DOI:
10.1039/D3MA00632H
(Review Article)
Mater. Adv., 2024,
5, 961-985
Current advancement in nanomaterial-based emerging techniques for the determination of aminoglycosides antibiotics for antibiotic resistance surveillances
Received
31st August 2023
, Accepted 11th December 2023
First published on 15th January 2024
Abstract
Antibiotics proved to be no less than a boon for humans after their evolution. Antibiotics shield humans against bacterial infection and save millions of lives. However, the unnecessary and un-prescribed use of these life-saving drugs by humans and animals also makes the bacteria resist the powerful effect of these antibiotics and they could fail in the further treatment of bacterial infection. Hence, it would be necessary to trace the residue of antibiotics remains in food samples to prevent bacterial resistance. To trace the residue of antibiotics in food samples, there is a urgent need to develop a point-of-care device (POC) that is portable, affordable, and highly sensitive. This review focused on the uses of aminoglycosides (AMGs) antibiotics in human and animals as well as the mode of action used by the antibiotics against the bacteria, defence mechanism used by bacteria to develop antibiotic resistance (ABR), reason for ABR followed by their consequences in the public health, and the economic burden. Through this review, we provide deep insights into the emerging optical and electrochemical methods used for the detection of AMGs antibiotics such as gentamicin (GENTA), kanamycin (KANA), streptomycin (STR), neomycin (NEO), tobramycin (TOB), and lincomycin (LNM) residue in food samples. The different sensing modes are evaluated for their potential significance in the enhancement of their sensitivity and selectivity. In this review, we have also focused on the opportunities and challenges for integrating optical and electrochemical sensors for detecting antibiotics in food samples and provide a data set used by artificial intelligence (AI) for the future surveillances and prediction of ABR.
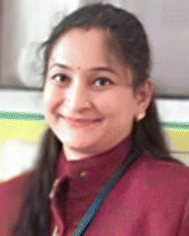
Reena K. Sajwan
| Reena K. Sajwan is a doctoral student at Special Centre of Nanoscience, Jawaharlal Nehru University, New Delhi, India and a recipient of DST- Women Scientist fellowship. She did MTech in Nanoscience and Technology from Guru Gobind Singh Indraprastha University, New Delhi in 2014 and BTech in Instrumentation Engineering from Hemvati Nandan Bahuguna Garhwal Central University, Srinagar, Uttarakhand, India in 2010. Her PhD research work is focus on the development of nanomaterials and nanocomposites based optical sensor used for the detection of antibiotics present in animal derived food samples which is useful in food safety application. She has published many research articles in peer-reviewed journals and filed on Indian patent. |
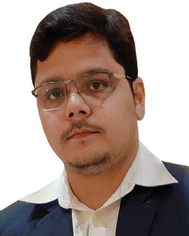
S. Z. H. Hashmi
| S. Z. H. Hashmi is currently working as Junior research fellow at Special Centre of Nanoscience, Jawaharlal Nehru University, New Delhi, India. He did his MTech in Nanoscience and Technology from Guru Gobind Singh Indraprastha University, New Delhi and BTech in Mechanical Engineering from Dr APJ Abdul Kalam Technical University, Lucknow, Uttar Pradesh. He has four years of research experience in the field of nanoscience and published three research articles in peer reviewed international journals and filed one Indian patent. His research work focused on the synthesis of nanocomposites and fabrication of sensors for detection of various environmental pollutants. |
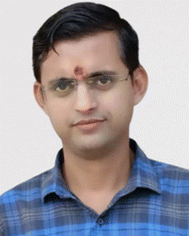
Jayendra Kumar Himanshu
| Jayendra Kumar Himanshu is a doctoral student at Mahatma Gandhi Central University. He completed his BSc Biotechnology from Jiwaji University in 2010 and MSc in Applied Microbiology from Vellore Institute of Technology in 2012. He also did MPhil in Life Sciences from Central University of Gujarat in 2015. Currently he is working as a research scholar at the Nanobio-Laboratory, Special Centre for Nanoscience, Jawaharlal Nehru University under the co-supervision of Dr Pratima R. Solanki. His research area involves fabrication of nanomaterials based electrochemical sensors for the detection of food contaminants. He has published a research article in an internationally recognised journal. |
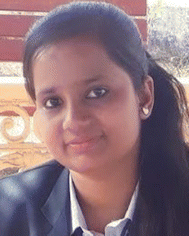
Anjali Kumari
| Anjali Kumari currently working as a project assistant in 3K Nano LLP led by Dr Pratima R Solanki. She did her MSc in Biotechnology from School of Biotechnology, Gautam Buddha University in 2023 and BSc (Biotechnology) from Vinoba Bhave University in the year 2020. She did her master dissertation on “Carbon Quantum Dots-Based “Turn-Off” Fluorescence-Sensing Probes for the Detection of Tetracycline in Food Samples” from Special Centre for Nanoscience, Jawaharlal Nehru University, New Delhi under the supervision of Dr Pratima R. Solanki. Her research area focused on the development of sensors for antibiotic detection in food samples. |
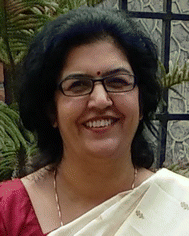
Pratima R. Solanki
| Pratima R. Solanki is working as an Assistant Professor at Special Centre for Nanoscience, Jawaharlal Nehru University, Delhi, India. Dr Solanki received her PhD degree in biological sciences from Maharishi Dayanand University, Haryana, India. She worked as a postdoctoral fellow at National Physical Laboratory, Delhi, Institute of Genomics and Integrative Biology, Delhi, and Tokyo Institute of Technology (TIT), Japan. She is the co-author of more than 200 research papers published in peer-reviewed journals with 11199 citations and with an H-index of 58 and more than 20 international book chapters. She is actively engaged in the research and development of biosensors utilizing nanostructured materials for healthcare and environmental monitoring. She has received Visitor Award 2019 from the Hon’ble President of India. |
1. Introduction
Antibiotics are natural and synthetic drugs that can affect the survival of microorganisms by inhibiting their growth or killing them. Antibiotics treat bacterial infection in humans and are useful for animal health and welfare.1 Among the diverse classes of antibiotics, aminoglycosides (AMGs) have played a significant role in combatting diseases due to their unique properties and mechanisms of action; they have bactericidal action (killing bacteria instead of growth termination), broad-spectrum activity (effective for both gram-positive and negative bacteria), rapid bacterial killing, and are also used in bacterial infections, which are resistive to other antibiotics.2,3
1.1. Discovery and development of AMGs antibiotics
AMGs were first isolated in the mid-20th century from Streptomyces bacteria.4 The discovery was serendipitous as researchers investigated soil samples for compounds with antibacterial properties. The early examples of AMGs included streptomycin (STR) and neomycin (NEO).5 STR, discovered in 1943, marked a breakthrough in treating tuberculosis, a deadly infection at the time.6 The development of NEO soon followed, expanding the arsenal of antibiotics against various pathogens.7
Use of AMGs antibiotics in humans.
The discovery of antibiotics is a blessing of science and invention. Antibiotics play a crucial role as life-saving drugs for humans and act as front-line warriors to protect humans from different bacterial infections. We all are surrounded by countless bacteria that cause several bacterial infections, which causes life-threatening infections, and antibiotics are the only medicines that can treat various kinds of bacterial infections like respiratory infections, pneumonia, sinusitis, bronchitis, urinary tract infections, skin and soft tissue, sepsis, meningitis, and bone and joint infections. While AMGs have been indispensable in treating bacterial infections, their use is not without challenges. One of the major concerns is the potential for adverse effects, particularly on the kidneys and auditory system. AMGs can cause nephrotoxicity (kidney damage) and ototoxicity (hearing loss) due to their accumulation in certain tissues.8
Use in livestock/animals.
AMGs antibiotics are commonly used to treat various bacterial infections in animals, particularly in veterinary medicine.9 AMGs antibiotics, such as gentamicin (GENTA), amikacin, and NEO, have been employed in veterinary practice to manage bacterial infections in livestock. These antibiotics exhibit a broad spectrum of activity against various bacteria, making them valuable tools in treating infections in animals.10 AMGs are effective in treating respiratory, gastrointestinal, and urogenital infections in livestock, helping to improve animal health and welfare.11 Antibiotics in animals are used not only for treating bacterial infections but also as growth promoters. AMGs can contribute to improved growth rates, increased food production efficiency, and enhance the mass of livestock animals.9,11
1.2. Mode of action of antibiotics
Before understanding antibiotic resistance, which bacteria developed against antibiotics to stop or terminate their bacterial inhibiting ability, it is important to first understand the mode of action of antibiotics. Antibiotics are classified based on their mode of action, which is the ability of antibiotics to terminate the growth of bacteria by interfering with the metabolic machinery of bacteria/microbes. The bacteria need this machinery to hold, protect, and generate a replica of it. Antibiotics are supposed to disrupt bacterial cells through one or more modes of action, as shown in Fig. 1 below.12,13
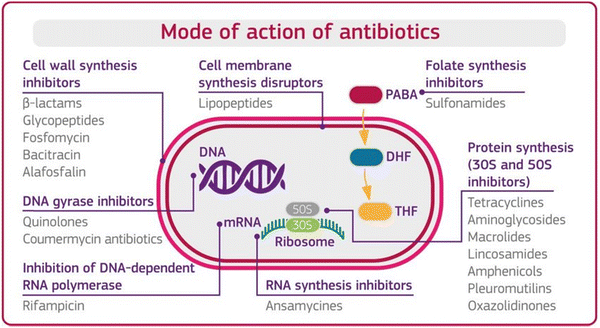 |
| Fig. 1 Mode of action of antibiotics. [Reproduced from ref. 12, copyright [2018]]. | |
Mode 1: Cell wall inhibition. The rigidity of the cell wall protects the bacteria, without which most bacteria break down and die. In this mode of action, the antibiotics inhibit the bacterial enzymes (peptidases), which help synthesize the cellular wall that synthesizes the rigid cellular wall and gives it rigidity.
Mode 2: Cell membrane disruptors. Antibiotics disrupt the integrity of the bacterial cell membrane. They interact with the lipids in the membrane, causing leakage of cellular contents and cell death.
Mode 3: Inhibition of protein synthesis. Ribosome is the main unit for protein synthesis. In this mode of action, antibiotics interfere with ribosomal function by binding with structural sub-units and inhibit the synthesis of proteins. AMGs antibiotics used this defence mechanism against the bacteria.
Mode 4: Interfere with genetic synthesis. In this mode, antibiotics inhibit the DNA polymerase enzyme, which helps to replicate the DNA sequence and multiply the number of resistive bacteria. The disruption of bacterial DNA synthesis can lead to cell death.
Mode 5: Inhibition of RNA synthesis. This mode of action stops the transcription process of messenger RNA and inhibits protein synthesis and cell death.
Mode 6: Inhibition of metabolic pathways. An antibiotic inhibits the enzyme responsible for the synthesis of the metabolic end product in a metabolic way and prevents the further synthesis of the end product.
1.3. Antibiotic resistance developed by AMGs antibiotics
Antibiotic resistance (ABR) is a growing global concern that threatens the effectiveness of medical treatments and public health.14 AMGs antibiotics, known for their potent bactericidal properties, have contributed significantly to the development of ABR.15 ABR can occur through various mechanisms like enzymatic inactivation (produced enzymes, which modified the antibiotics), alter the process of uptake and reflux, a mutation in DNA composition and transfer the resistive information to their neighbors through gene transfer.16 Due to their low bactericidal properties, antibacterial activity, cost, high stability, and broad spectrum effectiveness. AMGs are most widely used clinically in treating bacteria, Monas and Staphylococcus infections, and tuberculosis.17 In animal husbandry, AMGs are used to treat sepsis, peritonitis, enteritis, bovine mastitis, metritis, etc. However, AMGs are used not only for treating bacterial infections in animals but also as a growth promoter for the enhancement of animal mass, which eventually causes the release of excess amounts of these antibiotics into animal-derived food samples and contaminates the environment as well.18 Apart from the advantages, AMGs have serious side effects like toxicity to kidneys, muscle paralysis, ototoxicity, and damage of the vestibular nerve.19
In some cases, they can also cause shock and are sometimes responsible for death. Even a significantly smaller amount of antibiotic residue in food can trigger the ABR speed and become a significant health threat in the future. For that reason, many countries and regions have started making policies for the use of antibiotics and set the tolerance limit of antibiotics as the maximum residue limit (MRL) for AMGs residues in animal products (Table 1) according to Commission Regulation EU No. 37/2010 and Food Safety and Standards Authority of India number F.No. 1-100/SP(PAR)-Notification/Enf/FSSAI/2014.20,21 The simple and rapid detection of AMGs with high sensitivity and selectivity is essential for the routine.
