DOI:
10.1039/C5MB00007F
(Paper)
Mol. BioSyst., 2015,
11, 1012-1028
Hierarchical control of coherent gene clusters defines the molecular mechanisms of glioblastoma†
Received
5th January 2015
, Accepted 13th January 2015
First published on 4th February 2015
Abstract
Glioblastoma is a highly-aggressive and rapidly-lethal tumor characterized by resistance to therapy. Although data on multiple genes, proteins, and pathways are available, the key challenge is deciphering this information and identifying central molecular targets. Therapeutically targeting individual molecules is often unsuccessful due to the presence of compensatory and redundant pathways, and crosstalk. A systems biology approach that involves a hierarchical gene group networks analysis can delineate the coherent functions of different disease mediators. Here, we report an integrative networks-based analysis to identify a system of coherent gene modules in primary and secondary glioblastoma. Our study revealed a hierarchical transcriptional control of genes in these modules. We elucidated those modules responsible for conversion of the glioma-associated microglia/macrophages into glioma-supportive, immunosuppressive cells. Further, we identified clusters comprising mediators of angiogenesis, proliferation, and cell death for both primary and secondary glioblastomas. Data obtained for these clusters point to a possible role of transcription regulators that function as the gene modules mediators in glioblastoma pathogenesis. We elucidated a set of possible transcription regulators that can be targeted to affect the selected gene clusters at specific levels for glioblastoma. Our innovative approach to construct informative disease models may hold the key to successful management of complex diseases including glioblastoma and other cancers.
Introduction
Glioblastoma (GBM) is one of the most malignant cancers that is rapidly lethal and treatment-resistant. Similarly to other cancers, GBM has complex interactions with genetic and epigenetic pathways, signaling networks, and cellular levels, to name a few. Numerous gene-expression analyses have been conducted on GBM tumor tissues in the past decade. Extracting the knowledge from these data as well as associated integrative knowledge is an important task in GBM studies and in bioinformatics in general.
The prominent genetic alterations associated with GBM have been elucidated in numerous publications.1–6 These abnormalities include significantly increased angiogenesis, a high rate of tumor proliferation, anti-apoptosis gene activation, and invasive immunosuppressing microglia. GBM is a highly-vascular tumor associated with a high degree of microvascular proliferation. It is critical to understand the molecular pathways regulating these events, especially angiogenesis, because it represents a highly-attractive therapeutic target. In addition, GBM is characterized by high, unregulated cellular proliferation, owing primarily to alterations in the cell cycle regulatory genes. The process of tumorigenesis is often associated with cells evading apoptosis, which determines the susceptibility of cells to various chemotherapeutic agents and to radiation. Finally, one of the distinguishing features of GBM that makes it intractable is its high degree of infiltration into surrounding normal brain tissue.7
Considering the complex interplay of mediators involved in GBM pathophysiology, it is clear that examining individual signaling molecules or even pathways may not give information accurately representing the underlying molecular abnormalities. We believe that a comprehensive bioinformatics analysis using an integrative systems-biology approach may provide a means to define or delineate the roles of these and other signaling mediators in the pathophysiology and in turn prognosis of GBM. The systems biology approach allows us to analyze in conjunction gene expression, signaling and metabolic pathways, gene-regulation and protein-interaction networks, and gene-ontology data, to visualize and query these networks and pathways, and to predict new gene targets for the treatment of disease and the identification of pathways that can be affected by drugs. Here, using this approach, we present a network analysis that defines coherent gene modules (CGMs) and highlights the hierarchical control of them. Our analysis identifies “highly-connected” signaling entities for GBM. Furthermore, we define gene modules that comprise specific mediators involved in the pathophysiology of GBM. These data will not only improve our understanding of basic disease pathology but will also identify effective therapeutic targets for GBM.
Results
Microarray data analysis
We used microarray data obtained from the Affymetrix GeneChip® Human Genome U133 Plus 2.0 Array (Santa Clara, Calif., USA) deposited by Michal Grzmil and colleagues in the Gene Expression Omnibus (GEO) database (entry GEO15824, http://www.ncbi.nlm.nih.gov/geo/query/acc.cgi?acc=GSE15824). The microarray contains 54
675 probes and several sample groups, from which we used three groups: 12 samples from primary glioblastoma (GBM1), 3 samples from secondary (GBM2), and 2 samples from normal brains (Norm). For details see the Methods and materials section.
After Kruskal–Wallis nonparametric test of the ANOVA8 with p = 0.025 for the 2229 significant genes obtained (4%), we subjected this data to expression threshold filtering. Only differentially expressed genes that overcome the 1.5-fold threshold of GBM gene expression compared to normal were taken into account, separately for primary (GBM1) and secondary (GBM2) glioblastomas. 442 genes for GBM1 and 586 genes for GBM2 were the subjects of further analysis. For these genes the average values for each probe for the normal group (NormAVE) were calculated and their expressions were normalized to GBM1/NormAVE and GBM2/NormAVE rates (creating two sets of genes: GBM1 for primary, and GBM2 for secondary glioblastomas), which underwent further analysis. Some of the genes belong to both the GBM1 and GBM2 sets but their expressions significantly differ.
Hierarchical networks of TFs and TRs regulating glioblastoma genes
As described above, using BiologicalNetworks,9,10 we conducted analysis of calculated values for 12 sets of GBM1 and 3 sets of GBM2, using the method described earlier.11 On the basis of analysis of the microarray data and the existing Integrome database (IntegromeDB) of connectivity of transcription regulators (TRs) and transcription factors (TFs), the program created networks of “coherent gene modules” (CGMs), TFs, and TRs11 for both GBM1 and GBM2. One of the most important parameters in the analysis of TFs and TRs is their connectivity.
Primary GBM (GBM1).
The network for GBM1 contains 11 CGMs (generated by the BiologicalNetworks program), each including different genes from the aforementioned 442 gene set. All these genes are differentially expressed with a ratio of cancer expression to normal average expression of greater than 1.5 (Fig. 1).
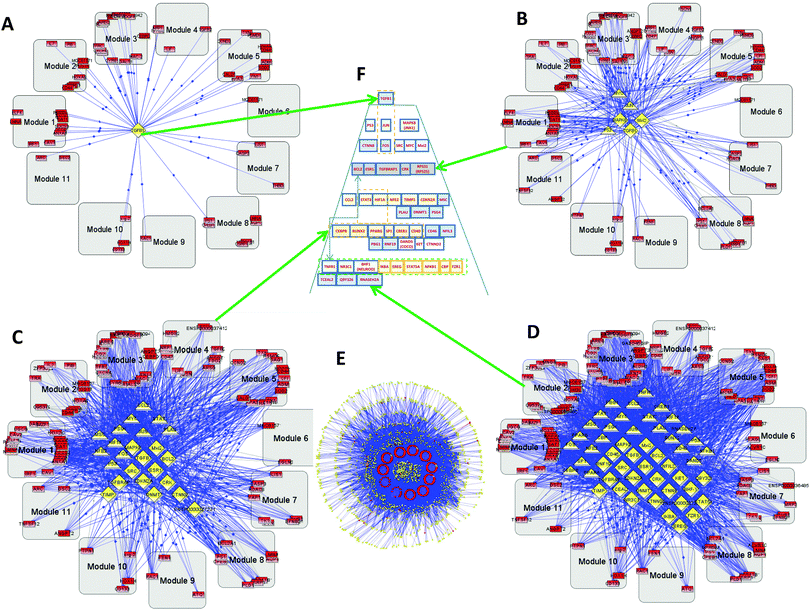 |
| Fig. 1 Primary glioblastoma (GBM1) hierarchy: gray rounded squares—coherent gene modules (CGMs), red rectangles—genes, yellow triangles—transcription factors (TFs), yellow diamonds—transcription regulators (TRs). (A) Level 1 hierarchical network. Several genes included in CGMs and the only top level TR–TGFB (TGFβ), (B) level 3 of hierarchy. (C) Level 9. (D) Level 12. These four panels (A–D) show gradual conversion from the top level (A) to the bottom (E). (E) Bottom level full network including 442 genes, 155 TFs, and 1605 TRs. (F) A hierarchical control pyramid of TFs and TRs that regulate all the CGMs. | |
The complete set includes 442 genes, 155 TFs, and 1605 TRs (Fig. 1E). Because of the number of connections involved, we cannot use image analysis and instead studied this computationally. Fig. 1A–D, show the TF–TR–CGM networks “sliced” on consecutive levels of network connectivity. The level of connectivity of a particular gene in the network has for a long time been considered as a parameter related to its importance. There exists a so-called centrality–lethality (C–L) rule, which states that highly-connected genes in a network are likely more important for cell viability.12,13 It has also been shown that the connectivity parameter for particular genes can be important not only for general cell function, but also for specified functions of the gene groups, like environmental response, cell cycle, DNA damage, etc.14 From our study on GBM1, we demonstrate that the TR with the greatest connectivity in this network is the transforming growth factor-beta (TGFB—TGFβ) that appears on the first layer (see Fig. 1A and Fig. 2). TGFB is increasingly recognized as an important player in GBM15,16 and our group is conducting clinical trials on TGFB inhibitors that show activity against GBM. Other slices of the connectivity pyramid were made on different levels: level 3 (Fig. 1B), level 9 (Fig. 1C), and level 12 (Fig. 1D). The central pyramid (Fig. 1F and Fig. 2) gives a hierarchical representation of the entire network of TFs and TRs that actually regulate all the CGMs, based on connectivity level. One can see that different levels have significant functional separation by their connectivity. A close-up of the top levels is shown in Fig. S1A (ESI†).
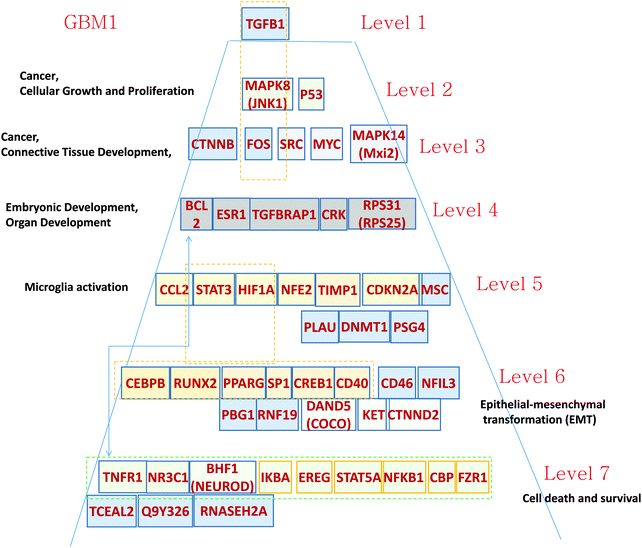 |
| Fig. 2 Hierarchical pyramid for primary glioblastoma. This figure shows a close-up of the control pyramid shown in Fig. 1F, including TFs and TRs. Each level is responsible for the control of specific functions. | |
To illustrate the gene functional distribution inside the modules, heat maps were created for every important CGM (Fig. S2 and S3, ESI†). The color represents the p-value and the size represents the quantity of genes with the mentioned function. In Fig. S2 (ESI†) one can see that in GBM1, genes from the CGM1 participate in proliferation, differentiation, and migration of cells with p-values 1.09 × 10−8, 3.18 × 10−5, and 4.85 × 10−5, respectively. Genes from CGM2 are responsible for abnormal morphogenesis and inflammation. Genes of CGM3 participate in development of blood vessels (p = 1.03 × 10−8), vasculogenesis (p = 1.86 × 10−8), angiogenesis (p = 3.43 × 10−5), cell differentiation, proliferation, migration, and movement (p = 2.16 × 10−7, 4.97 × 10−7, 3.02 × 10−7, and 3.74 × 10−7, respectively), and especially in proliferation of tumor cells (p = 3.85 × 10−5), metastasis (p = 1.97 × 10−5), and growth of tumor (p = 3.18 × 10−5). CGM5 genes participate in cell proliferation.
