DOI:
10.1039/C5RA10660E
(Paper)
RSC Adv., 2015,
5, 66947-66955
A value-added use of volatile turpentine: antifungal activity and QSAR study of β-pinene derivatives against three agricultural fungi†
Received
5th June 2015
, Accepted 24th July 2015
First published on 24th July 2015
Abstract
In consideration of turpentine and its analogues possessing some agricultural biological activity, persistent efforts to take advantage of renewable, abundant natural resources have been made. Three series of derivatives from β-pinene were synthesized and their fungicidal activities against Rhizoctonia solani, Fusarium graminearum, and Botrytis cinerea were investigated. Most of the synthesized compounds exhibited moderate to significant fungicidal activity. Among them, the acylthiourea derivatives from β-pinene showed more promising results than the other compounds. It was worth noting that compounds 7b and 7d displayed excellent fungicidal activity against Rhizoctonia solani, with IC50 values of 2.439 and 1.857 μg mL−1, which was close to or even better than the control triadimenol (1.945 μg mL−1, a commercial fungicide). The structure–activity relationship (SAR) analysis indicated that the compounds with more net positive charge possessed better fungicidal activity. The quantitative structure–activity relationship (QSAR) model (R2 = 0.9879, F = 348.41, S2 = 0.0047) was obtained through the best multi-linear regression. The built model revealed a strong correlation of fungicidal activity against Rhizoctonia solani with the molecular features of the title compounds. Additionally, the SAR and QSAR studies showed that the introduction of an electron-withdrawing group, which can increase the positive charge, was favorable towards the fungicidal activity. These encouraging results may provide an alternative, promising use of β-pinene through the design and exploration of eco-friendly fungicides with low toxicity and high efficiency.
1. Introduction
In the development of modern crop production, ensuring both food safety and disease prevention is a key issue. In traditional agriculture, chemical fungicides were used to protect crops from plant diseases. However, the abuse of a single synthesized fungicide has led to the development of resistance as well as increased side effects to the environment and harmless creatures.1–5 This is also one of the main reasons why efficient and eco-friendly fungicides need to be explored.
As a remarkable alternative means to classic agrochemicals, botanical fungicides have played an increasingly important role in integrated and ecological disease management.6 During the long-standing interaction between plants and the environment, some secondary metabolites have been produced, which endow with plants the ability to withstand adversity. Namely, plant secondary metabolites can protect plants from attacks by pests and diseases. Currently, a range of secondary metabolites from natural sources, such as avonoids, alkaloids and terpenoids, have been developed as the lead compounds for the preparation of potent fungicides of less or slower resistance and lower pollution.7,8
Turpentine is a renewable and abundant natural resource commodity in China. As a secondary metabolite secreted from some species of pine, turpentine has been proposed to have potential activity.9 As shown in Scheme 1, β-pinene is an important component of turpentine. Based on this promising reality, β-pinene can be used as the lead active material in some fields, such as a natural resource in the pharmaceutical industry, insecticides and fungicides in crop protection, repellents in health care, a polyterpine-resin in polymer materials, gum arabic food, and a solvent in paint and varnish.10,11 β-Pinene analogues have been reported to have a broad spectrum of bioactivity, demonstrating antibacterial,12–15 antifeedant,16–23 repellent,24–27 and antifungal properties.28 Among these analogues, cumic acid is a transformant of β-pinene in plants and it has good antimicrobial activity.29 Encouragingly, dehydrocumic acid, which has almost the same molecular structure as cumic acid, can be chemically synthesized from β-pinene.30 The development of an eco-friendly fungicide of high efficiency and selectivity from dehydrocumic acid was expected.
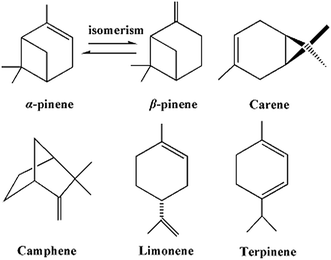 |
| Scheme 1 Chemical structures of the major constituents of turpentine. | |
In order to obtain novel natural product-based fungicides, three series of derivatives from β-pinene were synthesized on the basis of their molecular similarity. The fungicidal activities of the title compounds against three important agricultural pathogens Rhizoctonia solani (R. solani), Fusarium graminearum (F. graminearum) and Botrytis cinerea (B. cinerea) were investigated. Moreover, a QSAR study was also performed on all of the title compounds using the Gaussian and CODESSA software packages, which can account for the structural features responsible for the fungicidal activity. This exploration was expected to improve the added value of β-pinene as botanical fungicides in organic agriculture.
2. Experimental section
2.1. Synthetic procedures and identification
β-Pinene (1) was obtained from a commercial source (Wu Zhou Pine Chemicals Ltd, Guangxi, China) and used without further purification. All other chemicals used were of reagent grade. The Fourier transform infrared (FT-IR) spectra of the dried compounds were measured on a Nicolet IS10 (Nicolet, Madison, USA) spectrophotometer, using a KBr pellet technique. The 1H NMR spectra were recorded on a Bruker AV-300 (Bruker, Karlsruhe, Germany) nuclear magnetic resonance spectrometer with CDCl3 or DMSO-d6 as the solvent and TMS as an internal standard. The MS spectra were taken on an Agilent-5973 (Agilent, Santa Clara, USA) spectrophotometer. ESI mass spectral data were obtained on a Bruker Q-TOF mass spectrometer, equipped with an electrospray ionization source, operated in positive ion mode. The elemental analysis (C, H, and N) was carried out on a Vario EL-III (Elementar, Hanau, Germany) elemental analyzer and the results were in good agreement with the calculated values. X-ray intensity data were collected on a Bruker/Enraf-Nonius CAD-4 (Bruker, Karlsruhe, Germany) diffractometer. Melting points were taken on an XT-5 (Saiao, Beijing, China) apparatus and uncorrected. All reactions were traced by thin-layer chromatography (TLC) analysis, which was performed using Merck silica gel 60 GF254 plates, visualized under 254 nm UV light and eluted with a gradient of petroleum ether/acetone (v/v = 8
:
1). The preparation of the title compounds is shown in Scheme 2, and the substituted groups of the β-pinene derivatives are shown in Table 1. The pathogenic fungi were provided by the Research & Development Center of Biorational Pesticide, Northwest A & F University.