Table 1 Tolerance limit of AMGs antibiotics in food samples
AMGs antibiotics |
Animals |
Animal derived food samples |
Maximum tolerance limit (MRL) mg kg−1 |
FSSAI |
EU |
Streptomycin (STR) |
Cattle |
All edible animal |
|
|
Chicken |
Tissues/fats |
0.5 |
0.5 |
|
Milk |
0.2 |
0.2 |
Eggs |
2 |
— |
Neomycin (NEO) |
Cattle |
All edible animal |
|
|
Duck |
Tissues/fats |
0.5 |
0.5 |
Goat |
Kidney |
10 |
— |
Pork |
Milk |
1.5 |
1.5 |
Chicken |
Egg |
0.5 |
0.5 |
Kanamycin (KANA) |
Cattle |
Mussels/fats |
0.5 |
0.1 |
Pork |
Kidney |
10 |
0.6 |
Chicken |
Milk |
0.1 |
0.15 |
Egg |
0.5 |
— |
Gentamicin (GENTA) |
Cattle |
Mussels/fats |
0.5 |
0.1 |
Pork |
Milk |
0.1 |
— |
Chicken |
Egg |
0.5 |
— |
1.4. Defence mechanism of bacteria to developed ABR
ABR is developed when the bacteria develop the ability to defeat the mode of action of antibiotics to protect themselves. When the bacteria fight back and find new ways to survive, these strategies are said to be resistance mechanisms. Bacteria develop resistance through gene mutation, in which bacteria change the genetic information and transfer it from other bacteria to develop resistance.22,23 Various mechanisms are associated with muted antibacterial action, which diminishes the effect of antibiotics, as shown in Fig. 2.
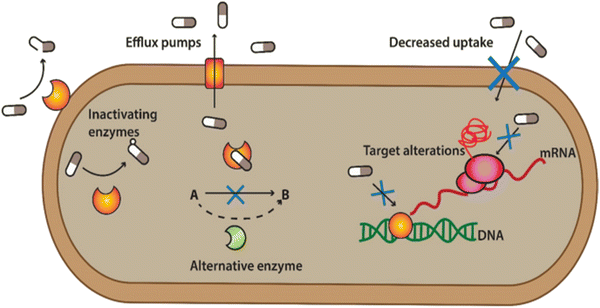 |
| Fig. 2 Defence mechanism of bacteria against antibiotics. [Reproduced from ref. 25 with permission from [Springer Nature], copyright [2022]]. | |
(1) Bacterial can flash back the antibiotics by activating the efflux pump: bacteria can produce pumps in their membrane or cell wall. These so-called efflux pumps are very common in bacteria and can transport a variety of compounds such as signal molecules and nutrients. Some of these pumps can also transport antibiotics out from the bacterium, lowering the antibiotic concentration inside the bacterial cell.
(2) Developing new cell process: through this process, bacteria decreased the membrane's permeability surrounding the bacterial cell. Specific changes in the bacterial membrane make it more difficult to pass through. In this way, less of the antibiotic gets into the bacteria.
(3) Destroy or break down the antibiotics by new protein: bacterial enzymes can inactivate antibiotics. Bacteria can sometimes produce enzymes capable of adding different chemical groups to antibiotics. This prohibits binding between the antibiotic and its target in the bacterial cell.
(4) Mutations in genes: changes in the composition or structure of the target in the bacterium (resulting from mutations in the bacterial DNA) can stop the antibiotic from interacting with the target. Alternatively, the bacteria can add chemical groups to the target structure, shielding it from the antibiotic.
(5) Changing the target so antibiotics no longer fit and do their jobs: sometimes, bacteria can produce a different variant of a structure it needs. For example, vancomycin-resistant bacteria make another cell wall compared to the susceptible bacteria. The antibiotic cannot interact as well with this type of cell wall.23,24
1.5. Transfer mechanism of resistance by bacteria
Antibiotics are used to kill the bacteria and terminate the growth of bacteria by inhibiting cell wall synthesis, discontinuing protein synthesis, altering cell membrane properties, changing the intake route, and effectively activating the reflux pump.26 However, the resistance developed by bacteria is the natural process adapted by bacteria to counter the effect of antibiotics. Bacteria produce these mechanisms using instructions carried in their DNA. The use of antibiotics in animals is not limited to treating bacterial infections but can also be used as a growth promotor. AMGs antibiotics are more prominently used in animal husbandry due to their low cost and are most effective against gram-positive and negative bacterium.27 The unconditional and uncontrolled use of antibiotics in animals can shoot up the bacterial resistance they developed against the antibiotics to nullify their effect. The resistive bacteria then transfer their resistivity through plasmid genes, carry resistive information to nearby bacteria, and accelerate the growth of resistive bacteria.27,28 Then, antibiotics have no long effect on the resistive bacteria and require a higher concentration of antibiotics, and resistive bacteria even grow in the sea of antibiotics, as shown in Fig. 3(A) and (B).
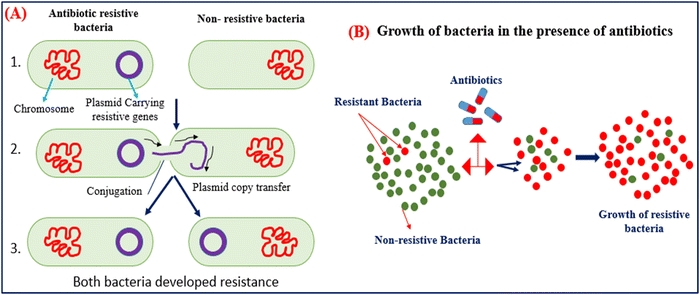 |
| Fig. 3 (A) Mechanism of gene transfer of resistive bacteria to nearby non-resistive bacteria. (B) Growth of bacteria in the presence of massive amounts of antibiotics. | |
1.6. Consequences of ABR
ABR has become a global threat, and the whole world is moving toward the silent pandemic of ABR. The residue of these excess antibiotics is presented in animal-derived food like milk, meat, and eggs. This extra amount of antibiotics further accelerated the rate of ABR and caused significant health problems; as a consequence, the burden of medical hospitality and economic growth increased very rapidly. Nowadays, every country around the world is facing the consequences of ABR, and low-income countries are affected more. In the U.S., more than 2.8 million people suffer from infections developed by resistive bacteria, and around 35
000 people die as a result of saviour-resistant bacterial infections.23 Due to ABR, around 7
000
000 people die globally due to the extensive rise of antibiotic-resistant superbug.27 The statistics said that if the ABR increased at this speed, then at the end of the year 2050, around 10 million people will be on the edge of dying every year, which makes it deadlier than cancer.29,30 World Bank also states that the global gross domastic product (GDP) will fall between 1.1% and 3.8% due to ABR by the year 2050. Unless action is taken, the global burden of deaths from ABR stands at a cumulative global economic output cost of $100 trillion USD.31,32
2. Conventional route for ABR
The unconditional use of AMGs antibiotics in animals is the reason for the development of ABR, and an excess amount of these antibiotics enters derived food samples like meat, milk and egg samples and contaminates them. The most common analytical methods were used for the detection of antibiotics residue in food samples like gas–liquid chromatography,33 mass spectroscopy- liquid chromatography,34,35 high-performance liquid chromatography (HPLC),36 gas chromatography–mass spectroscopy (GS- MS),37 capillary electrophoresis,38 and enzyme-linked immunosorbent (ELISA).39 These methods are sensitive but are time-consuming and expensive; they require expertise and special reagents and have lower detection limits, linear response, and lack of rapidity for practical clinical use; this is why it is not to be used for the spot detection of antibiotics. To overcome these problems, a fast, sensitive, and affordable method is required for the detection of antibiotic traces in food samples. Biosensors are important in fulfilling all the advanced requirements associated with well-defined modern sensors to achieve these properties. Biosensors come into view as the appropriate option or balancing analytical tools for antibiotic determination owing to their advantages over the analytical techniques.40 Further, the biosensor devices can be used as point of care devices (POC), providing easy, fast, and on-site detection facilities.
In past decade, electrochemical and optical techniques have been most widely used for the suitable and selective detection of antibiotics. Electrochemical methods have gained researchers' attention due to their high selectivity, sensitivity, cost-effectiveness, affordability, quick response, and most importantly, the user-friendly and simple design for detecting antibiotics.41–43 Optical biosensors can be distinct as sensors constructed on the optical principles and give biochemical interaction into a suitable output signal. Optical biosensors have a growing impact on conventional techniques for determining biological and chemical analytes due to their easy operation, simple instrumentation, and great sensitivity.44–46
The purpose of writing this review is to mainly focus on providing the collective information on AMGs antibiotics and their uses in humans and animals. The review article includes the mode of action of antibiotics against bacteria, reasons of ABR development, and their consequences in terms of health and economic burden. Apart from this, the articles provide insight into emerging nanomaterials used to develop biosensing platforms. The different sensing method, like optical sensing methods, includes colorimetric and fluorometric assays with chemiluminescence, surface-enhanced Raman scattering (SERS), and electrochemical analysis used widely for detecting AMGs antibiotics. The article's future approach is to integrate all the data sets achieved through the different sensing modes and methods with artificial intelligence (AI) technology. With the advancement of AI, the integrated sensing data sets help to predict ABR in the future.
The state-of-the-art presented in Scheme 1 demonstrates the nanomaterials-based detection approach for AMGs antibiotics, which are covered in this review article. The scheme has four major parts: the first one presented the verities of nanomaterials playing a very important role in the biosensing platform fabrication. The second one is biomolecules like enzymes, nucleic acid, antibody, aptamer, and molecularly imprinted polymers (MIP) (artificial antibodies), which play an important role in recognizing elements that provide selectivity and sensitivity to the developed system. The third part of the system is the different sensing methods, such as colorimetric assays, fluorometric assays, chemiluminescence, surface-enhanced Raman scattering (SERS), and electrochemical analysis. Fourth part associated with collective data set collected from different sensing methods was then further integrated with the modern AI technology, which significantly used the collective data set to predict ABR, their types, and the precautions to be taken in the future.
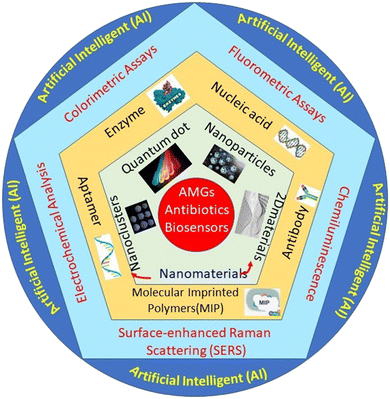 |
| Scheme 1 Systematic representation of the nanomaterials-based biosensor for the detection of AMGs antibiotics. | |
3. Role of nanomaterials in development of antibiotic biosensors
Nanomaterials (NMs) play a very important role in developing optical and electrochemical sensors for detecting antibiotics due to their excellent optical, electronic, and mechanical properties.47,48 NMs open up a new route in the fabrication of advanced and emerging biosensors due to their improved physical and chemical properties like improved surface reactivity, electronic conductivity, and chemical reactivity. There are different types of NMs that have been explored by researchers for the development of optical and electrochemical sensors.49 The most commonly used NMs are carbon quantum dots (CQDs), quantum dots (QDs), metal nanomaterials (MNMs), upconversion nanoparticles (UCNPs), organic fluorescent particles and many more that have significantly increased their consideration and applicability to several fields together with environmental and life sciences.50 These NMs were extensively utilized for the development of biosensors from the point of view of their outstanding mechanical, thermal, electronic, and optical properties for the detection of AMGs antibiotics.51
3.1 Quantum dots (QDs)
QDs are the majority used fashionable fluorescent NMs that describe excellent fluorescence property and have been engaged for a variety of applications. QDs are a quantized electronic structure in which the electrons are confined with respect to the motion in all three directions. The key building block are essentially used from the periodic groups of II–VI or III–V, with generally crystalline structures of a few 100–1000 atoms that make the final size in the range of 1–100 nm such as ZnO, CdS, and CdSe.52,53 This facilitates the unmatched fluorescence property due to the changeover of electrons inside the newly formed band gaps. QDs have numerous advantages as fluorescent NMs (FNMs) as compared to organic dyes.54 FNMs are advantageous over diverse applications like bioimaging and sensing to have brighter luminescence to raise the contrast and sensitivity. In order of luminescence, by changing the size of the QDs, the fluorescence emission wavelength of QDs can be tuned to different wavelengths of light with different excitation wavelengths.55 Thus, QDs prepared from the same group can emit dissimilar color of luminescence simply due to their difference in the size.
