Open Access Article
DOI:
10.1039/C1MB05205E
(Opinion)
Mol. BioSyst., 2012,
8, 22-26
How do dynamic cellular signals travel long distances?†
Received
27th May 2011
, Accepted 29th June 2011
First published on 18th July 2011
Abstract
Communication is essential. It is vital between cells in multi-cellular organisms, and within cells. A signaling molecule binds to a receptor protein, and initiates a cascade of dynamic events. Signaling is a multistep pathway, which allows signal amplification: if some of the molecules in a pathway transmit the signal to multiple molecules, the result can be a large number of activated molecules across the cell and multiple reactions. That is how a small number of extracellular signaling molecules can produce a major cellular response. The pathway can relay signals from the extracellular space to the nucleus. How do signals travel efficiently over long-distances across the cell? Here we argue that evolution has utilized three properties: a modular functional organization of the cellular network; sequences in some key regions of proteins, such as linkers or loops, which were pre-encoded by evolution to facilitate signaling among domains; and compact interactions between proteins which is achieved via conformational disorder.
Introduction
Cellular function necessitates transfer of information across long distances. How is the information transferred efficiently to achieve optimal cellular response? Tissues, cells, developmental stages, environments, systems and functions are diverse. The functions that can be performed by a multi-cellular organism are determined by the type and organization of the cells and their environment. Humans have about 100 trillion or 1014cells and a typical cell size is 10 μm; however, it can be much larger, reaching 100 μm. Cells shapes can vary; some, like neurons are typically elongated; others like the erythrocytes are equi-dimensional. Some cells are encased in rigid walls; others are flexible. One of the fundamental common properties of eukaryotes is their ability to maintain cell sizes and organelle volumes, and these relate to growth and differentiation states. Although the number of most organelles, like the endoplasmic reticulum and mitochondria, can vary substantially, cells maintain a roughly constant karyoplasmic ratio (the ratio of the nuclear volume to cell volume);1,2 that is, the nuclear size is proportional to the total cell size. The sizes of individual nuclei are apparently controlled by the relative amount of cytoplasm surrounding each nucleus, which relates the cytoplasm to nuclear size control.3–5 The large sizes and volumes of the cell and the organelles emphasize a key cellular dilemma: how to cross the huge distances that need be spanned in order to coordinate cellular function in the dynamic environment? To survive and optimally respond to morphogen signals entering the cell during development, changes in external physical conditions, in metabolic states, or in conditions inducing stress, cells need an efficient, dynamic system to transfer information. The cellular network largely consists of protein–protein interactions.6–14 It is extensive, and stretches from the extracellular domains of membrane receptors, through the cytoplasm to the nucleus (and other organelles), to turn on/off genes. To gauge the magnitude of the problem, we need to consider that the 10 μm to 100 μm are the measurements across the cell. However, signals do not travel across; instead, they go through loops (forward and backward),15,16 and complex and intricate schemes, which involve a large number of inter-connected events making those distances still larger.17How do signals travel over such distances?
The signaling cascade can be initiated by a signaling molecule (such as hormone, growth factor, an extra-cellular matrix component like fibronectin, cytokine, chemokine, neurotransmitter, neurotrophin, like nerve growth factor) or a ligand, interacting with the extra-cellular domains of a transmembrane receptor.18 Binding perturbs the domains. The local perturbation at the interaction sites propagates through the membrane-spanning segment of the receptor,19 and changes the conformation of the intra-cellular domains in the cytoplasm, and in this way conveys the signal from outside the cell. Some small signaling messengers like steroids can diffuse through the cell membrane and activate (or repress) the cellular response by stimulating the binding of their receptors to the regulatory DNA elements of steroid-responsive genes.20–23 While signaling may also be initiated inside the cell, for example following some mutational events, most signaling pathways are initiated by stimulation which arises by external morphogens.24–26 In all cases, a cascade of signaling events which takes place through multiple consecutive or concomitant interactions with other proteins may follow. Eventually, the signals could cross the nuclear membrane to trigger or inhibit gene activation.27 They can also lead to phosphorylation, ubiquitination, or other post-translational modification events; epigenetic events; replication; etc.28,29 In this way, a small signal can dynamically result in a large response, which may involve a change in the cell, either in the expression of the DNA in the nucleus or in the activity of enzymes in the cytoplasm. Signaling time scales vary: from milliseconds (for ion flux),30 minutes (for protein- and lipid-mediated kinase cascades), hours, or days (for steroid action and gene expression).23,31,32
Here, we posit that the propagation of signals over such distances is helped by three key factors: (i) the hierarchical spatial and modular organization of the cellular network which facilitate signaling across the global network of the cell (Fig. 1); (ii) the sequences of some key regions of proteins, such as linkers or loops which have been pre-encoded by evolution to facilitate signaling by increasing the populations of conformations which are ‘correctly’ oriented with respect to each other; (iii) compact interactions between proteins to facilitate propagation from one protein to another via conformational disorder.
