DOI:
10.1039/C3MB70240E
(Paper)
Mol. BioSyst., 2014,
10, 74-85
Ion-pairing chromatography on a porous graphitic carbon column coupled with time-of-flight mass spectrometry for targeted and untargeted profiling of amino acid biomarkers involved in Candida albicans biofilm formation†
Received
21st June 2013
, Accepted 2nd October 2013
First published on 3rd October 2013
Abstract
Background: Candida albicans, the most common fungal pathogen related to colonization and biofilm formation on the surfaces of indwelling medical devices, shows high resistance to the most commonly used antifungal drugs. In this study, an ion-pairing chromatography-porous graphitic carbon column coupled with a time-of-flight mass spectrometry (IP-PGC-TOF/MS) system was developed for targeted and untargeted profiling of metabolites involved in biofilm and planktonic growth of C. albicans. Using untargeted profiling analysis, 16 differential metabolites were screened and identified as potential biomarkers, most of which were amino acids or related compounds. Based on untargeted profiling analysis, targeted quantitative analysis of 22 amino acids was established and carefully evaluated using stable isotope-labeled internal standards. Among them, 9 amino acids that were not screened by untargeted profiling were further characterized as new biomarkers. Finally, a total of 25 potential biomarkers were screened using the combined targeted and untargeted strategy, among which 16 were characterized for the first time. Our results confirmed that amino acid metabolism and polyamine metabolism were at a high level in biofilms, except for some new biomarkers including ornithine, arginine and proline that were directly related to ornithine. Further experiments were carried out on the ornithine decarboxylase-negative (spe1Δ) mutant, and the results showed that the consumption of ornithine for putrescine biosynthesis has a significant impact on biofilm formation and may prove to be a drug target for resolving drug resistance of C. albicans. This study provides a systematic view of changes in amino acid metabolism during C. albicans biofilm formation by a combination of targeted and untargeted profiling using an original IP-PGC-TOF/MS method. It is a feasible approach for characterizing subtle variations and screening novel biomarkers from the microbial metabolome.
Introduction
Candida albicans is a pathogenic fungus that can be recognized by the innate immune system and causes life-threatening systemic infections in patients whose normal host defense mechanisms are impaired.1 In comparison with the planktonic counterparts, C. albicans cells in biofilm mode display different phenotypic traits, which are intensely resistant to both host immune factors and antimicrobial agents including amphotericin B and azoles.2,3 Knowing that C. albicans uses complex metabolic strategies to optimize biofilm growth during invasive disease and can use different carbon metabolic pathways at different stages of systemic infection,4 determination of the cellular metabolism and metabolic regulation has great importance for elucidating the mechanism of biofilm formation, and metabolomics researchers have been increasingly involved in the metabolic response analysis of C. albicans.5,6
Almost all metabolomics experiments fall into one of the two categories: metabolic profiling and quantitative metabolomics. Due to practical limitations, such as the absence of standard reference and the limitation of detection tools, most current studies on metabolic profiling use the mode of relative quantification,7–9 though it is unable to characterize accurately the contents of different types of metabolites in biological samples. On the other hand, quantitative metabolome data can be directly and precisely mapped onto the metabolic network, which facilitates many types of data analysis, such as identification of reporter reactions and network-embedded thermodynamic analysis.10–12 However, quantitative metabolomics requires pure standards, which are not commercially available for all intracellular metabolites and can be very costly for large scale metabolomics analyses.13 Given the current technical conditions, combining metabolic profiling analysis with quantification of targeted metabolites may be a compromised way to interpret the elaborate mechanism of metabolic states.
In our previous research, we applied GC-MS based untargeted metabolic profiling to compare the biofilm and planktonic cell C. albicans growth.14 We found that there was a striking difference in the metabolite profiles, ranging from energy metabolism to amino acid biosynthesis. Several amino acids, including alanine, aspartate, asparagine, threonine, valine and glycine, were upregulated almost during the whole process of biofilm development compared to planktonic cells, indicating that amino acid metabolism plays a critical role in C. albicans biofilm formation. Unfortunately, the global amino acid metabolic network cannot be definitely characterized and well elucidated, because some key amino acids such as arginine, citrulline, ornithine and proline are absent from the GC-MS spectra or cannot be characterized by multivariate statistics. The main reason could be ascribed to the imprecision of the two-step chemical derivation and relative quantification procedures, leading to incomplete elucidation of variations in amino acid metabolism during biofilm development. Thus, it is necessary to develop a simple and robust method that can monitor global amino acids in C. albicans cells and help understand the underlying mechanism of how amino acid metabolism participates in biofilm formation.
Due to the inability to separate more polar amino acids using conventional reversed-phase (RP)-LC, and amino acid signal suppression in electrospray ionization (ESI)-MS caused by co-elution of components in complex biological matrices, intracellular amino acids were generally analyzed by GC-MS.15–17 The pre-column derivatization procedure of samples is always required, although it is time-consuming and limits the throughput of metabolite profiling.18 Consequently, several LC-ESI-MS strategies for analysis of underivatized amino acids, such as hydrophilic interaction chromatography (HILIC)-MS,19,20 ion-pairing chromatography (IP) on RP-LC-MS21 and strong cation exchange (SCX)-MS,22 have been developed. Recently, the use of volatile ion pair reagents in LC-MS separation of various types of metabolites has been mentioned in some studies.13,21,23,24 Ion pair chromatography is one such type of chromatography that utilizes hydrophobic stationary phases and MS compatible solvent to separate ionic compounds by addition of ion pair reagents to the mobile phase. Through formation of ion pairs with oppositely charged ion pair reagents, the retention, response and selectivity of charged analytes could be dramatically improved.25 Owing to the good suitability with the ESI interface and the powerful separation ability of different types of metabolites with high polarity, the application of volatile ion pair reagents in LC-MS is a simple and reliable method for obtaining abundant metabolome information. However, the complete determination of amino acids by IP-RP-LC-MS might be prevented by coelutions and the limited pH range of the mobile phase, which masks the elution of some amino acids.26,27
It has been reported that a porous graphitic carbon (PGC) column can provide significantly better retention and selectivity for polar compounds.28,29 In addition to its strong reversed-phase behavior, delocalized π-electrons from the graphite can participate in the retention mechanism and even dominate it.30 As a result, PGC often exhibits unexpected properties and greater selectivity than a reversed-phase column toward aromatic or other conjugate systems and polar compounds.31,32 Chaimbault et al.33 used different ion pair reagents on a PGC stationary phase and showed that the IP-PGC system presented a totally different elution order and better separation efficiency for some strong polar components as compared with the IP-RP system. In addition, Petritis et al.34 used electrospray ionisation tandem mass spectrometry as a detector and demonstrated that the ion pair reagent NFPA was also suitable as an MS detector. In the present study, we chose time-of-flight mass spectrometry (TOFMS) as the detector, knowing that it can provide accurate mass measurements, as well as full scan spectral sensitivity, which is well-suited to perform screening and structure confirmation of untargeted compounds when it is combined with advanced LC techniques.21,35 Thus, the combination of LC and TOFMS can be expedient for sensitively tracing potential biomarkers.
In this study, an IP-PGC-TOF/MS system was developed for targeted and untargeted profiling of metabolites in biofilm and planktonic cell modes of C. albicans. The chromatographic conditions were carefully optimized for maximum resolution and suitability. Based on the results of untargeted profiling with IP-PGC-TOF/MS, amino acid metabolism was chosen for further targeted metabolite profiling using the same chromatographic conditions. A total of 22 key amino acids included in C. albicans central carbon metabolism were selected for quantitative analysis and calibrated by stable isotope-labeled amino acids. The mass-to-charge ratio (m/z) of all amino acids can be verified to within 1–5 ppm, thus reducing mis-identification of target amino acids in the presence of several co-eluting matrix components of similar molecular weight. These analyses led to a further evaluation of the mechanisms responsible for the phenotypic changes that occur during C. albicans biofilm development. To the best of our knowledge, this is the first time an IP-PGC-TOF/MS system has been developed and optimized for both targeted and untargeted profiling of metabolites from C. albicans.
