DOI:
10.1039/C1MB05246B
(Paper)
Mol. BioSyst., 2012,
8, 296-307
Is there a biological cost of protein disorder? Analysis of cancer-associated mutations†‡
Received
17th June 2011
, Accepted 21st August 2011
First published on 14th September 2011
Abstract
As many diseases can be traced back to altered protein function, studying the effect of genetic variations at the level of proteins can provide a clue to understand how changes at the DNA level lead to various diseases. Cellular processes rely not only on proteins with well-defined structure but can also involve intrinsically disordered proteins (IDPs) that exist as highly flexible ensembles of conformations. Disordered proteins are mostly involved in signaling and regulatory processes, and their functional repertoire largely complements that of globular proteins. However, it was also suggested that protein disorder entails an increased biological cost. This notion was supported by a set of individual IDPs involved in various diseases, especially in cancer, and the increased amount of disorder observed among disease-associated proteins. In this work, we tested if there is any biological risk associated with protein disorder at the level of single nucleotide mutations. Specifically, we analyzed the distribution of mutations within ordered and disordered segments. Our results demonstrated that while neutral polymorphisms were more likely to occur within disordered segments, cancer-associated mutations had a preference for ordered regions. Additionally, we proposed an alternative explanation for the association of protein disorder and the involvement in cancer with the consideration of functional annotations. Individual examples also suggested that although disordered segments are fundamental functional elements, their presence is not necessarily accompanied with an increased mutation rate in cancer. The presented study can help to understand how the different structural properties of proteins influence the consequences of genetic mutations.
Introduction
For several decades, molecular biology studies largely concentrated on globular proteins, based on the assumption that a well-defined structure is necessary for the proper function of proteins, and the loss of structure leads to the loss of function. In exploring the genetic background of various diseases similar biases were also present, by focusing on mutations that could be placed into structural context. With the increase of available genome sequences, it has become evident that a large number of naturally occurring proteins do not require a well-folded structure to fulfill their biological role.1–3 These intrinsically unstructured/disordered proteins (IUPs/IDPs) exist as highly flexible ensembles of rapidly interconverting conformations, even under physiological conditions.1–3 IDPs are surprisingly common, especially in higher eukaryotes,4,5 and are involved in many vital cellular functions. These include regulation, transcription and translation, signal transduction, protein phosphorylation, storage of small molecules, chaperone action, transport, and assembly of large multiprotein complexes.6 The increased flexibility of these proteins is pertinent for their specific functions and offers several functional advantages. IDPs provide a larger interaction surface area than globular proteins of similar length.7,8 They generally interact with their partners with relatively high specificity and low affinity and can bind to multiple partners.9,10 The plasticity of these proteins also enables them to adapt to the surface of their partners.11 They are often subject to various post-translational modifications that facilitate the regulation of their function in the cell.12,13 Consequently, disordered proteins can capture and integrate various signals in a complex way through their disordered segments and participate in a large number of interactions.13 These properties explain their prevalence in signaling and regulatory functions,5,14 as well as serving as hubs of interaction networks.15,16
Given the functional importance of disordered protein regions, their malfunction is expected to have serious biological consequences. IDPs indeed are often associated with various diseases, especially with cancer.17 This observation is supported by the list of IDPs, such as BRCA1, p27, p21 and CBP, that are involved in various forms of cancer. One of best characterized disordered proteins, p53, is directly inactivated in more than 50% of cancers.18 At a more general level, the higher proportion of disordered proteins among cancer associated proteins was also observed. According to the analysis of the SwissProt database, 79% of human cancer associated proteins have been classified as IDPs, compared to 47% of all eukaryotic proteins.19 The correlation between protein disorder and cancer was further underscored in the case of two common forms of generic alterations, chromosomal rearrangements20 and copy number variations.21 In addition to cancer, disordered proteins were also suggested to be common in diabetes and cardiovascular diseases.17,22 Several disordered proteins—such as Aβ, τ, α synuclein, and prion proteins—are involved in neurodegenerative diseases and are also prone to amyloid formation.23,24 Altogether, these results lead to the conclusion that protein disorder comes with a “biological cost” that is reflected in an increased risk of cancer and other diseases.2,17 This calls for the understanding of the role of protein disorder in various diseases.
Large scale sequencing efforts now enable us to explore the relationship between protein disorder and disease-causing genetic mutations at a more detailed level. The completion of the Human Genome Project is being followed by concerted efforts to categorize commonly occurring sequence alterations.25,26 As a result, the dbSNP database already contains more than 13 million sequence variations. Recently, dbSNP started to include personal genomics data by incorporating the results from the pilot study of 1000 Genomes Project.27 The rapid accumulation of DNA variation data enabled the evaluation of evolutionary constraints at the level of single nucleotide polymorphisms (SNPs).28 Furthermore, advances in sequencing technologies also opened new ways to explore how genetic changes lead to diseases. Before the Human Genome Project, the identification of potential cancer-causing genes often relied on prior assumptions about the approximate location of mutated regions in the genome or some information about their biological function.29 Consequently, traditional approaches could indirectly favor better-characterized ordered proteins and introduce a bias against disordered segments. Cancer genome projects can decipher the genetic background of cancer without such biases by directly analyzing the differences between cancer and normal cells at the DNA level.30 From the currently available studies of breast, colorectal,31,32 pancreatic cancers33 and glioblastoma,34 an unexpectedly complex landscape of cancer emerged. According to this, cancer is a result of the accumulation of a relatively large number of mutations each of which carries a small fitness advantage towards tumor progression. While there are a few frequently occurring mutations, the distribution of mutations is dominated by a much larger number of infrequently mutated genes.32
With the rapid explosion of data on sequence variations and the expanding catalogue of cancer-associated mutations, we can have a fresh look on how the structural properties of proteins determine the distribution of neutral and cancer-associated mutations. In this work we tested the hypothesis regarding the biological cost of protein disorder in terms of single point mutations. We considered cancer-associated proteins identified by traditional biochemical essays as well as by the various cancer genome projects. Using these datasets, the distributions of commonly occurring polymorphisms and cancer-associated mutations within ordered and disordered regions of proteins were investigated. A functionally relevant subclass of disordered segments corresponding to disordered binding regions was also studied in a similar manner. In order to explore indirect relationships between cancer and the structural state of proteins, we also considered functional categories of cancer-associated proteins. A closer look at interesting examples can give further insights into the role of protein disorder in cancer-associated proteins.
Results
We have compiled 12 datasets of cancer-associated proteins from various resources (see Data and methods and Table 1). The datasets differed in their size and the primary way the specific proteins were identified. It is worth noting that in this study, genetic variations were restricted to single amino acid substitutions, therefore proteins that were associated with cancer viachromosomal translocations or copy number variations were not considered.
Table 1 The number of proteins and residues for the 12 cancer-associated mutation databases and the polymorphism database. The number of mutations and/or polymorphisms are also shown where applicable
Datasets |
Number of |
Proteins
|
Residues |
Mutations |
Polymorphisms |
SP_cancer |
1403 |
1 250 776 |
5246 |
— |
SP_cancer_annotated |
113 |
91 683 |
1555 |
— |
SP_poly |
11 510 |
7 776 050 |
— |
36 583 |
CGP_br/col_1 |
924 |
795 543 |
1239 |
3536 |
CGP_br/col_2 |
1335 |
1 332 469 |
1739 |
6098 |
CGP_pan |
711 |
769 634 |
790 |
3848 |
CGP_glio |
1089 |
1 074 168 |
1195 |
5794 |
CGP_CAN_br/col_1 |
174 |
203 731 |
395 |
908 |
CGP_CAN_br/col_2 |
243 |
298 114 |
513 |
1372 |
CGP_CAN_pan |
64 |
72 317 |
130 |
289 |
CGP_CAN_glio |
36 |
43 031 |
77 |
210 |
COSMIC |
8957 |
6 898 559 |
22 708 |
26 435 |
COSMIC_census |
261 |
238 130 |
5375 |
673 |
The first type of dataset was collected from the SwissProt database,35 primarily from literature searches (SP_cancer). A subset of this dataset with specific annotation in the OMIM database was also considered (SP_cancer_annotated). These two datasets, especially the annotated subset, are expected to be dominated by the cancer mutations identified in more traditional ways. The second type of datasets was compiled from four cancer genome projects. Two of these corresponded to breast and colorectal cancers (CGP_br/col_1 and CGP_br/col_2),31,32 one to pancreatic cancer (CGP_pan)33 and another one to glioblastoma (CGP_glio).34 In each case, a subset of genes were selected that were more likely to contain driver mutations. These mutations are expected to actively contribute to the tumorigenesis as opposed to passenger mutations which occur purely by chance. These CAN sets were also analyzed separately (CGP_CAN). The largest dataset was compiled from the COSMIC database (COSMIC).36 It included cancer mutation data collected both from the literature and the outcomes of large-scale cancer genome projects. An additional dataset corresponded to a more restricted subset of proteins in COSMIC that were part of cancer census genes.37 These proteins could be casually linked to oncogenesis (COSMIC_census). The number of proteins, amino acids and mutations in each dataset are given in Table 1.