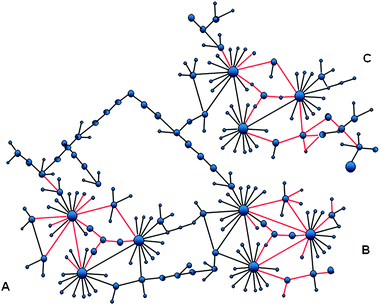 |
| Fig. 1 The modular organization of the cellular network can facilitate efficient signaling and cellular response. A node is a protein; an edge a protein–protein interaction. Spatially co-localized proteins are shown with the edges colored in red. Proteins in the module share a function. There are three modules in this network. Many of the interactions within the modules are co-localized in the cell; but no inter-modular interactions. Co-localization saves time because it avoids diffusion–collision in the crowded cellular environment. The most efficient co-localization is via covalent linkage; that is, gene fusion events. Protein–protein interaction is another way to co-localize proteins. | |
The three key factors that facilitate long distance communication in the
cell
:
(i) Hierarchical cellular network and co-localized functional modules.
The cellular network is hierarchical, and is constructed from modules. Proteins sharing a function are in the same module; however, not all proteins sharing a function are in the same module, and proteins with the same function can also be in other modules, in different combinations. The spatial co-localization33 avoids the collision and diffusion time of proteins and metabolites, which otherwise could be far away. It provides for more efficient feedback signaling and loops, which relate to information from a previous upstream pathway event to subsequent modification of the current events and back, relating downstream events to upstream ones.15,16,18 In addition, because modules often consist of large assemblies, the allosteric propagation transmits more rapidly. Both the feedforward path, which describes the signal propagation from the input to the output, and the feedback, which describes signal propagation in the reverse sense34 will be faster in a co-localized module.35,36 The modular organization is further helped by the crowded cell environment.37–41Fig. 1 illustrates a schematic diagram of this concept.
(ii) The sequences of single chain proteins may be pre-encoded to achieve shorter time scales even in large conformational changes by populating a series of states that avoid high barriers.
A common view holds that signaling proceeds through allosteric propagation, and that the signal can be amplified with each step of the cascade.17,42 The allosteric effect is initiated by some perturbation event: binding of some ligand, covalent change, etc.17,43–54 Binding perturbs the structure because surface atoms that previously were in contact with water or with other atoms in the protein are now in contact with ligand atoms. This local change in atomic interactions forces additional changes in atom–atom contacts in the binding site to accommodate these newly formed interactions. These changes create strain energy, because the binding site does not have favored interactions with the next layer of atoms. In this way, the strain energy keeps propagating across the structure. Propagation is through multiple pathways, major and minor. A covalent change such as that taking place by mutational event55 or post-translational modification works in a similar manner: in both cases, a side-chain is modified. It can get bulkier or smaller; it can introduce a charge or remove a charge. Thus, it can either create steric hindrance or ‘holes’; it can also change the electrostatic environment. All will perturb the structure, and create strain energy, which will propagate.43 Because allosteric propagation takes place through a change in atomic van der Waals contacts, propagation will be faster in compact environments. The interiors of folded domains are typically compact, with a strong hydrophobic core,56–58 permitting such propagation. We have recently argued that in multi-domain proteins, successive conformational states along major allosteric propagation pathways are pre-encoded in the linker sequence where each state is encoded by the previous one.59 The barriers between these states which are hierarchically populated are lower, achieving faster time scales even for large conformational changes. We also posited that evolution optimized the linker sequences and lengths for efficiency, which may explain why mutations in linkers can affect protein function.59 For fast time scales between triggering events and cellular response, which in multi-domain proteins typically involve a large conformational change, flexibility on its own may not constitute a good solution; the orientation of the domains with respect to each other needs to be optimal for function.