Materials and methods
The schematic flowchart of the metabolic profiling strategy used in this study is illustrated in Fig. 1.
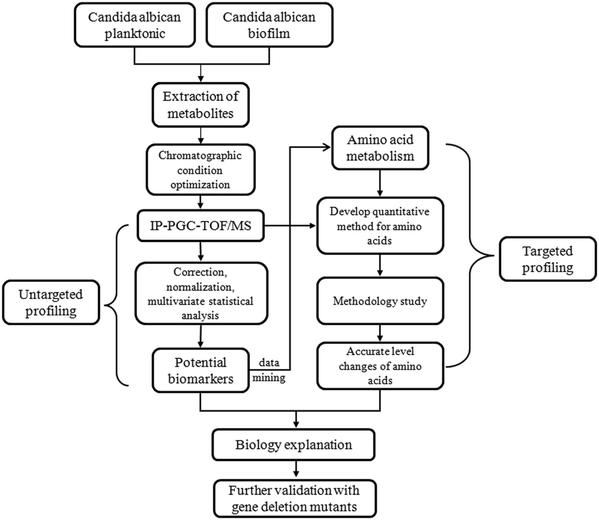 |
| Fig. 1 Schematic flowchart of the metabolic profiling strategy used in this study. Intracellular metabolites were extracted and analyzed by IP-PGC-TOFMS. Multivariate statistics were applied to extract meaningful information from the complex IP-PGC-TOFMS spectral data. Compounds with significant contributions to the variation of metabolic profiles between planktonic and biofilm cells were identified. Methods for quantitative analysis of 22 key amino acids in C. albicans metabolic pathways were established and carefully evaluated. Bioactivities of spe1Δ mutant biofilm cells were evaluated by CSH assay. | |
Chemicals
All metabolite standards were purchased from Aladdin reagent (Shanghai, China). Internal standards (IS) were isotope-labeled compounds (uniformly 13C > 98%, 15N > 98%) from Dibo chemical and technology Co., Ltd (Shanghai, China). Nonafluoropentanoic acid (NFPA) was obtained from Bailingwei chemical and technology Co., Ltd (Beijing, China). Solvents used for chromatography were of HPLC-MS grade (Merck, Darmstadt, Germany). Ultrapure water was prepared with a Milli-Q water purification system (Millipore, Bedford, MA, USA). Other reagents were of analytical grade.
Strains and culture conditions
Stock cultures of C. albicans strain SC5314 were routinely maintained on sabouraud dextrose agar, and propagated in yeast peptone dextrose (YPD) medium (1% w/v yeast extract, 2% w/v peptone, and 2% w/v dextrose) in an orbital shaker at 30 °C (200 rpm) for 16 h. Planktonic fermentation and biofilm culture were both performed at 37 °C (50 rpm) with the same initial cell density (1 × 106 cells mL−1) in RPMI-1640 medium (Gibco, Rockville, MD, USA). 250 mL tissue culture flasks (Nunc, Roskilde, Denmark) were used for biofilm cultivation. Following initial 1 h adhesion, the medium was aspirated, and non-adherent cells were removed and fresh RPMI-1640 was then added to the adherent cells. The flasks were incubated for further 24 h. The planktonic cells were cultivated in 250 mL flasks under the same cultivation conditions as those for the biofilm, except for the omitted adhesion and change of new medium step. Six biological replicates were prepared for each condition for a total of 12 samples. The samples used for validation of the method were cultivated under the conditions similar to those used for the above planktonic cell/biofilm samples, except that fermentation was performed at 30 °C (200 rpm).
Isolation of metabolites from biofilm and planktonic cultures
Metabolites were serially extracted essentially as described by Bajad et al.36 with slight modifications. In brief, at the end of the incubation period, either planktonic or biofilm cells were washed rapidly with precooled ultrapure sterilized water, followed by addition of 500 μL 80
:
20 methanol
:
water at −80 °C to the pellet. After 15 min sonication in an ice bath, the sample was spun in a micro-centrifuge at 13
200 rpm for 5 min at 4 °C to remove the soluble extract. The pellet was then re-suspended in 500 μL 80
:
20 methanol
:
water, sonicated for 15 min, and then centrifuged to yield a second clear extract, which was combined with the first extract. A total of 1 mL extract was obtained for each sample. At the end of the extraction step, either planktonic or resuspended biofilm cells were filtered through a filter (0.45 μm) and washed three times with ultrapure sterilized water. Cells were dried at room temperature to constant weight. The dry cell weight was measured at least three times, and the mean value was regarded as the biomass of each sample.
IP-PGC-TOF/MS analysis
All experiments were carried out on an Agilent 1100 series HPLC system (Agilent, Waldbronn, Germany) consisting of a quaternary pump, an on-line degasser, a well-plate autosampler, and a thermostatic column compartment. Detection was conducted by a 6220 TOF mass spectrometer (Agilent Technologies, Santa Clara, CA, USA) equipped with an electrospray ionization (ESI) interface. The chromatographic separation was carried out at 10 °C on a Hypercarb® PGC column (100 mm × 2.1 mm I.D., 5 μm). The mobile phase consisted of 25 mM aqueous nonafluoropentanoic acid (A) and ACN (B) with gradient elution: 0–8 min, 0–10% B; 8–16 min, 10–22% B; 16–25 min, 22–50% B; 25–32 min, 50% B, and the re-equilibration time of gradient elution was 10 min. The injected volume was 1 μL. The flow rate was set at 0.25 mL min−1 and directly introduced into the mass spectrometer.
TOFMS analysis was performed using full scan mode with the mass range set at m/z 50–1000 in positive ion mode. The ESI source conditions were as follows: drying gas (N2) flow rate: 10 L min−1; drying gas temperature: 350 °C; nebulizer gas pressure: 40 psi; capillary voltage: 400 V; fragmentor voltage: 110 V; skimmer voltage: 60 V; octopole RF: 250 V. All the data were processed by Agilent MassHunter Software (version B.02.00, Agilent Technologies). G1969-85000 tuning mix (Agilent Technologies) was used for lock mass calibration of our data.
Data processing for untargeted metabolite profiling
The raw data in the instrument specific format (.d) was converted to common data format (.mzdata) files by MassHunter software. The program XCMS (http://metlin.scripps.edu/download/) was applied for peak alignment of the data in the time domain and automatic integration and extraction of the peak intensities. Metabolites that did not exist in 80% of the samples in either group were filtered. The output data were normalized using IS and dry weight of each sample before being exported to multivariate data analysis. Then the output tables were introduced to SIMCA-P software (Version 11, Umetrics) for principal component analysis (PCA) and partial least squares discriminant analysis (PLS-DA). It should be noted that data were log transformed for multivariate analysis to eliminate the unit error. Variables that significantly contributed to the clustering and discrimination were identified according to a threshold of variable importance in the projection (VIP) values (VIP > 1), which could be generated after PLS-DA processing. Independent samples t-test was performed in succession by SPSS 13.0 for Windows (SPSS Inc., Chicago, IL, USA) to reveal the statistical differences for variables between planktonic cell and biofilm samples. P values of less than 0.05 were considered statistically significant.
Identification of metabolites of interest was based on matching the measured accurate mass and isotopic pattern with the database (METLIN/YMDB/KEGG), and was finally confirmed by comparing with the standard compounds. Metabolites without standard compounds were tentatively identified.