Level 1 of the TFs and TRs comprises TGFB.
Level 2 of the TFs and TRs includes p53, involved in the MAPK8 regulation pathway; MAPK8 phosphorylates p53 and also activates the TFs and TRs in a lower level. These genes are related to cancer, cellular growth, and proliferation.
Level 3 includes FOS, which is also in part activated by TGFB. FOS interacts with RAS–RAF–MEK, JNK, and MYC–MAX groups. These genes are related to cancer and connective tissue development.
Level 4 includes TGFBRAP1, which is involved in TGFB regulation.17 The level also includes TFs and TRs related to the AKT–mTOR–PI3K signaling pathway.18 v-Crk activates the PI3K/AKT pathway;19 BCL2 is activated by the AKT/mTOR pathway.20 These genes are related to development.
Level 5 contains a majority of genes related to microglia activation. It has been shown21 that microglia have become immunosuppressive. Glioma-associated macrophages (MFs) and microglial cells promote cancer by increasing pro-angiogenic factors and enhancing invasion.21–23 Expression of one of the microglia-activating genes HIF-1 is associated with CCL2 chemokine expression.24 CCL2 interacts with MSP-1 and modulates the microglia activation and proliferation.25 STAT activates creation of the immunosuppressive microglia that support cancer cell proliferation.26 Gabrusiewicz and Heimberger26 comment that “Mounting evidence indicates that the glioma microenvironment converts the glioma-associated microglia/macrophages (GAMs) into glioma-supportive, immunosuppressive cells.” This way the level 5 TFs and TRs can play a significant role in GBM development and need appropriate therapeutic intervention.
Level 6 of the TRs pyramid of GMB1 includes a majority of epithelial–mesenchymal transformation (EMT)-related genes. First, we note that the top gene TGFB is known to induce EMT and the level 6 and some other genes on different levels actually provide the signaling for this transformation. For example, PPARG is directly responsible for TGFB-induced EMT and consequent tumor invasion.27 The SP1 gene in level 6 cooperating with ZEB2 upregulates integrin alpha5 expression participation in EMT in cancer cells.28
Level 7 of the pyramid is mostly related to cell death. Some of the TFs and TRs activate cell death pathways: TNFR1, NR3C1, and BNF1 (NeuroD); and others (IKBA, EPEG, STAT5A, NFKB1, FZR1) inhibit this process.
The most important coherent gene modules responsible for carcinogenesis
In the previous section, we described and analyzed the “pyramid” hierarchy of the TR and TF networks (that control the CGMs) based on their connectivity. Here we analyze the most populated CGMs using Ingenuity Interactive Pathway Analysis (IPA) software (Ingenuity Systems, Inc., Redwood City, Calif.) and describe the influence of their interactions with the TRs and TFs in terms of their functions and their effects on tumorigenesis. The BiologicalNetworks software generated 11 CGMs based on 442 differentially expressed genes for GBM1, and 13 CGMs based on 586 genes for GBM2. We considered only statistically significant CGMs and describe them in ascending p-value order.
Analyzing the most populated CGMs using IPA, we obtained several gene networks. For further analysis we selected only statistically significant and functionally meaningful networks.
For GBM1 we selected CGMs 1 and 3, which are statistically significant. Note that the most populated CGM3 has the lowest p-values (highest 1/p values) for the functions of development of blood vessels (Fig. 3C). As noted by El Hallani and co-authors, GBM is one of the most angiogenic human tumors.29 The other main feature noted by these authors is the highest rate of endothelial proliferation. These factors are also among the most important functions of CGM3 (Fig. 3C). In the GBM1, CGM3 has 11 connections with a higher-level controlling TR–TGFB1—the maximum number of connections in comparison with other CGMs.
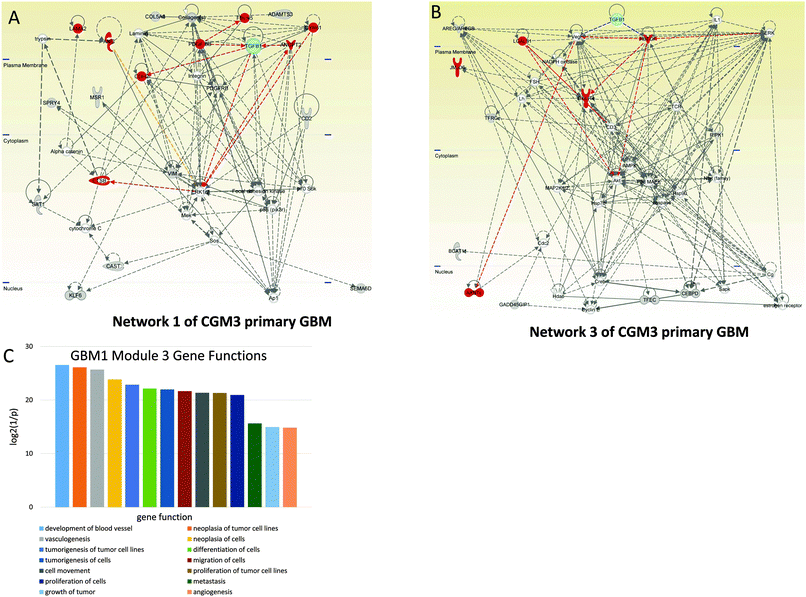 |
| Fig. 3 Angiogenesis-controlling CGM3s of GBM1. Networks of genes included in CGM3 used for analysis: cyan—TGFB1, red—genes responsible for angiogenesis. (A) Network 1. TGFB1 is the hub, regulating a number of highly-connected genes, TRs, and TFs: ERK1/2, CDH2, VIM, and PDGFRB. (B) Network 3. One can see that TGFB1 regulates two of the most important hubs—VEGF and HBEGFR—that have high connectivity. (C) Distribution of main gene functions for CGM3 of GBM1. | |
Angiogenic function of networks 1 and 3 of CGM3.
Highlighted in red in Fig. 3A and B are genes that participate in angiogenesis. The p-value of the angiogenic function of this module, estimated on the basis of activated genes, is 1.03 × 10−8. One can see eight such genes in network 1 (CGM3) and six in network 3 (CGM3).
Among the genes of network 1 are some well known mediators of angiogenesis, including ANGPT2 (angiopoietin 2), CDH2 (N-cadherin), CTSB (cathepsin B), CYR61 (cysteine-rich, angiogenic inducer 61), FBLN5 (fibulin 5), LAMA2 (laminin-alpha 2), and PDGFRB (platelet-derived growth factor receptor, beta polypeptide). These genes are regulated by TGFB1 and PDGF. The MEK–ERK1/2 pathway and Ap1 are also included in the group of genes that control the network.
Among the genes of network 3 (CGM3) are ARNTL (aryl hydrocarbon receptor nuclear translocator-like), CXCR4 (C-X-C chemokine receptor type 4), HBEGFR (heparin-binding EGF-like growth factor receptor), and LGALS1 (lectin, galactoside-binding 1). These genes are controlled by HBEGFR and VEGF, which in their turn are activated by TGFB1. AKT protein is involved in the expression of CXCR mRNA.30
Proliferation function of networks 1 and 2 of CGM1.
Proliferation is one of the most important functions of CGM1 of GBM1. Genes related to the proliferation of cells: ADAM12, ADAMTS9, ANXA1, CAV1, CAV2, CFB, CHI3L1, DAB2, ELF4, GALNS, GCH1, HOXB3, HOXB7, IFI30, IRF1, LEPREL1, LMNA, MYOF, MZF1, NAMPT, NELFE, PLA2G5, POSTN, SAT1, SOCS3, SOD2, UGCG, VAMP8, and VMP1 feature in this module. The p-value of this function for CGM1 is 1.9 × 10−8, which gives a clear functional assignment for this module GBM1. There are two main gene networks assigned to this module by the IPA program. Gene symbols in bold typeface are from network 1 (Fig. 4A), which is related to cell death, survival, and cellular movement. Italicized gene symbols are from network 2 (Fig. 4B), which is related to connective tissue development and function and organ development. The proliferation activity of CGM1 gene network 1 (Fig. 4A) is controlled by TGFB and PDGF in cooperation with MAPK, ERK1, and AP1 (a heterodimer most often containing JUN kinase and FOS). Brown-colored genes and gene connections are related to the proliferation process governed by this network (Fig. 4). AP1 is known to activate the proliferation of cells; the ETS gene in CGM1 is a well-known cancer-related TF that is responsible for proliferation and cell migration, along with ETS-related TF ELF4 (overexpressed in CGM1 of the GBM1). ETS activates the chitinase 3-like protein 1 (CHI3L1, also known as YKL-40) responsible for cancer progression and specifically for glioma cell migration.31 Note that PDGF protein, which is included in CGM1 network 1, controls specific mesenchyme proliferation and differentiation.
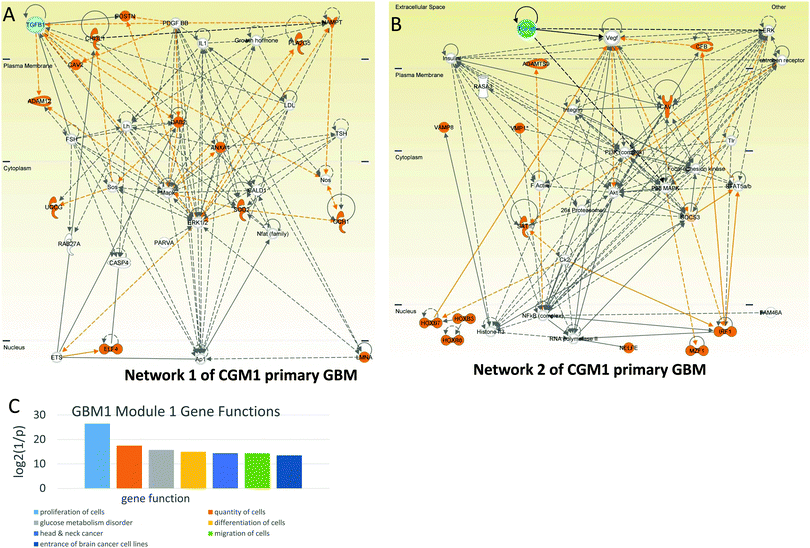 |
| Fig. 4 Cell proliferation-controlling CGM1s of GBM1. Networks of genes included in CGM1 taken for the analysis: cyan—TGFB1, orange—genes responsible for cell proliferation. (A) Network 1. TGFB1 is hub regulating a number of highly-connected genes: PDGFBB, DAB2, MAPK, and ERK1/2. (B) Network 2. One can see that TGFB1 regulates three of the most important hubs—VEGF, ERK, and p38 MAPK—that have high connectivity. (C) Distribution of main gene functions for CGM1 of GBM1. | |
Network 2 of CGM1 also contains a number of proliferation-related genes (these genes and their connections are colored brown in Fig. 4B). The top regulation of proliferation is conducted by TFGB1 and VEGF in cooperation with ERK, MAPK, AKT, and PI3K. Note that Ck2 kinase also activates the proliferation-related genes. It increases phosphorylation of the IRF (interferon-regulatory factor 1),32 SAT1,33 and HOXB7 (and most probably HPXB3 and HOX8) genes.32
Secondary GBM (GBM2).
