DOI:
10.1039/C1MD00210D
(Review Article)
Med. Chem. Commun., 2012,
3, 28-38
From the protein's perspective: the benefits and challenges of protein structure-based pharmacophore modeling
Received
17th August 2011
, Accepted 8th November 2011
First published on 12th December 2011
Abstract
A pharmacophore describes the arrangement of molecular features a ligand must contain to efficaciously bind a receptor. Pharmacophore models are developed to improve molecular understanding of ligand–protein interactions, and can be used as a tool to identify novel compounds that fulfil the pharmacophore requirements and have a high probability of being biologically active. Protein structure-based pharmacophores (SBPs) derive these molecular features by conversion of protein properties to reciprocal ligand space. Unlike ligand-based pharmacophore models, which require templates of ligands in their bioactive conformation, SBPs do not depend on ligand information. The current review describes the different steps in the construction of SBPs: (i) protein structure preparation, (ii) binding site detection, (iii) pharmacophore feature definition, and (iv) pharmacophore feature selection. We show that the SBP modeling workflow poses different challenges than ligand-based pharmacophore modeling, including the definition of protein pharmacophore features essential for ligand binding. A comprehensive overview of different SBP modeling and screening methods and applications is provided to illustrate that SBPs can be efficiently used for virtual screening, ligand binding mode prediction, and binding site similarity detection. Our review demonstrates that SBPs are valuable tools for hit and lead optimization, compound library design and target hopping, especially in cases where ligand information is scarce.
1. Introduction
The pharmacophore concept was first introduced in 1909 by Ehrlich who defined a pharmacophore as ‘a molecular framework that carries (phoros) the essential features responsible for a drug's (pharmacon) biological activity’.1 Later, the pharmacophore concept was more precisely described by Kier2 who stated that a drug must possess ‘(a) those atomic features suitable for the requisite drug–receptor interaction phenomena and (b) the appropriate spatial disposition of these features necessary to bring about the required simultaneous or required sequential interaction events with the receptor’.3 Gund further updated Kier's definition and described a pharmacophore in 1977 as “a set of structural features in a molecule that is recognized at a receptor site and is responsible for that molecule's biological activity”.4 Pharmacophores have proven to be extremely effective in silico filters in the search for bioactive molecules on several targets. Their use reduces the number of compounds and costs which have to be considered in large biophysical screenings.5–8 In contrast to e.g. topological/2D ligand similarity searches which take a whole-structure, ‘global,’ view on the activity of molecules, pharmacophores focus on ‘local’ similarity and study the molecular determinants and their specific arrangement required for biological activity.9 As a result they provide an explanation for the predicted activity of molecules. Ligand-based pharmacophores (LBPs), i.e., pharmacophore models derived from one or multiple active ligand(s), have been extensively used in the discovery and design of biologically active molecules.6Protein structure-based pharmacophores (SBPs), derived from the three-dimensional (3D) structure(s) of one or more protein target(s), are receiving more and more attention in the past few years.6,10 One of the reasons for the rising interest in SBPs is the significant increase in high resolution protein structures. Currently more than 75
000 three-dimensional structures of biological macromolecules (mostly proteins) are deposited in the Protein Databank (9th August 2011),11 leading to unprecedented understanding of these molecular drug targets. SBPs can be used as a tool to give insights into ligand–protein interactions and to enable large scale structural chemogenomics studies to identify new ligands for specific proteins (ligand profiling), or find new targets for specific ligands (target fishing).12–16 As such, there are three benefits of SBPs over LBPs: (i) SBPs allow the identification of novel scaffolds, as they are less biased towards existing ligand chemotypes. (ii) SBPs can be used to elucidate protein–ligand binding mode hypotheses within the protein structural framework,17–20 making SBPs suitable tools for structure-based ligand optimization. (iii) SBPs lead to better understanding of ligand binding sites. These insights can for example be used to find ligands for orphan receptors21 or to study ligand binding site similarities between different proteins to address cross-pharmacology22 and suggest new targets for existing drugs.23
Generally four different steps in the construction of SBPs can be distinguished: (i) protein structure preparation, (ii) binding site detection, (iii) pharmacophore feature definition, and (iv) pharmacophore feature selection (Fig. 1). Protein structure preparation and binding site detection are obviously specific aspects of SBP modeling. The protonation states of functional groups and conformation of the pharmacophore modeling template are clearly equally important determinants of LBPs and SBPs. For SBPs however, the possible variation in protonation states and conformations (at the protein backbone, sidechain, or polar hydrogen atoms level) is in principle larger, simply because ligand binding sites (in most cases) contain more atoms than ligands do. Whereas the features included in SBPs are generally the same as in LBPs, the initial number of features in SBPs is generally higher. As a result, pharmacophore feature selection (an important step in both LBP and SBP modeling workflows) requires a different approach and poses different challenges in LBP and SBP modeling protocols. Structural alignment of different ligands can be used to identify essential features in LBPs, and experimental data such as structure–activity relationships (SAR) can be used to further emphasize specific features in LBPs. Selection of essential features in SBPs is however not straightforward, even when guided directly by experimental data such as site-directed mutagenesis studies or indirectly by including amino acid sequence based knowledge or ligand information. The larger number of conformational and spatial possibilities in SBPs make the selection of pharmacophore features more complex than in LBPs.
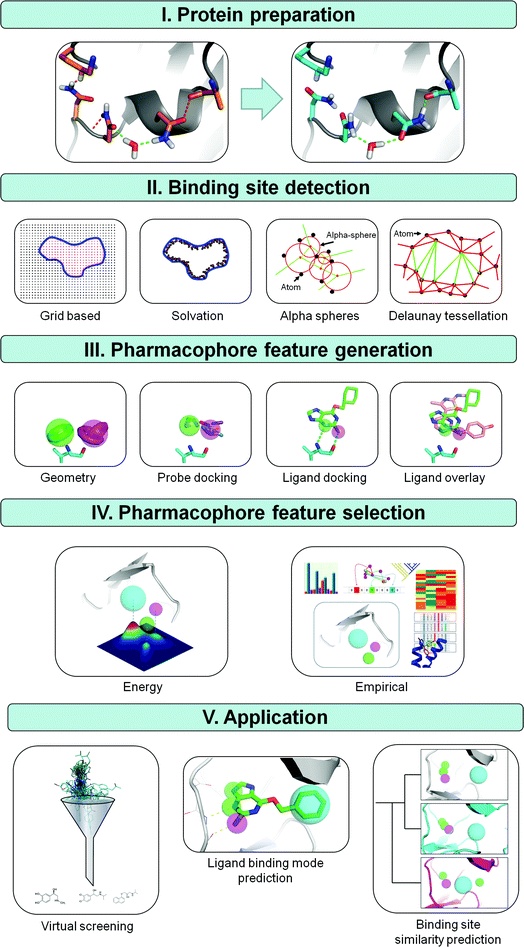 |
| Fig. 1 Methods for deriving structure based pharmacophores (SBPs). (I) The first step in the derivation of a SBP is the preparation of the protein structure. An example of PDB file 2BNU is shown with favorable interactions in green and unfavorable interactions in red. Three unfavorable interactions are observed in the original input file; an asparagine bumps into a lysine, a hydrogen of a neighboring asparagine clashes with the backbone and two partial negatively charged oxygens of an asparagine and backbone COMPOUND LINKS
Read more about this on ChemSpider
Download mol file of compoundalanine are in close proximity. Flipping these asparagines removes all unfavorable interactions and gains one additional favorable interaction.65 (II) Next, a cavity is defined using a grid-based, solvation, alpha sphere or the Delaunay tessellation based approach. (III) Pharmacophore features are subsequently derived by calculation of spatially favorable positions for functional groups by using known interaction geometries, probe docking, or by docking one or more ligands and extracting this information from ligand poses. (IV) To increase pharmacophore specificity an energy or statistics based method is finally applied to reduce the number of pharmacophore features in the final pharmacophore hypothesis. (V) Three possible applications for pharmacophores are virtual screening, ligand binding mode prediction and binding site similarity prediction. | |
The current review gives a comprehensive overview of different methodologies to construct SBPs and describes the specific benefits and challenges (Section 2). Furthermore, representative applications of SBPs are provided to illustrate that SBPs can be efficiently used for virtual screening, ligand binding mode prediction, and binding site similarity detection (Section 3). Our review demonstrates that SBPs are valuable tools for hit and lead optimization, compound library design and target hopping, especially in cases where ligand information is scarce.
2.