Preparation of standard solutions
Amino acid calibration and spike standards were prepared at physiological concentration ranges from commercially available standards. Amino acid mix #1 contained amino acids that are stable in 0.1% hydrochloric acid solution, such as the branched chain amino acids and the hydroxyl group containing amino acids, including Asp, Hyp, Ser, Gly, Thr, Glu, Ala, Pro, Val, Met, Tyr, Ile, Leu, Phe, His, Trp, Arg, and Lys. Amino acid mix #2 contained the amino acids that are unstable in an acid solution, such as Gln, Asn, Cit and Orn. All calibration stocks and working standards were stored at −40 °C until used. In addition to calibration standards, IS mixes (15N2-glutamine, 13C6-lysine, 15N4-arginine) were prepared in 0.1% hydrochloric acid solution and used for quantitative analysis.
Ions monitored for quantification of targeted amino acids
Ions monitored for quantification were extracted using Agilent MassHunter Software (version B.02.00, Agilent Technologies). Signals from both IS and targeted amino acids provided an accurate mass ± 0.01 Da extraction window (Table S1, ESI†).
Calibration curves
Stock solutions containing 22 amino acids were prepared by mixing #1 and #2 together. All amino acids would be within expected physiological concentrations. Most amino acids were calibrated using standard compounds in a 400-fold range from the minimum to the maximum content except that glycine, glutamic acid, valine, citrulline, leucine, isoleucine, phenylalanine, tryptophan were 200-fold. Linear fit was applied to quantify all the amino acids.
Method accuracy and precision
The standard solutions at low, medium and high concentrations were analyzed in a set of three on a single assay day to determine intra-day precision, and analyzed in triplicate on each of three consecutive days for inter-day variation. Accuracy of the method was verified by measuring recovery from intracellular samples spiked with all amino acids.
Construction of C. albicans SPE1 deletion mutants
The entire coding sequence of SPE1 was deleted from C. albicans strain SN152 by two-step homologous recombination using a fusion-PCR-based strategy.37,38 Briefly, using the genomic DNA of SN152 as a template, 5′ and 3′ flanking sequences immediately outside the SPE1 coding region were amplified using the primers listed in Table S2 (ESI†), where primers P1 and P2 were for the upstream fragment, and P3 and P4 were for the downstream fragment. Using P5 and P6 as the PCR primers, the auxotrophic markers LEU2 and ARG4 were amplified from plasmids pSN40 and pSN69, respectively. Fusion PCR strategy was used to combine the upstream and downstream fragments of SPE1 with the auxotrophic markers LEU2 using primer P1 and P4. Thus the knockout PCR cassette spe1Δ::LEU2 was obtained. The spe1Δ::ARG4 cassette was generated using a similar method. The SPE1 null mutant was generated by transforming SN152 with a spe1Δ::LEU2 fragment, followed by a spe1Δ::ARG4 fragment.
Cell surface hydrophobicity assay
The cell surface hydrophobicity (CSH) of C. albicans was measured by the water–hydrocarbon two-phase assay, as described.39 Briefly, the spe1Δ mutant and its wild-type cells were cultured as described above to form biofilms, and cells from the biofilms were removed from the flask surface with a sterile scraper to prepare a cell suspension in YPD medium (optical density at 600 nm of 1.0). A total of 1.2 mL suspension from each group was drawn into a clean glass tube and overlaid with 0.3 mL octane. The mixture was then vortexed for 3 min, and the aqueous phase OD600 was determined soon after the two phases had separated. The OD600 for the group without octane overlay in YPD medium was used as the negative control. Three repeats were performed for each group. The relative hydrophobicity was obtained as [(OD600 of the control − OD600 after octane overlay)/OD600 of the control] × 100.
Results and discussion
Optimization of chromatographic conditions
The main goal of the present work was to identify single LC conditions that enable efficient analysis of as many intracellular metabolites as possible, especially for the analysis of polar amino acids. Mass spectrometric detection was used to determine typical chromatography parameters, such as peak shape, retention time, and signal-to-noise ratio, for numerous different analytes in a single LC run. As shown in Fig. S1A (ESI†), three different column temperatures (10 °C, 20 °C, 30 °C) were evaluated at a moderate concentration of IP buffer of 15 mM NFPA, and the retention factors (k) of the first eight eluted polar amino acids were calculated by injection of the standard compounds. It gives a visualized outcome for amino acid analysis on a PGC support, and decreasing temperature plays an important role in improving separation. The impact of the NFPA concentration (10 mM, 15 mM, 20 mM, 25 mM and 30 mM) in the mobile phase on the retention factors of amino acids was also evaluated at 10 °C (Fig. S1B, ESI†). Higher concentrations of NFPA would make the mobile phase acidic (pH < 2). Owing to the specific properties of PGC that allow a large pH range (1–14) without damage, it was possible to work under such acidic conditions that cannot be realized on classical RP packing materials. The best retention behavior and separation were obtained with 25 mM NFPA. Thus, the final chromatographic conditions were performed as described in the “IP-PGC-TOF/MS analysis” section.
Untargeted profiling of planktonic and biofilm cells
Untargeted metabolic profiling of planktonic and biofilm samples was detected using the optimized IP-PGC-TOF/MS method. The typical chromatograms are shown in Fig. S2 (ESI†). The raw LC-MS data from metabolic profiling were pretreated following the procedure described in “Data processing for untargeted metabolite profiling”. Finally, a total of 1229 features were initially generated by processing the mass spectrometric data using XCMS, and after removing the IS and artifact peaks, 543 features were retained and used for subsequent multivariate analysis. PCA, an unsupervised method, was first performed for the separation of planktonic and biofilm groups (Fig. 2A) (R2 = 0.81). The supervised approach PLS-DA was also adopted, in comparison with the unsupervised approach PCA, similar separation results were observed (Fig. 2B) (R2 = 0.90, Q2 = 0.89). Both the PCA and PLS-DA models indicated that planktonic samples displayed characteristic metabolic features that were significantly different from those of the biofilm samples. To validate the model, permutation tests with 500 iterations were further performed. These permutation tests compared the goodness of fit of the original model with the goodness of fit of randomly permuted models. As shown in Fig. 2C, the validation plot indicates that the original model is valid. The criteria for validity are as follows: all the permuted R2(cum) and Q2(cum) values to the left are lower than the original point to the right, and the blue regression line of the Q2(cum) points has a negative intercept. In addition, metabolites with VIP greater than 1 were considered to have a statistically significant contribution to the model. The corresponding loading plot (Fig. 2D) was used to select potential biomarkers. According to the VIP value (>1), several variables were first selected as candidates for potential biomarkers. After merging the variables from the identical metabolites, fewer variables were collected. Differentially produced (P < 0.05) metabolites were considered significant. According to this algorithm, we found significantly differential variables that were considered differential metabolites in biofilm or planktonic cells during their growth process. A total of 16 potential biomarkers were identified and the results are shown in Fig. 3 and Table 1, which are combined to produce a visual summary of metabolites probably responsible for the phenotypic changes that occur during biofilm development. Isomeric leucine and isoleucine were identified by comparing with the standard compounds.