The global network for GBM2 contains 13 CGMs, each including different genes from the aforementioned 586 gene set (Fig. 5). All these genes are differentially expressed with a ratio of cancer expression to normal average expression of greater than 1.5. The full network is presented in Fig. 5E. It includes 586 genes, 147 TFs, and 1694 TRs. Fig. 5A–D show the TF–TR–CGM networks “sliced” on consecutive levels of network connectivity. The close-up of the top levels is presented in Fig. S1B (ESI†).
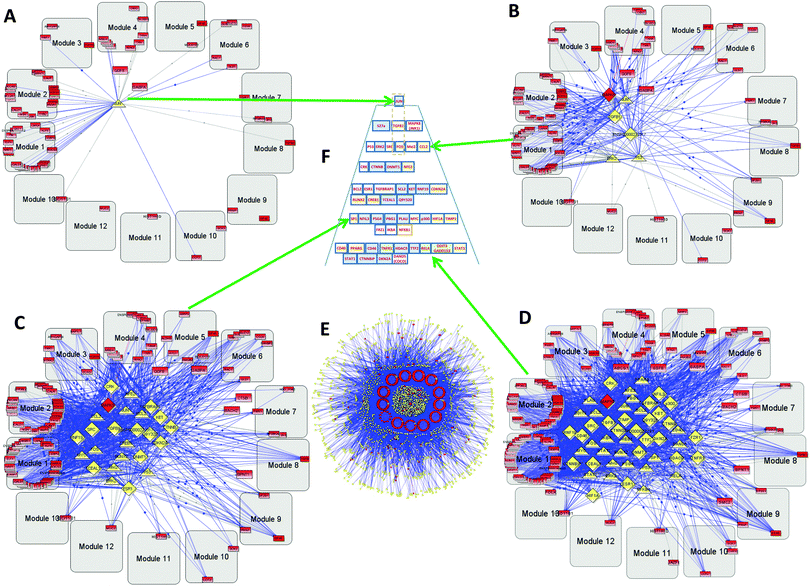 |
| Fig. 5 Secondary glioblastoma (GBM2) hierarchy: gray rounded squares—coherent gene modules (CGMs), red rectangles—genes, yellow triangles—transcription factors (TFs), yellow diamonds—transcription regulators (TRs). (A) Level 1 hierarchical network. Several genes included in the CGMs and the only top TR–JUN, (B) level 3 of hierarchy. (C) Level 9. (D) Level 12. These three panels (B and C) show gradual conversion from the top level (A) to the bottom (E). (E) Bottom level full network including 586 genes, 147 TFs, and 1694 TRs. (F) A hierarchical control pyramid of TFs and TRs that regulate all the CGMs. | |
The heat map of the most representative CGMs for GBM2 (Fig. S3, ESI†) shows that they play an important role in cell death. 146 genes of CGM1 are responsible for cell death (p = 2.93 × 10−8), necrosis (p = 4.58 × 10−7), and apoptosis (p = 9.01 × 10−7). 128 genes of CGM2 also participate in necrosis (p = 7.60 × 10−7), apoptosis (p = 5.17 × 10−6), and cell death (p = 2.23 × 10−5). A large number of the genes of CGM2 also trigger cell proliferation (p = 1.60 × 10−6).
The GBM2 controlling TF/TR pyramid (Fig. 5F) and CGMs are shown in Fig. 6. They were analyzed similarly to the GBM1 analysis reported above.
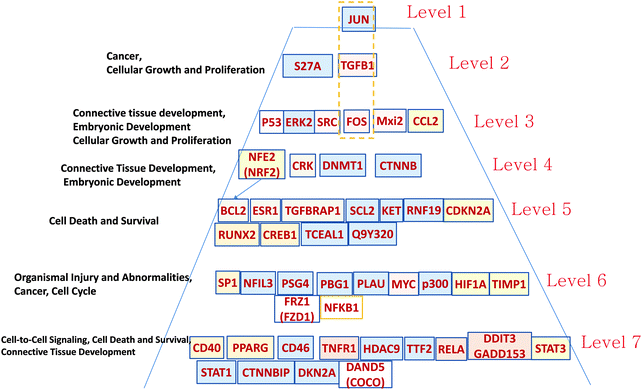 |
| Fig. 6 Hierarchical pyramid of secondary glioblastoma. This figure shows a close-up of the control pyramid shown in Fig. 5F, including TFs and TRs. Each level is responsible for the control of specific functions. | |
Level 1 of the TFs and TRs comprises JUN-kinase (JNK, MAPK8). Its interaction with FOS creates the most connected TF of GBM2.
Level 2 includes TGFB.
Level 3 includes p53 involved in the JNK (MAPK8) regulation pathway: MAPK8 phosphorylates p53 and also activates the TFs and TRs in a lower level. This level also includes FOS, which is activated by TGFB and interacts with MAPK8, and with the gene groups including RAS–RAF–MEK and MYC–MAX. Note that Mxi3 of the third level sustained ERK1/2 phosphorylation in the nucleus by preventing ERK1/2 from binding to phosphatases.34 Genes at this level are related to cellular growth and proliferation related to connective tissue development.
Level 4 includes TFs and TRs related to the AKT–mTOR–PI3K signaling pathway.18 v-Crk activates the PI3K/AKT pathway.19 Note also the DNMT1 methyltransferase gene that maintains DNA methylation status in tumor-suppressing genes. DNMT1 silencing significantly increases levels of apoptosis in cancer cells.35 NFE1 is involved in a set of cancer-related processes, including regulation of the anti-apoptotic BCL2.36 PI3K–AKT pathway genes affect NFE2.37 NFE2 also participates in oncogenesis, affecting the activity of the KRAS, BRAF, and MYC genes.38
Level 5 is also significantly related to the AKT–mTOR–PIR3 pathway. AKT activates CREB1, which regulates transcription of BCL2.20,39 CREB is a regulatory target for the protein kinase Akt/PKB;39 ESR1 activates PI3K.40,41 CDKN2A (cyclin-dependent kinase inhibitor 2A) is a tumor suppressor through binding of CDK4/6 and preventing their proper contact with these kinases. TGFBRAP1 (TRAP1) is involved in TGFB regulation.17
Level 6 includes NFIL3, overexpressed in various cancers, which supports tumor cell survival through repression of TRAIL.42 SP1 regulates known cancer-related genes: androgen receptor, TGFβ, c-Met, and others.43 It is interesting to note the presence of PSG4 (pregnancy specific beta-1-glycoprotein 4), which usually plays the role of immunomodulator to protect the growing fetus. We hypothesize that such a function can be mimicked to protect the growing tumor. PLAU (urokinase-type plasminogen activator) is involved in cancer cell proliferation. Tumor promoters HIF1A, MYC, p300, and NFKB1 are the well-known targets of anticancer medicine. FRZ1 mediates multidrug resistance in cancer through regulating of the WNT pathway.44
In Level 7 it is interesting to note the COCO (DAND5) gene that is known as a BMP (bone morphogenic protein–tumor suppressor) antagonist, which reactivates tumor cells leading to metastasis in lungs.45 It is possible that COCO plays a more important role in development of secondary GBM. This option has to be explored. It has been shown that PPARG plays a significant role in blocking the invasiveness of glioma cells, and that its agonists, such as pioglitazone (Actos), troglitazone (Rezulin), rosiglitazone (Avandia), and the experimental PPARγ (agonist ciglitazone46), can be useful for increasing its antitumor activity.
Above, we have presented some examples of important TFs and TRs from different layers of the pyramid. One can see that on all layers, known cancer-related proteins can be found. Our finding is that we have outlined that these genes can play a concerted role in secondary GBM, and the treatment of GBM would need to take an approach that targets most of them in order to effect outcomes.
Cell death-related functions of CGM1 of GBM2.
In spite of the aforementioned lack of samples, CGM1 of GBM2 shows very significant functional assignment. As assigned by IPA, the most important function for both CGM1 and CGM2 is “cell death,” with a p-value of 2.9 × 10−8 for CGM1 and a p-value of 2.35 × 10−7 for CGM2. There are a total of 37 genes in CGM1 and 29 genes in CGM2 that are assigned to the cell death function. IPA generated four networks for each module. CGM1 networks 4 and 2 and CGM2 networks 4 and 1 are statistically significant and were selected for further analysis. The CGM1 networks include 38 genes related to cell death. These genes are: ANG, ARHGAP18, CASP4, CASP7, CD44, CEBPD, CEBPG, CHI3L1, CTSS, CXCR4, CYR61, CYR64, DAB2, DRAM1, ELF4, FCGR2B, FSTL1, GZMA, HBEGFR, KLF6, LCP2, LGALS1, LGALS3, LMNA, LYZ, MICA, MSR1, NRP1, RAB27A, SAT1, SLC47A1, SOCS3, SOD2, TFEC, TNFSF12, TNFSF8, VIM, and YAP1. Genes with italicized symbols belong to network 4 (Fig. 7A), those in bold belong to network 2 (Fig. 7B).
