DOI:
10.1039/C3LC50487E
(Paper)
Lab Chip, 2013,
13, 3965-3975
A bioengineered heterotypic stroma–cancer microenvironment model to study pancreatic ductal adenocarcinoma†
Received
17th April 2013
, Accepted 30th July 2013
First published on 31st July 2013
Abstract
Interactions between neoplastic epithelial cells and components of a reactive stroma in pancreatic ductal adenocarcinoma (PDAC) are of key significance behind the disease's dismal prognosis. Despite extensive published research in the importance of stroma–cancer interactions in other cancers and experimental evidence supporting the importance of the microenvironment in PDAC progression, a reproducible three-dimensional (3D) in vitro model for exploring stroma–cancer interplay and evaluating therapeutics in a physiologically relevant context has been lacking. We introduce a humanized microfluidic model of the PDAC microenvironment incorporating multicellularity, extracellular matrix (ECM) components, and a spatially defined 3D microarchitecture. Pancreatic stellate cells (PSCs) isolated from clinically-evaluated human tissue specimens were co-cultured with pancreatic ductal adenocarcinoma cells as an accessible 3D construct that maintained important tissue features and disease behavior. Multiphoton excitation (MPE) and Second Harmonic Generation (SHG) imaging techniques were utilized to image the intrinsic signal of stromal collagen in human pancreatic tissues and live cell–collagen interactions within the optically-accessible microfluidic tissue model. We further evaluated the dose–response of the model with the anticancer agent paclitaxel. This bioengineered model of the PDAC stroma–cancer microenvironment provides a complementary platform to elucidate the complex stroma–cancer interrelationship and to evaluate the efficacy of potential therapeutics in a humanized system that closely recapitulates key PDAC microenvironment characteristics.
Introduction
Pancreatic ductal adenocarcinoma (PDAC) is the fourth most common cause of cancer death in the United States. The disease originates in the ductal epithelium and rapidly evolves from precursor lesions to fully invasive cancer in a manner difficult to detect. Once advanced, PDAC presents itself as one of the most dismal human cancers. It is one of the few human malignancies with nearly 100% mortality with a median survival time of less than 6 months and a 5-year survival rate of less than 4%.1 This is a result of the inherently aggressive disease biology and a pronounced resistance to conventional therapeutic regimens.2–4 Although a number of genetic and environmental factors have been implicated in PDAC carcinogenesis, exact causes and pathological mechanisms remain incompletely understood.
The tumor microenvironment facilitates aggressive carcinogenesis in many soft tissue cancers and now with the evidence of the interplay it has with cancer cells itself, is beginning to be perceived as a heterogeneous organ-like system.5 Recently, considerable attention has focused on stromal components of PDAC tumors, which envelopes the solid nest of cancer cells. The PDAC stroma is composed mainly of deregulated extracellular matrix (ECM) proteins, endothelial cells, inflammatory cells, fibroblasts, and pancreatic stellate cells (PSCs).5 Among all cancer types, PDAC exhibits the most dense desmoplastic stromal reaction and can account for up to 90% of the total tumor volume.1 The regulation of this key histological hallmark is largely attributed to the chronic and sustained activation of PSCs. PSCs exist in the periacinar space of normal pancreas in a quiescent state consisting of 4% of the total cell population and function to store vitamin A; however, during PDAC they undergo activation resulting in pathological consequences.6,7 They exhibit a highly proliferative myofibroblast-like (αSMA expressing) phenotype in terms of excessive contraction and aberrant deposition of ECM components such as collagen type I (ColI). Collectively, interactions among PSCs, cancer cells, ECM elements, and other microenvironment components have been shown to influence tumorigenesis, angiogenesis, therapeutic resistance, and metastasis in PDAC.8–17 Notably, there is growing evidence that the stroma, and stromal ECM reorganization in particular, plays an active role in cancer progression and invasion.18–20 Additionally, it is believed the fibrotic stroma induces high interstitial fluid pressures and microvasculature collapse, which together create a physical barrier against the systemic delivery of therapeutics.21,22 Due to the demonstrated importance of the stroma in PDAC progression, a new perspective coined the “stromal depletion hypothesis” has emerged which involves targeting both cellular and acellular stroma components to disrupt stroma–cancer pro-survival interactions.22–30
Despite the important role of the stroma in carcinogenesis, experimental models that accurately recreate the complex heterogeneity of the human PDAC microenvironment are lacking. As a result, opportunities to investigate and therapeutically target the most clinically relevant pathological mechanisms underlying the disease are missed. PDAC animal models that seek to recreate stroma–cancer interactions do exist.1,22,31 However, these animal models have significant limitations including cost, long latency, unpredictable characteristics, the inability to perform rapid experimental manipulations, and difficulty in correlating observed results with responses in human. Although no in vitro model is able to reproduce all aspects of in vivo physiology, such models do offer the potential for direct observation and systematic testing of hypotheses before advancing to preclinical studies. Traditionally, PDAC research has been performed in vitro using two-dimensional (2D) human cell culture systems.32–35 Although these systems are well referenced and have established protocols, there has been research based motivation to develop three-dimensional (3D) in vitro models. A number of studies have suggested that cells cultured on flat 2D substrates differ significantly in terms of morphology, behavior, cell–cell, and cell–matrix interactions compared to more realistic 3D environments.36–42 Additionally, 3D models have been shown to provide more in vivo-like responses to therapeutic agents compared to 2D cultures.43–45 In order to capture the advantages of three-dimensionality, intact PDAC tissue has been cultured ex vivo.46–48 Although advantageous in that the tissue retains its original 3D heterogeneous architecture, these models can be limited by tissue sourcing, low viability, and lack of experimental manipulation. An alternative model is the multicellular tumor spheroid, which contains a 3D aggregation of cancer cells that resemble solid tumors in terms of nutrient, oxygenation, and viability gradients.43,44,49,50 Spheroids typically though only consist of a homogenous cell type population and lack a controlled microarchitecture and significant ECM component. Another approach is to isolate pathophysiologically-relevant cell types and implant them into a reconstituted 3D gel of ECM-derived material (i.e. collagen, Matrigel) to form an organotypic culture.51,52 These models are advantageous in that the ECM composition and cell types can be tailored to the disease under investigation; however, they are traditionally limited in their ability to spatially pattern tumor components as found in vivo and perform live, dynamic imaging of the intact culture.
Microfluidic technology has enabled the development of promising in vitro platforms that address the challenges of reconstituting, integrating, and interrogating many variables of the tumor microenvironment.53,54 In addition to retaining the benefits of the aforementioned 3D models, microfluidic technology holds a number of additional advantages that makes it amendable to oncology research.55,56 For example, microfluidic platforms inherently have dimensions comparable to cells that allow for defined 3D cell patterning and construction of cell–cell interfaces by exploiting laminar flow. Additionally, optically accessible microfluidic devices enable in situ imaging of living cultures with high spatiotemporal resolution. For example, powerful nonlinear optical imaging techniques such as multiphoton excitation (MPE)57 and Second Harmonic Generation (SHG) imaging58 can be effectively employed. MPE can be used to excite cellular fluorescence and perform optical sectioning deep into culture and tumor constructs. Multiphoton scanning is also uniquely suited to image 3D collagen matrices since collagen fibers readily generate intrinsic SHG signals that can be discriminated without the need for labeling. When used simultaneously, MPE and SHG imaging enables fluorescent live cells to be imaged noninvasively in intact 3D environments to provide valuable insight into cell–ECM interplay. Microfluidic models have been developed to examine dynamic stroma–cancer interactions in a variety of cancer types.59–64 However despite similar evidence of the stromal role in PDAC, an effective microfluidic model of PDAC has not been generated to investigate the role of cell–ECM interactions during pathogenesis and to test therapeutics in a biologically-relevant setting.
