DOI:
10.1039/C4AN01978D
(Paper)
Analyst, 2015,
140, 2114-2120
Marker-free automated histopathological annotation of lung tumour subtypes by FTIR imaging
Received
29th October 2014
, Accepted 10th December 2014
First published on 10th December 2014
Abstract
By integration of FTIR imaging and a novel trained random forest classifier, lung tumour classes and subtypes of adenocarcinoma are identified in fresh-frozen tissue slides automated and marker-free. The tissue slices are collected under standard operation procedures within our consortium and characterized by current gold standards in histopathology. In addition, meta data of the patients are taken. The improved standards on sample collection and characterization results in higher accuracy and reproducibility as compared to former studies and allows here for the first time the identification of adenocarcinoma subtypes by this approach. The differentiation of subtypes is especially important for prognosis and therapeutic decision.
Introduction
Lung cancer is a major cause of cancer deaths worldwide.1 It was the most common cancer type in the world for several decades with an estimated 1.8 million new cases in 2012 (both sexes).2 The 5-year overall survival rate is 16.8% between 2004 and 2010 in the United States.3 There are two major types of lung cancer: small cell lung cancer (SCLC) and the non-small cell lung cancer types (NSCLC), with the main components being adenocarcinoma (ADC) and squamous cell carcinoma (SqCC). Semi-malignant carcinoid tumours with the typical and atypical variants and the benign hamartochondroma and thymoma were also investigated. Epidemiologic data clearly established cigarette smoking as the major cause of lung cancer.28 NSCLCs are mainly caused by tobacco consumption.10 SCLC is the fastest growing, most aggressive tumour and is treated in Germany according to the guidelines of the German Respiratory Society and the German Cancer Society with chemotherapy and radiation.4 The NSCLCs show, besides the main components adenocarcinoma and squamous cell carcinoma, also a third class, namely the large-cell carcinoma.5 A large challenge for pathologists is the inherent histological heterogeneity in the subsets of NSCLC.6,7 Exemplarily, adenocarcinoma shows several subtypes, with prognostic relevance for overall survival of patients.8 The subtypes of adenocarcinoma have different kinds of prognosis: poor (solid and micropapillary), favourable (nonmucinous lepidic), and intermediate (papillary and acinar).5,9,10 For the improvement of the classification of these subtypes immunohistochemical, histochemical and molecular diagnostic procedures can be used.11,12 But these characterizations are often not applied. They are time-consuming and expensive and often the low sample amount does not allow further studies.10
In addition to the lung tumour classes diffuse malignant mesothelioma (DMM) caused mostly by asbestos is identified, which can also be differentiated into subclasses.13 The DMM is a tumour of the pleura, but as the tumour grows, it replaces the pleural space, and the lung becomes entrapped. The gold standard for diagnosis is histology, including immunohistochemical panels.14
Since the newly proposed classification of adenocarcinoma of the lung might have a great impact on therapeutic decisions – even intraoperative – a fast, marker-free and automated – annotation would provide a large input in the healthcare system. FTIR imaging of tissue biopsies is a very promising emerging tool. The amount of tissue needed is very low as compared to conventional surgery; therefore endoscopic and minimally invasive techniques could be applied more frequently. In FTIR imaging these tissue slides of a biopsy are measured by an IR microscope spatially resolved. The spatially resolved spectra are assigned each to different index colours by unsupervised clustering algorithms in our hands, e.g. hierarchical cluster analysis (HCA).15 These result in index colour images of the tissue which are then compared to the later H&E stained tissue and annotated with the help of a pathologist. Thereby a database of characteristic spectral classes for the tissue components and especially for the tumour classes is implemented. Based on the established database, supervised algorithms – here the random forests – are trained. The random forest used here provides high robustness and excellent performance.16 Tumours are identified, thereby marker-free and automated in lung tissue slices from biopsies.