3.1.1 Carbon quantum dots (CQDs).
The unique properties of CQDs have encouraged wide studies on them due to their huge potential for a broad diversity of technical applications. The fluorescence emissions of CQDs have gained rising attention in recent years due to their excellent photo-luminescent properties.56 CQDs were discovered accidentally at the time of refinement of single-wall carbon nanotubes (SWCNTs) resulting from arc-discharge soot. After that, researchers used CQDs in various fields for sensing.57,58 Usually, the CQDs are NMs with semi-spherical shapes with particle sizes less than 10 nm, and they have excellent photoluminescence properties, which give emission in the visible region when excitation is applied.57 The CQDs have attractive tuneable photoluminescence properties, excitation and emission wavelengths, good photostability, biocompatibility, and less toxicity with comparatively small size. The enhancement in the photoluminescence of CQDs due to the confinement effect arises due to their small size and plays an important role in research.59 Different groups have done a variety of research and have found that the fluorescence intensity changes along with the size of the NPs. An additional feasible source of photoluminescence could be due to the presence of surface defects of the CQDs. The occurrence of surface deformities and functional groups of CQDs increased the electron sites.60
3.2 Metal-based nanoparticles (MNPs)
MNPs is a group of modified materials with exclusive physical and chemical properties, mostly dependent on their size, composition, shape, and structure.61 Tremendous growth has been achieved in the preparation of new MNPs and their prospective applications in miscellaneous fields such as in catalysis, electronics, sensors, and medicine.62 Due to their high surface area, MNPs have numerous absorption binding sites for antibodies, enzymes, and antigen with brilliant electron transfer kinetics. They have gained significant interest in constructing electrochemical biosensors for determining antibiotics.63 Gold (Au) and silver (Ag) NPs are the two varieties of MNPs well reported in the synthesis of fluorescent NMs.64 The metal NPs like MoS2 nanoflowers and nanosheet are also used to construct electrochemical biosensors.41,65
3.3 Up conversion nanoparticles (UCNPs)
Up-conversion nanoparticles (UCNPs) are a new group of fluorescent NMs in which larger wavelength radiation (lower energy), typically near-infrared (NIR) or IR light, is transformed to a lesser wavelength radiation (higher energy) such as visible light via a two-photon or multiple photon mechanism.66,67 This is recognized as an anti-Stokes mechanism that similarly desires the absorption of two or more comparable photons to offer significant energy for the upconversion of energy. It has gained considerable interest in diverse fields due to its less background distortion, good photostability, minimum toxicity, and high penetrating signals in tissues with low absorption.68 With the various advantages, UCNPs create new room for research compared to down-conversion FNMs, like dyes and FNMs. Usually, they are mostly used as fluorescent linker joint with biorecognition molecules for determining antibiotics.69
3.4 Organic fluorescent particles
Organic fluorescent particles are polymeric materials that show fluorescence.3 The mechanism of fluorescence in organic materials is different from that of metal nanoparticles or quantum dots.
Fluorescent molecularly imprinted polymers are being widely used for the sensing of AMGs. MIPs have the advantage of having multiple binding sites for a single target analyte, which imparts higher recognition selectivity to the optical sensing device.70 MIPs help to detect AMGs in complex biological samples. MIP-based optical sensors can be fabricated using two approaches. It was first the use of the fluorescent monomer to synthesize the MIP and, second, the incorporation of QDs during polymerization reaction while synthesizing MIP.71 The main advantage of using QDs-incorporated MIP is that it imparts high selectivity to the target analyte while maintaining high fluorescent properties of the QDs.72 This gives both high selectivity and high sensitivity to the fabricated optical sensor for the AMGs. The surface modification of QDs is required to improve the photostability and enable surface functionalization while synthesizing QDs-based MIP.73
4 Development of nanomaterial-based sensing techniques
4.1. Optical technique
The optical biosensor is a class of sensors that uses basic optical sensing phenomena to convert a biochemical interaction into a suitable output readout signal. The bimolecular interaction on the NMs surface alters the light characteristics of the NMs, such as intensity, and the bio-sensing event can be determined by the change in their optical properties, such as absorption, fluorescence, and refractive index.74 Optical biosensors have gained tremendous attention in antibiotic detection in recent decades due to their easy operation, handiness, and sensitivity.75 Using NMs in optical biosensors has opened new ways for selective and sensitive strategies in antibiotic determination. Based on the optical signal mechanism, constructing optical biosensors using NMs to determine antibiotics is generally categorized as a colorimetric, fluorometric, and chemiluminescence readout system.
4.1.1 Colorimetric-based mode for the detection of AMGs antibiotics.
Colorimetric read-out systems have gained tremendous consideration due to their inbuilt advantages such as easy formation, rapid detection, and most importantly, naked eye visualization and no need for sophisticated instruments. Colorimetry is a technique in which the determination of substance concentration is mainly done in the solution phase by comparison with the intensity of color, corresponding to the known concentration of the substance.76 In the observation of their properties, colorimetric biosensors are an extremely trendy method in optical biosensors for determining antibiotics. The developed technology or protocol can easily be transformed into POC devices.
Generally, colloidal AuNPs are engaged mostly in the colloidal phase-based biosensors and due to the aggregation of AuNPs in the presence of target molecules, the color changes from red to blue; hence, detection can be done through the color-changing phenomenon. Apyari et al., reported the colorimetric readout system for the determination of NEO. In this method, they used ethylenediaminetetraacetatedisodium salt (EDTA) as a masking agent to prevent aggregation due to the metal ions. The AuNPs was based on the aggregation of label-free AuNPs, in which the color of AuNPs changed along with the increasing concentration of NEO, and the color change is visualized with naked eyes.77 The developed sensor could detect the presence of NEO in the range of 0–73.2 nM with an LOD of 37.4 nM.
Further, Lai et al., used chitosan for the surface modification of AuNPs and enhanced the hydrogen bonding capability of AuNPs. Hydrogen-bonding interaction occurs between kanamycin (KANA) and chitosan, changing the color of the AuNPs from red to blue. The reported sensor shows the linear relation with KANA concentration in the range of 0.01–40 μM with an LOD of 8 nM. The sensor's applicability was tested in real spiked milk samples and found that it could be used for food monitoring applications.26 In another report, Gukowsky et al., reported a cysteamine-modified AuNPs as a colorimetric sensor for the detection of GENTA in the range of 0–200 nM in which the author detected the lower concentration of GENTA up to 12.45 nm.78 The reported work mentioned above used another ligand to improve the selectivity and detection range. But this extra step can make the sensor more laborious and complex, making it costlier. Thus, in this direction, Sajwan et al., reported AuNPs and graphene quantum dots (GQDs)-based dual mode colorimetric as well as fluorometric sensing methods for detecting GENTA without any modification to make the sensing system simple. In that work, the author reported the colorimetric change of AuNPs in the presence of GENTA by improving the detection range to 1.03–16.33 μM with an LOD of 422 nM (Fig. 4(A)). The presented method was validated in the presence of spiked milk and egg samples.79 In this work, the author used two NPs, AuNPs and GQDs, as colorimetric and fluorometric indicators. In other reports, the authors synthesized CQDs and used them as a reducing and stabilizing agent for the synthesis of Au@CQDs nanocomposites (NCs). Au@CQDs NCs are used for the detection of GENTA and KANA using colorimetric sensing mechanism. In this, the color of Au@CQDs NCs changed in the presence of antibiotics, as shown in Fig. 4(B). The developed sensor could detect two antibiotics in the 0–2000 nM range with LOD of 116 nM for GENTA and 195 nM for KANA, respectively. The system applicability was checked in the real spiked milk and egg samples.80
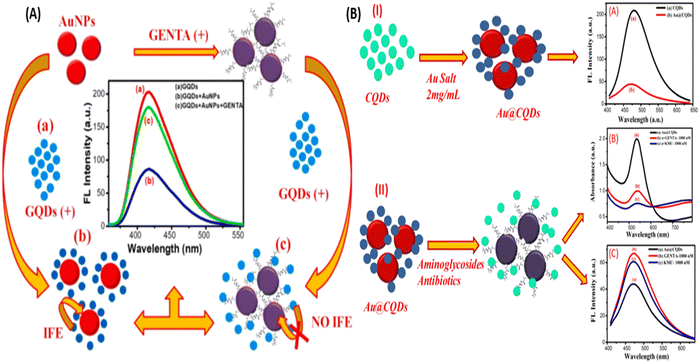 |
| Fig. 4 (A) AuNPs and colorimetric sensor for the detection of GENTA. [Reproduced from ref. 79 with permission from [Elsevier], copyright [2022]] and (B) Au@CQDs NCs-based colorimetric sensor for the detection of GENTA and KANA. [Reproduced from ref. 80 with permission from [Elsevier], copyright [2023]]. | |
Although AuNPs are most widely used for the development of colorimetric sensor, it also has the affinity to get aggregate in the presence of salt and other molecules present in a real system. Thus, to improve the selectivity of the sensors toward the target molecules, researchers have used biomolecules to improve the selectivity. Therefore, in this direction, many reporters have developed a colorimetric deletion method using aptamers to precisely determine antibiotics. Aptamers are single-stranded DNA (ssDNA) or RNA oligonucleotides, which can exclusively attach to the object molecules with excellent affinity, selectivity, and sensitivity.81–83 The aptamer can be cost-effectively synthesized and easily altered with signal moieties. In a classic AuNPs-based colorimetric readout system using the aptamer, the aptamer binds on the surface of AuNPs, which is specific to the target (antibiotics); in the absence of a target, the aptamer protects the AuNPs from aggregation in the presence of a salt. With the addition of target molecules, the aptamer shows specific affinity toward the target molecules, binds with it, and separates out from the surface of the AuNPs. In the salt's presence, AuNPs again aggregate and change their color,63,84 as represented in Fig. 5. Many researchers used this mechanism and developed AuNPs-based aptasensor to detect KANA,85 STR,86,87 and TOB.88,89 Shayesteh et al., used poly(diallyl dimethyl ammonium chloride) (PDDA) as an aggregating agent instead of NaCl. In this work, PDDA agglomerated AuNPs and changed their color from red to blue. When PolyA aptamer was immobilized on the surface of AuNPs, it prevented the aggregation of AuNPs due to the dense attachment of PDDA with the aptamer, as shown in Fig. 5(B).
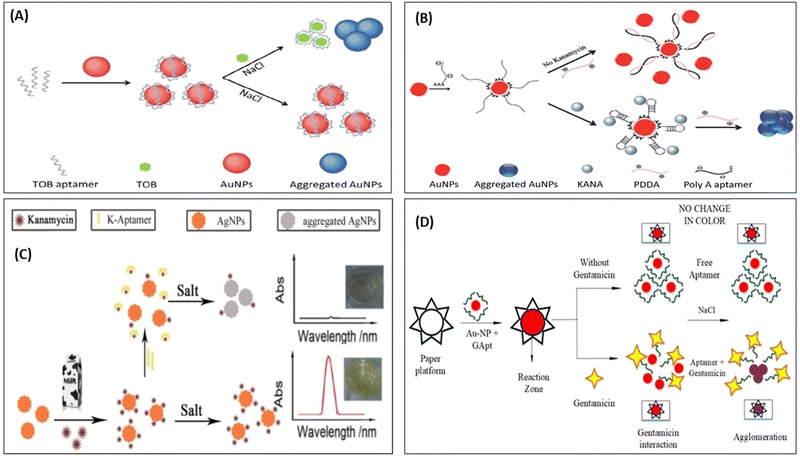 |
| Fig. 5 AuNPs-based colorimetric aptasensor for (A) TOB. [Reproduced from ref. 89 with permission from [Royal Society of Chemistry], copyright [2018]] and (B) KANA. [Reproduced from ref. 85 with permission from [Royal Society of Chemistry], copyright [2020]]. (C) Colorimetric aptasensor for KANA using AgNPs. [Reproduced from ref. 91 with permission from [Elsevier], copyright [2016]], and (D) paper-based aptasensor for GENTA. [Reproduced from ref. 92 with permission from [MDPI], copyright [2021]]. | |
In the presence of KANA, the aptamer traps KANA and changes their structure from linear to 3D. Thus, in the presence of KANA, no free aptamer was present, which formed the PDDA complex, and hence it again agglomerated AuNPs and changed the color. The AuNPs and AgNPs were also used to develop colorimetric aptasensors for AMGs antibiotics like KANA.90,91 Similarly, Ramalingam et al., followed the same and developed an aptasensor for detecting GENTA, as shown in Fig. 5(D). The developed paper-based aptasensor could detect the presence of GENTA in milk samples in the range of 1–3000 nM with an LOD of 300 nM in 2 min.92 This paper-based sensor is convenient, portable, affordable, and can be directly used as a POC device for detecting GENTA. All these set of detection data are further integrated with AI and used for the surveillance of ABR.