Here we report on the new development of a microfluidic model that incorporates the complexities of clinically-derived multicellularity and a relevant 3D ECM in a spatially controlled architecture to more closely recapitulate stroma–cancer interactions in the human PDAC microenvironment. Seeking to bridge the PDAC translational gap between traditional 2D in vitro models and animal models, we support the models utility by validating its components with clinically-diagnosed human tissues, demonstrating the ability to observe live cell–ECM interplay in real-time, and evaluating therapeutic response in a microenvironment context.
Materials and methods
Microfluidic device fabrication
Microfluidic devices were fabricated using polydimethylsiloxane (PDMS) soft lithography. An in-house photomask was designed using Adobe Illustrator (Adobe Systems Incorporated, San Jose, CA) and printed on a transparency using a 6000 dpi high-resolution printer (Imagesetter Incorporated, Madison, WI). SU8-100 photoresist (Microchem, Newton, MA) was spun, exposed, and developed to generate a negative master mold with 250 μm feature heights on a 3-in silicon wafer. Topographically, the pattern consists of three 750 μm wide inlet channels that converge to a single 2.25 mm wide central channel. Base PDMS and crosslinking agents (Sylgard 184 Silicon Elastomer Kit, Dow Corning, Midland, MI) were thoroughly mixed at a weight ratio of 10
:
1, degassed for 1 h under vacuum at room temperature, poured onto the master mold, and cured for at least 2 h at 60 °C. Upon removal from the master mold, access ports were formed using a 2 mm diameter circular biopsy punch. The PDMS was then corona-treated for 20 s (BD-20, Electro-Technic Products, Chicago, IL), irreversibly bonded to acid-washed glass slides or #1.5 coverglass (for MPE and SHG imaging), and autoclaved for 35 min.
Human cell culture
Human primary PSCs were isolated from clinically diagnosed pancreatic specimens after obtaining informed consent from patients with PDAC undergoing primary surgical resection at the University of Wisconsin Carbone Cancer Center (UWCCC). Tissue was obtained under a University of Wisconsin IRB human subjects exemption status (#2011-0587) for de-identified residual tissue not required for patient diagnosis by pathology. PSCs were isolated using an established outgrowth method from fresh tissue deemed fibrotic by gross examination at the time of surgery.65 All fresh tissue specimens used for PSC isolations were further confirmed by histological analysis. Following each isolation, PSC phenotype and purity was determined via morphological visualization and immunohistochemistry for vimentin (anti-vimentin, clone V9, DAKO) and alpha smooth muscle actin (αSMA) (anti-human αSMA, clone 1A4, DAKO). For immunostaining, PSCs were pelleted, supported in 2% agarose (UltraPure Agarose 1000, Invitrogen, Carlsbad, CA), fixed with 10% neutral buffered formalin, paraffin-embedded, and sectioned at 5 μm. In total, PSCs were isolated from four patients clinically diagnosed with resectable PDAC tumors (Table S1, ESI†). PSCs were maintained in complete media of DMEM/Ham's F-12 (1
:
1) (ATCC, Manassas, VA) supplemented with 10% fetal bovine serum (FBS, Hyclone) and antibiotic-antimycotic solution (100 IU penicillin, 100 μg mL−1 streptomycin, 25 ng mL−1 amphotericin B) (Cellgro). Only PSC populations between passage 2 and 6 were used for subsequent experiments to minimize uncertain variables. Commercially-available neonatal human dermal fibroblasts (NHDF, Lonza, Basel, Switzerland) and PANC-1 pancreatic ductal adenocarcinoma cells (ATCC) were routinely maintained in complete media and used for a maximum of 15 passages. All cells were cultured 37 °C in an incubator containing 5% CO2 and either passaged or used once 80% confluency was met in a 75 cm2 culture flask.
Acid-solubilized ColI (rat tail, high concentration, BD Biosciences) was neutralized with an equal amount of 100 mM HEPES buffer in 2× PBS and complete media. Sodium hyaluronate (HA, 150 kDa, Lifecore Biomedical, Chaska, MN) powder was dissolved in serum-free DMEM:F12 media. Six ECM mimics were formulated by maintaining a constant HA concentration of 2 mg mL−1, varying the ColI concentration (2–4 mg mL−1), and either including or omitting a pre-incubation step for 1 h at 4 °C before microfluidic loading (Table S2, ESI†). For cell-based studies, cells were detached from culture flasks by incubating with 0.05% trypsin–EDTA (1×) (Gibco). Cells were then diluted in complete culture media, counted, centrifuged at 1000 rpm for 5 min, and resuspended at a concentration of 5 × 106 cells mL−1. Cells and culture media were added to achieve final ColI and HA concentrations. The final concentration of PANC-1 cells was fixed at 1 × 106 cells mL−1. PSCs and NHDFs were used at either 1.25 × 105 or 2.5 × 105 cell/mL. In experiments where cell types needed to be fluorescently distinguished, cells were labeled with 5 μM CellTracker Green CMFDA (Invitrogen, Carlsbad, CA) according to manufacturer instructions before trypsinizing for resuspension in ColI/HA precursor solution.
Microfluidic device operation
Three microfluidic devices were loaded without cells for each of the six ECM conditions. A 15 μL ECM solution droplet was placed on each inlet. Due to PDMS hydrophobicity, the droplets remained positioned on the inlets without spontaneous flow into the microfluidic channel. Suction was applied by removing 18 μL of air from the outlet port using a manual pipette, which drew the three ECM solution droplets into the microfluidic channel simultaneously. Devices were then transferred to a 37 °C incubator for 15 min to induce ECM polymerization. Media was introduced via capillary forces by first adding a 30 μL droplet to the outlet. 10-μL media droplets were then added simultaneously to the flanking inlets using a multichannel pipette. The cultures were allowed to equilibrate for 24 h at 37 °C in humidified bioassay dishes (to circumvent detrimental effects of evaporation) before MPE and SHG imaging. For trilayer co-culture establishment, cell–ECM solutions were gently mixed via manual pipetting to obtain a uniform cell density. A 15 μL droplet of PANC-1-ECM solution was always placed on the middle inlet port. A 15 μL droplet of either NHDF-ECM or PSC-ECM solution was placed on the flanking inlet ports. Loading and ECM polymerization was accomplished in the same manner as the cell-free situations. After 24 h of culture, media was changed every day by removing existing droplets and simultaneously adding 10 μL droplets to the flanking inlet ports using a multichannel pipette.
Imaging the PDAC microenvironment
Trilayer patterning was visualized immediately after loading by imaging CellTracker Green CMFDA-labeled PSCs and unlabeled PANC-1 cells on an inverted epifluorescent microscope (Nikon Eclipse TE300) using a SPOT imaging system (Diagnostic Instruments, Inc., Sterling Heights, MI). To quantify culture contraction, a 4× brightfield image of the central culture channel was acquired at 24, 48, and 72 h after loading. The percent area of the channel occupied by cells-ECM was measured at each timepoint using the polygon manual drawing tool in ImageJ. The ability to introduce solution through the void space via capillary forces was also visualized. 24 h after culture establishment a 30 μL media droplet was added to the outlet and two 10 μL droplets of media containing 500 kDa FITC-conjugated dextran were simultaneously added to the flanking inlet ports. A series of fluorescent images was acquired at the central culture channel for 30 min to track the fluid flow. Using ImageJ, the average fluorescent intensity of each pixel column was determined and plotted as a function of channel width.
MPE and SHG imaging was performed using a custom built multiphoton optical workstation assembled around a Nikon Eclipse TE300 (Nikon, Melville, NY) at the LOCI (Laboratory for Optical and Computational Instrumentation, University of Wisconsin) imaging research facility. A Ti:sapphire laser (890 nm; Spectra-Physics-Millennium/Tsunami) was focused onto samples using either a Nikon S Fluor 20× air-immersion lens (NA = 0.75) or Nikon CFI Apo Lambda S LWD 40× water-immersion lens (NA = 1.15). A 445–20 nm narrow-band pass filter (Thin Field Imaging Technologies, Greenfield MA) was used to isolate the backscattered SHG signal. All MPE and SHG data was captured using WiscScan (http://loci.wisc.edu/software/wiscscan), a laser scanning software package developed at LOCI.