Protein disorder in cancer-associated proteins
We evaluated the disorder content in our datasets to confirm that protein disorder is common in human cancer-associated proteins.19 The length and average disorder content were analyzed in these datasets. As a reference, we used the complete human proteome downloaded from the SwissProt database.38 The disorder content was calculated using the IUPred disorder prediction method.39,40 The results were confirmed with two other popular disorder prediction methods, DISOPRED25 and VSL2.41
Fig. 1 shows the disorder content and the percentage of proteins with disordered regions over 30 residues, as well as the average length of proteins in the various datasets as compared to the average values of the human proteome obtained with IUPred. In contrast to earlier results,19 the percentage of disordered residues in these datasets was not significantly different compared to the background (Fig. 1 and Table S1 (ESI‡)). Significant differences were only observed in the case of two breast–colorectal datasets (CGP_br/col_2 and CGP_CAN_br/col_2) and the COSMIC census dataset. In the case of SP_cancer_annotated data, the disorder content actually decreased compared to the average disorder content in the human proteome, although this difference was not statistically significant. These results did not depend on the choice of the disorder prediction software, as DISOPRED2 and VSL2, two other fundamentally different methods produced remarkably similar outputs (see Fig. S1, ESI‡). It should be noted that Iakoucheva et al.19 compared the disorder content of cancer proteins to those of all eukaryotic proteins in the SwissProt database. This could explain why the differences in their work were much larger compared to our work.
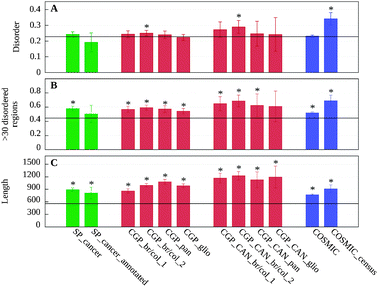 |
| Fig. 1 Average ratio of disordered residues (A), ratio of proteins containing >30 residue long disordered regions (B) and length (C) in the 12 datasets analyzed. Black horizontal lines represent the average values obtained for the proteins of the human proteome taken from SwissProt. Flags show the confidence interval of α = 0.01 calculated from the standard error of the mean of randomly selected samples from the human proteome (see Data and methods). Significant differences are marked with asterisks (see Table S1, ESI‡). | |
There was, however, a significant increase in the proportion of proteins containing long disordered segments among cancer-associated proteins compared to the human proteome. With the exception of SP_cancer_annotated and the CGP_CAN_glio datasets, all differences were significant. The results calculated with IUPred (Fig. 1B) were again confirmed by the two other prediction methods (Fig. S1, ESI‡). In agreement with earlier results,42 cancer-associated proteins were also significantly longer. The increase in length and in fraction of proteins with long disordered segments points to the increased modularity and complexity of cancer-associated proteins.
Polymorphisms in ordered and disordered regions
The rates of evolution are largely governed by the stringency of functional and structural constraints. As ordered and disordered segments in proteins have distinct properties in this regard, these characteristic differences are expected to be reflected in the distribution of genetic variations in these regions. To test this assumption, we analyzed the differences in the distribution of SNPs within disordered and ordered segments of cancer-associated proteins. Polymorphism data were collected from the SwissProt resource and the dbSNP database (release 132). On average, we observed around five polymorphisms per thousand amino acid positions, although this number varied slightly among the various datasets (see Table 1).
For each protein in our datasets, we tallied the number of observed polymorphisms in ordered and disordered segments. These numbers were compared to the expected number of polymorphisms based on the assumption that the mutations are distributed evenly in the sequence. The results presented in Fig. 2A show the relative difference between the observed and expected number of polymorphisms within both disordered and ordered segments predicted with IUPred. A more detailed account of the results for each dataset including the p-values showing the statistical significance is presented in Table S2 (ESI‡). The results indicate that a significantly larger number of polymorphisms fell within disordered segments compared to ordered regions. The enrichments ranged from 7.7% (CGP_CAN_pan) to 45.9% (CGP_CAN_glio) in the various datasets, with an average of 15.0% (see Table S2, ESI‡). With the exception of some of the CAN gene sets, the differences were statistically significant in all datasets and largely agreed for all three disorder prediction methods (see also Fig. S2A and S3A, ESI‡). These data indicate that in cancer-associated proteins, disordered regions generally are more tolerant to mutations compared to ordered proteins. This trend is in agreement with the lower evolutionary conservation of disordered proteins, observed at various levels.28,43–45
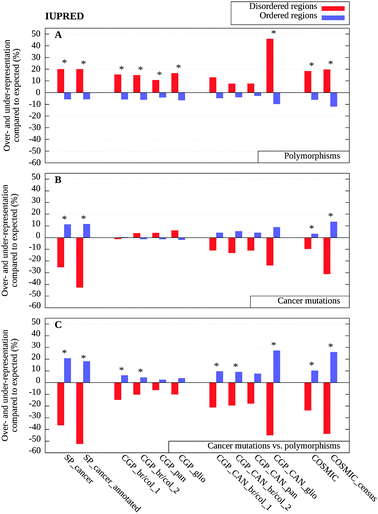 |
| Fig. 2 Over- and under-representation of mutations in disordered (red) and ordered regions (blue) calculated with IUPred, as compared to background distributions (see Data and methods). (A) The distribution of polymorphisms as compared to the uniform random distribution; (B) the distribution of cancer-associated mutations as compared to the uniform random distribution and (C) the distribution of cancer-associated mutations as compared to the expected values weighted by the distribution of polymorphisms shown in (A). Significant differences are marked with asterisks (see Table S2, ESI‡). | |
Cancer-associated mutations
As a next step we investigated if there is any preference of cancer-associated mutations towards order or disorder in proteins. The cancer-associated mutations collected from various sources were projected onto positions in the protein sequence, and the order/disorder status of the corresponding residues was determined by the IUPred disorder prediction algorithm. Similarly to polymorphisms, the observed number of mutations within ordered and disordered segments was compared to the expected number of mutations based on the assumption that the mutations are distributed evenly in the sequence.
Compared to polymorphisms, cancer-associated mutations followed a reversed trend and were more likely to appear within ordered regions (Fig. 2B). This tendency was strongest in the SwissProt datasets, but was also present in the four CGP_CAN, as well for the complete COSMIC dataset and its subset of cancer census proteins. The SwissProt and COSMIC datasets produced statistically significant differences (see Table S2, ESI‡). Results obtained with IUPred again were in agreement with results of the two other disordered prediction methods (Fig. S2 and S3, ESI‡). The complete dataset of cancer genomes showed a slightly different trend. In these cases, cancer-associated mutations were slightly tilted towards disordered segments. The weak preference of these sequence variations for disordered segments can be due to the higher number of randomly occurring passenger mutations present in these datasets. Indeed, the normalization which takes into account the uneven distribution of polymorphisms, compensated for this behavior. As a result, the underrepresentation of cancer-associated mutations within disordered regions became even more apparent and unequivocal within all datasets analyzed (Fig. 2C). The normalization also increased the statistical significance of the results (Table S2, ESI‡). The reversed trend was statistically significant in the manually curated datasets (SP_cancer, SP_cancer_annotated, COSMIC, and COSMIC_census). Some of the cancer genomes project also produced significant differences after the normalization, despite the increased noise present in these datasets due to the higher content of passenger mutations. Altogether, these results clearly contradicted the original hypothesis about the increased risk of cancer associated with protein disorder, at least in terms of single nucleotide mutations.