Protein structure based pharmacophore modeling methodology
In principle, the availability of a three dimensional protein structure or model is the only absolute pre-requisite for SBP methods. If the target protein structure is not known a protein structure model may be generated by homology modeling with the use of the sequence and the three dimensional structure of a close homolog.24 The subsequent steps in the SBP modeling workflow, protein preparation (2.1), binding site detection (2.2), pharmacophore feature definition (2.3), and pharmacophore feature selection (2.4) are outlined in Fig. 1 and discussed in this section. An overview and classification of the different SBP methods for binding site detection, feature definition and selection is presented in Table 1.
Table 1 Different structure based pharmacophore methodologies and their specifications
Method/software |
Protein preparation |
Binding site detection |
Feature definition |
Feature selection |
Resolutiona |
Automationb |
Reference |
High resolution methods require one high resolution structure from which all information is deduced. Medium resolution methods are able to combine different high resolution structures. Low resolution models do not require a high resolution structure, instead typically use interaction geometries, rotamer ensembles or simulations to cover a range of probable conformations.
Highly automated methods can produce pharmacophores by the push of a button and have a fully integrated workflow. Medium automation requires a call to separate procedures. Low automation methods require the user to specify at which location or residue a feature should be positioned without giving much guidance except possibly the coloring of surfaces according to calculated properties.
The method uses a fixed position for pharmacophore features and positions them solely based on sequence information.
The method requires a ligand.
|
Catalyst/Discovery Studio |
Yes |
Grid |
Geometry |
Energy |
Medium |
Medium |
50
|
Chemogenomics (Klabunde) |
No |
Fixedc |
Fixedc |
— |
Low |
High |
28
|
FLAP
|
Yes |
Grid |
Geometry |
Energy |
Medium |
Medium |
66 and 67
|
GBPM
|
Yes |
Grid |
Geometry |
Energyd |
Medium |
Medium |
68
|
HS-Pharm |
Yes |
Grid |
Geometry |
Empirical |
Medium |
Medium |
69
|
LigandScout |
Yes |
|
Ligand |
Energy |
High |
High |
70
|
MOE
|
Yes |
Alpha spheres |
Ligand |
Energy/manual |
High |
High |
52
|
MUSIC |
Yes |
Solvation |
Probe
|
Energy |
Low |
Medium |
47
|
Pocket v2 |
Yes |
Grid |
Geometry |
Energy |
Medium |
High |
71
|
Schrodinger |
Yes |
Grid |
Probe
|
Energy |
High |
Medium |
72
|
Ligand
|
Energy
|
High |
Snooker |
No |
Delaunay |
Geometry |
Empirical |
Low |
High |
73
|
Sybyl |
Yes |
Grid |
Geometry |
Knowledge |
Medium |
Low |
49
|
Solvation |
Ligand |
Energy/manual |
2.1
Protein structure preparation
2.1.1 Protonation states.
The first step in the construction of a SBP is the preparation of the protein structure (Fig. 1), including the consideration of non-protein groups (e.g.water molecules or cofactors), the determination of protonation states and positions of hydrogen atoms of protein residues, and the consideration of (alternative) protein conformations. Structures obtained by traditional X-ray crystallography and deposited in the PDB usually lack the positions of the hydrogens. These atoms are added according to basic pH dependent chemistry rules. Protonation states of aspartate, glutamate and histidine are typically solved by pKa predictions of the local structural environment while flipping of asparagine, COMPOUND LINKS
Read more about this on ChemSpider
Download mol file of compoundglutamine and histidine side chains as well as tautomer optimization for histidine are performed to optimize the hydrogen bonding network.25 An assessment of the accuracy of various methods for predicting hydrogen positions in protein structures has been reported by Forrest and Honig.26 A protein preparation step is recommended since many structures deposited in the PDB contain errors.27 An energy minimization is optional to obtain the protein structure (or protein–ligand complex in the case of a holo-structure) at the closest local minimum. Not all SBP methods require a correctly prepared protein structure (Table 1). Both the chemogenomics approaches as described by Klabunde et al.28 and Snooker29 do not require high resolution ‘cleaned’ protein structures. The chemogenomics method uses fixed pharmacophore positions and needs only a correlation matrix between available pharmacophore features plus the sequence to create a pharmacophore. Snooker uses a structural template together with the sequence to build an ensemble structure which contains all likely rotamers of all residues. Since Snooker uses fixed rules for residue interaction geometries which are tautomer and protonation state independent, a protein preparation step is not required.
2.1.2
Protein conformations.
Although LBP methods can in theory consider protein flexibility by aligning ensembles of molecular conformations, they typically assign primacy to the ‘biologically active’ ligand conformation and use only this to align multiple active molecules and deduce a pharmacophore.30,31 However, a recent systematic study suggests that ligands rarely bind in their lowest calculated energy conformation,32 obviously making the problem of selecting biologically active conformations for constructing LBPs more complicated. Alternatively, many applications allow an externally derived conformation of an active molecule to be used as a template in the alignment of active molecules from which the pharmacophore is derived. In SBP modelling, it has been widely accepted that the conformational flexibility of proteins has to be taken into account. Flexible docking algorithms typically consider a number of protein conformations and keep a limited number of residues flexible during the docking run.33–35 Carlson and Meagher developed an approach to incorporate protein flexibility in SBPs by generating pharmacophores from molecular dynamics (MD) simulations of protein–ligand complexes36 based on clustering of the aligned pharmacophores of different snapshots. It is also possible to align the results of docking simulations, rendering this method suitable for the modeling of flexible proteins. Loving et al.37 and Salam et al.38 for example used Phase39 to flexibly dock ligands/fragments and generate pharmacophore sites based on the docking solutions. They subsequently decomposed the Glide XP docking scores to energy terms for all individual probe fragments (hydrogen bond acceptors/donors, positive and negative ionizable groups and hydrophobic and aromatic interactions) and mapped these onto the different pharmacophore sites to rank the features. Using this approach Loving et al. reproduced the binding modes of 12 targets and recovered known actives from a database screen,37 while Salam et al. generated 30 successful reduced pharmacophore definitions and used them in enrichment studies.38 Geometry based methods also allow the generation of ‘consensus’ pharmacophores by overlaying protein structures and thus interaction maps. Molecular interaction fields (MIFs),40LUDI41,42 and Snooker interaction maps generated from different models of the same protein can be superposed to extract the most robust regions of interactions. Snooker by default uses the rotamer statistics as obtained from a rotamer library43 to build an ensemble model for each protein.
2.2
Binding site detection
The second step in the SBP modeling workflow (Fig. 1) is to define the location of the ligand binding site by the application of binding site detection algorithms. Retrospective studies show that these algorithms perform very well in the case of ligand bound crystal structures, correctly detecting up to 95% of the ligand binding pockets, but are less accurate for apo-structures.44 Structure based binding site detection can be divided into energy based methods (including solvation methods) and geometry based methods (including grid based, alpha sphere, Delaunay tessellation) as summarized in Fig. 1 and Table 1. Energy-based algorithms, like PocketFinder,45 SuperStar,46 and MUSIC,47 the solvation method of SiteID (Tripos) and SiteMap (Schrödinger) try to describe the local surface properties of a cavity by the simulation of solvent molecule interactions with the protein surface. SiteMap48 uses a grid to sample binding site properties but is nevertheless fully based on interaction energy calculation. MUSIC47 floods the pocket with probe fragments instead of solvent molecules and simulates the interactions of those fragments in short MD-simulations. Geometry based methods can be divided into two categories: discrete grid-based sampling and analytic methods like Delaunay tessellation and alpha sphere based methods. Grid-based methods available in SiteID (Tripos49), GRID40 and in the binding site analysis module of InsightII (Accelrys50) typically select grid points close to but not overlapping with protein atoms, and define the pocket after a flood filling algorithm of grid points located in a cavity, where cavity grid points are defined as grid points with a substantial number of contacts with the protein. Snooker identifies the protein pocket by a Delaunay tessellation51 of Cα-atoms and a calculated mean side chain atom, followed by the indexing of tetrahedra with at least one ‘long’ edge (Fig. 1). Delaunay tessellation implies the generation of an aggregate of space-filling irregular tetrahedra. These tetrahedra are deduced from a set of coordinates such that for each tetrahedron, the four vertices are on the circumspheres while no other vertices are inside the circumsphere. It is used to identify all tetrahedra which span large distances and cover potential ligand binding pockets. Finally, all indexed tetrahedra having a triangle in common are merged and the largest merged volume is defined as the pocket, while all non-indexed tetrahedra form the protein volume. SiteFinder (MOE52), PASS,53SURFNET,54 LIGSITE,55 APROPOS,56 and CAST57,58 use alpha complexes to detect pockets. Alpha shapes are an extension of the convex hulls proposed by Edelsbrunner and Mucke.59 Alpha spheres associated with 4 atoms can be generated from the simplices of a Delaunay tessellation (Fig. 1). Solvent exposed alpha spheres and those corresponding to inaccessible areas (small spheres) are removed and the pocket is detected by aggregation of remaining nearby alpha spheres. Two reviews summarizing and explaining ligand-binding site detection have been written by Prymula et al.60 and Henrich et al.61
2.3 Pharmacophore feature definition
In the third step in the SBP flow scheme (Fig. 1), pharmacophore features are derived from the co-crystallized ligand or from the ligand binding site (determined in the previous step) itself (Table 1). Protein structure based pharmacophore methods typically use geometric entities, such as spheres, vectors and planes with given attributes to characterize favorable interactions. The commonly used interaction types include H-bond acceptors, H-bond donors, positive and negative ionizable groups, lipophilic regions and aromatic rings. Their positions are set according to either positions of co-crystallized ligands or basic interaction geometry rules.