To image collagen in human pancreatic tissue, three normal and three PDAC H&E slides (5 μm cryosections) were made from tissue of consented patients (Table S3, ESI†). For each slide, intact ducts were kindly identified and marked by Dr Agnes Loeffler (Department of Pathology and Laboratory Medicine, University of Wisconsin). Three images were acquired for each duct: a brightfield image (by attaching a Canon Vixia HF S20 digital camera to the microscope eyepiece), an unfiltered MPE image, and a filtered SHG image. Brightfield images were used to verify that the data was captured in a region containing either normal or PDAC ducts. The unfiltered MPE images were used to identify the epithelial cells forming the ducts. The stroma–epithelial boundaries were manually drawn and then applied to the corresponding SHG images for subsequent signal analysis using ctFIRE version 1.1 and CurveAlign version 2.2, which are both programs developed by LOCI (http://loci.wisc.edu/software/ct-fire, http://loci.wisc.edu/software/curvelet-based-alignment-analysis). ctFIRE was used to extract individual collagen fibers from the SHG data after thresholding signal background. CurveAlign was then used to angle orientations relative to the duct boundaries.
For each ECM condition loaded into the microfluidic devices, three SHG z-stacks (50 μm step size) were acquired along the length of the central culture channel at 2, 4, and 6 mm from the point where the inlet channels converge. The SHG stacks were then z-projected using a maximum intensity approach. When microfluidic cultures were imaged to characterize live cell–collagen interactions, the excitation source remained tuned to 890 nm and a 520–35 nm filter was used to discriminate MPE from SHG.
Paclitaxel dose–response assays
The efficacy of the common cancer therapeutic paclitaxel (LC Laboratories, Woburn, MA) was determined in a conventional 2D microplate set-up. PSCs (2000 cells per well) and PANC-1 cells (4000 cells per well) were plated in 96-well plates and allowed to adhere for 24 h. Paclitaxel was dissolved in DMSO, added to fresh media, and administered to the wells at 0.1, 1, 10, 100, and 1000 nM. The final concentration of DMSO was 0.1% v/v. After 48 h of incubation, metabolic activity was measured using a CellTiter-Blue assay (Promega, Madison, WI) with a FLUOstar Omega plate reader (BMG Labtech, Offenburg, Germany). The half maximal inhibitory drug concentration (IC50) was determined using a 3-parameter fit (GraphPad Prism, Graphpad Software, La Jolla, CA).
Paclitaxel response assays were also performed with the microfluidic model. Trilayer cultures with an ECM composition of 2 mg mL−1 ColI and 2 mg mL−1 HA, a PSC density of 2.5 × 105 cells mL−1, and a PANC-1 density of 1 × 106 cells mL−1 were allowed to contract for 24 h after loading. Existing media droplets were removed and replaced with 1, 10, and 100 nM paclitaxel. A 0.1% DMSO vehicle control was also included. All treatment groups were done in triplicate. After 48 h of incubation, cell viability was determined by washing the cultures once with 1× PBS and incubating with LIVE/DEAD® reagent (2 μM calcein-AM and 4 μM ethidium homodimer-1) (Invitrogen, Carlsbad, CA) for 30 min. Cultures were then washed again with 1× PBS. A “live” and corresponding “dead” fluorescent image was acquired at two regions in the central channel with a FITC-Texas Red multiband filter block (Nikon). All image acquisition settings were kept constant. Images were then imported into ImageJ, thresholded to omit background signal, and processed for total integrated intensity (brightness). The cell-ECM area after paclitaxel treatment was measured as previously described.
Statistical analysis
For microfluidic experiments, data is presented as the mean ± standard deviation for three independent devices for each condition. Differences between groups were evaluated using an unpaired Student's t-test where a value of p < 0.05 was considered to be statistically significant.
Results and discussion
Design and loading of the 3D microfluidic trilayer device
In contrast to traditional oncology research platforms that tend to focus solely on the genetic and biological characteristics of the cancer cells, this work sought to develop and characterize a microfluidic in vitro model of PDAC that consists of stromal elements and cancer cells in a spatially-defined 3D configuration representative of the in vivo microenvironment. To achieve this, our system employs an efficient PDMS microfluidic device consisting of three inlet channels that converge to form a single central culture channel (Fig. 1A). The device is intended to accept three cell–ECM solution droplets at the inlet ports, and the flanking inlet ports are spaced to be compatible with a multichannel pipette. The ability of the user to interface with the device via simple pipetting is advantageous in that it eliminates the cumbersome need for tubing or specialized instruments that may make many microfluidic systems unattractive. Also, it opens the possibility for implementing liquid handling technology, which could increase throughput and parallelization of culture loading and analysis. As air is drawn from the outlet port, the cell–ECM solution droplets flow into the device and pattern as a continuous trilayer over the length of the central culture channel due to laminar flow (Fig. 1B). Exploiting this phenomenon during the loading process allows for the initial compartmentalization of different cell types. By using a reconstituted ECM solution as a viscous cell carrier, the rate of cell settling due to gravity is reduced. Therefore, in situ polymerization of the ECM results in the establishment of a reproducible 3D trilayer co-culture.
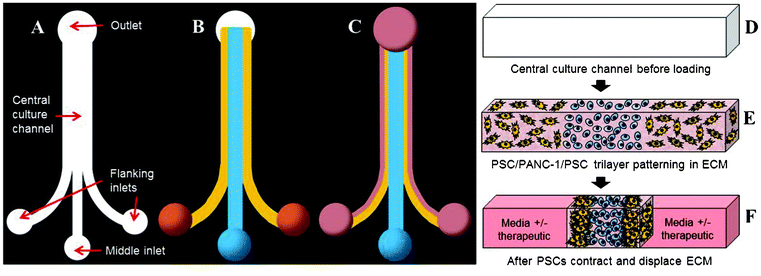 |
| Fig. 1 Design and operation of the microfluidic device. Depicted is the entire topography of an empty device (A), a device after trilayer patterning (B), and a contracted trilayer culture with media (pink) added (C). Three cell-ECM droplets are placed on the inlet ports and simultaneously drawn into the central culture channel from the outlet. Three distinct layers are patterned on the basis of laminar flow and polymerized in situ. Following ECM contraction, space devoid of cells-ECM is created on each side of the trilayer and becomes filled with media to maintain, manipulate, and analyze the culture. This process of culture establishment is also depicted in cross-section (D–F). | |
Sourcing of disease-relevant human cells and tissues
To accurately recapitulate the multicellular feature of the PDAC microenvironment, using pathophysiologically-relevant cell types within the microfluidic device is a must (Fig. 2). We selected the adherent PANC-1 human pancreatic ductal adenocarcinoma cell line that is derived from a poorly differentiated primary PDAC tumor and possesses an activating KRAS mutation and loss of tumor suppressors CDKN2A/p16 and TP53.66 As a representative stromal cell type, primary human PSCs were used based on their central role in sustaining the PDAC microenvironment.7 Early PSC research has been restricted to rat PSCs; however, the dynamic molecular regulation of stroma–cancer interactions is likely to be different between humans and other animal models.11,67 To date, we have isolated and preserved primary cancer-associated PSCs from four different patients clinically diagnosed with PDAC. For each isolation, PSC phenotype was confirmed via light microscopy (loss of retinoid droplets and increase in myofibroblast morphology, Fig. 2A and B) and immunostaining of characteristic vimentin (∼100%) and smooth muscle actin (80–90%) cytoskeletal markers (Fig. S1, ESI†). Since all patients provided informed consent, complete pathology reports make it possible to relate PSC behavior within our model to a clinically-validated, detailed disease state from which the cells were sourced (Table S1, ESI†).
