Automated vehicles and electrification of transport
Received
16th July 2014
, Accepted 11th November 2014
First published on 11th November 2014
Abstract
Global greenhouse gas (GHG) emission targets can only be met by significantly decarbonising road transport. The only long term way to do this is via the electrification of powertrains combined with the production of low carbon electricity or hydrogen. Current assumptions and models, such as the IEA BLUE Map, demonstrate that this is technically possible, but assume growth in demand for transport services will only double by 2035 and triple by 2050, largely driven by growth in developing economies. However, another transport revolution, automated vehicles, could drive growth in transport services significantly further, which without electrification will have a large negative impact on efforts to curb transport related emissions. In contrast, it is shown in this paper that automated vehicles could significantly improve the economics of electric vehicles, and therefore make the electrification of powertrains more likely, which could help reduce emissions. Despite this uncertainty, little work has been done on understanding how these factors will affect each other, particularly the timing and uptake of automated vehicles and their effect on future transport related GHG emissions and economics, yet the impact on transport policy, infrastructure and society will be profound and should be of interest to policy makers, the automotive and energy industries, and society as a whole.
Broader context
Improving vehicle efficiency and alternatively fuelled vehicles are essential in order to reduce global greenhouse gas emissions targets from transport. However, efforts to do this risk being undermined by increasing demand for transport services. Automated vehicles and electrification of powertrains are two major revolutions in automotive technology that have begun already and will happen over the next few decades. Automated vehicles could significantly increase demand for transport services, making reducing emissions harder. However, this paper demonstrates analytically that automated vehicles, if they increase average vehicle utilisation, could significantly change the economics of alternatively fuelled vehicles, particularly battery electric vehicles, making them by far the cheapest option in the medium to long term. This could therefore make reducing emissions from transport easier. Battery electric vehicles, by being cheaper, could also increase the total availability of transport services and make the benefits of automated vehicles available to a larger percentage of the global population. This paper highlights that little work has been done on considering how these two transport revolutions will affect each other, despite the fact they are going to be occurring at the same time.
|
Introduction
Our vehicles are currently powered by burning fossil fuels in internal combustion engines, which makes a significant contribution towards both local air pollution and GHG emissions.1 An over-reliance on a single energy source also puts our transport systems at significant risk from future oil shocks and declines in the production of conventional fuels.2 International efforts to meet all three of these major challenges have identified that the electrification of powertrains is necessary in the long term (30–40 years) if we are to clean the air in our cities, prevent the worst excesses of climate change and diversify and secure our future energy supplies.3 Electrification in this context can take multiple forms, and does not necessarily mean solely battery electric vehicles, but that the majority of the vehicle power comes originally from electricity,4 and hence could also be plug-in-hybrids or fuel cell vehicles. At the same time it is predicted by the International Energy Agency (IEA), in their business-as-usual scenario, that the passenger vehicle fleet will need to double to 1.7 billion in 2035 and triple by 2050 in order to sustain even modest global economic growth.3 More accurately this should be considered that demand for transport services will increase, which the IEA then assumes means more vehicles. How this increase in demand for transport services is going to be delivered at the same time as managing global GHG emissions to minimize climate change is not clear.
Electrification is necessary as even if the average efficiency of combustion engine is doubled, and the oil industry's most optimistic assumptions of oil supply in 2035 are met,2 it will not be possible to meet the demand projected by the IEA with incumbent technologies. Therefore a lack of mature alternatives could in the long run result in significantly less growth in the availability of transport services than predicted by the IEA. This would result in many negative consequences. In all these reports, models and assumptions,5 automated vehicles do not appear to have been considered. For example the 2007 IPCC report on mitigation6 mentioned many measures that can reduce demand for travel and/or car usage and improve driving style in addition to new technologies that can all reduce GHG emissions. Although they state that their effectiveness may be counteracted by growth in the sector, automated vehicles are not mentioned.