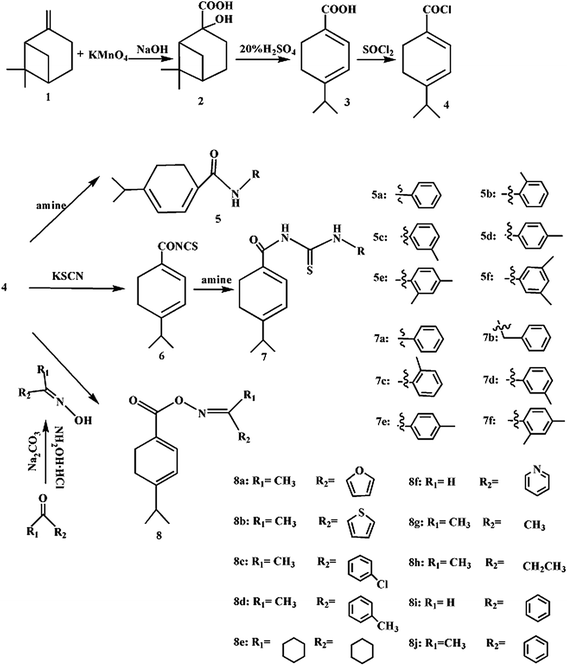 |
| Scheme 2 Preparation of the title compounds. | |
Table 1 The substituted groups of the β-pinene derivatives
2.1.1. Synthesis of 2-hydroxy-6,6-dimethylbicyclo[3.1.1]heptane-2-carboxylic acid (2). β-Pinene was oxidized by basic potassium permanganate in the homogeneous solution of water/tert-butanol (v/v = 1
:
2). KMnO4 (12.0 g, 0.076 mol) and NaOH (1.5 g, 0.038 mol) were dissolved in the mixture of water (100 mL) and t-BuOH (50 mL). While stirring vigorously, β-pinene (5.2 g, 0.038 mol) was added. The reaction was maintained at 15–25 °C for 0.5 h, and then was heated to 80 °C. After hot filtration, cooling, acidification, and vacuum drying, the crude compound was precipitated.31 The target chemical 2 was obtained by recrystallization with ethanol/toluene to give colorless crystals (0.30 mm × 0.20 mm × 0.10 mm in size) suitable for X-ray single crystal diffraction. The crystal structure was solved by direct methods and refined on F2 using all data by full-matrix least squares procedures with SHELXS 97.
2.1.2. Synthesis of 4-isopropylcyclohexa-1,3-dienecarboxylic acid (3). A solution of 40 g concentrated sulfuric acid in 100 mL water was added dropwise into a solution of compound 2 (10.0 g, 0.054 mol) at room temperature. Then, the reaction was heated to 75 °C. After reacting for 6 h, the mixture was recrystallized with water/ethanol (v/v = 1
:
1) to give a white flaky solid 3.
2.1.3. Synthesis of 4-isopropylcyclohexa-1,3-dienecarbonyl chloride (4). In a 250 mL flask with a water-cooled condenser, thermometer, drying tube and dropping funnel, compound 3 (5.0 g, 0.027 mol) was dissolved in CH2Cl2 (50 mL). Thionyl chloride (0.082 mmol) was added dropwise within 1 h and refluxed for 4 h at 65 °C. The chloride (4) was obtained after removing the solvent and excess thionyl chloride.
2.1.4. Synthesis of 4-isopropylcyclohexa-1,3-dienecarboxamides (5a–f). A solution of the above chloride (4) in 10 mL of CH2Cl2 was added dropwise to a solution of 40 mmol of amine and 40 mmol of triethylamine in 40 mL of CH2Cl2 within 30 minutes at room temperature. After reacting for 12 h, the residue was dried with anhydrous MgSO4 and purified by ethanol crystallization to give the six resulting compounds 5a–f.13
2.1.5. Synthesis of 4-isopropylcyclohexa-1,3-dienecarboxthioureas (7a–f). A solution of KSCN (3.0 g, 0.03 mol) and anhydrous 100 mL CH2CN was added to a 250 mL flask equipped with a water-cooled condenser, thermometer, drying tube, and dropping funnel. After reacting for 24 h at 70 °C, the intermediate compound 6 was collected by filtration and used without further purification. Then the arylamine (0.03 mol) was added and the resulting mixture was refluxed for 2 h. The six resulting compounds 7a–f were obtained after drying with anhydrous MgSO4 and purification by silica gel chromatography [ethyl acetate/petroleum ether (v/v = 1
:
10)].32
2.1.6. Synthesis of oximyl 4-isopropylcyclohexa-1,3-dienecarboxylates (8a–j). A solution of dihydrocumyl chloride in 50 mL of CH2Cl2 was added dropwise to a solution of 0.030 mol of oxime and 0.040 mol of triethylamine in 40 mL of CH2Cl2 within 30 minutes at 15–25 °C. After that, the reaction mixture was kept at room temperature for 2 h and washed with distilled water. The residue was dried with anhydrous MgSO4 and purified by silica gel chromatography [ethyl acetate/petroleum ether (v/v = 1
:
10)] to give the ten resulting compounds 8a–j.30
2.2. Biological assay
The fungicidal effect was determined by the inhibition of mycelia radial growth. The test chemicals were dissolved in DMSO at various concentrations (2560 μg mL−1, 1280 μg mL−1, 640 μg mL−1, 320 μg mL−1, 160 μg mL−1). The solutions were diluted to one tenth with Potato-Dextrose Agar (PDA) at 50 °C to give the required drug-containing medium at a series of concentrations (256 μg mL−1, 128 μg mL−1, 64 μg mL−1, 32 μg mL−1, 16 μg mL−1). The evaluations were based on a percentage scale of 0–100, where 0 = no activity and 100 = complete eradication. The standard deviations of the tested biological values were ±5%. The IC50 values were calculated by probit analysis. For comparative purposes, the commercial product triadimenol was tested under the same conditions.