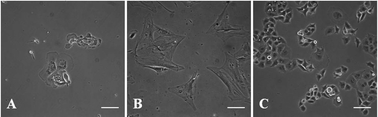 |
| Fig. 2 Morphological characterization of human PSCs and PANC-1 cells. Quiescent PSCs show retinoid droplets (A) that diminish as activated PSCs display a myofibroblast morphology (B). PANC-1 cells display a cobblestone morphology with extensive cell–cell contacts (C). All cells are shown on 2D tissue culture plastic before microfluidic culture. Scale bars represent 100 μm. | |
Disease-relevant ECM composition within the PDAC model
Using a 3D ECM as a part of an in vitro model has been shown to elicit cell behaviors more representative of that observed in vivo. Additionally, the molecular composition, mechanical properties, and structural organization of the ECM surrounding cells have major effects on cell behavior.19 Since each tissue has a characteristic ECM, a focus of our model development was to appropriately select an ECM mimic that best recapitulates that found in human PDAC. In terms of the ECM molecular composition, a hybrid of ColI and HA was chosen to represent the abnormal ECM of the PDAC microenvironment found in vivo. ColI is the most abundant ECM polymer found in vivo and has been widely used for cell culture. In regards to the PDAC microenvironment, extracellular ColI is over-secreted by activated PSCs and a predominant component of the desmoplastic stroma involved in cell proliferation, invasion and metastasis, impeding systemic delivery of therapeutics to tumors, and promoting resistance to gemcitabine treatment.27,45,68–72 Also, collagen organization is significantly altered in tumor tissue relative to normal tissue and has been implicated as a clinical biomarker for diagnosis and staging, underscoring its importance to the tumor microenvironment.73–75 Likewise, the other component of our ECM mimic, HA, is a large glycosaminoglycan known to be up-regulated in the extracellular space of PDAC and thought to impede drug delivery by functioning as an immobile, gel-like phase due to negative charge repulsion and water sequestration.22,76,77 HA can also biochemically interact with CD44-positive cells of the PDAC microenvironment to promote therapeutic resistance.78,79
In addition to molecular composition, the ability to modulate ECM organization within the model is important to investigate the role of ECM within the PDAC microenvironment. In regards to the cancer microenvironment, increasing evidence supports that collagen fibers are highly aligned around tumors and may be implicated in cancer cell migration.20,73,75 By imaging clinically-evaluated human pancreatic tissue sections, our SHG data suggests that distinct differences in collagen organization exist around normal and PDAC ducts. Specifically, collagen fibers appear to uniquely organize into aligned, elongated, linear patterns around PDAC ducts whereas collagen fibers are arranged in a loose, concentric pattern around normal ducts (Fig. 3, Fig. S2 and S3, Movies S1 and S2, ESI†). Although additional characterization is needed to support the existence of tumor-associated collagen signatures in human PDAC, the ability to change ECM characteristics within a cell-based model is absolutely necessary to study the unclear physiological role of collagen organization in PDAC. Using SHG as a means to characterize the 3D ColI component in cell-free ColI/HA ECMs, we show that in vitro polymerization parameters can be modulated to generate ECM mimics of different collagen architectural patterns within our microfluidic model (Fig. 4). Holding HA concentration (2 mg mL−1) constant and maintaining a physiological pH (7.0) of the pre-polymerized ECM formulations, we show that collagen architecture can be influenced by collagen concentration and pre-incubation of the solutions at 4 °C before loading. At a collagen concentration of 2 mg mL−1 with no incubation, we observed linearized collagen fibers distributed throughout the central culture channel (Fig. 4A). When an incubation period is applied, the fibers appear to maintain a linear morphology and increase in length (Fig. 4B). This observation is likely due to a longer nucleation phase promoting molecular assembly and is consistent with previous reports in microsystems.80 Increasing collagen content to 3 mg mL−1 and applying no incubation results in a denser network of linear fibers (Fig. 4C); however, incubation attenuates the ability of linear fibers to form under the same composition (Fig. 4D). A similar inability for linear fibers to form is also observed at a higher collagen concentration of 4 mg mL−1 (Fig. 4E and F). The ability to modulate collagen architecture within the model, demonstrated here, will be necessary to study cellular behaviors in response to specific ECM landscapes. As additional knowledge is gained about collagen organization in human tissue, different model ECMs could be rationally formulated by further altering polymerization parameters (i.e. pH, temperature, salt concentration, flow rate)81 to investigate stroma–cancer microenvironment interactions and cell migration under conditions that better emulate PDAC ECM architecture.
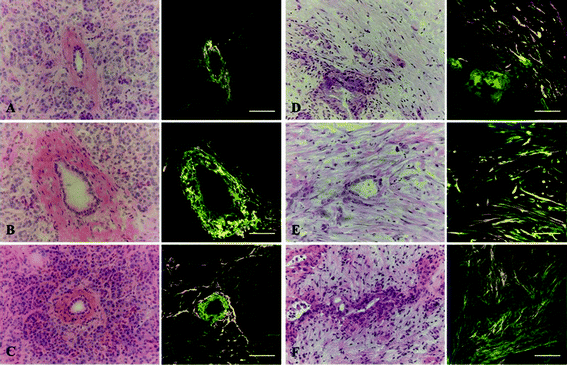 |
| Fig. 3 MPE and SHG imaging of human pancreatic tissues. Three normal ducts (A–C) and three PDAC ducts (D–F) from different individuals were identified. Shown is the H&E image of each duct. Each duct was imaged for eosin fluorescence (green) and SHG signal (orange), which were then merged to depict collagen fibers in relation to epithelial cells comprising the duct. Collagen appears organized in a loose, concentric pattern around normal ducts but becomes linearized and aligned around PDAC ducts. Scale bars represent 100 μm. | |
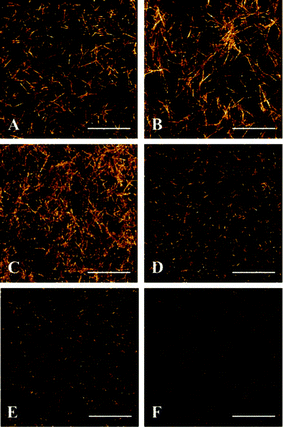 |
| Fig. 4 Collagen architecture modulated by polymerization conditions. Six ECM formulations were loaded into microfluidic channels as follows: (A) 2 mg mL−1 ColI + 2 mg mL−1 HA, no incubation, (B) 2 mg mL−1 ColI + 2 mg mL−1 HA, 1 h incubation at 4 °C before loading, (C) 3 mg mL−1 ColI + 2 mg mL−1 HA, no incubation, (D) 3 mg mL−1 ColI + 2 mg mL−1 HA, 1 h incubation at 4 °C before loading, (E) 4 mg mL−1 ColI + 2 mg mL−1 HA, no incubation, (F) 4 mg mL−1 ColI + 2 mg mL−1 HA, 1 h incubation at 4 °C before loading. Shown are representative z-stack projections of the SHG data obtained within the central culture channel. Scale bars represent 25 μm. | |
Establishment and live cell imaging of the PDAC microenvironment model
The use of microfluidic technology enables PDAC-relevant cell types, embedded in a ColI/HA ECM mimic, to be cultured in a specific 3D architecture for precise and dynamic imaging of microenvironment entities. Due to the simultaneous flow of three solution droplets into the device, compartmentalization of three parallel cell-ECM layers (a central PANC-1 layer, two flanking PSC layers) is achieved solely by laminar flow and without the need for artificial physical barriers to confine the cell-laden ECM layers (Fig. 1, Fig. 5A and B). After patterning and ECM polymerization, activated PSCs form cellular projections, interact with the collagen network, and contract the ECM away from the PDMS sidewalls towards the central PANC-1 layer to create a compressed trilayer culture (Fig. 1, Fig. 5C and D). This approach is uniquely critical in that culture establishment is governed by the intrinsic contractile behavior of activated PSCs (αSMA-positive) and requires minimal exogenous intervention during the process.82,83 This contracted trilayer co-culture mimics the spatial relationship between PDAC tumor cell types in vivo where dense stromal components encompass disseminating cancer cells (Fig. S4, ESI†). Importantly, the juxtaposed trilayer microarchitecture of the culture remains intact after ECM contraction and enables intimate paracrine and juxtacrine signaling mechanisms between PSCs and cancer cells, which are important in maintaining the malignant phenotype.33,34,84 From an analytical perspective, preserved compartmentalization allows for imaging of distinct microregions of the reconstructed microenvironment in a precise and quantitative manner. For example, the ability for PSCs or cancer cells to remodel or migrate though the 3D ECM under different culture conditions could be independently monitored. Even though the initial culture establishment is simple, PSC-mediated contraction and displacement of the ECM away from the PDMS sidewalls creates space devoid of ECM and suspended PSCs on each side of the trilayer culture. This results in a more complex system in which the void space becomes continuously filled with media and can be used to rapidly exchange fluid via capillary forces for in situ media changes or administration of therapeutic agents (Fig. 1, Fig. S5, ESI†). Additionally, any agents added to the culture must first traverse the stromal cell layers before reaching the central cancer cell layer. As observed in vivo, this both spatially models the pathway of interstitial transit following vascular delivery and creates a situation where agents may bind or sequester to stromal components before encountering cancer cells.
