DOI:
10.1039/C5MB00024F
(Opinion)
Mol. BioSyst., 2015,
11, 1487-1493
Why phosphoproteomics is still a challenge
Received
10th January 2015
, Accepted 13th March 2015
First published on 13th March 2015
Abstract
Despite continuous improvements phosphoproteomics still faces challenges that are often neglected, e.g. partially poor recovery of phosphopeptide enrichment, assessment of phosphorylation stoichiometry, label-free quantification, poor behavior during chromatography, and general limitations of peptide-centric proteomics. Here we critically discuss current limitations that need consideration in both qualitative and quantitative studies.
Introduction
Protein activity is mainly modulated by dynamic reversible (and irreversible) post-translational modifications (PTMs).1 Phosphorylation is a reversible PTM involved in numerous regulatory mechanisms in eukaryotic cells, such as cell division, apoptosis, response to extracellular signals and growth factor stimulation,2 and is regulated by the interplay of protein kinases and phosphatases.3,4
The fundamental importance of protein phosphorylation and the continuous development in the field of protein mass spectrometry render phosphoproteomics a valuable tool in current life science, allowing the study of regulatory changes over time and/or between conditions. However, despite its routine use in many laboratories around the world several limitations still have to be faced.5,6
Among those are (i) the analysis of low abundant proteins in the light of the vast dynamic range of cells, (ii) the low stoichiometry of phosphorylation, (iii) potentially impaired digestion efficiency,7 (iv) phosphopeptide losses during sample preparation and chromatography,8–11 (v) impaired ionization efficiency of phosphopeptides, (vi) the peculiar behavior of the labile phosphate group upon collision induced dissociation, often resulting in poor quality MS/MS spectra that can impair both identification of the peptide sequence and the (vii) correct localization of the phosphorylation site(s),12–14 and (viii) the general limitations of peptide-centric proteomics.15
Taken together, these issues can complicate the identification and quantification of phosphopeptides (and particularly phosphorylation sites) compared to their non-phosphorylated counterparts, and can produce wrong, misleading and inaccurate identification and quantification results.
Nevertheless, some issues can be (partially) addressed by improved methods for sample preparation, phosphopeptide enrichment, MS analysis, and computational analysis of MS-derived phosphoprotemics data.16
Here, we discuss current obstacles that can emerge in phosphoproteomic analysis and demonstrate why beside all the advances achieved in recent years, phosphoproteomics is still a challenge.
Sample amount, sample preparation and noise
Phosphorylation is a fast and dynamic process that, like any enzyme-driven biochemical reaction, can be prone to errors.17 Consequently, it is conceivable that within a cell certain levels of protein phosphorylation might occur without major functional relevance. However, for a given site the degree of phosphorylation, i.e. the stoichiometry that reflects how many copies of a protein are phosphorylated at that amino acid at a given time point, might represent a threshold that may distinguish relevant from non-functional phosphorylation events. Many studies use large amounts of protein starting material (often in the mg range) to enrich and identify as many phosphopeptides as possible, often leading to the identification of 10
000s of phosphorylation sites. As information about stoichiometry is almost always missing, it may be argued how many of these phosphorylation sites really derive from specific phosphorylation events, and how many are indeed just ‘noise’ derived from more-or-less random phosphorylation events, without functional consequence. Such random events however may be detected because of the extremely high sensitivity of modern mass spectrometers. If present on (relatively) high abundant proteins, these random events may interfere with the identification of phosphorylation sites on low abundant proteins.
Obtaining temporal profiles of protein phosphorylation upon specific treatment may be one strategy to dissect and interpret the huge amount of data obtained using modern phosphoproteomics approaches.18,19
Moreover, sample preparation steps prior to LC-MS analysis can have a huge impact on the final results. Recently, Mertins et al. investigated the proteome and the phosphoproteome of human ovarian tumor and breast cancer tissue after defined ischemic events. They demonstrated that, while the proteome remains unchanged for 60 min after tissue removal from its blood supply, up to 24% of the phosphoproteome showed rapid phosphorylation changes, particularly in pathways relevant for cancer. Thus, it is recommended to exhaustively examine and optimize protocols for sample collection before analyzing the phosphoproteome.20
Popular sample preparation protocols such as Filter Aided Sample Preparation (FASP) involve the use of urea, which is a potent chaotropic agent.21,22 In aqueous solution urea dissociates over time, a reaction that is accelerated upon heating, such as often done e.g. during the reduction of disulfide bonds. Notably, one of its degradation products, isocyanate reacts with primary amines such as protein N-termini and ε-amines of lysines, inducing in vitro carbamylation. Thus, if used without care urea (e.g. by using non-freshly prepared urea buffers or by heating urea buffers) can easily induce artificial carbamylation of primary amines, thereby reducing identification rates and interfering with several stable isotope labeling strategies that target Lys residues.23 Especially in case of low complexity, samples are sometimes separated using SDS-PAGE followed by silver staining, in-gel digestion and LC-MS analysis in order to identify phosphorylation sites. However, as demonstrated by Gharib et al. certain silver staining protocols can induce artificial sulfation which is almost isobaric to phosphorylation and thus can be easily mistaken for the latter.24
Generally, when looking for quantitative changes in protein phosphorylation upon specific treatment/stimulation of samples, special attention has to be paid to the experimental design in order to (i) guarantee maximal reproducibility, (ii) not induce alterations of phosphorylation profiles, (iii) reduce sample losses, especially for low sample amounts, and (iv) not introduce artificial modifications.