Automated vehicles will change our world
Automated vehicles will be a technology revolution that will affect every facet of our lives. Although many previous transport technologies have revolutionized the way we travel, such as the invention of the railway, aviation, mass production, containerization, roads and the motorways, as yet nothing has been able to break the link between needing one driver hour for every vehicle hour except in very controlled environments or applications. This link has given rise to a phenomenon known as constant average travel time, which is that the average travel time per person has remained constant at about an hour a day for at least the past 30 years over, and the number of trips has remained roughly constant at one thousand trips per year, although distance has increased by 50% because we can travel faster.7 Travel time appears to be mostly independent of all other indicators, with the world average commuting time being around 40 minutes whether you are in Africa, Europe or America.8 This is because everyone has an equal number of hours in each day, and travel has a cost in terms of competing with other activities for time, such that the marginal value of travel declines as total travel time increases.7 If it is assumed that reducing the negative cost of travel; by making it possible to use that time to undertake other activities simultaneously, will significantly increasing the percentage of our time that we are prepared to spend travelling. Then an unintended consequence of automated vehicles is that they are likely to significantly increase demand for transport services.9 This could make the IEA predictions of increases in demand for transport services a significant under-estimate. However, automated vehicles also have the potential to decrease the energy consumption per kilometre due to more efficient driving and reducing congestion, but by making travel cheaper and increasing the number of vehicles we can fit on our roads, will further increase demand. Therefore how automated vehicles could affect the assumptions and calculations of future GHG emissions is highly complicated. However, on the upside, breaking the link between a unit of human effort and a unit of economic activity by a significant amount, what defines a technology revolution,10 could trigger a technology revolution and revitalize our economies.
Automated driving systems can be classified according to the degree of automation versus human control according to Society of Automotive Engineers (SAE) standard J3016,11 as summarised in Table 1, with the term autonomous vehicles being either level 4 high automation or level 5 full automation. Although full automation has numerous challenges to overcome and might be a long way off the transition has already begun, with the emergence of multiple advanced driver assistance, partial and conditional automation systems which are becoming standard,12 many of which are stepping stones towards high or full automation as described in Table 1.
Table 1 Summary of the SAE J3016 standards for automated driving system
SAE level & name |
SAE narrative definition |
0 – No automation |
The full-time performance by the human driver of all aspects of the dynamic driving task, even when enhanced by warning or intervention systems |
1 – Driver assisted |
The driving mode-specific execution by a driver assistance system of either steering or acceleration/deceleration using information about the driving environment and with the expectation that the human driver perform all remaining aspects of the dynamic driving task |
2 – Partial automation |
The driving mode-specific execution by one or more driver assistance systems of both steering and acceleration/deceleration using information about the driving environment and with the expectation that the human driver performs all remaining aspects of the dynamic driving task |
3 – Conditional automation |
The driving mode-specific performance by an automated driving system of all aspects of the dynamic driving task with the expectation that the human driver will respond appropriately to a request to intervene |
4 – High automation |
The driving mode-specific performance by an automated driving system of all aspects of the dynamic driving task, even if a human driver does not respond appropriately to a request to intervene |
5 – Full automation |
The full-time performance by an automated driving system of all aspects of the dynamic driving task under all roadway and environmental conditions that can be managed by a human driver |
However, there is a risk that public perception of initiatives like the ‘google self-driving car’ oversimplify the challenges both technical,13 control14 and legal15 that remain ahead in order to introduce high or full automation. Industry predictions for high or full automation range from the near-future of 2020 to a more cautious second half of the twenty-first century, and so although driving is likely to become more automated and drivers are likely to become accustomed to automated driving in some environments, for example on flowing motorways, it is hard to predict how long it will take before all journeys can be driven autonomously.16 The DARPA Grand Challenge and Urban Challenge competitions led the way in translating research on automated driving into real world conditions.17 Since then, many projects have made significant progress in recent years, for example the AdaptIVe project, a consortium of 29 partners, and its predecessor, interactIVe, developing technologies for partial and highly automated driving on motorways, urban scenarios, and close-distance manoeuvres,18,19 or the V-charge project which focussed on developed an electric automated car outfitted with close-to-market sensors.13
The effect of automated vehicles on the electrification of transport
In previous work a number of influential reports were reviewed and the results confirmed that predict that there will be no significant difference between conventional and alternative powertrain costs by 2035.4 However, all these studies aggregate behaviour into a single market average, and assume current behavioral patterns, vehicle ownership models, and vehicle utilisation rates, will continue with no change. None of these assumptions are necessarily true. Limited data has shown that innovative ownership & business models like car clubs can increase vehicle utilisation20 and spread out the fixed costs of vehicle ownership.21 Therefore if it is assumed that automated vehicles will have a similar, if not greater, effect,9 then automated vehicles will substantially change the economics of alternative powertrains. The effect of increasing vehicle utilisation rates will improve the economics of low running cost vehicles more than others, and changing ownership models will improve the economics of high capital cost vehicles more than others, both of which are characteristics of BEVs.