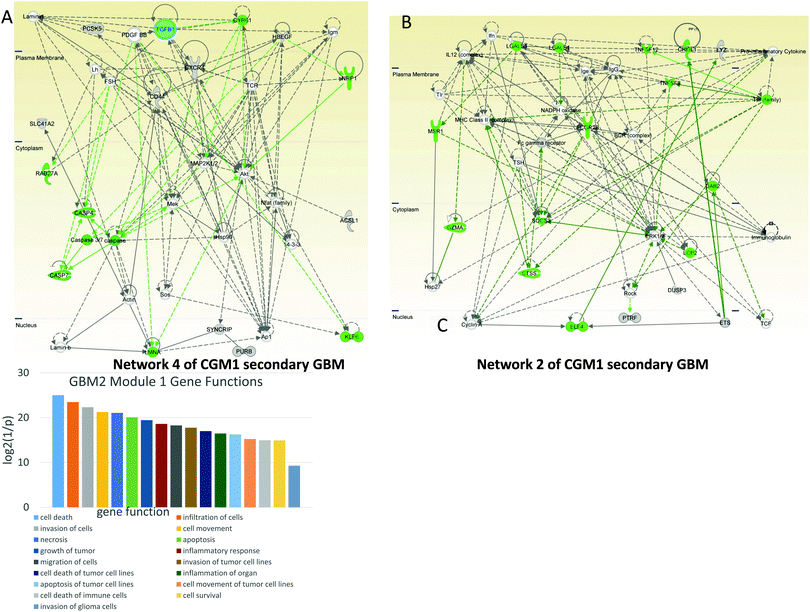 |
| Fig. 7 Cell death-controlling CGM1s of GBM2. Networks of genes included in CGM1 taken for the analysis: cyan—TGFB1, green—genes, TFs, and TRs responsible for cell death and survival. (A) Network 4. Cell death-related genes including caspases are regulated by TGFB1 and PDGFBB. (B) Network 2 is significantly related to cell death and the immune response; it includes tumor necrosis factor (TNF) and its family, which regulate hubs such as ERK1/2, IgG, SOCS3, and MHC Class II complex. (C) Distribution of main gene functions for CGM1 of GBM2. | |
In network 4 of CGM1 (Fig. 7A) cell death-related genes (colored green) including caspases are regulated by TGFB1 and PDGFBB. The latter decreases expression of CASP4.47 PDGFBB also increases the activity of proto-oncogene BCD (KLF6).48 The MEK gene decreases the apoptotic activity of caspase3/7.49 NRP1 (neuropilin 1) expression is increased by HBEGFR.50 Overall MEK, MAP2K1/2, and AKT participate significantly in cell death signaling.
Network 2 of CGM1 (Fig. 7B) is significantly related to cell death and immune responses. The BCR–ABL/GRB2 complex recruits SOS, and the BCR–ABL/GRB2/SOS complex activates the RAS, MEK, and MAPK pathways.51 The tumor necrosis factor (TNF)-related pathway sends a cell death signal that is overridden by ERK1/2 activation amplified by the same TNF genes.52 Activation of the RhoA/ROCK signaling pathway has been shown to contribute to dissociation-induced apoptosis of embryonic and neural stem cells.53 Expression of ROCK is increased by ERK1/2.54 At the same time, as a negative feedback loop, RHO kinase (ROCK) decreases the activity of ERK1/2.55 Studies have shown a significant role for the BCR complex that increases expression of MHC (major histocompatibility complex) class II gene56 and activates ERK1/2 protein.57 Moreover, FC-gamma receptor can be a target for anticancer therapy.58
CGM2 of the GBM2 contains 29 genes related to cell death: ANTXR2, ANXA1, B2M, BCL2A1, CALR, CASP4, CD8A, CDCP1, CEBPD, CHI3L1, DAB2, DPYD, EMP3, GLIPR1, GLRX, IL33, MSN, NAMPT, PECAM1, PLAGL1, PRKAA1, RAB32, RASSF3, SOD2, SWAP70, TGFB2, THBD, TYMP, VAV3. The genes in bold typeface are in network 1 and those italicized are in network 4. The cell death-related genes are colored green in both networks (Fig. 8A and B).
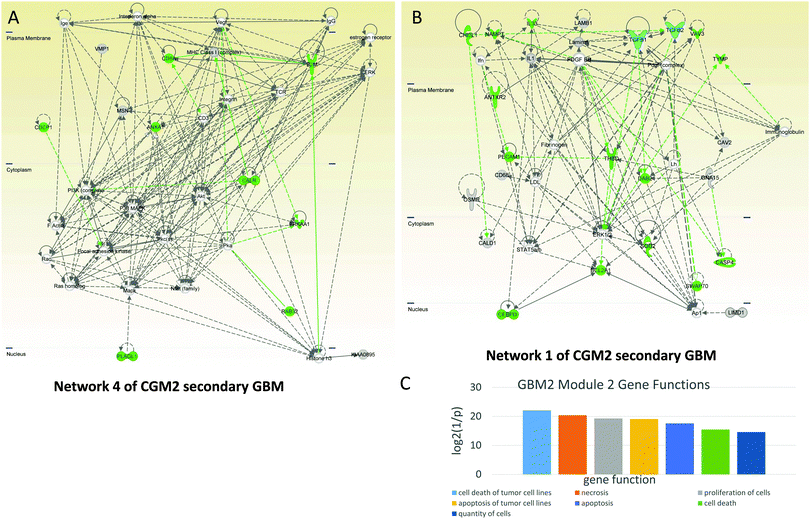 |
| Fig. 8 Cell death- and immune response-controlling CGM2 of GBM2. Networks of genes included in CGM2 taken for the analysis: cyan—TGFB1 and TGFB2 TRs, green—genes, TFs, and TRs responsible for cell death and survival. (A) Network 4. Cell death-related genes including caspases are regulated by TGFB1 and PDGFBB. It also contains immune response genes: MHC Class 1 and activating it B2M, immunoglobulins, TCR, and CD8A. (B) Networks 1’s cell death function is controlled by TGFB1 and TGFB2 in contact with PDGF. Complex Ap1 is involved in this network regulation. (C) Distribution of main gene functions for CGM2 of GBM2. | |
Network 1 of CGM2 of GBM2 (Fig. 8B) contains a number of cell death-related genes (highlighted in green). It is controlled by TGFB1 and TGFB2 in contact with PDGF. Complex Ap1 is also involved in this network regulation. CASP4 is a pro-apoptotic protein. CHI3L1 (chitinase 3-like 1, cartilage glycoprotein 39) promotes cancer cell proliferation, macrophage recruitment, and angiogenesis. PECAM1, known as cluster of differentiation 31 (CD31), is also involved in angiogenesis.
Inflammation and immune response functions of CGM1 of GBM2.
Network 2 of CGM1 of the GBM2 is significantly related to inflammation and the immune response. This can be explained by the presumption that the growing GBM2 activates the immune system, which tries to fight the growing tumor.
Network 4 of CGM2 of the GBM2 also contains immune response genes: MHC Class 1, activating it beta-2-microglobulin (B2M), immunoglobulins, T-cells receptor recognizing antigens (TCR), and CD8A (CD8 antigen is a cell surface glycoprotein found on most cytotoxic T lymphocytes that mediates efficient cell–cell interactions within the immune system). CD3 (together with other members of this family) couples the antigen response to intracellular pathways. ANXA1 (annexin A1) is also important for the immune response and inhibits the NFKB pathway that can lead to proliferation in cancer. It is interesting to note that interference of active CD3 by antibody increases activation of AKT.59 This indicates that activated CD3 can inhibit the AKT pathway, while MAPK is activated by the TCR complex.60
Correlation of top level network genes to survival and GBM subtypes
We looked at the top level genes in the networks identified above and tested whether they correlated with survival or subgroups in the Cancer Genome Atlas (TCGA) dataset (http://cancergenome.nih.gov). Using the SurExpress platform, we found that MAPK8, SRC, CCL2, and MAPK14 expression levels are related to patients' survival for general GBM. The volcano plots in the insets show that for MAPK8, SRC, and MAPK14, a higher expression level of these genes in the tumor correlates with the increase of survival, and for CCL this correlates with the decrease of survival (Fig. S4, ESI†). These results, contradicting the initial “naïve” hypothesis of direct correlation of oncogene expression with patient survival, are discussed in the Discussion section.
Using cBioPortal portal (http://www.cbioportal.org), we confirmed that MAPK8, SRC, and CCL2 expression levels were associated with the overall survival and disease-free status of GBM patients (Fig. S5, ESI†). Several top-level network genes also are correlated with survival in different molecular subtypes in the TCGA dataset (Fig. S6, ESI†). For TGFB1, TP53, CTNNB1, SRC, and MYC the expression of these genes corresponds to the increase of survival only for the neural subtype of GBM. For other subtypes we see various correlations that are interesting and may lead to more comprehensive understanding of the GBM molecular mechanism (Fig. S6, ESI†).
Strategies for drug administration
Based on our analysis of the genes controlling connections, one can make a decision according to the level of hierarchy of the genes involved in GBM. Table 1 shows the possible drugs to target the top five levels of the GBM1 pyramid (Fig. 2). Note that we propose drugs only for the selected genes of each level. The current state of drug development does not provide a reliable drug solution for all the genes elucidated in the pyramid but it gives a possible motivation for drug design based on the identification of specific genes in the top levels.
Table 1 Possible drugs for intervention at top levels of the GBM1 and GBM2 TFs and TRs hierarchy
Pyramid level |
Target gene |
Drug |
Approval status |
Description |
Ref. |
1 |
TGFB |
LY2157299 |
Trials II |
LY2157299 is an inhibitor of TGFB with IC50 = 56 nM. |
79
|
2 |
MAPK |
Trametinib and selumetinib |
Trametinib FDA approved |
The MAPK/Ras/Raf/Mek/Erk pathway is one of the targets. MEK inhibitors are trametinib and selumetinib. |
80
|
3 |
CTNNB |
Celecoxib |
FDA approved |
Celecoxib—the selective COX2 inhibitor celecoxib has been FDA approved in patients with FAP as it has been shown to reduce polyp formation. It has also been shown to reduce nuclear β-catenin (CTNNB1) in colorectal cancer cell lines similarly to other NSAIDs. |
81
|
3 |
SRC |
Dasatinib (Sprycel), saracatinib, bositinib |
Dasatinib FDA approved |
Constitutively-active v-Src [SRC] protein increases the activation of estrogen receptor alpha [ESR1] protein, which is mediated by Mekk [MAP3K1] protein. ESR1 can also be a target for drug intervention. |
82
|
Dasatinib is an oral multi-BCR/Abl and Src family tyrosine kinase inhibitor approved for first-line use in patients with chronic myelogenous leukemia (CML). |
83
|
3 |
Mxi2 |
Trametinib and selumetinib |
Trametinib FDA approved |
Mxi2 sustains ERK1/2 phosphorylation in the nucleus by preventing ERK1/2 binding to phosphatases. The MAPK/Ras/Raf/Mek/Erk pathway is one of the targets. MEK inhibitors are trametinib and selumetinib. |
84
|
4 |
BCL2 |
Obatoclax |
Trials I and II |
BCL2 inhibitor with Ki = 220 nM. |
85,86
|
4 |
CRK |
Tozasertib (VX-680) |
Trials I and II |
Tozasertib—Aurora kinase inhibitor that reduces phosphorylation of CRK. |
87
|
5 |
CDKN2A |
Everolimus deforolimus, temsirolimus, tacrolimus, ridaforolimus |
Everolimus FDA approved |
Akt increases activation of CDKN2A through the PI3K/AKT/mTOR pathway: everolimus (zortress, certican, afinitor), deforolimus, temsirolimus, tacrolimus, ridaforolimus. |
88
|
5 |
STAT3 |
Celecoxib |
FDA approved |
Celecoxib (a COX-2 inhibitor) with a novel function to target STAT3 (predictive STAT3 binding dissociation constant Kd = 8.6 μM). |
89
|
3 |
ERK2 |
Trametinib and selumetinib |
Trametinib FDA approved |
The MAPK/Ras/Raf/Mek/Erk pathway is one of the targets. MEK inhibitors are trametinib and selumetinib. |
80
|
4 |
ESR1 |
Tamoxifen |
|
Tamoxifen—ESR antagonist (selective estrogen receptor modulators (SERM)). |
90
|
Discussion
GBM is an aggressive cancer, characterized by a high degree of treatment resistance and a dismal prognosis. The lack of effective therapeutic options makes GBM a major unmet clinical need. In this study, we identified the GBM molecular networks using the unique multidimensional networks of TFs and TRs and coherent gene modules, including co-regulated genes. Such analysis made it possible for us to find unexpected points of intervention and combinations of drugs that serve as effective GBM therapeutics.