Phosphorylation stoichiometry, digestion efficiency and peptide-centric phosphoproteomics
As mentioned before, during sample preparation in proteomic studies there are initial and crucial steps that directly affect the reliability of the final results. Are the cells effectively lysed and is the activity of endogenous kinases and particularly phosphatases inhibited? Is the sample properly digested? The underlying steps should be carefully controlled, as they are important for the success of proteomics studies.16,25,26
For shotgun proteomics and phosphoproteomics trypsin is the protease-of-choice due to its availability, high specificity and cleavage properties, resulting in peptides with homogenous properties such as an average length of ∼14 amino acids.25,27 However, proteolytic cleavage can be strongly impaired in proximity of phosphoamino acids, leading to missed cleavage sites and complicating phosphoproteome analysis. We recently evaluated this effect in detail using synthetic phosphopeptides, demonstrating that it not only depends on the position of the phosphoamino acid, but on its very nature (Ser/Thr/Tyr). Thus, reduced digestion efficiency can be partially compensated by using optimized digestion conditions, such as the addition of organic solvents or simply by increasing the amount of protease.7 Indeed, it is recommended to make use of relative high concentrations of trypsin up to 1
:
20 (enzyme
:
protein, wt
:
wt), whereas often used concentrations of 1
:
50 or even 1
:
100 can lead to a substantially reduced cleavage efficiency and therefore loss of sensitivity.
Notably, this interference of proteolytic digestion by protein phosphorylation can strongly affect the determination of phosphorylation stoichiometry. To determine, for a given protein, how many copies/which share is phosphorylated at a given position and time point is still one of the most challenging issues in the field of phosphoproteomics. One strategy to assess the degree of phosphorylation is based on the usage of synthetic stable isotope labeled analogues of endogenous phosphopeptides and their non-phosphorylated counterparts, in order to absolutely quantify both versions and thus assess the phosphorylation degree.28 However, the impairment of digestion can strongly interfere with this strategy. For affected phosphorylation sites the digestion can be up to 10 times more efficient in absence of a phosphorylation, such that the mere comparison of phosphorylated and non-phosphorylated fully tryptic peptides can lead to wrong results. In other strategies, the sample is split in two aliquots, one of which is treated with phosphatase prior to LC-MS. The increase of the non-phosphorylated peptide signal upon treatment with phosphatase can then be used to deduce the degree of phosphorylation.29 But also here impaired digestion efficiency will affect the quantitative results and even more importantly, after phosphatase treatment different phospho-isoforms of the same peptide will contribute to the same non-phosphorylated signal and therefore cannot be distinguished. This shortcoming is a direct consequence of the general limitations of peptide-centric shotgun proteomics. Although often neglected, in phosphoproteomics peptides rather than phosphorylation sites are quantified. As a consequence the up-regulation of a specific phosphopeptide does not necessarily correlate with an increase of the particular phosphorylation site, as depicted in Fig. 1. Moreover, in conventional bottom-up peptide-centric proteomics is it unclear how many of the different phosphorylation sites/peptides often detected for a single protein really occur on the very same protein molecule.
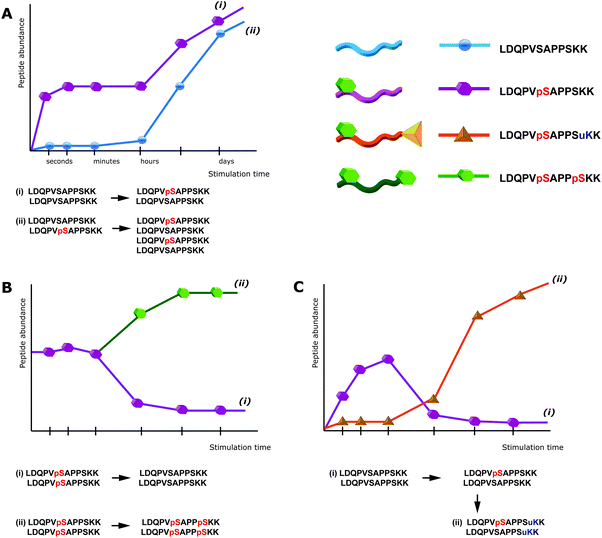 |
| Fig. 1 Peptide-centric bottom-up proteomics can lead to ambiguous results. (a) Upon stimulation of cells an increase in the signal of a specific phosphopeptide can be induced by: phosphorylation of the previously non-modified amino acid, i.e. an increased phosphorylation stoichiometry (i), or especially after prolonged time of stimulation an increased expression of the protein itself, whereas the stoichiometry remains unchanged (ii). (b) The down-regulation of a specific phosphopeptide does not necessarily result from a decrease in the abundance of the corresponding phosphorylation site (i), but could be induced by the phosphorylation of a another residue within the same peptide, leading to an increase in the intensity of the doubly-phosphorylated peptide (ii), that might even escape detection via mass spectrometry. (c) PTM crosstalk can further interfere with the detection of phosphorylation sites. An apparent down-regulation of phosphorylation (i) might be the consequence of an additional PTM on the same peptide (ii), this, however, would usually escape detection. pS = phosphorylated Ser, uK = ubiquitinated Lys. | |