Disordered binding regions
As disordered proteins are quite heterogeneous both in terms of their structural and functional properties, deviations from the general behavior can occur in certain cases. We specifically analyzed predicted disordered binding regions that are expected to be enriched in functionally relevant sites. Disordered proteins often function via binding to other macromolecules that involves a disorder-to-order transition.2,10 Although binding to other macromolecules can induce a transition to a fully or at least partially ordered structure in the case of many IDPs, their complexes have distinct properties compared to complexes formed by ordered proteins.46,47 The actual binding regions often correspond to short, localized elements in the sequence and have unique sequence properties compared to both ordered and disordered segments in general.48 Using a sequence based prediction method, called ANCHOR,48,49 we examined the distribution of polymorphisms and cancer-associated mutations within disordered binding regions.
Based on the predictions, a distinct group was formed from the residues of disordered binding regions. Residues not predicted as disordered binding sites were divided into two separate groups depending on whether they were predicted as disordered or as ordered. Disordered binding residues are usually part of a disordered segment, however, in some cases they can also correspond to local dips in the prediction profile in which case they are predicted as ordered.50 Therefore, both disordered and ordered datasets contained fewer residues compared to the previous analysis. The results for the three groups are presented in Fig. 3A–C and Table S3 (ESI‡). There are significant differences among the three sets in the distributions of observed SNPs (Fig. 3A), with the exception of the small CAN gene sets. While SNPs were clearly overrepresented in disordered segments and underrepresented in ordered regions, disordered binding regions fell between these two categories, but their behavior was still closer to disordered segments. One of the CAN gene sets (pancreatic cancer) differed slightly from this trend, in this case more SNPs were observed in disordered binding regions than in disordered segments in general.
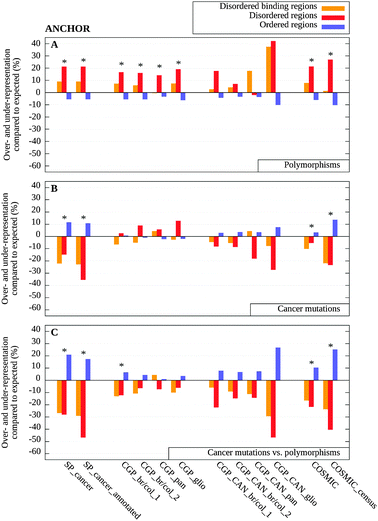 |
| Fig. 3 Over- and under-representation of mutations in disordered binding regions (orange), disordered (red) and ordered regions (blue) calculated with ANCHOR, as compared to background distributions (see Data and methods). (A) The distribution of polymorphisms as compared to the uniform random distribution; (B) the distribution of cancer-associated mutations as compared to the uniform random distribution and (C) the distribution of cancer-associated mutations as compared to the expected values weighted by the distribution of polymorphisms shown in (A). Significant differences are marked with asterisks (see Table S3, ESI‡). | |
The distribution of cancer-associated mutations within disordered binding regions was largely similar to that of disordered regions in general, with some differences in the case of the cancer genome datasets (Fig. 3B). These deviations can also be attributed to the increased number of passenger mutations within disordered segments and disappeared when the uneven distribution of polymorphisms was taken into account. In this normalized data, disordered binding regions had a smaller depletion of cancer-associated mutations in most cases compared to disordered regions in general (Fig. 3C). This behavior was expected for regions with increased functional importance.
Functional correlations
We also analyzed cancer-associated proteins in terms of their functional categories and their number of protein–protein interactions. First, we assessed which functional groups were overrepresented within cancer-associated proteins. For this analysis, the GeneOntology functional categories were used (see Data and methods). The occurrence of each of the considered 50 biological processes and 41 molecular functions in the COSMIC_census dataset was compared to the expected occurrence of these functions in the human proteome. The list of biological processes and molecular functions that exhibited statistically significant differences is shown in Table 2. The significantly enriched processes among cancer-associated proteins included signal transduction, involvement in cell-cycle and proliferation, DNA- and protein binding, phosphorylation and regulation of transcription. These proteins on the other hand were significantly depleted in transport processes in general and particularly in ion transport. In other cases, the differences were not significant at the α = 0.01 level. In general, our results are in complete agreement with an earlier study,42 and correlate well with the functional enrichments of disordered proteins.5,14
Table 2 List of GO biological processes and molecular functions that are significantly over- or under-represented in the COSMIC census database as compared to the human proteome. p-values were obtained using the exact Fisher test (see Data and methods)
|
GO ID |
Description |
Number of COSMIC census proteins with the given term |
Expected number of proteins with the given term |
p-value |
Over- or under-representation |
Biological processes
|
GO:0007165 |
Signal transduction
|
51 |
26 |
1.418 × 10−3 |
0.96 |
GO:0008283 |
Cell proliferation
|
17 |
4 |
3.055 × 10−3 |
3.25 |
GO:0006811 |
Ion transport
|
0 |
8 |
3.696 × 10−3 |
−1.00 |
GO:0006810 |
Transport
|
9 |
24 |
5.370 × 10−3 |
−0.63 |
GO:0007049 |
Cell cycle
|
20 |
7 |
8.084 × 10−3 |
1.86 |
Molecular functions
|
GO:0005515 |
Protein binding
|
184 |
65 |
1.305 × 10−26 |
1.83 |
GO:0003677 |
DNA binding
|
84 |
27 |
4.907 × 10−10 |
2.11 |
GO:0000166 |
Nucleotide binding
|
72 |
25 |
6.844 × 10−8 |
1.88 |
GO:0004672 |
Protein kinase activity
|
36 |
6 |
5.573 × 10−7 |
5.00 |
GO:0003700 |
Transcription factor activity |
44 |
12 |
3.463 × 10−6 |
2.67 |
GO:0016301 |
Kinase activity
|
37 |
8 |
3.192 × 10−6 |
3.63 |
GO:0016740 |
Transferase activity
|
48 |
18 |
5.276 × 10−5 |
1.67 |
GO:0030528 |
Transcription regulator activity
|
17 |
5 |
7.340 × 10−3 |
2.40 |
Cancer-associated proteins represent a specific group of proteins that are enriched in certain functions, contain more disordered regions, generally are longer and involved in a larger number of interactions (25.5 per protein as compared to 5.5 per protein in the human proteome). However, all these features also correlate with each other. To untangle these complicated relationships, we studied the association between these distinct features. Specifically, we considered the length of the protein, the ratio of its residues residing in disordered segments or disordered binding regions, the number of cancer-associated mutations taken from the COSMIC census database and the number of protein–protein interactions as well as the above identified significant functional classes (see Data and methods). The mutual information and the Jaccard distances were calculated between all pairs of features. The obtained distances between the different features are shown in Table 3. These distances were also subject to multidimensional scaling to reduce the dimensionality to two. The resulting scaled location of each feature is presented in Fig. 4.
Table 3 Jaccard distances of the 6 features calculated on the COSMIC census database as compared to the human proteome (see Data and methods)
|
Length |
Disorder % |
Binding regions % |
COSMIC census mutations |
Interactions |
Functions |
Length |
0.0000 |
0.9871 |
0.9860 |
0.9597 |
0.9776 |
0.9157 |
Disorder % |
|
0.0000 |
0.5170 |
0.9753 |
0.9896 |
0.9208 |
Binding regions % |
|
|
0.0000 |
0.9732 |
0.9860 |
0.9162 |
COSMIC census mutations |
|
|
|
0.0000 |
0.9444 |
0.8808 |
Interactions |
|
|
|
|
0.0000 |
0.8670 |
Functions |
|
|
|
|
|
0.0000 |
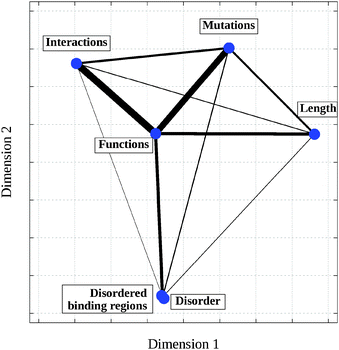 |
| Fig. 4 Two-dimensional mapping of various features based on the distances calculated on the COSMIC census database relative to the human proteome. Coordinates were obtained using multidimensional scaling (see Data and methods) by projecting the original Jaccard distances into two dimensions. The widths of the connecting lines are inversely proportional to the original Jaccard distances (see Table 3). | |
It can be seen that the association between the ratios of residues in disordered regions and disordered binding sites is the highest indicating the relatively constant ratio of disordered residues that are involved in binding. Apart from this strong association, the functional features shared the most information with all the other features. This indicated the central role of function that largely determines the disorder content together with the amount of disordered binding regions, the number of protein–protein interactions, the required length for a given protein and its involvement in cancer. These data suggest that the association between increased amount of protein disorder and cancer in terms of single nucleotide mutations is indirect.