Spectral histopathology was applied recently to differentiate lung tumour classes.17 This approach was also applied to other entities, which have shown the broad potential of FTIR imaging for clinical diagnostics.18–22 However, most of these studies in early times have not used the methodical standards of clinical or epidemiological studies (e.g. study design, standard operating procedures – SOP, sample history). Many of the mentioned studies used tissue micro arrays (TMA), which are sometimes too small to provide an accurate diagnosis for highly heterogenic tumours.10,29 In the clinical epidemiological study presented here the tissue slices are well documented and minimized to the smallest possible variances in clinical everyday business. Meta data, for example, pre-treatment and smoking status, were recorded and regarded in the analysis. The meta data together with the highly accurate clinical histopathology used for the annotation is crucial for the next step after identification of cancer classes, the exact subtyping of lung cancer. This is challenging due to the high inherent histological heterogeneity of lung cancer subtypes.6,7
Bird et al. have shown on Biomax TMAs that spectral histopathology (SHP) is able to separate the major classes (adenocarcinoma – ADC, squamous cell carcinoma – SqCC, small cell carcinoma – SCLC and bronchioloalveolar carcinoma – BAC (no longer used by WHO)) of lung cancer with high sensitivity, specificity and accuracy for small cell carcinoma vs. non-small cell carcinoma (91.2%, 98.0% and 94.6%) and quite low sensitivity, specificity and accuracy for adenocarcinoma vs. bronchioloalveolar carcinoma (88.8%, 47.2% and 68.0%).17 This work inspired us to not only develop a SHP-based classifier that annotates the five tumour classes, but also go a step further and characterize also histopathological subtypes. Here, we focus on the subtypes of adenocarcinoma. The aim of the presented study is to provide a marker-free, automated diagnosis of lung cancer subtypes of adenocarcinoma, which agrees with the latest pathological gold standard.8 Based on these feasibility data, FTIR might be able to fasten diagnosis at the point of care and to optimize therapy decisions in personalized medicine.
The fresh frozen tissue samples taken during surgery and biopsy are characterized by a pathologist, all within our consortium PURE. Based on the annotation of the pathologists, representative spectra for the different classes and subtypes are selected. These spectra were used for the training of a supervised classifier, random forest (RF). To account for the high heterogeneity of lung cancer, it is necessary to create a hierarchical decision tree of several random forests. In the first level of decision, healthy and pathologically relevant is differentiated. The pathologically relevant regions are annotated in the second level of decision to the five tumour classes. In the third level of decision, subtypes for each tumour class are annotated. This study shows for the first time the automated and marker-free subtyping of a lung tumour class based on FTIR imaging.
Methods
Sample acquisition
The tissue samples are harvested within the context of surgical or bronchoscopic interventions following standard operating procedures (SOP). The SOP was developed together with the clinicians in the starting phase of the presented study to fit the best quality of fresh frozen samples. Bird et al. (2012)17 used paraffinized commercially available tissue microarray samples from Biomax. We decided to use larger fresh frozen tissue samples because they provide an improved pathological annotation, a recorded clinical history of the patient, and the corresponding meta data (for example, sex, age, smoker etc.) and enables further analysis by proteomics and next generation sequencing. After harvesting the tissue samples were cooled at 4 °C as fast as possible and transported within about 10 minutes to the pathologist, who decided which tissue material is to be used for diagnostics (priority) or this study. The tissue is washed with isotonic saline and afterwards frozen for cutting in the cryostat. The largest allowed time up to this point is 30 minutes. The thin tissue slices were deposited on LowE slides (Kevley, Chesterland, OH, USA). The LowE slides were chosen because of low costs and because they are rather similar to the clinically used glass slides. For every new sample the cryostat was cleaned and the blade was changed to reduce the carryover of cells from different specimens. Afterwards the samples are stored at −80 °C till measurement. All steps were done as fast as possible and are well documented. The SOP guarantees high quality samples with very low degradation of the tissue and its proteins, DNA and RNA. It was developed under consideration of molecular biological standards.
Histological staining
The tissue samples were stained with Hematoxylin and Eosin (H&E) after FTIR-spectroscopic measurement. The use of the same samples allows more precise overlays between the spectral image and the classical stained image. For the staining the tissue samples were washed with Milli-Q water, stained for 50 seconds with Harris Hematoxylin (VWR, Germany), washed with water, counterstained with eosin (Merck, Germany), dehydrated with increasing gradients of alcohol, and mounted with Euparal (ROTH, Germany). The stained sections were imaged automatically with an Olympus BX43 microscope.