Apart from these reports, some researchers reported that KANA and GENTA themselves induced the color change of AuNPs without adding NaCl.26,80 Zhou et al., investigated the influence of different aptamers adsorbed on the surface of AuNPs in the presence of KANA and NaCl. The concentration of KANA varies from 1 pM to 0.1 mM. The results show the color change of AuNPs in the presence of KANA at a concentration 0.1 μM. However, on the addition of NaCl, the color change is more significant at a concentration of 0.1 μM.93 Here, the author reported that in the presence of different aptamers, AuNPs shows same color change in the presence of KANA, as shown in part I in Fig. 6(C). This means that KANA has a great affinity toward the AuNPs or can agglomerate AuNPs even in the presence of the aptamer, whether it is specific to KANA or not. Similar results have been reported for the detection of KANA.94,95
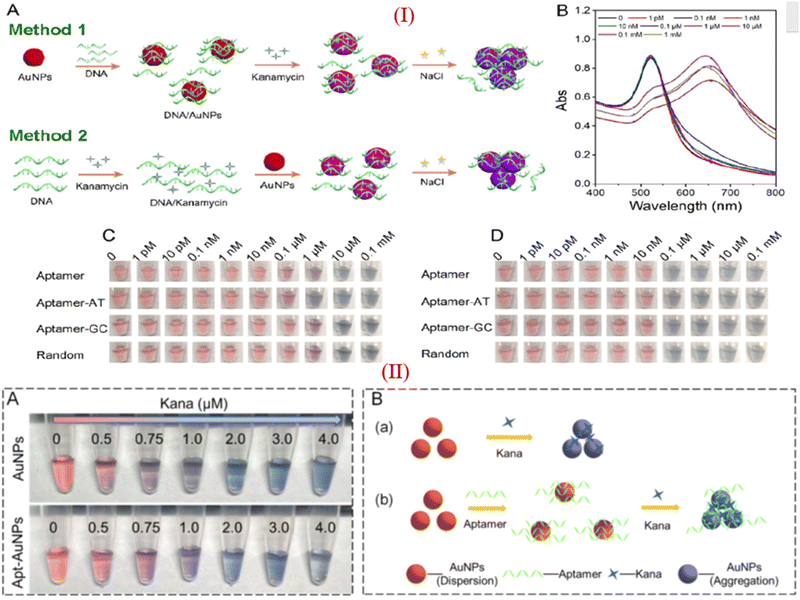 |
| Fig. 6 (I) (A) Different approache used for colorimetric assays of KANA, (B) Absorption spectra of AuNPs in presence of KANA. Colorimetric change of AuNPs after treating with the aptamer with increasing concentration of KANA in the (C)absence and (D) presence of NaCl with different aptamer. [Reproduced from ref. 93 with permission from [American Chemical Society], copyright [2020]]. (II) (A)color change of AuNPs in the presence of KANA without and with the presence of aptamer. (B) systamatic representation of colorimatric apasensor for KANA [Reproduced from ref. 94 with permission from [Elsevier], copyright [2022]]. | |
Apart from the color change properties, AuNPs also shows the catalytic activity and its peroxidase mimetic property was in the development of biosensing assays,96 as shown in Fig. 7. Wang et al., used AuNPs as a catalytic agent, which accelerated the catalytic reaction between 3,3′,5,5′-tetramethylbenzidine (TMB) and H2O2. In the presence of KANA, AuNPs change the surface properties, producing OH˙ radicals and Au3+ ions in the solution and speeding up the catalytic reactions between TMB and H2O2. The reported method shows high sensitivity, and the selectivity toward the KANA varies from 0 to 1000 nM and could detect the lowest value of KANA up to 0.1 nM,97 represented in Fig. 7(A). In a similar way, Tavakoli et al., used AuNPs and explored their catalytic activity in three-way junction aptamer pockets. In the absence of TOB, the AuNPs surface was prevented by the three-way junction pockets and reduced the catalytic activation of 4-nitrophenol in the presence of NaBH4. When TOB was added to the solution, the junction pocket does not form and 4-nitrophenol accesses AuNPs and enhances the catalytic activity in the presence of NaBH4. The aptasensing platform detects TOB in the range that varies from 4 to 32 μM with an LOD of 1.16 μM.98 Zhao et al., reported a colorimetric biosensor using competitive recognition between KANA magnetic beads-kanamycin (MBs-KANA) and biotin-dUTP embedded aptamer and terminal deoxynucleotidyl transferase (TdT) with signal-mediated amplification strategy. Without KANA, aptamers recognize MBs-KANA and TdT can amplify oligonucleotides to the 3′-OH ends of aptamers. The developed assays produced color change after horseradish peroxidase (HRP) catalysis when the substrate forms linkage between biotin and streptavidin (SA)-HRP. In the presence of the KANA, the aptamer binds with KANA rather than MBs-KANA, and the aptamer was removed by magnetic separation. Here, the aptamer is no longer attached with MBs-KANA, which results in a reduction in the amplification of the catalytic signal. The developed colorimetric competitive assays are highly sensitive to KANA and can lower the value of KANA to 9 pM.99
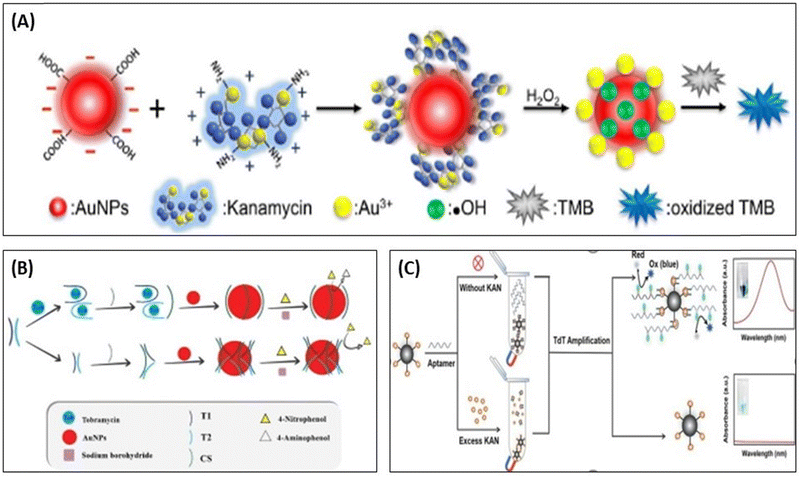 |
| Fig. 7 (A) Detection of KANA using the catalytic activity of AuNPs with TMB. [Reproduced from ref. 97 with permission from [Elsevier], copyright [2017]]. (B) colorimetric aptasensor for TOB using enzyme-like activity of AuNPs. [Reproduced from ref. 98 with permission from [Elsevier], copyright [2022]]. (C) Competitive strategy for the development of MBs-KANA-based colorimetric aptasensor for KANA using HRP. [Reproduced from ref. 99 with permission from [Elsevier], copyright [2023]]. | |
In the past decade, tremendous work has been reported on the development of colorimetric sensors for detecting the AMGs class of antibiotics. Some of the colorimetric sensors are summarized in Table 2.
Table 2 Colorimetric sensor for the detection of AMGs antibiotics
Materials |
Analyte |
Recognition element |
Real sample |
Linear range |
LOD |
Ref. |
AuNPs |
KANA |
Aptamer |
Food products |
10–100 nM |
25 nM |
64
|
AgNPs |
KANA |
Aptamer |
Milk |
103.2 nm–1.2 μM |
5.37 nM |
100
|
AuNPs, HCR |
KANA |
Aptamer |
Milk |
1–40 μM |
0.68 μM |
101
|
AuNPs, MMS |
KANA |
Aptamer |
Milk, honey |
5–500 nM |
4.96 nM |
102
|
AuNPs |
KANA |
Aptamer |
Milk, honey |
1–30 nM |
0.0778 nM |
103
|
AgNPs |
KANA |
Aptamer |
Milk |
85–1000 nM |
4.5 nM |
100
|
AgNPs |
KANA |
|
Milk |
960 nM–20 μM |
960 nM |
104
|
Chlortetracycline-AgNPs |
KANA |
— |
Water |
0.12–0.48 nM |
0.12 nM |
105
|
Chitosan-AuNPs |
KANA |
|
Milk |
10–40 M |
8 nM |
26
|
AuNPs |
KANA |
|
Milk, water |
100–2000 nM |
4 nM |
106
|
Au@CQDs |
KANA |
|
Milk, egg |
0–2000 nM |
195 nM |
80
|
AuNPs-TMB |
KANA |
|
— |
0–1000 nM |
0.1 nM |
97
|
AuNPs |
STR |
|
Milk |
0.0001 nM–0.5 μM |
147.87 nM |
107
|
AuNPs |
STR |
Aptamer |
Milk |
0.18–1 μM |
47.2 nM |
86
|
AuNPs |
STR |
Aptamer |
Milk |
5.2 pM–34.4 nM |
1.7 pM |
108
|
Ag NPs |
STR |
— |
Milk |
0.05–0.65 nM |
0.036 nM |
109
|
Chlortetracycline-Ag NPs |
STR |
|
Water |
2–11 nM |
2.0 nM |
105
|
AuNPs |
TOB |
Aptamer |
Milk, chicken eggs |
40–200 nM |
23.3 nM |
89
|
Hemin DNAzyme |
TOB |
DNA |
Milk, lake water |
20–800 nM |
12.24 nM |
110
|
AuNPs |
TOB |
Aptamer |
Milk, egg |
40–200 nM |
23.3 nM |
89
|
AuNPs |
TOB |
Aptamer |
Honey |
100–1400 nM |
37.9 nM |
88
|
Cys-AuNPs |
GENTA |
— |
Milk |
12.45–200 nM |
12.45 nM |
78
|
AuNPs |
GENTA |
— |
Milk, egg, water |
1.03–16.33 μM |
422 nM |
79
|
Au@CQDs NCs |
GENTA |
— |
Milk egg |
0–2000 nM |
116 nM |
80
|
4.1.2. Fluorometric-based mode of detection for AMGs antibiotics.
The fluorescence technique is one of the most popular optical methods used mainly in developing biosensors for determining antibiotics by considering its wonderful features like simple operation, quick hybridization kinetics, and convenient automation.111 Such biosensors are typically constructed with the help of a fluorophore, which acts as a donor and a quencher used as an acceptor. However, FNMs are very important in developing fluorometric sensor/biosensors. The sensing mechanism of fluorometric sensors are mostly based on fluorescence quenching processes like static quenching, dynamic quenching, inner filter effect (IFE), photo-induced electron transfer (PET), and fluorescence resonance energy transfer (FRET).112 Based on these processes, fluorescent sensors can be developed directly or using biomolecules. In the direct sensor, direct interaction occurs between the host or donor materials and the analyte, which changes the fluorescence intensity of the donor. The fluorometric sensors are in two ways based on the change in their fluorescence intensity after adding antibiotics to the surface-modified FNMs, as shown in Fig. 8(A). The mode to develop acceptor–donor-based pairs fulfills the criteria of IFE and FRET-based systems. In the FRET system, when these two substances come close to each other, the energy transfer between them depends on the distance between the FRET pair. At the excitation wavelength, the resonance energy of the donor transfers to the acceptor; hence, the donor's fluorescence intensity decreased in the presence of the acceptor.113 In this system, the antibiotics are bound with a donor and a suitable acceptor. Upon the binding of biomolecules with the donor or acceptor responsible for the conformational change of the antibiotics, the donor is at a proximity with acceptor in such a way that the energy transfer takes place between them and hence the fluorescence intensity of the donor decreased and can be known as “signal-off”. When the target molecules are introduced in the system, the aptamer binds with the specified target and decreases the close proximity, where the energy transfer efficiency decreases; hence, the fluorescence intensity of the donor increased in the presence of the target molecules and system is said to be in the on mode, as shown in Fig. 8(B).
