DOI:
10.1039/C5RA20185C
(Paper)
RSC Adv., 2015,
5, 107020-107030
Calculation of protein–ligand binding affinities based on a fragment quantum mechanical method†
Received
29th September 2015
, Accepted 1st December 2015
First published on 3rd December 2015
Abstract
An electrostatically embedded generalized molecular fractionation with conjugate caps (EE-GMFCC) method (J. Phys. Chem. A, 2013, 117, 7149) has been successfully used for efficient linear-scaling quantum mechanical (QM) calculations of protein energies. Furthermore, an efficient approach that combined the EE-GMFCC method with a conductor-like polarizable continuum model (CPCM), termed as EE-GMFCC-CPCM (J. Chem. Phys., 2013, 139, 214104), was developed for ab initio calculations of the electrostatic solvation energy of proteins. In this study, we applied the EE-GMFCC-CPCM method for the calculation of binding affinities of 14 avidin–biotin analogues. The calculation delineated the contributions of interaction energy and electrostatic solvation energy to the binding affinity. The binding affinity of each ligand bound to avidin was calculated at the HF/6-31G* and B3LYP/6-31G* levels with empirical dispersion corrections, respectively. The correlation coefficient (R) between the calculated binding energies and experimental values is 0.75 at the HF/6-31G*-D level based on single complex structure calculations, as compared to 0.73 of the force field result. On the other hand, the correlation coefficient between the calculated binding energies and the experimental values is 0.85 at the B3LYP/6-31G*-D level based on single complex structure calculations, and this correlation can be further improved to 0.88 when multiple snapshots are considered. Our study demonstrates that the EE-GMFCC-CPCM method is capable of providing reliable predictions of binding affinities for various ligands bound to the same target.
1. Introduction
Accurate prediction of the binding affinity for a ligand bound to a macromolecule is of great interest in computational chemistry and biology, which also has broad applications in drug discovery and molecular design.1 Many efforts have been made in developing theoretical methods to estimate accurate binding affinity. Currently, most theoretical methods employed molecular mechanical (MM) force fields which are built on pairwise atomic interaction potentials.2–4 Despite the success of classical force field methods for calculation of interaction energies in biomolecules, they still have significant limitations. The standard nonpolarizable force fields lack of electronic polarization effect and thus do not include the many-body interaction, which plays a critical role in determining molecular interactions.5,6 Explicit inclusion of polarization effect has been shown to improve the accuracy of the force fields in estimating the binding affinities of protein–ligand complexes.7–9 However, the deviations from experimental results in well-converged free energy calculations have still been attributed to the imperfect force fields.10,11 Some semiempirical approaches, which exploit PM6 Hamiltonian, or density functional tight-binding method have also been developed and performed successfully in calculation of protein–ligand binding affinities.12–16
In recent years, quantum mechanical (QM) methods emerged as accurate and powerful approaches for prediction of protein–ligand binding affinities.11 Such methods determine the potential energy of the system at the ab initio level, which are intrinsically transferable and also systematically improvable.6 However, biological systems are in general too large for a full QM treatment. One popular approach of applying QM calculation on biological molecules is the hybrid QM/MM method,17 in which only a small subsystem is treated by QM and the rest atoms are described using MM. At present, the QM/MM calculations could provide a powerful means for theoretical study of biological systems. Another approach is applying full QM calculations based on the fragmentation method, in which the whole system is decomposed into small subsystems and the calculation of the large system is performed on each subsystem individually.18 Several fragmentation methods have been applied to protein–ligand interaction calculations, including the divide-and-conquer method,19–21 the fragment molecular orbital (FMO) method,22–24 the molecular fractionation with conjugate caps (MFCC) method,25–29 and related approaches.6,30–32
The MFCC method is a fragment-based approach developed in our group, and initially it was employed to calculate the protein–ligand interaction. Then the MFCC approach was extended to compute the total energy of proteins at diverse ab initio levels.33–37 In the following development, we proposed an efficient generalized molecular fractionation with conjugate caps/molecular mechanics (GMFCC/MM) scheme.38 Subsequently, in order to further improve the accuracy of the GMFCC/MM method, recently we developed the electrostatically embedded generalized molecular fractionation with conjugate caps (EE-GMFCC) method for accurate calculation of the protein energy.39 Numerical tests have shown that the EE-GMFCC method is computationally efficient and the protein energies calculated by EE-GMFCC are in excellent agreement with the full system QM calculations. The overall mean unsigned error (MUE) for 18 real three-dimensional proteins of up to 1142 atoms is only 2.39 kcal mol−1 with respect to the full system HF/6-31G* calculations.39
Rigorous representation of solvation is important for theoretical study of molecular properties in solution. Incorporating the fragmentation methods with solvation models made an important pace toward rigorous study of protein in realistic environment.40,41 The density-based polarized continuum model (PCM)42–45 is one of the most widely used solvation methods in QM calculation. In 2006, Mei et al. proposed a practical MFCC-CPCM method which combined the MFCC approach with the conductor-like PCM model46 to calculate the electrostatics solvation energy of proteins.40 More recently, a more accurate approach that combines the EE-GMFCC method with CPCM, termed EE-GMFCC-CPCM, is developed for ab initio calculation of the electrostatic solvation energy of proteins.47 As compared to standard full system CPCM calculations, EE-GMFCC-CPCM shows clear improvement over the MFCC-CPCM method for both the total electrostatic solvation energy and its components (the polarized solute–solvent reaction field energy and wavefunction distortion energy of the solute).
In this study, we calculated the binding affinities of protein–ligand complexes at two QM levels by using EE-GMFCC and EE-GMFCC-CPCM methods based on the thermodynamic cycle.48,49 To test the performance of our approach, we studied the affinities of the biotin, as well as 13 biotin analogues, bound to the avidin protein, whose experimental binding affinities are available.50–53 The binding affinities based on single snapshot approximation were obtained first. For better inclusion of the conformational sampling on the protein–ligand binding structures, the ensemble-averaged binding affinities over multiple snapshots obtained from molecular dynamics (MD) simulations were also calculated for comparison. We also compared the results calculated from different QM levels. Finally, the role of different free energy components in determining the protein–ligand binding affinity is discussed.