Examples
Besides analyzing the general features of cancer-associated proteins, a few examples are also presented here to gain further insights into how disordered regions and their binding sites contribute to the function of these proteins. The examples were selected from the COSMIC dataset and stand out with the largest number of mutations falling into ordered (p53, PTEN) or disordered regions (β-catenin, ACP). The domain structure (according to PFAM51), the predicted disordered regions and disordered binding regions and the distribution of cancer-associated mutations are shown in Fig. 5. Interestingly, these proteins basically contained no neutral polymorphisms.
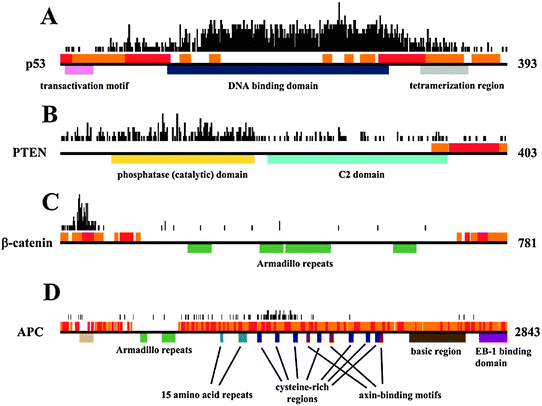 |
| Fig. 5 Domain structure, location of disordered binding regions and disordered segments and the number of cancer-associated mutations per position shown for (A) p53, (B) PTEN, (C) β-catenin and (D) APC. Black horizontal lines mark the full length proteins, colored boxes below show the various Pfam domains, red and orange boxes above show the disordered and disordered binding regions, respectively. The black boxes above the structural descriptions show the number of known cancer-associated mutations for each residue. | |
p53.
The largest number of mutations occurred within p53 (TP53). It is a transcription factor that regulates a large number of genes (>100 genes) and controls a number of key tumor suppressing functions such as cell cycle arrest, DNA repair, senescence and apoptosis.52,53 p53 protein is expressed at a low level in normal cells and at a high level in response to DNA damage and oncogenic transformation. Whilst the activation of p53 often leads to apoptosis, p53 inactivation facilitates tumor progression. From structural point of view, it is composed of the central DNA binding domain that is largely ordered, and the disordered N- and C-termini (Fig. 5A).54,55 These disordered regions harbor several binding sites. Specifically, binding partners for the N-terminal regions include MDM2, RPA 70N and the B subunit of RNA polymerase II.48 The C-terminal contains the tetramerization domain that becomes ordered only upon forming a tetramer. The dynamic nature of this complex, however, is underlined by the presence of the nuclear localization signal hidden within this structure.56 A remarkable example for the plasticity of disordered binding regions is presented by a short segment near the end of the sequence that was observed to bind to several partners, such as S100β, CBP, Cyclin A2 and sirtuin, in different local conformations.11 Although p53 contains a significant amount of disorder that is essential for its central role, cancer-associated mutations are concentrated within the ordered DNA binding domain.54
PTEN
.
PTEN is also among the most frequently inactivated tumor suppressor genes in various cancers, with the second largest number of mutations collected in COSMIC. The PTEN gene encodes a dual specificity phosphatase that can act on both proteins and phosphoinositide substrates.57,58 It negatively regulates the intracellular level of phosphatidylinositol-3,4,5-triphosphate in cells and functions as a tumor suppressor by negatively regulating Akt/PKB signaling pathways. PTEN contains two key domains, the phosphatase (catalytic) domain, and the C2 (lipid membrane-binding) domain (Fig. 5B).59 The C-terminal region is disordered, and the very end of the sequence contains the disordered binding region that can form a complex with the PDZ domain.60 The observed cancer-associated mutations occur throughout the length of PTEN, but they are enriched in the C2 and in especially the phosphatase domains (Fig. 5B). Therefore, this protein is another example where cancer-associated mutations clearly prefer ordered regions. Although p53 and PTEN were thought to act independently as tumor suppressors, with an interesting twist, it turned out that they can interact both directly and indirectly.61 The sites of the physical interaction were localized within the C2 domain of PTEN and the C-terminal region of p53, which is involved in multiple interactions.62 The complex crosstalk between these two proteins is also supported by the recent finding demonstrating that PTEN and p53 somatic mutations are mutually exclusive in the case of human breast cancers.63
β-catenin
.
β-catenin (CTNNB1) goes against the general trend with a significantly higher number of cancer-associated mutations falling into disordered segments. β-catenin is an essential structural component of the cadherin-based cell adhesion complex, and it is also involved in the Wnt/Wingless growth factor signaling pathway.64 In cell adhesion, β-catenin helps link cadherin adhesion molecules to cytoskeletal actin filaments. In its signal transduction role, β-catenin functions as a transcriptional co-activator of target genes involved in cell differentiation and proliferation.65 The core region of β-catenin is composed of 12 copies of a 42 amino acid sequence motif known as an armadillo repeat (Fig. 5C). These repeats form a superhelix of helices that features a long, positively charged groove.66 This groove mediates the interaction of β-catenin with several unrelated partners, largely based on charge complementarity. Besides the central repeat region, the protein also contains short disordered segments on both termini. Nearly all cancer-associated mutations are located in the N-terminal disordered region (Fig. 5C). Of particular importance is the second predicted disordered binding site that also contains a short linear motif, the DSGxxS diphosphodegron. This region is recognized by the SCF-β-TrCP E3 ligase with the binding site located at the top face of the βTrCP1 WD40 β-propeller.67 The complex formation targets β-catenin for proteasome destruction depending on the phosphorylation state of its degron.68 Mutations in this region can lead to malignant transformation by increasing the cytoplasmic pool of β-catenin. This prompts its translocation to the nucleus, where it activates downstream elements of the Wnt pathway leading to cell overgrowth.64
APC
.
A large number of cancer-associated mutations within disordered regions are also present in another key element of the Wnt signalling pathway, the adenomatous polyposis coli (APC) protein.69 Mutations of this protein frequently occur in colorectal tumors.70APC is a large (2843 residues) protein with several putative functions in cell cycle control, differentiation, migration, apoptosis, and the maintenance of chromosomal stability. It acts as a tumor suppressor based on its ability to bind to β-catenin and to promote its rapid degradation.71,72 By downregulating CTNNB1, ACP acts as a negative regulator in Wnt signaling. The central region of APC contains multiple β-catenin interaction motifs, including three 15 amino acid repeats and seven 20 amino acid cysteine-rich repeats.73,74 The large majority of cancer-associated mutations are located within the first three 20 amino acid repeats (Fig. 5D). The protein contains several additional domains or motifs, such as the oligomerization domain, armadillo repeats, axin binding repeats, basic region, and EB-1 interaction domain. With the exception of the armadillo repeats, these regions are largely disordered and contain several binding regions. Nevertheless, the cancer-associated mutations are significantly less frequent within these regions. This also indicates that it is not disorder in itself, but it is rather the specific function that can pose an increased biological risk in this case.
Discussion
IDP regions are important elements of cancer-associated proteins.12 In general, disordered proteins are fundamentally different from globular proteins both in their structural and functional properties. This necessitates the understanding of how these regions contribute to the development of cancer.