Petri dishes containing 10 mL of the drug-containing agar medium were inoculated by placing 6 mm fungus-coated discs upside down on the agar surface. The Petri dishes containing 10 mL DMSO were set as the negative control. The plates were incubated at 30 °C and the mycelia radial growth was measured after three days. The inhibition rate (%) was calculated by (Tzone − Czone)/Tzone × 100, where Tzone was the inhibition zone of the test compounds, and Czone was the inhibition zone of the negative control. The IC50 values of the compounds were calculated with the SPSS Statistic program version 17.0.33
2.3. Building and validation of the QSAR model
The general procedure of QSAR model building and validation is shown in Fig. 1. Firstly, the optimal conformers of the title compounds with the lowest energy were computed at the DFT/6-31G (d) level using the Gaussian 03W package of programs.34 Secondly, the calculated results were changed into a form compatible with CODESSA 2.7.15 using Ampac 9.1.3. Thirdly, all of the molecular descriptors involved in these compounds were calculated by CODESSA 2.7.15. In order to find out which structural features play an important role in the fungicidal activity against R. solani, the best multiple regression analysis was selected to generate the QSAR model. In this model, the statistical criteria were indicated by the squared correction coefficient (R2), the squared standard error of the estimates (S2), and the Fisher significance ratio (F). The tested IC50 values were converted into the corresponding log
IC50 values and used as dependent variables in the QSAR studies. The quality of the final model was determined using both an internal validation and the “leave-one-out” cross-validation methods.
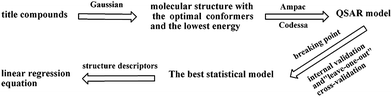 |
| Fig. 1 The general procedure of QSAR model building and validation. | |
3. Results and discussion
3.1. Synthesis
A polymerization reaction easily took place due to an inappropriate temperature, time, and concentration of H2SO4 in the synthesis of compound 3. During the reaction process, too high a temperature led to the appearance of a yellow and globular material; too long a reaction time led to the dark product. The white flake solid (compound 3) was obtained with the optimum reaction conditions: 80 °C, 20% H2SO4, and 6 h. The infrared spectrum data showed that the absorption peak of the hydroxyl (3100 cm−1) disappeared, and a carbon–carbon double bond (1572 cm−1) appeared.
The oil-soluble acyl chloride and water-soluble KSCN could not react homogeneously. The anhydrous CH2CN was chosen to make a homogeneous reaction. The intermediate isothiocyanate needed not to be purified and was used directly in the synthesis of acylthiourea derivatives from β-pinene (7a–f).
The preparation of the oximes played an important role in the synthesis of the title compounds 8a–j. According to the reported literature, the yield and compatibility of the reaction were affected by the solvent and base.35 In the present work, ethanol and sodium carbonate were selected as a suitable solvent and base in preparing the compounds 8a–j.
3.2. Crystal structure analysis
The target chemical 2 was obtained by recrystallization with ethanol/toluene to give colorless crystals suitable for X-ray single crystal diffraction, and the XRD peaks were observed for values of 2θ in the range of 10–13°, θmax = 25.3°, θmin = 1.6°. The crystallographic parameters were shown as: a = 26.796 (5) Å, b = 6.6560 (13) Å, c = 12.250 (3) Å, β = 112.23 (3)°, V = 2022.5 (9) Å3, Z = 8, μ = 0.009 mm−1, T = 293 K, R[F2 > 2σ(F2)] = 0.056, wR(F2) = 0.153, S = 1.00, λ = 0.71073 Å, F000 = 800.
3.3. Fungicidal activity and structure–activity relationships (SAR)
3.3.1. Fungicidal activity of the title compounds against R. solani. The results of the fungicidal activity against R. solani are summarized in Table 2, from which it can be seen that the title compounds exhibited certain fungicidal activity against R. solani. The introduction of the acyl thiourea group increased the fungicidal activity against R. solani significantly. Compounds 7a–f exhibited about a 90% inhibition rate against R. solani at 64 μg mL−1. It was notable that the IC50 values of 7b and 7d were similar to or lower than triadimenol, a commercialized fungicide. Compared with compound 3, all the derivatives had a more significant fungicidal activity. There were several postulations about the mechanisms elucidating the antimicrobial activity.36 The most acceptable mechanism is the interaction between the positively charged molecules and the negatively charged microbial cell membrane. The compounds having protonated groups can generate electrostatic forces with the electrostatic negative charges on the microbial cell surfaces.37 Based on this electrostatic interaction mechanism, the analogues with more positive charge would possess a higher antimicrobial activity. The introduction of amide, acyl thiourea, and oxime groups into compound 3 increased its cationic centers and its solubility in organic and aqueous media, which strengthened the net positive charge and led to a better fungicidal activity. With regards to the different kinds of derivatives, the order of the fungicidal activity level was acyl thiourea (7a–f) > oxime ester (8a–j) > amide (5a–f), which complied with the above mechanism; for the same kind of derivatives, the activity results also met the mechanism well. Taking the oxime derivatives from β-pinene as an example, compounds 8c, 8i, 8j, and 8d with electron-withdrawing groups have better activity than other compounds with electron-donating groups.