2. Theory and methodology
2.1. The EE-GMFCC method
Detailed description of the EE-GMFCC method can be found in ref. 39. Here, we just give a brief review. In the framework of the EE-GMFCC method, the protein with N residues is divided into N − 2 individual fragments by cutting through the peptide bond (excluding the first and the last peptide bonds) and a pair of conjugate caps is used to saturate each fragment. Hydrogen atoms are added to terminate the molecular caps to avoid dangling bonds (see Fig. 1a). Considering the importance of two-body interaction,54,55 rigorous treatment of the short-range non-neighboring interaction is necessary. In our EE-GMFCC scheme, two non-neighboring residues, whose minimal distance falls within a defined distance threshold λ (4.0 Å was used in this work based on our previous study39), are considered to be in close contact (defined as Gconcap) and their interaction is calculated at the QM level (see Fig. 1b). All of the fragment calculations are embedded in the electrostatic field of the point charges representing the remaining fragments of the protein. The total energy of protein can then be expressed by the following formulation |
 | (1) |
where Ẽ(Cap*i−1AiCapi+1) represents the summation of the self-energy of fragment (the ith residue Ai capped with a left cap Cap*i−1 and a right cap Capi+1) and the interaction energy between fragment and background charges. The self-energy of concap along with the interaction energy between concap and background charges Ẽ(Cap*iCapi+1) need to be deducted. The third term is the two-body interaction between two nonneighboring residues (i and j) in close contact (within the distance threshold λ). The doubly counted interaction between distant non-neighboring residues is deducted by charge–charge interactions approximately in the last term EDC. In our study, Amber94 (ref. 56) charge model is adopted for describing the embedding field. For the protein–ligand binding system, the total energy of the complex can be derived using the following formula, |
 | (2) |
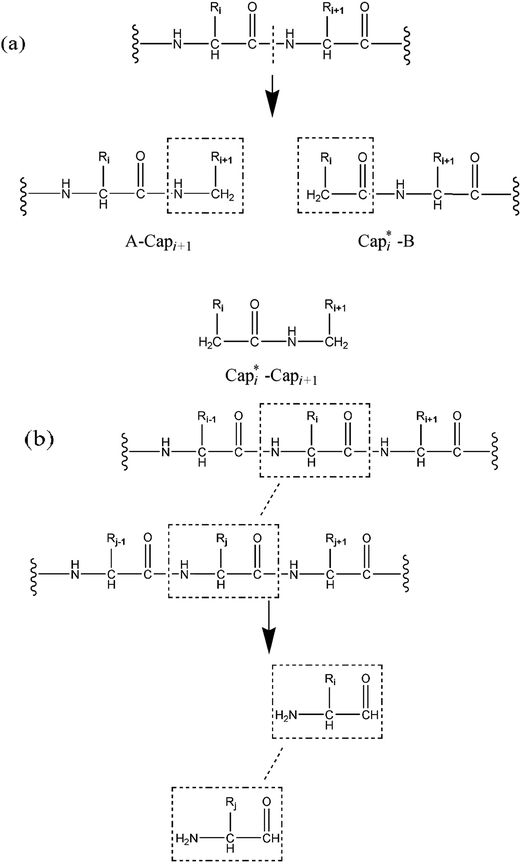 |
| Fig. 1 (a) EE-GMFCC scheme in which the peptide bond is cut and the fragments are capped with Capi+1 and its conjugate Cap*i, where subscript i denotes the ith amino acid in the given protein. The concap is defined as the fused molecular species of Cap*i − Capi+1. (b) The generalized concap (Gconcap) scheme and the atomic structure of the Gconcap. | |
Eqn (2) is similar to eqn (1), while two additional terms were added to include the contribution from the ligand. Ẽ(lig) represents the self-energy of ligand and the interaction energy between the ligand and its environment represented by the background charges. The two-body contribution, Ẽlig,i − Ẽlig − Ẽi, is also included to cover the quantum effect between the ligand and its nearby residues.
2.2. The EE-GMFCC-CPCM method
Ref. 47 provides detailed information of the EE-GMFCC-CPCM method. Here, a brief summary is given for clarification. Normally, the electrostatic solvation energy (G(ele)) is obtained by, |
G(ele) = E(wfd) + G(es)
| (3) |
where E(wfd) and G(es) represent the wave function distortion energy and the electrostatic solute–solvent interaction energy, respectively.
Based on the EE-GMFCC approach, the wave function distortion energy E(wfd) can be approximately obtained through
|
 | (4) |
where Δ
Ẽ represents the difference of the fragment energy in gas phase and solution phase, which consists of the self-energy of each fragment and the interaction energy between the fragment and its corresponding background charges. Owing to the fixed charge model used in this study, the doubly counted charge–charge interaction energy (the last term in
eqn (1) and
(2)) is exactly cancelled out between the gas phase and solution phase in the current EE-GMFCC-CPCM calculations. In addition,
NF,
NC and
NGC denote the number of capped fragments (including the single ligand), concaps and Gconcaps, respectively. On the other hand, the electrostatic solute–solvent interaction energy
G(es) is given by
|
 | (5) |
where
qμ and
rμ represent the induced surface charges and their positions, respectively, which are determined through the CPCM method.
46,57 Zα and
Rα are the nuclear charges and their corresponding coordinates,
ρ(
r) is the electron density at position
r, and
ϕ(
rμ) is the electrostatic potential on cavity surface site
μ generated by both the nuclei and the electrons of the solute, which can be derived from the EE-GMFCC approach,
|
 | (6) |
In the EE-GMFCC-CPCM approach, the QM calculation of each fragment is conducted in the presence of the surface charges. The newly obtained wavefunction is then employed to determine the surface charges of the CPCM equation.57,58 The procedure is repeated in a self-consistent fashion until the final electrostatic solute–solvent interaction energy and the surface charges reach convergence, as in the standard self-consistent reaction field (SCRF) calculation.46,57
2.3. Binding affinity calculation
In this study, the binding affinity (ΔGbind) between a receptor (P) and a ligand (L) in a complex form (PL) is formulated based on the thermodynamic cycle48 as shown in Fig. 2, |
ΔGbind = G(PL) − G(P) − G(L)
| (7) |
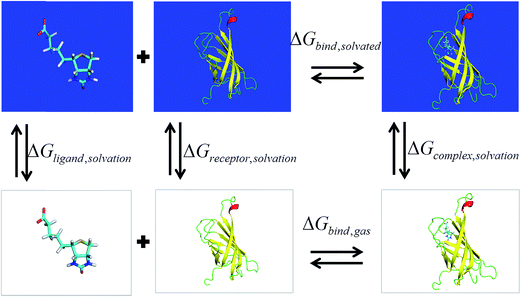 |
| Fig. 2 Thermodynamic cycle for binding free energy calculation of a receptor–ligand complex. Solvated systems are shown in blue boxes, while systems in the gas phase are in white boxes. | |
The free energy of each component is estimated as
|
ΔG = Δ(Egas + Gsolv + Gnp) − TΔS
| (8) |
where
Egas =
EEE-GMFCC +
Edispersion is the energy of the molecule in gas phase, which includes the molecular electronic energy calculated by the EE-GMFCC method and the dispersion correction energy obtained from the density functional dispersion correction (DFT-D3) method,
59 which can also be used to calculate the dispersion correction for HF;
Gsolv is the electrostatic solvation energy, computed by the EE-GMFCC-CPCM method;
Gnp is the non-electrostatic solvation energy contribution, which is calculated by the non-electrostatic term in the CPCM model, and
S is the conformational entropy. For the atomic radii, both
Gsolv and
Gnp calculations use united-atom topological model (UATM).