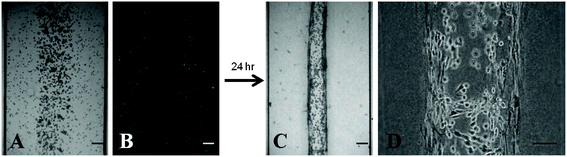 |
| Fig. 5 Establishment of the PDAC microenvironment culture. (A) Trilayer patterning of PSCs and PANC-1 cells suspended in an ECM composed of 2 mg mL−1 ColI + 2 mg mL−1 HA immediately after loading and polymerization within the device. All cells display a rounded morphology. (B) PSCs were dyed with CellTracker Green to demonstrate the ability to spatially pattern separate layers of PSCs and PANC-1 cells without the need for artificial barriers. (C) Contracted trilayer culture 24 h after loading. (D) Two dense PSC layers flank a central PANC-1 layer. Scale bars represent 100 μm. | |
We have routinely shown that PSCs contract the ECM rapidly within the first 24 h following culture loading before reaching a state of tensional homeostasis when using culture parameters of 2 mg mL−1 ColI, 2 mg mL−1 HA, 1 h incubation at 4 °C, 1 × 106 PANC-1 cells mL−1, and 2.5 × 105 PSCs/mL. A concentration of 2 mg mL−1 of ColI was chosen based on previously-used experimental applications and our observations that linear fibers are formed under these conditions comparable to fiber morphology seen in human PDAC tissue.22,80 A HA concentration of 2 mg mL−1 was chosen based on previous observations that its presence can elevate interstitial fluid pressures in a subcutaneous PDAC mouse model.22 We have shown that the rate of ECM contraction is dependent on mesenchymal cell type and loading density (Fig. S6 and S7, ESI†). Also ECM contraction is known to depend on ECM composition and polymerization parameters (data not shown).80,85,86 By using the void space created by ECM displacement for media changes, we have maintained high culture viability beyond 7 days as also observed in other microsystems.64
It has been shown that active ECM synthesis, deposition, and remodeling by cells accompany cancer progression.19,71,87 Traditionally, cell–ECM interplay has been studied at static timepoints by performing standard 2D histopathological imaging techniques on intact tissue and in vitro tissue constructs. Recently, multiphoton microscopy has enabled unprecedented insight into the dynamic cellular processes that occur within the tumor microenvironment.88 MPLSM can be used to simultaneously generate MPE of fluorescent probes and SHG signals from fibrillar collagen deep within intact tissues and constructs. Since MPE and SHG emission signals can be spectrally separated using standard filters, nonlinear imaging can provide noninvasive information regarding the structural reorganization of 3D collagen networks by living cells (Fig. 6). Taking advantage of our optically-accessible model, we have shown that SHG signal increases at the periphery of PSC cytoplasmic protrusions compared to around PANC-1 cells after 24 h (Fig. 6B and 6C). At this timepoint, it is likely that the increase in SHG signal is a result of increased local ColI density following PSC contraction of the ECM network. With extended culture time, we would also expect additional fibrillar collagen deposition and rearrangement to contribute to the SHG signal. Additionally, we observed that cell-mediated collagen remodeling around PSCs is more evident compared to around NHDFs after 24 h (Fig. 6A), which underscores the importance of employing disease-relevant stromal cell types to best recapitulate the pathological behavior of the PDAC microenvironment.
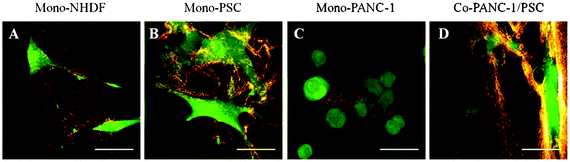 |
| Fig. 6 Representative live cell–collagen interactions within the microfluidic device. All cells were labeled with CellTracker Green and cultured for 24 h in 2 mg mL−1 ColI + 2 mg mL−1 HA. MPE was used to generate cell-labeled fluorescence (green) while SHG imaging was used to generate the intrinsic signal of collagen (orange). Higher SHG signal intensity is observed around PSC cell boundaries compared to around NHDFs likely due to the activated, contractive behavior of the PSCs. Compared to around PSCs, PANC-1 cells form 3D nests with minimal collagen remodeling after 24 h. Scale bars represent 100 μm. | |
Dose–response of the PDAC microenvironment model to paclitaxel
A major motivation to develop in vitro pathophysiologically-relevant human disease models is to provide alternative platforms to evaluate the preclinical efficacy of therapeutic candidates. Traditionally, the experimental continuum consists of lead discovery, cell-based assays, animal assays, and finally human trials. Unfortunately, many cancer therapeutics fail to translate in clinical trials due to lack of efficacy. This gap between preclinical promise and clinical reality arises in part from the inability of traditional preclinical models to realistically represent the heterogeneity of the human tumor microenvironment. Notably, the majority of existing in vitro platforms investigate cellular responses while mainly considering factors intrinsic to the cancer cells (i.e. genetics and epigenetics) and neglecting the influence of extrinsic factors in the microenvironmental niche.89
To demonstrate our models adaptability to testing therapeutics in a microenvironment context, paclitaxel was chosen as a prototypical anticancer agent. Paclitaxel induces cell cycle arrest and apoptosis by stabilizing microtubules. Recently, nab-paclitaxel, an albumin-bound formulation of paclitaxel, has been shown to selectively target and deplete PDAC stroma desmoplasia in preclinical trials.90 For comparison, we performed paclitaxel cytotoxicity assays on PANC-1 cells and PSCs using a conventional 2D microplate set-up. Results showed that paclitaxel effectively inhibited the cell survival and proliferation of both PSCs (IC50 = 0.197 nM) and PANC-1 cells (IC50 = 0.050 nM) and (Tables S4 and S5, ESI†). Based on these results, microfluidic cultures were allowed to contract for 24 h, treated with paclitaxel concentrations between 0 and 100 nM, and incubated for the same amount of time (48 h). Using simultaneous LIVE/DEAD® staining of viable and non-viable cells, decreasing viability was observed with increasing paclitaxel concentrations (Fig. 7A–C). Interestingly, trilayer compactness appeared to be disrupted by paclitaxel treatment as well. In particular, a greater percent area of cells-ECM was observed after paclitaxel treatment indicating expansion of the initially contracted cultures (Fig. 7D–F). Taken together, this data suggests that in addition to cytotoxicity, the model may be used to determine the biophysical effect (i.e. ECM compactness, collagen reorganization) of therapeutics on the PDAC microenvironment. Therapeutic targeting of the tumor stroma in addition to neoplastic cells is an innovative approach that has shown recent promise in the management of PDAC. The ability to perturb and characterize the ECM, as demonstrated possible in our model, may provide innovative means of elucidating the mechanisms behind cell–ECM interplay and developing novel therapeutic regimens designed to target both cells and ECM elements of the PDAC microenvironment.