Table 2 Fungicidal activity of compounds against R. solani
No. |
Compd |
Fungicidal activity (%) at a concentration of (μg mL−1) |
IC50 |
y = ax + b |
R2 |
log IC50 |
256 |
128 |
64 |
32 |
16 |
1 |
3 |
100 |
67 |
40 |
20 |
0 |
92.712 |
y = 0.236x − 2.722 |
0.977 |
1.967 |
2 |
5a |
100 |
70 |
53 |
31 |
24 |
69.777 |
y = 0.175x − 1.445 |
0.952 |
1.844 |
3 |
5b |
100 |
70 |
50 |
30 |
23 |
72.485 |
y = 0.177x − 1.528 |
0.971 |
1.860 |
4 |
5c |
100 |
72 |
53 |
30 |
25 |
68.481 |
y = 0.177x − 1.456 |
0.964 |
1.836 |
5 |
5d |
100 |
70 |
50 |
30 |
23 |
72.485 |
y = 0.177x − 1.528 |
0.971 |
1.860 |
6 |
5e |
100 |
70 |
48 |
30 |
24 |
73.144 |
y = 0.177x − 1.525 |
0.986 |
1.864 |
7 |
5f |
100 |
72 |
54 |
33 |
25 |
66.345 |
y = 0.174x − 1.382 |
0.961 |
1.822 |
8 |
7a |
100 |
98 |
89 |
76 |
58 |
3.017 |
y = 0.235x − 0.102 |
0.990 |
0.480 |
9 |
7b |
100 |
98 |
89 |
77 |
58 |
2.439 |
y = 0.236x − 0.083 |
0.987 |
0.387 |
10 |
7c |
100 |
97 |
87 |
73 |
57 |
3.284 |
y = 0.221x − 0.099 |
0.990 |
0.516 |
11 |
7d |
100 |
98 |
89 |
78 |
58 |
1.857 |
y = 0.237x − 0.063 |
0.983 |
0.269 |
12 |
7e |
100 |
97 |
87 |
73 |
57 |
3.284 |
y = 0.221x − 0.099 |
0.990 |
0.516 |
13 |
7f |
100 |
97 |
86 |
72 |
57 |
3.770 |
y = 0.218x − 0.112 |
0.994 |
0.576 |
14 |
8a |
100 |
95 |
75 |
53 |
33 |
32.604 |
y = 0.206x − 1.089 |
0.991 |
1.513 |
15 |
8b |
100 |
95 |
76 |
55 |
33 |
31.371 |
y = 0.207x − 1.051 |
0.985 |
1.497 |
16 |
8c |
100 |
95 |
80 |
61 |
33 |
27.470 |
y = 0.211x − 0.948 |
0.957 |
1.439 |
17 |
8d |
100 |
95 |
76 |
57 |
33 |
30.444 |
y = 0.206x − 1.011 |
0.980 |
1.484 |
18 |
8e |
100 |
93 |
70 |
50 |
30 |
36.840 |
y = 0.200x − 1.155 |
0.984 |
1.567 |
19 |
8f |
100 |
95 |
75 |
54 |
33 |
32.146 |
y = 0.206x − 1.069 |
0.989 |
1.507 |
20 |
8g |
100 |
95 |
74 |
52 |
32 |
33.902 |
y = 0.207x − 1.138 |
0.992 |
1.530 |
21 |
8h |
100 |
94 |
72 |
51 |
32 |
35.174 |
y = 0.201x − 1.114 |
0.992 |
1.546 |
22 |
8i |
100 |
95 |
79 |
60 |
32 |
28.749 |
y = 0.211x − 0.995 |
0.960 |
1.459 |
23 |
8j |
100 |
95 |
77 |
58 |
32 |
30.230 |
y = 0.208x − 1.026 |
0.972 |
1.480 |
24 |
Triadimenol |
100 |
98 |
89 |
76 |
59 |
1.945 |
y = 0.235x − 0.065 |
0.992 |
0.289 |
3.3.2. Fungicidal activity of the title compounds against F. graminearum. The results of the fungicidal activity of the title compounds against F. graminearum are listed in Table 3, from which it is shown that compounds 3, 5a–f, 7a–f and 8a–j exhibited low fungicidal activity against F. graminearum. The death rate of most compounds was about 30–40% at 256 μg mL−1, which was less effective than the fungicidal activity against R. solani.
Table 3 Fungicidal activity of compounds against F. graminearum
Compd |
Fungicidal activity (%) at a concentration of (μg mL−1) |
Compd |
Fungicidal activity (%) at a concentration of (μg mL−1) |
256 |
128 |
64 |
256 |
128 |
64 |
3 |
20 |
0 |
— |
7f |
43 |
20 |
0 |
5a |
30 |
10 |
0 |
8a |
38 |
20 |
0 |
5b |
30 |
10 |
0 |
8b |
40 |
20 |
0 |
5c |
30 |
10 |
0 |
8c |
40 |
20 |
0 |
5d |
30 |
10 |
0 |
8d |
40 |
20 |
0 |
5e |
30 |
10 |
0 |
8e |
40 |
20 |
0 |
5f |
30 |
10 |
0 |
8f |
40 |
20 |
0 |
7a |
44 |
20 |
0 |
8g |
37 |
20 |
0 |
7b |
45 |
20 |
0 |
8h |
36 |
20 |
0 |
7c |
43 |
20 |
0 |
8i |
40 |
20 |
0 |
7d |
45 |
20 |
0 |
8j |
40 |
20 |
0 |
7e |
30 |
20 |
0 |
Triadimenol |
100 |
100 |
89 |
3.3.3. Fungicidal activity of the title compounds against B. cinerea. The results of the fungicidal activity of the title compounds against B. cinerea are listed in Table 4, from which it can be seen that all of the test compounds exhibited low fungicidal activity against B. cinerea. These compounds have the lowest fungicidal activity against B. cinerea, and the death rate was about 20–30% at 256 μg mL−1.
