The histone H3 N-terminal tail: a computational analysis of the free energy landscape and kinetics†
Received
30th March 2015
, Accepted 23rd April 2015
First published on 24th April 2015
Abstract
Histone tails are the short peptide protrusions outside of the nucleosome core particle and they play a critical role in regulating chromatin dynamics and gene activity. A histone H3 N-terminal tail, like other histone tails, can be covalently modified on different residues to activate or repress gene expression. Previous studies have indicated that, despite its intrinsically disordered nature, the histone H3 N-terminal tail has regions of notable secondary structural propensities. To further understand the structure–dynamics–function relationship in this system, we have carried out 75.6 μs long implicit solvent simulations and 29.3 μs long explicit solvent simulations. The extensive samplings allow us to better characterize not only the underlying free energy landscape but also kinetic properties through Markov state models (MSM). Dihedral principal component analysis (dPCA) and locally scaled diffusion map (LSDMap) analysis yield consistent results that indicate an overall flat free energy surface with several shallow basins that correspond to conformations with a high α-helical propensity in two regions of the peptide. Kinetic information extracted from Markov state models reveals rapid transitions between different metastable states with mean first passage times spanning from several hundreds of nanoseconds to hundreds of microseconds. These findings shed light on how the dynamical nature of the histone H3 N-terminal tail is related to its function. The complementary nature of dPCA, LSDMap and MSM for the analysis of biomolecules is also discussed.
Introduction
In eukaryotic cells, highly conserved histone proteins provide the basic scaffold to package the genome inside the nucleus, while playing a critical role in regulating gene activity at the same time. A DNA segment of approximately 146 base-pairs wraps tightly around a histone hetero-octomer consisting of two subunits of each type of histone protein (H2A, H2B, H3 and H4) to form a nucleosome, which can further fold into higher order chromatin fibers.1,2 The structure of the nucleosome core particle (NCP) has been resolved experimentally and also studied using molecular simulations.3–6 The histone terminal tails extending out of the NCP and adjacent regions are critical for chromatin remodeling and thus regulation of gene transcription.1 The histone tails can be covalently modified upon receiving upstream signals. Post-translational modifications, such as methylation, acetylation and phosphorylation, can alter the charges on specific residues, leading to changes in the binding affinity with other functional consequences.7,8 Even though the histone tails are of intrinsically disordered nature, some can still transiently assume secondary structural elements according to previous experimental and computational studies.9–13 However, the dynamic properties of histone tails, such as the kinetics of transitions among different metastable conformations, remain to be elucidated.
A histone H3 N-terminal tail, in particular, can be covalently modified with various patterns and is of great interest to both experimental and computational studies. For instance, methylation of the K4 residue is linked to gene activation while methylation of K9 or K27 is linked to gene repression.14–16 Methylation of K9 is a specific target for heterochromatin protein 1 (HP1).15,17,18 In the NMR structure of its complex with HP1, the region from K4 to S10 showed an extended structure with K4 and K9 both in the dimethylated form.19 In another study, an 8-residue variant of the peptide with trimethylated K4 showed an extended β-strand conformation from A1 to T6 in complex with an ING2 (inhibitor of growth family, member 2) plant homeodomain (PHD) finger.20 An experimental study using circular dichroism (CD), however, showed that some α-helix content is present in histone H3/H4 tails, even though it was difficult to selectively isolate H3 or H4 tails.9 Previous computational studies11,13 showed that the histone H3 tail populates α-helical conformations, and that post-translational modifications only have a marginal influence on the most populated conformations.11 The degree of sampling in these pioneering computational studies was somewhat limited, despite the use of replica exchange techniques,13 leading to some regions of the free energy surface only sparsely sampled. Moreover, the kinetics of transitions among the various conformations were not explored. In this study, we use the Markov state model (MSM), dihedral angle principal component analysis (dPCA) and locally scaled diffusion map (LSDMap) to gain a further understanding of the free energy landscape and dynamics of this peptide.
MSMs have been used to extract long time scale kinetic information from many short MD simulation trajectories.21–27 Here we run extensive explicit solvent simulations on the H3 N-terminal tail using graphic processing units, with seeds from intensively sampled implicit solvent conformational ensembles. We extract kinetic information from the MSM and characterize the kinetically metastable states. No metastable state has a dominant population and the peptide makes transitions between different states at the microsecond time scale. dPCA and LSDMap also reveal a generally flat free energy surface with low barriers between the basins. The low free energy barriers and rapid transitions between different metastable conformations are consistent with the functional requirement of the H3 tail to respond to different covalent modifications and binding partners.
Computational methods
Simulation protocol
Due to the lack of a defined experimental structure, we build a stretched initial structure of the histone H3 N-terminal tail according to its amino acid sequence,28 with the C-terminal capped with an N-methyl group. A 38 residue construct is used (with the sequence: ARTKQ TARKS TGGKA PRKQL ATKAA RKSAP ATGGV KKP), which is slightly longer than the biochemically isolated one by trypsination. This sequence preserves the structural geometry of the tail and is of the same length as the construct used in another computational study,13 making it straightforward to compare the current and previous results. All simulations are carried out using the Amber14 MD package on graphic processing units.29,30 The ff99SBnmr1 force field31 is used because recent benchmark calculations suggested that this force field provides reliable structural propensities with both explicit and implicit solvent simulations.32 We carry out both implicit and explicit solvent simulations on the system in this work.