60 All QM calculations were carried out at the HF/6-31G* and B3LYP/6-31G* levels using the Gaussian 09 program.
61
2.4. System preparation
The avidin–biotin complex (Fig. 3) is one of the most remarkable protein–ligand binding systems in nature, with a strong binding affinity of around 20 kcal mol−1.62 Elucidating the binding mechanism of this classical system has motivated a variety of experimental and theoretical investigations.51,52 In this work, we computed the binding affinities of biotin and 13 biotin analogues (Fig. 4) bound to avidin. The initial coordinates of 14 ligand–avidin structures were obtained from Jia et al.'s study,53 which assessed the applicability of polarized protein-specific charges in linear interaction analysis by performing molecular dynamics (MD) simulations for these complexes. All complexes with biotin analogues were generated based on the avidin–biotin crystal structure (PDB ID: 1AVD). Hydrogen atoms were added to the protein using the Leap module in AMBER11 program.63 H++ software64 was utilized to determine the protonation states of titratable residues. The geometry of each ligand was optimized at the B3LYP/6-31G* level. Ligands were initially assigned RESP charge.65,66 Other force field parameters were taken from the general AMBER force field (GAFF).67 Partial charges of the protein were assigned with the AMBER03 force field.68
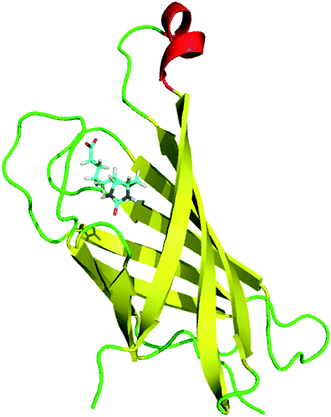 |
| Fig. 3 The X-ray crystal structure of avidin–biotin binding complex. The biotin is shown in sticks. | |
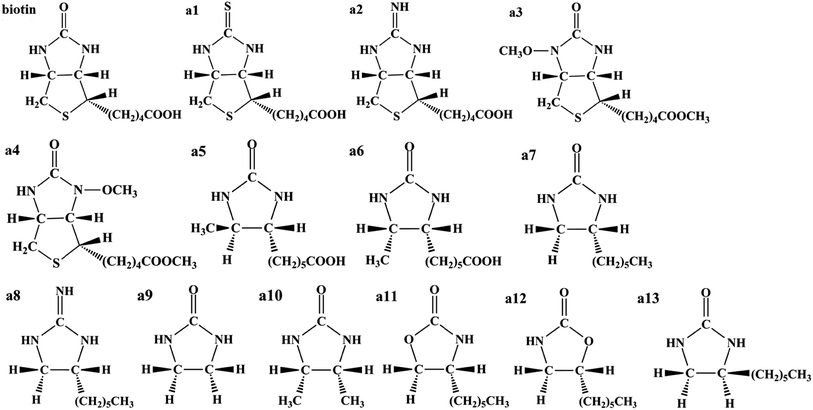 |
| Fig. 4 The structures of biotin and 13 biotin analogues used in this study. | |
2.5. MD simulation and MM/PBSA calculation
In MD simulations, each complex was soaked in a periodic truncated octahedral box of TIP3P water with 13 Å buffer. Counterions were added to neutralize the whole system. Two steps of minimizations were carried out to optimize the initial structure. In the first step, the protein was restrained and all other molecules were relaxed using steep descent method followed by conjugate gradient minimization. In the second step, the whole system was optimized. Then the system was gradually heated to 300 K in 1.2 ns, and the time step was 1 fs during heating. A 2 ns simulation in canonical ensemble was carried out to equilibrate the whole system at the target temperature, which was followed by an 8 ns production simulation in NPT ensemble with a 2 fs time step. Temperature was regulated by Langevin dynamics69 with a collision frequency of 1.0 ps−1. The pressure was regulated using Berendsen's barostat.70 SHAKE algorithm was applied to constrain the covalent bonds involving hydrogen atoms.71 Particle mesh Ewald72 with a cutoff of 9.0 Å in real space was utilized to calculate long range Coulomb interaction. van der Waals (VDW) interaction was truncated at 9.0 Å. Snapshots from the last 6 ns trajectory were evenly extracted for further analysis. All the MD simulations were carried out by AMBER11 program.63
Owing to the high computational cost for QM calculation on such large systems, the single-snapshot approach was firstly chosen for calculation of the binding affinity using the EE-GMFCC-CPCM method. The single-snapshot approximation has been proved to be useful in a previous work by Sandberg et al.73 In this study, the time-averaged binding affinities were first calculated through the MM/PBSA approach.48 Subsequently, the snapshot, whose binding affinity was closest to the time-averaged binding affinity, was selected in each complex. In MM/PBSA calculation, the conformational entropy was not included, because of the expensive computation and large fluctuation of this term. The value of the exterior dielectric constant was set to 80, and the solute dielectric constant was set to 1. The nonpolar solvation term in MM/PBSA is calculated from the solvent-accessible surface area (SASA):74 ΔGnp = γ × ΔSASA, where γ = 0.0072 kcal mol−1 Å−2. 300 snapshots evenly extracted from the last 6 ns trajectories were used for calculation. Finally, for each complex, the snapshot which gave the closest binding affinity to its corresponding time-averaged value from MM/PBSA was extracted for subsequent QM calculation. This protocol implicitly includes conformational sampling by matching the computed average value. To include the conformational sampling effect, two additional snapshots, whose calculated binding affinities in MM/PBSA are next closest to their corresponding time-averaged value, were extracted from MD trajectory in each binding complex. Therefore, the average binding affinity over three representative snapshots in each complex was obtained through the EE-GMFCC-CPCM approach.
3. Results and discussion
We computed the binding affinities of biotin and 13 biotin analogues bound to avidin using the EE-GMFCC-CPCM method at the HF/6-31G*-D and B3LYP/6-31G*-D levels, and compared to the experimental results. In 2010, Soderhjelm et al. reported the quantum chemical estimates of protein–ligand binding affinities,11 in which they calculated the binding of seven biotin analogues bound to avidin using the PMISP/MM (polarizable multiple interactions with supermolecular pairs) approach30 at the MP2/cc-pVTZ level, however they found that their method gave poor absolute affinities, which can be seen from the correlation coefficient between the experimental and calculated values (R2 = 0.27). In this study, we do not include the entropy because of the inaccuracy and expensive calculation. The absolute binding affinities calculated in this study are also away from the experimental values (see Tables 1–4). One reason causing the large deviation is that the entropic change upon ligand binding is not included in this study. Furthermore, the binding mechanism of biotin involves a mobile loop that is expected to be in an open conformation in the unbound avidin, and changed to a closed conformation upon ligand binding.75 The contribution of the loop's conformational change to the binding free energy, which is around 27.0 kcal mol−1 based on a previous study,76 is also not considered. Therefore, the deviations of absolute binding affinities from experiment are reasonable. The calculated binding affinities obtained from MM/PBSA approach are shown in Table 1, and the correlation coefficient R between the experimental and calculated values is 0.73, as shown in Fig. 5. Table 2 shows the results obtained at the HF/6-31G*-D level based on the single-snapshot approach (see Section 2.5). The correlation coefficient R between the experimental and calculated affinities is 0.75 (see Fig. 6). From Soderhjelm et al.'s calculation, the correlation R is also 0.75 for seven analogues, when the entropy is excluded. The results obtained at the B3LYP/6-31G*-D level based on the single protein–ligand structure are shown in Table 3 and Fig. 7, and the correlation coefficient R is 0.85. When three snapshots are considered, the correlation is further improved to 0.88 as shown in Table 4 and Fig. 7. Hence, we conclude that the QM method outperforms empirical approaches in predicting the protein–ligand binding affinity for these binding complexes.