(iii) Interfaces of conformationally disordered proteins can help efficient signaling.
To propagate in the cellular network, signals need to cross smoothly between molecules, as if they were a single molecule. Intrinsically disordered proteins fit this bill. Disordered proteins exist in two states: unfolded on their own; or folded and bound. They get stabilized upon binding because they create a hydrophobic core at their interfaces,60–66 similar to the core that exists in folded domains.67 Comparison of the interface sizes of several disordered and stable (ordered) proteins in their complexed states has established that disordered proteins tend to have large interface-to-surface ratios whereas stable proteins have smaller ratios.61,62,68 Disordered monomers can be distinguished from ordered monomers on the basis of the per-residue surface and interface areas, which are significantly larger for disordered proteins.61,62Signaling proteins frequently have disordered domains (or segments) which fold upon binding.69,70 The large, tight interfaces that they create allow efficient signal propagation across proteins sharing a pathway. We propose that given the complexity of the cellular network and the immense distances that need to be spanned, evolution has made use of this property. This could be a main reason for the increasing disorder during evolution.71 The increasing complexity of the network in higher organisms increased the number of interactions and thus their regulation and control, i.e. increased number of modules, pathways, and loops.
The signal can be amplified with each step of the cascade
Above, we suggested that rapid signaling across the cell is helped by three factors: (i) the network organization, which is hierarchical and modular; (ii) pre-encoded sequences in regions which can govern the conformational transitions in protein molecules, such as linkers or loops; and (iii) conformational disorder which helps by creating tightly packed interfaces and in this way facilitate signaling across protein–protein interfaces. Nonetheless, the distances are large, and a large number of interactions may need to take place starting from the extra-cellular stimulation event to regulation of gene activation. In addition, the ability to modulate the signal is important, because it affords further regulation and control in higher organisms. Allostery is a key factor in such modulation.
Allostery is a phenomenon that reflects the behavior of the ensemble. An allosteric event always changes, or shifts the populations of the states: rare, high energy states can become more populated. Allosteric events that are functionally-relevant increase the populations of those states where the ligand binding site is in a binding-ready conformation. This can take place through the population shift of the network as a consequence of a chain of events; it can be enhanced by additional allosteric events such as binding of a small molecule, metabolite, or a post-translational modification, such as phosphorylation, glycosylation, methylation, nitrosylation, etc. Moreover, numerous proteins, such as p53 and histones, present a large number of combinations of post-translational modifications, which can be manifested in alteration of preferred states. However, not all such events relate to allosteric effects; for example, phosphorylation may be recognized patterns for other protein factors either to bind or not to bind, if these events blocked the active sites.17
Intrinsically disordered proteins and conformational selection
Currently the proposition that binding takes place through a conformational selection and population shift mechanism60,72,73 is widely accepted.74–76 According to this mechanism that we have proposed over a decade ago, the protein exists in a broad range of conformational states. Among these, there could be ones which are complementary to a ligand's shape and chemistry even though they may have a low population. The ligand will preferentially bind these states. Binding will stabilize them. This will lead to a population shift toward these conformers, which will propagate the binding reaction. The main difference between intrinsically disordered proteins and folded proteins is in the stability: while folded proteins are stable on their own in solution, this is not the case for disordered proteins, which are at the extreme end of the stability spectrum. The fact that no structure is observed (which is why they are called ‘disordered’) implies that these proteins exist in a broad range of states where the stabilities of the states are quite similar and the barriers between the states are low.60,77,78 Nonetheless, all proteins follow the same physical principles, and the basic mechanisms are universal. Intrinsically disordered proteins also have states which are preferentially selected for binding.60 While the populations of these states are small which makes them unobservable by experiments, thus evoking an ‘induced fit’-type mechanism, they nevertheless exist, and are stabilized upon binding. With the increased power of experimental techniques, particularly NMR, these rare states are becoming observed, which substantiates a selection mechanism. Their lack of stability on their own implies small hydrophobic cores and typically extended states.77–80 The stability is gained upon interaction.80–85 Moreover, disordered proteins present ensembles separated by low barriers, which implies that they sample significantly more conformational states, thus also signaling at higher rates.
