. and Chang Rundgren S. N., (2013), Representations of chemical bonding models in school textbooks-help or hindrance for understanding? Chem. Educ. Res. Pract., 14, 589–606.
- Bodemer D. and Faust U., (2006), External and mental referencing of multiple representations, Comput. Hum. Behav., 22, 27–42.
- Bodner G. M. and Domin D. S., (2000), Mental models: the role of representations in problem solving in chemistry, University Chemistry Education, 4, 24–30.
- Bowen C. W., (1990), Representational systems used by graduate students while problem solving in organic synthesis, J. Res. Sci. Teach., 27, 351–370.
- Brown T. L., LeMay H. E., Bursten B. E., Murphy C. J. and Woodward P. M., (2011), Chemistry – the central science, Prentice Hall.
- Cheng M. and Gilbert J. K., (2009), in Gilbert J. K. and Treagust D. F. (ed.), Multiple Representations in Chemical Education, Berlin/Heidelberg: Springer, pp. 191–208.
- Chi M. T. H., Feltovitch P. J. and Glaser R., (1981), Categorization and Representation of Physics Problems by Experts and Novices, Cognitive Sci., 5, 121–152.
- Chung W. C., (2013), Three-dimensional atomic orbital plots in the classroom using Winplot, J. Chem. Educ., 90, 1090–1092.
- Cohen J., (1988), Statistical Power Analysis for the Behavioral Sciences, Hillsdale, NJ: Lawrence Erlbaum Associates.
- Cooper M. M., Underwood S. M. and Hilley C. Z., (2012), Development and validation of the implicit information from Lewis structures instrument (IILSI): do students connect structures with properties? Chem. Educ. Res. Pract., 13, 195–200.
- Corbett A. and Anderson J., (2001), Proceedings of CHI 2001, ACM Press.
- Corbett A. T., Koedinger K. and Hadley W. S., (2001), in Goodman P. S. (ed.), Technology enhanced learning: opportunities for change., Mahwah, NJ, US: Lawrence Erlbaum Associates Publishers, pp. 235–263.
- Davidowitz B. and Chittleborough G., (2009), in Gilbert J. K. and Treagust D. F. (ed.), Multiple representations in chemical education, Dordrecht, Netherlands: Springer, pp. 169–191.
- De Jong O. and Taber K. S., (2014), in Lederman N. and Abell S. K. (ed.), Handbook of Research on Science Education, New York, NY: Routledge, pp. 457–480.
- Dori Y. J. and Barak M., (2001), Virtual and physical molecular modeling: fostering model perception and spatial understanding, Educational Technology & Society, 4, 61–74.
- Eastwood M. L., (2013), Fastest fingers: a molecule-building game for teaching organic chemistry, J. Chem. Educ., 90, 1038–1041.
- Eichler J. F. and Peeples J., (2013), Online Homework Put to the Test: A Report on the Impact of Two Online Learning Systems on Student Performance in General Chemistry, J. Chem. Educ., 90, 1137–1143, DOI:10.1021/ed3006264.
- Eilam B., (2013), in Treagust D. F. and Tsui C.-Y. (ed.), Multiple Representations in Biological Education, Dordrecht, Netherlands: Springer, pp. 55–73.
- Furio C., Calatayud M. L., Barcenas S. L. and Padilla O. M., (2000), Functional fixedness and function reduction as common sense reasonings in chemical equilibrium and in geometry and polarity of molecules, Sci. Educ., 84, 545–565.
- Gadgil S., Nokes-Malach T. J. and Chi M. T., (2012), Effectiveness of holistic mental model confrontation in driving conceptual change, Learn. Instr., 22, 47–61.
- Gegenfurtner A., Lehtinen E. and Säljö R., (2011), Expertise differences in the comprehension of visualizations: a meta-analysis of eye-tracking research in professional domains, Educ. Psychol. Rev., 23, 523–552.
- Gibson E. J., (1969), Principles of perceptual learning and development, New York: Prentice Hall.
- Gibson E. J., (2000), Perceptual learning in development: some basic concepts, Ecol. Psychol., 12, 295–302.
- Goldstone R. L. and Barsalou L. W., (1998), Reuniting perception and conception, Cognition, 65, 231–262.
- Hinze S. R., Williamson V. M., Deslongchamps G., Shultz M. J., Williamson K. C. and Rapp D. N., (2013), Textbook treatments of electrostatic potential maps in general and organic chemistry, J. Chem. Educ., 90, 1275–1281.