Ordered and disordered proteins are expected to differ in terms of their tolerance to mutations. The basic assumption is that neutral polymorphisms are less likely to occur in positions with stronger structural and functional constraints. In globular proteins, functionally relevant sites are often restricted to a few residues that form the active site, but nearly all residues contribute to the formation of the 3D structure at some level.75,76 This represents a large evolutionary constraint for globular proteins. Functionally important residues of IDPs, such as residues directly involved in binding or undergoing post-translational modifications, can experience constraints similar to the active sites of globular proteins. In terms of structural constraints, however, mutations generally are expected to have a smaller impact on the structural properties of disordered segments, due to the lack of a well-defined structure. The increased evolutionary constraints of ordered residues compared to disordered ones have been observed at various evolutionary distances, ranging from human polymorphisms,28 to the divergence between mouse and human.43 Similar conclusions were drawn from the comparison of evolutionarily related sequences from different organisms that indicated that disordered segments were generally less conserved.44,45 Deviations from this trend were observed in only a few cases and were mostly attributed to the involvement in protein–protein interactions.44
In complete agreement with this view, the larger tolerance to mutations of disordered segments was also present in cancer-associated proteins. Our results showed that a significantly fewer number of SNPs were observed in ordered regions compared to disordered regions in cancer-associated proteins. In contrast, cancer-associated mutations were more likely to occur within ordered segments. This effect was even larger, when the uneven distribution of polymorphisms was taken into account. These results suggest that disordered residues are more tolerant to mutations at two levels. Firstly, disordered regions can allow a larger number of genetic variations without affecting the function. Secondly, if a mutation occurs, it is more likely to cause cancer if the affected residue is located within an ordered region. The lower sensitivity of disordered regions to genetic variations is likely to originate from the specific structural properties of these regions. The analysis of disordered binding regions showed that functionally relevant sites within disordered regions can slightly deviate from this behavior. Disordered binding regions could be placed between disordered regions in general and ordered regions, both in terms of the appearance of polymorphisms and cancer associated mutations. These suggest stronger evolutionary constraints within disordered binding regions, in accordance with their functional importance. Nevertheless, within the broader context of binding regions, only a few residues might be directly responsible for the specificity of the binding.77 These residues could present even higher evolutionary constraints.
While results obtained on the various datasets agreed quite well, there were some variations. These differences can be associated with potential biases of the datasets. For example, since cancer genome projects rely on identifying nucleotide changes between normal and cancer cell lines at the level of genome, differences can also occur by random sites that are not actively involved in tumorigenesis. Mutations that occur randomly throughout the sequence do not bias our results, although they could decrease the statistical significance of the observed differences. However, we observed that neutral SNPs were not distributed randomly, but were more likely to occur within disordered regions. We accounted for this by using a different type of normalization. This leads to a more consistent picture with more pronounced differences, showing that cancer-associated mutations are more likely to occur within ordered regions. The normalization had the largest effect on the pure data of cancer genomes projects, where a higher number of non-disease causing mutations were expected. In the other cases, the results did not change much. Nevertheless, passenger mutations can also be present in the other databases. This is supported by the fact that only a few neutral polymorphisms were described in the case of our examples while they had the highest number of cancer-associated mutations. Due to the potential problems of passenger mutations, we used the term “cancer-associated mutation” throughout the manuscript. To weed out these mutations, further studies are needed. One of the important conclusions of our work is that such random mutations are not distributed evenly and affect disordered regions even more. This phenomenon should be taken into account in selecting driver mutations.
Other databases may suffer from different types of biases. For example, the SP_cancer_annotated dataset had a smaller percentage of disordered residues, in contrast to the increase of protein disorder in all other datasets. The preference of cancer associated mutations for ordered residues was also unusually high in this case. We suspect that the slightly different behavior in this case originates from the experimental biases of traditional approaches that could have favored ordered proteins. We could observe some differences within the various cancer genome projects as well, for example in the distribution of disordered binding regions (Fig. 3). The results obtained for breast and colorectal cancers agreed well in the two cases, but there were some differences when the CAN gene sets of glioblastoma and pancreatic cancer were considered. Although larger statistical variations can be expected in these cases due to the small size of these datasets, the results caution us that different types of cancer might be associated with different molecular and functional properties. Nevertheless, the 12 datasets analyzed in this work presented quite a consistent picture altogether, despite their different sizes, origins, and potential biases. The consistency of these results lends confidence to our findings, showing that while in cancer genes neutral polymorphisms are more likely to occur within disordered regions, cancer-associated mutations are more common in ordered regions.
Our general finding is in contrast with the results obtained in the analyses of another major form of genetic aberrations leading to cancer, chromosomal translocations. In this case, a direct link between disorder and cancer was found.20 This was rationalized based on that ordered proteins are more likely to be misfolded and degraded as a result of translocation, while disordered proteins could survive with an aberrant function.2,20 A third form of commonly occurring genetic variations is copy number variation (CNV), which corresponds to the enrichment or depletion of certain genomic regions. CNVs are frequently observed in cancer and other diseases. In a recent study, a strong correlation between dosage sensitive gene products and protein disorder was found, and it was related to the interaction promiscuity of IDPs.21 Interestingly, in two of the analyzed examples mutations affected disordered regions that regulated the level of β-catenin, a central element of the Wnt signalling pathway. These examples are in agreement with the observation that disordered proteins are generally under tight cellular control.78,79 In contrast, the level of p53 is regulated by MDM2.52 The specific binding site, however, did not show an increased rate of cancer-associated mutations (Fig. 5A). In order to resolve these seemingly contradictory results, cancer-associated mutations have to be placed into a network context. The network view was also suggested to be crucial in order to reduce the complexity of the landscape of cancer genomes.33
In conclusion, our results clearly show that protein disorder in itself is not responsible for the increased biological risk in terms of cancer-associated mutations. It seems plausible that the functional involvement of a protein determines both its disorder content and its involvement in cancer, thus presenting a correlation between these two features, without an existing casual link between them. Our study was restricted to single amino acid changes, however, other type of genetic alterations can also lead to cancer. A strong association between protein disorder and cancer was suggested in copy number variations or chromosomal translocations. The exploration of the role of protein disorder in these cases necessitates many further studies and taking into account the specific functions of these proteins and the way they are regulated. The present work, nevertheless, demonstrated that genetic mutations affect ordered and disordered regions in different ways, in accordance with the distinct structural and functional properties of these segments. In order to understand the background of various diseases, these differences have to be taken into account.
Data and methods
Datasets
SwissProt cancer datasets.
We used three different resources to collect various cancer-associated genetic variations. The first dataset was downloaded from the UniProt/SwissProt Knowledgebase35 and was derived primarily from literature reports using strict inclusion criteria. This dataset contains polymorphisms with no clinical relevance, disease related amino acid mutations and some unclassified variants. Cancer-associated mutations were collected from the pre-compiled database available at http://www.uniprot.org/docs/humsavar. In the full dataset (SP_cancer) those entries were kept, where the ‘Disease name’ field either matched one of the selection keywords (‘cancer’, ‘tumor’, ‘lymphoma’, ‘leukemia’, ‘carcinoma’, ‘glioma’, ‘glioblastoma’, ‘melanoma’ and ‘sarcoma’) or had an OMIM reference to a type of cancer (checked on the http://www.omim.org/ site). A smaller list was also created by selecting the mutations from SP_cancer that had ‘Disease’ annotations in the database omitting ones with ‘Unclassified’ tags (SP_cancer_annotated).
Cancer genome project datasets.
The second type of datasets corresponded to four cancer genome projects collecting the result of comprehensive genome-wide analyses. Two of these studies described the mutations of breast and colorectal cancer (CGP_br/col_1 and CGP_br/col_2 datasets31,32), one focused on pancreatic cancer (CGP_pan dataset33) and one on glioblastoma (CGP_glio dataset34). In these studies, somatic mutations in cancer were determined by sequencing the major fraction of human genes and identifying nucleotide changes. Any alterations that were also present in normal samples or could be found in single-nucleotide polymorphism (SNP) databases were removed. The list of somatic mutations could still contain nonfunctional “passenger” alterations. To distinguish genes likely to contribute to tumorigenesis from those in which passenger mutations occurred by chance, a list of candidate cancer genes (CAN genes) was established based on the probability that the number of mutations in a given gene was greater than expected from the background mutation rate. The mutations described in these selected genes were used to compile the four datasets CGP_CAN_br/col_1, CGP_CAN_br/col_2, CGP_CAN_pan and CGP_CAN_glio. The list of gene identifiers and the nucleotide changes were downloaded from the supplementary materials of the original publications.
COSMIC.
The third dataset was collected from the COSMIC database.36 This is currently the most comprehensive catalogue of somatic mutations in cancer. Data are gathered from two sources, publications in the scientific literature (v52 contains 11
437 curated articles) and the full output of the genome-wide screens from the Cancer Genome Project (CGP) at the Sanger Institute, UK. This dataset also incorporated the outcome of cancer genome projects. A small subset of the COSMIC database was also part of the cancer census datasets that were casually linked to oncogenesis.37 These genes constituted the COSMIC_census dataset.
Although there could be some overlap between the three datasets, we opted to keep them separately in order to be able to observe any potential biases. Our analysis was restricted to single missense substitutions. Altogether we analyzed 12 different datasets. The number of proteins and mutations in each dataset are listed in Table 1.