Using an integrative systemic approach, we have elucidated and examined hierarchical network pyramids including TRs and TFs that serve as driving forces for the activation of specific CGMs. The analysis of genes at different layers of these pyramids for primary and secondary GBMs has identified a vast majority of TFs and TRs related to cancers. Interestingly, some of the mediators that our analysis uncovered, such as PSG4 or COCO, have been shown to be associated with GBM for the first time. At the same time, data suggests their possible role in the pathogenesis of primary and/or secondary GBMs.
Our analysis based on the hierarchical pyramid revealed TGFB to have the highest connectivity of all molecules identified in the coherent gene modules for primary GBM. TGFB has been shown in unrelated studies to be important in the pathogenesis of cancers61 and specifically GBM,15,16 but it has never been demonstrated to be one of the key genes responsible for GBM. Inhibitors of TGFB are being developed and tested in various preclinical and clinical studies. One such inhibitor, LY2157299, a TGFβ receptor type I (R1) inhibitor, is currently being tested in a phase I/II clinical study for GBM, in combination with radiation and chemotherapy (ClinicalTrials.gov identifiers NCT01582269 and NCT01220271). This drug has potent antitumor activity in animal models of cancer. These observations suggest that the 3D network analysis we present here can identify therapeutically relevant molecules that can form effective clinical targets.
Following the identification of specific CGMs, we used detailed IPA-driven network analyses to pinpoint specific genes. We identified clusters comprising different genes linked to angiogenesis for both primary and secondary GBM. A cancer characterized by high vascularity, GBM shows overexpression of VEGF, an important regulator of tumor-associated angiogenesis. Anti-angiogenesis therapies targeting VEGF are being used in GBM with variable success. Monoclonal antibodies to VEGF,62 small molecule inhibitors of VEGFR,63 and decoy receptors inhibiting VEGF activity64 represent major therapeutic approaches currently being tested to target the VEGF/VEGFR pathway. Multiple phase I/II studies using the VEGF inhibitor bevacizumab (Avastin) for recurrent GBM show modest improvement in progression-free survival.65
In addition, in keeping with the highly-proliferative nature of GBM, one of the high scoring networks that IPA analysis identified for primary GBM was related to proliferation (CGM1). Two mutations commonly seen in most cancers including GBM are those of the retinoblastoma (RB) and p53 pathways.66,67 In addition to these two tumor suppressor pathway abnormalities, GBM also features increased expression and/or activity of mitogens and associated membrane receptors with their downstream signaling pathways, such as MAPK, PI3K/PTEN, and different RTKs.1 Not surprisingly, in our analyses, one proliferative gene network in primary GBM (network 1 of CGM1) was controlled by TGFB and PDGF, in cooperation with the MAPK pathway. Similarly, network 2 of CGM1 in primary GBM comprised of proliferation-related genes that were regulated by TGFB1 and VEGF in cooperation with the MAPK pathway, AKT and PI3K. These data from our network analyses in primary GBM point to the importance of the TGFB and VEGF molecules in regulating proliferation, an important phenotypic feature of GBM.
On the other hand, network analyses on secondary GBM revealed a network that was linked to cell death (network 4 of CGM1) and comprised of 38 genes related to cell death or apoptosis. There are several molecular mediators of apoptosis, prominent among which is the Bcl2 family of proteins comprising both pro- and anti-apoptotic members. It has been shown that the treatment resistance seen in recurrent GBM is primarily related to an anti-apoptotic shift in the dynamics of the Blc2 family members.68 However, counter-intuitively, increased expression of anti-apoptotic Bcl2 members has also been shown to improve prognosis in GBM.69 Interpretation of data on apoptotic mediators may not be straightforward and may be influenced by different signaling mediators. In our study, TGFB1 and PDGFB regulated many of these cell death-related genes, including caspases and other proto-oncogenes. The net effect was reduced apoptosis in cells, leading to uncontrolled growth. Secondary GBM was also characterized by the presence of a set of genes that was significantly related to immune response, in addition to cell death (network 2 of CGM1). Together, our network analysis data are in line with the high inflammation, immune response, and anti-apoptotic effects observed in GBM.
Notably, when we analyzed survival data in GBM patients with specific genetic aberrations (TCGA data analyses, Fig. S4–S6, ESI†), we observed that low expression of MAPK8, MAPK14, and SRC correlated with high risk and shorter overall survival in patients. On the other hand, low expression of CCL2 correlated with higher risk and shorter overall survival. The apparent discrepancy wherein high-risk patients (shorter overall survival) demonstrated low MAPK8 and MAPK14 expression can be explained by the fact that highly-proliferative cells have higher expression of these kinases. However, these cells, though more aggressive, are preferentially targeted by anti-proliferative agents, resulting in elimination of cell populations with high kinase expression. Furthermore, these kinases are also targets of many molecularly-targeted agents in clinical practice. As a consequence, though high MAPK8 and MAPK14 expression confers an aggressive phenotype (supported by our data here), these cells are better targeted by current therapies and hence may demonstrate an apparent contradiction in the form of longer survival in patients with higher expression.
Conclusions
Cancer, particularly GBM, is a complex disease that involves the interplay of various genes and proteins. Understanding this complexity will help decipher the exact molecular mechanisms responsible for GBM and will facilitate optimal therapeutic targeting. However, this complexity is difficult to comprehend and unlikely to be understood solely by focusing on individual molecules or even single pathways. Our approach of identifying major multilevel hierarchical networks and coherent gene modules within these networks would improve our understanding for clinical translation. This analysis is ready to be introduced in concrete medical practice.
A global view of the network of signaling molecules presents a viable mode of studying cancer. We believe that this approach will lead to the construction of informative disease models that facilitate development of novel strategies for clinical management, including optimal individualized therapies for different patients.
Methods and materials
The strategy
Our strategy is based on application of the contemporary systems-biology programs to the results of microarray data analyses, and consists of the following steps: (A) initial processing of microarray data (which continues by the three branches); (B) functional and hierarchical network analysis and network visualization; (C) elucidation of genes associated with brain cancers, namely, primary and secondary glioblastomas and analysis of pathways and networks containing these genes; and (D) development strategies of drug administration.
Step A includes: (1) calculation of mean from replicates, (2) selecting a set of genes by significance, and (3) calculating ratios of cancer expression value to normal average expression value. In step B we selected the most higher differentially expressed genes and analyzed them with the BiologicalNetworks program,9–11 creating an integrative multilevel hierarchical pyramid of coherent gene modules, TFs and TRs. In step C we elucidated and analyzed the signaling networks and pathways generated from genes extracted from the multilevel hierarchical pyramid using Ingenuity Pathway Analysis software (IPA®, Ingenuity Systems Inc., Redwood City, Calif.). Outcomes of this analysis allowed us to elucidate specific gene–TF interactions for each glioblastoma and to catch sight of differences between them. Analysis of obtained pathways with IPA led to listing the biological processes characteristic of each of them. Step D showed further directions for developing strategies of drug administration based on analysis of hierarchical networks.
Sample preparation, microarray and data analysis
We used microarray data published by Michal Grzmil and colleagues.70 As mentioned in ref. 70, primary glioblastoma tissues were obtained from the operating room in line with the guidelines of the Ethical Committee of the University Hospitals of Basel and Dusseldorf, and were processed as described by Maier and co-authors.71 The microarray was created by RNA hybridization, as described in ref. 72. The Affymetrix GeneChip® Human Genome U133 Plus 2.0 Array (Santa Clara, Calif., USA), and its data was deposited in the Gene Expression Omnibus (GEO) database (entry GEO15824, http://www.ncbi.nlm.nih.gov/geo/query/acc.cgi?acc=GSE15824). The microarray contains 54
675 probes and three sample groups: 12 samples from primary glioblastoma (GBM1), 3 samples from secondary (GBM2), and 2 samples from normal brains (Norm) (other samples present in the microarray were omitted due to being beyond the study scope).
Because the data distribution is non-normal, the data were subjected to a one-factor Kruskal–Wallis nonparametric test8 with MultiExperiment Viewer (MeV), v. 4.8.1.73 Like most nonparametric tests, it is performed on ranked data. The test statistic for a Kruskal–Wallis test is given by:
H = ((12/(N × (N + 1))) × (∑(Ti2/ni))) − 3 × (N + 1) |
where
N is the total number of participants, so
N = 17, and
ni is the number of samples in each group, so
n1 = 12,
n2 = 3, and
n3 = 2;
Ti is an average rank for the all samples. Significance was based on an input alpha:
p < 0.025.
Gene–TF network elucidation
To find genes that are regulated with the same transcription factors (TFs) and novel potential regulators of significant genes and TFs in glioblastoma, we used the BiologicalNetworks program,9–11 an environment for integrative systems biology research. Genes exhibiting correlated expression patterns may be co-regulated by common TFs or may be functionally related, forming a functional module or a molecular complex.74 Finding the transcription factor binding sites (TFBSs) involves a number of problems.75 For example, because of the ambiguity of binding TFs, the number of false positives and false negatives can be unexpectedly high.75 Evolutionary conserved motifs or regions in the promoters of the homologous genes may function as TFBSs. In an attempt to identify those genes that are considered significant for glioblastoma, a systematic, large-scale search for TFs, their respective binding sites (i.e., TFBSs), and for a phylogenetically conserved promoter structures was conducted.11 Starting with a set of gene pairs found to be differentially expressed in glioblastoma and normal tissues, BiologicalNetworks identified orthologous promoter regions from three species (H. sapiens, Mus musculus, and Rattus norvegicus), using a systematic approach. TFBSs common to promoter regions across species were first identified for individual genes. Using a modified phylogenetic footprinting method, a search was conducted for TFBSs that are enriched in the region of 6000 bases upstream of each transcription start site to 500 bases downstream of each transcription start site of every gene in the gene pairs found, and are conserved in the human, mouse, and rat genomes.