The initial structure is minimized and equilibrated using the GB7 generalized Born implicit solvent model.33 An initial 2 μs long simulation is then carried out. The conformations in the trajectory are clustered using the K-means clustering algorithm implemented in the MMTSB Tool Set,34 and seed conformations are randomly chosen from different clusters for parallel production runs. In total, 75.6 μs long simulations are collected. In these simulations, no cutoff is used for the non-bonded interactions. 0.15 M of NaCl is applied using a modified generalized Born model based on the Debye–Hückel limiting law for ionic screening of electrostatic interactions.35 The SHAKE algorithm36 is used to constrain all bonds involving hydrogen atoms. An integration time step of 2 fs is used. Langevin dynamics with a collision frequency of 20 ps−1 is used to keep the temperature at 300 K.
For explicit solvent simulations, the seed conformations are taken from the entire implicit solvent simulation ensemble following the same clustering and selection procedure. This seeding procedure enhances sampling by starting simulations from as diverse a set of conformations as possible; as shown in the ESI,† the propensities of secondary structures from the implicit and explicit solvent simulations are generally similar after extensive sampling, supporting the use of implicit solvent models to seed explicit solvent simulations. The seed conformations are solvated with TIP3P water,37 with a box size of around 70 × 70 × 70 Å3 using periodic boundary conditions. The system is neutralized with counterions and an additional 0.15 M NaCl is added to mimic the physiological conditions. The electrostatic interactions are calculated using the particle mesh Ewald method with a grid spacing of about 1.0 Å. The cutoff for van der Waals interactions is set at 10 Å. The system is first minimized with the fixed protein and then for the entire system. 1 ns NPT simulation is carried out to equilibrate the volume of the system. Production simulations are run in the NVT ensemble at 300 K using the weak-coupling algorithm38 with a time constant of 5.0 ps. A total of 29.3 μs of trajectories are collected for analysis.
Dihedral principal component analysis (dPCA)
The explicit solvent simulations are analyzed using principal component analysis based on backbone dihedral angles.39,40 Since the histone H3 tail frequently undergoes folding/unfolding in the simulations, the backbone dihedral angles are better coordinates for the analysis compared to Cartesian coordinates. If we denote each backbone dihedral angle as φi, we have 75 [2 × number of residues (38) − 1] backbone dihedral angles in total, lacking the ϕ angle for the first residue. Due to the periodicity of dihedral angles, we transform each angle into its cosine and sine values,
q2n−1 = cos φn, |
| q2n = sin φn, | (1) |
where n = 1,…, 75. The correlated internal motions can then be represented by the covariance matrix | σij = 〈(qi − 〈qi〉)(qj − 〈qj〉)〉, | (2) |
where q1,…, q150 are the transformed cosine and sine values for the dihedral angles. By diagonalizing the covariance matrix, we obtain 150 pairs of eigenvalues and eigenvectors. The conformations in the ensemble are then projected onto the two eigenvectors with the largest eigenvalues. The free energy surface of the system is then calculated as | ΔG(Q1,Q2) = −kBT[ln ρ(Q1,Q2) − ln ρmax], | (3) |
where ρ is an estimate of the probability density function obtained after binning the data. ρmax is the maximum density, which has the free energy minimum with ΔG set to 0.
Locally scaled diffusion map
The conformation ensemble of the histone H3 N-terminal tail is further analyzed using LSDMap. LSDMap is a multiscale method to determine the collective reaction coordinates of macromolecular dynamics.41 For computational efficiency, the ensemble is subsampled and a total of 11
731 conformations are used for the calculation. The transition probability between two conformations is described by the kernel K, | 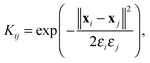 | (4) |
where ∥xi − xj∥ is the root mean square deviation (RMSD) between the two conformations xi and xj based on backbone atoms (CA, C, N, and O) and CB. εi and εj are the respective local scales. The local scales for every point in the data set are estimated according to the previously proposed procedure.41 The elements in the kernel matrix characterize the ease of a conformation to diffuse into another. The kernel can be normalized and converted to a Markov matrix, from which we compute the first few largest eigenvalues and the corresponding eigenvectors. The eigenvectors represent the diffusion coordinates. For technical details, we refer the interested reader to ref. 41.
MSM construction and validation
For the construction of MSM, conformations in the 29.3 μs long explicit solvent simulation trajectory are saved every 50 ps, resulting in ∼586 thousand conformations. These conformations are clustered into 8000 microstates using the hybrid k-centers/k-medoids clustering method24 based on the RMSD of backbone atoms (CA, C, N, and O) and CB. The number of transitions between the microstates at an interval of a certain lag time is counted. The count matrix is then symmetrized and normalized to obtain the transition probability matrix (T). The Markov time, the lag time at which the model is Markovian, is determined by examining the implied time scales at different lag times. At a specific lag time, the implied time scales can be calculated as: |  | (5) |
where κ is a relaxation time scale, τ is the lag time, and μ(τ) is an eigenvalue for the transition matrix T(τ). If the model is Markovian at a certain lag time, the relaxation time scales should remain constant when using longer lag times, satisfying the Chapman–Kolmogorov equation. Here we use the smallest lag time at which the implied time scales level off to build the microstate MSM for further analysis. The microstate MSM is used to calculate the kinetic properties. To facilitate interpretation, the 8000 state microstate model is coarse grained to 150 macrostates using the Bayesian agglomerative clustering engine (BACE),42 which has been shown to be a robust method in a recent study that compared different coarse graining protocols.43 The population of each macrostate is the sum of the equilibrium populations of the microstates belonging to this macrostate. The MSMBuilder software is used to construct the model.21,24,44
Mean first passage time (MFPT) calculations
MFPT is defined as the average transition time between a pair of states in an MSM. The MFPT from initial state i to final state j can be determined by solving the following set of equations: |  | (6) |
where τ is the lag time and T(τ) is the corresponding transition probability matrix.45 To compute the MFPT from the microstate MSM, we first set the MFPTs of all the microstates within the destination macrostate to be zero. MFPTs starting from each microstate in the starting macrostate are then calculated. A weighted average is then obtained as MFPTif:
, where pl is the normalized population of microstate l within macrostate i.