We further note that the ‘induced fit’-type mechanism generally takes place if the ligand concentration or the affinity is very high. Because the kinetics of conformational selection is slower than that of the induced fit, due to the need to overcome barriers, one observes the induced fit, which may lead to a mis-assignment of the mechanism.
Conclusions
Signaling is complex; it involves an intricate web of communication that governs the cellular activities and coordinates the diverse cell functions. Cells need to perceive and respond to their microenvironment: this is the basis of development, tissue repair, immunity and homeostatis. Errors in cellular information processing can lead to disease. While much of the focus has been on individual parts of cell signaling pathways, putting the parts in the framework of the system helps in the understanding of the underlying structure of the cell signaling networks, how the information is transmitted, and how changes in the networks may affect the transmission and flow of information. Networks are complex systems; this is not only because of the large number of modules and interactions that they contain. In particular, this complexity derives from their fundamental dynamic properties. Cellular networks should be inherently dynamic because cells need to differentially respond under different stages of organism development, stress, and overall eternally changing conditions. This implies that to study network organization, efficient methods for detecting network ‘communities’ and community overlaps are essential.36,86,87 Concentration is a key factor in affecting network dynamics. For example, a high concentration of a transcription factor will populate all chromatin-exposed DNA response elements (REs); on the other hand, if the concentration is limited, only high affinity REs will be populated. Analysis of the cell signaling networks requires experimental and theoretical approaches. These would help in assembling, organizing and making sense of the signaling data. Within this framework, a key challenge is to understand how cells exploit the individual units for efficient communication given cellular complexity and sheer sizes. Here we posited that evolution has not only optimized the conformational ensembles of large loops, linkers and disordered regions to facilitate signaling; in addition, it made use of the series of conformational states and their dynamics for signaling.
Signaling can be initiated by a signaling molecule which binds to a receptor protein. Signaling is a multistep pathway which allows signal amplification. Amplification takes place because some of the molecules in a pathway can transmit the signal to multiple molecules of the next component in the cascade. The outcome can be a large number of activated molecules at the end of the pathway. In this way a small number of extracellular signal molecules can produce a large cellular response. Pathways can relay signals from the extracellular space to the nucleus. However, to be functionally relevant, signaling should take place over short time scales. Here we asked how signal propagation can take place over such long distances from the binding of the signaling molecule to the extra-cellular domain of a receptor to a cellular response, which often involves activation/repression of gene expression.
We proposed that three major components help in efficient signaling: the hierarchical modular functional organization of the cellular network; the amino acid sequences of some regions of proteins, which play key roles in allosteric transitions, such as linkers or loops; and compact interactions between proteins, which may take place particularly via intrinsically disordered proteins. These could facilitate allosteric propagation.
Acknowledgements
I am grateful to my group and collaborators, for all the discussion that we have had over the years. This project has been funded in whole or in part with Federal funds from the National Cancer Institute, National Institutes of Health, under contract number HHSN261200800001E. The content of this publication does not necessarily reflect the views or policies of the Department of Health and Human Services, nor does mention of trade names, commercial products, or organizations imply endorsement by the U.S. Government. This research was supported (in part) by the Intramural Research Program of the NIH, National Cancer Institute, Center for Cancer Research.
References
-
E. B. Wilson, The karyoplasmic ratio, in The Cell in Development and Heredity, The Macmillan Company, New York, 1925, pp. 727–733 Search PubMed.
- T. Cavalier-Smith, Ann. Bot., 2005, 95, 147–175 CrossRef CAS.
- P. Jorgensen, N. P. Edgington, B. L. Schneider, I. Rupes, M. Tyers and B. Futcher, Mol. Biol. Cell, 2007, 18, 3523–3532 CrossRef CAS.
- F. R. Neumann and P. Nurse, J. Cell Biol., 2007, 179, 593–600 CrossRef CAS.
- M. D. Huber and L. Gerace, J. Cell Biol., 2007, 179, 583–584 CrossRef CAS.
- C. J. Tsai, S. L. Lin, H. J. Wolfson and R. Nussinov, Crit. Rev. Biochem. Mol. Biol., 1996, 31, 127–152 CrossRef CAS.