Derivation of pharmacophores from ligands is ostensibly straightforward with features positioned at functional groups of the ligand. Ligand based pharmacophore modeling tools are generally similar although they may produce slightly different pharmacophores due to differences in feature definitions and algorithmic search strategies.62,63 An excellent review providing a detailed explanation of the available molecular alignment techniques has been written by Lemmen and Lengauer.64 As each software package has differences in feature definition criteria, it is appropriate to use the same algorithm for both pharmacophore elucidation and pharmacophore searching, in order to ensure compatibility. If the structure of a protein–ligand complex is available, a pharmacophore can be constructed by positioning features at the functional groups of ligands (Table 1).
Structure based pharmacophore derivation from apo structures is in contrast more challenging. The number of potential interacting residues in a ligand binding site is typically larger than the number of observed interactions in protein–ligand complexes. Furthermore it is not straightforward to determine the optimal interaction geometry and there is no guarantee that ligands will interact at the predicted favorable sites of interaction. Some targets even have diverse ligand-binding modes and require a set of different pharmacophores to cover the interaction observed for all ligands.74–80 Pharmacophore feature placement is therefore less accurate in structure based pharmacophore methods and tolerances of pharmacophore features are typically less strict. FLAP,66,67GBPM,68 Pocket V2,71 Discovery Studio (Accelrys50), Sybyl (Tripos49) and Snooker29 can create SBPs without the use of any ligand information and apply knowledge from residue based interaction geometries to predict likely interactions and their locations. MUSIC47 and Schrodinger37,38 also do not require information about known actives and use a multiple copy simultaneous search (MCSS) method81 to identify energetically favorable positions and properties of probes to generate pharmacophore features.38 In the MCSS method a protein's active site is filled with thousands of copies of organic functional groups which are allowed to energy-minimize onto the protein surface. Groups that minimize at the same location and bind most tightly are subsequently converted into pharmacophore features. MOE52 and LigandScout70 at least require one known active ligand and a protein structure. Both can be used to generate a binding mode hypothesis and extract pharmacophore features from the modelled interactions. An improved pharmacophore can potentially be defined if multiple ligands are docked into the receptor active site and if only the conserved interactions are translated into pharmacophore features. Two recent comparative reviews on pharmacophore elucidation methods have recently been published by Luu et al. and Wallach.82,83
2.4 Selection of essential pharmacophore features
To obtain valid binding mode hypotheses and subsequent compound library enrichment it is important to select only those features that correlate with biological activity (step 4 in the SBP modeling workflow (Fig. 1)). Three different approaches can be defined to select essential pharmacophore features (Fig. 1 and Table 1): (i) using interaction energy calculations (2.4.1), (ii) using protein–ligand interaction information (2.4.2), and (iii) based on analysis of amino acid sequence variation (2.4.3). Finally, SBPs can be refined by training pharmacophore models with known active compounds (2.4.4) and by complementing SBPs with shape restraints (2.4.5).
2.4.1 Energy-based selection.
Several SBP methods select pharmacophore features based on their (potential) interaction energy with ligands (Table 1). In cases where (the binding mode of) only one ligand is known, protein–ligand interaction energies can be estimated to prioritize interactions and discard those with small contributions to the overall binding energy. Methods using probe docking or simulation typically select features at positions where probes have high interaction energies. Here the possibility also exists to examine multiple protein structures obtained viaexperimental methods or homology modeling. Such structures can be overlaid after which ‘hot spots’ can be identified which represent conserved or highly favourable interactions. Most challenging is the selection of features after a geometry-based feature definition. Often, these methods place many pharmacophore features in the binding pocket and provide little information on which features to select. Sybyl49 allows the user to manually pick the residues which correlate with binding activity and provides guidance via the calculation of surface properties. FLAP66,67 uses GRID40 to generate molecular interaction fields (MIFs) which are condensed into discrete points representing the most favorable interactions. Pocket v.271 uses a similar approach and generates a scored grid with the Pocket program to rank the protein–ligand interactions observed in the structure and utilizes this to automatically reduce the multitude of features to a reasonable number. Pocket v.2 also has the ability to suggest new binding spots besides the pharmacophore features already represented by a protein–ligand complex. Accelrys provides Ludi interaction maps41 for H-bond donor, H-bond acceptor and hydrophobic interactions. These are extracted from distributions of non-bonded contacts generated by a search through the Cambridge Structural Database42 (which contains statistics about small molecule crystal structures) or generated by the application of interaction geometry rules which typically describe desired distances and angles between interacting pairs. Features are most likely in denser areas of those distributions. An alternative approach to GRID, LUDI and scored grids of the Pocket program is the extended electron distribution (XED).84 In contrast to the three methods previously described, XED generates field points based on a quantum orbital model. This enables the generation of multipoles for electronegative atoms with Π orbitals resulting in a potentially more precise description of the desired sites of interaction.
2.4.2 Selection based on protein–ligand interaction information.
If multiple ligands are available and binding mode hypotheses are generated, features which correlate with conserved protein–ligand interactions may be identified (Table 1). McGregor for example generated a pharmacophore for small molecule protein kinase inhibitors after extraction of conserved interactions in 220 kinase crystal structures.85 Fingerprint methods like SIFt,86SQUID87 and FLAP66 encode protein–ligand interactions in binary bit strings and have been shown to be very useful for such analysis. The fingerprints typically contain information on the residue numbers and interaction type (HBA, HBD, positive ionisable, negative ionisable, hydrophobic, aromatic) observed in a protein–ligand complex. HS-Pharm69 prioritizes cavity atoms that should be targeted for ligand binding, by training machine learning algorithms with atom based fingerprints of known ligand-binding pockets and was shown to have better enrichment curves for 2 out of 3 targets compared to docking algorithms.69 An overview of pharmacophore methods classified according to the pharmacophore comparison method (alignment/fingerprint based) can be found in a recent review by Luu et al.82
2.4.3 Selection based on variation of proteinamino acids in the ligand binding site.
Amino acid sequence variations can be used to evaluate the role of individual amino acid residues in the binding site and select those that are important for ligand binding. These data can be derived from single nucleotide polymorphisms (SNPs), site-directed mutagenesis studies, or sequence alignments of protein families. Snooker prioritizes cavity residues by analysis of a multiple sequence alignment (MSA) of a large set of homologous protein sequences. Klabunde28 uses a set of 10 homology models and 3 X-ray structures to generate 35 single-feature pharmacophore elements associated with a sequence motif, the assumption being that certain sequence motifs at fixed positions are by definition important for ligand binding. Methods that use knowledge based prioritization of interactions (Table 1) are usually less dependent on the accuracy of the structures and models and are therefore better suited for protein families for which little structural data are available, like the G-protein coupled receptor (GPCR) family.
2.4.4 Training of SBPs with known actives.
Known active compounds can be used to select specific (combinations of) pharmacophore features and to include shape restraints in the SBP model. Purely structure based pharmacophore models can be tested and optimized by searching a set of known active compounds and selecting only combinations of pharmacophore features which correlate to actives. Accelrys offers for example the Ligand Profiler Protocol to create a heatmap of ligandsvs. pharmacophores. In this way, retrospective virtual screening studies can be used to identify pharmacophore features which discriminate known ligands from inactive (or decoy) molecules.69 Snooker has been used to select compound sets by using ligand-based shape constraints based on poses of the active compound in different sub-pharmacophores. The scoring function used by FLAP can be trained by supplying a set of known actives and known inactives and simultaneously minimizing the fraction of false positives and false negatives.