- Höst G. E., Schönborn K. J. and Palmerius K. E. L., (2012), Students' Use of Three Different Visual Representations To Interpret Whether Molecules Are Polar or Nonpolar, J. Chem. Educ., 89, 1499–1505.
- Johnson C. I. and Mayer R. E., (2010), Applying the self-explanation principle to multimedia learning in a computer-based game-like environment, Comput. Hum. Behav., 26, 1246–1252.
- Johnstone A. H., (1991), Why is science difficult to learn? Things are seldom what they seem, J. Comput. Assist. Lear., 7, 75–83.
- Justi R., Gilbert J. K. and Ferreira P. F., (2009), in Gilbert J. K. and Treagust D. F. (ed.), Multiple Representations in Chemical Education, Berlin/Heidelberg: Springer, pp. 285–307.
- Kellman P. J. and Garrigan P. B., (2009), Perceptual learning and human expertise, Phys. Life Rev., 6, 53–84.
- Kellman P. J. and Massey C. M., (2013), Perceptual Learning, cognition, and expertise, Psychol. Learn. Motiv., 558, 117–165.
- Kellman P. J., Massey C. M. and Son J. Y., (2009), Topics in Cognitive Science, vol. 2, pp. 285–305.
- Koedinger K. R., Corbett A. T. and Perfetti C., (2012), The Knowledge-Learning-Instruction Framework: Bridging the Science-Practice Chasm to Enhance Robust Student Learning, Cognitive Sci., 36, 757–798.
- Kozma R. B. and Russell J., (1997), Multimedia and understanding: Expert and novice responses to different representations of chemical phenomena, J. Res. Sci. Teach., 34, 949–968.
- Kozma R. and Russell J., (2005a), in Mayer R. E. (ed.), The Cambridge handbook of multimedia learning, New York, NY: Cambridge University Press, pp. 409–428.
- Kozma R. and Russell J., (2005b), in Gilbert J. (ed.), Visualization in science education, Dordrecht, Netherlands: Springer, pp. 121–145.
- Kozma R., Chin E., Russell J. and Marx N., (2000), The roles of representations and tools in the chemistry laboratory and their implications for chemistry learning, J. Learn. Sci., 9, 105–143.
- Linenberger K. J. and Bretz S. L., (2012), Generating cognitive dissonance in student interviews through multiple representations, Chem. Educ. Res. Pract., 13, 172–178.
- Loudon M., (2009), Organic Chemistry, Roberts and Company Publishers.
- Luxford C. J., (2013), Use of multiple representations to explore students' understandings of covalent and ionic bonding as measured by the bonding representations inventory, Doctoral dissertation, Miami University.
- Luxford C. J., Crowder M. W. and Bretz S. L., (2011), A Symmetry POGIL Activity for inorganic chemistry, J. Chem. Educ., 89, 211–214.
- Massey C. M., Kellman P. J., Roth Z. and Burke T., (2011), in Stein N. L. and Raudenbush S. W. (ed.), Developmental cognitive science goes to school, New York, NY: Routledge, pp. 235–249.
- Matta C. F. and Gillespie R. J., (2002), Understanding and interpreting molecular electron density distributions, J. Chem. Educ., 79, 1141–1152.
- Michalchik V., Rosenquist A., Kozma R., Kreikemeier P. and Schank P., (2008), in Gilbert J. K., Reiner M. and Nakhleh M. B. (ed.), Visualization: theory and practice in science education, Dordrecht, Netherlands: Springer, pp. 233–282.
- Moore J. W., Stanitski C. L. and Jurs P. C., (2010), Chemistry: The Molecular Science, Cengage Learning.
- Moreira R. F., (2013), A Game for the early and rapid assimilation of organic nomenclature, J. Chem. Educ., 1035–1037.
- Peters M., Laeng B., Latham K., Jackson M., Zaiyouna R. and Richardson C., (1995), A Redrawn Vandenberg & Kuse Mental Rotations Test: Different Versions and Factors that affect Performance, Brain Cognition, 28, 39–58.
- Rau M. A., (under review), A Framework for Discipline-Specific Grounding of Educational Technologies with Multiple Visual Representations, IEEE Transactions on Learning Technologies, submitted.
- Rau M. A., Aleven V., Rummel N. and Pardos Z., (2014), How Should Intelligent Tutoring Systems Sequence Multiple Graphical Representations of Fractions? A Multi-Methods Study, Int. J. Artif. Intelligence Educ., 24, 125–161.