- O. Keskin, B. Ma, K. Rogale, K. Gunasekaran and R. Nussinov, Phys. Biol., 2005, 2, S24–S35 CrossRef CAS.
- N. Tuncbag, A. Gursoy and O. Keskin, Phys. Biol., 2011, 8, 03500 CrossRef.
- J. G. Lees, J. K. Heriche, I. Morilla, J. A. Ranea and C. A. Orengo, Phys. Biol., 2011, 8, 035008 CrossRef CAS.
- S. Navlakha and C. Kingsford, PLoS Comput. Biol., 2011, 7, e1001119 CAS.
- A. Jaimovich and N. Friedman, Curr. Opin. Biotechnol., 2011, 22, 87–93 CrossRef CAS.
- S. Lievens, S. Eyckerman, I. Lemmens and J. Tavernier, Expert Rev. Proteomics, 2010, 7, 679–690 CrossRef CAS.
- J. R. Perkins, I. Diboun, B. H. Dessailly, J. G. Lees and C. Orengo, Structure, 2010, 18, 1233–1243 CrossRef CAS.
- K. Kaizu, S. Ghosh, Y. Matsuoka, H. Moriya, Y. Shimizu-Yoshida and H. Kitano, Mol. Syst. Biol., 2010, 6, 415 CrossRef.
- M. C. Good, J. G. Zalatan and W. A. Lim, Science, 2011, 332, 680–686 CrossRef CAS.
- S. G. Peisajovich, J. E. Garbarino, P. Wei and W. A. Lim, Science, 2010, 328, 368–372 CrossRef CAS.
- B. Ma and R. Nussinov, Proc. Natl. Acad. Sci. U. S. A., 2009, 106, 6887–6888 CrossRef CAS.
- S. E. Acuner Ozbabacan, A. Gursoy, O. Keskin and R. Nussinov, Curr. Opin. Drug Discovery Dev., 2010, 13, 527–537 Search PubMed.
- C. J. Tsai, A. Del Sol and R. Nussinov, Mol. BioSyst., 2009, 5, 207–216 RSC.
- J. Wollam and A. Antebi, Annu. Rev. Biochem., 2011, 80, 885–916 CrossRef CAS.
- S. H. Meijsing, M. A. Pufall, A. Y. So, D. L. Bates, L. Chen and K. R. Yamamoto, Science, 2009, 324, 407–410 CrossRef CAS.
- H. Gronemeyer and W. Bourguet, Sci. Signaling, 2009, 73, pe34 CrossRef.
- R. W. Chow, D. J. Handelsman and M. K. Ng, Endocrinology, 2010, 151, 2411–2422 CrossRef CAS.
- A. Gallet, Trends Cell Biol., 2011, 21, 238–246 CrossRef CAS.
- A. F. Schier, Cold Spring Harbor Perspect. Biol., 2009, 1, a003459 CrossRef.
- E. Kutejova, J. Briscoe and A. Kicheva, Curr. Opin. Genet. Dev., 2009, 19, 315–322 CrossRef CAS.
- M. D. Galbraith and J. M. Espinosa, Curr. Opin. Genet. Dev., 2011, 21, 160–166 CrossRef CAS.
- X. Yan and Y. G. Chen, Biochem. J., 2011, 434, 1–10 CrossRef CAS.
- V. W. Zhou, A. Goren and B. E. Bernstein, Nat. Rev. Genet., 2011, 12, 7–18 CrossRef CAS.
- M. L. Tierney, Biochim. Biophys. Acta, Biomembr., 2011, 1808, 667–673 CrossRef CAS.
- D. G. Spiller, C. D. Wood, D. A. Rand and M. R. White, Nature, 2010, 465, 736–745 CrossRef CAS.
- J. M. Ankers, D. G. Spiller, M. R. White and C. V. Harper, Curr. Opin. Biotechnol., 2008, 19, 375–380 CrossRef CAS.
- J. Kuriyan and D. Eisenberg, Nature, 2007, 450, 983–990 CrossRef CAS.
- C. O. Tan and J. A. Taylor, J. Physiol., 2011, 589(Pt 8), 1867 CAS.
- A. Kovács, R. Palotai, M. S. Szalay and P. Csermely, PLoS One, 2010, 5, e12528 Search PubMed.