Results and discussion
In the following, we first comment on the degree of sampling and validation of the Markov model. Then we characterize the free energy landscape by analyzing the conformational features of macro states from MSM and comparing the observations to the free energy surface computed by dPCA and LSDMap. Finally, we discuss the kinetics of conformational transitions by computed MFPTs.
Convergence and model validation
An adequate sampling is critical for extracting valid equilibrium and kinetic information from molecular simulations. The various analyses in this work are based on the fairly long (∼30 μs) explicit solvent simulations, which are seeded by different conformations from a longer set of (∼80 μs) implicit solvent simulations. To evaluate the convergence of the simulations, we monitor the radius of gyration (Rg) distribution and the dPCA free energy surface when only part of the simulation data is used. As shown in Fig. S1 and S2 (ESI†), when only 15 μs, 20 μs or 25 μs of the explicit solvent data is used, the Rg distributions remain nearly the same; the free energy landscapes obtained using dPCA are also similar in terms of the main free energy basins and their connectivity. Thus we conclude that the degree of sampling carried out here is adequate to draw meaningful conclusions.
In the MSM analysis, for the model to be Markovian, it should satisfy the Chapman–Kolmogorov equation and the relaxation time scales should remain constant at different lag times. Instead of spot-checking specific states, we conduct a global check on the MSM by observing the implied time scale plot at different lag times. As shown in Fig. 1(a), the implied time scales for the 8000 microstate model reach a plateau at about 6 ns, which is used to construct the microstate MSM. The quantitative properties, including the MFPTs, are calculated from this model.
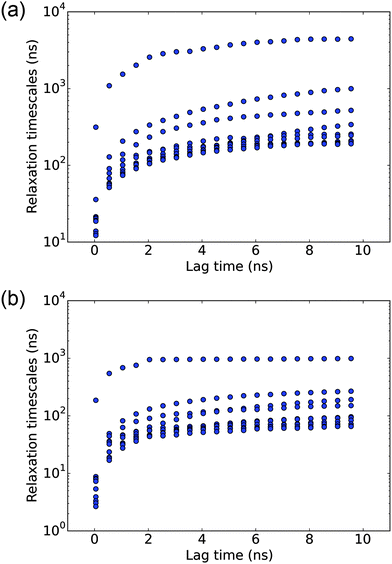 |
| Fig. 1 Analysis of the Markov state model (MSM) with different lag times. (a) Implied time scale plot for the 8000-state microstate MSM. (b) Implied time scale plot for the 150-state macrostate MSM. In each case, the slowest eigenmodes of the corresponding transition matrix are analyzed. | |
To better elucidate the free energy landscape, we coarse grain the 8000 microstate model to a 150 state macrostate MSM using the BACE coarse graining method. The implied time scales for the 150 state model, as shown in Fig. 1(b), also level off at about 6 ns. The relaxation time scales are slightly shorter than those of the microstate MSM. As noted in a previous study,27 this discrepancy results from lumping very small microstates with the large ones and may be corrected by keeping those small microstates as independent states. Here, we will only use the macrostate MSM for the purpose of elucidating key features of the free energy landscape.
Analysis of the free energy landscape
The equilibrium populations of the kinetically metastable states obtained from the macrostate MSM (Fig. 2) show that the free energy surface is rugged without a single dominant well. The most populated macro state has a population of about 3.4%. This is physically reasonable considering the high structural flexibility of the histone tail.
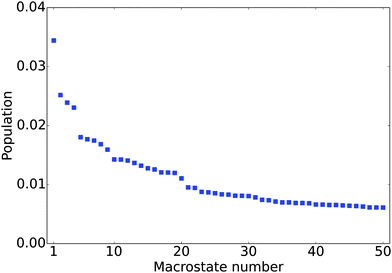 |
| Fig. 2 Equilibrium population of the 50 most populated macrostates. | |
To gain a better understanding of the characteristics of the major free energy basins, we further investigate the structural features of the top 9 most populated macro states (there is a small gap in population between the 9th and 10th states, see Fig. 2). As shown in Fig. 3, most of the structures in these basins have two α-helix regions, one from residue 2 to residue 11 and another from residue 17 to residue 28; the second α-helix segment has a higher propensity to be folded. The other regions mainly assume coil and turn structures. The most populated state, as shown in Fig. 3(a), has an N-terminal α-helix region with an average probability of 0.41 and a second α-helix region with an average probability of 0.77. It has an average Rg of 18.04 Å. The 4th most populated state has a very low probability for the first α-helix (Fig. 3(d)). The Rg's among the top nine states range from 15.09 Å to 19.49 Å, with the standard deviation of ∼1.5 Å. An outlier is the 9th state, which only has a single short α-helix between residues 20 and 26; it also features a much more collapsed structure with an average Rg of 10.00 ± 0.14 Å (Fig. 3(i)).