Furthermore, if automated vehicles are used in fleets of vehicles operating as point-to-point on-demand car clubs, then this mode of operation would significantly improve the battery utilisation in BEVs, further improving their economics. The most well-known disadvantage of BEVs is range anxiety which forces vehicle manufacturers to design BEVs with heavy and costly battery packs capable of driving a hundred kilometres or more, despite the fact that on average most people only drive 30–50 kilometres per day.22 This has a serious detrimental impact on battery utilisation and hence BEV economics. A hybrid fleet with vehicles with different ranges and battery recharging requirements has recently been modeled, and supported the hypothesis that hybrid and BEVs with different ranges can be used in routing problems successfully.23 This would enable the full range of the BEVs in a fleet to be maximised and potentially used multiple times a day, significantly increasing the battery utilisation, whilst long distances journeys (>160 km) could still be met by a small number of vehicles powered by chemical fuels, as these typically represent only 12% of all vehicle kilometres undertaken.22
How significant this effect could be is shown in Fig. 1, which plots the battery utilisation for different vehicles based upon average vehicle usage data extracted from the UK National Travel Survey, as described in previous work.22 All assumptions and the cost model used are described in detail in previous work.4
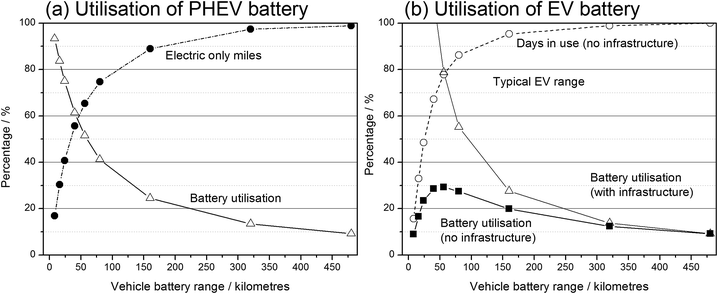 |
| Fig. 1 (a) Battery utilisation as a function of vehicle range for a plug-in-hybrid (PHEV) (Δ) also showing electric only range (●), and (b) for an electric vehicle (EV) with (Δ) and without (■) infrastructure (i.e. fast charging) also showing days in use when without infrastructure (○). | |
For a plug-in-hybrid (PHEV) there is an optimum battery size around 5–15 kW h depending on vehicle size, with an electric only range of 24–56 km, giving a battery utilisation between 50–70% per day. For an electric vehicle (EV) with a typical range of 160 km, battery utilisation for an average vehicle user will be around 20% without infrastructure, i.e. assuming the vehicle owner can only charge the vehicle overnight at home and will only drive if they have sufficient range. With charging infrastructure, then battery utilisation will increase to around 28%, but still significantly below a PHEV. Smaller battery packs could in theory have very high utilisation, but would require multiple and regular charging throughout the day, especially for long journeys, impractical for most drivers, however this could happen in the case of roadbed inductive charging.24Table 2 shows some typical commercially available vehicles, their battery size and typical electric only range. It should be noted that actual electric range can vary considerably when taking into account real world behaviour, traffic congestion and road type.25
Table 2 Typical electric and plug-in-hybrid electric vehicle battery size and electric only range
Type |
Model |
Nominal battery size |
Typical electric only range |
PHEV18 |
Toyota prius plug-in hybrid |
4.4 kWh |
10 km (EPA)26 |
PHEV32 |
Ford fusion energi |
7.6 kWh |
30 km (EPA)26 |
PHEV40 |
Mitsubishi outlander PHEV |
12 kWh |
52 km (Mitsubishi)27 |
PHEV56 |
2015 Chevrolet volt |
17 kWh |
61 km (EPA)26 |
BEV120 |
2013 Nissan LEAF |
24 kWh |
134 km (EPA)26 |
BEV333 |
Tesla model S |
60 kWh |
333 km (Tesla)28 |
Fig. 2 shows how larger battery packs increase the running cost per kilometre considerably. The cost over 5 years (100% depreciation of powertrain cost and fuel cost only) for ‘low’ yearly mileage of 13
216 km is compared to a vehicle utilisation rate increased by 5 times to a ‘high’ yearly mileage of 66
064 km. This is indicative only, as how much automated vehicles could increase vehicle utilisation rates has not been studied. Energy costs of 28.5 $ GJ−1 for petrol (4.5 $ Gallon−1) and 35.9 $ GJ−1 for electricity, and fuel consumption of 1.7 MJ km−1 (58 mpg) for an ICE, 1.34 MJ km−1 (73 mpg) (petrol mode) and 0.79 MJ km−1 (125 mpg) (electric mode) for a PHEV, and 0.6 MJ km−1 (165 mpg) for a BEV, were assumed.4 Over 5 years for a BEV with a 160 km range and ‘low’ mileage the cost would be 17.3 cents km−1 compared to the cheapest option, the ICE, at 10.8 cents km−1. However, if a ‘high’ mileage is used, the battery utilisation for the BEV is increased from 23% to 113% and the cost per kilometre is now the cheapest at 5.2 cents km−1 compared to the next cheapest option, the PHEV with a 24 km battery range costing 5.6 cents km−1. If the vehicle lasts longer than 5 years, the ongoing running cost per kilometre for the BEV is only 2.1 cents km−1 compared to 3.4 cents km−1 for the PHEV. This clearly demonstrates that changing vehicle utilisation rates changes the economics of alternative powertrains unequally.