- P. Ronhovde and Z. Nussinov, Phys. Rev. E: Stat., Nonlinear, Soft Matter Phys., 2010, 81(4 Pt 2), 046114 CrossRef.
- R. J. Ellis, Trends Biochem. Sci., 2001, 26, 597–604 CrossRef CAS.
- P. Tompa and P. Csermely, FASEB J., 2004, 18, 1169–1175 CrossRef CAS.
- J. A. Dix and A. S. Verkman, Annu. Rev. Biophys., 2008, 37, 247–263 CrossRef CAS.
- H. X. Zhou, G. Rivas and A. P. Minton, Annu. Rev. Biophys., 2008, 37, 375–397 CrossRef CAS.
- W. Wang, W. X. Xu, Y. Levy, E. Trizac and P. G. Wolynes, Proc. Natl. Acad. Sci. U. S. A., 2009, 106, 5517–5522 CrossRef CAS.
- R. G. Smock and L. M. Gierasch, Science, 2009, 324, 198–203 CrossRef CAS.
- A. del Sol, C. J. Tsai, B. Ma and R. Nussinov, Structure, 2009, 17, 1042–1050 CrossRef CAS.
- C. J. Tsai, A. del Sol and R. Nussinov, J. Mol. Biol., 2008, 378, 1–11 CrossRef CAS.
- Q. Cui and M. Karplus, Protein Sci., 2008, 17, 1295–1307 CrossRef CAS.
- M. S. Formaneck, L. Ma and Q. Cui, Proteins, 2006, 63, 846–867 CrossRef CAS.
- G. Maksay, Prog. Biophys. Mol. Biol., 2011 Search PubMed , Jan 9.
- S. R. Tzeng and C. G. Kalodimos, Curr. Opin. Struct. Biol., 2011, 21, 62–67 CrossRef CAS.
- G. Kar, O. Keskin, A. Gursoy and R. Nussinov, Curr. Opin. Pharmacol., 2010, 10, 715–722 CrossRef CAS.
- P. I. Zhuravlev and G. A. Papoian, Q. Rev. Biophys., 2010, 43, 295–332 CrossRef CAS.
- G. Zocchi, Annu. Rev. Biophys., 2009, 38, 75–88 CrossRef CAS.
- M. J. Whitley and A. L. Lee, Curr. Protein Pept. Sci., 2009, 10, 116–127 CrossRef CAS.
- L. Swint-Kruse and K. S. Matthews, Curr. Opin. Microbiol., 2009, 12, 129–137 CrossRef CAS.
- D. M. Leitner, Annu. Rev. Phys. Chem., 2008, 59, 233–259 CrossRef CAS.
- N. Sinha and R. Nussinov, Proc. Natl. Acad. Sci. U. S. A., 2001, 98, 3139–3144 CrossRef CAS.
- E. E. Lattman, K. M. Fiebig and K. A. Dill, Biochemistry, 1994, 33, 6158–6166 CrossRef CAS.
- H. S. Chan, S. Bromberg and K.
A. Dill, Philos. Trans. R. Soc. London, Ser. B, 1995, 348, 61–70 CrossRef CAS.
- K. A. Dill, K. M. Fiebig and H. S. Chan, Proc. Natl. Acad. Sci. U. S. A., 1993, 90, 1942–1946 CrossRef CAS.
- B. Ma, C. J. Tsai, T. Haliloglu and R. Nussinov, Structure, 2011 DOI:10.1016/j.str.2011.06.002.
- C. J. Tsai, B. Ma, Y. Y. Sham, S. Kumar and R. Nussinov, Proteins, 2001, 44, 418–427 CrossRef CAS.
- K. Gunasekaran, C. J. Tsai and R. Nussinov, J. Mol. Biol., 2004, 341, 1327–1341 CrossRef CAS.
- K. Gunasekaran, C. J. Tsai, S. Kumar, D. Zanuy and R. Nussinov, Trends Biochem. Sci., 2003, 28, 81–85 CrossRef CAS.
- S. Brocca, L. Testa, F. Sobott, M. Samalikova, A. Natalello, E. Papaleo, M. Lotti, L. De Gioia, S. M. Doglia, L. Alberghina and R. Grandori, Biophys. J., 2011, 100, 2243–2252 CrossRef CAS.