2.4.5 Shape restraints.
Shape and volume are valuable concepts in drug design as outlined in a review by Nicholls et al.88 Both concepts provide guidance for scaffold ‘decoration’ with chemical groups for virtual screening and lead optimization and can also be used in library design, ligand fitting, pose prediction, or active site description. The selectivity of pharmacophores can also be increased by the addition of shape restraints. Greenidge et al.89 showed that the number of false positives could be decreased by a factor of 2–5 by the application of excluded volumes while the number of true positives remains nearly unchanged. Klabunde et al.28 indicate that the enrichment they obtain is largely due to the addition of shape restraints to their initial pharmacophores. The program FLAP has the ability to describe the shape of the binding site by using shape probes in the GRID force field on which the fingerprints are based.66,90 Restraints can be added at positions occupied by the receptor, by space not occupied by a set of known actives or by setting a minimum shape similarity (volume overlap) to a reference compound. Using a shape restraint often ensures that especially large molecules with many interacting groups can only match the pharmacophore features in a conformation which is complementary to the protein binding site and does not match purely by increased probability. Rella et al.91 showed the contribution of different shape restraints for COMPOUND LINKS
Read more about this on ChemSpider
Download mol file of compoundangiotensin converting enzyme 2 inhibitors. First they generated a pharmacophore comprising 5 features (two hydrogen bond acceptors (HBAs), two hydrophobic groups and one zinc binding group) and screened a compound library of 3.8 M compounds of which they retrieved 1 M compounds. By the addition of a shape restraint set at 130% of the co-crystallized ligand volume, a further reduction to 91
000 compounds was achieved. Filtering of this set with 25 excluded volume spheres placed at positions occupied by the receptor gave 56
000 compounds while only 38
000 compounds were selected after the reduction in HBA tolerances to 1.3 Å. A final shape filter of 110% and 100% of the reference structure volume reduced the selection to 35
000 and 16
665 compounds, respectively. Seventeen compounds were selected based on high fit values as well as diverse structures and subjected to experimental validation in a bioassay. All these compounds showed an inhibitory effect on ACE2 activity. Some virtual screening methodologies are even entirely based on shape, like the ROCS method from OpenEye and Shape4 developed by Ebalunode et al.92 Both have been reported to perform well in terms of virtual screening.93,94 However to derive specific shape restraints the conformation and binding mode of at least one known active molecule are required and these methods have therefore limited compatibility with structure based pharmacophores.
3. Applications of SBPs
SBPs are developed to improve molecular understanding of ligand–protein interactions (3.1). As already mentioned in Section 1, SBPs can be used for virtual screening (3.2), ligand binding mode prediction (3.3), and binding site similarity detection (3.4). The lower part of Fig. 1 gives a pictorial description of three different uses of SBPs and an overview of relevant articles concerning the application of structure-based pharmacophores is presented in Table 2. The application papers discussed in this review are diverse with respect to the generation method, target family, available data and used software and will be discussed in more detail in this section. The SBP models described in the current section furthermore exemplify: (1) some of the potential advantages of SBPs over LBPs; (2) the discovery of novel scaffolds different from known chemotypes; (3) the elucidation of protein–ligand binding modes, and (4) better understanding of ligand binding sites.
Table 2 Examples of structure based pharmacophore studies demonstrating the potential and wide variety of applications of SBPs for different protein targets
Target |
Family |
Input |
Method |
Predictionc |
Result |
Reference |
The chemogenomics approach has fixed positions for features and positions features depending on the protein sequence.
Features are positioned on the protein (do not describe desired ligand features).
VS: virtual screening, BM: binding mode hypothesis generation, BS similarity: binding site similarity prediction.
|
AlaR |
Racemase
|
X-Ray |
Overlay |
VS |
19 Compounds selected for testing |
121
|
C3AR1
|
GPCR
|
— |
Chemoprintsa |
VS |
4 New ligands found |
28
|
Various |
Me–Lys binders |
X-Ray |
Protein
b
|
BS similarity |
Similar sites identified |
139
|
CDK2
|
Transferase
|
X-Ray |
Overlay |
Model validation |
Recognition known ligands |
140
|
CHK1
|
Transferase
|
X-Ray |
Overlay |
VS |
Enrichment > 9-fold |
122
|
DHFR-TS |
Synthase
|
X-Ray |
Complex |
BM + affinity |
Correct prediction 6 ligands |
141
|
DNMT1
|
Transferase
|
Model |
Probe
|
BM + VS |
Explanatory pharmacophore model derived |
142
|
HIV-1 RT |
Transcriptase |
X-Ray-NMR |
Geometry |
BM
|
HIV-1RT superligand generated |
138
|
HtrA |
Protease
|
Model |
Geometry |
VS |
6 New ligands found |
120
|
Kinase
|
Transferase
|
X-Ray |
Geometry |
BS similarity |
Relevant clustering of kinase families |
90
|
Kinase
|
Transferase
|
X-Ray |
Geometry |
BS similarity |
Bio-isosters proposed for multiple targets |
143
|
Thrombin |
Protease
|
|
|
|
|
|
Kv1.5
|
Potassium channel
|
Model |
Geometry |
VS |
19 New ligands found |
124
|
NK1R
|
GPCR
|
Model |
Overlay |
VS |
1 New ligands found |
123
|
NS3
|
Protease/helicase/NTPase |
X-Ray |
Overlay |
BM + VS |
15 Compounds selected for testing, ligand interacting residues identified |
135
|
Renin
|
Angiotensinogenase |
X-Ray |
Interaction |
BM + VS |
2 New ligands found |
136
|
RSK2
|
Transferase
|
Model |
Overlay |
VS + BM |
2 New ligands found |
144
|
Thrombin |
Protease
|
X-Ray |
Geometry |
VS + scaffold hopping |
Enrichment > 15-fold, successful scaffold replacement |
137
|
TrXr, HIV-1N |
Reductase–Integrase |
X-Ray |
Geometry |
VS |
1 New ligand found |
119
|
Various |
— |
X-Ray |
Complex |
VS |
Average 40-fold enrichment |
38
|
Various |
— |
X-Ray |
Geometry |
VS |
Average 17-fold enrichment |
67
|
3.1 SBPs versus LBPs
While comparativeligand- and structure-based pharmacophore modelling studies are relatively scarce,95 more and more protocols are reported in which LBP and SBPs are combined.96–99 This is in line with recent comparative virtual screening studies which show that ligand- and protein structure-based methods are complementary approaches in identifying different ligand chemotypes.99–101
Thangapandian et al.95 report a comparative study of ligand- and structure-based pharmacophores for the design of novel histone deacetylase 8 inhibitors. The LBP comprised 4 features and retrieved 117 compounds of which 87 were active (corresponding to an ∼8-fold enrichment over random picking), while the SBP contained 6 features and retrieved 74 compounds of which 63 were active (corresponding to a comparable enrichment value of ∼10). Kumar et al.96 describe a method which combines LBPs and SBPs in order to identify additional interaction sites with c-Jun N-terminal kinase-3 that cannot be derived by ligand-based approaches alone. Using a training set of 21 c-Jun N-terminal kinase-3 inhibitors a LBP of 4 features was derived and used to construct a quantitative pharmacophore model to predict the affinities of 85 inhibitors with a correlation coefficient r2 of 0.846. Conserved hydrogen bond interactions with the hinge region were subsequently identified by docking of the 85 inhibitors into the kinase binding site and a SBP was derived which contained three additional features (2 donors and 1 acceptor) compared to the previously constructed LBP. Griffith et al.97 and Singh et al.102 have described protocols that combine the speed of LBPs with the ability of SBPs to predict ligand binding modes and to discover novel molecules.
Comparative retrospective virtual screening studies report similar99 or somewhat higher100,101 overall enrichment for ligand-based methods compared to structure-based virtual screening approaches.101 The performance of different ligand-based103,104 and docking-based methods105 as well as the relative performance of ligand- vs. structure-based methods99–101 can however be highly target dependent, justifying the use of both ligand-based and structure-based drug design techniques as complementary ligand discovery tools. Evers et al.100 for example performed a retrospective virtual screening study on four biogenic amine-binding G-protein coupled receptors (GPCRs) in which three ligand-based methods (LBPs,106,107 2D,108 and 3D similarity searches109) were compared with docking110–112 in homology models. They showed that ligand-based methods outperform structure based methods, although structure-based methods still have satisfying enrichment factors (up to 60% of actives in the top-ranked 1% of the screened database). Krüger and Evers99 compared docking,113–116 3D similarity searches117 and 2D similarity searches118 and reported almost equal enrichments. Hit lists obtained from different algorithms were however complementary and the combination of different approaches is likely to result in more (and more diverse) actives.