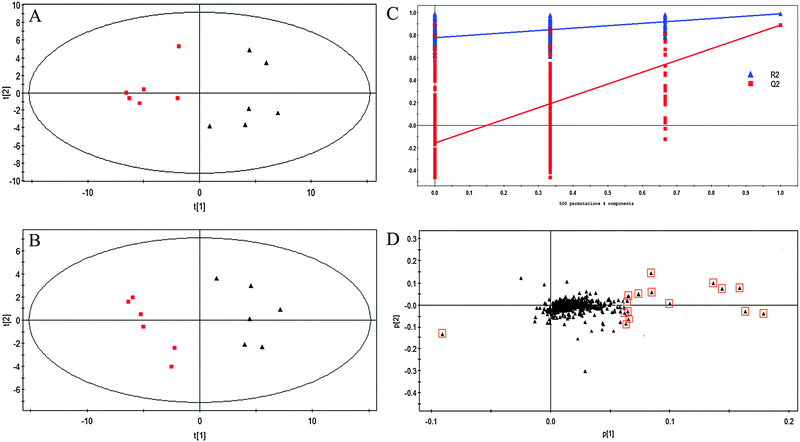 |
| Fig. 2 PCA scores plot (A), PLS-DA scores plot (B) of IP-PGC-TOF/MS data sets from the biofilm (▲) and planktonic ( ) groups. (C) Validation plot obtained from 500 permutation tests. The marked triangles in loading scores plot (D) from PLS-DA model are the 16 ions with VIP values > 1 and may be regarded as potential biomarkers. | |
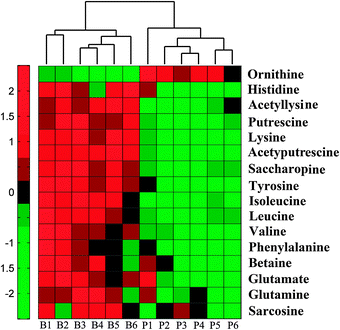 |
| Fig. 3 Colors in metabolite arrays represent x-fold change in the untargeted metabolite levels of biofilm (B) development or planktonic culture (P). Red indicates increased levels of metabolites; green indicates decreased levels of metabolites. The two groups can be well recognized by cluster analysis of 16 potential biomarkers. | |
Table 1 Identification of significantly differential metabolites between biofilm and planktonic cells
No. |
m/z |
t
R (min) |
Compound |
Trendsa |
Fold |
P valueb |
Direction of changes compared with planktonic cells. (↑), up-regulated; (↓), down-regulated.
P values were calculated from the Student's test, which was performed using SPSS software.
|
1 |
118.0858 |
3.28 |
Betaine |
↑ |
1.55 |
1.92 × 10−2 |
2 |
90.0535 |
3.95 |
Sarcosine |
↑ |
1.24 |
2.28 × 10−2 |
3 |
147.0754 |
8.53 |
Glutamine |
↑ |
1.36 |
1.12 × 10−2 |
4 |
133.0959 |
9.72 |
Ornithine |
↓ |
0.46 |
5.02 × 10−3 |
5 |
148.0595 |
9.93 |
Glutamate |
↑ |
1.26 |
8.98 × 10−3 |
6 |
89.1073 |
10.21 |
Putrescine |
↑ |
5.58 |
1.17 × 10−7 |
7 |
118.0848 |
10.43 |
Valine |
↑ |
1.66 |
7.52 × 10−3 |
8 |
131.1156 |
11.35 |
N-Acetylputrescine |
↑ |
4.33 |
2.53 × 10−9 |
9 |
147.1128 |
12.57 |
Lysine |
↑ |
2.08 |
1.35 × 10−8 |
10 |
132.1002 |
13.05 |
Leucine |
↑ |
1.56 |
3.26 × 10−4 |
11 |
132.1003 |
14.01 |
Isoleucine |
↑ |
1.59 |
5.37 × 10−5 |
12 |
189.1208 |
14.70 |
Acetyllysine |
↑ |
2.24 |
7.00 × 10−5 |
13 |
156.0753 |
16.73 |
Histidine |
↑ |
1.29 |
5.93 × 10−3 |
14 |
277.136 |
16.99 |
Saccharopine |
↑ |
2.26 |
8.44 × 10−6 |
15 |
166.0841 |
23.36 |
Phenylalanine |
↑ |
1.94 |
1.60 × 10−2 |
16 |
182.0794 |
24.06 |
Tyrosine |
↑ |
1.76 |
3.09 × 10−5 |
In our previous research,14 nine amino acids (alanine, asparagine, γ-aminobutyrate, glutamate, isoleucine, glycine, sarcosine, serine and threonine) were identified as significant differential metabolites in 24 h cultivated planktonic and biofilm cells, and all of them were up-regulated in biofilm cells. However, although derivatized GC/MS methods for metabolomic studies have been well established and widely used, there still exist many puzzles yet to be uncovered. During derivatization and GC analysis, degradation or rearrangement reactions can occur. For example, arginine and citrulline could be decomposed, while asparagine could be converted to aspartate, and glutamate could partly lose H2O and rearrange forming pyroglutamate, which is also produced from glutamine through loss of NH3.40,41 In this study, all the key amino acids in central carbon metabolism were directly characterized using the IP-PGC-TOF/MS untargeted method, while tricarboxylic acid (TCA) cycle and glycolysis metabolites were at low responses in the spectra as compared with GC-MS. After multivariate statistical analysis, it was interesting to find that most of the identified potential biomarkers were of amino acids or related compounds, suggesting that IP-PGC-TOF/MS is a potentially valuable method for interpreting changes in amino acid metabolites during the process of biofilm formation. Some new potential biomarkers were also found, including N-acetylputrescine, glutamine, histidine, acetyllysine, saccharopine and ornithine, indicating that IP-PGC-TOF/MS has enriched the spectra of amino acid metabolism. However, it remains difficult to derive causal relationships between observations and perturbations due to the imprecision of metabolic profiling for detecting subtle variations in metabolites.13,42 Thus, more accurate targeted analyses of amino acids are needed to characterize the subtle variations in different types of metabolites in both samples and help interpret the pathway of amino acid metabolism in a more comprehensive way.
Targeted profiling of amino acids in planktonic and biofilm cells
Methodology investigation.
When the final chromatographic conditions were optimized and established, all the targeted 22 amino acids eluted within 32 min. Extracted ion chromatograms of the 22 targeted amino acids and 3 IS are shown in Fig. S3 (ESI†). Further experiments on methodology were also investigated, including calibration, limits of detection and quantification, accuracy and precision. Table S3 (ESI†) displays the curve fit used and the correlation coefficient obtained by each amino acid of different concentrations. Linear regression analysis from calibration curves showed that the correlation coefficient R2 was between 0.9972 and 0.9999. The limits of detection (LOD) and quantification (LOQ) were determined at a signal-to-noise ratio (S/N) of 3 and 10, respectively. As shown in Table S3 (ESI†), the LODs varied from 2 to 50 ng mL−1 and the LOQs varied from 6 to 173 ng mL−1. RSDs of the intra-day and inter-day measurements were all less than 3% for the 22 amino acids (Table S4, ESI†). Spike recoveries for all targeted amino acids were within 80–120% (Table S5, ESI†).
Quantitative analysis of the biofilm and planktonic cells.
The developed IP-PGC-TOF/MS method was subsequently applied to simultaneous quantification of the 22 amino acids in six batches of biofilm and planktonic cells. The accurate content and the variation trend of each amino acid are shown in Table 2 and Fig. 4, respectively. As shown in Table 2, the accurate content of the 22 key amino acids in C. albicans varied dramatically from 0.33 to 43.47 μg mg−1. Fig. 4 shows that the absolute content of 19 of the 22 amino acids in the biofilm group was significantly different from that in the planktonic group (P < 0.05, n = 6). Of the 19 amino acids, 12 were characterized only in the targeted quantification mode. Except for ornithine, arginine and praline, most amino acids were at high levels in biofilm cells, and therefore any subtle variation tendency of each targeted amino acid could be evaluated, which helps us to understand the mechanism more profoundly by reconstructing more elaborate metabolic pathways.
Table 2 The absolute content of the 22 amino acids in biofilm and planktonic cells (μg mg−1)
Compound |
Biofilm (μg mg−1) |
RSD% |
Planktonic cells (μg mg−1) |
RSD% |
P valuea |
Sample sizeb |
P values were calculated from the independent Student's t test, which was performed using SPSS software.