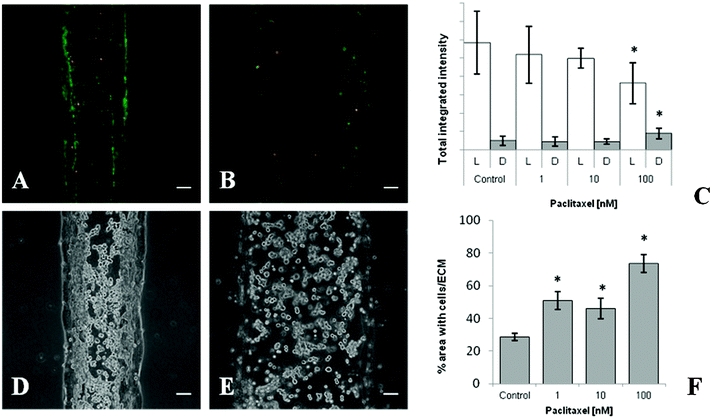 |
| Fig. 7 Response of the PDAC microenvironment model to paclitaxel treatment. Representative LIVE/DEAD® images of trilayer culture region after 48 h treatment with (A) 0.1% DMSO vehicle control and (B) 100 nM paclitaxel. (C) Brightness analysis of LIVE/DEAD® signal after increasing concentrations of paclitaxel treatment. Representative images showing structural integrity of the trilayer culture region after 48 h treatment with (D) 0.1% DMSO vehicle control and (E) 100 nM paclitaxel. (F) Quantified cell/ECM area as an indication of trilayer compactness. Each data point represents the mean ± standard deviation for three independently treated cultures, * p < 0.05 vs. control. Scale bars represent 100 μm. | |
Conclusion
Although accumulating evidence underscores the importance stroma–cancer interactions in PDAC, a robust in vitro model that represents the heterogeneity of the permissive tumor microenvironment is currently lacking. In order to translate findings from basic cellular research into clinical applications, human cell-based models need to recapitulate the inherent complexity of the PDAC microenvironment and at the same time accommodate real-time experimental intervention and observation. We developed and characterized an in vitro microfluidic model of the PDAC stroma–cancer microenvironment that incorporates the in vivo complexities of multicellularity, ECM components, and a rationally-defined 3D microarchitecture. The humanized PDAC model is expected to provide an enhanced “in vivo-like” surrogate to monitor and help accelerate understanding of dynamic pathological interactions between PSCs, cancer cells, and ECM components within the PDAC microenvironment. Validation against clinically-evaluated human tissues, characterization of cell–ECM interactions, and proof-of-concept assays for therapeutic efficacy support the model's potential applicability to basic PDAC research and therapeutic development.
Abbreviations
αSMA | alpha smooth muscle actin |
ColI | collagen type I |
ECM | extracellular matrix |
HA | hyaluronan |
MPE | multiphoton excitation |
NA | numerical aperture |
PDAC | pancreatic ductal adenocarcinoma |
PSC | pancreatic stellate cell |
SHG | second harmonic generation |
Acknowledgements
This work was financially supported by the University of Wisconsin-Madison and UW School of Pharmacy. The authors would like to thank the UWCCC Translational Science BioCore and Experimental Pathology Laboratory for services used to complete this research. These services are supported in part by NIH/NCI P30 CA014520-UW Comprehensive Cancer Center Support. The authors thank Dr Paolo Provenzano for useful conversations and the Williams Laboratory at the UW-Madison for assistance with microfluidic fabrication. The authors also thank the Laboratory for Optical and Computational Instrumentation (LOCI) for assistance with MPE/SHG imaging and data processing.
References
- A. Neesse, P. Michl, K. K. Frese, C. Feig, N. Cook, M. A. Jacobetz, M. P. Lolkema, M. Buchholz, K. P. Olive, T. M. Gress and D. A. Tuveson, Gut, 2011, 60, 861–868 CrossRef.
- M. Hidalgo, N. Engl. J. Med., 2010, 362, 1605–1617 CrossRef CAS.
- J. E. Shea, K. H. Nam, N. Rapoport and C. L. Scaife, HPB, 2011, 13, 153–157 CrossRef.
- T. Conroy, F. Desseigne, M. Ychou, O. Bouche, R. Guimbaud, Y. Becouarn, A. Adenis, J.-L. Raoul, S. Gourgou-Bourgade, C. de la Fouchardiere, J. Bennouna, J.-B. Bachet, F. Khemissa-Akouz, D. Pere-Verge, C. Delbaldo, E. Assenat, B. Chauffert, P. Michel, C. Montoto-Grillot, M. Ducreux, U. Grp Tumeurs Digestives and P. Intergrp, N. Engl. J. Med., 2011, 364, 1817–1825 CrossRef CAS.
- M. Erkan, S. Hausmann, C. W. Michalski, A. A. Fingerle, M. Dobritz, J. Kleeff and H. Friess, Nat. Rev. Gastroenterol. Hepatol., 2012, 9, 454–467 CrossRef CAS.
- M. Erkan, N. Weis, Z. Pan, C. Schwager, T. Samkharadze, X. H. Jiang, U. Wirkner, N. A. Giese, W. Ansorge, J. Debus, P. E. Huber, H. Friess, A. Abdollahi and J. Kleeff, Mol. Cancer, 2010, 9, 88 CrossRef.
- M. Erkan, G. Adler, M. V. Apte, M. G. Bachem, M. Buchholz, S. Detlefsen, I. Esposito, H. Friess, T. M. Gress, H.-J. Habisch, R. F. Hwang, R. Jaster, J. Kleeff, G. Kloeppel, C. Kordes, C. D. Logsdon, A. Masamune, C. W. Michalski, J. Oh, P. A. Phillips, M. Pinzani, C. Reiser-Erkan, H. Tsukamoto and J. Wilson, Gut, 2012, 61, 172–178 CrossRef CAS.
- J. M. Bailey, B. J. Swanson, T. Hamada, J. P. Eggers, P. K. Singh, T. Caffery, M. M. Ouellette and M. A. Hollingsworth, Clin. Cancer Res., 2008, 14, 5995–6004 CrossRef CAS.
- M. B. Omary, A. Lugea, A. W. Lowe and S. J. Pandol, J. Clin. Invest., 2007, 117, 50–59 CrossRef CAS.
- A. Vonlaufen, P. A. Phillips, Z. Xu, D. Goldstein, R. C. Pirola, J. S. Wilson and M. V. Apte, Cancer Research, 2008, 68, 7707–7710 CrossRef CAS.
- M. G. Bachem, M. Schunemann, M. Ramadani, M. Siech, H. Beger, A. Buck, S. X. Zhou, A. Schmid-Kotsas and G. Adler, Gastroenterology, 2005, 128, 907–921 CrossRef CAS.
- H. Tian, C. A. Callahan, K. J. DuPree, W. C. Darbonne, C. P. Ahn, S. J. Scales and F. J. de Sauvage, Proc. Natl. Acad. Sci. U. S. A., 2009, 106, 4254–4259 CrossRef CAS.
- K. Walter, N. Omura, S. M. Hong, M. Griffith, A. Vincent, M. Borges and M. Goggins, Clin. Cancer Res., 2010, 16, 1781–1789 CrossRef CAS.
- M. Erkan, J. Kleeff, A. Gorbachevski, C. Reiser, T. Mitkus, I. Esposito, T. Giese, M. W. Buchler, N. A. Giese and H. Friess, Gastroenterology, 2007, 132, 1447–1464 CrossRef CAS.
- T. S. Mantoni, S. Lunardi, O. Al-Assar, A. Masamune and T. B. Brunner, Cancer Res., 2011, 71, 3453–3458 CrossRef CAS.
- M. M. Erkan, C. Reiser-Erkan, C. W. Michalski, S. Deucker, D. Sauliunaite, S. Streit, I. Esposito, H. Friess and J. Kleeff, Neoplasia, 2009, 11, 497–508 CAS.
- D. Mahadevan and D. D. Von Hoff, Mol. Cancer Ther., 2007, 6, 1186–1197 CrossRef CAS.