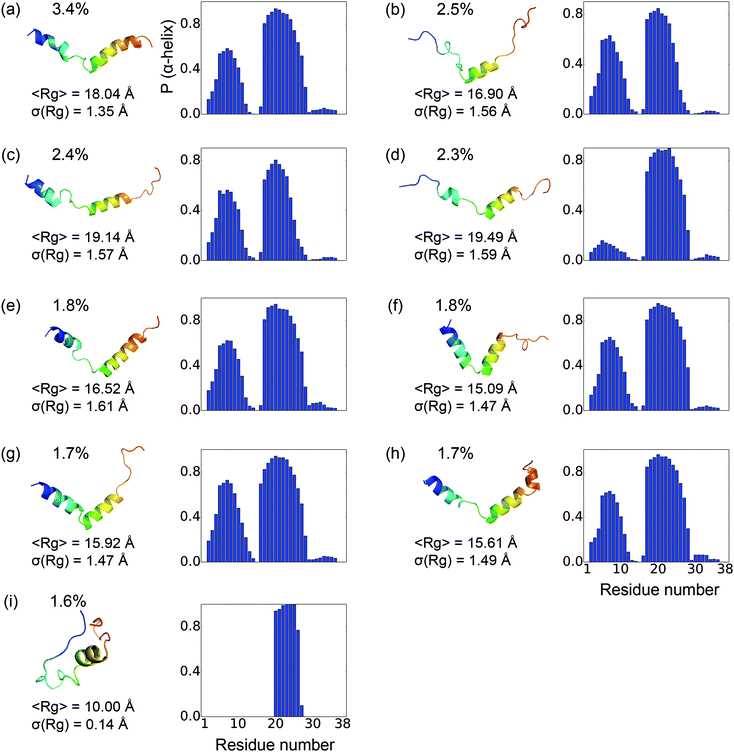 |
| Fig. 3 Representative structures and structural features for the nine most populated macrostates. The population for each state is labeled on the top-left. The representative structures are colored in rainbow with blue denoting the N-terminus and red for the C-terminus. To the right is the probability for each residue to be α-helical in each state. The mean and standard deviation of the radius of gyration (Rg) for the conformations in each state are also listed. | |
In Fig. 4, the Rg values for the 150 macro states are plotted in the order of their populations. There is not a strong correlation between the level of compactness and population. Most of the macrostates have Rg values between 12 and 18 Å, with substantial standard deviations. The only exception is the already noted 9th state, which features only very compact conformers with a small (0.14) standard deviation in Rg. Several other macro states also have an average Rg of around 10–11 Å but with substantially higher standard deviations. The fact that the standard deviations in Rg for most macro states are fairly large (∼1–2 Å) suggests that many conformations of different Rg's interconvert rapidly (e.g., when the isomerization only involves simple bond rotations etc., see discussions below). Many compact structures are assigned to different macro states, which explains that the macro states with low average Rg's are not highly populated.
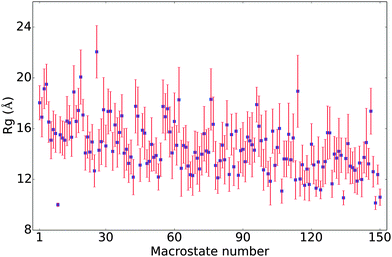 |
| Fig. 4 The average Rg's of the 150 metastable states in the MSM; the states are numbered based on their populations, with the first state being most populated and the last being least populated. The error bars are twice the standard deviation of the Rg's among conformations in each state. | |
To better understand the overall structural propensities of the peptide, we investigate the secondary structure distribution for the entire conformational ensemble (Fig. 5). On average, about 21 residues are coil and about 10 residues assume an α-helix structure (Fig. 5(a)). The most α-helical regions are observed from residues 5 to 9 and residues 17 to 26, with the rest mainly being coils and turns (Fig. 5(b)). The most populated macro states have a higher content of α-helix structures than the average macro states. The analysis of the implicit solvent simulations shows a similar pattern (see Fig. S3, ESI†). In the previous computational study with a 16-residue H3 N-terminal tail variant, the region from residues 4 to 11 showed a high propensity to assume an α-helix structure,11 corresponding to the first α-helical fragment in this study. In another computational study using the same construct as this work, 2–3 regions strongly favoring the α-helix structure were observed,13 in qualitative agreement with our findings.
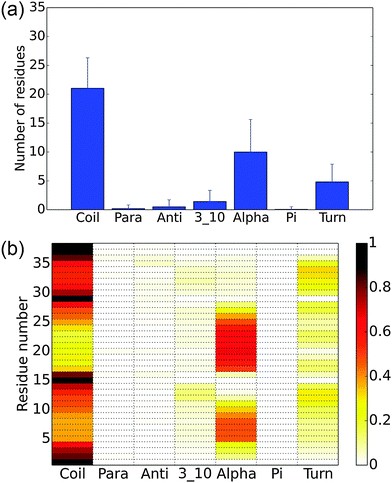 |
| Fig. 5 Secondary structure of the histone H3 tail in explicit solvent simulations. (a) The average number of residues having a specific type of secondary structure. The error bars show the standard deviation. (b) The probability for each residue to have each type of secondary structure. | |
Free energy landscape analyzed using dPCA
Dihedral PCA decomposes the free energy surface into three major basins. As shown in Fig. 6, the free energy surface is well connected and the barriers between the major basins are around 0.5–1.5 kcal mol−1. The largest basin, labeled ‘ii’ in the figure, has the characteristic structural feature of two α-helical segments as observed for the top-populated macro states from MSM (Fig. 3). The second largest basin, labeled ‘i’ in the figure, has very compact conformations. It lacks the N-terminal helix but maintains the second helical segment, similar to the 9th macro state from MSM. The conformations in basin ‘iii’ are quite open in nature and have a lower helical content than those in basin ‘ii’. Even though it is difficult to make a one to one mapping between the free energy basins from dPCA and the macro states from MSM, the dPCA results confirm the overall feature of the free energy landscape implied by the MSM analysis. In the study by Papoian and co-workers,13 the free energy landscape was also analyzed using dPCA, which showed dispersed free energy basins with depths of around 1–3 kcal mol−1. The free energy surface in the current work is better connected due to the more extensive sampling, although the gross features are fairly similar. The ensemble average Rg of 15.2 Å is, however, higher than that reported in the previous study, which was 11.6 Å.