Sample size was calculated from the results of independent Student's t test by power analysis module of PASS 12 software.
|
Glycine |
7.860 |
3.17 |
5.370 |
0.75 |
1.42 × 10−6 |
1.00 |
Serine |
25.76 |
4.65 |
19.59 |
2.17 |
3.21 × 10−7 |
1.00 |
Alanine |
20.42 |
4.27 |
19.53 |
5.30 |
1.35 × 10−1 |
0.36 |
Hydroxyproline |
1.150 |
2.54 |
1.110 |
4.65 |
2.40 × 10−1 |
0.42 |
Threonine |
3.420 |
6.85 |
2.620 |
2.04 |
1.03 × 10−5 |
0.99 |
Asparagine |
7.440 |
3.45 |
5.650 |
1.85 |
2.17 × 10−8 |
1.00 |
Proline |
4.250 |
4.41 |
5.010 |
1.64 |
3.61 × 10−6 |
1.00 |
Aspartic acid |
12.99 |
4.13 |
12.41 |
0.35 |
6.45 × 10−2 |
0.70 |
Glutamine |
8.740 |
3.56 |
7.530 |
0.96 |
3.52 × 10−6 |
0.99 |
Ornithine |
2.420 |
5.26 |
4.890 |
1.88 |
3.88 × 10−12 |
1.00 |
Glutamic acid |
18.87 |
1.53 |
14.90 |
2.41 |
1.26 × 10−9 |
1.00 |
Valine |
1.150 |
2.96 |
0.640 |
4.66 |
8.16 × 10−11 |
1.00 |
Citrulline |
2.670 |
5.07 |
1.840 |
4.25 |
1.40 × 10−7 |
1.00 |
Leucine |
8.080 |
1.15 |
5.130 |
3.84 |
1.45 × 10−11 |
1.00 |
Lysine |
43.47 |
3.52 |
20.63 |
2.35 |
9.15 × 10−12 |
1.00 |
Methionine |
0.401 |
4.87 |
0.330 |
4.21 |
2.58 × 10−2 |
1.00 |
Isoleucine |
9.210 |
2.05 |
5.621 |
3.29 |
1.45 × 10−11 |
1.00 |
Histidine |
25.17 |
1.14 |
19.04 |
0.83 |
5.98 × 10−13 |
1.00 |
Arginine |
15.91 |
3.99 |
17.43 |
3.56 |
1.90 × 10−3 |
0.96 |
Phenylalanine |
3.250 |
4.34 |
1.492 |
3.18 |
5.75 × 10−11 |
1.00 |
Tyrosine |
1.940 |
1.51 |
1.043 |
4.12 |
1.15 × 10−12 |
1.00 |
Tryptophan |
0.810 |
3.14 |
0.400 |
4.58 |
2.34 × 10−11 |
1.00 |
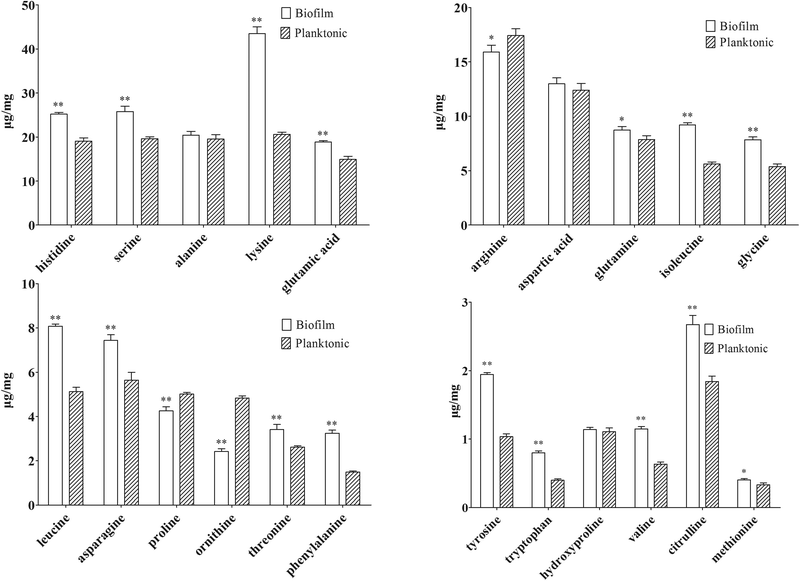 |
| Fig. 4 Absolute content of 22 amino acids in biofilm cells and planktonic cells (n = 6) measured by IP-PGC-TOF/MS. * indicates P < 0.05 and ** indicates P < 0.01 compared with values from planktonic cells. | |
Discussion on the mechanism of biofilm formation by reconstructing metabolic pathways.
Amino acids are not only cell signaling molecules but also regulators of gene expression and cascades of protein phosphorylation. Additionally, amino acids are key precursors for synthesis of low-molecular-weight nitrogenous substances with each having biological importance.43 Combining targeted and untargeted profiling data together, 25 amino acids or related metabolites were selected as potential biomarkers, 16 of which were newly characterized in this study, and the schematic pathway of differential metabolites was re-constructed (Fig. 5). In comparison with previous data, glutamine, citrulline, leucine, lysine, methionine, histidine, saccharopine, acetyllysine, tyrosine and tryptophan were characterized and screened as new potential amino acid biomarkers, and these biomarkers were at high levels in C. albicans biofilms. This result is consistent with the previous microarray data44,45 showing that MET3 and HOM6 (methionine biosynthesis), HIS1 (histidine biosynthesis), ARO8 (aromatic amino acid biosynthesis), LEU1 (3-isopropylmalate dehydratase), TRP4 (tryptophan biosynthesis) and BAT22 (branched chain amino acid aminotransferase) were all overexpressed in intermediate or mature phases of biofilms. Jeniel et al.46 found that genes involved in amino acid biosynthesis were up-regulated in the transcriptional analysis of C. albicans biofilms as compared with planktonic cells. In addition, reduced respiration47 and less reactive oxygen species activity48 were observed in biofilm cells, indicating inhibition of the TCA cycle (also confirmed in our previous study14). Thus, the increased amino acids in biofilms implied a reduced consumption of amino acids due to the inhibited TCA cycle activity. What's more, the extracellular matrix (ECM) increased during the biofilm formation process when cells adhered to the surface, the increased intracellular pool of amino acids that prepare the biofilm for the large increase in biomass is, in part, due to the accumulation of large amounts of carbohydrate-rich EMC.45
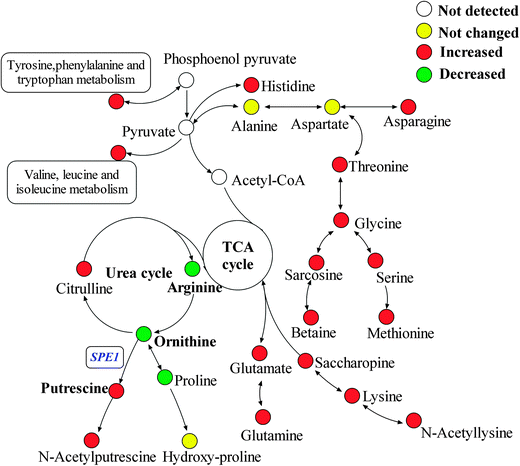 |
| Fig. 5 Schematic overview of differences in metabolites changes between biofilm and planktonic cells. Metabolites in red (higher in biofilm cells) and green (higher in planktonic cells) represent those differential metabolites selected by IP-PGC-TOF/MS targeted and untargeted profiling. | |
Several amino acid-related metabolites were identified by untargeted profiling, including betaine, putrescine, N-acetylputrescine, acetyllysine and saccharopine. Saccharopine and acetyllysine are both lysine-related metabolites. Saccharopine is an intermediate of the amino adipic acid pathway of lysine biosynthesis, and acetyllysine is a degradation product of lysine. Quantitative analysis showed that lysine increased by 2.11 fold in biofilm cells (shown in Table 2). Along with up-regulation of saccharopin and acetyllysine in biofilm cells, activation of the lysine biosynthesis/degradation pathway was observed. Previous research showed that Gcn4p, a general regulator of amino acid biosynthesis, was required for normal biofilm formation,45 and lysine biosynthesis was highly regulated by Gcn4p in yeast.49
N-Acetylputrescine is produced from putrescine catalyzed by the diamine acetyltransferase, while putrescine is produced from ornithine initiated by ornithine decarboxylase (ODC). Ornithine is the only amino acid that was first found to be down-regulated in biofilm cells by both targeted and untargeted profiling approaches using IP-PGC-TOF/MS. Targeted quantitative analysis showed that arginine and proline that directly related to ornithine were slightly down-regulated. Thus, a metabolite transformation trend that the up-regulation of putrescine results in a waning down-regulation of the corresponding precursors was observed in our research. If it proves to be true, an induced ODC activity should be observed and the important role of the ornithine-putrescine pathway during biofilm formation should be further understood.