Table 1 The calculated binding free energy decomposition (in kcal mol−1) using the MM/PBSA approach
Biotin & analogues |
ΔEgas |
ΔGsolv |
ΔGnp |
ΔEele + ΔGsolv |
ΔGcalc |
ΔGexp |
ΔEele |
ΔEvdw |
Biotin |
−153.78 |
−27.89 |
154.67 |
−4.24 |
0.89 |
−31.24 |
−20.40 |
a1 |
−159.35 |
−32.35 |
163.06 |
−4.37 |
3.71 |
−33.01 |
−16.90 |
a2 |
−177.00 |
−31.48 |
176.13 |
−4.32 |
−0.87 |
−36.68 |
−14.30 |
a3 |
−35.42 |
−40.58 |
47.65 |
−5.26 |
12.23 |
−33.61 |
−8.80 |
a4 |
−33.73 |
−38.45 |
49.16 |
−5.26 |
15.43 |
−28.28 |
−12.20 |
a5 |
−148.83 |
−29.02 |
140.76 |
−4.33 |
−8.06 |
−41.41 |
−16.50 |
a6 |
−156.18 |
−28.26 |
148.32 |
−4.26 |
−7.86 |
−40.38 |
−14.00 |
a7 |
−31.25 |
−27.00 |
36.64 |
−4.13 |
5.39 |
−25.74 |
−11.10 |
a8 |
−33.06 |
−28.60 |
42.20 |
−4.18 |
9.14 |
−23.64 |
−7.40 |
a9 |
−23.59 |
−11.38 |
21.64 |
−2.03 |
−1.95 |
−15.35 |
−4.50 |
a10 |
−26.77 |
−16.95 |
27.66 |
−2.82 |
0.89 |
−18.87 |
−6.40 |
a11 |
−18.88 |
−25.33 |
22.10 |
−4.16 |
3.23 |
−26.27 |
−5.00 |
a12 |
−28.58 |
−25.36 |
39.57 |
−4.05 |
11.00 |
−18.41 |
−7.40 |
a13 |
−30.81 |
−23.55 |
30.26 |
−4.04 |
−0.55 |
−28.13 |
−9.10 |
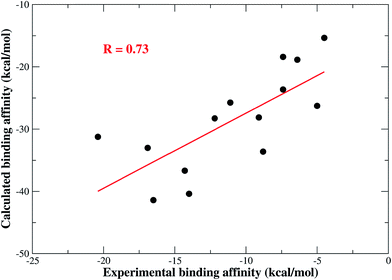 |
| Fig. 5 The correlation between the experimental and calculated binding affinities using the MM/PBSA approach. | |
Table 2 The calculated binding free energy decomposition (in kcal mol−1) based on the single protein–ligand structure at the HF/6-31G*-D level
Biotin & analogues |
ΔEgas |
ΔGsolv |
ΔGnp |
ΔEEE-GMFCC + ΔGsolv |
ΔGcalc |
ΔEEE-GMFCC |
ΔEdispersion |
Biotin |
−137.64 |
−74.80 |
150.76 |
26.13 |
13.12 |
−35.55 |
a1 |
−133.49 |
−80.05 |
160.99 |
24.56 |
27.50 |
−27.98 |
a2 |
−167.70 |
−77.57 |
174.83 |
25.84 |
7.13 |
−44.61 |
a3 |
−22.84 |
−79.76 |
75.36 |
29.38 |
52.52 |
2.14 |
a4 |
−14.81 |
−77.57 |
42.66 |
29.02 |
27.85 |
−20.69 |
a5 |
−143.14 |
−69.49 |
154.21 |
25.91 |
11.07 |
−32.51 |
a6 |
−149.47 |
−58.96 |
148.07 |
24.21 |
−1.40 |
−36.16 |
a7 |
−26.94 |
−52.79 |
35.70 |
21.75 |
8.76 |
−22.28 |
a8 |
−24.22 |
−56.26 |
45.39 |
26.75 |
21.17 |
−8.34 |
a9 |
−20.81 |
−24.76 |
22.74 |
11.47 |
1.93 |
−11.36 |
a10 |
−24.68 |
−36.64 |
25.08 |
15.23 |
0.40 |
−21.02 |
a11 |
−8.53 |
−45.97 |
21.74 |
23.89 |
13.21 |
−8.87 |
a12 |
−29.68 |
−43.19 |
42.04 |
23.51 |
12.36 |
−7.32 |
a13 |
−31.82 |
−45.13 |
26.17 |
23.04 |
−5.65 |
−27.74 |
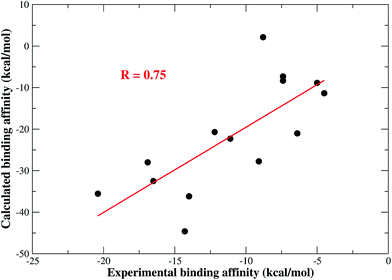 |
| Fig. 6 The correlation between the experimental and calculated binding affinities based on the single protein–ligand structure at the HF/6-31G*-D level. | |
Table 3 The calculated binding free energy decomposition (in kcal mol−1) based on the single protein–ligand structure at the B3LYP/6-31G*-D level
Biotin & analogues |
ΔEgas |
ΔGsolv |
ΔGnp |
ΔEEE-GMFCC + ΔGsolv |
ΔGcalc |
ΔEEE-GMFCC |
ΔEdispersion |
Biotin |
−161.98 |
−57.70 |
139.10 |
26.13 |
−22.88 |
−54.45 |
a1 |
−156.35 |
−62.77 |
144.79 |
24.56 |
−11.56 |
−49.77 |
a2 |
−191.01 |
−58.51 |
161.27 |
25.84 |
−29.74 |
−62.41 |
a3 |
−42.35 |
−65.52 |
41.83 |
29.38 |
−0.52 |
−36.66 |
a4 |
−36.53 |
−63.65 |
36.99 |
29.02 |
0.46 |
−34.17 |
a5 |
−166.34 |
−53.91 |
143.29 |
25.91 |
−23.05 |
−51.05 |
a6 |
−170.49 |
−53.36 |
135.69 |
24.21 |
−34.80 |
−63.95 |
a7 |
−40.67 |
−43.12 |
31.06 |
21.75 |
−9.61 |
−30.98 |
a8 |
−39.50 |
−44.82 |
40.66 |
26.75 |
1.16 |
−16.91 |
a9 |
−29.49 |
−19.08 |
19.93 |
11.47 |
−9.56 |
−17.17 |
a10 |
−36.35 |
−29.07 |
21.69 |
15.23 |
−14.66 |
−28.5 |
a11 |
−20.46 |
−38.22 |
18.46 |
23.89 |
−2.00 |
−16.33 |
a12 |
−39.37 |
−36.29 |
36.28 |
23.51 |
−3.09 |
−15.87 |
a13 |
−43.05 |
−37.16 |
22.60 |
23.04 |
−20.45 |
−34.57 |
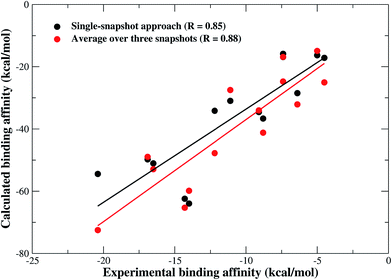 |
| Fig. 7 The correlation between the experimental and calculated binding affinities at the B3LYP/6-31G*-D level. | |
Table 4 The calculated binding free energy decomposition (in kcal mol−1) averaging over three snapshots at the B3LYP/6-31G*-D level
Biotin & analogues |
ΔEgas |
ΔGsolv |
ΔGnp |
ΔEEE-GMFCC + ΔGsolv |
ΔGcalc |
ΔEEE-GMFCC |
ΔEdispersion |
Biotin |
−182.88 |
−60.90 |
146.10 |
25.17 |
−36.78 |
−72.51 |
a1 |
−162.96 |
−60.37 |
148.66 |
25.72 |
−14.30 |
−48.95 |
a2 |
−190.24 |
−63.04 |
163.07 |
24.92 |
−27.17 |
−65.29 |
a3 |
−47.02 |
−66.33 |
41.38 |
30.76 |
−5.64 |
−41.21 |
a4 |
−47.80 |
−67.19 |