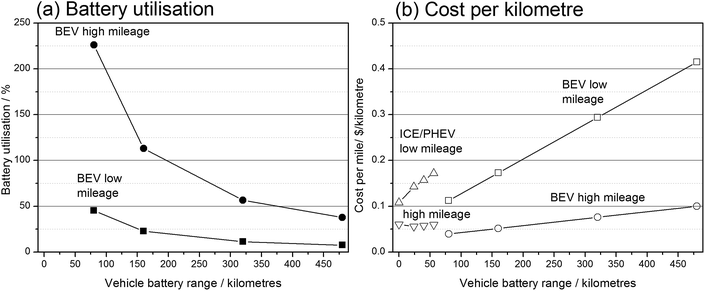 |
| Fig. 2 (a) Battery utilisation for low (■) and high mileage (●), and (b) cost per km as a function of vehicle battery range assuming a single overnight charge for ICE/PHEV with low (Δ) and high mileage (∇) and cost per km for a BEV with unlimited charging for low (□) and high mileage (○). | |
Conclusions
Changing vehicle utilisation rates has been demonstrated to change the economics of alternative powertrains unequally. On a cost per kilometre basis, increasing vehicle utilisation rates spreads out the fixed costs of the powertrain and increases the battery utilisation rate which significantly improves the economics of BEVs and to a lesser extent PHEVs, but in both cases significantly more than ICE vehicles. If it is assumed that automated vehicles will substantially increase vehicle utilisation, then automated vehicles could make BEVs the winner in the long term. This could have a large positive impact on efforts to curb global GHG emissions. However, automated vehicles by mitigating the negative cost of travel, by making it possible to use that time to undertake other activities simultaneously, and by increasing vehicle densities on roads and mitigating congestion, could significantly increase demand for transport services making current predictions of future transport demand unrealistic. This could have a large negative impact on efforts to curb global GHG emissions. However, despite this uncertainty, there has been little work done to date on understanding potential transition pathways that include automated vehicles and electrification together, even though it is clear they are both going to happen at the same time. The potential effect of automated vehicles on behavioral patterns particularly vehicle ownership and vehicle utilisation rates, as well as overall demand for transport services, is in urgent need of study. Combined with electrification automated vehicles could make it easier to reduce global GHG emissions, make our transport systems more energy secure, improve safety, solve local air quality problems, solve congestion and meet future growth in mobility services. Or they could make it worse. We can't afford to leave it to chance.
Acknowledgements
The author G.J. Offer would like to thank the EPSRC for funding his EPSRC career acceleration fellowship, award number EP/I00422X/1. The author would also like to thank the anonymous peer reviewers for their advice and assistance making the paper better.
Notes and references
- D. a. Howey, Policy: A challenging future for cars, Nat. Clim. Change, 2011, 2, 28–29 CrossRef.
- S. Sorrell, R. Miller, R. Bentley and J. Speirs, Oil futures: A comparison of global supply forecasts, Energy Policy, 2010, 38, 4990–5003 CrossRef PubMed.
-
F. Birol, World energy outlook 2012, 2012 Search PubMed.
- M. Contestabile, G. J. Offer, R. Slade, F. Jaeger and M. Thoennes, Battery electric vehicles, hydrogen fuel cells and biofuels. Which will be the winner?, Energy Environ. Sci., 2011, 4, 3754 Search PubMed.
- M. Tran, D. Banister, J. D. K. Bishop and M. D. McCulloch, Realizing the electric-vehicle revolution, Nat. Clim. Change, 2012, 2, 328–333 CrossRef.