Polymorphisms.
In the case of SP datasets, the polymorphisms present in the SwissProt resource were also collected in the SP_poly dataset and were used as reference.35 In all other cases, polymorphisms were collected using the UCSC Genome Browser.80 Single genes were mapped to the genomic location corresponding to the UCSC Santa Cruz hg19/GRCh37 build. Those sequences, that could not be mapped, were changed or retracted, were discarded from further analyses. The polymorphism data were obtained by mapping the SNPs of dbSNP (release 132)25 to the genomic coordinates. This release contained over 13 million SNPs. It also incorporated the results of the 1000 Genomes pilot projects that collected variations via whole genome shotgun sequencing from two families with high coverage and 179 individuals with low coverage.27 We used the Common SNPs corresponding to uniquely mapped variants that appear in at least 1% of the population. The commonness of these variations suggests that these are likely to be neutral polymorphisms with no clinical relevance. To ensure the quality of the polymorphisms data, we only used validated SNPs.
All cancer associated mutations and polymorphisms were transformed into a common format specifying the used identifier of the sequence, the sequence position of the mutation and the original and mutated amino acids. This format enabled a simple selection at the level of unique mutations, therefore identical polymorphisms were only counted once. The numbers of polymorphisms are also listed in Table 1.
Human proteome.
The proteins of the human proteome were downloaded from the “complete proteome” page of the UniProt database. Only reviewed entries were kept, resulting in a dataset of 20
232 proteins.
Functional annotations
Functional classifications were based on GeneOntology (GO)81 terms assigned to human proteins in UniProt. We retrieved all GO terms for all proteins in the human proteome and mapped them to high level GO terms described in the Generic GOslim subset of GO. This subset contained 127 terms covering all three parts of GO annotations: biological processes (50 terms), cellular components (36 terms) and molecular functions (41 terms). All proteins from COSMIC, were possible, were mapped to UniProt sequences and were assigned the relevant GOslim terms.
Interactions
Protein–protein interactions were taken from the current release of the IntAct database (www.ebi.ac.uk/intact/).82
Statistical analysis
Comparison of the 12 cancer databases and the human proteome.
The average ratio of disordered residues, ratio of proteins containing >30 residue long disordered regions and average length were calculated in the 12 cancer datasets analyzed (Fig. 1). These averages were compared to the average values calculated in the human proteome. For each of the 12 datasets, standard errors of the mean were calculated by selecting 10
000 random samples from the human proteome of the same size as the respective dataset. In each of the 10
000 random selections, the means were calculated. From these means the standard error of the mean was established and used to test the difference between the random samples and the database average. The mean values, standard errors and the appropriate p-values are shown in Table S1 (ESI‡). Fig. 1 shows the confidence intervals of α = 0.01 (corresponding to 2.576 standard errors) in each case.
Over- and under-representation of polymorphisms and cancer-associated mutations.
For each protein in our dataset, the sequences were downloaded from the Uniprot database or the UCSC Genome Browser. Using the sequence, the IUPred method39,40 was used to assess which residues were part of disordered regions. These results were also calculated with two other disorder prediction methods, DISOPRED25 and VSL2.41 The ANCHOR method48,49 was used to predict regions involved in disordered binding regions. While there are several methods to predict disordered residues, ANCHOR is the only publicly available method for the prediction of disordered binding regions. For each protein, the number of polymorphisms and cancer-associated mutations within these regions were calculated. These numbers were compared to the expected number of mutations calculated in the following way: to calculate the expected number of mutations for ordered and disordered regions, the number of observed mutations was divided according to the ratio of ordered and disordered residues in the given sequence. This model takes into account that the number of mutations can change from one protein to another. The number of expected and observed mutations was summed up separately for ordered and disordered segments. Using these numbers, the statistical significance of the differences in the two distributions was assessed by the χ2 test.
In this null model we assumed that the selection pressure on disordered and ordered regions is the same, and the probability that a mutation occurs in ordered or disordered regions is equal. We expect that the observed differences are mainly the result of selection acting at the protein level. It should be noted that other factors can also contribute to the selections, for example, by affecting the stability of DNA, mRNA, or interactions with regulatory factors. We checked that taking into account the different codon usage, or differences in transition–transversion rates does not affect our results.
In the case of cancer-associated mutations, an additional model was used to calculate the expected number of mutations. This took into account the uneven distribution of polymorphisms between ordered and disordered regions. The model was based on a normalization factor calculated from the ratio of the observed number of SNPs relative to their expected number. The normalization factor was calculated for disordered and ordered residues, in each dataset. The expected number of mutations was recalculated by weighting them according to the normalization factor for disordered and ordered residues within each dataset. Using these references, the statistical significance could be calculated similarly to the previous case. Unfortunately, current data do not enable us to calculate this factor for proteins individually. However, when datasets were divided into subgroups, for example based on the number of mutations, the results did not change.
Distributions of functional categories.
The distribution of each GO term was analyzed using the COSMIC_census dataset. To determine significantly over- or under-represented terms, the distribution of these terms in the human proteome was used as a reference. A random subset was selected from the human proteome dataset and was parsed for occurrence numbers of each term. This was repeated 100 times and then the average occurrence of each term was calculated. These occurrence numbers were compared to the occurrence numbers in the COSMIC_census dataset using left and right sided Fisher tests to assign significance values to the under- and over-representation of terms.
Features.
The calculated length, ratio of disordered residues and disordered binding residues, interaction numbers and the number of COSMIC census mutations for COSMIC census proteins and the randomly selected reference human proteins were categorized into 5 bins to provide a coarse-grained description. The boundaries of the bins for each feature are shown in Table S4 (ESI‡). The sixth feature describing the functional involvement of the proteins was represented by ‘functional profiles’. These profiles were calculated based on the significantly over- and under-represented GO terms shown in Table 3. For each protein, a 13 element binary vector was assigned that showed which of the 13 considered GO terms the protein was annotated with.
Mutual information and Jaccard distance.
The association between different features calculated on proteins was measured by calculating the mutual information (I(X,Y)) between all X and Y pairs of features using the standard formula:
where p′(x) and p′′(y) are the probability distributions of the features X and Y respectively and p(x,y) is their joint probability distribution. As the maximal information of different features can vary (and hence their maximal mutual information can also vary), to be able to compare the association of different parameter pairs directly, the mutual information was scaled:
where H(X,Y) is the joint entropy of X and Y:
The resulting D(X,Y) Jaccard distance is a universal metric with D(X,Y) = 1 if X and Y are completely independent and D(X,Y) = 0 if X and Y are identical.
The multidimensional scaling of the obtained distances was calculated using the R package.
Acknowledgements
This work was sponsored by the Hungarian Scientific Research Fund (OTKA) [grant number K 72569]. The Bolyai Janos fellowship for Z.D. and Charles Simonyi fellowship for I.S. are also gratefully acknowledged. We would like to thank Lajos Kalmár for his critical comments on the project.
References
- A. K. Dunker, J. D. Lawson, C. J. Brown, R. M. Williams, P. Romero, J. S. Oh, C. J. Oldfield, A. M. Campen, C. M. Ratliff, K. W. Hipps, J. Ausio, M. S. Nissen, R. Reeves, C. Kang, C. R. Kissinger, R. W. Bailey, M. D. Griswold, W. Chiu, E. C. Garner and Z. Obradovic, J. Mol. Graphics Modell., 2001, 19, 26–59 CrossRef CAS.
- H. J. Dyson and P. E. Wright, Nat. Rev. Mol. Cell Biol., 2005, 6, 197–208 CrossRef CAS.
- P. Tompa, Trends Biochem. Sci., 2002, 27, 527–533 CrossRef CAS.
- A. K. Dunker, Z. Obradovic, P. Romero, E. C. Garner and C. J. Brown, Genome Inf. Ser. Workshop Genome Inf., 2000, 11, 161–171 CAS.
- J. J. Ward, J. S. Sodhi, L. J. McGuffin, B. F. Buxton and D. T. Jones, J. Mol. Biol., 2004, 337, 635–645 CrossRef CAS.
- P. Tompa, FEBS Lett., 2005, 579, 3346–3354 CrossRef CAS.
- K. Gunasekaran, C. J. Tsai, S. Kumar, D. Zanuy and R. Nussinov, Trends Biochem. Sci., 2003, 28, 81–85 CrossRef CAS.