FTIR-imaging
FTIR-imaging was performed in transflection (reflection-absorption) of LowE slides. It is known that there is an inherent problem of transflection-mode infrared spectroscopic microscopy which leads to a shift in the ratio of absorption bands.23,24 In our study this effect does not affect the identification of lung tumour subtypes, because the thickness of the samples is quite stable; therefore the electric field standing wave is not influencing the analysis. Two spectrometers of different manufacturers were used. The first instrument was a Bruker (Ettlingen, Germany) Hyperion 3000 infrared microscope equipped with a 64 × 64 nitrogen-cooled mercury–cadmium–telluride (MCT) focal plane array (FPA) detector and the second instrument was an Agilent (Santa Clara, California, USA) Model Cary 620 infrared microscope equipped with a 128 × 128 pixel liquid nitrogen-cooled (MCT) focal plane array detector, henceforth referred to as the “Hyperion” and “Cary”. Wavenumbers have been collected between 2700–950 cm−1 (Hyperion) and between 3700–950 cm−1 (Cary) at a spectral resolution of 4 cm−1. 32 scans (Hyperion) or 128 scans (Cary) were co-added for sample and background spectra. The mapped pixel resolution is ∼2.7 μm (Hyperion) and ∼5.5 μm (Cary), so the tissue sampling area is nearly 172 × 172 μm (Hyperion) and 715 × 715 μm (Cary) for each FPA-field. This leads to oversampling due to a much lower optical resolution in the infrared. The results in this study and in previous studies show that the oversampling has no effect on the cancer detection.16 All index colour images presented in this publication are from the original dataset of unbinned data. The instrument and the microscope chamber of both, the Bruker and the Cary instrument, are continuously purged with dry air to avoid spectral contributions of atmospheric water. Furthermore we installed a 24/7 liquid nitrogen cooling supply (Norhof; Maarssen, Netherlands) at both systems, which enables us to measure constantly 24 hours a day, 7 days a week.
The Fourier transformation was performed with a power phase correction and Blackman–Harris 3-term apodization for the Hyperion. The system is controlled by an Opus, Bruker, macro that enables us to measure any number of FPA fields of 64 × 64 pixels in one measurement, which were stitched automatically after Fourier transformation in Matlab (MathWorks, Natick, Massachusetts, USA). Each raw spectral vector consisted of 1362 data points (resolution 4 cm−1, zero-filling 4, upper limit 3949 cm−1). For the Cary, the Fourier transformation was done using Mertz phase correction and Blackman–Harris 4-term apodization. The measurements were done in mosaic mode of the Agilent software. Individual mosaic tiles, each measuring 128 × 128 pixels, were stitched automatically after measurements. Each raw spectral vector consisted of 1428 data points (resolution 4 cm−1, zero-filling 4, upper limit 5266 cm−1). The stitching for both instrument datasets was performed in Matlab.
Spectral pre-processing and analysing
The raw datasets with up to 15 million spectra with around 1400 spectral elements each were pre-processed in Matlab as follows. The pre-processing step is necessary due to dispersion effects (e.g. rMie scattering) and variation in the slice thickness of the samples. After stitching the raw datasets a quality test is performed. This test sorts out the background, disturbed spectra from the vicinity of voids or cracks in the tissue, or pixel spectra from highly spherical, small cells such as lymphocytes, which may exhibit strong scattering artefacts that have been attributed to “resonance Mie” scattering.25 The spectra were tested for signal to noise ratio and signal level. All spectra that succeed the quality test were subjected to an EMSC-based Mie and resonance-Mie scattering correction26 from 2300 to 950 cm−1, with one iteration step. A higher number of iteration steps (up to 20) were tested but due to low scattering effects it does not alter the final classification. For up to twenty million spectra per measurement this also saves time in the processing. Unsupervised hierarchical and k-means clustering and supervised Random Forests (RF)13 were performed in the spectral range of 1800 to 950 cm−1. The unsupervised methods are performed on the second derivative of smoothed spectra. The smoothing was done using a 9 point Savitzky–Golay filter.27 The training dataset of the Random Forests was created from unsmoothed absorption spectra. For the first level RF (healthy/pathologic) the spectra are interpolated down to 100 equidistant data points and for the second (tumour classes) and third RF (subtyping) down to 385 from 1800–950 cm−1. These adjusted the spectra were measured on the Hyperion and the Cary to a similar format and save the calculation time in the first level RF. A separate feature selection was not performed. The RF algorithm carries out a weighting of features based on Gini importance and chooses points randomly for decision.15 Accordingly the high number of trees, here 500 are used, makes the feature selection redundant. Second derivatives on smoothed spectra were tested for the training dataset but do not lead to higher accuracy for the RF. To determine the accuracy of the training dataset a ten times Monte Carlo cross validation was used which yielded accuracies of more than 97%.