38.89 |
28.33 |
−8.91 |
−47.77 |
a5 |
−169.27 |
−51.81 |
140.82 |
27.33 |
−28.45 |
−52.93 |
a6 |
−166.48 |
−55.50 |
138.09 |
24.04 |
−28.39 |
−59.85 |
a7 |
−41.62 |
−41.48 |
32.08 |
23.55 |
−9.54 |
−27.47 |
a8 |
−39.62 |
−46.17 |
36.93 |
24.08 |
−2.69 |
−24.78 |
a9 |
−38.41 |
−20.90 |
22.87 |
11.38 |
−15.54 |
−25.06 |
a10 |
−42.77 |
−29.65 |
24.68 |
15.61 |
−18.09 |
−32.13 |
a11 |
−20.31 |
−38.63 |
20.11 |
23.90 |
−0.20 |
−14.93 |
a12 |
−36.77 |
−37.40 |
33.58 |
23.71 |
−3.19 |
−16.88 |
a13 |
−41.88 |
−45.37 |
29.74 |
23.49 |
−12.14 |
−34.02 |
3.1. Energy decomposition
Our present calculation provides decomposition of the binding affinity into contributions from the solute interaction energy ΔEgas, the polar and nonpolar solvation energy ΔGsolv and ΔGnp. In all cases, we observe favorable changes in the interaction energy, along with unfavorable changes in the polar and nonpolar solvation energy. For the interaction energy ΔEEE-GMFCC, which is calculated through our EE-GMFCC approach, it contributes a maximum of −167.70 kcal mol−1 for analogue a2 and a minimum of −8.53 kcal mol−1 for analogue a11 at the HF/6-31G*-D level (Table 2). It is worth noting that, this energy term differs significantly between the neutral and charged ligands. It is more negative for the charged ligands (biotin, a1, a2, a5, a6), which ranges from −133.49 to −167.70 kcal mol−1. However, it significantly decreases to −8.53 to −31.82 kcal mol−1 for the neutral ligands (a3, a4, a7–a13). The electrostatic solvation energy (ΔGsolv) is calculated using the EE-GMFCC-CPCM approach. As can be seen from the Tables 1–3, these positive polar solvation energies are closely correlated with the gas phase interaction energies, which cancel out the contribution of the gas phase interaction energy changes to some extent. For HF results in Table 2, the polar solvation energies are 148.07–174.83 kcal mol−1 for the charged ligands, which are more positive than those (21.74–75.36 kcal mol−1) for the neutral ligands. This shows a similar feature as PMISP/MM does. In Soderhjelm et al.'s study, he also pointed that the polar solvation energy obtained from PMISP/MM/PCM is always more positive for the charged ligands than for the neutral ones.11
The gas phase interaction energy is increased for each complex in B3LYP/6-31G*-D calculation as shown in Table 3. For charged ligands, the interaction energies (ΔEEE-GMFCC) are within −156.35 to −191.01 kcal mol−1, and the maximum energy is increased to −191.01 kcal mol−1 for a2 as compared to the corresponding HF calculation. ΔEEE-GMFCC given by B3LYP also becomes more negative for the neutral ligand than that from the HF calculation. The minimum ΔEEE-GMFCC is also decreased to −20.46 kcal mol−1 for a11. Compared to the more negative molecular electronic energy change, the polar solvation energy for each ligand does not become more positive. It is worth noting that, the polar solvation energy for each ligand from B3LYP is less positive than that from HF. The most positive polar solvation energy is 161.27 kcal mol−1 for a2 using B3LYP, whereas it is 174.83 kcal mol−1 for the same analogue using HF. The least positive one is 18.46 kcal mol−1 for a11 in B3LYP, whereas it is 21.74 kcal mol−1 for the same analogue in HF. For B3LYP, it gives more negative molecular electronic energy (ΔEEE-GMFCC), but with less positive polar solvation energy (ΔGsolv), which can explain the more favorable binding affinity obtained from B3LYP.
In the PCM model, the nonpolar solvation energy is calculated from three components: the energy cost of making a cavity in the solvent, the dispersion interactions between the solute and the solvent, and the exchange repulsion.60 We calculated the nonpolar solvation energy using the PCM model implemented in Gaussian 09 program.61 As can be seen from Tables 2 and 3, the nonpolar solvation energy is 11.47–29.38 kcal mol−1; it has positive sign and is larger in magnitude compared to the nonpolar solvation energy calculated by MM/PBSA. In MM/PBSA approach, the nonpolar solvation energy is estimated from the solvent-accessible surface area (SASA) of the molecule. The SASA energy estimate is −2.03 to −5.26 kcal mol−1, as shown in Table 1.