One strategy to address these issues may be the use of (multiple) alternative proteases30,31 and so-called middle-down proteomics, where rather large peptides of ≥50 amino acids are analyzed.32 Top-down proteomics of intact proteins might be the method-of-choice for analyzing samples of low complexity or single proteins, but as enrichment methods are comparably inefficient on the protein level, large-scale top-down phosphoproteomics will require substantial improvements in order to deal with the problems of dynamic range and low stoichiometry. Nevertheless, the use of (multiple) alternative proteolytic enzymes also comes with the advantage that areas of the proteome that have been inaccessible due to generation of too long or too short peptides, may be analyzed by shotgun proteomics. Already in 2001, Lehman and co-workers speculated that trypsin may not be the method-of-choice for analyzing protein phosphorylation – as it can often generate large peptides with low quality MS/MS spectra – and demonstrated that the broad specificity of the protease elastase can yield better results. In this context, we evaluated whether under optimized conditions the non-specific serine protease subtilisin may be useful for large-scale phosphoproteomics. Indeed, subtilisin enables reproducible digestion and furthermore grants access to new phosphorylation sites that are “hidden” from conventional tryptic digestion.33
However, the peptide centric nature of typical bottom-up workflows might not allow completely solving the aforementioned problems for large-scale phosphoproteomics.
The need for enrichment
The substoichiometric abundance of protein phosphorylation has led to the development of specific enrichment techniques, which are nowadays typically applied on the peptide level in order to specifically target phosphopeptides. Among those, the most popular protocols make use of immobilized metal ion affinity chromatography (IMAC),34–36 metal oxide affinity chromatography (MOAC) such as TiO2,37 strong cation exchange chromatography (SCX),38 strong anion exchange chromatography (SAX)39,40 electrostatic repulsion–hydrophilic interaction chromatography (ERLIC),41,42 phosphotyrosine immunoprecipitation,43 Ti4+/Zr4+-IMAC44,45 or combinations of those. Novel methods and protocols for enrichment are frequently published, however, all these methods have in common that they work best when combined with optimized and reasonable sample preparation and LC-MS analysis workflows. Then, in combination with state-of-the-art instrumentation, phosphopeptide yields in the range of 100 sites per 1 μg of sample can be obtained.42,46,47 In general, the aforementioned methods for enrichment seem to have different preferences to more efficiently enrich for specific subsets of the phosphoproteome and thus may be combined to yield a higher coverage. Whereas phosphopeptide recovery varies between the different methods and respective methodical variations, and furthermore depends on the sample and its complexity, even under optimal conditions recovery is clearly below 50% for most phosphopeptides, as can be derived from spike-in experiments with synthetic peptides. Particularly methods that primarily enrich but not additionally fractionate, such as IMAC and MOAC should be combined with subsequent (or prior) fractionation e.g. by Hydrophilic Interaction Liquid Chromatography (HILIC), SCX18 or high pH RP48 to increase the number of identified phosphopeptides. Notably, ERLIC provides both, highly selective enrichment and fractionation (e.g. separating singly from multiply phosphorylated peptides) at once.42
Generally, phosphopeptide enrichment is accompanied by the loss of information about the non-phosphorylated counterpart and therefore cannot readily be used for determining stoichiometry.
Challenges in separation, fragmentation and identification of phosphopeptides
Several properties of phosphopeptides complicate their analysis by LC-MS. Metal ions on the surfaces of the HPLC flow path, or within solvents can lead to losses due to the formation of phosphopeptide–metal ion complexes, which can be partially circumvented by the addition of EDTA to the LC buffers.49 In this context, Lehmann and co-workers investigated in detail the effect of additives given to the sample before LC-MS analysis. They demonstrated that citrate can overcome the adsorption of phosphopeptides to surfaces and is compatible with LC-MS.10
Especially multi-phosphorylated peptides can smear substantially during reversed phase chromatography, which in severe cases can dramatically impair their identification (see Fig. 2).