Results
Tissue slices of 92 patients (101 samples) were measured. Meta data, e.g. medication, the previous illness or the last chemotherapy, smoking status, age, sex, etc., were recorded for each patient. 20 patients were chosen for the training dataset. The patients differ in smoking status, sex, cancer types, grading, and staging. All of them did not have any previous treatment like chemotherapy (Table 1). This exclusion criterion was chosen to minimize disturbances of the reference data by the patient's treatment. Furthermore, patients chosen as healthy are non-smokers to minimize possible inflammatory markers in the spectra.
Table 1 Patients included in this study. The first row presents all patients. The second row shows the training set
No. charge |
No. sample |
Smoking state |
Sex |
Non-smoker |
Smoker |
Unknown |
Ex-smoker > 10 years |
Ex-smoker 5–10 years |
Ex-smoker < 5 years |
Ex-smoker u |
m |
f |
u |
92 |
101 |
18 |
30 |
5 |
17 |
7 |
17 |
7 |
68 |
30 |
3 |
20 |
20 |
6 |
8 |
1 |
1 |
1 |
2 |
1 |
15 |
4 |
1 |
For the subtyping of a tumour class, the accurate detection of pathologic regions and their tumour class has to be performed in the first step. The first level RF distinguishes between healthy tissue, pathologically noticeable tissue, blood, and calcifications. The second level RF distinguishes between the tumour classes, inflammation/necrosis, spreading pleura, and lymphocyte aggregate. Such levels were also identified before mostly on Biomax samples.17 Here we show for the first time a third level RF, the subtyping of adenocarcinoma as illustrated in Fig. 1. Adenocarcinomas were chosen in this study because their subtyping is of prognostic value. The different subtypes of adenocarcinoma have the following different kinds of diagnosis: poor (solid and micropapillary), favourable (nonmucinous lepidic), and intermediate (papillary and acinar).5,9,10
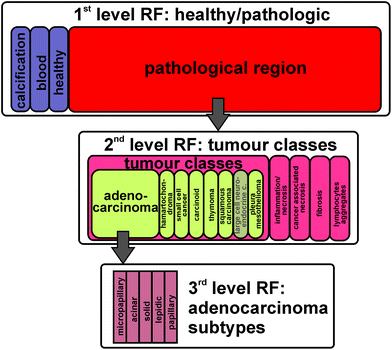 |
| Fig. 1 A set of three rfs was trained: 1st level: healthy/pathologic decision; 2nd level: tumour class decision; 3rd subtyping of adenocarinomas. | |
The first level RF: healthy/pathologic
The first level RF separates healthy tissue, pathologically noticeable tissue, blood, and calcifications. Blood and calcifications are typical confounders in the analysis of lung tissue. Healthy tissue includes all pathologically normal tissue types while pathologically noticeable tissue means all tissues that are altered like inflammation, necrosis or cancer. In Fig. 2 the separation between healthy (green) and pathologically noticeable regions (red) is shown exemplarily. Compared with the H&E-stained image (A) the index colour image (B) reflects the morphology very precisely. The spectra of the noticeable pathological region are then analysed further on the second level RF.