- B. Mészáros, P. Tompa, I. Simon and Z. Dosztányi, J. Mol. Biol., 2007, 372, 549–561 CrossRef.
- A. K. Dunker, E. Garner, S. Guilliot, P. Romero, K. Albrecht, J. Hart, Z. Obradovic, C. Kissinger and J. E. Villafranca, Pac. Symp. Biocomput., 1998, 473–484 CAS.
- H. J. Dyson and P. E. Wright, Curr. Opin. Struct. Biol., 2002, 12, 54–60 CrossRef CAS.
- C. J. Oldfield, J. Meng, J. Y. Yang, M. Q. Yang, V. N. Uversky and A. K. Dunker, BMC Genomics, 2008, 9(suppl 1), S1 CrossRef.
- L. M. Iakoucheva, P. Radivojac, C. J. Brown, T. R. O'Connor, J. G. Sikes, Z. Obradovic and A. K. Dunker, Nucleic Acids Res., 2004, 32, 1037–1049 CrossRef CAS.
- C. A. Galea, Y. Wang, S. G. Sivakolundu and R. W. Kriwacki, Biochemistry, 2008, 47, 7598–7609 CrossRef CAS.
- H. Xie, S. Vucetic, L. M. Iakoucheva, C. J. Oldfield, A. K. Dunker, V. N. Uversky and Z. Obradovic, J. Proteome Res., 2007, 6, 1882–1898 CrossRef CAS.
- C. Haynes, C. J. Oldfield, F. Ji, N. Klitgord, M. E. Cusick, P. Radivojac, V. N. Uversky, M. Vidal and L. M. Iakoucheva, PLoS Comput. Biol., 2006, 2, e100 Search PubMed.
- Z. Dosztányi, J. Chen, A. K. Dunker, I. Simon and P. Tompa, J. Proteome Res., 2006, 5, 2985–2995 CrossRef.
- V. N. Uversky, C. J. Oldfield and A. K. Dunker, Annu. Rev. Biophys., 2008, 37, 215–246 CrossRef CAS.
- B. Vogelstein, D. Lane and A. J. Levine, Nature, 2000, 408, 307–310 CrossRef CAS.
- L. M. Iakoucheva, C. J. Brown, J. D. Lawson, Z. Obradovic and A. K. Dunker, J. Mol. Biol., 2002, 323, 573–584 CrossRef CAS.
- H. Hegyi, L. Buday and P. Tompa, PLoS Comput. Biol., 2009, 5, e1000552 Search PubMed.
- T. Vavouri, J. I. Semple, R. Garcia-Verdugo and B. Lehner, Cell (Cambridge, Mass.), 2009, 138, 198–208 CAS.
- Y. Cheng, T. LeGall, C. J. Oldfield, A. K. Dunker and V. N. Uversky, Biochemistry, 2006, 45, 10448–10460 CrossRef CAS.
- V. N. Uversky, Front. Biosci., 2009, 14, 5188–5238 CrossRef CAS.
- P. Tompa, FEBS J., 2009, 276, 5406–5415 CrossRef CAS.
- S. T. Sherry, M. H. Ward, M. Kholodov, J. Baker, L. Phan, E. M. Smigielski and K. Sirotkin, Nucleic Acids Res., 2001, 29, 308–311 CrossRef CAS.
- D. F. Conrad, J. E. Keebler, M. A. Depristo, S. J. Lindsay, Y. Zhang, F. Casals, Y. Idaghdour, C. L. Hartl, C. Torroja, K. V. Garimella, M. Zilversmit, R. Cartwright, G. A. Rouleau, M. Daly, E. A. Stone, M. E. Hurles and P. Awadalla, Nat. Genet., 2011, 43, 712–714 CrossRef CAS.
- The 1000 Genomes Project Consortium, Nature, 2010, 467, 1061–1073 CrossRef.
- J. Liu, Y. Zhang, X. Lei and Z. Zhang, Genome Biol., 2008, 9, R69 CrossRef.
- A. Bardelli and V. E. Velculescu, Curr. Opin. Genet. Dev., 2005, 15, 5–12 CrossRef CAS.
- H. Ledford, Nature, 2010, 464, 972–974 CrossRef CAS.
- T. Sjoblom, S. Jones, L. D. Wood, D. W. Parsons, J. Lin, T. D. Barber, D. Mandelker, R. J. Leary, J. Ptak, N. Silliman, S. Szabo, P. Buckhaults, C. Farrell, P. Meeh, S. D. Markowitz, J. Willis, D. Dawson, J. K. Willson, A. F. Gazdar, J. Hartigan, L. Wu, C. Liu, G. Parmigiani, B. H. Park, K. E. Bachman, N. Papadopoulos, B. Vogelstein, K. W. Kinzler and V. E. Velculescu, Science, 2006, 314, 268–274 CrossRef.
- L. D. Wood, D. W. Parsons, S. Jones, J. Lin, T. Sjoblom, R. J. Leary, D. Shen, S. M. Boca, T. Barber, J. Ptak, N. Silliman, S. Szabo, Z. Dezso, V. Ustyanksky, T. Nikolskaya, Y. Nikolsky, R. Karchin, P. A. Wilson, J. S. Kaminker, Z. Zhang, R. Croshaw, J. Willis, D. Dawson, M. Shipitsin, J. K. Willson, S. Sukumar, K. Polyak, B. H. Park, C. L. Pethiyagoda, P. V. Pant, D. G. Ballinger, A. B. Sparks, J. Hartigan, D. R. Smith, E. Suh, N. Papadopoulos, P. Buckhaults, S. D. Markowitz, G. Parmigiani, K. W. Kinzler, V. E. Velculescu and B. Vogelstein, Science, 2007, 318, 1108–1113 CrossRef CAS.
- S. Jones, X. Zhang, D. W. Parsons, J. C. Lin, R. J. Leary, P. Angenendt, P. Mankoo, H. Carter, H. Kamiyama, A. Jimeno, S. M. Hong, B. Fu, M. T. Lin, E. S. Calhoun, M. Kamiyama, K. Walter, T. Nikolskaya, Y. Nikolsky, J. Hartigan, D. R. Smith, M. Hidalgo, S. D. Leach, A. P. Klein, E. M. Jaffee, M. Goggins, A. Maitra, C. Iacobuzio-Donahue, J. R. Eshleman, S. E. Kern, R. H. Hruban, R. Karchin, N. Papadopoulos, G. Parmigiani, B. Vogelstein, V. E. Velculescu and K. W. Kinzler, Science, 2008, 321, 1801–1806 CrossRef CAS.
- D. W. Parsons, S. Jones, X. Zhang, J. C. Lin, R. J. Leary, P. Angenendt, P. Mankoo, H. Carter, I. M. Siu, G. L. Gallia, A. Olivi, R. McLendon, B. A. Rasheed, S. Keir, T. Nikolskaya, Y. Nikolsky, D. A. Busam, H. Tekleab, L. A. Diaz, Jr., J. Hartigan, D. R. Smith, R. L. Strausberg, S. K. Marie, S. M. Shinjo, H. Yan, G. J. Riggins, D. D. Bigner, R. Karchin, N. Papadopoulos, G. Parmigiani, B. Vogelstein, V. E. Velculescu and K. W. Kinzler, Science, 2008, 321, 1807–1812 CrossRef CAS.
- Y. L. Yip, M. Famiglietti, A. Gos, P. D. Duek, F. P. David, A. Gateau and A. Bairoch, Hum. Mutat., 2008, 29, 361–366 CrossRef CAS.
- S. A. Forbes, N. Bindal, S. Bamford, C. Cole, C. Y. Kok, D. Beare, M. Jia, R. Shepherd, K. Leung, A. Menzies, J. W. Teague, P. J. Campbell, M. R. Stratton and P. A. Futreal, Nucleic Acids Res., 2011, 39, D945–D950 CrossRef.
- P. A. Futreal, L. Coin, M. Marshall, T. Down, T. Hubbard, R. Wooster, N. Rahman and M. R. Stratton, Nat. Rev. Cancer, 2004, 4, 177–183 CrossRef CAS.
- UniProt Consortium, Nucleic Acids Res., 2011, 39, D214–D219 CrossRef.
- Z. Dosztányi, V. Csizmók, P. Tompa and I. Simon, Bioinformatics, 2005, 21, 3433–3434 CrossRef.