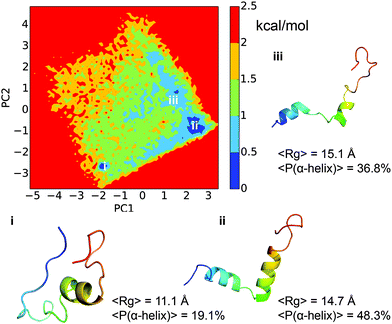 |
| Fig. 6 Free energy profile of the histone H3 tail dynamics projected onto two main dPCA principal components. Representative structures from the three main free energy basins are shown in rainbow colors, with blue denoting the N-terminus and red for the C-terminus. The average Rg and the α-helix content are also shown for each basin. | |
Free energy surface analyzed using LSDMap
The LSDMap shows two major basins on the free energy surface (Fig. 7). The barrier between these two basins is around 2–2.5 kcal mol−1. The small basin (basin ‘i’) has very compact structures, with an average Rg of 10.0 Å. However, the structures have a low α-helix content (∼18.2%) and are largely in random coils. The large basin (basin ‘ii’) has an average Rg of 16.9 Å and an α-helix content of 33.5%, showing that the structures are more open with two main α-helix segments. The time scale separation, as shown in Fig. 7a, indicates that a single time scale appears to dominate the dynamics. The projection of the free energy landscape onto the first diffusion coordinate and Rg (Fig. 8) shows that the first diffusion coordinate corresponds to the collapse/expansion of the peptide.
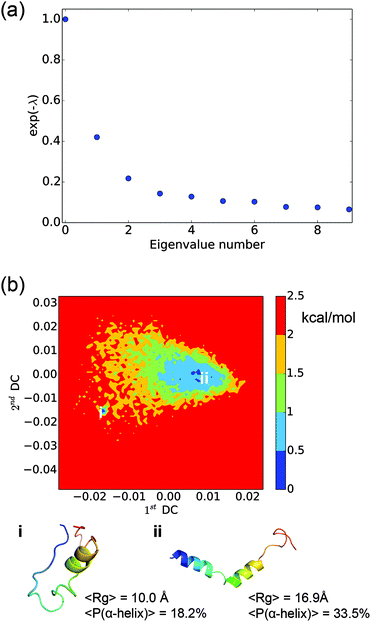 |
| Fig. 7 Results from the locally scaled diffusion map (LSDMap) analysis. (a) The exponential of the negative of the Fokker–Planck operator eigenvalues as a function of the eigenvalue number. The zero-th eigenvalue corresponds to the Boltzmann distribution. The major spectral gap between the first and second eigenvalues suggests that there is a single time scale that tends to dominate the dynamics. (b) Free energy landscape of the histone H3 tail projected onto the first and second diffusion coordinates. Representative structures for the two main free energy basins are shown in rainbow colors, with blue denoting the N-terminus and red for the C-terminus. The average Rg and the α-helix content are also shown for each basin. | |
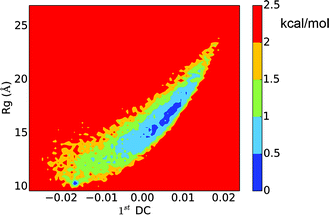 |
| Fig. 8 Free energy landscape of the histone H3 tail projected onto the first diffusion coordinate and Rg. | |
Comparing the free energy landscape analyzed using LSDMap and dPCA, based on the average Rg and the α-helix content, basin ‘i’ on the LSDMap corresponds to basin ‘i’ on the dPCA free energy surface, and basin ‘ii’ on the LSDMap corresponds to basins ‘ii’ and ‘iii’ on the dPCA free energy surface. The basin ‘i’ on both free energy surfaces feature very compact conformations, similar to the 9th-populated macro state from MSM (Fig. 3). The other basins have higher Rg values, similar to other top-populated MSM macro states.
MFPTs between different metastable states
Kinetic information is extracted from the MSM by calculating the MFPTs between different metastable states. As shown in Fig. 9, the MFPTs between these 150 metastable states range from hundreds of nanoseconds to hundreds of microseconds. Most of the transitions are on the order of several microseconds. The fastest transition occurs at ∼178 ns and the slowest at ∼242 μs. The fastest transition corresponds to the conversion from the 14th most populated state to the most populated state. Both of these two states have quite an open structure (with Rg's of 16.46 Å and 18.04 Å, respectively) and two helical regions. The slowest transition is from the 122nd most populated state to the 9th most populated state. Both of these two states have very compact structures, with Rg's of 11.31 Å and 10.00 Å, respectively. These observations are plausible considering that the transition between the open structures might simply involve the rotation of several single bonds while that between the compact structures may have to involve the breaking of electrostatic interactions and hydrogen bonds. For example, the representative structure in the 122nd most populated state is a compact random coil structure (Fig. S4(a), ESI†), while that for the 9th most populated state has an α-helical region (Fig. S4(b), ESI†). This conformational transition involves breaking of the original contacts in the compact random coil structure and the formation of an α-helix fragment.