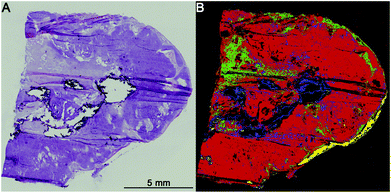 |
| Fig. 2 1st level rf: healthy/pathologic decision. Colour scheme: pathologic regions (red), healthy (green), and calcifications (blue). Shown is a hamartochondroma: (A) H&E stained and (B) index colour image of the 1st level rf. | |
The second level RF: pathological classification
The second level RF separates the tumour classes, inflammation/necrosis, spreading pleura, and lymphocyte aggregates. Detectable tumour classes are the non-small cell lung carcinomas (NSCLC), adenocarcinoma (ADC) and squamous cell carcinoma (SqCC), the small cell lung cancer (SCLC), hamartochondroma, carcinoids, thymoma, large cell neuroendocrine carcinoma (not shown, because only one patient is in the study), and diffuse malignant mesothelioma (DMM). The different tumour classes as identified by spectral histopathology in comparison with the H&E stained samples are shown in Fig. 3. The index colour images represent the result of the second level RF at which each colour represents a specific tumour. Inflammation and necrosis, which are abundant in low differentiated tumours, are shown in blue and dark green, respectively. All tumour classes identified by spectral pathology agree precisely with the diagnosis of the pathologists based on the H&E-stained image also shown in Fig. 3. Healthy and other tissue spectra are not regarded in the second level RF. Therefore these tissue regions are represented in black. In Fig. 3a SCLC is shown. It represents in the Western world around 13% of all lung cancers. Almost all patients have a history of smoking. SCLC is very aggressive. One-third of the patients have a localised disease, which results in high mortality. The shown SCLC in Fig. 3a is taken from such a localized disease. It is nicely identified and annotated by our classifier. Because most patients with SCLC are treated with radiation and chemotherapy, only three samples of fresh frozen tissue from untreated patients were available besides the training sample. The validation sample (shown in Fig. 3) can be identified as small cell lung cancer. Less than 2% of all observed spectra have a false positive assignment to tumour classes different from SCLC.
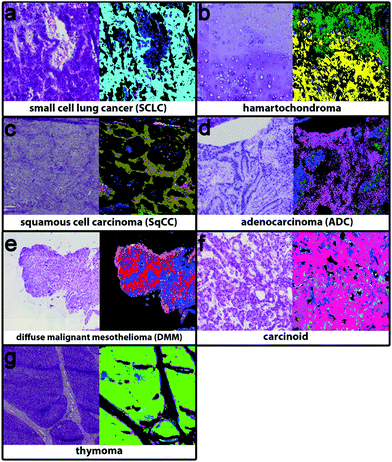 |
| Fig. 3 2nd level rf: tumour class decision. (a) Small cell cancer (cyan), (b) hamartochondroma (yellow), (c) squamous cell carcinoma (olive), (d) carcinoid (magenta), (e) pleura mesothelioma (red), (f) adenocarcinoma (pink), and (g) thymoma (light green), and inflammation/necrosis (blue and dark green). | |
Hamartochondroma shown in Fig. 3b in yellow is a benign lung tumour whose origin is mesenchymal. Two of the seven measured samples were used for the training, one smoker and one non-smoker. The spectra of this class differ clearly from the other tumour classes. The five validation samples are identified correctly by our system. The comparison of the H&E-stained tissue with the index colour image supports this. For precise diagnosis of NSCLC in the first step SqCC and ADC have to be differentiated. Samples of eight patients with SqCC were measured (two as reference spectra and the remaining six for validation). All patients are smokers or ex-smokers. This is expected, because SqCCs are usually associated with tobacco abuses. The SqCC (olive, Fig. 3c) annotation is supported by the H&E stained image. The SqCC is clearly distinguished from ADCs and carcinoids. Only 8% of all measured spectra deviate and are assigned to ADC and carcinoid, whereas the pathologist assigned it to SqCC. 40% of all lung cancers are ADCs, mostly associated with smoking. Among all ADC patients (n = 52) in the study, only 3 (5.8%) non-smokers were detected. Eight of the patients were used as reference data (smokers, ex- and non-smokers). 49 samples were used for validation. The ADC, shown in Fig. 3d, is represented in magenta and shows precise agreement with the H&E image. All validation patients for ADC are correctly identified. The remaining tumour classes are DMM, carcinoid and thymoma (Fig. 3e–g). The DMM class shown in red relies on 2 reference samples and is validated by 10. Even if for thymoma only two samples were available, the index colour image (thymoma – light green) agrees nicely with the H&E image. In order to regard all tumour classes, thymoma is included on the 2nd level even if the patient number is low. Summing up, the implemented RF based classifiers identify all lung cancer classes except large cell neuroendocrine carcinoma, because only one patient was included in this analysis. The tumour classes can be identified with a high accuracy of 96% as compared to the final diagnosis of the pathologist. Bird et al. (2012)17 provided sensitivity and specificity values for their lung cancer classifier. They used binary classifiers but our data show that even for the healthy/pathologic decision the results improve largely if we use more than two classes besides healthy and pathologically relevant as shown in Fig. 1. For multiclass classifiers the sensitivity and specificity cannot be calculated and so it cannot be presented here.