Table 4 Fungicidal activity of compounds against B. cinerea
Compd |
Fungicidal activity (%) at a concentration of (μg mL−1) |
Compd |
Fungicidal activity (%) at a concentration of (μg mL−1) |
256 |
128 |
64 |
256 |
128 |
64 |
3 |
10 |
0 |
— |
7f |
33 |
20 |
0 |
5a |
20 |
10 |
0 |
8a |
38 |
10 |
0 |
5b |
20 |
10 |
0 |
8b |
30 |
10 |
0 |
5c |
20 |
10 |
0 |
8c |
30 |
10 |
0 |
5d |
20 |
10 |
0 |
8d |
30 |
10 |
0 |
5e |
20 |
10 |
0 |
8e |
30 |
10 |
0 |
5f |
20 |
10 |
0 |
8f |
30 |
10 |
0 |
7a |
34 |
20 |
0 |
8g |
27 |
10 |
0 |
7b |
35 |
20 |
0 |
8h |
26 |
10 |
0 |
7c |
33 |
20 |
0 |
8i |
30 |
10 |
0 |
7d |
35 |
20 |
0 |
8j |
30 |
10 |
0 |
7e |
30 |
20 |
0 |
Triadimenol |
100 |
100 |
89 |
3.4. QSAR study on the fungicidal activity against R. solani
In the QSAR study, the selection of the structure descriptors was an important step to determine the molecular features correlating to the activity. The descriptors can be categorized as constitutional, topological, geometrical, electrostatic, quantum chemical, and thermodynamic. In this research, the most important factors were the geometry and charge distribution, which involved some descriptors, such as the HOMO energy (the energy of the highest occupied molecular orbital), LUMO energy (the energy of the lowest occupied molecular orbital), dipole moment, etc. Many regression approaches, such as the best multi-linear, multi-linear regression, principal component analysis, partial least square regression, and heuristic regression are available in the CODESSA 2.7.15 software. In view of the number of samples and descriptors involved in this work, the best multi-linear regression was chosen.
Determining the number of descriptors is an another important step. A simple rule called the “breaking point” was used in the improvement of the statistical quality of the model. As shown in Fig. 2, the R2 value of the best multi-linear regression had a dramatic increase before the number of the descriptors reached 4. The significance of the descriptors within the model was reflected in the t-test. Descriptors with high t values were accepted and those with low t values were rejected. After the number of the descriptors reached a certain value, the improvement of the regression model became less insignificant (ΔR2 < 0.02–0.04). Furthermore, another point worth noting was that the number of descriptors conformed to the linear regression given by eqn (1):
where
N is the number of samples and
k is the number of descriptors. Consequently, the final QSAR model with four descriptors was selected as the best model. The values of the four descriptors of sample compounds are listed in
Table 5.
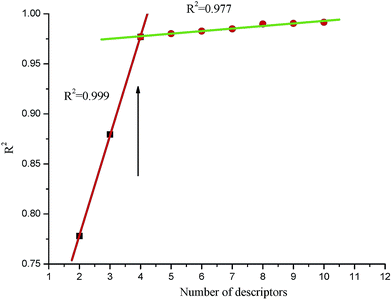 |
| Fig. 2 The “breaking point” rule results. | |
Table 5 Fungicidal activity and structural descriptors of the title compounds
No. |
Compd |
log IC50 |
Structure descriptors |
HOMO |
DM |
qOmax |
qmin |
1 |
5a |
1.844 |
−0.3512 |
−0.2067 |
0.3524 |
−0.3512 |
2 |
5b |
1.860 |
−0.3534 |
−0.2067 |
0.3523 |
−0.3534 |
3 |
5c |
1.836 |
−0.3523 |
−0.2067 |
0.3524 |
−0.3523 |
4 |
5d |
1.860 |
−0.3525 |
−0.2067 |
0.3520 |
−0.3525 |
5 |
5e |
1.864 |
−0.3546 |
−0.2066 |
0.3519 |
−0.3546 |
6 |
5f |
1.822 |
−0.3534 |
−0.2067 |
0.3521 |
−0.3534 |
7 |
7a |
0.480 |
−0.2713 |
−0.2072 |
0.2748 |
−0.2713 |
8 |
7b |
0.387 |
−0.2710 |
−0.2070 |
0.2522 |
−0.2768 |
9 |
7c |
0.516 |
−0.2725 |
−0.2072 |
0.2745 |
−0.2725 |
10 |
7d |
0.269 |
−0.2718 |
−0.2072 |
0.2748 |
−0.2718 |
11 |
7e |
0.516 |
−0.2718 |
−0.2072 |
0.2749 |
−0.2718 |
12 |
7f |
0.576 |
−0.2731 |
−0.2072 |
0.2746 |
−0.2731 |
13 |
8a |
1.513 |
−0.2896 |
−0.2095 |
0.3705 |
−0.2896 |
14 |
8b |
1.497 |
−0.2917 |
−0.4339 |
0.3685 |
−0.4339 |
15 |
8c |
1.439 |
−0.2922 |
−0.2071 |
0.3706 |
−0.2922 |
16 |
8d |
1.484 |
−0.2950 |
−0.2070 |
0.3696 |
−0.2950 |
17 |
8e |
1.567 |
−0.2963 |
−0.2070 |
0.3697 |
−0.2963 |
18 |
8f |
1.507 |
−0.2873 |
−0.2073 |
0.3706 |
−0.2873 |
19 |
8g |
1.530 |
−0.2945 |
−0.2086 |
0.3698 |
−0.2945 |
20 |
8h |
1.546 |
−0.2970 |
−0.2071 |
0.3691 |
−0.2970 |
21 |
8i |
1.459 |
−0.2907 |
−0.2072 |
0.3697 |
−0.2907 |
22 |
8j |
1.480 |
−0.2940 |
−0.2071 |
0.3697 |
−0.2940 |
The best statistical model for the log
IC50 data had the following statistical characteristics: R2 = 0.9879, F = 348.41, S2 = 0.0047. This model included four descriptors in descending order according to their statistical significance, which is shown in Table 6, and the regression coefficients X and their standard errors ΔX were also listed.