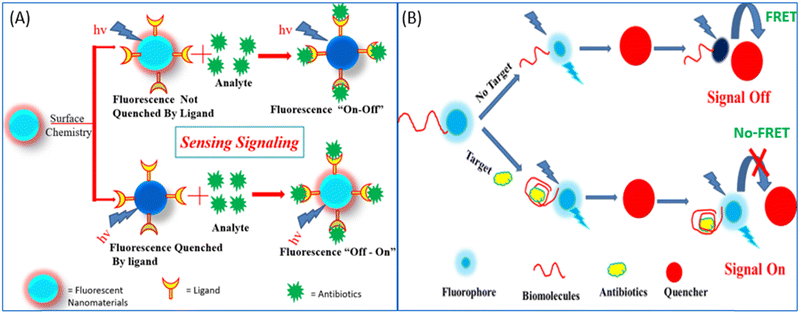 |
| Fig. 8 (A) Fluorescence “on–off”/“off–on” mechanism for the detection of antibiotics. (B) Donor–acceptor-based FRET sensing strategy. | |
Researchers in the past few years have broadly used NMs for the development of fluorescent biosensors with due regard to their unmatched optical and electronic properties. In the past few decades, due to the unmatched photoluminescence properties of NMs, it has gained significant interest in forming novel fluorescent biosensors with the aptamer as a recognition element for more specific antibiotic detection.114,115 Researchers have used GO and graphene, which acts as a more effective acceptor utilizing the electron or energy transfer mechanisms,114 as represented in Fig. 9. Li et al., used UCNPs and graphene sheets to construct donor–acceptor FRET pairs to detect KANA. The specific KANA aptamer could bind on the surface of the UCNPs using EDC/NHS cross-linking mechanism. Firstly, in the presence of graphene, the fluorescence intensity of UCNPs is quenched due to the FRET mechanism, and after the introduction of KANA, the fluorescence intensity was recovered back. The developed system detected low concentration of KANA up to 9 pM, as shown in Fig. 9(A).116 Similarly, Wang et al., reported an MoS2 nanosheet (NS) and aptamer-modified CQDs-based FRET system to determine KANA. The fluorescence intensity of CQDs decreased with the addition of MoS2 NS due to the energy transfer between them. When KANA is introduced into the system, then the aptamer-modified CQDs gets attached to KANA due to the higher affinity of the aptamer toward the target molecules and recovered the fluorescence intensity of CQDs. The FRET-based system shows the linear response with KANA concentration varying from 4 to 25 μM and LOD of 1.1 μM;65 the syntagmatic representation is presented in Fig. 9(B). Apart from FNMs, AuNPs also plays a very important role in the development of a fluorometric aptasensor, and numerous researchers have used AuNPs to develop fluorescent aptasensors, as shown in Fig. 9(C) and (D). Sun et al., used fluorescein (FAM)-labeled AuNPs for the fluorometric detection of KANA. In this FAM aptamer itself as the donor, their emission spectra significantly overlapped with the absorption spectra of AuNPs and constructed an FRET pair. When the aptamer is added to AuNPs, it is adsorbed on the surface of AuNPs and the fluorescence intensity of the FAM-labeled aptamer is decreased. But in the presence of KANA, the aptamer detached from the AuNPs and the fluorescence intensity of the FAM aptamer was recovered back. The aptasensor could detect the KANA in the range of 0.1 pM–0.1 μM with an LOD of 0.1 pM.117
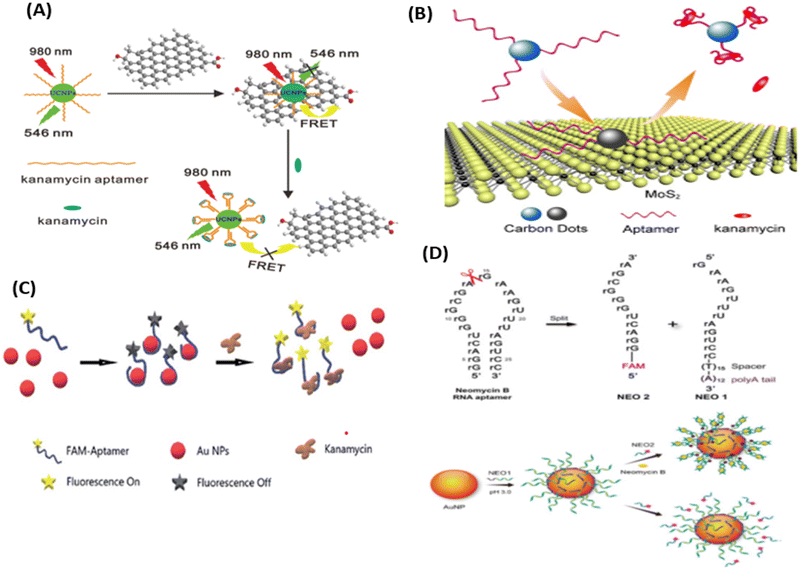 |
| Fig. 9 (A) UCNPs-based aptasensor for KANA. [Reproduced from ref. 116 with permission from [Elsevier], copyright [2014]]. (B) FRET-based system for the determination of KANA. [Reproduced from ref. 65 with permission from [Royal Society of Chemistry], copyright [2016]]. (C) Detection of KANA using FAM aptamer modified AuNPs. [Reproduced from ref. 117 with permission from [Royal Society of Chemistry], copyright [2021]]. (D) AuNPs-based RNA aptasensor for NEO B. [Reproduced from ref. 118 with permission from [Springer Nature], copyright [2016]]. | |
Similarly, Ling et al., used AuNPs and RNA as recognizing agents. In this work, before attaching to the AuNPs, NEO RNA spilt into two parts as NEO1 and NEO2. NEO1 is attached with the thymidine (T15) spacer and the polyaenosine (A12) tail, which is used as an effective anchoring block for potential adsorption on the surface of AuNPs. NEO1 adsorbed on the surface of AuNPs and self-assembled with NEO2 in the presence of NEO B and dissociated in the absence of NEO B. The self-assembly has binding sites for NEO through a conformational structural change in the secondary structure, due to which NEO2 (FAM aptamer) comes close to AuNPs, decreasing the fluorescence intensity of the FAM aptamer. Due to the self-assembly of the RNA aptamer, the fluorescence intensity of the FAM aptamer decreases with the increasing concentration of NEO B, which varies from 0 to 50 μM with an LOD of 0.01 μM.118
Fan et al., reported the detection of NEO using CdTe QDs modified with mercaptosuccinic acid. It was reported that the NEO is detected with both visual analysis and fluorescent signals. The fluorescence of the sensor changed from bright yellow to dark red. The LOD of the sensor reported is 0.18 nM. Sajwan et al., reported the detection of gentamycin using a composite of GQDs-AuNPs, which illustrates the importance of tuning the optical properties of the QDs. Synthesizing QDs composites with metal nanoparticles provides the dual nature of fluorometric donors and acceptors as well as colorimetric detection. This hybrid sensor gives quantitative and qualitative GENTA assays in real food samples. The hybrid sensor shows the lower LOD of 422 nM and 493 nM in colorimetric and fluorometric readout methods, respectively. The quenching mechanism reported in this article is based on IFE. This article reports a recovery efficiency of 95.74%.80 The works reported in the last decade are summarized in Table 3.
Table 3 Fluorometric sensing of AMGs antibiotics
Materials |
Analyte |
Recognition element |
Real sample |
Linear range |
LOD |
Ref. |
CDs, AuNPs |
KANA |
Aptamer |
Milk |
0.04–0.24 μM |
18 nM |
119
|
MSNs, rhodamine B |
KANA |
Aptamer |
Serum |
24.75–137.15 nM |
7.5 nM |
120
|
CNPs |
KANA |
|
Milk |
0.0021 pM–2.06 pM |
0.0001 pM |
121
|
CuSNPs |
KANA |
Aptamer |
Serum |
0.04–20 nM |
26 pM |
122
|
MIP, CdSe QDs |
KANA |
Aptamer |
Water, milk, urine |
0.086–17 μM |
22 nM |
123
|
GOQDs, SSB |
KANA |
Aptamer |
Milk |
0.021 pM–185.7 nM |
12.38 pM |
124
|
berberine |
KANA |
Aptamer |
Serum, milk |
5.0–71.0 nM |
2.3 nM. |
125
|
GO |
KANA |
Aptamer |
Milk |
0.200–200 μM |
0.026 μM |
126
|
Au@CQDs |
KANA |
— |
Milk, egg |
0–2000 nM |
120 nM |
80
|
Au-GQDs |
GENTA |
— |
Milk, egg, water |
1.06–16.55 μM |
493 nM |
79
|
Au@CQDs |
GENTA |
— |
Milk, egg |
0–2000 nM |
133 nM |
80
|
Quantum dot |
STR |
Aptamer |
Milk |
171.9 pM–171.9 nM |
51.58 pM |
127
|
SYBR gold |
STR |
Aptamer |
Serum and milk |
0–4000 nM |
54.5 nM |
128
|
DNA-binding dye |
STR |
Aptamer |
Milk and chicken |
100–100 000 nM |
94 nM |
129
|
AuNPs |
NEO B |
RNA aptamer |
Milk |
0.1 to 10 μM |
0.01 μM |
118
|
MIP |
NEO B |
|
Milk |
3.3–1627 nM |
0.26 nM |
73
|
MIP |
LIN |
|
Egg |
22.5–225 nM |
16.2 nM |
130
|
4.1.3 Molecularly imprinted polymers (MIP)-based fluorescent biosensors.
In recent years, MIP-based sensors have gained researcher's attention due to their high rate of stability, low-cost synthesis, and sensor development. MIPs are the polymers synthesized in the presence of analytes to printed cavities within the polymer matrix, which are associated with the target molecules and act as an artificial antibody. Fluorometric sensing uses fluorescence nanomaterials such as QDs73,131,132 and fluorescent metal–organic framework (MOF) NPs.130 Typically, He and Du et al., developed a fluorometric probe based on a europium metal–organic framework coated with molecularly imprinted polymers (Eu(BTC)-MIP) for the detection lincomycin (LNM). The europium metal–organic framework-based MIP provides specific binding sites for LNM. The fluorescence intensity of MIP decreased in the presence of LNM due to the entrapment of LNM into the cavity exists in MIP. The MIP sensor could detect the presence of LNM in the range from 24.60 nM to 245.98 nM with LOD 16.2 nM and validate the sensor in real egg samples.130 The dual-recognition MIP sensor was reported to improve the selectivity of AMGs antibiotics.73,123 As an example of this for example, Geng et al., fabricated dual-recognition MIP for KANA using CdSe QDs as a fluorescent indicator and thiol-modified aptamer as dual recognition elements. The author used “thio-lene” click reaction to attach the aptamer in the polymer matrix. The MIP was prepared in the presence of the analyte and the aptamer to play the role of dual recognition and improve the selectivity of the sensor. CdSe QDs emitted strong red emission at about 530 nm when excited at 365 nm. The fluorescence intensity of MIP was decreased with different concentration of KANA. The developed sensor could detect the presence of KANA in the range of 103.2 nM–20.64 μM with a detection limit of 26.83 nM.123 Van et al., reported the detection of NEO using SiO2-fluorescent MIP. The fluoresent MIP (FMIP) was synthesized on the surface of the SiO2-QDs by the co-condensation method. The SiO2 QDs were synthesized using the Stober method. Briefly, the functional monomer phenylboronic acid appended triethoxysilane was used to synthesize the FMIP. The developed FMIP sensor provides a selective, sensitive, accurate, rapid, and robust solution for detecting NEO in complex biological samples. The comparative data are summarized in Table 3.