Binding sites and corresponding TFs were filtered for p < 10−3 and examined for consistency.11
Gene–TF networks and pathway analysis
For network and pathway analysis the Ingenuity Interactive Pathway Analysis (IPA) package (Ingenuity Systems, Inc., Redwood City, Calif., USA) was used.
Statistical analysis
Because the microarray data has a non-normal distribution and often a small sample size (in our case 2, 3, and 12), we used the Kruskal–Wallis nonparametric test8—a one-factor analysis for multiple samples with non-normal distributions. In BiologicaNetworks, for Gene Ontology term overrepresentation analysis the Fisher's exact test was used.76 To build correlation networks the Pearson's correlations were calculated.76
Cancer genome atlas (TCGA) analysis
We investigated whether the expression levels of genes identified above in network analysis correlated with GBM patients’ clinical outcomes by SurvExpress.77 Biomarker and Cox Survival Analysis were censored by survival months, and patients’ survival data were stratified by death. Genes in the GBM pyramid levels 1, 2, and 3 were analyzed, in total 538 samples of TCGA.
The Kaplan–Meier survival curve comparison and the Cox proportional-hazards model were used to investigate the gene expression level and patients’ outcome according to their molecular subtypes.78 The Glioblastoma Bio Discovery Portal (GBM-BioDP) was queried and visualized for association of gene expression levels with clinical outcomes. The mRNA levels were defined in 3-platform aggregates and participants were patients in the Verhaak extended database from TCGA (http://robtcga.nci.nih.gov).
We also investigated Kaplan–Meier Overall Survival and Disease-Free Survival data comparing gene expression levels with patient survival and disease-free survival by cBioPortal (http://www.cbioportal.org). The cBioPortal is developed and maintained by the Computational Biology (cBio) Center at Memorial Sloan-Kettering Cancer Center. We analyzed and grouped patient data according to their gene expression levels as: up-regulated, normal and down-regulated (mRNA/miRNA expression Z-scores > 1, >−1 and <1, <−1, respectively).
Acronyms and abbreviations
ANGPT2 | Angiopoietin 2 |
ANXA1 | Annexin A1 |
ARNTL | Aryl hydrocarbon receptor nuclear translocator-like |
B2M | beta-2 microglobulin |
BMP | Bone morphogenic protein |
C–L | Centrality–lethality |
CD8A | Cluster of differentiation 8A |
CD31 | Cluster of differentiation 31 |
CDH2 | Neural cadherin (N-cadherin) |
CDKN2A | Cyclin-dependent kinase inhibitor 2A |
CGM | Coherent gene module |
CHI3L1 | Chitinase 3-like protein 1 |
CTSB | Cathepsin B |
CSCR4 | C-X-C Chemokine receptor type 4 |
CYR61 | Cysteine-rich, angiogenic inducer, 61 |
DB | Database |
EMT | Epithelial–mesenchymal transformation |
FBLN5 | Fibulin 5 |
GAM | Glioma-associated microglia/macrophages |
GBM | Glioblastoma multiform |
GBM1 | Primary glioblastoma |
GBM2 | Secondary glioblastoma |
GEO | Gene expression omnibus |
HBEGFR | Heparin-binding EGF-like growth factor receptor |
IPA | Interactive pathway analysis |
JMJD6 | Jumonji domain containing 6 |
LAMA2 | Laminin, alpha 2 |
LGALS1 | Lectin, galactoside-binding, soluble, 1 |
MeV | MultiExperiment viewer (bioconductor) |
MF | Macrophage |
NRP1 | Neuropilin 1 |
NormAVE | Average value for the Normal sample group |
PDGFRB | Platelet-derived growth factor receptor, beta polypeptide |
PLAU | Urokinase-type plasminogen activator |
PSG4 | Pregnancy specific beta-1-glycoprotein 4 |
TDEC | Tumor-derived endothelial cell |
TCR | T cell receptor |
TF | Transcription factor |
TGFB (TGFβ) | Transforming growth factor, beta |
TNF | Tumor necrosis factor |
TR | Transcription regulator |
VEGF | Vascular endothelial growth factor |
Acknowledgements
We gratefully acknowledge support by grants from Voices Against Brain Cancer to S. Kesari. We cordially thank Michael Baitaluk and Sergey Kozhenkov from San Diego Supercomputer Center at University of California, San Diego, for their generous support with the BiologicalNetworks program and their responsiveness.
References
- F. B. Furnari, T. Fenton, R. M. Bachoo, A. Mukasa, J. M. Stommel, A. Stegh, W. C. Hahn, K. L. Ligon, D. N. Louis, C. Brennan, L. Chin, R. A. DePinho and W. K. Cavenee, Malignant astrocytic glioma: genetics, biology, and paths to treatment, Genes Dev., 2007, 21, 2683–2710 CrossRef CAS PubMed.
- S. Kesari, Understanding glioblastoma tumor biology: the potential to improve current diagnosis and treatments, Semin. Oncol., 2011, 38(suppl 4), S2–S10 CrossRef PubMed.
- Y. P. Ramirez, J. L. Weatherbee, R. T. Wheelhouse and A. H. Ross, Glioblastoma multiforme therapy and mechanisms of resistance, Pharmaceuticals, 2013, 6, 1475–1506 CrossRef PubMed.
- P. Wesseling, D. J. Ruiter and P. C. Burger, Angiogenesis in brain tumors; pathobiological and clinical aspects, J. Neuro-Oncol., 1997, 32, 253–265 CrossRef CAS.
- P. Y. Wen and S. Kesari, Malignant Gliomas in Adults, N. Engl. J. Med., 2008, 359, 492–507 CrossRef CAS PubMed.
- D. J. Brat and E. J. Van Meir, Vaso-occlusive and pro-thrombotic mechanisms associated with tumor hypoxia, necrosis and accelerated growth in glioblastoma, Lab. Invest., 2004, 84, 397–405 CrossRef CAS PubMed.
- F. Lefranc, J. Brotchi and R. Kiss, Possible future issues in the treatment of glioblastomas: special emphasis on cell migration and the resistance of migrating glioblastoma cells to apoptosis, J. Clin. Oncol., 2005, 23, 2411–2422 CrossRef CAS PubMed.
- W. H. Kruskal and W. A. Wallis, Use of ranks in one-criterion variance analysis, J. Am. Stat. Assoc., 1952, 47, 583–621 CrossRef.
- S. Kozhenkov, Y. Dubinina, M. Sedova, A. Gupta, J. Ponomarenko and M. Baitaluk, BiologicalNetworks 2.0—an integrative view of genome biology data, BMC Bioinformatics, 2010, 11, 610 CrossRef PubMed.
- M. Baitaluk, S. Kozhenkov, Y. Dubinina and J. Ponomarenko, IntegromeDB: an integrated system and biological search engine, BMC Genomics, 2012, 13, 35 CrossRef CAS PubMed.
- I. F. Tsigelny, V. L. Kouznetsova, M. Baitaluk and J.-P. Changeux, A hierarchical coherent-gene-group model for brain development, Genes, Brain and Behavior, 2013, 12, 147–165 CrossRef CAS PubMed.
- H. Jeong, S. P. Mason, A. L. Barabasi and Z. N. Oltvai, Lethality and centrality in protein networks, Nature, 2001, 411, 41–42 CrossRef CAS PubMed.
- J. Kim, I. Kim, S. K. Han, J. U. Bowie and S. Kim, Network rewiring is an important mechanism of gene essentiality change, Sci. Rep., 2012, 2, 900 Search PubMed.
- M. R. J. Carlson, B. Zhang, Z. Fang, P. S. Mischel, S. Horvath and S. F. Nelson, Gene connectivity, function, and sequence conservation: predictions from modular yeast co-expression networks, BMC Genomics, 2006, 7, 40 CrossRef PubMed.
- W. Wick, U. Naumann and M. Weller, Transforming growth factor-beta: a molecular target for the future therapy of glioblastoma, Curr. Pharm. Des., 2006, 12, 341–349 CrossRef CAS.
- A. Bruna, R. S. Darken, F. Rojo, A. Ocaña, S. Peñuelas, A. Arias, R. Paris, A. Tortosa, J. Mora, J. Baselga and J. Seoane, High TGFbeta-Smad activity confers poor prognosis in glioma patients and promotes cell proliferation depending on the methylation of the PDGF-B gene, Cancer Cell, 2007, 11, 147–160 CrossRef CAS PubMed.
- J. U. Wurthner, D. B. Frank, A. Felici, H. M. Green, Z. Cao, M. D. Schneider, J. G. McNally, R. J. Lechleider and A. B. Roberts, Transforming growth factor-beta receptor-associated protein 1 is a Smad4 chaperone, J. Biol. Chem., 2001, 276, 19495–19502 CrossRef CAS PubMed.
-
X. He, Identification
of the novel Akt2 interacting proteins RPS25 and eIF3c: characterization of interaction and physiological consequences, Doctoral Degree Dissertation, Department of Biology, Eberhard Karls University of Tübingen, Germany, 2007 Search PubMed.
- T. Akagi, K. Murata, T. Shishido and H. Hanafusa, v-Crk activates the phosphoinositide 3-kinase/AKT pathway by utilizing focal adhesion kinase and H-Ras, Mol. Cell. Biol., 2002, 22, 7015–7023 CrossRef CAS.
- S. Pugazhenthi, A. Nesterova, C. Sable, K. A. Heidenreich, L. M. Boxer, L. E. Heasley and J. E. Reusch, Akt/protein kinase B up-regulates Bcl-2 expression through cAMP-response element-binding protein, J. Biol. Chem., 2000, 275, 10761–10766 CrossRef CAS PubMed.
- A. Wu, J. Wei, L. Y. Kong, Y. Wang, W. Priebe, W. Qiao, R. Sawaya and A. B. Heimberger, Glioma cancer stem cells induce immunosuppressive macrophages/microglia, Neurooncology, 2010, 12, 1113–1115 CAS.
- J. W. Pollard, Tumour-educated macrophages promote tumour progression and metastasis, Nat. Rev. Cancer, 2004, 4, 71–78 CrossRef CAS PubMed.
- M. Sliwa, D. Markovic, K. Gabrusiewicz, M. Synowitz, R. Glass, M. Zawadzka, A. Wesolowska, H. Kettenmann and B. Kaminska, The invasion promoting effect of microglia on glioblastoma cells is inhibited by cyclosporin A, Brain, 2007, 130, 476–489 CrossRef PubMed.
- G. J. Baay-Guzman, I. G. Bebenek, M. Zeidler, R. Hernandez-Pando, M. I. Vega, E. A. Garcia-Zepeda, G. Antonio-Andres, B. Bonavida, M. Riedl, E. Kleerup, D. P. Tashkin, O. Hankinson and S. Huerta-Yepez, HIF-1 expression is associated with CCL2 chemokine expression in airway inflammatory cells: implications in allergic airway inflammation, Respir. Res., 2012, 13, 60 CrossRef CAS PubMed.
- A. E. Hinojosa, B. Garcia-Bueno, J. C. Leza and J. L. M. Madrigal, CCL2/MCP-1 modulation of microglial activation and proliferation, J. Neuroinflammation, 2011, 8, 77 CrossRef CAS PubMed.