However, today, for the prognosis of the cancer and for the therapeutic decision, the accurate subtyping of the tumour classes is important. In principle, immunohistochemical and molecular biological diagnostics can be used for subtyping. But these methods are time demanding, expensive and often not as precise as recommended.10 Therefore, here a third level RF is applied to distinguish exemplarily between subtypes of adenocarcinomas.
The third level RF: subtypes of adenocarcinoma
The results for the subtyping of ADCs are presented in Fig. 4. The subtype identification of ADC can be used as a prognostic marker. Lepidic ADC has the lowest mortality rate; it is increased in acinar than in papillary, micropapillary, and finally solid subtypes.5 70 to 90% of ADCs show not only one subclass but a heterogeneous mixture. Therefore all subtypes have to be regarded in the analysis. Sometimes annotations of the NSCLC's subtype are too challenging due to such a heterogeneity and then the tumours are classified as NSCLC-NOS (NSCLC, not otherwise specified). For this challenging prognostic diagnosis spectral histopathology is quite promising to support this challenging diagnostic decision.
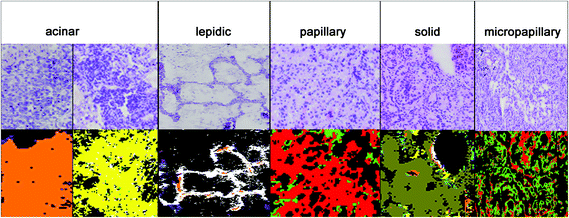 |
| Fig. 4 3rd level rf: adenocarcinoma subtyping. Presented here, starting with the lowest up to the highest mortality, are acinar (orange and yellow), lepidic (white), papillary (red), solid (olive), and micropapillary (green). | |
In Fig. 4, the diagnosis of the ADC subtypes is compared to the corresponding H&E stained images. The index colour images of the third level RF identified the correct subtypes: solid (olive), micropapillary (light green), papillary (red), lepidic (white), and acinar (yellow and orange). The acinar subtype is actually highly heterogenic and it has to be represented by two classes. All cases have been annotated correctly as compared to the clinical pathological gold standards used today.
The subtypes are identified automated and marker-free without further treatment of the tissue slice. We think that this is a breakthrough because for the first time not only cancer classes are distinguished with a high accuracy of 97%, but also subclasses of ADC tumours with an accuracy of 95%. The subclassification is a prognostic marker.
Conclusion
In this study we present a new marker-free and automated diagnostic tool for point of care decisions based on FTIR imaging. Not only all lung tumour classes are annotated but also for the first time the prognostic subtypes of adenocarcinoma. Using the newly proposed classification, solid, micropapillary, papillary, lepidic, and acinar subtypes are identified. The approach will allow reducing the intra- and inter-operator variability due to its reproducibility, objectivity, and improved accuracy over present-day methodologies in the lung tumour diagnostics. This is an emerging need in personalized medicine. The approach has to be validated in a larger and independent study in the future.
Acknowledgements
This research was funded by the German Social Accident Insurance (DGUV; project FP339A). The responsibility for this publication and the presented results is in the hands of the authors. Further, we have to thank Max Diem and Cireca for their assistance.
References
- G. Cox, J. L. Jones, A. Andi, D. A. Waller and K. J. O'Byrne, Thorax, 2001, 56, 561–566 CrossRef CAS.
-
J. Ferlay, I. Soerjomataram, M. Ervik, R. Dikshit, S. Eser, C. Mathers, M. Rebelo, D. M. Parkin, D. Forman and F. Bray, GLOBOCAN 2012 v1.0, Cancer Incidence and Mortality Worldwide: IARC CancerBase No. 11 [Internet]. Lyon, France: International Agency for Research on Cancer; 2013. Available from: http://globocan.iarc.fr, accessed on 26/11/2014.