Table 6 The best four-descriptor model
Descriptor no. |
X |
±ΔX |
t-Test |
Descriptor |
Energy of the highest occupied molecular orbital in atomic units. Dipole moment. Max. net atomic charge for an O atom. Min. net atomic charge. |
0 |
−8.3866 |
1.5216 |
−5.5119 |
Intercept |
1 |
−3.9598 × 101 |
1.1968 × 101 |
−3.3086 |
HOMOa |
2 |
−1.9346 × 101 |
7.4859 |
−2.5843 |
DMb |
3 |
8.8104 |
4.5244 × 10−1 |
19.4733 |
qOmaxc |
4 |
3.0670 × 101 |
1.1916 × 101 |
2.5738 |
qmind |
The values of the experimental and predicted log
IC50 are listed in Table 7, and the plot of the comparison between the predicted and experimental values is shown in Fig. 3. The four-descriptor QSAR model equation is described in the following eqn (2)
|
log IC50 = −8.3866 − 39.598 × HOMO − 19.346 × DM + 8.8104 × qOmax + 30.670 × qmin
| (2) |
N = 22, R2 = 0.9879, F = 348.41, S2 = 0.0047 |
Table 7 The difference between the experimental log
IC50 and predicted log
IC50
No. |
Compd |
Calc. log IC50 |
Exp. log IC50 |
Difference |
No. |
Compd |
Calc. log IC50 |
Exp. log IC50 |
Difference |
1 |
5a |
1.8522 |
1.9670 |
−0.1148 |
12 |
7f |
0.4792 |
0.4792 |
0.5760 |
2 |
5b |
1.8710 |
1.8480 |
0.0270 |
13 |
8a |
1.5159 |
1.5159 |
1.5130 |
3 |
5c |
1.8620 |
1.8600 |
0.0020 |
14 |
8b |
1.4970 |
1.4970 |
1.4970 |
4 |
5d |
1.8603 |
1.8360 |
0.0243 |
15 |
8c |
1.4936 |
1.4936 |
1.4390 |
5 |
5e |
1.8762 |
1.8640 |
0.0122 |
16 |
8d |
1.5078 |
1.5078 |
1.4840 |
6 |
5f |
1.8692 |
1.8220 |
0.0472 |
17 |
8e |
1.5203 |
1.5203 |
1.5670 |
7 |
7a |
0.4649 |
0.4800 |
−0.0151 |
18 |
8f |
1.4537 |
1.4537 |
1.5070 |
8 |
7b |
0.3879 |
0.3870 |
0.0009 |
19 |
8g |
1.5361 |
1.5361 |
1.5300 |
9 |
7c |
0.4730 |
0.5160 |
−0.0430 |
20 |
8h |
1.5232 |
1.5232 |
1.5460 |
10 |
7d |
0.4694 |
0.2690 |
0.2004 |
21 |
8i |
1.4742 |
1.4742 |
1.4590 |
11 |
7e |
0.4702 |
0.5160 |
−0.0458 |
22 |
8j |
1.5017 |
1.5017 |
1.4800 |
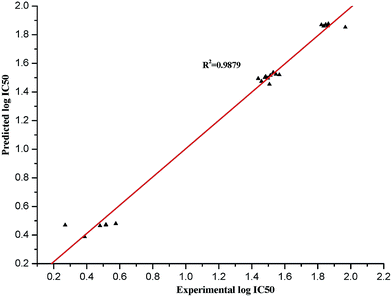 |
| Fig. 3 Experimental log IC50 versus predicted log IC50. | |
The developed QSAR model was validated by both internal validation and “leave-one-out” cross-validation methods. In the internal validation, the compounds were divided into three subsets: A, B, and C. The compounds 1, 4, 7, 10, etc., belonged to subset A; 2, 5, 8, 11, etc., belonged to subset B; and 3, 6, 9, 12, etc., belonged to subset C. Two subsets, (A and B), (B and C), or (A and C) were selected as the training set, and the remaining subset was treated as the test set. The correlation equation, obtained from each of the training sets using the same descriptors, was used to predict values of the corresponding test sets. The internal validation results are listed in Table 8.
Table 8 Internal validation of the QSAR modela
Training set |
N |
R2 |
F |
S2 |
Test set |
N |
R2 |
F |
S2 |
Compounds A: 1, 4, 7, 10, 13, 16, 19, 22, compounds B: 2, 5, 8, 11, 14, 17, 20, compounds C: 3, 6, 9, 12, 15, 18, 21. |
A + B |
15 |
0.9800 |
340.78 |
0.0043 |
C |
7 |
0.9843 |
345.90 |
0.0045 |
B + C |
14 |
0.9711 |
339.58 |
0.0041 |
A |
8 |
0.9814 |
343.25 |
0.0044 |
A + C |
15 |
0.9724 |
339.63 |
0.0050 |
B |
7 |
0.9793 |
341.47 |
0.0043 |
Average |
|
0.9745 |
340.00 |
0.0045 |
Average |
|
0.9817 |
343.54 |
0.0044 |
The difference between RTraining2 and RTest2 were within 5% for the three sets, and the average values of RTraining2 = 0.9745 and RTest2 = 0.9817 were very similar to the integrated R2 value, which signified that the obtained model possessed the predictive power of three-fold cross-validation. In a similar way to the internal validation, the “leave-one-out” method can be implemented. Every fourth compound (1, 5, 9, 13, 17, 21) was put into an external test set, and the remaining compounds were left in the training set. With the same four descriptors, the R2 of the training set was 0.9640, and the R2 of the test set was 0.9533, which also indicated that the obtained QSAR model was satisfactory.