The absence of ODC and putrescine affects biofilm formation.
Martinez et al.50 reported that the dimorphic transition of C. albicans could be inhibited by ODC inhibitors, which to some extent suggest the significance of the ODC activity during biofilm formation. In this study, an ODC-negative (spe1Δ mutant) was constructed and used for further investigation of its impact on biofilm development. We first quantified the levels of ornithine and putrescine in biofilm and planktonic cells cultured by the spe1Δ mutant and its wild-type. As shown in Fig. 6A, similar to C. albicans SC5314, the level of ornithine was lower in wild-type C. albicans biofilms than in planktonic cells (2.61 μg mg−1vs. 4.73 μg mg−1). It was found to be accumulated in the spe1Δ mutant biofilms as compared with planktonic cells (5.68 μg mg−1vs. 2.17 μg mg−1). On the other hand, putrescine was significantly up-regulated in wild-type biofilms 4.91-fold (0.58 to 6.85 μg mg−1). In spe1Δ mutant, putrescine only raised by 1.76-fold (0.25 to 0.44 μg mg−1) and the total content was far less than that in its wild-type. These results indicate that ODC function was vital for ornithine consumption and putrescine biosynthesis, especially during biofilm formation. Electron microscope images in Fig. 6B show the biofilms cultured by wild-type and spe1Δ mutant at 24 h. In the biofilm formed by the wild-type strain, long tubular hyphae and high cell density were clearly displayed, while biofilm formation was significantly inhibited in the spe1Δ mutant, which was mainly composed of pseudohyphae and yeast cells. When 0.2 mM putrescine was added to the medium to compensate for the deletion, the biofilm appearance of spe1Δ mutant was apparently converted to its wild-type. It is therefore confirmed that the consumption of ornithine for putrescine biosynthesis contributes to biofilm formation in C. albicans.
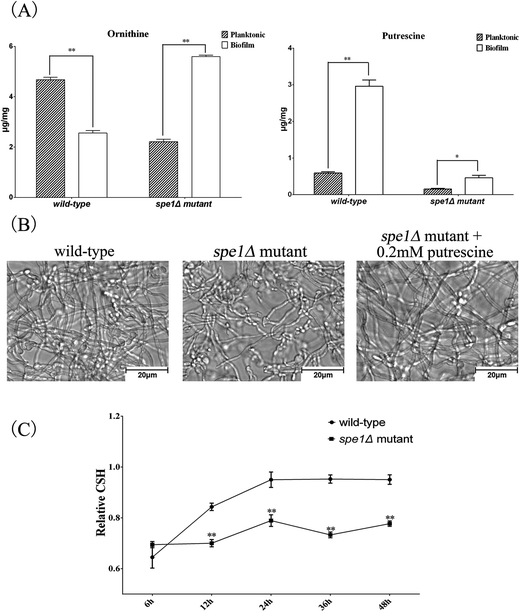 |
| Fig. 6 (A) The content of ornithine and putrescine in wild-type and spe1Δ null mutant cells measured by IP-PGC-TOF/MS. * indicates P < 0.05 and ** indicates P < 0.01 compared with values from planktonic cells. (B) Lack of ODC and putrescine affects biofilm formation. Electron microscope image of a biofilm formed by the wild-type, spe1Δ null mutant cells and spe1Δ null mutant + 0.2 mM putrescine respectively. (C) The cell surface hydrophobicity (CSH) of the biofilms was measured by the water–hydrocarbon two-phase assay. All results are mean ± SD of the three independent experiments. ** indicates P < 0.01 compared with values from wild-type cells. | |
To examine the mechanism by which SPE1 inhibited biofilm formation, CSH of biofilm was also measured by the biphasic separation method. CSH is considered an important factor in the adhesion of C. albicans due to its contribution to the interaction between the cell surface and the flask surface.39,51 A positive correlation between biofilm formation abilities and CSH has been found.52 Here we determined the CSH of biofilms of spe1Δ mutant and its wild-type. As shown in Fig. 6C, there was a positive correlation between the cultivated time and CSH of biofilms for wild-type C. albicans, while CSH of the spe1Δ mutant always remained at a relatively low level. All these results indicate that deletion of the SPE1 gene (or ODC) has a significant impact on C. albicans biofilm formation. Our result is consistent with a previous report53 on microarray analysis of C. albicans biofilms, in which the SPE1 gene was overexpressed in biofilms compared with the planktonic mode. Knowing that polyamines compensate for anionic charges in fungal structures, and that the SPE1 gene (or ODC) plays a pivotal role in cell wall organization and can be considered a potential drug target for disturbing C. albicans biofilm growth, it is worthwhile to further examine the effects of existing anti-fungal drugs on SPE1 or ODC, and new ODC inhibitors can be designed for target clinical applications.
Conclusion
An IP-PGC-TOF/MS system has been developed for targeted and untargeted profiling of metabolites in biofilm and planktonic modes of C. albicans. The inherent property of the PGC column makes it suitable for analysis of polar compounds along with volatile ion-pair agent NFPA. Chromatographic conditions were carefully optimized. Through untargeted profiling analysis, 16 differential metabolite markers were identified, based on which a quantitative analysis method of 22 amino acids was established and evaluated. The robustness, repeatability and sensitivity of the method have all been tested, with satisfactory results. A total of 25 metabolites with significant differences (P < 0.05) were selected as potential biomarkers, among which 16 were characterized and screened as biofilm related biomarkers for the first time. A schematic overview of metabolite changes between biofilm and planktonic cells was reconstructed according to the targeted and untargeted profiling data, whereby subtle changes during biofilm formation could be clearly characterized. In addition to inhibition of the TCA cycle and preparation of biomass accumulation, most amino acids and related metabolites were accumulated in the biofilm formation process, except for ornithine, proline and arginine. Meanwhile, polyamine related metabolites were also found to be at high levels in biofilm cells, indicating activation of ODC, which was tested by the spe1Δ null mutant, confirming that the consumption of ornithine for putrescine biosynthesis and SPE1 overexpression contribute to biofilm formation in C. albicans. Consequently, the combination of targeted and untargeted metabolic profiling analyses provides new insights to the evaluation of the mechanism underlying phenotypic changes that occur during C. albicans biofilm development. Our results indicate that ornithine related substances play important roles in biofilm formation and the potential drug target SPE1 (or ODC) deserves further study.
Acknowledgements
This work was supported by the National Natural Science Foundation of China (NSFC) [No. 81273474 and 81271798].
References
- M. G. Netea, G. D. Brown, B. J. Kullberg and N. A. Gow, An integrated model of the recognition of Candida albicans by the innate immune system, Nat. Rev. Microbiol., 2008, 6, 67–78 CrossRef CAS PubMed.
- J. Chandra, P. K. Mukherjee, S. D. Leidich, F. F. Faddoul, L. L. Hoyer, L. J. Douglas and M. A. Ghannoum, Antifungal resistance of candidal biofilms formed on denture acrylic in vitro, J. Dent. Res., 2001, 80, 903–908 CrossRef CAS PubMed.