- J. Wei, K. Gabrusiewicz and A. Heimberger, The controversial role of microglia in malignant gliomas, Clin. Dev. Immunol., 2013, 2013, 285246 Search PubMed.
- L. C. Lin, S. L. Hsu, C. L. Wu, W. C. Liu, H. T. Chiu and C. M. Hsueh, Peroxisome proliferator-activated receptor Gamma responsible for TGFβ-induced epithelial mesenchymal transition (EMT) and tumor invasion of NSCLC cells (H460), Eur. J. Cancer Suppl., 2009, 7, 124 CrossRef.
- E. H. Nam, Y. Lee, Y. K. Park, J. W. Lee and S. Kim, ZEB2 upregulates integrin α5 expression through cooperation with Sp1 to induce invasion during epithelial–mesenchymal transition of human cancer cells, Carcinogenesis, 2012, 33, 563–571 CrossRef CAS PubMed.
- S. El Hallani, B. Boisselier, F. Peglion, A. Rousseau, C. Colin, A. Idbaih, Y. Marie, K. Mokhtari, J. L. Thomas, A. Eichmann, J. Y. Delattre, A. J. Maniotis and M. Sanson, A new alternative mechanism in glioblastoma vascularization: tubular vasculogenic mimicry, Brain, 2010, 133, 973–982 CrossRef PubMed.
- R. J. Phillips, J. Mestas, M. Gharaee-Kermani, M. D. Burdick, A. Sica, J. A. Belperio, M. P. Keane and R. M. Strieter, Epidermal growth factor and hypoxia-induced expression of CXC chemokine receptor 4 on non-small cell lung cancer cells is regulated by the phosphatidylinositol 3-kinase/PTEN/AKT/mammalian target of rapamycin signaling pathway and activation of hypoxia inducible factor-1alpha, J. Biol. Chem., 2005, 280, 22473–22481 CrossRef CAS PubMed.
- S. K. Singh, R. Bhardwaj, K. M. Wilczynska, C. I. Dumur and T. Kordula, A complex of nuclear factor I-X3 and STAT3 regulates astrocyte and glioma migration through the secreted glycoprotein YKL-40, J. Biol. Chem., 2011, 286, 39893–39903 CrossRef CAS PubMed.
- F. Meggio and L. A. Pinna, One-thousand-and-one substrates of protein kinase
CK2?, FASEB J., 2003, 17, 349–368 CrossRef CAS PubMed.
- L. Bordin, C. Vargiu, S. Colombatto, G. Clari, G. Testore, A. Toninello and M. A. Grillo, Casein kinase 2 phosphorylates recombinant human spermidine/spermine N1-acetyltransferase on both serine and threonine residues, Biochem. Biophys. Res. Commun., 1996, 229, 845–851 CrossRef CAS PubMed.
- B. Casar, J. Rodríguez, G. Gibor, R. Seger and P. Crespo, Mxi2 sustains ERK1/2 phosphorylation in the nucleus by preventing ERK1/2 binding to phosphatases, Biochem. J., 2012, 441, 571–578 CrossRef CAS PubMed.
- Y. Zhang, F. Q. Chen, Y. H. Sun, S. Y. Zhou, T. Y. Li and R. Chen, Effects of DNMT1 silencing on malignant phenotype and methylated gene expression in cervical cancer cells, J. Exp. Clin. Cancer Res., 2011, 30, 98 CrossRef CAS PubMed.
- S. K. Niture and A. K. Jaiswal, Nrf2 protein up-regulates antiapoptotic protein Bcl-2 and prevents cellular apoptosis, J. Biol. Chem., 2012, 287, 9873–9886 CrossRef CAS PubMed.
- P. Shelton and A. K. Jaiswal, The transcription factor NF-E2-related Factor 2 (Nrf2): a protooncogene?, FASEB J., 2013, 27, 414–423 CrossRef CAS PubMed.
- R. M. Perera and N. Bardeesy, Cancer: when antioxidants are bad, Nature, 2011, 475, 43–44 CrossRef CAS PubMed.
- K. Du and M. Montminy, CREB is a regulatory target for the protein kinase Akt/PKB, J. Biol. Chem., 1998, 273, 32377–32379 CrossRef CAS PubMed.
- T. Simoncini, A. Hafezi-Moghadam, D. P. Brazil, K. Ley, W. W. Chin and J. K. Liao, Interaction of oestrogen receptor with the regulatory subunit of phosphatidylinositol-3-OH kinase, Nature, 2000, 407, 538–541 CrossRef CAS PubMed.
- P. Mannella and R. D. Brinton, Estrogen receptor protein interaction with phosphatidylinositol 3-kinase leads to activation of phosphorylated Akt and extracellular signal-regulated kinase 1/2 in the same population of cortical neurons: a unified mechanism of estrogen action, J. Neurosci., 2006, 26, 9439–9447 CrossRef CAS PubMed.
- M. Keniry, M. M. Pires, S. Mense, C. Lefebvre, B. Gan, K. Justiano, Y. K. Lau, B. Hopkins, C. Hodakoski, S. Koujak, J. Toole, F. Fenton, A. Calahan, A. Califano, R. A. DePinho, M. Maurer and R. Parsons, Survival factor NFIL3 restricts FOXO-induced gene expression in cancer, Genes Dev., 2013, 27, 916–927 CrossRef CAS PubMed.
- U. T. Sankpal, S. Goodison, M. Abdelrahim and R. Basha, Targeting Sp1 transcription factors in prostate cancer therapy, Med. Chem., 2011, 7, 518–525 CrossRef CAS.
- H. Zhang, X. Zhang, X. Wu, W. Li, P. Su, H. Cheng, L. Xiang, P. Gao and G. Zhou, Interference of Frizzled 1 (FZD1) reverses multidrug resistance in breast cancer cells through the Wnt/β-catenin pathway, Cancer Lett., 2012, 323, 106–113 CrossRef CAS PubMed.
- H. Gao H, G. Chakraborty, A. P. Lee-Lim, Q. Mo, M. Decker, A. Vonica, R. Shen, E. Brogi, A. H. Brivanlou and F. G. Giancotti, The BMP inhibitor Coco reactivates breast cancer cells at lung metastatic sites, Cell, 2012, 150, 764–779 CrossRef PubMed.
- J. E. Cobb, S. G. Blanchard, E. G. Boswell, K. K. Brown, P. S. Charifson, J. P. Cooper, J. L. Collins, M. Dezube, B. R. Henke, E. A. Hull-Ryde, D. H. Lake, J. M. Lenhard, W. Oliver Jr, J. Oplinger, M. Pentti, D. J. Parks, K. D. Plunket and W. Q. Tong, N-(2-Benzoylphenyl)-L-tyrosine PPARgamma agonists. 3. Structure–activity relationship and optimization of the N-aryl substituent, J. Med. Chem., 1998, 41, 5055–5069 CrossRef CAS PubMed.
- N. Kaplan-Albuquerque, Y. E. Bogaert, V. Van Putten, M. C. Weiser-Evans and R. A. Nemenoff, Patterns of gene expression differentially regulated by platelet-derived growth factor and hypertrophic stimuli in vascular smooth muscle cells: markers for phenotypic modulation and response to injury, J. Biol. Chem., 2005, 280, 19966–19976 CrossRef CAS PubMed.
- J. W. Tullai, M. E. Schaffer, S. Mullenbrock, S. Kasif and G. M. Cooper, Identification of transcription factor binding sites upstream of human genes regulated by the phosphatidylinositol 3-kinase and MEK/ERK signaling pathways, J. Biol. Chem., 2004, 279, 20167–20177 CrossRef CAS PubMed.
- R. R. Mattingly, J. M. Kraniak, J. T. Dilworth, P. Mathieu, B. Bealmear, J. E. Nowak, J. A. Benjamins, M. A. Tainsky and J. J. Reiners, The mitogen-activated protein kinase/extracellular signal-regulated kinase kinase inhibitor PD184352 (CI-1040) selectively induces apoptosis in malignant schwannoma cell lines, J. Pharmacol. Exp. Ther., 2006, 316, 456–465 CrossRef CAS PubMed.
- P. Kurschat, D. Bielenberg, M. Rossignol-Tallandier, A. Stahl and M. Klagsbrun, Neuron restrictive silencer factor NRSF/REST is a transcriptional repressor of neuropilin-1 and diminishes the ability of semaphorin 3A to inhibit keratinocyte migration, J. Biol. Chem., 2006, 281, 2721–2729 CrossRef CAS PubMed.
- D. Cilloni and G. Saglio, Molecular pathways: BCR-ABL, Clin. Cancer Res., 2012, 18, 930–937 CrossRef CAS PubMed.
- S. E. Tran, T. H. Holmstrom, M. Ahonen, V. M. Kahari and J. E. Eriksson, MAPK/ERK overrides the apoptotic signaling from Fas, TNF, and TRAIL receptors, J. Biol. Chem., 2001, 276, 16484–16490 CrossRef CAS PubMed.
- L. Zhang, J. M. Valdez, B. Zhang, L. Wei, J. Chang and L. Xin, ROCK inhibitor Y-27632 suppresses dissociation-induced apoptosis of murine prostate stem/progenitor cells and increases their cloning efficiency, PLoS One, 2011, 6, e18271 CAS.
- E. Sahai, M. F. Olson and C. J. Marshall, Cross-talk between Ras and Rho signalling pathways in transformation favours proliferation and increased motility, EMBO J., 2001, 20, 755–766 CrossRef CAS PubMed.
- S. Zhou, A. Schmelz, T. Seufferlein, Y. Li, J. Zhao and M. G. Bachem, Molecular mechanisms of low intensity pulsed ultrasound in human skin fibroblasts, J. Biol. Chem., 2004, 279, 54463–54469 CrossRef CAS PubMed.
- R. J. Benschop, D. Melamed, D. Nemazee and J. C. Cambier, Distinct signal thresholds for the unique antigen receptor-linked gene expression programs in mature and immature B cells, J. Exp. Med., 1999, 190, 749–756 CrossRef CAS.
- A. Jacob, D. Cooney, M. Pradhan and K. M. Coggeshall, Convergence of signaling pathways on the activation of ERK in B cells, J. Biol. Chem., 2002, 277, 23420–23426 CrossRef CAS PubMed.
- P. M. Hogarth and G. A. Pietersz, Fc receptor-targeted therapies for the treatment of inflammation, cancer and beyond, Nat. Rev. Drug Discovery, 2012, 11, 311–331 CrossRef CAS PubMed.
- K. W. Kim, M. L. Cho, M. K. Park, C. H. Yoon, S. H. Park, S. H. Lee and H. Y. Kim, Increased interleukin-17 production via a phosphoinositide 3-kinase/Akt and nuclear factor kappaB-dependent pathway in patients with rheumatoid arthritis, Arthritis Res., 2005, 7, R139–R148 CrossRef CAS PubMed.