The generally rapid transitions observed here are consistent with the relatively low barriers between free energy basins obtained using dPCA and LSDMap. This feature might be due to the abundant positive charges on the histone H3 N-terminal tail (it has four Arg residues and eight Lys residues, and no Asp/Glu). The generally repulsive electrostatic interactions make it harder to form many distinct compact structures. This is also supported by the relatively open conformations in the most populated macro states. The transitions likely mainly involve the partial folding and unfolding of the α-helix segments, movements around the linkage between these two segments, and also the flexible C-terminus.
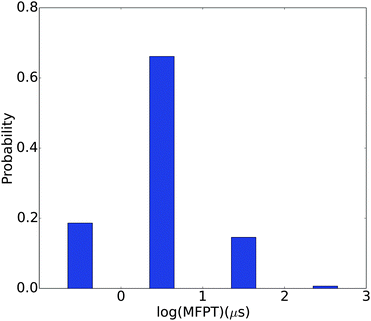 |
| Fig. 9 Distribution of MFPTs between the 150 different macrostates. | |
Concluding remarks
In this study, we carry out extensive explicit solvent simulations on the histone H3 N-terminal tail and construct the MSM to describe its dynamics. The MSM shows that the peptide has a rather flat free energy surface with shallow free energy basins separated by low barriers. The conformations in the main metastable states show two α-helix prone segments. The overall secondary structural propensity is consistent with observations from previous experimental and computational studies.9,11,13 The free energy surfaces obtained using dPCA and LSDMap show similar features, with conformations in the free energy basins exhibiting partial helical contents. The MFPTs computed from MSM indicate that the transitions between different metastable states range from hundreds of nanoseconds to hundreds of microseconds.
The rapid transition between different conformational states of the histone H3 N-terminal tail may well indicate how this peptide functions. The low free energy barrier between different conformational states can be easily perturbed by various modifications on different residues. The binding between the peptide and its counterpart may also involve an induced fitting process. For instance, in the NMR structure of a K4 and K9 dimethylated form in complex with HP1 and the crystal structure of K4 trimethylated form in complex with the ING2 PHD finger, the histone H3 N-terminal tail in general showed extended structures.19,20 Since chemical modifications were not found to have a major impact on the structure of this peptide,11 these structural variations observed in different experiments are expected to be due largely to binding to other proteins, which led to the conformational transition from helical to extended structures. The resolved region in the experimental structures largely corresponds to the first α-helical fragment in this study. It is worth noting that the N-terminal helical region is more labile than the second helical region. In the 9th most populated macrostate in the MSM and the basins labeled ‘i’ on the free energy surfaces analyzed with dPCA and LSDMap, the structures all have an extended N-terminus very similar to that found in the experimental structures. The side chains of K4 and K9 orient away from the helical region (Fig. S4(b–d), ESI†), which might facilitate the modifications and the binding process. The conformational transitions of the peptide in the induced-fit process can be facilitated by the intrinsically fast kinetics of the peptide. A similar discussion has been made for other intrinsically disordered proteins, whose conformations respond actively to solution conditions, self aggregation and binding to other proteins.27,46,47 These studies and the current work highlight how the internal dynamics of this important class of peptides contribute to the function.
From a technical perspective, the present work illustrates the advantages of integrating MSM, dPCA and LSDMap to study the free energy landscape and kinetics of a flexible system. dPCA is computationally efficient since it relies only on structural information and provides a compact description of the free energy landscape. LSDMap goes beyond dPCA by considering the “kinetic connectivity” among different conformations, thus the dominant eigenvectors better describe the collective dynamics of the system. MSM explicitly considers microscopic kinetic information for the interconversion among metastable states and therefore leads to a more complete description of the free energy landscape, although at the cost of requiring a more thorough sampling. In the current work, the results from the three methods are largely consistent in terms of dominant structural features, although MSM clearly contains richer information regarding the kinetic stability and accessibility of different populations. The integration of the three methods provides us with a means of cross validation and a more complete characterization of the free energy landscape and kinetics of the histone H3 N-terminal tail.
Acknowledgements
Discussions with Prof. Xuhui Huang and Dr. Qin Qiao regarding MSM are greatly acknowledged. The initial work on the extensive sampling was supported by grant DMS-1160360 from the National Science Foundation, and the data analysis was supported by grant CHE-1300209. Computational resources from the Extreme Science and Engineering Discovery Environment (XSEDE), which is supported by NSF grant number OCI-1053575, are greatly appreciated; computations are also supported in part by NSF through a major instrumentation grant (CHE-0840494) to the chemistry department, and the GPU computing facility supported by the Army Research Office (W911NF-11-1-0327).
References
- K. Luger and T. J. Richmond, The histone tails of the nucleosome, Curr. Opin. Genet. Dev., 1998, 8, 140–146 CrossRef CAS.
- R. D. Kornberg and Y. Lorch, Twenty-Five Years of the Nucleosome, Fundamental Particle of the Eukaryote Chromosome, Cell, 1999, 98, 285–294 CrossRef CAS.
- K. Luger, A. W. Mäder, R. K. Richmond, D. F. Sargent and T. J. Richmond, Crystal structure of the nucleosome core particle at 2.8 A resolution, Nature, 1997, 389, 251–260 CrossRef CAS PubMed.
- T. J. Richmond and C. A. Davey, The structure of DNA in the nucleosome core, Nature, 2003, 423, 145–150 CrossRef CAS PubMed.
- C. K. Materese, A. Savelyev and G. A. Papoian, Counterion Atmosphere and Hydration Patterns near a Nucleosome Core Particle, J. Am. Chem. Soc., 2009, 131, 15005–15013 CrossRef CAS PubMed.