- J. W. Costerton, P. S. Stewart and E. P. Greenberg, Bacterial biofilms: a common cause of persistent infections, Science, 1999, 284, 1318–1322 CrossRef CAS.
- C. J. Barelle, C. L. Priest, D. M. Maccallum, N. A. Gow, F. C. Odds and A. J. Brown, Niche-specific regulation of central metabolic pathways in a fungal pathogen, Cell. Microbiol., 2006, 8, 961–971 CrossRef CAS PubMed.
- M. Kamthan, G. Mukhopadhyay, N. Chakraborty, S. Chakraborty and A. Datta, Quantitative proteomics and metabolomics approaches to demonstrate N-acetyl-D-glucosamine inducible amino acid deprivation response as morphological switch in Candida albicans, Fungal Genet. Biol., 2012, 49, 369–378 CrossRef CAS PubMed.
- T. L. Han, R. D. Cannon and S. G. Villas-Boas, The metabolic response of Candida albicans to farnesol under hyphae-inducing conditions, FEMS Yeast Res., 2012, 12, 879–889 CrossRef CAS PubMed.
- J. K. Nicholson, J. C. Lindon and E. Holmes, ‘Metabonomics’: understanding the metabolic responses of living systems to pathophysiological stimuli via multivariate statistical analysis of biological NMR spectroscopic data, Xenobiotica, 1999, 29, 1181–1189 CrossRef CAS PubMed.
- W. B. Dunn, D. Broadhurst, P. Begley, E. Zelena, S. Francis-McIntyre, N. Anderson, M. Brown, J. D. Knowles, A. Halsall, J. N. Haselden, A. W. Nicholls, I. D. Wilson, D. B. Kell and R. Goodacre, Procedures for large-scale metabolic profiling of serum and plasma using gas chromatography and liquid chromatography coupled to mass spectrometry, Nat. Protocols, 2011, 6, 1060–1083 CAS.
- M. M. Koek, R. H. Jellema, J. van der Greef, A. C. Tas and T. Hankemeier, Quantitative metabolomics based on gas chromatography mass spectrometry: status and perspectives, Metabolomics, 2011, 7, 307–328 CrossRef CAS PubMed.
- K. G. Zulak, A. M. Weljie, H. J. Vogel and P. J. Facchini, Quantitative 1H NMR metabolomics reveals extensive metabolic reprogramming of primary and secondary metabolism in elicitor-treated opium poppy cell cultures, BMC Plant Biol., 2008, 8, 5 CrossRef PubMed.
- I. R. Lanza, S. Zhang, L. E. Ward, H. Karakelides, D. Raftery and K. S. Nair, Quantitative metabolomics by H-NMR and LC-MS/MS confirms altered metabolic pathways in diabetes, PLoS One, 2010, 5, e10538 Search PubMed.
- A. Kummel, S. Panke and M. Heinemann, Putative regulatory sites unraveled by network-embedded thermodynamic analysis of metabolome data, Mol. Syst. Biol., 2006, 2, 2006.0034 CrossRef PubMed.
- J. M. Buscher, D. Czernik, J. C. Ewald, U. Sauer and N. Zamboni, Cross-platform comparison of methods for quantitative metabolomics of primary metabolism, Anal. Chem., 2009, 81, 2135–2143 CrossRef CAS PubMed.
- Z. Zhu, H. Wang, Q. Shang, Y. Jiang, Y. Cao and Y. Chai, Time Course Analysis of Candida albicans Metabolites during Biofilm Development, J. Proteome Res., 2013, 6, 2375–2385 CrossRef PubMed.
- K. F. Smart, R. B. Aggio, J. R. Van Houtte and S. G. Villas-Boas, Analytical platform for metabolome analysis of microbial cells using methyl chloroformate derivatization followed by gas chromatography-mass spectrometry, Nat. Protocols, 2010, 5, 1709–1729 CAS.
- H. Li, M. L. Ma, S. Luo, R. M. Zhang, P. Han and W. Hu, Metabolic
responses to ethanol in Saccharomyces cerevisiae using a gas chromatography tandem mass spectrometry-based metabolomics approach, Int. J. Biochem. Cell Biol., 2012, 44, 1087–1096 CrossRef CAS PubMed.
- L. V. Roze, A. Chanda, M. Laivenieks, R. M. Beaudry, K. A. Artymovich, A. V. Koptina, D. W. Awad, D. Valeeva, A. D. Jones and J. E. Linz, Volatile profiling reveals intracellular metabolic changes in Aspergillus parasiticus: veA regulates branched chain amino acid and ethanol metabolism, BMC Biochem., 2010, 11, 33 CrossRef PubMed.
- Y. Liu, T. Chen, Y. Qiu, Y. Cheng, Y. Cao, A. Zhao and W. Jia, An ultrasonication-assisted extraction and derivatization protocol for GC/TOFMS-based metabolite profiling, Anal. Bioanal. Chem., 2011, 400, 1405–1417 CrossRef CAS PubMed.
- T. Langrock, P. Czihal and R. Hoffmann, Amino acid analysis by hydrophilic interaction chromatography coupled on-line to electrospray ionization mass spectrometry, Amino Acids, 2006, 30, 291–297 CrossRef CAS PubMed.
- M. Kato and A. Takatsu, Amino acid analysis by hydrophilic interaction chromatography coupled with isotope dilution mass spectrometry, Methods Mol. Biol., 2012, 828, 55–62 CAS.
- M. Armstrong, K. Jonscher and N. A. Reisdorph, Analysis of 25 underivatized amino acids in human plasma using ion-pairing reversed-phase liquid chromatography/time-of-flight mass spectrometry, Rapid Commun. Mass Spectrom., 2007, 21, 2717–2726 CrossRef CAS PubMed.
- B. Thiele, K. Fullner, N. Stein, M. Oldiges, A. J. Kuhn and D. Hofmann, Analysis of amino acids without derivatization in barley extracts by LC-MS-MS, Anal. Bioanal. Chem., 2008, 391, 2663–2672 CrossRef CAS PubMed.
- R. M. Seifar, C. Ras, J. C. van Dam, W. M. van Gulik, J. J. Heijnen and W. A. van Winden, Simultaneous quantification of free nucleotides in complex biological samples using ion pair reversed phase liquid chromatography isotope dilution tandem mass spectrometry, Anal. Biochem., 2009, 388, 213–219 CrossRef CAS PubMed.
- P. Kiefer, N. Delmotte and J. A. Vorholt, Nanoscale ion-pair reversed-phase HPLC-MS for sensitive metabolome analysis, Anal. Chem., 2011, 83, 850–855 CrossRef CAS PubMed.
- J. Stahlberg, Retention models for ions in chromatography, J. Chromatogr., A, 1999, 855, 3–55 CrossRef CAS.
- P. Chaimbault, K. Petritis, C. Elfakir and M. Dreux, Determination of 20 underivatized proteinic amino acids by ion-pairing chromatography and pneumatically assisted electrospray mass spectrometry, J. Chromatogr., A, 1999, 855, 191–202 CrossRef CAS.
- M. Piraud, C. Vianey-Saban, K. Petritis, C. Elfakir, J. P. Steghens and D. Bouchu, Ion-pairing reversed-phase liquid chromatography/electrospray ionization mass spectrometric analysis of 76 underivatized amino acids of biological interest: a new tool for the diagnosis of inherited disorders of amino acid metabolism, Rapid Commun. Mass Spectrom., 2005, 19, 1587–1602 CrossRef CAS PubMed.
- Y. Q. Xia, M. Jemal, N. Zheng and X. Shen, Utility of porous graphitic carbon stationary phase in quantitative liquid chromatography/tandem mass spectrometry bioanalysis: quantitation of diastereomers in plasma, Rapid Commun. Mass Spectrom., 2006, 20, 1831–1837 CrossRef CAS PubMed.