- Z. Dosztányi, V. Csizmók, P. Tompa and I. Simon, J. Mol. Biol., 2005, 347, 827–839 CrossRef.
- K. Peng, P. Radivojac, S. Vucetic, A. K. Dunker and Z. Obradovic, BMC Bioinf., 2006, 7, 208 CrossRef.
- S. J. Furney, D. G. Higgins, C. A. Ouzounis and N. Lopez-Bigas, BMC Genomics, 2006, 7, 3 CrossRef.
- Y. Xia, E. A. Franzosa and M. B. Gerstein, PLoS Comput. Biol., 2009, 5, e1000413 Search PubMed.
- C. J. Brown, S. Takayama, A. M. Campen, P. Vise, T. W. Marshall, C. J. Oldfield, C. J. Williams and A. K. Dunker, J. Mol. Evol., 2002, 55, 104–110 CrossRef CAS.
- C. J. Brown, A. K. Johnson, A. K. Dunker and G. W. Daughdrill, Curr. Opin. Struct. Biol., 2011, 21, 441–446 CrossRef CAS.
- B. Mészáros, P. Tompa, I. Simon and Z. Dosztányi, J. Mol. Biol., 2007, 372, 549–561 CrossRef.
- B. Mészáros, I. Simon and Z. Dosztányi, Phys. Biol., 2011, 8, 035003 CrossRef.
- B. Mészáros, I. Simon and Z. Dosztányi, PLoS Comput. Biol., 2009, 5, e1000376 Search PubMed.
- Z. Dosztányi, B. Mészáros and I. Simon, Bioinformatics, 2009, 25, 2745–2746 CrossRef.
- Y. Cheng, C. J. Oldfield, J. Meng, P. Romero, V. N. Uversky and A. K. Dunker, Biochemistry, 2007, 46, 13468–13477 CrossRef CAS.
- R. D. Finn, J. Mistry, J. Tate, P. Coggill, A. Heger, J. E. Pollington, O. L. Gavin, P. Gunasekaran, G. Ceric, K. Forslund, L. Holm, E. L. Sonnhammer, S. R. Eddy and A. Bateman, Nucleic Acids Res., 2010, 38, D211–D222 CrossRef CAS.
- C. A. Brady and L. D. Attardi, J. Cell Sci., 2010, 123, 2527–2532 CrossRef CAS.
- T. Soussi and K. G. Wiman, Cancer Cell, 2007, 12, 303–312 CrossRef CAS.
- A. C. Joerger and A. R. Fersht, Adv. Cancer Res., 2007, 97, 1–23 CrossRef CAS.
- S. Bell, C. Klein, L. Muller, S. Hansen and J. Buchner, J. Mol. Biol., 2002, 322, 917–927 CrossRef CAS.
- C. Addison, J. R. Jenkins and H. W. Sturzbecher, Oncogene, 1990, 5, 423–426 CAS.
- M. C. Hollander, G. M. Blumenthal and P. A. Dennis, Nat. Rev. Cancer, 2011, 11, 289–301 CrossRef CAS.
- T. Maehama and J. E. Dixon, Trends Cell Biol., 1999, 9, 125–128 CrossRef CAS.
- J. O. Lee, H. Yang, M. M. Georgescu, A. Di Cristofano, T. Maehama, Y. Shi, J. E. Dixon, P. Pandolfi and N. P. Pavletich, Cell (Cambridge, Mass.), 1999, 99, 323–334 CAS.
- W. Feng, H. Wu, L. N. Chan and M. Zhang, J. Biol. Chem., 2008, 283, 23440–23449 CrossRef CAS.
- L. C. Trotman and P. P. Pandolfi, Cancer Cell, 2003, 3, 97–99 CrossRef CAS.
- D. J. Freeman, A. G. Li, G. Wei, H. H. Li, N. Kertesz, R. Lesche, A. D. Whale, H. Martinez-Diaz, N. Rozengurt, R. D. Cardiff, X. Liu and H. Wu, Cancer Cell, 2003, 3, 117–130 CrossRef CAS.
- K. Kurose, K. Gilley, S. Matsumoto, P. H. Watson, X. P. Zhou and C. Eng, Nat. Genet., 2002, 32, 355–357 CrossRef CAS.
- L. Shapiro, Structure (London), 1997, 5, 1265–1268 CAS.
- B. M. Gumbiner, Curr. Opin. Cell Biol., 1995, 7, 634–640 CrossRef CAS.
- A. H. Huber, W. J. Nelson and W. I. Weis, Cell (Cambridge, Mass.), 1997, 90, 871–882 CAS.
- G. Wu, G. Xu, B. A. Schulman, P. D. Jeffrey, J. W. Harper and N. P. Pavletich, Mol. Cell, 2003, 11, 1445–1456 CrossRef CAS.
- M. Al-Fageeh, Q. Li, W. M. Dashwood, M. C. Myzak and R. H. Dashwood, Oncogene, 2004, 23, 4839–4846 CrossRef CAS.
- P. Polakis, Biochim. Biophys. Acta, 1997, 1332, F127–F147 CAS.
- K. W. Kinzler and B. Vogelstein, Cell (Cambridge, Mass.), 1996, 87, 159–170 CAS.
- B. Rubinfeld, B. Souza, I. Albert, O. Muller, S. H. Chamberlain, F. R. Masiarz, S. Munemitsu and P. Polakis, Science, 1993, 262, 1731–1734 CAS.
- L. K. Su, B. Vogelstein and K. W. Kinzler, Science, 1993, 262, 1734–1737 CAS.
- Y. Xing, W. K. Clements, I. Le Trong, T. R. Hinds, R. Stenkamp, D. Kimelman and W. Xu, Mol. Cell, 2004, 15, 523–533 CrossRef CAS.
- K. Eklof Spink, S. G. Fridman and W. I. Weis, EMBO J., 2001, 20, 6203–6212 CrossRef CAS.
- M. Guharoy and P. Chakrabarti, Proc. Natl. Acad. Sci. U. S. A., 2005, 102, 15447–15452 CrossRef CAS.
- M. Landau, I. Mayrose, Y. Rosenberg, F. Glaser, E. Martz, T. Pupko and N. Ben-Tal, Nucleic Acids Res., 2005, 33, W299–W302 CrossRef CAS.
- M. Fuxreiter, P. Tompa and I. Simon, Bioinformatics, 2007, 23, 950–956 CrossRef CAS.
- J. Gsponer, M. E. Futschik, S. A. Teichmann and M. M. Babu, Science, 2008, 322, 1365–1368 CrossRef CAS.
- M. M. Babu, R. van der Lee, N. S. de Groot and J. Gsponer, Curr. Opin. Struct. Biol., 2011, 21, 432–440 CrossRef CAS.
- J. Z. Sanborn, S. C. Benz, B. Craft, C. Szeto, K. M. Kober, L. Meyer, C. J. Vaske, M. Goldman, K. E. Smith, R. M. Kuhn, D. Karolchik, W. J. Kent, J. M. Stuart, D. Haussler and J. Zhu, Nucleic Acids Res., 2011, 39, D951–D959 CrossRef.
- M. Ashburner, C. A. Ball, J. A. Blake, D. Botstein, H. Butler, J. M. Cherry, A. P. Davis, K. Dolinski, S. S. Dwight, J. T. Eppig, M. A. Harris, D. P. Hill, L. Issel-Tarver, A. Kasarskis, S. Lewis, J. C. Matese, J. E. Richardson, M. Ringwald, G. M. Rubin and G. Sherlock, Nat. Genet., 2000, 25, 25–29 CrossRef CAS.
- B. Aranda, P. Achuthan, Y. Alam-Faruque, I. Armean, A. Bridge, C. Derow, M. Feuermann, A. T. Ghanbarian, S. Kerrien, J. Khadake, J. Kerssemakers, C. Leroy, M. Menden, M. Michaut, L. Montecchi-Palazzi, S. N. Neuhauser, S. Orchard, V. Perreau, B. Roechert, K. van Eijk and H. Hermjakob, Nucleic Acids Res., 2010, 38, D525–D531 CrossRef CAS.
Footnotes |
† Published as part of a Molecular BioSystems themed issue on Intrinsically Disordered Proteins: Guest Editor M. Madan Babu. |
‡ Electronic supplementary information (ESI) available. See DOI: 10.1039/c1mb05246b |
|
This journal is © The Royal Society of Chemistry 2012 |
Click here to see how this site uses Cookies. View our privacy policy here.