3.2 Virtual screening (VS) for new ligands
Structure based pharmacophores (SBPs) are very well suited to combine the efficient screening methodologies of pharmacophores with structural information obtained from X-ray crystallization and NMR spectroscopy efforts. In this section 7 virtual screening studies are described28,38,67,119–123 which used different methods (geometry (3.2.1), probe (3.2.2), complex (3.2.3), overlay (3.2.4), and chemogenomics (3.2.5) based) to derive and select pharmacophore features (Fig. 1).
3.2.1 VS using geometry based SBP.
Tintori et al. used GRID to generate SBPs and showed that they could identify active molecules for the thioredoxin reductase enzyme in a virtual screen and discovered novel classes of active compounds able to inhibit complex formation between HIV-1 1N and viral host.119 Pirard et al. used PASS (Putative Active Site with Spheres)53 to identify the binding site and GRID to generate molecular interaction fields.124 Manual selection of minima from the GRID energy maps and virtual screening with UNITY49 resulted in 19 novel potassium channel blockers. Cross et al. used FLAP to generate SBPs for 13 different targets extracted from the DUD (Directory of Useful Decoys) and showed enrichment values of approximately 17 fold over random at a false positive rate of 1%.67 Significantly, they retrieved a variety of chemotypes demonstrating that lead-hopping and scaffold hopping into different chemical classes with SBPs are feasible.67,125 Löwer et al. used PocketPicker126,127 to extract binding pockets and generated interaction points complementary to the protein residues using Ludi rules.42,128 A pharmacophore was identified in regions of high interaction point density by the LIQUID program.129 With this SBP six compounds (from 22 selected in silico hits) were identified that were able to block E-cadherin cleavage by HtrA.120
3.2.2 VS using probe based SBP.
Mustata and Briggs used molecular dynamics (MD) simulations to take protein flexibility into account in the construction of SBPs.121 Ligbuilder130 was used to generate property maps of the individual frames of the simulation of COMPOUND LINKS
Read more about this on ChemSpider
Download mol file of compoundalanine racemase. Subsequently a dynamic pharmacophore was extracted using a MCSS approach81 and the resulting SBP was used to identify compounds from ACD for experimental validation (results not reported).
3.2.3 VS using complex based SBP.
Salam et al. derived SBPs from 30 different protein–ligand complexes and used the energetic terms computed by the Glide XP scoring function to rank the importance of pharmacophore features.38 In a subsequent virtual screen they obtained enrichment values ranging from 3 to 100 (average 40) at 1% of the decoy database screened.38
3.2.4 VS using overlay based SBP.
Chen et al. derived a common feature pharmacophore by clustering multiple structure based pharmacophore features from different Chk1–ligand complexes in comparable binding modes.122 This pharmacophore was used in combination with excluded volumes and shape constraints and showed an enrichment factor of >9 in a virtual screen. Evers et al. used the MOBILE method131 to generate a NK1 receptor model that was able to accommodate known NK1 antagonists from structurally diverse classes. Using mutational data from the literature and the features common to all known NK1 antagonists considered, they deduced a pharmacophore model which was used to select 7 compounds for biochemical testing, 1 of which showed affinity in the submicromolar range.123
3.2.5 VS using chemoprint based SBP.
Klabunde et al. used homology models and corresponding binding mode hypotheses to derive pharmacophore features associated with specific amino acid sequence motifs.28 These so-called chemoprints were used to generate SBPs for the Urotensin-II receptor and Complement component 3a receptor 1 (C3AR1). Additional shape restraints were extracted from binding mode hypotheses of known active compounds and a virtual screen resulted in the successful retrospective identification of 81 COMPOUND LINKS
Read more about this on ChemSpider
Download mol file of compoundurotensin-II receptor ligands and in a prospective identification of 4 C3AR1 ligands.
3.3
Ligand binding mode prediction
In contrast to ligand-based pharmacophores, structure-based pharmacophores add to the understanding of the interaction of a (set of) ligand(s) with the protein. This is beneficial for affinity and selectivity prediction, defining Structure–Activity Relationships (SAR), and hit optimization. SBPs can also be used for a complete exploration of the binding pocket and enable the targeting of residues that have not previously been utilized in interactions with ligands. A prerequisite for all these studies is the correct prediction of the ligand binding. So far only a few studies have shown that SBPs are capable to reproduce ligand binding modes of experimentally determined protein–ligand complexes. Loving et al.37 showed that they could reproduce the binding modes observed in 12 different protein–ligand complexes, while Sanders et al. used SBPs to correctly predict the binding modes of a set of β-2-adrenergic agonists and antagonists/inverse agonists. SBPs can also be used in pharmacophore constrained docking and several studies have shown that pharmacophore constraints can significantly improve binding mode predictions and virtual screening enrichment.39,132–134 In this section several different methods to derive and use SBPs in binding mode prediction will be discussed.
3.3.1
Ligand BM prediction using geometry based SBP.
Kaczor and Matosiuk have derived a SBP from a LUDI interaction map of the NS3 binding site.135 This SBP was successfully used to identify new ligand interacting residues for this protein. Thangapandian et al. used Discovery Studio to generate LUDI maps of the crystal structure of renin with co-crystallized COMPOUND LINKS
Read more about this on ChemSpider
Download mol file of compoundaliskiren and used the cluster pharmacophore tool to generate pharmacophore features.136 Superimposition and analysis of two other structures with co-crystallized ligands overlaid on the aliskiren structure resulted in the selection of representative pharmacophoric features of catalytic importance. Ahlström et al. constructed a thrombin SBP model based on GRID molecular interaction fields (MIFs) for ligand scaffold replacements of active molecules.137 A similarity search of curated scaffolds resulted in thrombin-derived scaffolds among the top solutions and docking of the entire molecules with replaced scaffolds showed feasible binding patterns. Griffith et al. used the UNITY-3D module of Sybyl to generate a ‘superligand’ from different crystal and NMR structures to facilitate the design of structurally diverse inhibitors that interact with residues of HIV1-RT (mutants) in a novel manner.138
3.3.2 Binding mode prediction using complex based SBP.
Schormann et al. used LigandScout to extract pharmacophores for 8 different co-crystallized DHFR inhibitors from the same chemical series.141 All pharmacophores contained 5 hydrophobic features, 2 donors and 1 negative ionizable feature. This pharmacophore was used together with docking to generate a ligand alignment and allow quantitative structure–activity relation (QSAR) modeling with HASL145 to predict affinity values for different inhibitors. Yoo et al. used the energy-optimized pharmacophore (e-pharmacophore)37,38 approach that is based on Glide XP energy terms to extract the most favorable sites of interaction from 14 docked inhibitors in human DNA methyltransferase 1 (hDNMT1) and derived an explanatory pharmacophore for hDNMT1 inhibitors.142
3.3.3 Binding mode prediction using overlay based SBP.
Zou et al. generated a SBP for CDK2 inhibition and reduced the number of features by selection of the top ranked 7 features as found in the 124 protein–ligand complexes.140 They showed that this most-frequent-feature pharmacophore encompasses previously reported ligand-based pharmacophore models. This pharmacophore was successfully used to discriminate CDK2 inhibitors from inactives and predict activities in retrospective virtual screening studies.140 Nguyen et al. constructed a homology model of the RSK2N-terminal kinase domain and optimized the models for different classes of active molecules, mimicking the ligand induced structural changes of the ATP-binding site of RSK2.144 A common pharmacophore was subsequently constructed from 5 consistently recurring protein–ligand interactions.144
3.4
Ligand binding site comparison
Many different methods to compare ligand binding sites based on pharmacophore or pharmacophore related properties have been developed in the past decade (for extensive reviews see Henrich et al.61 and Kellenberger et al.146). Most methods, like FuzCav,147 CavBase148 and PocketMatch,149 compare binding sites by assigning pharmacophore features directly to the protein. Campagna-Slater identified ligand binding sites that are chemically similar to known methyl-lysine binding domains using such SBP models.139 The KRIPO method, developed by Ritschel et al., uses protein–ligand interaction features derived from the ligand binding site to create 3D-pharmacophore fingerprints. This method has been successfully used to identify similar binding pockets and suggest structural modifications to ligands based on presumed bioisosteres.143 Sciabola et al. used FLAP to compare protein binding sites and were able to cluster kinase protein families in a relevant manner, predict ligand activity across related targets and perform protein–protein virtual screening.90 These methods are suitable for the identification of proteins which can be selectively targeted and compounds which might have activity on proteins with pharmacophorically similar binding sites.