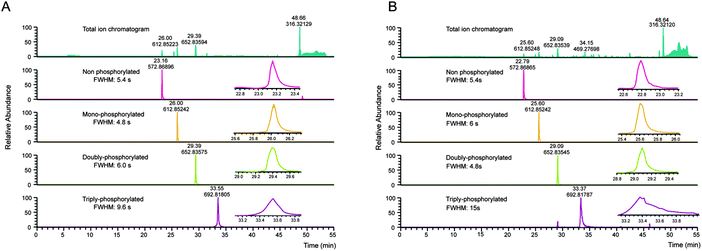 |
| Fig. 2 Column performance affects detection of multiply phosphorylated peptides. A set of synthetic peptides, KVTQTIITLK, KVtQTIITLK, KVtQTIItLK, KVtQtIItLK (lower case letters indicate phosphorylation sites) was analyzed by LC-MS on the same instrument on a new main column (a), and after 1.5 months of use (b). Even with an optimal LC system (a), the full width at half maximum (FWHM) increases slightly with the number of phosphorylation sites. However, with column ageing (b), the FWHM of the triply-phosphorylated peptide is further increasing, resulting in a reduced maximum intensity and therefore loss-of-sensitivity. While the given example still shows a relatively good separation considering the number of phosphorylation sites, non-optimal LC conditions can lead to FWHM in the min range and consequently to a complete absence of multiphosphorylated peptide identifications in complex samples. | |
Another frequently discussed issue is the ionization efficiency of phosphopeptides. Using synthetic peptides Steen et al. showed that lower ionization/detection efficiencies of phosphopeptides compared to their counterparts could not be substantiated. In LC-MS some phosphopeptides showed better ionization/detection than their cognates.50 In contrast, Choi et al. demonstrated that ionization/detection efficiency negatively correlates with the number of phosphorylation sites,11 which, however, could be a consequence of poor chromatography. Indeed, both studies agree that in complex mixtures with non-phosphorylated peptides, phosphopeptides show reduced ionization efficiencies.
Another issue that complicates phosphopeptide identification is the labile character of the phosphoester bond which, depending on the type of fragmentation used, can severely impair and alter the fragmentation behavior when compared to non-modified peptides.13 Still, the most popular technique to induce fragmentation of peptide ions is collision-induced dissociation (CID),51 which can be categorized into two subclasses, ion trap CID and beam-type CID,52 as conducted in quadrupoles. In ion traps, upon excitation peptides experience hundreds of light collisions with an inert gas, each collision transferring internal energy to the peptide. As the phosphoester bond is highly labile, it tends to break first, leading to a so-called neutral loss of phosphoric acid (98 Da) from the precursor. Phosphopeptide CID MS/MS spectra are often dominated by this neutral loss signal and show only minor fragmentation. Moreover, ion trap CID is often accompanied by additional neutral losses of water. In beam-type CID (also called higher energy CID or HCD) an electrical potential is used to accelerate peptide ions towards the inert gas, leading to fewer but higher energy collisions. Consequently, the presence of the neutral loss precursor is substantially reduced leading to richer fragmentation patterns.
Beside the fragmentation mode, the occurrence of the neutral loss precursor depends on peptide sequence and charge state (more prominent for +2 than +3 charge states), with phosphotyrosine showing only minor neutral losses of 80 Da, corresponding to meta-phosphoric acid. Beside the neutral loss of the precursor ion, fragment ions containing the phosphoamino acid can be present as intact (i.e. with phospho moiety) and neutral loss form, both in ion trap and beam-type CID. Notably, neutral losses of phosphopeptides reduce spectrum quality, as the overall signal intensity is distributed over more fragment ions when compared to non-phosphorylated peptides, therefore also complicating spectrum identification. Indeed, for two peptides sharing the same sequence but having different phosphorylation sites, such as IsAS and ISAs (lowercase indicating the phosphoamino acid), the occurrence of neutral losses can complicate the exact localization of the phospho moiety substantially, as demonstrated in Table 1.
Table 1 Under CID conditions, different phospho-isoforms can produce the same m/z for different b- and y-ions. The two phosphorylated peptides IsAS and ISAs can produce b- and y-ions that are isobaric but have a different origin (labeled in bold), owing to the neutral losses of phosphoric acid (H3PO4) and water (H2O). For instance, IsAS y1-H2O and ISAs y1-H3PO4 at 88.0393 m/z are not distinguishable. Besides, both peptides also share isobaric b- and y-ions of the same origin (italicized). Theoretical fragment masses were calculated using MS Product (http://prospector.ucsf.edu/prospector/cgi-bin/msform.cgi?form=msproduct)
IsAS |
ISAs |
m/z |
Fragment ion |
m/z |
Fragment ion |
88.0393
|
y1-H2O
|
88.0393
|
y1-H3PO4
|
106.0499 |
y1 |
159.0764
|
y2-H3PO4
|
159.0764
|
y2-H2O
|
183.1128
|
b2-H2O
|
177.0870 |
y2 |
186.0162 |
y1 |
183.1128
|
b2-H3PO4
|
201.1234 |
b2 |
246.1084
|
y
3
-H
3
PO
4
|
246.1084
|
y
3
-H
3
PO
4
|
254.1499
|
b
3
-H
2
O
|
254.1499
|
b
3
-H
2
O
|
281.0897 |
b2 |
257.0533 |
y2 |
326.0748
|
y
3
-H
2
O
|
272.1605 |
b3 |
344.0853
|
y
3
|
326.0748
|
y
3
-H
2
O
|
352.1268 |
b3 |
344.0853
|
y
3
|
359.1925
|
MH-H
3
PO
4
|
359.1925
|
MH-H
3
PO
4
|
439.1588 |
MH-H2O |
439.1588 |
MH-H2O |
457.1694 |
MH |
457.1694 |
MH |
In contrast, electron transfer dissociation (ETD) can preserve labile side chain modifications such as phosphorylation53 and can therefore facilitate phosphospeptide identification. However, ETD fragmentation works best with charge states above +2, is less efficient than CID and thus slightly less sensitive. The recently introduced EThcD combines ETD and HCD to generate both b/y and c/z ions and provides data-rich MS/MS spectra that can yield higher peptide sequence coverage and more confident localization of phosphorylation sites.54
Localization of phosphoamino acids
Although fragmentation modes such as beam-type CID, ETD, and EThcD yield data-rich fragmentation spectra that facilitate the localization of phosphoamino acids, common search algorithms still can mis-localize the phosphorylation site within a given peptide sequence. Thus, in the past decade a couple of algorithms12,18,55–57 that focus on a more reliable localization have been developed and have substantially improved the quality of phosphopeptide identifications. But even with improved algorithms, peptide spectrum matches can be prone to false positive identifications. Thus, phosphorylation site localization can be wrong58 and – owing to differences in quality and signal/noise – even redundant MS/MS spectra of the same synthetic phosphopeptide can yield different phosphoamino acid localizations or probabilities. This issue particularly complicates label free quantitative phosphoproteomics, as discussed below.