- J. Wang, T. Lin, J. Lai, Z. Cai and M. S. Yang, Analysis of adenosine phosphates in HepG-2 cell by a HPLC-ESI-MS system with porous graphitic carbon as stationary phase, J. Chromatogr., B: Anal. Technol. Biomed. Life Sci., 2009, 877, 2019–2024 CrossRef CAS PubMed.
- E. Forgacs and T. Cserhati, Dependence of the retention of some barbituric acid derivatives on a porous graphitized carbon column on their physicochemical parameters, J. Pharm. Biomed. Anal., 1992, 10, 861–865 CrossRef CAS.
- B. Rhourri-Frih, P. Chaimbault, D. Dequeral, P. Andre and M. Lafosse, Investigation of porous graphitic carbon for triterpenoids and natural resinous materials analysis by high performance liquid chromatography hyphenated to mass spectrometry, J. Chromatogr., A, 2012, 1240, 140–146 CrossRef CAS PubMed.
- C. West, C. Elfakir and M. Lafosse, Porous graphitic carbon: a versatile stationary phase for liquid chromatography, J. Chromatogr., A, 2010, 1217, 3201–3216 CrossRef CAS PubMed.
- P. Chaimbault, K. Petritis, C. Elfakir and M. Dreux, Ion-pair chromatography on a porous graphitic carbon stationary phase for the analysis of twenty underivatized protein amino acids, J. Chromatogr., A, 2000, 870, 245–254 CrossRef CAS.
- K. Petritis, P. Chaimbault, C. Elfakir and M. Dreux, Parameter optimization for the analysis of underivatized protein amino acids by liquid chromatography and ionspray tandem mass spectrometry, J. Chromatogr., A, 2000, 896, 253–263 CrossRef CAS.
- X. Chen, Z. Lou, H. Zhang, G. Tan, Z. Liu, W. Li, Z. Zhu and Y. Chai, Identification of multiple components in Guanxinning injection using hydrophilic interaction liquid chromatography/time-of-flight mass spectrometry and reversed-phase liquid chromatography/time-of-flight mass spectrometry, Rapid Commun. Mass Spectrom., 2011, 25, 1661–1674 CrossRef CAS PubMed.
- S. U. Bajad, W. Lu, E. H. Kimball, J. Yuan, C. Peterson and J. D. Rabinowitz, Separation and quantitation of water soluble cellular metabolites by hydrophilic interaction chromatography-tandem mass spectrometry, J. Chromatogr., A, 2006, 1125, 76–88 CrossRef CAS PubMed.
- S. M. Noble and A. D. Johnson, Strains and strategies for large-scale gene deletion studies of the diploid human fungal pathogen Candida albicans, Eukaryotic Cell, 2005, 4, 298–309 CrossRef CAS PubMed.
- L. Yan, P. Cote, X. X. Li, Y. Y. Jiang and M. Whiteway, PalI domain proteins of Saccharomyces cerevisiae and Candida albicans, Microbiol. Res., 2012, 167, 422–432 CrossRef CAS PubMed.
- Y. Cao, B. Dai, Y. Wang, S. Huang, Y. Xu, Y. Cao, P. Gao, Z. Zhu and Y. Jiang, In vitro activity of baicalein against Candida albicans biofilms, Int. J. Antimicrob. Agents, 2008, 32, 73–77 CrossRef CAS PubMed.
- H. Kaspar, K. Dettmer, Q. Chan, S. Daniels, S. Nimkar, M. L. Daviglus, J. Stamler, P. Elliott and P. J. Oefner, Urinary Amino Acid Analysis: A Comparison of iTRAQ-LC-MS/MS, GC-MS, and Amino Acid Analyzer, J. Chromatogr., B: Anal. Technol. Biomed. Life Sci., 2009, 877, 1838–1846 CrossRef CAS PubMed.
- M. F. Almstetter, P. J. Oefner and K. Dettmer, Metabolic Fingerprinting Using Comprehensive Two-Dimensional Gas Chromatography – Time-of-Flight Mass Spectrometry, Methods Mol. Biol., 2012, 815, 399–411 CAS.
- F. Xu, L. Zou and C. N. Ong, Multiorigination of Chromatographic Peaks in Derivatized GC/MS Metabolomics: A Confounder That Influences Metabolic Pathway Interpretation, J. Proteome Res., 2009, 8, 5657–5665 CrossRef CAS PubMed.
- G. Wu, Amino acids: metabolism, functions, and nutrition, Amino Acids, 2009, 37, 1–17 CrossRef CAS PubMed.
- J. E. Nett, A. J. Lepak, K. Marchillo and D. R. Andes, Time course global gene expression analysis of an in vivo Candida biofilm, J. Infect. Dis., 2009, 200, 307–313 CrossRef CAS PubMed.
- K. M. Yeater, J. Chandra, G. Cheng, P. K. Mukherjee, X. Zhao, S. L. Rodriguez-Zas, K. E. Kwast, M. A. Ghannoum and L. L. Hoyer, Temporal analysis of Candida albicans gene expression during biofilm development, Microbiology, 2007, 153, 2373–2385 CrossRef CAS PubMed.
- J. E. Nett, A. J. Lepak, K. Marchillo and D. R. Andes, Time Course Global Gene Expression Analysis of an In Vivo CandidaBiofilm, J. Infect. Dis., 2009, 200, 307–313 CrossRef CAS PubMed.
- J. Bonhomme, M. Chauvel, S. Goyard, P. Roux, T. Rossignol and C. d'Enfert, Contribution of the glycolytic flux and hypoxia adaptation to efficient biofilm formation by Candida albicans, Mol. Microbiol., 2011, 80, 995–1013 CrossRef CAS PubMed.
- C. J. Seneviratne, Y. Wang, L. Jin, Y. Abiko and L. P. Samaranayake,
Candida albicans biofilm formation is associated with increased anti-oxidative capacities, Proteomics, 2008, 8, 2936–2947 CrossRef CAS PubMed.
- S. Busch, H. B. Bode, A. A. Brakhage and G. H. Braus, Impact of the cross-pathway control on the regulation of lysine and penicillin biosynthesis in Aspergillus nidulans, Curr. Genet., 2002, 42, 209–219 Search PubMed.
- J. P. Martinez, J. L. Lopez-Ribot, M. L. Gil, R. Sentandreu and J. Ruiz-Herrera, Inhibition of the dimorphic transition of Candida albicans by the ornithine decarboxylase inhibitor 1,4-diaminobutanone: alterations in the glycoprotein composition of the cell wall, J. Gen. Microbiol., 1990, 136, 1937–1943 CrossRef CAS PubMed.
- Y. H. Samaranayake, P. C. Wu, L. P. Samaranayake and M. So, Relationship between the cell surface hydrophobicity and adherence of Candida krusei and Candida albicans to epithelial and denture acrylic surfaces, APMIS, 1995, 103, 707–713 CrossRef CAS.
- X. Li, Z. Yan and J. Xu, Quantitative variation of biofilms among strains in natural populations of Candida albicans, Microbiology, 2003, 149, 353–362 CrossRef CAS PubMed.
- S. Garcia-Sanchez, S. Aubert, I. Iraqui, G. Janbon, J. M. Ghigo and C. d'Enfert,
Candida albicans Biofilms: a Developmental State Associated with Specific and Stable Gene Expression Patterns, Eukaryotic Cell, 2004, 3, 536–545 CrossRef CAS.
Footnotes |
† Electronic supplementary information (ESI) available. See DOI: 10.1039/c3mb70240e |
‡ Xiaofei Chen and Haitang Wu contributed equally to this work. |
|
This journal is © The Royal Society of Chemistry 2014 |
Click here to see how this site uses Cookies. View our privacy policy here.