4.1.4 Chemiluminescence-based mode of detection for AMGs antibiotics.
Chemiluminescence (CL) is as a result of some chemical reaction. The energy needed comes from the chemical reaction, in which the reaction produced some new molecules that can have its electron in the excited state after it is formed.133,134 When this excited state electron decays down to the initial state, then the excess amount of energy is released in terms of light. CL is the conversion of chemical energy directly into light as a result of a chemical reaction.134 In 1928, Albrecht reported the CL phenomenon for the first time.135 The CL-based detection technique is broadly used in various fields, mainly in optical biosensing systems. Their excellent properties like high sensitivity, longer dynamic range, simple operation, and cost effectiveness play a great and significant role in analytical techniques.136 In comparison with fluorescence, CL has an advantage over fluorescence-based detection technique, which is that no external source of light is required. In the past years, CL biosensors together with nanomaterials have been broadly utilized in the field of biosensing due to their high sensitivity, wide linear range, simple equipment, and easy operation.134 In this regard, Wan et al., reported luminol-potassium periodate-Mn2+ CL system, in which the luminescence increased in the presence of STR.134 It was well investigated that luminol-H2O2 is fit for the CL reactions but in the absence of the catalyst, it shows very weak emission. However, gold nanoclusters (AuNCs) are known for having the best catalytic properties in luminol-H2O2 reactions to enhance the emission intensity.137 Yao et al., used two single DNAs. DNA1 was the amino-modified aptamer for KANA attached on the magnetic bead through the amidation process. However, DNA2 was used as the prepared AuNCs through the UV-light irradiation process. The AuNCs catalyzed the luminol-H2O2 reaction to emit the light. The KANA aptamer magnetic beads were then hybridized with the DNA-template AuNCs probe. The hybridization process was used for the CL-based detection of KANA. When the aptamer was immobilized on the surface of magnetic beads bound to the target molecules, which was KANA, it results in the release of AuNCs in the solution. The magnetic beads were separated out from the solution with the help of an external magnetic separation process. Then, AuNCs were left-out in the suspension and triggered the catalytic activity to enhance the CL emission of the luminol-H2O2 reaction. The developed strategy helped in the detection of KANA in the range of 0–4.4 nM with an LOD of 0.035 nM.138 Sun et al., detected STR using the CL process. In this work, 3D graphene oxide was functionalized with β-cyclodextrins (β-CD) and ionic liquids (IL) to form graphene oxide aerogel (β-CD/IL@GOGA). Then, the STR-aptamer and G-quadruplexDNAzyme were immobilized on the surface of β-CD/IL@GOGA. In this work, STR-aptamer (STR-Apt) was used for the specific binding to STR molecules; however, G-quadruplexDNAzyme was used to catalyze the luminol-H2O2 reaction to enhance the selectivity and the sensitivity analysis of STR. In the presence of STR, G-DNAzyme leaves the surface of STR-Apt/β-CD/IL@GOGA due to the higher affinity of the aptamer toward the analyte. Then, the released G-DNAzyme catalyzes the CL reaction. The CL-based sensor detects the presence of STR in the range from 1.4 pM to 2.8 nM with an LOD of 92 fM and tested in the real milk and cucumber samples.139 The summarized data are provided in Table 4 below.
Table 4 Chemiluminescence-based sensing for AMGs antibiotics
Materials |
Analyte |
Recognition element |
Real sample |
Linear range |
LOD |
Ref. |
AuNCs-catalyzed luminol |
KANA |
Aptamer |
Milk |
0.2–4.4 nM |
0.035 nM |
138
|
AgNPs |
KANA |
Aptamer |
Milk |
0.8–170 nM |
0.1 nM |
140
|
Opto-microfluidic system combined with SERS |
KANA |
|
Milk, orange juice, water |
1–100 nM |
0.75 nM |
141
|
DNAzyme-catalyzed luminol |
STR |
Aptamer |
Cucumber and milk |
1.4 μM–2.8 nM |
0.092 μM |
139
|
Strep-luminol-NaIO4–Mn2+ |
STR |
|
Milk |
10–1700 nM |
5.16 nM |
142
|
4.1.5 Surface-enhanced Raman spectroscopy (SERS)-based mode of detection of AMGs antibiotics.
SERS is a surface-sensitive technique that increases Raman scattering by molecules adsorbed on rough metal surfaces or by nanostructures like plasmonic AuNPs.143 The Raman signal was generated when the Raman probe was placed in a hotspot between the two metal NPs. Au and Ag metal NPs are most widely used in SERS for the detection of small molecules, biomolecules, and various other analytes.143–145 Shi et al., fabricated a lateral flow immunoassay (LFI) sensor using 4-aminothiophenol (PATP) surface-modified AuNPs as a Raman probe in this direction. Modified AuNPs surface immobilized with monoclonal antibody specific for NEO was used as a Raman probe for the selective detection of NEO. The appearance of the test line corresponding to the Raman signal at a peak at about 1078 cm−1 is used for the quantitative detection of NEO. The reported method is very fast and gave the results within 15 min with an LOD of 0.3514 pM.146 Jiang et al., used DNA-modified Au and AgNPs with the Raman-reported Cy3 to detect KANA. In the presence of increasing concentration of KANA, the Raman intensity of Cy3 decreased. The developed aptasensor could detect KANA in a very low amount of even 293.1 pM.147 Recently, Bi et al., reported a simple SERS-based sensor for the simultaneous detection of TOB and GENTA using bovine serum albumin (BSA)-coated AgNPs modified with γ-Al2O3. The sensor shows good linearity with Raman signals vs. the concentration of GENTA and the TOB varies in the range of 66.7 nM–2 μM and 6.67 nM–0.3 μM, respectively. The sensor could detect the lowest amount of GENTA as 11.88 nM and TOB as 1.26 nM.148 The accumulative data are summarized in Table 5 below.
Table 5 SERS-based biosensor for AMGs antibiotics
Materials |
Analyte |
Recognition element |
Real sample |
Linear range |
LOD |
Ref. |
SERS |
KANA |
Aptamer |
Milk |
0.017–170 nM |
0.0015 nM |
149
|
Cy3, Au, AgNPs |
KANA |
Aptamer |
Milk |
13.76–412.8 nM |
293.1 pM |
147
|
SERS based on LFA |
NEO |
Antibody |
— |
1.4–140 nM |
0.0003 nM |
146
|
BSA-AgNPs γ-Al2O3 |
GENTA |
— |
— |
66.7 nM–2 μM |
11.88 nM |
148
|
TOB |
— |
— |
6.67 nM–0.3 μM |
1.26 nM |
4.2 Electrochemical techniques
An electrochemical biosensor encompasses an electrochemical transducer where an electrode assumes the role of the transduction component. In line with the 1999 IUPAC recommendation, an electrochemical biosensor is a self-contained apparatus capable of furnishing specific quantitative or semi-quantitative analytical data. This is achieved utilizing a biological recognition element (biological receptor) that connects with an electrochemical transducer element.150
Working principle.
Electrochemical biosensors are based on the electrochemical reactions that occur at an electrode's surface when exposed to a solution containing the target analyte. The bioreceptor is immobilized on the surface of the electrode and selectively binds to the target analyte. The binding event causes a change in the electrochemical properties of the electrode, which is measured as an electrical signal that reflects the interaction between the target and the analyte, and it is directly proportional to the analyte concentration. This current can be correlated with the concentration of the electroactive species present at which it is generated. Identifying electrical attributes (like resistance, current, potential, capacitance, and impedance),151–154 which are discerned and quantified through diverse methods such as potentiometric, conductometric, amperometric, or voltametric155,156 having high sensitivity and selectivity. Fig. 10 represents the diagrams of (a) amperometric/voltametric, (b) potentiometric, (c) conductometric, and (d) impedometric biosensors (equivalent circuit).
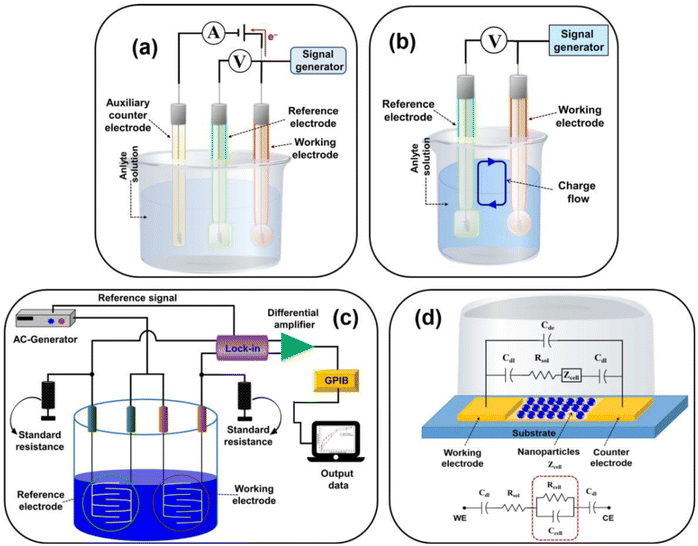 |
| Fig. 10 Representing the diagram of (a) amperometric/voltametric, (b) potentiometric, (c) conductometric biosensors, and (d) equivalent circuit of the impedometric biosensor. [Reproduced from ref. 157 with permission from [MDPI], copyright [2021]]. | |
Electrochemical sensor investigations employ an electrochemical cell, a critical component for the detection of antibiotics. The electrodes are pivotal in the performance of electrochemical cells and biosensors. This electrochemical cell, serving as the experimental, comprises three essential elements: a working electrode (WE), a reference electrode (RE), and a counter electrode (CE). The working electrode (WE) serves as the site where the specific reaction of interest occurs.158 In an electrochemical system featuring three electrodes, the designation of the working electrode as cathodic or anodic depends on whether the reaction happening on it involves reduction or oxidation.159 The reference electrode (RE) establishes a consistent potential within the electrochemical cell.160 The counter electrode (also referred to as the auxiliary electrode) is responsible for completing the current circuit within the electrochemical cell. Typically crafted from an inert material such as platinum, gold, graphite, or glassy carbon, it remains uninvolved in the electrochemical reaction itself.161 The current flow occurs between the working electrode (WE) and the counter electrode (CE).
4.2.1 Amperometric-based sensing.
Amperometric biosensors measure the current generated when the target analytes are electrochemically reduced or oxidized at an electrode surface.162 These sensors' high sensitivity, quick response, and broad dynamic range make them very popular for antibiotic detection. Working electrodes for amperometric sensors used to detect antibiotics are often constructed of conductive materials like carbon-based materials such as carbon nanotubes or graphene and noble metals such as nickel, platinum, and gold because they are inexpensive, have a vast potential window, are chemically inert, have a low background current, and can be used in a variety of sensing and detection applications.163 To increase the working electrode surface's selectivity toward the target antibiotic, specific biomolecules, such as antibodies, aptamer, or DNA probes, are typically added. The target antibiotic is either reduced or oxidized at the working electrode surface in the amperometric sensors' detection principle. The working electrode is surrounded by a reference electrode and a counter electrode, which provide a stable reference potential and complete the electrochemical circuit. The current results are inversely proportional to the amount of antibiotic present in the sample.43 Techniques like cyclic voltammetry, square wave voltammetry, or differential pulse voltammetry, which give additional details about the redox process and increase the sensor's sensitivity and selectivity, can be used to boost the sensor's performance. According to Wei et al., an amperometric approach was used to produce a label-free immunosensor for KANA detection. This research used a graphene sheet sensing platform that also contained PtNPs and Nafion-thionine on GCE electrode. Adsorption was used to immobilize the antibody, and thionine was used as an electron mediator to enhance the transfer of electrons between platinum and graphene. The sensor detects KANA with an LOD of 0.006 ng mL−1 and linear range from 20.64 nM to 24.76 μM.164
4.2.2 Potentiometric-based sensing.
Electrochemical devices such as potentiometric biosensors use an ion-selective membrane or electrode to monitor the potential difference created by an electrochemical cell. Due to their ease of use, affordability, and high sensitivity, these sensors are frequently utilized for antibiotic detection.165 Ion-selective electrodes (ISEs), which only react to certain ions found in antibiotics, are frequently used in potentiometric sensors for antibiotic detection. Glass membrane electrodes and solid-state electrodes are the two categories into which the ISEs can be divided. Antibiotics can be detected using glass membrane electrodes, such as pH glass electrodes, based on pH changes brought on by their ionization.166 On the other hand, ion-selective membranes made of certain materials that specifically bind to the target antibiotic ions are used in solid-state electrodes. There are several methods that may be used to improve the selectivity and sensitivity of potentiometric sensors, including the use of ionophores, MIP, and nanomaterials.167 Ionophores are specialized molecules that can only bind to particular ions, which enhances the sensor's sensitivity. In the study by Yu et al. they developed a novel aptasensor array using a 4-channel screen-printed carbon electrode. This array featured a dual-internal calibration system to simultaneously detect STR and KANA. Two channels were dedicated to assembling the aptamer for these targets, while the other two served as calibration channels. These calibration channels utilized immobilized all-A sequences to minimize background matrix effects, thus enhancing the detection accuracy. Ultimately, this aptasensor array exhibited remarkable sensitivity in detecting STR and KANA under optimized conditions, achieving detection limits of 9.66 pM and 5.24 pM, respectively. Additionally, the linear response ranges were found to be from 10 pM to 10 μM for STR and 10 pM to 1 μM for KANA.168