- M. A. Shields, S. Dangi-Garimella, A. J. Redig and H. G. Munshi, Biochem. J., 2012, 441, 541–552 CrossRef CAS.
- M. Egeblad, M. G. Rasch and V. M. Weaver, Curr. Opin. Cell Biol., 2010, 22, 697–706 CrossRef CAS.
- H. O. Lee, S. R. Mullins, J. Franco-Barraza, M. Valianou, E. Cukierman and J. D. Cheng, BMC Cancer, 2011, 11, 245 CrossRef CAS.
- T. Stylianopoulos, J. D. Martin, V. P. Chauhan, S. R. Jain, B. Diop-Frimpong, N. Bardeesy, B. L. Smith, C. R. Ferrone, F. J. Hornicek, Y. Boucher, L. L. Munn and R. K. Jain, Proc. Natl. Acad. Sci. U. S. A., 2012, 109, 15101–15108 CrossRef CAS.
- P. P. Provenzano, C. Cuevas, A. E. Chang, V. K. Goel, D. D. Von Hoff and S. R. Hingorani, Cancer Cell, 2012, 21, 418–429 CrossRef CAS.
- P. Olson and D. Hanahan, Science, 2009, 324, 1400–1401 CrossRef CAS.
- K. P. Olive, M. A. Jacobetz, C. J. Davidson, A. Gopinathan, D. McIntyre, D. Honess, B. Madhu, M. A. Goldgraben, M. E. Caldwell, D. Allard, K. K. Frese, G. DeNicola, C. Feig, C. Combs, S. P. Winter, H. Ireland-Zecchini, S. Reichelt, W. J. Howat, A. Chang, M. Dhara, L. Wang, F. Rueckert, R. Gruetzmann, C. Pilarsky, K. Izeradjene, S. R. Hingorani, P. Huang, S. E. Davies, W. Plunkett, M. Egorin, R. H. Hruban, N. Whitebread, K. McGovern, J. Adams, C. Iacobuzio-Donahue, J. Griffiths and D. A. Tuveson, Science, 2009, 324, 1457–1461 CrossRef CAS.
- G. L. Beatty, E. G. Chiorean, M. P. Fishman, B. Saboury, U. R. Teitelbaum, W. Sun, R. D. Huhn, W. Song, D. Li, L. L. Sharp, D. A. Torigian, P. J. O'Dwyer and R. H. Vonderheide, Science, 2011, 331, 1612–1616 CrossRef CAS.
- B. Diop-Frimpong, V. P. Chauhan, S. Krane, Y. Boucher and R. K. Jain, Proc. Natl. Acad. Sci. U. S. A., 2011, 108, 2909–2914 CrossRef CAS.
- M. Magzoub, S. Jin and A. S. Verkman, Faseb Journal, 2008, 22, 276–284 CrossRef CAS.
- E. E. Merika, K. N. Syrigos and M. W. Saif, Gastroenterol. Res. Pract., 2012 DOI:10.1155/2012/781765.
- K. Garber, Journal of the National Cancer Institute, 2010, 102, 448–450 CrossRef.
- A. Dimou, K. N. Syrigos and M. W. Saif, Therapeutic Advances in Medical Oncology, 2012, 4, 271–279 CrossRef.
- P. A. Perez-Mancera, C. Guerra, M. Barbacid and D. A. Tuveson, Gastroenterology, 2012, 142, 1079–1092 CrossRef.
- G. Feldmann, S. Rauenzahn and A. Maitra, Expert Opin. Drug Discovery, 2009, 4, 429–443 CrossRef CAS.
- R. F. Hwang, T. Moore, T. Arumugam, V. Ramachandran, K. D. Amos, A. Rivera, B. Ji, D. B. Evans and C. D. Logsdon, Cancer Res., 2008, 68, 918–926 CrossRef CAS.
- H. Fujita, K. Ohuchida, K. Mizumoto, T. Egami, K. Miyoshi, T. Moriyama, L. Cui, J. Yu, M. Zhao, T. Manabe and M. Tanaka, Cancer Sci., 2009, 100, 2309–2317 CrossRef CAS.
- H. Hosoya, K. Kadowaki, M. Matsusaki, H. Cabral, H. Nishihara, H. Ijichi, K. Koike, K. Kataoka, K. Miyazono, M. Akashi and M. R. Kano, Biochem. Biophys. Res. Commun., 2012, 419, 32–37 CrossRef CAS.
- K. M. Yamada and E. Cukierman, Cell, 2007, 130, 601–610 CrossRef CAS.
- J. B. Kim, Semin. Cancer Biol., 2005, 15, 365–377 CrossRef.
- F. Pampaloni, E. G. Reynaud and E. H. K. Stelzer, Nat. Rev. Mol. Cell Biol., 2007, 8, 839–845 CrossRef CAS.
- L. C. E. Windus, D. L. Kiss, T. Glover and V. M. Avery, Exp. Cell Res., 2012, 318, 2507–2519 CrossRef CAS.
- Y. Matsuda, T. Ishiwata, Y. Kawamoto, K. Kawahara, W.-X. Peng, T. Yamamoto and Z. Naito, Med. Mol. Morphol., 2010, 43, 211–217 CrossRef.
- S. Ghosh, G. C. Spagnoli, I. Martin, S. Ploegert, P. Demougin, M. Heberer and A. Reschner, J. Cell. Physiol., 2005, 204, 522–531 CrossRef CAS.
- K. S. M. Smalley, M. Lioni and M. Herlyn, In Vitro Cell. Dev. Biol.: Anim., 2006, 42, 242–247 CrossRef CAS.
- I. Dufau, C. Frongia, F. Sicard, L. Dedieu, P. Cordelier, F. Ausseil, B. Ducommun and A. Valette, BMC Cancer, 2012, 12, 15 CrossRef CAS.
- D. Loessner, K. S. Stok, M. P. Lutolf, D. W. Hutmacher, J. A. Clements and S. C. Rizzi, Biomaterials, 2010, 31, 8494–8506 CrossRef CAS.
- H. Miyamoto, T. Murakami, K. Tsuchida, H. Sugino, H. Miyake and S. Tashiro, Pancreas, 2004, 28, 38–44 CrossRef CAS.
- F. Esni, Y. Miyamoto, S. D. Leach and B. Ghosh, Methods in molecular medicine, 2005, 103, 259–271 Search PubMed.
- B. Rubio-Viqueira, H. Mezzadra, M. E. Nielsen, A. Jimeno, X. Zhang, C. Iacobuzio-Donahue, A. Maitra, M. Hidalgo and S. Altiok, Mol. Cancer Ther., 2007, 6, 515–523 CrossRef CAS.
- J. J. Tentler, A. C. Tan, C. D. Weekes, A. Jimeno, S. Leong, T. M. Pitts, J. J. Arcaroli, W. A. Messersmith and S. G. Eckhardt, Nat. Rev. Clin. Oncol., 2012, 9, 338–350 CrossRef CAS.
- T. Ohmori, J. L. Yang, J. O. Price and C. L. Arteaga, Exp. Cell Res., 1998, 245, 350–359 CrossRef CAS.
- A. Takagi, M. Watanabe, Y. Ishii, J. Morita, Y. Hirokawa, T. Matsuzaki and T. Shiraishi, Anticancer Research, 2007, 27, 45–53 CAS.
- F. E. M. Froeling, T. A. Mirza, R. M. Feakins, A. Seedhar, G. Elia, I. R. Hart and H. M. Kocher, Am. J. Pathol., 2009, 175, 636–648 CrossRef CAS.
- L. F. Sempere, J. R. Gunn and M. Korc, Cancer Biol. Ther., 2011, 12, 198–207 CrossRef CAS.
- D. Wlodkowic and J. M. Cooper, Curr. Opin. Chem. Biol., 2010, 14, 556–567 CrossRef CAS.
- M. Marimuthu and S. Kim, Anal. Biochem., 2011, 413, 81–89 CrossRef CAS.