- Rau M. A., Michaelis J. E. and Fay N., (2015), Connection making between multiple graphical representations: a multi-methods approach for domain-specific grounding of an intelligent tutoring system for chemistry, Comput. Educ., 82, 460–485.
- Richman H. B., Gobet F., Staszewski J. J. and Simon H. A., (1996), in Ericsson K. A. (ed.), The road to excellence? The acquisition of expert performance in the arts and sciences, sports and games, Mahwah, NJ: Erlbaum Associatees, pp. 167–187.
- Ritter S., Anderson J. R., Koedinger K. R. and Corbett A. T., (2007), Cognitive tutor: applied research in mathematics education, Psychon. Bull. Rev., 14, 249–255.
- Schönborn K. J. and Anderson T. R., (2006), The importance of visual literacy in the education of biochemists, Biochem. Mol. Biol. Educ., 34, 94–102.
- Seufert T., (2003), Supporting Coherence Formation in Learning from Multiple Representations, Learn. Instr., 13, 227–237.
- Shanks D., (2005), in Lamberts K. and Goldstone R. (ed.), Handbook of Cognition, London: Sage, pp. 202–220.
- Shusterman G. P. and Shusterman A. J., (1997), Teaching chemistry with electron density models, J. Chem. Educ., 74, 771–776.
- Stieff M., (2005), Connected chemistry—a novel modeling environment for the chemistry classroom, J. Chem. Educ., 82, 489–493.
- Stieff M., (2007), Mental rotation and diagrammatic reasoning in science, Learn. Instr., 17, 219–234.
- Stieff M., (2013), Sex differences in the mental rotation of chemistry representations, J. Chem. Educ., 90, 165–170.
- Stieff M., Hegarty M. and Deslongchamps G., (2011), Identifying representational competence with multi-representational displays, Cognition and Instruct., 29, 123–145.
- Strickland A. M., Kraft A. and Bhattacharyya G., (2010), What happens when representations fail to represent? Graduate students' mental models of organic chemistry diagrams, Chem. Educ. Res. Pract., 11, 293–301.
- Taber K. S., (2009), in Gilbert J. K. and Treagust D. F. (ed.), Multiple Representations in Chemical Education, Berlin/Heidelberg: Springer, pp. 75–105.
- Taber K. S., (2013), Revisiting the chemistry triplet: drawing upon the nature of chemical knowledge and the psychology of learning to inform chemistry education, Chem. Educ. Res. Pract., 14, 156–168.
- Talanquer V., (2013), Chemistry education: ten facets to shape us, J. Res. Math. Educ., 90, 832–838.
- Uttal D. H. and O’Doherty K., (2008), in Gilbert J. (ed.), Visualization: Theory and Practice in Science Education, Netherlands: Springer, pp. 53–72.
- Uttal D. H., Meadow N. G., Tipton E., Hand L. L., Alden A. R., Warren C. and Newcombe N. S., (2013), The malleability of spatial skills: a meta-analysis of training studies, Psychol. Bull., 139, 352–402.
- van der Meij J. and de Jong T., (2006), Supporting students' learning with multiple representations in a dynamic simulation-based learning environment, Learn. Instr., 16, 199–212.
- Van der Meij J. and de Jong T., (2011), The effects of directive self-explanation prompts to support active processing of multiple representations in a simulation-based learning environment, J. Comput. Assist. Lear., 27, 411–423.
- VanLehn K., (2011), The relative effectiveness of human tutoring, intelligent tutoring systems and other tutoring systems, Educ. Psychol., 46, 197–221.
- Wai J., Lubinski D. and Benbow C. P., (2009), Spatial ability for STEM domains: aligning over 50 years of cumulative psychological knowledge solidifies its importance, J. Educ. Psychol., 101, 817–835.
- Wibraham A. C., (2005), Chemistry, Prentice Hall.
- Williamson V. M., (2014), in Devetak I. and Aleksij S. Dordrecht (ed.), Learning with Understanding in the Chemistry Classroom, Netherlands: Springer, pp. 193–208.
- Wu H. K. and Shah P., (2004), Exploring visuospatial thinking in chemistry learning, Sci. Educ., 88, 465–492.
- Wu H. K., Krajcik J. S. and Soloway E., (2001), Promoting understanding of chemical representations: students' use of a visualization tool in the classroom, J. Res. Sci. Teach., 38, 821–842.
|
This journal is © The Royal Society of Chemistry 2015 |
Click here to see how this site uses Cookies. View our privacy policy here.