- Y. Wang, J. C. Fisher, R. Mathew, L. Ou, S. Otieno, J. Sublet, L. Xiao, J. Chen, M. F. Roussel and R. W. Kriwacki, Nat. Chem. Biol., 2011, 7, 214–221 CrossRef CAS.
- Z. Zhang and W. Wriggers, Biochemistry, 2011, 50, 2144–2156 CrossRef CAS.
- T. Mittag, L. E. Kay and J. D. Forman-Kay, J. Mol. Recognit., 2010, 23, 105–116 CAS.
- C. J. Tsai and R. Nussinov, Protein Sci., 1997, 6, 1426–1437 CrossRef CAS.
- B. Mészáros, P. Tompa, I. Simon and Z. Dosztányi, J. Mol. Biol., 2007, 372, 549–561 CrossRef.
- M. M. Babu, R. van der Lee, N. S. de Groot and J. Gsponer, Curr. Opin. Struct. Biol., 2011 Search PubMed , Apr 20.
- J. Liu, N. B. Perumal, C. J. Oldfield, E. W. Su, V. N. Uversky and A. K. Dunker, Biochemistry, 2006, 45, 6873–6888 CrossRef CAS.
- A. Tóth-Petróczy, C. J. Oldfield, I. Simon, Y. Takagi, A. K. Dunker, V. N. Uversky and M. Fuxreiter, PLoS Comput. Biol., 2008, 4, e1000243 Search PubMed.
- B. Ma, S. Kumar, C. J. Tsai and R. Nussinov, Protein Eng., Des. Sel., 1999, 12, 713–720 CrossRef CAS.
- S. Kumar, B. Ma, C. J. Tsai, N. Sinha and R. Nussinov, Protein Sci., 2000, 9, 10–19 CrossRef CAS.
- D. D. Boehr, R. Nussinov and P. E. Wright, Nat. Chem. Biol., 2009, 5, 789–796 CrossRef CAS.
- T. Wlodarski and B. Zagrovic, Proc. Natl. Acad. Sci. U. S. A., 2009, 106, 19346–19351 CrossRef CAS.
- J. Gsponer, J. Christodoulou, A. Cavalli, J. M. Bui, B. Richter, C. M. Dobson and M. Vendruscolo, Structure, 2008, 16, 736–746 CrossRef CAS.
- D. Shortle, Adv. Protein Chem., 2002, 62, 1–23 CrossRef CAS.
- D. Shortle and M. S. Ackerman, Science, 2001, 293, 487–489 CrossRef CAS.
- B. A. Shoemaker, J. J. Portman and P. G. Wolynes, Proc. Natl. Acad. Sci. U. S. A., 2000, 97, 8868–8873 CrossRef CAS.
- Z. T. Campbell, T. O. Baldwin and O. Miyashita, Biophys. J., 2010, 99, 4012–4019 CrossRef CAS.
- C. W. Lee, M. A. Martinez-Yamout, H. J. Dyson and P. E. Wright, Biochemistry, 2010, 49, 9964–9971 CrossRef CAS.
- H. J. Dyson and P. E. Wright, Nat. Rev. Mol. Cell Biol., 2005, 6, 197–208 CrossRef CAS.
- P. E. Wright and H. J. Dyson, Curr. Opin. Struct. Biol., 2009, 19, 31–38 CrossRef CAS.
- T. Mittag, L. E. Kay and J. D. Forman-Kay, J. Mol. Recognit., 2009, 23, 105–116 Search PubMed.
- V. N. Uversky and A. K. Dunker, Biochim. Biophys. Acta, Proteins Proteomics, 2010, 1804, 1231–1264 CrossRef CAS.
- P. Ronhovde and Z. Nussinov, Phys. Rev. E: Stat., Nonlinear, Soft Matter Phys., 2009, 80, 016109 CrossRef.
- G. Palla, I. Derenyi, I. Farkas and T. Vicsek, Nature, 2005, 435, 814–818 CrossRef CAS.
Footnote |
† Published as part of a Molecular BioSystems themed issue on Intrinsically Disordered Proteins: Guest Editor M Madan Babu. |
|
This journal is © The Royal Society of Chemistry 2012 |
Click here to see how this site uses Cookies. View our privacy policy here.