Importantly, public databases with PTM information can still contain site localizations that either have not been validated with specific algorithms or have not reported certain probabilities, and thus might include a substantial amount of wrong or non-confident phosphorylation sites. With the introduction of guidelines for uploading LC-MS raw data to open repositories59,60 for most proteomics journals, and very recently the possibility for automated and quality controlled reanalysis of such large-scale data sets,61 the quality of data in public databases should improve considerably.
Phosphopeptide quantification
Quantitative phosphoproteomics allows elucidating changes in protein phosphorylation between different samples. Several MS-based quantification methods have been used for this purpose, including chemical and metabolic stable-isotope labeling strategies such as iTRAQ,62 TMT,63 dimethyl or SILAC.64,65 Generally, SILAC is well-suited for quantitative phosphoproteomics in cell culture experiments, however, in contrast to chemical labeling techniques SILAC cannot be directly used for clinical samples such as blood cells, biopsies or primary tissue.19,20
Label free quantification represents an increasingly used approach for quantification, which on the one hand has no general limitations with regard to the number of samples or replicates, but on the other hand demands a great deal of the experimental design and quality control to ensure reproducibility and to avoid the introduction of biases. Primarily label free phosphoproteomics not only requires a reproducible sample preparation and LC-MS analysis with minor deviations in retention times and elution profiles, but furthermore has to deal with the issues of confident phosphorylation site localization. This is particularly challenging, as different phospho-isoforms of the same peptide can have highly similar retention times or even (partially) overlap in their elution profiles, rendering discrimination across multiple runs extremely difficult.66 Moreover, the aforementioned varying and sometimes low recovery of phosphopeptides might be a major issue, as slight variations in peptide signals of already low intensity disproportionately impair quantification reproducibility. Thus, an important step towards more robust label free phosphoproteomics of limited sample amounts might be to further improve phosphopeptide recovery.
Recently, Graaf et al. performed label free phosphoproteomics of 108 samples based on Ti+4-IMAC, analyzed the samples using decision tree-based ion-trap CID or ETD fragmentation to obtain data-rich MS/MS spectra, and performed the data analysis using MaxQuant67 to assess the phosphorylation site localization.68 As such studies demand a great deal of reproducibility and quality control, chemical labeling techniques that allow multiplexing might facilitate the reliable quantification of phosphopeptides across samples.
Conclusion
Here, we described several issues that have to be faced in modern phosphoproteomics in order to generate robust and reliable data. Although some of those have been partially addressed and solved in recent years, they still represent common pitfalls when doing quantitative phosphoproteomics. Owing to the large amount of data generated in current (and past) phosphoproteomics studies and their impact on public protein databases and in the literature, it is imperative that researchers pay special attention to the quality and reliability of the study design, experimental procedures and obtained data. Otherwise, even despite the use of state-of-the-art equipment, protocols, and software tools flawed data can be easily generated. This is particularly important with the advent of clinical studies that (i) are often limited in sample amount, (ii) generally have a much higher variation than the bulk of cell culture-based phosphoproteomic studies, and (iii) consequently are much more demanding in terms of robust protocols and data.
Considering the time, money, and effort spent for studying just a single phosphorylation site and its function, it is mandatory to constantly and critically assess the applied strategies.
Abbreviations
CID | Collision induced dissociation |
HCD | Higher collision induced dissociation |
ETD | Electron transfer dissociation |
SILAC | Stable isotopic label by amino acids in cell culture |
iTRAQ | Isobaric tag for relative and absolute quantification |
LC-MS | Liquid chromatography online mass spectrometry |
IMAC | Immobilized metal ion affinity chromatography |
MOAC | Metal oxide affinity chromatography |
ERLIC | Electrostatic repulsion–hydrophilic interaction liquid chromatography |
HILIC | Hydrophilic interaction liquid chromatography |
SCX | Strong cation exchange chromatography |
EDTA | Ethylenediaminetetraacetic acid |
TMT | Tandem mass tag |
Acknowledgements
The financial support by the Ministerium für Innovation, Wissenschaft und Forschung des Landes Nordrhein-Westfalen is gratefully acknowledged and by the ISAS Integrated Research Project ‘Monitoring Signaling Events’. We furthermore would like thank the Italian Proteomics Association (ItPA) for the great meeting in June 2014. In addition, we also thank our colleges for vivid daily discussions, in particular Stefan Loroch, Oliver Pagel, Clarissa Dickhut and Humberto Gonczarowska Jorge.