-
N. Howlader, A. M. Noone, M. Krapcho, J. Garshell, D. Miller, S. F. Altekruse, C. L. Kosary, M. Yu, J. Ruhl, Z. Tatalovich, A. Mariotto, D. R. Lewis, H. S. Chen, E. J. Feuer and K. A. Cronin, SEER Cancer Statistics Review, 1975–2011. Based on November 2013 SEER data submission, National Cancer Institute, Bethesda, MD, 2014 Search PubMed.
- G. Goeckenjan, H. Sitter, M. Thomas, D. Branscheid, M. Flentje, F. Griesinger, N. Niederle, M. Stuschke, T. Blum, K. M. Deppermann, J. H. Ficker, L. Freitag, A. S. Lübbe, T. Reinhold, E. Späth-Schwalbe, D. Ukena, M. Wickert, M. Wolf, S. Andreas, T. Auberger, R. P. Baum, B. Baysal, J. Beuth, H. Bickeböller, A. Böcking, R. M. Bohle, I. Brüske, O. Burghuber, N. Dickgreber, S. Diederich, H. Dienemann, W. Eberhardt, S. Eggeling, T. Fink, B. Fischer, M. Franke, G. Friedel, T. Gauler, S. Gütz, H. Hautmann, A. Hellmann, D. Hellwig, F. Herth, C. P. Heussel, W. Hilbe, F. Hoffmeyer, M. Horneber, R. M. Huber, J. Hübner, H. U. Kauczor, K. Kirchbacher, D. Kirsten, T. Kraus, S. M. Lang, U. Martens, A. Mohn-Staudner, K. M. Müller, J. Müller-Nordhorn, D. Nowak, U. Ochmann, B. Passlick, I. Petersen, R. Pirker, B. Pokrajac, M. Reck, S. Riha, C. Rübe, A. Schmittel, N. Schönfeld, W. Schütte, M. Serke, G. Stamatis, M. Steingräber, M. Steins, E. Stoelben, L. Swoboda, H. Teschler, H. W. Tessen, M. Weber, A. Werner, H. E. Wichmann, E. Irlinger Wimmer, C. Witt and H. Worth, Pneumologie, 2011, 65, 39–59 CAS.
- W. D. Travis, E. Brambilla and G. J. Riely, J. Clin. Oncol., 2013, 31, 992–1001 CrossRef CAS PubMed.
- P. S. Loo, S. C. Thomas, M. C. Nicolson, M. N. Fyfe and K. M. Kerr, J. Thorac. Oncol., 2010, 5, 442–447 CrossRef PubMed.
- V. L. Roggli, R. T. Vollmer, S. D. Greenberg, M. H. McGavran, H. J. Spjut and R. Yesner, Hum. Pathol., 1985, 16, 569–579 CrossRef CAS.
- W. D. Travis, N. Rekhtman, G. J. Riley, K. R. Geisinger, H. Asamura, E. Brambilla, K. Garg, F. R. Hirsch, M. Noguchi, C. A. Powell, V. W. Rusch, G. Scagliotti and Y. Yatabe, J. Thorac. Oncol., 2010, 5, 411–414 CrossRef PubMed.
- A. Yoshizawa, N. Motoi, G. J. Riely, C. S. Sima, W. L. Gerald, M. G. Kris, B. J. Park, V. W. Rusch and W. D. Travis, Mod. Pathol., 2011, 24, 653–664 CrossRef CAS PubMed.
- W. D. Travis, E. Brambilla, M. Noguchi, A. G. Nicholson, K. R. Geisinger, Y. Yatabe, D. G. Beer, C. A. Powell, G. J. Riely, P. E. Van Schil, K. Garg, J. H. M. Austin, H. Asamura, V. W. Rusch, F. R. Hirsch, G. Scagliotti, T. Mitsudomi, R. M. Huber, Y. Ishikawa, J. Jett, M. Sanchez-Cespedes, J.-P. Sculier, T. Takahashi, M. Tsuboi, J. Vansteenkiste, I. Wistuba, P. C. Yang, D. Aberle, C. Brambilla, D. Flieder, W. Franklin, A. Gazdar, M. Gould, P. Hasleton, D. Henderson, B. Johnson, D. Johnson, K. Kerr, K. Kuriyama, J. S. Lee, V. A. Miller, I. Petersen, V. Roggli, R. Rosell, N. Saijo, E. Thunnissen, M. Tsao and D. Yankelewitz, J. Thorac. Oncol., 2011, 6, 244–285 CrossRef PubMed.