- A. S. Naura, H. Kim, J. Ju, P. C. Rodriguez, J. Jordan, A. D. Catling, B. M. Rezk, Z. Y. Abd Elmageed, K. Pyakurel, A. F. Tarhuni, M. Q. Abughazleh, Y. Errami, M. Zerfaoui, A. C. Ochoa and A. H. Boulares, Minocycline blocks asthma-associated inflammation in part by interfering with the T cell receptor-nuclear factor κB-GATA-3-IL-4 axis without a prominent effect on poly(ADP-ribose) polymerase, J. Biol. Chem., 2013, 288, 1458–1468 CrossRef CAS PubMed.
- R. Derynck, R. J. Akhurst and A. Balmain, TGF-beta signaling in tumor suppression and cancer progression, Nat. Genet., 2001, 29, 117–129 CrossRef CAS PubMed.
- F. Winkler, S. V. Kozin, R. T. Tong, S. S. Chae, M. F. Booth, I. Garkavtsev, L. Xu, D. J. Hicklin, D. Fukumura, E. Di Tomaso, L. L. Munn and R. K. Jain, Kinetics of vascular normalization by VEGFR2 blockade governs brain tumor response to radiation: role of oxygenation, angiopoietin-1, and matrix metalloproteinases, Cancer Cell, 2004, 6, 553–563 CAS.
- T. T. Batchelor, A. G. Sorensen, E. Di Tomaso, W. T. Zhang, D. G. Duda, K. S. Cohen, K. R. Kozak, D. P. Cahill, P. J. Chen, M. Zhu, M. Ancukiewicz, M. M. Mrugala, S. Plotkin, J. Drappatz, D. N. Louis, P. Ivy, D. T. Scadden, T. Benner, J. S. Loeffler, P. Y. Wen and R. K. Jain, AZD2171, a pan-VEGF receptor tyrosine kinase inhibitor, normalizes tumor vasculature and alleviates edema in glioblastoma patients, Cancer Cell, 2007, 11, 83–95 CrossRef CAS PubMed.
- J. Folkman, Angiogenesis: an organizing principle for drug discovery?, Nat. Rev. Drug Discovery, 2007, 6, 273–286 CrossRef CAS PubMed.
- M. H. Cohen, Y. L. Shen, P. Keegan and R. Pazdur, FDA drug approval summary: bevacizumab (Avastin) as treatment of recurrent glioblastoma multiforme, Oncologist, 2009, 14, 1131–1138 CrossRef CAS PubMed.
- E. E. Schmidt, K. Ichimura, G. Reifenberger and V. P. Collins, CDKN2 (p16/MTS1) gene deletion or CDK4 amplification occurs in the majority of glioblastomas, Cancer Res., 1994, 54, 6321–6324 CAS.
- K. Ueki, Y. Ono, J. W. Henson, J. T. Efird, A. von Deimling and D. N. Louis, CDKN2/p16 or RB alterations occur in the majority of glioblastomas and are inversely correlated, Cancer Res., 1996, 56, 150–153 CAS.
- H. Strik, M. Deininger, J. Streffer, E. Grote, J. Wickboldt, J. Dichgans, M. Weller and R. Meyermann, BCL-2 family protein expression in initial and recurrent glioblastomas: modulation by radiochemotherapy, J. Neurol., Neurosurg. Psychiatry, 1999, 67, 763–768 CrossRef CAS.
- F. E. McDonald, J. W. Ironside, A. Gregor, B. Wyatt, M. Stewart, R. Rye, J. Adams and H. W. Potts, The prognostic influence of bcl-2 in malignant glioma, Br. J. Cancer, 2002, 86, 1899–1904 CrossRef CAS PubMed.
- M. Grzmil, P. Morin Jr., M. M. Lino, A. Merlo, S. Frank, Y. Wang, G. Moncayo and B. Hernmings, MAP kinase-interacting kinase 1 regulates SMAD2-dependent TGF-β signaling pathway in human glioblastoma, Cancer Res., 2011, 71, 2392–2402 CrossRef CAS PubMed.
- D. Maier, D. Comparone, E. Taylor, Z. Zhang, O. Gratzl, E. G. Van Meir, R. J. Scott and A. Merlo, New deletion in low-grade oligodendroglioma at the glioblastoma suppressor locus on chromosome 10q25–26, Oncogene, 1997, 15, 997–1000 CAS.
- M. Grzmil, T. Rzymski, M. Milani, A. L. Harris, R. G. Capper, N. J. Saunders, A. Salhan, J. Ragoussis and C. J. Norbury, An oncogenic role of eIF3e/INT6 in human breast cancer, Oncogene, 2010, 29, 4080–4089 CrossRef CAS PubMed.
- A. I. Saeed, V. Sharov, J. White, J. Li, W. Liang, N. Bhagabati, J. Braisted, M. Klapa, T. Currier, M. Thiagarajan, A. Sturn, M. Snuffin, A. Rezantsev, D. Popov, A. Ryltsov, E. Kostukovich, I. Borisovsky, Z. Liu, A. Vinsavich, V. Trush and J. Quackenbush, TM4: a free, open-source system for microarray data management and analysis, Biotechniques, 2003, 34, 374–378 CAS.
- S. Fessele, H. Maier, C. Zischek, P. J. Nelson and T. Werner, Regulatory context is a crucial part of gene function, Trends Genet., 2002, 18, 60–63 CrossRef CAS.
- E. Barta, Comparative genomics-based orthologous promoter analysis using the DoOP database and the DoOPSearch web tool, Methods Mol. Biol., 2007, 395, 319–328 CAS.
- M. Baitaluk, M. Sedova, A. Ray and A. Gupta, BiologicalNetworks: visualization and analysis tool for systems biology, Nucleic Acids Res., 2006, 34, W466–W471 CrossRef CAS PubMed.
- R. Aguirre-Gamboa, H. Gomez-Rueda, E. Martinez-Ledesma, A. Martinez-Torteya, R. Chacolla-Huaringa, A. Rodriguez-Barrientos, J. G. Tamez-Pena and V. Trevino, SurvExpress: an online biomarker validation tool and database for cancer gene expression data using survival analysis, PLoS One, 2013, 8, e74250 CAS.
- R. G. Verhaak, K. A. Hoadley, E. Purdom, V. Wang, Y. Qi, M. D. Wilkerson, C. R. Miller, L. Ding, T. Golub, J. P. Mesirov, G. Alexe, M. Lawrence, M. O'Kelly, P. Tamayo, B. A. Weir, S. Gabriel, W. Winckler, S. Gupta, L. Jakkula, H. S. Feiler, J. G. Hodgson, C. D. James, J. N. Sarkaria, C. Brennan, A. Kahn, P. T. Spellman, R. K. Wilson, T. P. Speed, J. W. Gray, M. Meyerson, G. Getz, C. M. Perou and D. N. Hayes, Integrated genomic analysis identifies clinically relevant subtypes of glioblastoma characterized by abnormalities in PDGFRA, IDH1, EGFR, and NF1, Cancer Cell, 2010, 17, 98–110 CrossRef CAS PubMed.
- R. J. Akhurs and A. Hata, Targeting the TGFβ signalling pathway in disease, Nat. Rev. Drug Discovery, 2012, 11, 790–811 CrossRef PubMed.
- J. R. Infante, K. P. Papadopoulos, J. C. Bendell, A. Patnaik, H. A. Burris 3rd, D. Rasco D, S. F. Jones, L. Smith, D. S. Cox, M. Durante, K. M. Bellew, J. J. Park, N. T. Le and A. W. Tolcher, A phase 1b study of trametinib, an oral Mitogen-activated protein kinase kinase (MEK) inhibitor, in combination with gemcitabine in advanced solid tumours, Eur. J. Cancer, 2013, 49, 2077–2085 CrossRef CAS PubMed.
- J. J. Xia, L. B. Pei, J. P. Zhuang, Y. Ji, G. P. Xu, Z. P. Zhang, N. Li and J. L. Yan, Celecoxib inhibits β-catenin-dependent survival of the human osteosarcoma MG-63 cell line, J. Int. Med. Res., 2010, 38, 1294–1304 CrossRef CAS PubMed.
- W. Feng, P. Webb, P. Nguyen, X. Liu, J. Li, M. Karin and P. J. Kushner, Potentiation of estrogen receptor activation function 1 (AF-1) by Src/JNK through a serine 118-independent pathway, Mol. Endocrinol., 2001, 15, 32–45 CrossRef CAS PubMed.
- FDA. FDA approves additional medical indication for Sprycel, FDA, http://www.fda.gov, retrieved 22 March 2014.
- B. Casar, J. Rodríguez, G. Gibor, R. Seger and P. Crespo, Mxi2 sustains ERK1/2 phosphorylation in the nucleus by preventing ERK1/2 binding to phosphatases, Biochem. J., 2012, 441, 571–578 CrossRef CAS PubMed.
- M. Nguyen, R. C. Marcellus, A. Roulston, M. Watson, L. Serfass, S. R. Murthy Madiraju, D. Goulet, J. Viallet, L. Bélec, X. Billot, S. Acoca, E. Purisima, A. Wiegmans, L. Cluse, R. W. Johnstone, P. Beauparlant and G. C. Shore, Small molecule obatoclax (GX15-070) antagonizes MCL-1 and overcomes MCL-1-mediated resistance to apoptosis, Proc. Natl. Acad. Sci. U. S. A., 2007, 104, 19512–19517 CrossRef CAS PubMed.
- F. Basit, S. Cristofanon and S. Fulda, Obatoclax (GX15-070) triggers necroptosis by promoting the assembly of the necrosome on autophagosomal membranes, Cell Death Differ., 2013, 20, 1161–1673 CrossRef CAS PubMed.
- S. Okabe, T. Tauchi, Y. Tanaka, S. Kimura, T. Maekawa and K. Ohyashiki, Activity of histone deacetylase inhibitors and an Aurora kinase inhibitor in BCR-ABL-expressing leukemia cells: Combination of HDAC and Aurora inhibitors in BCR-ABL-expressing cells, Cancer Cell Int., 2013, 13, 32 CrossRef CAS PubMed.
- C. O'Neill, Y. Li and X. L. Jin, Survival signaling in the preimplantation embryo, Theriogenology, 2012, 77, 773–784 CrossRef PubMed.
- L. Lin, H. Li, M. Xu, Z. Zhao, V. Olson, Y. Xu, C. Li and J. Lin, Abstract 4772: novel drug discovery approach targeting STAT3 for breast cancer therapy using MLSD and drug repositioning, Cancer Res., 2012, 72(suppl 1), 4772 Search PubMed.
- V. C. Jordan, Tamoxifen (ICI46, 474) as a targeted therapy to treat and prevent breast cancer, Br. J. Pharmacol., 2006, 147(suppl 1), S269–S276 CAS.
Footnote |
† Electronic supplementary information (ESI) available. See DOI: 10.1039/c5mb00007f |
|
This journal is © The Royal Society of Chemistry 2015 |
Click here to see how this site uses Cookies. View our privacy policy here.