4. Conclusion
The current review describes the different steps in the construction of SBPs: (i) protein structure preparation, (ii) binding site detection, (iii) pharmacophore feature definition, and (iv) pharmacophore feature selection. SBP generation typically starts with a protein preparation step to correct and optimize the starting structure. Subsequently the ligand binding pocket can be defined by a pocket detection algorithm and several strategies can be used to convert the protein properties to ligand. A choice of method can be made depending on the resolution of the available protein structure or model and the availability of known active ligands and corresponding binding mode hypotheses. Geometric methods to derive SBPs are typically the least restrictive and are together with the probe based method the only approaches which can be applied in the absence of known ligands. A real challenge in SBP design is the reduction of the typically high number of features in a structure based pharmacophore to only those features which are related to biological activity. Energy based methods to construct SBPs in these cases typically depend on the accuracy of the input structure and the binding mode hypothesis generated, while statistics based measures, such as those relying on the protein–ligand complex or protein variance information, are generally more robust with a greater capability to deal with low resolution or low quality structures. The relatively simple concept of a pharmacophore makes it an attractive tool for various research applications. Several studies have described the successful use of SBPs for binding mode hypotheses generation, virtual screening and binding site similarity calculations, demonstrating that SBPs are valuable tools for hit and lead optimization, compound library design and target hopping, especially in cases where ligand information is scarce.
Abbreviations
LBP
|
ligand based pharmacophore; |
SBP
| structure based pharmacophore; |
QSAR
| quantitative structure–activity relationship; |
SAR | structure–activity relationship; |
HBD
|
hydrogen bond donor; |
HBA |
hydrogen bond acceptor; |
MD | molecular dynamics; |
MIFs | molecular interaction fields; |
MCSS | multiple copy simultaneous search; |
XED
| extended election distribution; |
SNPs
|
single nucleotide polymorphisms; |
MSA
| multiple sequence alignment |
Acknowledgements
The authors would like to thank Tina Ritschel and Sander Nabuurs for critical reading of the manuscript. This work is supported by the Top Institute Pharma [project number D1.105: the GPCR Forum]. CdG is supported by The Netherlands Organization for Scientific Research (NWO) through VENI grant 700.59.408.
References
- P. Ehrlich, Ber. Dtsch. Chem. Ges., 1909, 42, 17–47 Search PubMed.
- L. B. Kier, Mol. Pharmacol., 1967, 3, 487–494 Search PubMed.
- L. B. Kier, Pure Appl. Chem., 1973, 35, 509–520 Search PubMed.
- P. Gund, Prog. Mol. Subcell. Biol., 1977, 11, 117–143 Search PubMed.
- T. Langer, Mol. Inf., 2010, 29, 470–475 Search PubMed.
- A. R. Leach, V. J. Gillet, R. A. Lewis and R. Taylor, J. Med. Chem., 2010, 53, 539–558 CrossRef CAS.
- H. Sun, Curr. Med. Chem., 2008, 15, 1018–1024 Search PubMed.
-
Success Stories of Computer-Aided Design, ed. H. Kubinyi, Wiley-Interscience, New York, 2006 Search PubMed.
- H. Eckert and J. Bajorath, Drug Discovery Today, 2007, 12, 225–233 CrossRef CAS.
- T. Langer, Mol. Inf., 2010, 29, 470–475 Search PubMed.
- F. C. Bernstein, T. F. Koetzle, G. J. Williams, E. F. Meyer, Jr, M. D. Brice, J. R. Rodgers, O. Kennard, T. Shimanouchi and M. Tasumi, J. Mol. Biol., 1977, 112, 535–542 CAS.
- D. Rognan, Mol. Inf., 2009, 29, 176–187 Search PubMed.
- D. Rognan, Br. J. Pharmacol., 2007, 152, 38–52 CrossRef CAS.
- S. Ekins, J. Mestres and B. Testa, Br. J. Pharmacol., 2007, 152, 9–20 CrossRef CAS.
- S. Ekins, J. Mestres and B. Testa, Br. J. Pharmacol., 2007, 152, 21–37 Search PubMed.
- J. Meslamani and D. Rognan, J. Chem. Inf. Model., 2011, 51, 1593–1603 Search PubMed.
- A. C. Pike, A. M. Brzozowski, J. Walton, R. E. Hubbard, T. Bonn, J. A. Gustafsson and M. Carlquist, Biochem. Soc. Trans., 2000, 28, 396–400 CrossRef CAS.
- A. C. Pike, A. M. Brzozowski and R. E. Hubbard, J. Steroid Biochem. Mol. Biol., 2000, 74, 261–268 CrossRef CAS.
- C. de Graaf and D. Rognan, J. Med. Chem., 2008, 51, 4978–4985 Search PubMed.
- D. Moras and H. Gronemeyer, Curr. Opin. Cell Biol., 1998, 10, 384–391 Search PubMed.
- A. Evers, G. Hessler, H. Matter and T. Klabunde, J. Med. Chem., 2005, 48, 5448–5465 CrossRef.
- F. Milletti and A. Vulpetti, J. Chem. Inf. Model., 2010, 50, 1418–1431 Search PubMed.
- V. J. Haupt and M. Schroeder, Briefings Bioinf., 2011, 12, 312–326 Search PubMed.
- C. N. Cavasotto and S. S. Phatak, Drug Discovery Today, 2009, 14, 676–683 Search PubMed.
- R. W. Hooft, C. Sander and G. Vriend, Proteins, 1996, 26, 363–376 Search PubMed.
- L. R. Forrest and B. Honig, Proteins, 2005, 61, 296–309 Search PubMed.
- R. P. Joosten, T. A. te Beek, E. Krieger, M. L. Hekkelman, R. W. Hooft, R. Schneider, C. Sander and G. Vriend, Nucleic Acids Res., 2011, 39, D411–419 Search PubMed.
- T. Klabunde, C. Giegerich and A. Evers, J. Med. Chem., 2009, 52, 2923–2932 Search PubMed.
- M. P. Sanders, S. Verhoeven, C. De Graaf, L. Roumen, B. Vroling, S. Nabuurs, J. D. Vlieg and J. P. Klomp, J. Chem. Inf. Model., 2011, 51, 2277–2292 Search PubMed.
-
L. B. Kier, Fundamental Concepts in Drug–Receptor Interactions, Academic Press, London, New York, 1970 Search PubMed.
-
L. B. Kier and L. H. Hall, Molecular Orbital Theory in Drug Research, Academic Press, New York, 1971 Search PubMed.
- E. Perola and P. S. Charifson, J. Med. Chem., 2004, 47, 2499–2510 CrossRef CAS.
- H. Alonso, A. A. Bliznyuk and J. E. Gready, Med. Res. Rev., 2006, 26, 531–568 CrossRef CAS.
- H. A. Carlson, Curr. Opin. Chem. Biol., 2002, 6, 447–452 Search PubMed.
- M. L. Teodoro and L. E. Kavraki, Curr. Pharm. Des., 2003, 9, 1635–1648 Search PubMed.
- K. L. Meagher and H. A. Carlson, J. Am. Chem. Soc., 2004, 126, 13276–13281 Search PubMed.
- K. Loving, N. K. Salam and W. Sherman, J. Comput.-Aided Mol. Des., 2009, 23, 541–554 Search PubMed.
- N. K. Salam, R. Nuti and W. Sherman, J. Chem. Inf. Model., 2009, 49, 2356–2368 Search PubMed.
- S. L. Dixon, A. M. Smondyrev, E. H. Knoll, S. N. Rao, D. E. Shaw and R. A. Friesner, J. Comput.-Aided Mol. Des., 2006, 20, 647–671 Search PubMed.
- P. J. Goodford, J. Med. Chem., 1985, 28, 849–857 CrossRef CAS.
- H. J. Bohm, J. Comput.-Aided Mol. Des., 1994, 8, 623–632 CrossRef CAS.
- H. J. Bohm, J. Comput.-Aided Mol. Des., 1992, 6, 61–78 CAS.
- S. C. Lovell, J. M. Word, J. S. Richardson and D. C. Richardson, Proteins, 2000, 40, 389–408 CrossRef CAS.
- P. Schmidtke, C. Souaille, F. Estienne, N. Baurin and R. T. Kroemer, J. Chem. Inf. Model., 2010, 50, 2191–2200 Search PubMed.