- S. Sharma, F. Ding and N. V. Dokholyan, Multiscale Modeling of nucleosome dynamics, Biophys. J., 2007, 92, 1457–1470 CrossRef CAS PubMed.
- B. D. Strahl and C. D. Allis, The language of covalent histone modifications, Nature, 2000, 403, 41–45 CrossRef CAS PubMed.
- R. Margueron, P. Trojer and D. Reinberg, The key to development: interpreting the histone code?, Curr. Opin. Genet. Dev., 2005, 15, 163–176 CrossRef CAS PubMed.
- J. L. Baneres, A. Martin and J. Parello, The N tails of histones H3 and H4 adopt a highly structured conformation in the nucleosome, J. Mol. Biol., 1997, 273, 503–508 CrossRef CAS PubMed.
- X. Wang, S. C. Moore, M. Laszckzak and J. Ausió, Acetylation increases the alpha-helical content of the histone tails of the nucleosome, J. Biol. Chem., 2000, 275, 35013–35020 CrossRef CAS PubMed.
- H. Liu and Y. Duan, Effects of posttranslational modifications on the structure and dynamics of histone H3 N-terminal Peptide, Biophys. J., 2008, 94, 4579–4585 CrossRef CAS PubMed.
- D. Yang and G. Arya, Structure and binding of the H4 histone tail and the effects of lysine 16 acetylation, Phys. Chem. Chem. Phys., 2011, 13, 2911–2921 RSC.
- D. A. Potoyan and G. A. Papoian, Energy landscape analyses of disordered histone tails reveal special organization of their conformational dynamics, J. Am. Chem. Soc., 2011, 133, 7405–7415 CrossRef CAS PubMed.
- B. D. Strahl, R. Ohba, R. G. Cook and C. D. Allis, Methylation of histone H3 at lysine 4 is highly conserved and correlates with transcriptionally active nuclei in Tetrahymena, Proc. Natl. Acad. Sci. U. S. A., 1999, 96, 14967–14972 CrossRef CAS.
- J. Nakayama, J. C. Rice, B. D. Strahl, C. D. Allis and S. I. Grewal, Role of histone H3 lysine 9 methylation in epigenetic control of heterochromatin assembly, Science, 2001, 292, 110–113 CrossRef CAS PubMed.
- K. Plath, J. Fang, S. K. Mlynarczyk-Evans, R. Cao, K. A. Worringer, H. B. Wang, C. C. de la Cruz, A. P. Otte, B. Panning and Y. Zhang, Role of histone H3 lysine 27 methylation in X inactivation, Science, 2003, 300, 131–135 CrossRef CAS PubMed.
- A. J. Bannister, P. Zegerman, J. F. Partridge, E. A. Miska, J. O. Thomas, R. C. Allshire and T. Kouzarides, Selective recognition of methylated lysine 9 on histone H3 by the HP1 chromo domain, Nature, 2001, 410, 120–124 CrossRef CAS PubMed.
- M. Lachner, N. O'Carroll, S. Rea, K. Mechtler and T. Jenuwein, Methylation of histone H3 lysine 9 creates a binding site for HP1 proteins, Nature, 2001, 410, 116–120 CrossRef CAS PubMed.
- P. R. Nielsen, D. Nietlispach, H. R. Mott, J. Callaghan, A. Bannister, T. Kouzarides, A. G. Murzin, N. V. Murzina and E. D. Laue, Structure of the HP1 chromodomain bound to histone H3 methylated at lysine 9, Nature, 2002, 416, 103–107 CrossRef CAS PubMed.
- P. V. Pena, F. Davrazou, X. B. Shi, K. L. Walter, V. V. Verkhusha, O. Gozani, R. Zhao and T. G. Kutateladze, Molecular mechanism of histone H3K4me3 recognition by plant homeodomain of ING2, Nature, 2006, 442, 100–103 CAS.
- G. R. Bowman, X. Huang and V. S. Pande, Using generalized ensemble simulations and Markov state models to identify conformational states, Methods, 2009, 49, 197–201 CrossRef CAS PubMed.
- G. R. Bowman, K. A. Beauchamp, G. Boxer and V. S. Pande, Progress and challenges in the automated construction of Markov state models for full protein systems, J. Chem. Phys., 2009, 131, 124101 CrossRef PubMed.
- F. Noe and S. Fischer, Transition networks for modeling the kinetics of conformational change in macromolecules, Curr. Opin. Struct. Biol., 2008, 18, 154–162 CrossRef CAS PubMed.
- K. A. Beauchamp, G. R. Bowman, T. J. Lane, L. Maibaum, I. S. Haque and V. S. Pande, MSMBuilder2: Modeling Conformational Dynamics at the Picosecond to Millisecond Scale, J. Chem. Theory Comput., 2011, 7, 3412–3419 CrossRef CAS PubMed.
- M. Senne, B. Trendelkamp-Schroer, A. S. J. S. Mey, C. Schuette and F. Noe, EMMA: A Software Package for Markov Model Building and Analysis, J. Chem. Theory Comput., 2012, 8, 2223–2238 CrossRef CAS.
- V. A. Voelz, G. R. Bowman, K. Beauchamp and V. S. Pande, Molecular Simulation of ab Initio Protein Folding for a Millisecond Folder NTL9(1-39), J. Am. Chem. Soc., 2010, 132, 1526–1528 CrossRef CAS PubMed.
- Q. Qiao, G. R. Bowman and X. Huang, Dynamics of an intrinsically disordered protein reveal metastable conformations that potentially seed aggregation, J. Am. Chem. Soc., 2013, 135, 16092–16101 CrossRef CAS PubMed.