Some structural features could make a difference to the fungicidal activity by interpreting the descriptors involved in the QSAR model. The first important descriptor was the HOMO energy, which is directly related to the ionization potential of the compounds.38,39 In Fig. 4, the HOMO energy maps for compounds 7b and 7d are shown. In eqn (2), the HOMO energy and activity are negatively correlated, which suggested that the electron withdrawing substitution groups of the derivatives are beneficial for the fungicidal activity against R. solani. The conclusion obtained from the QSAR study partially met the above SAR study result.
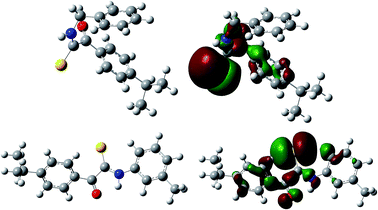 |
| Fig. 4 Optimized structures and HOMO energy maps for compounds 7b and 7d from the DFT calculations of Gaussian 03W. The green parts represent positive molecular orbitals, and the red parts represent negative molecular orbitals. | |
The second important descriptor was the dipole moment, which is a measure of the polarization between the positive and negative electrical charges in a system.40,41 The C
O, C
S, and N–H groups exhibited permanent polarization because of a significant electronegativity difference between the atoms. Among the title compounds, the acylthiourea derivatives from β-pinene displayed the better fungicidal activity, which illustrated that the dipole moment played a critical role in modulating the activity.
The 3rd and 4th important descriptors were the maximum net atomic charge for an O atom and the minimum net atomic charge. Both of these two descriptors can give an expression of the features of the charge distribution in the molecules. In eqn (2), the appearance of a positive sign in the model indicated that a molecule with a higher descriptor value had a higher log
IC50. On the contrary, a negative sign in the model indicated that a molecule with a lower descriptor value had a higher log
IC50.
In summary, both the SAR and QSAR studies indicated that the electron withdrawing substitution groups of the β-pinene derivatives had a positive effect towards the fungicidal activity. In the light of these results, further research on the correlation of SAR and QSAR is expected to carry on, and the design and exploration of potentially efficient fungicides would be implemented.
4. Conclusions
For the purpose of increasing the added value of turpentine, three series of β-pinene analogues were prepared and their fungicidal activity against three common agricultural fungi was determined. The acylthiourea derivatives from β-pinene 7a–f displayed more significant activity than the other derivatives. It was worth noting that compounds 7b and 7d exhibited excellent activity. Both the SAR and QSAR studies indicated that the structural features had an apparent influence on the fungicidal activity. From these satisfying consequences, some insight into the exploitation of eco-friendly fungicides from natural products with low toxicity and high efficiency can be gained.
Acknowledgements
The present research was supported by the National Nature Science Foundation of China (Project No. 31401783 and Project No. 31400509) and the Research Fund for the Doctoral Program of Higher Education of Northwest A&F University (Project No. Z109021501).
Notes and references
- G. P. Dively and A. Kamel, J. Agric. Food Chem., 2012, 60, 4449–4456 CrossRef CAS PubMed
. - M. Ishtiaq, M. A. Saleem and M. Razaq, Crop Prot., 2012, 33, 13–20 CrossRef CAS PubMed
. - J. D. Rios-Diez and C. I. Saldamando-Benjumea, J. Econ. Entomol., 2011, 104, 1698–1705 CrossRef CAS
. - J. Y. Shim, Y. A. Kim, Y. T. Lee, B. D. Hammock and H. S. Lee, J. Agric. Food Chem., 2010, 58, 5241–5247 CrossRef CAS PubMed
. - G. Tanner and C. Czerwenka, J. Agric. Food Chem., 2011, 59, 12271–12277 CrossRef CAS PubMed
. - M. B. Isman, Annu. Rev. Entomol., 2006, 51, 45–66 CrossRef CAS PubMed
. - C. L. Cantrell, F. E. Dayan and S. O. Duke, J. Nat. Prod., 2012, 75, 1231–1242 CrossRef CAS PubMed
. - D. B. Turley, Q. Chaudhry, R. W. Watkins and F. E. I. Deswarte, Ind. Crops Prod., 2006, 24, 238–243 CrossRef CAS PubMed
. - E. A. Martinuzzi and J. C. Arago, US Pat., 3, 112, 347, November 26, 1963
. - C. Chapuis and P. A. Blanc, US Pat., 5, 696, 075, December 9, 1997.
- E. H. Uhing, US Pat. 4, 758, 684, July 19, 1988.