- J. An, M. Totrov and R. Abagyan, Mol. Cell. Proteomics, 2005, 4, 752–761 CrossRef CAS.
- M. L. Verdonk, J. C. Cole and R. Taylor, J. Mol. Biol., 1999, 289, 1093–1108 CrossRef CAS.
- H. A. Carlson, K. M. Masukawa, K. Rubins, F. D. Bushman, W. L. Jorgensen, R. D. Lins, J. M. Briggs and J. A. McCammon, J. Med. Chem., 2000, 43, 2100–2114 Search PubMed.
- T. Halgren, Chem. Biol. Drug Des., 2007, 69, 146–148 Search PubMed.
- Tripos, http://tripos.com/, 2011.
- Accelrys, http://accelrys.com/products/discovery-studio/, 2011.
- B. Delaunay, Izv. Akad. Nauk SSSR, Otd. Mat. Estestv. Nauk, 1934, 7, 793–800 Search PubMed.
- C. C. Group, http://www.chemcomp.com/software.htm, 2011.
- G. P. Brady, Jr and P. F. Stouten, J. Comput.-Aided Mol. Des., 2000, 14, 383–401 Search PubMed.
- R. A. Laskowski, J. Mol. Graphics, 1995, 13, 323–330 CrossRef CAS , 307–328.
- M. Hendlich, F. Rippmann and G. Barnickel, J. Mol. Graphics Modell., 1997, 15, 359–363 CrossRef CAS , 389.
- K. P. Peters, J. Fauck and C. Frommel, J. Mol. Biol., 1996, 256, 201–213 Search PubMed.
- J. Liang, H. Edelsbrunner and C. Woodward, Protein Sci., 1998, 7, 1884–1897 Search PubMed.
- T. A. Binkowski, S. Naghibzadeh and J. Liang, Nucleic Acids Res., 2003, 31, 3352–3355 Search PubMed.
- H. Edelsbrunner and L. Mucke, ACM Trans. Graphics, 1994, 13, 43–72 Search PubMed.
- K. Prymula, T. Jadczyk and I. Roterman, J. Comput.-Aided Mol. Des., 2011, 25, 117–133 Search PubMed.
- S. Henrich, O. M. Salo-Ahen, B. Huang, F. F. Rippmann, G. Cruciani and R. C. Wade, J. Mol. Recognit., 2010, 23, 209–219 Search PubMed.
- G. Wolber, T. Seidel, F. Bendix and T. Langer, Drug Discovery Today, 2008, 13, 23–29 CrossRef CAS.
- G. M. Spitzer, M. Heiss, M. Mangold, P. Markt, J. Kirchmair, G. Wolber and K. R. Liedl, J. Chem. Inf. Model., 2010, 50, 1241–1247 Search PubMed.
- C. Lemmen and T. Lengauer, J. Comput.-Aided Mol. Des., 2000, 14, 215–232 CrossRef CAS.
- J. M. Word, S. C. Lovell, J. S. Richardson and D. C. Richardson, J. Mol. Biol., 1999, 285, 1735–1747 CrossRef CAS.
- M. Baroni, G. Cruciani, S. Sciabola, F. Perruccio and J. S. Mason, J. Chem. Inf. Model., 2007, 47, 279–294 Search PubMed.
- S. Cross, M. Baroni, E. Carosati, P. Benedetti and S. Clementi, J. Chem. Inf. Model., 2010, 50, 1442–1450 Search PubMed.
- F. Ortuso, T. Langer and S. Alcaro, Bioinformatics, 2006, 22, 1449–1455 Search PubMed.
- C. Barillari, G. Marcou and D. Rognan, J. Chem. Inf. Model., 2008, 48, 1396–1410 Search PubMed.
- Inteligand, http://www.inteligand.com/ligandscout/, 2011.
- J. Chen and L. Lai, J. Chem. Inf. Model., 2006, 46, 2684–2691 Search PubMed.
- Schrodinger, http://www.schrodinger.com/, 2011.
- Z. Chen, G. Tian, Z. Wang, H. Jiang and J. Shen, J. Chem. Inf. Model., 2010, 50, 615–625 Search PubMed.
- A. Al-Nadaf, G. Abu Sheikha and M. O. Taha, Bioorg. Med. Chem., 2010, 18, 3088–3115 Search PubMed.
- M. O. Taha, A. G. Al-Bakri and W. A. Zalloum, Bioorg. Med. Chem. Lett., 2006, 16, 5902–5906 CrossRef CAS.
- M. O. Taha, Y. Bustanji, A. G. Al-Bakri, A. M. Yousef, W. A. Zalloum, I. M. Al-Masri and N. Atallah, J. Mol. Graphics Modell., 2007, 25, 870–884 Search PubMed.
- M. O. Taha, Y. Bustanji, M. A. Al-Ghussein, M. Mohammad, H. Zalloum, I. M. Al-Masri and N. Atallah, J. Med. Chem., 2008, 51, 2062–2077 CrossRef CAS.
- M. O. Taha, L. A. Dahabiyeh, Y. Bustanji, H. Zalloum and S. Saleh, J. Med. Chem., 2008, 51, 6478–6494 Search PubMed.
- M. O. Taha, M. Tarairah, H. Zalloum and G. Abu-Sheikha, J. Mol. Graphics Modell., 2010, 28, 383–400 Search PubMed.
- I. Wallach and R. Lilien, J. Chem. Inf. Model., 2009, 49, 2116–2128 Search PubMed.
- A. Miranker and M. Karplus, Proteins, 1991, 11, 29–34 CAS.
- T. T. Luu, N. O. Malcolm and K. Nadassy, Comb. Chem. High Throughput Screening, 2011, 14, 488–489 Search PubMed.
- I. Wallach, Drug Dev. Res., 2011, 72, 17–25 Search PubMed.
- T. Cheeseright, M. Mackey, S. Rose and A. Vinter, Expert Opin. Drug Discovery, 2007, 2, 131–144 Search PubMed.
- M. J. McGregor, J. Chem. Inf. Model., 2007, 47, 2374–2382 Search PubMed.
- Z. Deng, C. Chuaqui and J. Singh, J. Med. Chem., 2004, 47, 337–344 CrossRef CAS.
- S. Renner and G. Schneider, J. Med. Chem., 2004, 47, 4653–4664 CrossRef CAS.
- A. Nicholls, G. B. McGaughey, R. P. Sheridan, A. C. Good, G. Warren, M. Mathieu, S. W. Muchmore, S. P. Brown, J. A. Grant, J. A. Haigh, N. Nevins, A. N. Jain and B. Kelley, J. Med. Chem., 2010, 53, 3862–3886 CrossRef CAS.
- P. A. Greenidge, B. Carlsson, L. G. Bladh and M. Gillner, J. Med. Chem., 1998, 41, 2503–2512 Search PubMed.
- S. Sciabola, R. V. Stanton, J. E. Mills, M. M. Flocco, M. Baroni, G. Cruciani, F. Perruccio and J. S. Mason, J. Chem. Inf. Model., 2010, 50, 155–169 Search PubMed.
- M. Rella, C. A. Rushworth, J. L. Guy, A. J. Turner, T. Langer and R. M. Jackson, J. Chem. Inf. Model., 2006, 46, 708–716 Search PubMed.
- J. O. Ebalunode, Z. Ouyang, J. Liang and W. Zheng, J. Chem. Inf. Model., 2008, 48, 889–901 Search PubMed.
- P. C. Hawkins, A. G. Skillman and A. Nicholls, J. Med. Chem., 2007, 50, 74–82 CrossRef CAS.
- A. Nicholls, G. B. McGaughey, R. P. Sheridan, A. C. Good, G. Warren, M. Mathieu, S. W. Muchmore, S. P. Brown, J. A. Grant, J. A. Haigh, N. Nevins, A. N. Jain and B. Kelley, J. Med. Chem., 2010, 53, 3862–3886 CrossRef CAS.
- S. Thangapandian, S. John, S. Sakkiah and K. W. Lee, Eur. J. Med. Chem., 2010, 45, 4409–4417 Search PubMed.
- B. V. Kumar, R. Kotla, R. Buddiga, J. Roy, S. S. Singh, R. Gundla, M. Ravikumar and J. A. Sarma, J. Mol. Model., 2011, 17, 151–163 Search PubMed.
- R. Griffith, T. T. Luu, J. Garner and P. A. Keller, J. Mol. Graphics Modell., 2005, 23, 439–446 Search PubMed.