- L. Mariño-Ramírez, B. Hsu, A. D. Baxevanis and D. Landsman, The Histone Database: a comprehensive resource for histones and histone fold-containing proteins, Proteins, 2006, 62, 838–842 CrossRef PubMed.
- A. W. Götz, M. J. Williamson, D. Xu, D. Poole, S. Le Grand and R. C. Walker, Routine Microsecond Molecular Dynamics Simulations with AMBER on GPUs. 1. Generalized Born, J. Chem. Theory Comput., 2012, 8, 1542–1555 CrossRef PubMed.
- R. Salomon-Ferrer, A. W. Goetz, D. Poole, S. Le Grand and R. C. Walker, Routine Microsecond Molecular Dynamics Simulations with AMBER on GPUs. 2. Explicit Solvent Particle Mesh Ewald, J. Chem. Theory Comput., 2013, 9, 3878–3888 CrossRef CAS.
- D.-W. Li and R. Brueschweiler, NMR-Based Protein Potentials, Angew. Chem., Int. Ed., 2010, 49, 6778–6780 CrossRef CAS PubMed.
- K. A. Beauchamp, Y.-S. Lin, R. Das and V. S. Pande, Are Protein Force Fields Getting Better? A Systematic Benchmark on 524 Diverse NMR Measurements, J. Chem. Theory Comput., 2012, 8, 1409–1414 CrossRef CAS PubMed.
- J. Mongan, C. Simmerling, J. A. McCammon, D. A. Case and A. Onufriev, Generalized Born model with a simple, robust molecular volume correction, J. Chem. Theory Comput., 2007, 3, 156–169 CrossRef CAS PubMed.
- M. Feig, J. Karanicolas and C. L. Brooks, 3rd MMTSB Tool Set: enhanced sampling and multiscale modeling methods for applications in structural biology, J. Mol. Graphics Modell., 2004, 22, 377–395 CrossRef CAS PubMed.
- J. Srinivasan, M. W. Trevathan, P. Beroza and D. A. Case, Application of a pairwise generalized Born model to proteins and nucleic acids: inclusion of salt effects, Theor. Chem. Acc., 1999, 101, 426–434 CrossRef CAS.
- J.-P. Ryckaert, G. Ciccotti and H. J. Berendsen, Numerical integration of the cartesian equations of motion of a system with constraints: molecular dynamics of n-alkanes, J. Comput. Phys., 1977, 23, 327–341 CrossRef CAS.
- D. J. Price and C. L. Brooks, A modified TIP3P water potential for simulation with Ewald summation, J. Chem. Phys., 2004, 121, 10096–10103 CrossRef CAS PubMed.
- H. J. Berendsen, J. P. M. Postma, W. F. van Gunsteren, A. DiNola and J. Haak, Molecular dynamics with coupling to an external bath, J. Chem. Phys., 1984, 81, 3684–3690 CrossRef CAS PubMed.
- Y. G. Mu, P. H. Nguyen and G. Stock, Energy landscape of a small peptide revealed by dihedral angle principal component analysis, Proteins, 2005, 58, 45–52 CrossRef CAS PubMed.
- A. Altis, P. H. Nguyen, R. Hegger and G. Stock, Dihedral angle principal component analysis of molecular dynamics simulations, J. Chem. Phys., 2007, 126, 244111 CrossRef PubMed.
- M. A. Rohrdanz, W. Zheng, M. Maggioni and C. Clementi, Determination of reaction coordinates via locally scaled diffusion map, J. Chem. Phys., 2011, 134, 124116 CrossRef PubMed.
- G. R. Bowman, Improved coarse-graining of Markov state models via explicit consideration of statistical uncertainty, J. Chem. Phys., 2012, 137, 134111 CrossRef PubMed.
- G. R. Bowman, L. Meng and X. Huang, Quantitative comparison of alternative methods for coarse-graining biological networks, J. Chem. Phys., 2013, 139, 121905 CrossRef PubMed.
- X. Huang, G. R. Bowman, S. Bacallado and V. S. Pande, Rapid equilibrium sampling initiated from nonequilibrium data, Proc. Natl. Acad. Sci. U. S. A., 2009, 106, 19765–19769 CrossRef CAS PubMed.
- N. Singhal, C. D. Snow and V. S. Pande, Using path sampling to build better Markovian state models: predicting the folding rate and mechanism of a tryptophan zipper beta hairpin, J. Chem. Phys., 2004, 121, 415–425 CrossRef CAS PubMed.
- R. van der Lee, M. Buljan, B. Lang, R. J. Weatheritt, G. W. Daughdrill, A. K. Dunker, M. Fuxreiter, J. Gough, J. Gsponer and D. T. Jones,
et al., Classification of Intrinsically Disordered Regions and Proteins, Chem. Rev., 2014, 114, 6589–6631 CrossRef CAS PubMed.
- Z. A. Levine, L. Larini, N. E. LaPointe, S. C. Feinstein and J.-E. Shea, Regulation and aggregation of intrinsically disordered peptides, Proc. Natl. Acad. Sci. U. S. A., 2015, 112, 2758–2763 CrossRef CAS PubMed.
Footnote |
† Electronic supplementary information (ESI) available: The analysis of convergence of simulations and secondary structure propensities of the histone H3 tail from implicit solvent simulations are included. Also shown are representative structures for the 9th and 122nd macro states, which feature the slowest transition in the MFPT analysis. See DOI: 10.1039/c5cp01858g |
|
This journal is © the Owner Societies 2015 |
Click here to see how this site uses Cookies. View our privacy policy here.