The DFT-D3 method has been widely used to accurately model the important London dispersion interactions for noncovalent binding. As shown in Tables 2 and 3, the dispersion interaction energy plays an important role in protein–ligand binding complexes. The dispersion interaction added to HF/6-31G* ranges from −24.76 to −80.05 kcal mol−1, while for dispersion correction of B3LYP/6-31G*, the range is from −19.08 to −65.52 kcal mol−1. Therefore, there is significant amount of dispersion energy in the protein–ligand binding complex, which cannot be well captured by traditional HF and B3LYP calculations.
3.2. Binding affinities averaging over multiple protein–ligand binding structures
Binding free energy predictions are usually made on large collections of equilibrated structures from MD simulation. The backbone root-mean-square deviations (RMSDs) during MD simulation for 14 binding complexes are shown in Fig. S1 of ESI.† To further validate the equilibrium, we extended MD simulations to 20 ns. From the plot of the backbone RMSDs, we can see that the equilibrium for each system was mostly reached after the first 3 ns simulation. Due to the expensive cost of QM calculations, only one single snapshot which gave the closest binding affinity to its corresponding time-averaged value in MM/PBSA was firstly extracted for each system. This single-snapshot approximation has implicitly included conformational sampling by matching the computed average value. For better including the effect of conformational sampling, another two representative snapshots were selected from the MD simulation for each system (see Section 2.5). The average binding affinities obtained from B3LYP/6-31G*-D level are shown in Table 4. Importantly, with three representative protein–ligand configurations, we achieved a better correlation (R = 0.88, see Fig. 7) between the experimental and calculated binding affinities, as compared to the single configuration result. Therefore, we conclude that the ensemble-averaged binding affinity over multiple snapshots generally gives more reliable result than that from single structure calculation.
3.3. Correlation analysis
The correlations between the experimental and calculated binding affinities have been obtained. Nevertheless, it is worth investigating what energy components play a more important role in predicting the protein–ligand binding affinity. Therefore, we tested how well a scoring function could perform if it used only one or two of these energy components. We first analyzed the energy components from the single structure results. We found that, the molecular electronic energy change (ΔEEE-GMFCC) correlates well with the experimental binding affinities, with the correlation coefficient of 0.84 for HF and 0.85 for B3LYP, respectively. The dispersion correction energy term (ΔEdispersion) does not correlate so well with the experimental binding affinities as the molecular electronic energy change does. The correlation of that obtained from HF is 0.75. In contrast, the correlation obtained from B3LYP is 0.72. However, the total interaction energy in gas phase (ΔEgas = ΔEEE-GMFCC + ΔEdispersion) correlates better with the experimental values. The correlation coefficients (R) are 0.89 for both HF and B3LYP results. The nonpolar solvation energy (ΔGnp) correlates poorly with the experimental binding affinities, with the correlation coefficient of −0.49. For the polar solvation energy (ΔGsolv), the correlation is good, with R = −0.86 for HF and R = −0.87 for B3LYP. The solvation energy (ΔGsolv + ΔGnp) also correlates fairly well with the experimental binding values. For both HF and B3LYP results, the correlation is around R = −0.88. Because the positive polar solvation energy is closely associated with the negative molecular electronic energy change, and they counteract with each other to some extent. We calculated the energy term of ΔEEE-GMFCC + ΔGsolv, and this energy term obtained from B3LYP is more negative than that from HF, as shown in Tables 2 and 3. Nevertheless, this energy term does not correlate well with the experimental binding values, with a correlation coefficient R = −0.08 for HF and R = 0.57 for B3LYP.
For the energy components averaged over three protein–ligand structures at the B3LYP/6-31G*-D level, they show a similar pattern to those from the single-snapshot results. The correlations of electronic energy change and dispersion correction energy term with the experimental binding affinities are 0.88 and 0.71, respectively. The interaction energy achieves a really good correlation with the experimental values as R = 0.91. For the polar and nonpolar solvation terms, the correlations are −0.88 and −0.50, respectively. In addition, the sum of these two terms (ΔGsolv + ΔGnp) achieves −0.89 for the correlation with respect to the experiment. For the energy term ΔEEE-GMFCC + ΔGsolv, the correlation with the experiment is 0.74, which is better than the corresponding single structure result (0.57). In summary, both the interaction energy and solvation energy play important roles in predicting the protein–ligand binding energy, and both of them are good indicators of binding affinity for these binding complexes.
4. Conclusion
In this study, we carried out full ab initio methods for accurate prediction of protein–ligand binding affinities. To this aim, we utilized our recently developed EE-GMFCC and EE-GMFCC-CPCM methods to calculate the interaction energy and electrostatic solvation energy, respectively. In our previous work, these two methods have been demonstrated to give accurate and reliable molecular electronic energy and electrostatic solvation energy compared to full system QM calculations. Herein, we tested our approaches at different QM levels. We also include the nonpolar solvation energy and empirical dispersion correction (for HF and DFT) in calculations of binding affinity. The nonpolar solvation energy is obtained through the PCM model, and the dispersion correction energy is obtained through the DFT-D3 method. These methods are combined to evaluate binding affinities based on the snapshots obtained from the empirical MM/PBSA approach.
Our approach has been tested for 14 avidin–biotin and biotin analogue complexes whose experimental binding affinities are available. The calculated binding affinities, obtained from the single snapshot and averaging over multiple snapshots, have been analyzed respectively. The result shows that the binding free energy averaged over three snapshots achieved a better correlation (R = 0.88 at the B3LYP/6-31G*-D level) with the experimental values than the result obtained from the single snapshot. In contrast, the correlation obtained from MM/PBSA is only 0.73, which shows the limitation of the empirical force field in describing the protein–ligand binding interactions. Our approach shows the capability of ab initio methods for accurate prediction of the protein–ligand binding affinities. QM calculations also demonstrate that both the interaction energy and solvation energy play important roles in capturing the protein–ligand binding. The accurate description of the interaction energy and solvation terms is of great importance in developing an effective scoring function.
Acknowledgements
This work was supported by the National Natural Science Foundation of China (Grants No. 21433004 and 21303057) and Shanghai Putuo District grant (2014-A-02). X. H. is also supported by the Specialized Research Fund for the Doctoral Program of Higher Education (Grant No. 20130076120019) and the Fundamental Research Funds for the Central Universities. We thank the Supercomputer Center of East China Normal University for providing us computational time.
References
- H. S. Muddana and M. K. Gilson, J. Chem. Theory Comput., 2012, 8, 2023–2033 CrossRef CAS PubMed.
- B. R. Brooks, R. E. Bruccoleri, B. D. Olafson, D. J. States, S. Swaminathan and M. Karplus, J. Comput. Chem., 1983, 4, 187–217 CrossRef CAS.
- D. A. Pearlman, D. A. Case, J. W. Caldwell, W. S. Ross, T. E. Cheatham, S. Debolt, D. Ferguson, G. Seibel and P. Kollman, Comput. Phys. Commun., 1995, 91, 1–41 CrossRef CAS.
- A. T. Hagler, E. Huler and S. Lifson, J. Am. Chem. Soc., 1974, 96, 5319–5327 CrossRef CAS PubMed.