- L. Tan, H. Geppert, M. T. Sisay, M. Gutschow and J. Bajorath, ChemMedChem, 2008, 3, 1566–1571 Search PubMed.
- D. M. Kruger and A. Evers, ChemMedChem, 2010, 5, 148–158 CrossRef.
- A. Evers, G. Hessler, H. Matter and T. Klabunde, J. Med. Chem., 2005, 48, 5448–5465 CrossRef.
- M. von Korff, J. Freyss and T. Sander, J. Chem. Inf. Model., 2009, 49, 209–231 Search PubMed.
- N. Singh, G. Cheve, D. M. Ferguson and C. R. McCurdy, J. Comput.-Aided Mol. Des., 2006, 20, 471–493 Search PubMed.
- J. Kirchmair, S. Distinto, P. Markt, D. Schuster, G. M. Spitzer, K. R. Liedl and G. Wolber, J. Chem. Inf. Model., 2009, 49, 678–692 Search PubMed.
- A. J. Kooistra, T. W. Binsl, J. H. van Beek, C. de Graaf and J. Heringa, J. Chem. Inf. Model., 2010, 50, 1772–1780 Search PubMed.
- N. Moitessier, P. Englebienne, D. Lee, J. Lawandi and C. R. Corbeil, Br. J. Pharmacol., 2008, 153(Suppl. 1), S7–26 CrossRef CAS.
- O. Guner, O. Clement and Y. Kurogi, Curr. Med. Chem., 2004, 11, 2991–3005 Search PubMed.
- O. F. Guner, Curr. Comput.-Aided Drug Des., 2011, 7, 158 Search PubMed.
- K. Roy, Mol. Diversity, 2004, 8, 321–323 Search PubMed.
- C. Lemmen, T. Lengauer and G. Klebe, J. Med. Chem., 1998, 41, 4502–4520 CrossRef CAS.
- I. Halperin, B. Ma, H. Wolfson and R. Nussinov, Proteins, 2002, 47, 409–443 CrossRef CAS.
- D. B. Kitchen, H. Decornez, J. R. Furr and J. Bajorath, Nat. Rev. Drug Discovery, 2004, 3, 935–949 CrossRef CAS.
- C. Sotriffer and G. Klebe, Farmaco, 2002, 57, 243–251 Search PubMed.
- R. A. Friesner, J. L. Banks, R. B. Murphy, T. A. Halgren, J. J. Klicic, D. T. Mainz, M. P. Repasky, E. H. Knoll, M. Shelley, J. K. Perry, D. E. Shaw, P. Francis and P. S. Shenkin, J. Med. Chem., 2004, 47, 1739–1749 CrossRef CAS.
- G. Jones, P. Willett, R. C. Glen, A. R. Leach and R. Taylor, J. Mol. Biol., 1997, 267, 727–748 CrossRef CAS.
- A. N. Jain, J. Med. Chem., 2003, 46, 499–511 CrossRef CAS.
- B. Kramer, M. Rarey and T. Lengauer, Proteins, 1999, 37, 228–241 CrossRef CAS.
- T. S. Rush, 3rd, J. A. Grant, L. Mosyak and A. Nicholls, J. Med. Chem., 2005, 48, 1489–1495 CrossRef.
- G. Hessler, M. Zimmermann, H. Matter, A. Evers, T. Naumann, T. Lengauer and M. Rarey, J. Med. Chem., 2005, 48, 6575–6584 Search PubMed.
- C. Tintori, V. Corradi, M. Magnani, F. Manetti and M. Botta, J. Chem. Inf. Model., 2008, 48, 2166–2179 Search PubMed.
- M. Lower, T. Geppert, P. Schneider, B. Hoy, S. Wessler and G. Schneider, PLoS One, 2011, 6, e17986 Search PubMed.
- G. I. Mustata and J. M. Briggs, J. Comput.-Aided Mol. Des., 2002, 16, 935–953 Search PubMed.
- X. M. Chen, T. Lu, S. Lu, H. F. Li, H. L. Yuan, T. Ran, H. C. Liu and Y. D. Chen, J. Mol. Model., 2010, 16, 1195–1204 Search PubMed.
- A. Evers and G. Klebe, J. Med. Chem., 2004, 47, 5381–5392 Search PubMed.
- B. Pirard, J. Brendel and S. Peukert, J. Chem. Inf. Model., 2005, 45, 477–485 Search PubMed.
- S. Cross and G. Cruciani, Drug Discovery Today, 2010, 7, 213–219 Search PubMed.
- M. Weisel, E. Proschak and G. Schneider, Chem. Cent. J., 2007, 1, 7 Search PubMed.
- M. Weisel, E. Proschak, J. M. Kriegl and G. Schneider, Proteomics, 2009, 9, 451–459 Search PubMed.
- C. Bissantz, B. Kuhn and M. Stahl, J. Med. Chem., 2010, 53, 5061–5084 CrossRef CAS.
- Y. Tanrikulu, M. Nietert, U. Scheffer, E. Proschak, K. Grabowski, P. Schneider, M. Weidlich, M. Karas, M. Gobel and G. Schneider, ChemBioChem, 2007, 8, 1932–1936 Search PubMed.
- R. Wang, Y. Gao and L. Lai, J. Mol. Model., 2000, 6, 498–516 CrossRef CAS.
- A. Evers, H. Gohlke and G. Klebe, J. Mol. Biol., 2003, 334, 327–345 Search PubMed.
- H. Claussen, M. Gastreich, V. Apelt, J. Greene, S. A. Hindle and C. Lemmen, Curr. Drug Discovery Technol., 2004, 1, 49–60 Search PubMed.
- S. A. Hindle, M. Rarey, C. Buning and T. Lengaue, J. Comput.-Aided Mol. Des., 2002, 16, 129–149 Search PubMed.
- D. Joseph-McCarthy, B. E. T. Thomas, M. Belmarsh, D. Moustakas and J. C. Alvarez, Proteins, 2003, 51, 172–188 Search PubMed.
- A. Kaczor and D. Matosiuk, FEMS Immunol. Med. Microbiol., 2010, 58, 91–101 Search PubMed.
- S. Thangapandian, S. John, S. Sakkiah and K. W. Lee, Eur. J. Med. Chem., 2011, 46, 2469–2476 Search PubMed.
- M. M. Ahlstrom, M. Ridderstrom, K. Luthman and I. Zamora, J. Chem. Inf. Model., 2005, 45, 1313–1323 Search PubMed.
- R. Griffith, T. T. Luu, J. Garner and P. A. Keller, J. Mol. Graphics Modell., 2005, 23, 439–446 Search PubMed.
- V. Campagna-Slater, A. G. Arrowsmith, Y. Zhao and M. Schapira, J. Chem. Inf. Model., 2010, 50, 358–367 Search PubMed.
- J. Zou, H. Z. Xie, S. Y. Yang, J. J. Chen, J. X. Ren and Y. Q. Wei, J. Mol. Graphics Modell., 2008, 27, 430–438 Search PubMed.
- N. Schormann, O. Senkovich, K. Walker, D. L. Wright, A. C. Anderson, A. Rosowsky, S. Ananthan, B. Shinkre, S. Velu and D. Chattopadhyay, Proteins, 2008, 73, 889–901 Search PubMed.
- J. Yoo and J. L. Medina-Franco, J. Comput.-Aided Mol. Des., 2011, 25, 555–567 Search PubMed.
- T. Ritschel, D. J. Wood, J. de Vlieg and M. Wagener, J. Cheminf., 2011, 3, 37 Search PubMed.
- T. L. Nguyen, R. Gussio, J. A. Smith, D. A. Lannigan, S. M. Hecht, D. A. Scudiero, R. H. Shoemaker and D. W. Zaharevitz, Bioorg. Med. Chem., 2006, 14, 6097–6105 Search PubMed.
- A. M. Doweyko, J. Med. Chem., 1994, 37, 1769–1778 Search PubMed.
- E. Kellenberger, C. Schalon and D. Rognan, Curr. Comput.-Aided Drug Des., 2008, 4, 209–220 Search PubMed.
- N. Weill and D. Rognan, J. Chem. Inf. Model., 2010, 50, 123–135 Search PubMed.
- S. Schmitt, D. Kuhn and G. Klebe, J. Mol. Biol., 2002, 323, 387–406 Search PubMed.
- K. Yeturu and N. Chandra, BMC Bioinf., 2008, 9, 543 Search PubMed.
|
This journal is © The Royal Society of Chemistry 2012 |
Click here to see how this site uses Cookies. View our privacy policy here.