-
B. Metz, O. Davidson, P. Bosch, R. Dave and L. Meyer, Climate change 2007, Mitigation of climate change, 2007, at http://www.ipcc.ch/pdf/assessment-report/ar4/wg3/ar4_wg3_full_report.pdf Search PubMed.
-
D. Metz, Travel Time Constraints in Transport Policy, 2003 Search PubMed.
- (Sheffield), S. G. & Newman, M. Commuting Time. Bridg. Mag, 2006 (2006), at http://www.worldmapper.org.
-
S. Le Vine and J. Polak, Automated Cars: A smooth ride ahead?, 2014 Search PubMed.
- C. Perez, Technological revolutions and techno-economic paradigms, Cambridge J. Econ, 2009, 34, 185–202 CrossRef PubMed.
- J3016: Taxonomy and Definitions for Terms Related to On-Road Motor Vehicle Automated Driving Systems - SAE International, 2014, at http://standards.sae.org/j3016_201401/.
- J. Piao and M. McDonald, Advanced Driver Assistance Systems from Autonomous to Cooperative Approach, Transplant. Rev., 2008, 28, 659–684 CrossRef.
-
P. Furgaley
et al., Toward automated driving in cities using close-to-market sensors: An overview of the V-Charge Project, in Intelligent Vehicles Symposium, ( 2013), at http://ieeexplore.ieee.org/xpls/abs_all.jsp?arnumber=6629566 Search PubMed.
-
B. Vanholme, D. Gruyer, B. Lusetti and S. Glaser, Highly Automated Driving on Highways Based on Legal Safety, 14, pp. 333–347, ( 2013) Search PubMed.
- B. W. Smith, Automated Vehicles are Probably Legal in the United States, SSRN Electron. J., 2012, 1–112 CAS.
-
G. Yeomans, Autonomous vehicles Handing over control: Opportunities and Risks for Insurance. ( 2014). at http://www.lloyds.com/%7E/media/lloyds/reports/emergingriskreports/autonomousvehiclesfinal.pdf.
-
G. Seetharaman, A. Lakhotia and E. P. Blasch, Unmanned vehicles come of age: The darpa grand challenge, Computer, Long. Beach. Calif, 39, pp. 26–29 2006 Search PubMed.
-
M. Miglietta, G. Burzio and A. Saroldi, Continuous Driver Assistance: Technology Leadership Brief, 2012, DOI:10.4271/2012-01-9014.
-
F. Fahrenkrog and A. Zlocki, interactIVe - accident avoidance by active intervention for Intelligent Vehicles, in 22. Aachner Kolloquium conference proceedings, 2013 Search PubMed.
- C. Morency, M. Trépanier and B. Martin, Object-Oriented Analysis of Carsharing System, Transp. Res. Rec., 2008, 2063, 105–112 CrossRef PubMed.
-
M. Duncan, The cost saving potential of carsharing in a US context, Transportation (Amst), 38, pp. 363–382, 2010 Search PubMed.
- G. J. Offer, M. Contestabile, D. a. Howey, R. Clague and N. P. Brandon, Techno-economic and behavioural analysis of battery electric, hydrogen fuel cell and hybrid vehicles in a future sustainable road transport system in the UK, Energy Policy, 2011, 39, 1939–1950 CrossRef CAS PubMed.
- A. Juan, J. Goentzel and T. Bektaş, Routing fleets with multiple driving ranges: Is it possible to use greener fleet configurations?, Appl. Soft Comput., 2014, 21, 84–94 CrossRef PubMed.
- M. Yilmaz and P. T. Krein, Review of Battery Charger Topologies, Charging Power Levels, and Infrastructure for Plug-In Electric and Hybrid Vehicles, IEEE Trans. Power Electron, 2013, 28, 2151–2169 CrossRef.
- R. Shankar and J. Marco, Method for estimating the energy consumption of electric
vehicles and plug-in hybrid electric vehicles under real-world driving conditions, IET Intell. Transp. Syst., 2013, 7, 138–150 CrossRef PubMed.
-
http://www.fueleconomy.gov, 2014, at http://www.fueleconomy.gov/feg/findacar.shtml.
- Mitsubishi Outlander P. H. E. V., 2014. at http://www.mitsubishi-cars.co.uk/outlander/specifications.aspx?trim=PHEVGX4hs.
- Tesla Model S. (2014). at http://www.teslamotors.com/models/design.
|
This journal is © The Royal Society of Chemistry 2015 |