4.2.3 Voltammetry base sensing.
A method of measuring the current passing through an electrode about the applied voltage is called voltammetry. Different voltammetry techniques, such as cyclic voltammetry (CV), differential pulse voltammetry (DPV), and square wave voltammetry (SWV), can be used to find aminoglycoside antibiotics.169 The analyte's redox behavior or its interaction with specific receptors or nanomaterials is the key component of the detection approach. To increase the sensitivity and selectivity of voltammetry sensors for AMGs detection, nanomaterials have recently been introduced into these devices.170 The selectivity of voltammetric sensors is further enhanced by functionalizing electrodes modified with nanomaterials having recognition components, such as aptamer or antibodies specific to AMGs.171 The measurement of AMGs in complex samples is made possible by variations in the electrochemical signal caused by the interaction of the recognition element and the target analyte. Qin et al., reported that thionine-functionalized graphene (GR-TH) and hierarchical nanoporous PtCu alloy were electrophoretically deposited onto a GCE substrate and immobilized the aptamer for the electrochemical detection of KANA. This aptasensor demonstrates a wide detection span ranging from 1.03 nM to 103.2 μM and an LOD of 0.867 nM in animal-derived food.172
4.2.4. Impedometric-based sensing.
A quick method for characterizing the composition and functioning of electrodes functionalized with biomaterials is known as electrochemical impedance spectroscopy.173 The impedance of the electrode interface between the electrode and the solution containing the target analyte is measured by impedometric biosensors. The bioreceptor is immobilized on the electrode's surface, which is usually made of gold. Target analyte binding to the bioreceptor alters the electrode interface's dielectric characteristics, where an impedance analyzer may be detected as a change in impedance. Impedometric biosensors track how the target antibiotic interacts with an electrochemical system to alter its impedance. These biosensors measure the complex impedance, which consists of capacitance and resistance, at various frequencies. It offers details about the system's electrical characteristics, such as capacitance and resistance, which are connected to the presence and level of aminoglycoside antibiotics. The electrode surface is functionalized with recognition components or modified with nanomaterials to increase the sensitivity and specificity of impedance spectroscopy-based sensors. Changes in the interfacial characteristics brought on by the binding of AMGs to the recognition element affect the impedance spectra. The concentration of AMGs may be assessed using a variety of impedance characteristics, including charge transfer resistance, Warburg impedance (diffusion process occurring at the electrode–electrolyte interface),174 and double-layer capacitance, as shown in Fig. 11. The binding kinetics and processes by which AMGs and the recognition element interact are also well understood by frequency-dependent measurements. EIS is a crucial technique for examining and comprehending the interfacial characteristics of specific biorecognition processes, such as antigen–antibody capture, the construction of aptasensor and immunosensor, or the recognition of particular proteins, receptors, nucleic acids, or whole cells.175,176 Zhu et al., reported a DNA-based aptamer for KANA that is covalently immobilized onto the conducting polymer poly-[2,5-di-(2-thienyl)-1H-pyrrole-1-(p-benzoic acid)] (poly-DPB), with AuNPs drop cast on the SPE substrate. This aptasensor demonstrates a wide detection span ranging from 0.05 mM to 9.0 mM with an LOD of 9.4 ± 0.4 nM in milk.177
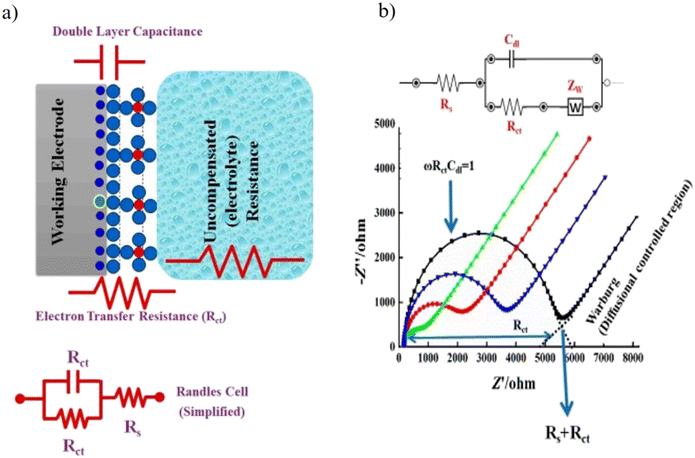 |
| Fig. 11 (a) A simple scheme to describe the EIS circuit and the redox reaction takes place at the surface of working electrodes in a conventional-electrochemical cell (i.e., three-electrode system). Rct is the charge transfer resistance, Rs is the electrolyte resistance, and Cdl is the capacitance double layer. (b) Experimental and simulated impedance spectra showing a simplified Randles equivalent circuit for an electrochemical system. [Reproduced from ref. 174 with permission from [MDPI], copyright [2021]]. | |
4.3.5. Conductometric based sensing.
Electrical conductivity or resistivity detection is measured against the analyte concentration (target antibiotic) when the potential is applied between the reference electrode and polymer-modified electrode. These biosensors require applications in antibiotic sensing because of their simplicity, low cost, and label-free detection. Conductometric biosensors for antibiotic detection frequently use microfabricated electrodes or interdigitated electrode architectures. These electrodes are functionalized with particular recognition components to specifically bind the target antibiotic, such as antibodies, enzymes, or DNA probes. A change in resistance or impedance can be used to measure how the binding event affects the sensor's conducting qualities. One of its benefits is the capacity of conductometric sensors to detect the analyte in real-time without the need for redox processes or electrochemical indicators. The key benefits of conductometric devices include not needing a reference electrode, being cost-effective, enabling miniaturization, and providing a direct electrical response.162 However, compared to other electrochemical sensing methods, their selectivity is less because several resistances need to be considered (electrical resistance, inverse of conductivity), and the sensitivity is also less.178 The interaction between the selective layer and the analyte can influence any individual component or their combinations mentioned above. This interaction makes it challenging to differentiate between various ions or molecules of interest. Therefore, it is crucial to integrate recognition elements with high selectivity into the selective layer. This incorporation aims to improve the overall sensor performance. Nanomaterials, surface modification methods, and signal amplification techniques are strategies to improve the sensitivity and selectivity of conductometric sensors. The electrochemical-based sensor for the detection of AMGs antibiotics is summarized in Table 6.
Table 6 Electrochemical sensor for the detection of AMGs antibiotics
Electrode |
Technique |
Nano-materials |
Analyte |
Recognition element |
Detection range |
LOD |
Real sample |
Ref. |
GCE |
DPV |
PPy3C/ERGO |
STR |
Aptamer |
— |
0.6 nM |
Honey and porcine kidney |
179
|
GCE |
DPV |
NPPtTi/Au@MWCNTs–Fe3O4 |
STR |
Aptamer |
— |
0.02 nM |
Milk |
180
|
GCE |
DPV |
GRFe3O4-AuNPs/PCNR |
STR |
Aptamer |
— |
0.05 nM |
Milk |
181
|
— |
DPV |
Porous carbon nanorods, gold NPs, and copper oxide-functionalized MWCNT |
STR |
Aptamer |
85.97 pM–515.8 nM |
61.9 pM |
Milk and honey |
182
|
GCE |
CV/DPV |
Au@MWCNTs-Fe3O4 composite and NP-PtTi alloy |
STR |
Aptamer |
85.97 pM–171.9 nM |
11.41 nM |
— |
183
|
GCE |
EIS |
(AuNPs) and (GQD-SH) |
STR |
Aptamer |
0.171 pM–1.2 nM |
— |
— |
184
|
— |
CV |
MIPs/GRMWCNTs/CS-SNP and |
NEO |
MIP |
— |
7.65 nM |
Honey and milk |
185
|
Paper strips |
Chronoamperometry/CV |
SWNTs |
NEO |
Antibody |
323.0 pM–203.3 nM |
65.1 pM |
Milk |
186
|
SPE |
LSV |
Poly-DPB/AuNPs |
KANA |
Aptamer |
|
9.5 nM |
Milk |
187
|
SPE |
LSV/CV |
DPB(AuNP) |
KANA |
Aptamer |
0.05 mM–9.0 mM |
9.47 ± 0.4 nM |
Milk |
177
|
GCE |
SWV/CV |
WGS/PB-CTS/NPG film |
KANA |
Antibody |
41.2 pM–28.9 nM |
13.02 nM |
Animal-derived foods |
188
|
GCE |
SWV/CV |
Ag@Fe3O4 NPs and TH-GS |
KANA |
Antibody |
103.2 pM–33.02 nM |
30.95 pM |
Pork meat |
189
|
GCE |
DPV |
VS2/AuNPs NCs and CoFe2O4 nanozyme |
KANA |
Aptamer |
1 pM–1 μM |
0.5 pM |
Milk |
190
|
GCE |
CV/EIS |
GR-TH composite and HNP-PtCu alloy |
KANA |
Aptamer |
1.0 μM–103.2 mM |
0.86 μm |
Animal-derived food |
172
|
ITO |
DPV |
MWCNTs–MoS2NCs |
GENTA |
Antibody |
2.1 μM–83.75 μM |
81.69 nM |
Milk, orange juice |
41
|
5. Role of artificial intelligence (AI) and internet of things (IOT)
In response to ABR crisis, there is an urgent need of developing a standardized system with on-site detection, user-friendly interface, constant surveillance, real-time data monitoring, and remote data analysis system, which can be achieved with the synchronized integration of machine learning, artificial intelligence (AI), and internet of things (IOT).191 Constant real time surveillance is the key strategy in reducing ABR in poultry and milk products. ABR data collected with real-time surveillance provide a vivid profile of ABR transmission through milk and poultry products, which helps identify the hot spots that require immediate intervention and assess the impact of intervention actions.191 This article explains different strategies to analyze the data using AI, deep learning, and data transmission to/from remote locations using IOT for better surveillance.191 Due to recent advances in computer science and programming, AI has a significant and versatile role to play in human intelligence-stimulation systems and its research. The human intelligence and simulation processes include speech recognition, visual perception, natural language processing, and decision-making according to perceived data.191,192 For the AI system's growth, two parameters are necessary: the metadata available from various health records and the enhancement of the processing power of computers. These two aspects of AI inevitably combine with complicated mathematical algorithms, neural networks (NN), and machine/deep learning.193 This is especially true with the development of deep NN designs, where the sophistication (commonly known as the number of factors the networks must learn) has skyrocketed in the previous decade.194
These analyzed data are interpreted and displayed using IOT devices, such as intelligent and wearable sensors, which offer the real-time monitoring of patients and environments.195 These devices can help track the spread of resistant strains, monitor patient adherence to prescribed antibiotic regimens, and provide continuous data for analysis. The seamless integration of IOT into healthcare infrastructure enhances the surveillance and control of ABR.196,197
6. Challenges and alternative approaches for the detection of AMGs antibiotics
Since AMGs antibiotics are essential in the fight against bacterial infections, it is important to develop a system that could trace the residue of these antibiotics in animal-derived food samples and make the system helpful in healthcare and food safety applications. This review summarized the different and majorly used techniques used to detect AMGs side antibiotics. POC are essentially required to enhance ABR surveillance and make antibiotic detection affordable and user-friendly. However, it is difficult to achieve POC since the miniaturization of the sensing equipment is costly for optical and electrochemical techniques, and a very highly scientific approach is required for microfluidic-based colorimetric sensor. There are a number of other difficulties with the existing AMGs detection techniques, from time-consuming procedures to sensitivity problems. Here, in this section, we have discussed the challenges faced by these methods in the form of tables, which provide information about the strengths and weaknesses of the reported ways that may influence the performance of the developed biosensors in Table 7 and also discussed the alternative approaches that hold promise for the future of AMGs detection.
Table 7 Comparison between the strengths and weaknesses of different sensing methods
Technique |
Materials |
Strengths |
Weaknesses |
Ref. |
Colorimetric analysis |
AuNPs |
Cost effective, simple operation, and affordable, suitable for qualitative and quantitative analysis. Visible color changes make operation easy and friendly, low cost, fast response |
Limited sensitivity, less specificity, limited dynamic range, interference from turbidity and background color |
198
|
Fluorometric analysis |
Fluorescent carbon dots QDs |
High sensitivity and selectivity, wide dynamic range for quantification, multiplexing capabilities for multiple analytes, suitable for real-time monitoring, lower interference from turbidity or color |
Requires fluorophores and specific excitation sources, quenching effects can affect accuracy; background fluorescence can be problematic. |
199
|
Chemiluminescence |
Black phosphorus quantum dots |
High sensitivity and low background noise, suitable for quantification in low concentrations, wide dynamic range and multiplexing capabilities, real-time and robust detection, widely used in clinical diagnostics. |
Limited shelf-life of reagents, relatively complex protocols, requires specific chemiluminescent substrates, and limited applicability to certain sample matrices. |
200
|
Surface-enhanced Raman scattering (SERS) |
Graphene oxide (GO) and AuNPs |
High specificity, sensitive for trace analysis, and minimal sample preparation, suitable for complex samples and multiplexing, non-destructive and label-free. |
Requires specialized substrates (e.g., nanoparticles) limited signal enhancement in some cases, background fluorescence can be an issue, instrumentation can be costly, data analysis can be complex. |
141
|
Electrochemical analysis |
0-D, 1-D, and 2-D nanomaterials |
High sensitivity and specificity, quantitative analysis with low detection limits, real-time monitoring and fast response, wide applicability to various analytes and matrices. |
Electrode fouling can impact results, electrode selection and maintenance are crucial, may require complex sample pre-treatment, limited multiplexing capabilities |
191
|
The alternative approach used for the detection of AMGs antibiotics is to develop POC devices that are user-friendly, portable, affordable, and can be used for onsite detection. These point of care devices can have colorimetric, optical, and electrochemical sensing depending on economic feasibility and portability. Multiplex sensor can also be fabricated, which can sense more than one antibiotic simultaneously. These sensors can use microfluidics technology for manufacturing multiplex sensors.