- Y. J. Cui, X. P. Rao, S. B. Shang, J. Song and Y. Q. Gao, Lett. Drug Des. Discovery, 2013, 10, 102–110 CAS
. - Y. Q. Gao, S. B. Shang, D. Wang and Y. j. Cui, Lett. Drug Des. Discovery, 2013, 10, 613–619 CrossRef CAS
. - J. A. Grodnitzky and J. R. Coats, J. Agric. Food Chem., 2002, 50, 4576–4580 CrossRef CAS PubMed
. - J. Li, G. M. Xiao, S. B. Shang and X. P. Rao, Lett. Drug Des. Discovery, 2014, 11, 59–66 CrossRef CAS
. - Z. N. Juárez, L. R. Hernández, H. Bach and E. Sánchez-Arreola, Ind. Crops Prod., 2015, 74, 178–182 CrossRef PubMed
. - C. Josep and T. Yudelsy, Phytochem. Anal., 2005, 16, 67–70 CrossRef PubMed
. - M. Yahyaa, D. Tholl, G. Cormier, R. Jensen and P. W. Simon, J. Agric. Food Chem., 2015, 63, 4870–4878 CrossRef CAS PubMed
. - G. V. Kokurkina, M. D. Dutov, S. A. Shevelev, S. V. Popkov, A. V. Zakharov and V. V. Poroikov, Eur. J. Med. Chem., 2011, 46, 4374–4382 CrossRef CAS PubMed
. - E. Paruch, J. Nawrot and C. Wawrzenczyk, Pest Manage. Sci., 2001, 57, 776–780 CrossRef CAS PubMed
. - R. K. Satdive, D. P. Fulzele and S. Eapen, J. Biotechnol., 2007, 128, 281–289 CrossRef CAS PubMed
. - Z. D. Wang, J. Song, Z. J. Han, Z. K. Jiang, W. Q. Zheng, J. Z. Chen, Z. Q. Song and S. B. Shang, J. Agric. Food Chem., 2008, 56, 11361–11366 CrossRef CAS PubMed
. - N. A. Mohamed and N. A. Abd El-Ghany, Int. J. Biol. Macromol., 2012, 50, 1280–1285 CrossRef CAS PubMed
. - N. C. Kasuga, K. Sekino, M. Ishikawa, A. Honda, M. Yokoyama, S. Nakano, N. Shimada, C. Koumo and K. Nomiya, J. Inorg. Biochem., 2003, 96, 298–310 CrossRef CAS
. - W. Thorsell and H. Tunon, Phytomedicine, 1998, 5, 307–310 CrossRef CAS
. - Z. D. Wang, Z. K. Jiang and Z. Q. Song, Chin. J. Hyg. Insectic. Equip., 2004, 10, 37–40 CAS
. - Z. D. Wang, J. Song, J. Z. Chen, Z. Q. Song, S. B. Shang, Z. K. Jiang and Z. J. Han, Bioorg. Med. Chem. Lett., 2008, 18, 2854–2859 CrossRef CAS PubMed
. - D. Pitarokili, M. Couladis, N. Petsikos-Panayotarou and O. Tzakou, J. Agric. Food Chem., 2002, 50, 6688–6691 CrossRef CAS PubMed
. - I. A. Southwell and T. M. Flynn, Xenobiotica, 1980, 10, 17–23 CrossRef CAS
. - Y. Q. Gao, J. Song, S. B. Shang, D. Wang and J. Li, BioResources, 2012, 7, 4150–4160 Search PubMed
. - Y. Q. Gao, S. B. Shang, X. Xu, X. P. Rao and H. X. Wang, Acta Crystallogr., Sect. E: Struct. Rep. Online, 2009, 65, o2748 CAS
. - Y. Q. Gao, J. Li, S. B. Shang and D. Wang, Lett. Drug Des. Discovery, 2015, 12, 241–249 CAS
. - SPSS Inc., SPSS Base version 17.0 for user’s guide, SPSS Inc., Chicago IL, 2008 Search PubMed
. - M. J. Frisch, G. W. Trucks, H. B. Schlegel, G. E. Scuseria, M. A. Robb, J. R. Cheeseman, J. A. Montgomery, T. Vreven, K. N. Kudin, J. C. Burant, J. M. Millam, S. S. Iyengar, J. Tomasi, V. Barone, B. Mennucci, M. Cossi, G. Scalmani, N. Rega, G. A. Petersson, H. Nakatsuji, M. Hada, M. Ehara, K. Toyota, R. Fukuda, J. Hasegawa, M. Ishida, T. Nakajima, Y. Honda, O. Kitao, H. Nakai, M. Klene, X. Li, J. E. Knox, H. P. Hratchian, J. B. Cross, V. Bakken, C. Adamo, J. Jaramillo, R. Gomperts, R. E. Stratmann, O. Yazyev, A. J. Austin, R. Cammi, C. Pomelli, J. W. Ochterski, P. Y. Ayala, K. Morokuma, G. A. Voth, P. Salvador, J. J. Dannenberg, V. G. Zakrzewski, S. Dapprich, A. D. Daniels, M. C. Strain, O. Farkas, D. K. Malick, A. D. Rabuck, K. Raghavachari, J. B. Foresman, J. V. Ortiz, Q. Cui, A. G. Baboul, S. Clifford, J. Cioslowski, B. B. Stefanov, G. Liu, A. Liashenko, P. Piskorz, I. Komaromi, R. L. Martin, D. J. Fox, T. Keith, M. A. Al-Laham, C. Y. Peng, A. Nanayakkara, M. Challacombe, P. M. W. Gill, B. Johnson, W. Chen, M. W. Wong, C. Gonzalez and J. A. Pople, Gaussian, Inc., Wallingford CT, 2004
. - J. Li, X. P. Rao, S. B. Shang, Y. Q. Gao and B. L. Song, J. Chem. Soc. Pak., 2012, 34, 217–222 CAS
. - I. Aranaz, R. Harris and A. Heras, Curr. Org. Chem., 2010, 14, 308–330 CrossRef CAS
. - A. Nadia, A. Mohamed Nahed and A. El-Ghany, Cellulose, 2012, 19, 1879–1891 CrossRef
. - D. Sharma, B. Narasimhan, P. Kumar and A. Jalbout, Eur. J. Med. Chem., 2009, 44, 1119–1127 CrossRef CAS PubMed
. - P. Sharma, A. Kumar, S. Upadhyay, V. Sahu and J. Singh, Eur. J. Med. Chem., 2009, 44, 251–259 CrossRef CAS PubMed
. - E. Koschier and K. Sedy, Crop Prot., 2003, 22, 929–934 CrossRef CAS
. - D. Ma, A. K. Bhattacharjee, R. K. Gupta and J. M. Karle, Am. J. Trop. Med., 1999, 60, 1–6 CAS
.
Footnotes |
† Electronic supplementary information (ESI) available: IR, 1H NMR, MS, and elemental analysis data for the target compounds. See DOI: 10.1039/c5ra10660e |
‡ The authors contributed equally to this work and should be considered co-first authors. |
|
This journal is © The Royal Society of Chemistry 2015 |