- N. Huang, C. Kalyanaraman, K. Bernacki and M. P. Jacobson, Phys. Chem. Chem. Phys., 2006, 8, 5166–5177 RSC.
- P. Soderhjelm, F. Aquilante and U. Ryde, J. Phys. Chem. B, 2009, 113, 11085–11094 CrossRef PubMed.
- D. Jiao, P. A. Golubkov, T. A. Darden and P. Ren, Proc. Natl. Acad. Sci. U. S. A., 2008, 105, 6290–6295 CrossRef CAS PubMed.
- Y. Tong, Y. Mei, Y. L. Li, C. G. Ji and J. Z. H. Zhang, J. Am. Chem. Soc., 2010, 132, 5137–5142 CrossRef CAS PubMed.
- J. F. Liu, X. He and J. Z. H. Zhang, J. Chem. Inf. Model., 2013, 53, 1306–1314 CrossRef CAS PubMed.
- D. A. Pearlman and P. S. Charifson, J. Med. Chem., 2001, 44, 3417–3423 CrossRef CAS PubMed.
- P. Soderhjelm, J. Kongsted and U. Ryde, J. Chem. Theory Comput., 2010, 6, 1726–1737 CrossRef PubMed.
- J. Fanfrlik, A. K. Bronowska, J. Rezac, O. Prenosil, J. Konvalinka and P. Hobza, J. Phys. Chem. B, 2010, 114, 12666–12678 CrossRef CAS PubMed.
- P. S. Brahmkshatriya, P. Dobes, J. Fanfrlik, J. Rezac, K. Paruch, A. Bronowska, M. Lepsik and P. Hobza, Curr. Comput.-Aided Drug Des., 2013, 9, 118–129 CrossRef CAS.
- A. S. Christensen, M. Elstner and Q. Cui, J. Chem. Phys., 2015, 143 Search PubMed.
- K. E. Riley and P. Hobza, J. Phys. Chem. B, 2008, 112, 3157–3163 CrossRef CAS PubMed.
- C. G. Bresnahan, C. R. Reinhardt, T. G. Bartholow, J. P. Rumpel, M. North and S. Bhattacharyya, J. Phys. Chem. A, 2015, 119, 172–182 CrossRef CAS PubMed.
- H. M. Senn and W. Thiel, Angew. Chem., Int. Ed., 2009, 48, 1198–1229 CrossRef CAS PubMed.
- X. He, T. Zhu, X. W. Wang, J. F. Liu and J. Z. H. Zhang, Acc. Chem. Res., 2014, 47, 2748–2757 CrossRef CAS PubMed.
- K. Raha and K. M. Merz, J. Am. Chem. Soc., 2004, 126, 1020–1021 CrossRef CAS PubMed.
- W. T. Yang, Phys. Rev. Lett., 1991, 66, 1438–1441 CrossRef CAS PubMed.
- X. He and K. M. Merz, J. Chem. Theory Comput., 2010, 6, 405–411 CrossRef CAS PubMed.
- K. Kitaura, E. Ikeo, T. Asada, T. Nakano and M. Uebayasi, Chem. Phys. Lett., 1999, 313, 701–706 CrossRef CAS.
- K. Fukuzawa, Y. Mochizuki, S. Tanaka, K. Kitaura and T. Nakano, J. Phys. Chem. B, 2006, 110, 16102–16110 CrossRef CAS PubMed.
- D. G. Fedorov and K. Kitaura, J. Phys. Chem. A, 2007, 111, 6904–6914 CrossRef CAS PubMed.
- D. W. Zhang, Y. Xiang and J. Z. H. Zhang, J. Phys. Chem. B, 2003, 107, 12039–12041 CrossRef CAS PubMed.
- D. W. Zhang and J. Z. H. Zhang, J. Chem. Phys., 2003, 119, 3599–3605 CrossRef CAS.
- D. W. Zhang, Y. Xiang, A. M. Gao and J. Z. H. Zhang, J. Chem. Phys., 2004, 120, 1145–1148 CrossRef CAS.
- X. He, Y. Mei, Y. Xiang, D. W. Zhang and J. Z. H. Zhang, Proteins: Struct., Funct., Bioinf., 2005, 61, 423–432 CrossRef CAS PubMed.
- Y. Mei, X. He, Y. Xiang, D. W. Zhang and J. Z. H. Zhang, Proteins: Struct., Funct., Bioinf., 2005, 59, 489–495 CrossRef CAS.
- P. Soderhjelm and U. Ryde, J. Phys. Chem. A, 2009, 113, 617–627 CrossRef.
- R. P. A. Bettens and A. M. Lee, J. Phys. Chem. A, 2006, 110, 8777–8785 CrossRef CAS.
- R. P. A. Bettens and A. M. Lee, Chem. Phys. Lett., 2007, 449, 341–346 CrossRef CAS.
- X. H. Chen, Y. K. Zhang and J. Z. H. Zhang, J. Chem. Phys., 2005, 122, 184105 CrossRef PubMed.
- X. He and J. Z. H. Zhang, J. Chem. Phys., 2005, 122, 031103 CrossRef PubMed.
- A. M. Gao, D. W. Zhang, J. Z. H. Zhang and Y. K. Zhang, Chem. Phys. Lett., 2004, 394, 293–297 CrossRef CAS.
- X. H. Chen and J. Z. H. Zhang, J. Chem. Phys., 2006, 125, 044903 CrossRef CAS PubMed.
- Y. Mei, X. He, C. G. Ji, D. W. Zhang and Z. H. Z. John, Prog. Chem., 2012, 24, 1058–1064 CAS.
- X. He and J. Z. H. Zhang, J. Chem. Phys., 2006, 124, 184703 CrossRef PubMed.
- X. W. Wang, J. F. Liu, J. Z. H. Zhang and X. He, J. Phys. Chem. A, 2013, 117, 7149–7161 CrossRef CAS PubMed.
- Y. Mei, C. G. Ji and J. Z. H. Zhang, J. Chem. Phys., 2006, 125, 094906 CrossRef PubMed.
- C. G. Ji, Y. Mei and J. Z. H. Zhang, Biophys. J., 2008, 95, 1080–1088 Search PubMed.
- R. Cammi and J. Tomasi, J. Comput. Chem., 1995, 16, 1449–1458 CrossRef CAS.
- E. Cances, B. Mennucci and J. Tomasi, J. Chem. Phys., 1997, 107, 3032–3041 CrossRef CAS.
- G. Scalmani and M. J. Frisch, J. Chem. Phys., 2010, 132, 114110 CrossRef PubMed.
- A. W. Lange and J. M. Herbert, J. Phys. Chem. Lett., 2010, 1, 556–561 CrossRef CAS.
- V. Barone and M. Cossi, J. Phys. Chem. A, 1998, 102, 1995–2001 CrossRef CAS.
- X. Y. Jia, X. W. Wang, J. F. Liu, J. Z. H. Zhang, Y. Mei and X. He, J. Chem. Phys., 2013, 139, 214104 CrossRef PubMed.