References
- J. M. Burkhart, S. Gambaryan, S. P. Watson, K. Jurk, U. Walter, A. Sickmann, J. W. Heemskerk and R. P. Zahedi, Circ. Res., 2014, 114, 1204–1219 CrossRef CAS PubMed
.
- R. H. Newman, J. Zhang and H. Zhu, Front. Genet., 2014, 5, 263 Search PubMed
.
- J. Reinders and A. Sickmann, Proteomics, 2005, 5, 4052–4061 CrossRef CAS PubMed
.
- E. Maes, K. Tirez, G. Baggerman, D. Valkenborg, L. Schoofs, J. R. Encinar and I. Mertens, Mass Spectrom. Rev., 2014 DOI:10.1002/mas.21440
.
- J. Yang, Q. M. Zou, S. X. Cai, G. Guo and Y. H. Zhu, Shengwu Gongcheng Xuebao, 2003, 19, 244–248 CAS
.
- N. Li, Methods Mol. Biol., 2012, 876, 17–32 CAS
.
- C. Dickhut, I. Feldmann, J. Lambert and R. P. Zahedi, J. Proteome Res., 2014, 13, 2761–2770 CrossRef CAS PubMed
.
- J. Kim, D. G. Camp 2nd and R. D. Smith, J. Mass Spectrom., 2004, 39, 208–215 CrossRef CAS PubMed
.
- T. Nakamura, K. T. Myint and Y. Oda, J. Proteome Res., 2010, 9, 1385–1391 CrossRef CAS PubMed
.
- D. Winter, J. Seidler, Y. Ziv, Y. Shiloh and W. D. Lehmann, J. Proteome Res., 2009, 8, 418–424 CrossRef CAS PubMed
.
- H. Choi, H. S. Lee and Z. Y. Park, Anal. Chem., 2008, 80, 3007–3015 CrossRef CAS PubMed
.
- S. A. Beausoleil, J. Villen, S. A. Gerber, J. Rush and S. P. Gygi, Nat. Biotechnol., 2006, 24, 1285–1292 CrossRef CAS PubMed
.
- P. J. Boersema, S. Mohammed and A. J. Heck, J. Mass Spectrom., 2009, 44, 861–878 CrossRef CAS PubMed
.
- A. M. Palumbo and G. E. Reid, Anal. Chem., 2008, 80, 9735–9747 CrossRef CAS PubMed
.
- A. S. Venne, L. Kollipara and R. P. Zahedi, Proteomics, 2014, 14, 513–524 CrossRef CAS PubMed
.
- S. Loroch, C. Dickhut, R. P. Zahedi and A. Sickmann, Electrophoresis, 2013, 34, 1483–1492 CrossRef CAS PubMed
.
- J. A. Ubersax and J. E. Ferrell Jr., Nat. Rev. Mol. Cell Biol., 2007, 8, 530–541 CrossRef CAS PubMed
.
- J. V. Olsen, B. Blagoev, F. Gnad, B. Macek, C. Kumar, P. Mortensen and M. Mann, Cell, 2006, 127, 635–648 CrossRef CAS PubMed
.
- F. Beck, J. Geiger, S. Gambaryan, J. Veit, M. Vaudel, P. Nollau, O. Kohlbacher, L. Martens, U. Walter, A. Sickmann and R. P. Zahedi, Blood, 2014, 123, e1–e10 CrossRef CAS PubMed
.
- P. Mertins, F. Yang, T. Liu, D. R. Mani, V. A. Petyuk, M. A. Gillette, K. R. Clauser, J. W. Qiao, M. A. Gritsenko, R. J. Moore, D. A. Levine, R. Townsend, P. Erdmann-Gilmore, J. E. Snider, S. R. Davies, K. V. Ruggles, D. Fenyo, R. T. Kitchens, S. Li, N. Olvera, F. Dao, H. Rodriguez, D. W. Chan, D. Liebler, F. White, K. D. Rodland, G. B. Mills, R. D. Smith, A. G. Paulovich, M. Ellis and S. A. Carr, Mol. Cell. Proteomics, 2014, 13, 1690–1704 CAS
.
- J. R. Wisniewski, A. Zougman, N. Nagaraj and M. Mann, Nat. Methods, 2009, 6, 359–362 CrossRef CAS PubMed
.
- L. L. Manza, S. L. Stamer, A. J. Ham, S. G. Codreanu and D. C. Liebler, Proteomics, 2005, 5, 1742–1745 CrossRef CAS PubMed
.