- D. J. Beebe, G. A. Mensing and G. M. Walker, Annu. Rev. Biomed. Eng., 2002, 4, 261–286 CrossRef CAS.
- E. W. K. Young and D. J. Beebe, Chem. Soc. Rev., 2010, 39, 1036–1048 RSC.
- W. Denk, J. H. Strickler and W. W. Webb, Science, 1990, 248, 73–76 CAS.
- P. J. Campagnola and L. M. Loew, Nat. Biotechnol., 2003, 21, 1356–1360 CrossRef CAS.
- K. E. Sung, N. Yang, C. Pehlke, P. J. Keely, K. W. Eliceiri, A. Friedl and D. J. Beebe, Integr. Biol., 2011, 3, 439–450 RSC.
- Y.-C. Toh, C. Zhang, J. Zhang, Y. M. Khong, S. Chang, V. D. Samper, D. van Noort, D. W. Hutmacher and H. Yu, Lab Chip, 2007, 7, 302–309 RSC.
- C. P. Huang, J. Lu, H. Seon, A. P. Lee, L. A. Flanagan, H.-Y. Kim, A. J. Putnam and N. L. Jeon, Lab Chip, 2009, 9, 1740–1748 RSC.
- M. Domenech, R. Bjerregaard, W. Bushman and D. J. Beebe, Integr. Biol., 2012, 4, 142–152 RSC.
- M. S. Kim, J. H. Yeon and J. K. Park, Biomed. Microdevices, 2007, 9, 25–34 CrossRef CAS.
- T. Liu, B. Lin and J. Qin, Lab Chip, 2010, 10, 1671–1677 RSC.
- M. G. Bachem, E. Schneider, H. Gross, H. Weidenbach, R. M. Schmid, A. Menke, M. Siech, H. Beger, A. Grunert and G. Adler, Gastroenterology, 1998, 115, 421–432 CrossRef CAS.
- E. L. Deer, J. Gonzalez-Hernandez, J. D. Coursen, J. E. Shea, J. Ngatia, C. L. Scaife, M. A. Firpo and S. J. Mulvihill, Pancreas, 2010, 39, 425–435 CrossRef CAS.
- M. V. Apte, P. S. Haber, T. L. Applegate, I. D. Norton, G. W. McCaughan, M. A. Korsten, R. C. Pirola and J. S. Wilson, Gut, 1998, 43, 128–133 CrossRef CAS.
- S. Dangi-Garimella, S. B. Krantz, M. R. Barron, M. A. Shields, M. J. Heiferman, P. J. Grippo, D. J. Bentrem and H. G. Munshi, Cancer Res., 2011, 71, 1019–1028 CrossRef CAS.
- T. Armstrong, G. Packham, L. B. Murphy, A. C. Bateman, J. A. Conti, D. R. Fine, C. D. Johnson, R. C. Benyon and J. P. Iredale, Clin. Cancer Res., 2004, 10, 7427–7437 CrossRef CAS.
- E. Ryschich, A. Khamidjanov, V. Kerkadze, M. W. Buchler, M. Zoller and J. Schmidt, Pancreas, 2009, 38, 804–810 CrossRef CAS.
- E. Brown, T. McKee, E. diTomaso, A. Pluen, B. Seed, Y. Boucher and R. K. Jain, Nat. Med., 2003, 9, 796–800 CrossRef CAS.
- T. Imamura, H. Iguchi, T. Manabe, G. Ohshio, T. Yoshimura, Z. H. Wang, H. Suwa, S. Ishigami and M. Imamura, Pancreas, 1995, 11, 357–364 CrossRef CAS.
- P. P. Provenzano, K. W. Eliceiri, J. M. Campbell, D. R. Inman, J. G. White and P. J. Keely, BMC Med., 2006, 4, 38 CrossRef.
- O. Nadiarnykh, R. B. LaComb, M. A. Brewer and P. J. Campagnola, BMC Cancer, 2010, 10, 94 CrossRef.
- M. W. Conklin, J. C. Eickhoff, K. M. Riching, C. A. Pehlke, K. W. Eliceiri, P. P. Provenzano, A. Friedl and P. J. Keely, Am. J. Pathol., 2011, 178, 1221–1232 CrossRef.
- A. D. Theocharis, M. E. Tsara, N. Papageorgacopoulou, D. D. Karavias and D. A. Theocharis, Biochim. Biophys. Acta, Mol. Basis Dis., 2000, 1502, 201–206 CrossRef CAS.
- M. A. Jacobetz, D. S. Chan, A. Neesse, T. E. Bapiro, N. Cook, K. K. Frese, C. Feig, T. Nakagawa, M. E. Caldwell, H. I. Zecchini, M. P. Lolkema, P. Jiang, A. Kultti, C. B. Thompson, D. C. Maneval, D. I. Jodrell, G. I. Frost, H. M. Shepard, J. N. Skepper and D. A. Tuveson, Gut, 2013, 62, 112–120 CrossRef CAS.
- J. Ringel, J. Rychly, B. Nebe, C. Schmidt, P. Muller, J. Emmrich, S. Liebe and M. Lohr, Cell and Molecular Biology of Pancreatic Carcinoma: Recent Developments in Research and Experimental Therapy, 1999, 880, 238–242 CAS.
- S. P. Hong, J. Wen, S. Bang, S. Park and S. Y. Song, Int. J. Cancer, 2009, 125, 2323–2331 CrossRef CAS.
- K. E. Sung, G. Su, C. Pehlke, S. M. Trier, K. W. Eliceiri, P. J. Keely, A. Friedl and D. J. Beebe, Biomaterials, 2009, 30, 4833–4841 CrossRef CAS.
- C. B. Raub, V. Suresh, T. Krasieva, J. Lyubovitsky, J. D. Mih, A. J. Putnam, B. J. Tromberg and S. C. George, Biophys. J., 2007, 92, 2212–2222 CrossRef CAS.
- A. Masamune, K. Kikuta, M. Satoh, K. Satoh and T. Shimosegawa, Br. J. Pharmacol., 2003, 140, 1292–1302 CrossRef CAS.
- A. Masamune and T. Shimosegawa, J. Gastroenterol., 2009, 44, 249–260 CrossRef.
- A. Y. Wehr, E. E. Furth, V. Sangar, I. A. Blair and K. H. Yu, Pancreas, 2011, 40, 557–566 CrossRef.
- W. Tan and T. A. Desai, Tissue Eng., 2003, 9, 255–267 CrossRef CAS.
- S. T. Kreger and S. L. Voytik-Harbin, Matrix Biol., 2009, 28, 336–346 CrossRef CAS.
- P. P. Provenzano, D. R. Inman, K. W. Eliceiri, S. M. Trier and P. J. Keely, Biophys. J., 2008, 95, 5374–5384 CrossRef CAS.
- P. P. Provenzano, K. W. Eliceiri and P. J. Keely, Trends Cell Biol., 2009, 19, 638–648 CrossRef CAS.
- E. S. Nakasone, H. A. Askautrud, T. Kees, J.-H. Park, V. Plaks, A. J. Ewald, M. Fein, M. G. Rasch, Y.-X. Tan, J. Qiu, J. Park, P. Sinha, M. J. Bissell, E. Frengen, Z. Werb and M. Egeblad, Cancer Cell, 2012, 21, 488–503 CrossRef CAS.
- D. D. Von Hoff, R. K. Ramanathan, M. J. Borad, D. A. Laheru, L. S. Smith, T. E. Wood, R. L. Korn, N. Desai, V. Trieu, J. L. Iglesias, H. Zhang, P. Soon-Shiong, T. Shi, N. V. Rajeshkumar, A. Maitra and M. Hidalgo, J. Clin. Oncol., 2011, 29, 4548–4554 CrossRef CAS.
Footnote |
† Electronic supplementary information (ESI) available. See DOI: 10.1039/c3lc50487e |
|
This journal is © The Royal Society of Chemistry 2013 |
Click here to see how this site uses Cookies. View our privacy policy here.