- P. A. Kollman, I. Massova, C. Reyes, B. Kuhn, S. H. Huo, L. Chong, M. Lee, T. Lee, Y. Duan, W. Wang, O. Donini, P. Cieplak, J. Srinivasan, D. A. Case and T. E. Cheatham, Acc. Chem. Res., 2000, 33, 889–897 CrossRef CAS PubMed.
- J. M. Wang, T. J. Hou and X. J. Xu, Curr. Comput.-Aided Drug Des., 2006, 2, 287–306 CrossRef CAS.
- O. Livnah, E. A. Bayer, M. Wilchek and J. L. Sussman, Proc. Natl. Acad. Sci. U. S. A., 1993, 90, 5076–5080 CrossRef CAS.
- B. Kuhn and P. A. Kollman, J. Med. Chem., 2000, 43, 3786–3791 CrossRef CAS PubMed.
- B. Kuhn and P. A. Kollman, J. Am. Chem. Soc., 2000, 122, 3909–3916 CrossRef CAS.
- X. Y. Jia, J. Zeng, J. Z. H. Zhang and Y. Mei, J. Comput. Chem., 2014, 35, 737–747 CrossRef CAS PubMed.
- N. J. Mayhall and K. Raghavachari, J. Chem. Theory Comput., 2012, 8, 2669–2675 CrossRef CAS PubMed.
- H. A. Le, H. J. Tan, J. F. Ouyang and R. P. A. Bettens, J. Chem. Theory Comput., 2012, 8, 469–478 CrossRef CAS PubMed.
- W. D. Cornell, P. Cieplak, C. I. Bayly, I. R. Gould, K. M. Merz, D. M. Ferguson, D. C. Spellmeyer, T. Fox, J. W. Caldwell and P. A. Kollman, J. Am. Chem. Soc., 1996, 118, 2309 CrossRef CAS.
- M. Cossi, N. Rega, G. Scalmani and V. Barone, J. Comput. Chem., 2003, 24, 669–681 CrossRef CAS PubMed.
- H. Li, C. S. Pomelli and J. H. Jensen, Theor. Chem. Acc., 2003, 109, 71–84 CrossRef CAS.
- S. Grimme, J. Antony, S. Ehrlich and H. Krieg, J. Chem. Phys., 2010, 132, 154104 CrossRef PubMed.
- V. Barone, M. Cossi and J. Tomasi, J. Chem. Phys., 1997, 107, 3210–3221 CrossRef CAS.
- M. J. Frisch, G. W. Trucks, H. B. Schlegel, G. E. Scuseria, M. A. Robb, J. R. Cheeseman, G. Scalmani, V. Barone, B. Mennucci, G. A. Petersson, H. Nakatsuji, M. Caricato, X. Li, H. P. Hratchian, A. F. Izmaylov, J. Bloino, G. Zheng, J. L. Sonnenberg, M. Hada, M. Ehara, K. Toyota, R. Fukuda, J. Hasegawa, M. Ishida, T. Nakajima, Y. Honda, O. Kitao, H. Nakai, T. Vreven, J. J. A. Montgomery, J. E. Peralta, F. Ogliaro, M. Bearpark, J. J. Heyd, E. Brothers, K. N. Kudin, V. N. Staroverov, T. Keith, R. Kobayashi, J. Normand, K. Raghavachari, A. Rendell, J. C. Burant, S. S. Iyengar, J. Tomasi, M. Cossi, N. Rega, J. M. Millam, M. Klene, J. E. Knox, J. B. Cross, V. Bakken, C. Adamo, J. Jaramillo, R. Gomperts, R. E. Stratmann, O. Yazyev, A. J. Austin, R. Cammi, C. Pomelli, J. W. Ochterski, R. L. Martin, K. Morokuma, V. G. Zakrzewski, G. A. Voth, P. Salvador, J. J. Dannenberg, S. Dapprich, A. D. Daniels, O. Farkas, J. B. Foresman, J. V. Ortiz, J. Cioslowski and D. J. Fox, Gaussian, Inc., Wallingford CT, 2010.
- I. J. General, R. Dragomirova and H. Meirovitch, J. Phys. Chem. B, 2012, 116, 6628–6636 CrossRef CAS PubMed.
- D. A. Case, T. E. Cheatham, T. Darden, H. Gohlke, R. Luo, K. M. Merz, A. Onufriev, C. Simmerling, B. Wang and R. J. Woods, J. Comput. Chem., 2005, 26, 1668–1688 CrossRef CAS PubMed.
- R. Anandakrishnan, B. Aguilar and A. V. Onufriev, Nucleic Acids Res., 2012, 40, W537–W541 CrossRef CAS PubMed.
- C. I. Bayly, P. Cieplak, W. D. Cornell and P. A. Kollman, J. Phys. Chem., 1993, 97, 10269–10280 CrossRef CAS.
- P. Cieplak, W. D. Cornell, C. Bayly and P. A. Kollman, J. Comput. Chem., 1995, 16, 1357–1377 CrossRef CAS.
- J. M. Wang, R. M. Wolf, J. W. Caldwell, P. A. Kollman and D. A. Case, J. Comput. Chem., 2004, 25, 1157–1174 CrossRef CAS PubMed.
- V. Hornak, R. Abel, A. Okur, B. Strockbine, A. Roitberg and C. Simmerling, Proteins: Struct., Funct., Bioinf., 2006, 65, 712–725 CrossRef CAS PubMed.
- R. W. Pastor, B. R. Brooks and A. Szabo, Mol. Phys., 1988, 65, 1409–1419 CrossRef.
- H. J. C. Berendsen, J. P. M. Postma, W. F. van Gunsteren, A. DiNola and J. R. Haak, J. Chem. Phys., 1984, 81, 3684–3690 CrossRef CAS.
- J. P. Ryckaert, G. Ciccotti and H. J. C. Berendsen, J. Comput. Phys., 1977, 23, 327–341 CrossRef CAS.
- T. Darden, D. York and L. Pedersen, J. Chem. Phys., 1993, 98, 10089–10092 CrossRef CAS.
- D. J. Sandberg, A. N. Rudnitskaya and J. A. Gascon, J. Chem. Theory Comput., 2012, 8, 2817–2823 CrossRef CAS PubMed.
- J. Weiser, P. S. Shenkin and W. C. Still, J. Comput. Chem., 1999, 20, 217–230 CrossRef CAS.
- I. J. General, R. Dragomirova and H. Meirovitch, J. Phys. Chem. B, 2011, 115, 168–175 CrossRef CAS PubMed.
- I. J. General and H. Meirovitch, J. Chem. Phys., 2011, 134, 025104 CrossRef PubMed.
Footnote |
† Electronic supplementary information (ESI) available. See DOI: 10.1039/c5ra20185c |
|
This journal is © The Royal Society of Chemistry 2015 |
Click here to see how this site uses Cookies. View our privacy policy here.