- L. Kollipara and R. P. Zahedi, Proteomics, 2013, 13, 941–944 CrossRef CAS PubMed
.
- M. Gharib, M. Marcantonio, S. G. Lehmann, M. Courcelles, S. Meloche, A. Verreault and P. Thibault, Mol. Cell. Proteomics, 2009, 8, 506–518 CAS
.
- J. M. Burkhart, C. Schumbrutzki, S. Wortelkamp, A. Sickmann and R. P. Zahedi, J. Proteomics, 2012, 75, 1454–1462 CrossRef CAS PubMed
.
- S. Gallien, A. Bourmaud and B. Domon, J. Proteome Res., 2014, 13, 2688–2695 CrossRef CAS PubMed
.
- L. Switzar, M. Giera and W. M. Niessen, J. Proteome Res., 2013, 12, 1067–1077 CrossRef CAS PubMed
.
- A. N. Kettenbach, J. Rush and S. A. Gerber, Nat. Protoc., 2011, 6, 175–186 CrossRef CAS PubMed
.
- D. Domanski, L. C. Murphy and C. H. Borchers, Anal. Chem., 2010, 82, 5610–5620 CrossRef CAS PubMed
.
- N. Taouatas, A. F. Altelaar, M. M. Drugan, A. O. Helbig, S. Mohammed and A. J. Heck, Mol. Cell. Proteomics, 2009, 8, 190–200 CAS
.
- J. M. Gilmore, A. N. Kettenbach and S. A. Gerber, Anal. Bioanal. Chem., 2012, 402, 711–720 CrossRef CAS PubMed
.
- C. Wu, J. C. Tran, L. Zamdborg, K. R. Durbin, M. Li, D. R. Ahlf, B. P. Early, P. M. Thomas, J. V. Sweedler and N. L. Kelleher, Nat. Methods, 2012, 9, 822–824 CrossRef CAS PubMed
.
- J. H. Gonczarowska, M. Dell'Aica, C. Dickhut and R. P. Zahedi, Methods Mol. Biol. Search PubMed
, in press.
- S. B. Ficarro, M. L. McCleland, P. T. Stukenberg, D. J. Burke, M. M. Ross, J. Shabanowitz, D. F. Hunt and F. M. White, Nat. Biotechnol., 2002, 20, 301–305 CrossRef CAS PubMed
.
- L. Andersson and J. Porath, Anal. Biochem., 1986, 154, 250–254 CrossRef CAS
.
- H. Zhou, M. Ye, J. Dong, E. Corradini, A. Cristobal, A. J. Heck, H. Zou and S. Mohammed, Nat. Protoc., 2013, 8, 461–480 CrossRef CAS PubMed
.
- M. W. Pinkse, P. M. Uitto, M. J. Hilhorst, B. Ooms and A. J. Heck, Anal. Chem., 2004, 76, 3935–3943 CrossRef CAS PubMed
.
- B. A. Ballif, J. Villen, S. A. Beausoleil, D. Schwartz and S. P. Gygi, Mol. Cell. Proteomics, 2004, 3, 1093–1101 CAS
.
- S. B. Ficarro, Y. Zhang, M. J. Carrasco-Alfonso, B. Garg, G. Adelmant, J. T. Webber, C. J. Luckey and J. A. Marto, Mol. Cell. Proteomics, 2011, 10, O111.011064, DOI:10.1074/mcp.O111.01106
.
- M. Dong, M. Ye, K. Cheng, C. Song, Y. Pan, C. Wang, Y. Bian and H. Zou, J. Proteome Res., 2012, 11, 4673–4681 CrossRef CAS PubMed
.
- A. J. Alpert, Anal. Chem., 2008, 80, 62–76 CrossRef CAS PubMed
.
- S. Loroch, R. P. Zahedi and A. Sickmann, Anal. Chem., 2015, 87, 1596–1604, DOI:10.1021/ac502708m
.
- J. Rush, A. Moritz, K. A. Lee, A. Guo, V. L. Goss, E. J. Spek, H. Zhang, X. M. Zha, R. D. Polakiewicz and M. J. Comb, Nat. Biotechnol., 2005, 23, 94–101 CrossRef CAS PubMed
.
- S. Feng, M. Ye, H. Zhou, X. Jiang, X. Jiang, H. Zou and B. Gong, Mol. Cell. Proteomics, 2007, 6, 1656–1665 CAS
.
- H. Zhou, M. Ye, J. Dong, G. Han, X. Jiang, R. Wu and H. Zou, J. Proteome Res., 2008, 7, 3957–3967 CrossRef CAS PubMed
.
- S. B. Ficarro, Y. Zhang, M. J. Carrasco-Alfonso, B. Garg, G. Adelmant, J. T. Webber, C. J. Luckey and J. A. Marto, Mol. Cell. Proteomics, 2011, 10, O111 011064 Search PubMed
.
- C. Dickhut, S. Radau and R. P. Zahedi, Methods Mol. Biol., 2014, 1156, 417–430 Search PubMed
.
- C. Song, M. Ye, G. Han, X. Jiang, F. Wang, Z. Yu, R. Chen and H. Zou, Anal. Chem., 2010, 82, 53–56 CrossRef CAS PubMed
.