- J. Yoo, J. H. Jung, M. A. Lee, K. J. Seo, B. Y. Shim, S. H. Kim, D. G. Cho, M. Im Ahn, C. H. Kim, K. D. Cho, S. J. Kang and H. K. Kim, J. Korean Med. Sci., 2007, 22, 318 CrossRef.
- J. Jagirdar, Arch. Pathol. Lab. Med., 2008, 132, 384–396 Search PubMed.
- C. Bianchi and T. Bianchi, Ind. Health, 2007, 45, 379–387 CrossRef.
- A. N. Husain, T. V. Colby, N. G. Ordóñez, T. Krausz, A. Borczuk, P. T. Cagle, L. R. Chirieac, A. Churg, F. Galateau-Salle, A. R. Gibbs, A. M. Gown, S. P. Hammar, L. A. Litzky, V. L. Roggli, W. D. Travis and M. R. Wick, Arc. Pathol. Lab. Med., 2009, 133, 1317–1331 Search PubMed.
- L. Breiman, Mach. Learn., 2001, 45, 5–32 CrossRef.
- A. Kallenbach-Thieltges, F. Großerüschkamp, A. Mosig, M. Diem, A. Tannapfel and K. Gerwert, J. Biophotonics, 2013, 6, 88–100 CrossRef PubMed.
- B. Bird, M. Miljković, S. Remiszewski, A. Akalin, M. Kon and M. Diem, Lab. Invest., 2012, 92, 1358–1373 CrossRef PubMed.
- M. Diem, M. Miljković, B. Bird, T. Chernenko, J. Schubert, E. Marcsisin, A. Mazur, E. Kingston, E. Zuser, K. Papamarkakis and N. Laver, Spectrosc. Int. J., 2012, 27, 463–496 CrossRef CAS PubMed.
- L. Chiriboga, P. Xie, H. Yee, V. Vigorita, D. Zarou, D. Zakim and M. Diem, Biospectroscopy, 1998, 4, 47–53 CrossRef CAS.
- C. Petibois, B. Drogat, A. Bikfalvi, G. Déléris and M. Moenner, FEBS Lett., 2007, 581, 5469–5474 CrossRef CAS PubMed.
- E. Gazi, M. Baker, J. Dwyer, N. P. Lockyer, P. Gardner, J. H. Shanks, R. S. Reeve, C. A. Hart, N. W. Clarke and M. D. Brown, Eur. Urol., 2006, 50, 750–761 CrossRef PubMed.
- O. J. Old, L. M. Fullwood, R. Scott, G. R. Lloyd, L. M. Almond, N. A. Shepherd, N. Stone, H. Barr and C. Kendall, Anal. Methods, 2014, 6, 3901 RSC.
- P. Bassan, J. Lee, A. Sachdeva, J. Pissardini, K. M. Dorling, J. S. Fletcher, A. Henderson and P. Gardner, Analyst, 2012, 138, 144 RSC.
- M. Miljkovic, B. Bird and M. Diem, Analyst, 2012, 137, 3954–3964 RSC.
- P. Bassan, H. J. Byrne, F. Bonnier, J. Lee, P. Dumas and P. Gardner, Analyst, 2009, 134, 1586–1593 RSC.
- P. Bassan, A. Kohler, H. Martens, J. Lee, H. J. Byrne, P. Dumas, E. Gazi, M. Brown, N. Clarke and P. Gardner, Analyst, 2010, 135, 268–277 RSC.
- A. Savitzky and M. J. E. Golay, Anal. Chem., 1964, 36, 1627–1639 CrossRef CAS.
- S. S. Hecht, J. Natl. Cancer Inst., 1999, 91(14), 1194–1210 CrossRef CAS PubMed.
- C. E. Gillett, R. J. Springall, D. M. Barnes and A. M. Hanby, J. Pathol., 2000, 192, 549–553 CrossRef CAS.
Footnote |
† Both authors contributed equally. |
|
This journal is © The Royal Society of Chemistry 2015 |
Click here to see how this site uses Cookies. View our privacy policy here.