- S. Liu, C. Zhang, J. L. Campbell, H. Zhang, K. K. Yeung, V. K. Han and G. A. Lajoie, Rapid Commun. Mass Spectrom., 2005, 19, 2747–2756 CrossRef CAS PubMed
.
- H. Steen, J. A. Jebanathirajah, J. Rush, N. Morrice and M. W. Kirschner, Mol. Cell. Proteomics, 2006, 5, 172–181 CAS
.
- R. N. Hayes and M. L. Gross, Methods Enzymol., 1990, 193, 237–263 CAS
.
- K. Biemann, Methods Enzymol., 1990, 193, 455–479 CAS
.
- J. E. Syka, J. J. Coon, M. J. Schroeder, J. Shabanowitz and D. F. Hunt, Proc. Natl. Acad. Sci. U. S. A., 2004, 101, 9528–9533 CrossRef CAS PubMed
.
- C. K. Frese, H. Zhou, T. Taus, A. F. Altelaar, K. Mechtler, A. J. Heck and S. Mohammed, J. Proteome Res., 2013, 12, 1520–1525 CrossRef CAS PubMed
.
- M. M. Savitski, S. Lemeer, M. Boesche, M. Lang, T. Mathieson, M. Bantscheff and B. Kuster, Mol. Cell. Proteomics, 2011, 10, M110 003830 Search PubMed
.
- T. Taus, T. Kocher, P. Pichler, C. Paschke, A. Schmidt, C. Henrich and K. Mechtler, J. Proteome Res., 2011, 10, 5354–5362 CrossRef CAS PubMed
.
- M. Vaudel, D. Breiter, F. Beck, J. Rahnenfuhrer, L. Martens and R. P. Zahedi, Proteomics, 2013, 13, 1036–1041 CrossRef CAS PubMed
.
- H. Wiese, K. Kuhlmann, S. Wiese, N. S. Stoepel, M. Pawlas, H. E. Meyer, C. Stephan, M. Eisenacher, F. Drepper and B. Warscheid, J. Proteome Res., 2014, 13, 1128–1137 CrossRef CAS PubMed
.
- J. A. Vizcaino, E. W. Deutsch, R. Wang, A. Csordas, F. Reisinger, D. Rios, J. A. Dianes, Z. Sun, T. Farrah, N. Bandeira, P. A. Binz, I. Xenarios, M. Eisenacher, G. Mayer, L. Gatto, A. Campos, R. J. Chalkley, H. J. Kraus, J. P. Albar, S. Martinez-Bartolome, R. Apweiler, G. S. Omenn, L. Martens, A. R. Jones and H. Hermjakob, Nat. Biotechnol., 2014, 32, 223–226 CrossRef CAS PubMed
.
- L. Martens, H. Hermjakob, P. Jones, M. Adamski, C. Taylor, D. States, K. Gevaert, J. Vandekerckhove and R. Apweiler, Proteomics, 2005, 5, 3537–3545 CrossRef CAS PubMed
.
- M. Vaudel, J. M. Burkhart, R. P. Zahedi, E. Oveland, F. S. Berven, A. Sickmann, L. Martens and H. Barsnes, Nat. Biotechnol., 2015, 33, 22–24, DOI:10.1038/nbt.3109
.
- P. L. Ross, Y. N. Huang, J. N. Marchese, B. Williamson, K. Parker, S. Hattan, N. Khainovski, S. Pillai, S. Dey, S. Daniels, S. Purkayastha, P. Juhasz, S. Martin, M. Bartlet-Jones, F. He, A. Jacobson and D. J. Pappin, Mol. Cell. Proteomics, 2004, 3, 1154–1169 CAS
.
- A. Thompson, J. Schafer, K. Kuhn, S. Kienle, J. Schwarz, G. Schmidt, T. Neumann, R. Johnstone, A. K. Mohammed and C. Hamon, Anal. Chem., 2003, 75, 1895–1904 CrossRef CAS
.
- H. Zhu, S. Pan, S. Gu, E. M. Bradbury and X. Chen, Rapid Commun. Mass Spectrom., 2002, 16, 2115–2123 CrossRef CAS PubMed
.
- S. E. Ong, B. Blagoev, I. Kratchmarova, D. B. Kristensen, H. Steen, A. Pandey and M. Mann, Mol. Cell. Proteomics, 2002, 1, 376–386 CAS
.
- F. Beck, U. Lewandrowski, M. Wiltfang, I. Feldmann, J. Geiger, A. Sickmann and R. P. Zahedi, Proteomics, 2011, 11, 1099–1109 CrossRef CAS PubMed
.
- J. Cox and M. Mann, Nat. Biotechnol., 2008, 26, 1367–1372 CrossRef CAS PubMed
.
- E. L. de Graaf, P. Giansanti, A. F. Altelaar and A. J. Heck, Mol. Cell. Proteomics, 2014, 13, 2426–2434 CAS
.
Footnote |
† Equal contribution. |
|
This journal is © The Royal Society of Chemistry 2015 |
Click here to see how this site uses Cookies. View our privacy policy here.