7. Conclusion
AMGs antibiotics are valuable antibacterial drugs employed in many areas of human activity. They are effective against the broad spectra of bacteria but must be used with care due to their low therapeutic indices. Since they are relatively low-cost, cases of unlawful use of these drugs have been reported. This review article focused on the use of the AMGs antibiotics in human and animal husbandry, mode of actions used by the antibiotics against the bacteria, the defence mechanism adopted by the bacteria to protect themselves against the effect of antibiotics, and to developed ABR, followed by their consequences and raising the economic burden on the nation. Through this review, we collectively gathered information about the recently developed optical and electrochemical sensors/biosensor that have been used for the selective and sensitive detection of AMGs antibiotics. The NMs like CQDs, MNPs, QDs, and UCNPs have been widely used in the fabrication of biosensors to detect AMGs antibiotics. The optical sensing mechanism in NMs-biosensor, i.e., colorimetric, fluorometric, chemiluminescence, SERS, and SPR, were used for the detection of AMGs antibiotics developed in recent years, with their application discussed in this review. Fascinatingly, a lot of label-free, one-step analysis, and reagent-free strategies have been developed using NMs. On the other hand, to put into practice the NMs-based biosensor for the detection of AMGs antibiotic in real sample, it is essentially required to understand the application's main aim. The colorimetric assays made the detection procedure simple as well as the naked eye visualization of color formation and color change in the presence of the target antibiotics. There are certain limitations with AuNPs-based colorimetric tests because AuNPs are agglomerated in the presence of salt and some other molecules in real samples. The fluorescent biosensor can be employed as a practical and appropriate detection technique to get over these issues. Although the fluorometric read out system has a spectrophotometry setup, it is a more reliable and continent method for determining antibiotics. In the fluorometric biosensor, MIP can also be used to detect antibiotics. MIP can be used as artificial antibodies, and the sensing is based on the lock and key-based interaction mechanisms. In this, when the analyte binds with the trapping site created during the synthesis, the fluorescence intensity of MIP changes. Another sort of luminescence phenomenon-based detection technique is chemiluminescence, where the luminescence is largely caused by a chemical process; in this process, it is not necessary to excite NMs with an external energy source like a spectrophotometer. In the SERS-based technique, the detection was done by observing the change in the Raman signals. Apart from the optical sensing mode, the electrochemical sensing method is also most commonly featured for detecting AMGs class of antibiotics. A larger range of detection and increased sensitivity are the two benefits of the electrochemical-based approach. In the electrochemical-based sensor, the sensing was performed using amperometric, voltametric, potentiometric, conductometric, and impedometric mode of detection. Among these methods, voltametric and electrochemical mode of detection are most commonly used. The biosensors developed on different sensing methodologies can be further implemented with the POC devices for easy and on site detection. Thus, in the review, we have summarized the collective information about the various detection methods used to determine the traces of AMGs antibiotics in food samples.
8. Future prospective and challenges
The significant challenge with antibiotic biosensors based on NMs is their practical applicability for the on-spot determination of an analyte in real-time with real environments. Real-time analysis is the ultimate aim of the entire manufactured biosensing device to be used by the end user. Regrettably, to date, modernization is constrained to the laboratory scale and is unable to reach that users who desperately need this quick and smart detection method. Thus, a more efficient method that enables the rapid construction of a massive number of NMs-based biosensors with excellent specificity at relatively low cost is still highly desired for the real-time analysis of diseases, which is the condition for the booming marketable applicability of deterministic devices. Potential hard work has been done for the specialized development of NMs and construct antibiotic biosensors that are more applicable. The relevance of the developed biosensors in real samples and the biorecognition elements should be even better since they ought to be liberal to real environmental situations.
On the other hand, we expect the research in NMs-based biosensors for the determination of AMGs antibiotic concentration will still be fascinating and ultimately become an efficient habitual analysis technique for the future point of view. In the future, the developed biosensors’ protocols further integrated with POC devices, which improvised the significance of the biosensing technology with regard to the benefits of society. With this review, we have summarized collective information on the various detection methods used to determine the trace of AMGs antibiotics in the food samples. The collective information provided in this review can be used to track the ABR, and the data can be further used for the surveillance of ABR in society. One promising way to improve our knowledge of antibiotics and reaction to ABR is to integrate AI technologies for the future study of collective data on ABR and their identification. A critical task is how to associate this information with AI systems. AI systems need a variety of comprehensive sets of data on ABR patterns and their detection and integration database, guaranteeing compatibility for AI analysis. The second significant parameter of this is identifying relevant features, including genetic information, microbial characteristics, patient demographics, and details about the detection methods, which are crucial to the AI systems' predictions and performance. After that, depending on the nature and data set, we consider utilizing several machine learning methods, such as clustering, regression, and classification. Based on the collective data sets and their interpretation, the main objectives are that the developing models may forecast ABR, examine detection patterns, and find correlations in the data; use AI algorithms to track trends in ABR and conduct real-time surveillance; react to new resistance patterns; and enable healthcare practitioners to keep ahead of growing dangers, which entails regularly updating the model with new data. Thus, in this review, we have provided information about the uses of AMGs antibiotics in humans and animals, the mode of actions used by the antibiotics against the bacteria, the defence mechanism of bacteria to develop ABR, followed by their consequences. The review provides collective data sets on the detection of AMGs antibiotics with different sensing methods with their sensing range, LOD, and applicability of sensors in real samples. This review can be used as one of the significant sources of the data set associated with AI to help in the prediction of ABR in the future.
Availability of data and material
Availability of data and material in this work are properly cited.
Funding
No funding was received for this review article.
Conflicts of interest
The authors declared that there is no conflict of interest.
Acknowledgements
R. K. S. is very thankful to DST-Women scientist fellowship for providing financial support. P. R. S. and S. Z. H. H is thankful to DHR – GIA grant for the project [R11013/46/2021-GIA/HR]. J. K. H. is thankful to UGC for Non-Net fellowship.
References
- S. Bin Zaman, M. A. Hussain, R. Nye, V. Mehta, K. T. Mamun and N. Hossain, Cureus, 2017, 9(6), e1403 Search PubMed.
- G. V. De Gaetano, G. Lentini, A. Famà, F. Coppolino and C. Beninati, Antibiotics, 2023, 12, 965 CrossRef CAS PubMed.
- Y. Zhou, Y. Ji and Z. Cao, Appl. Sci., 2020, 10, 6579 CrossRef CAS.
- A. H. Elmaidomy, N. H. Shady, K. M. Abdeljawad, M. B. Elzamkan, H. H. Helmy, E. A. Tarshan, A. N. Adly, Y. H. Hussien, N. G. Sayed and A. Zayed, RSC Adv., 2022, 12, 29078–29102 RSC.
- M. Imchen, J. Moopantakath, R. Kumavath, D. Barh, S. Tiwari, P. Ghosh and V. Azevedo, Front. Genet., 2020, 11, 563975 CrossRef CAS PubMed.
-
E. S. Armstrong, C. F. Kostrub, R. T. Cass, H. E. Moser, A. W. Serio and G. H. Miller, Aminoglycosides, in Antibiotic Discovery and Development, ed. T. J. Dougherty and M. J. Pucci, Springer, 2012, pp. 229–269 Search PubMed.
-
V. G. Gupta and A. Pandey, New and future developments, Microbial Biotechnology and bioengineering: Microbial secondary metabolites biochemistry and applications, Elsevier, 2019 Search PubMed.
- M. Jiang, F. Taghizadeh and P. S. Steyger, Front. Cell. Neurosci., 2017, 11, 362 CrossRef PubMed.
- E. Van Duijkeren, C. Schwarz, D. Bouchard, B. Catry, C. Pomba, K. E. Baptiste, M. A. Moreno, M. Rantala, M. Ružauskas and P. Sanders, J. Antimicrob. Chemother., 2019, 74, 2480–2496 CrossRef CAS.
-
S. Giguère, J. F. Prescott and P. M. Dowling, Antimicrobial therapy in veterinary medicine, John Wiley & Sons, 2013 Search PubMed.
- E. P. Martínez, S. E. Golding, J. van Rosmalen, C. Vinueza-Burgos, A. Verbon and G. van Schaik, Prev. Vet. Med., 2023, 213, 105858 CrossRef.
-
I. Sanseverino, A. Navarro Cuenca, R. Loos, D. Marinov and T. Lettieri, Publ. Off. Eur. Union.
- A. L. Fymat, Biomed. J. Sci. Tech. Res., 2017, 1, 1–16 Search PubMed.
- R. Laxminarayan, P. Matsoso, S. Pant, C. Brower, J.-A. Røttingen, K. Klugman and S. Davies, Lancet, 2016, 387, 168–175 CrossRef.
- N. Wang, J. Luo, F. Deng, Y. Huang and H. Zhou, Front. Pharmacol., 2022, 13, 839808 CrossRef CAS.
- J. Davies, J. Infect. Dis., 1971, 124, S7–S10 CrossRef CAS PubMed.
- M. E. Huth, K. H. Han, K. Sotoudeh, Y. J. Hsieh, T. Effertz, A. A. Vu, S. Verhoeven, M. H. Hsieh, R. Greenhouse and A. G. Cheng, J. Clin. Invest., 2015, 125, 583–592 CrossRef PubMed.
- F. Farouk, H. M. E. Azzazy and W. M. A. Niessen, Anal. Chim. Acta, 2015, 890, 21–43 CrossRef CAS.
- M. P. Mingeot-Leclercq, Y. Glupczynski and P. M. Tulkens, Antimicrob. Agents Chemother., 1999, 43, 727–737 CrossRef CAS PubMed.
- E. Nowacka-Kozak, A. Gajda and M. Gbylik-Sikorska, Molecules, 2023, 28, 4595 CrossRef CAS.
- R. Mani and M. Wallace, FSSAI Notifies Tolerance Limits for Additional Antibiotics, Global Agricultural Information Network (GAIN) Report Number: IN8107, 2018, 1–3 Search PubMed.
- R. I. Aminov, Environ. Microbiol., 2009, 11, 2970–2988 CrossRef CAS.
- C. for D. C. and P. (U.S.),; and. N. C. for E. Z. and I. D. (U. S.). D. of H. Q. P. A. R. C. and S. U., Antibiotic resistance threats in the United States, 2019, United States, 2019.
- C. for D. C. and Prevention, Antibiotic resistance threats in the United States, 2019, US Department of Health and Human Services, Centres for Disease Control and …, 2019.
- C. Mutuku, Z. Gazdag and S. Melegh, World J. Microbiol. Biotechnol., 2022, 38, 152 CrossRef CAS PubMed.
- C. Lai, X. Liu, L. Qin, C. Zhang, G. Zeng, D. Huang, M. Cheng, P. Xu, H. Yi and D. Huang, Microchim. Acta, 2017, 184, 2097–2105 CrossRef CAS.
- B. J. Langford and K. L. Schwartz, Can. Commun. Dis. Rep., 2018, 44, 277 CrossRef CAS.
- K. L. Schwartz and S. K. Morris, Curr. Infect. Dis. Rep., 2018, 20, 1–10 CrossRef.
- M. E. A. de Kraker, A. J. Stewardson and S. Harbarth, PLoS Med., 2016, 13, e1002184 CrossRef.
- J. Pérez, F. J. Contreras-Moreno, F. J. Marcos-Torres, A. Moraleda-Muñoz and J. Muñoz-Dorado, Comput. Struct. Biotechnol. J., 2020, 18, 2547–2555 CrossRef.
- The world Bank, By 2050, drug-resistant infections could cause global economic damage on par with 2008 financial crisis, New York, 2016.
- R. J. Fair and Y. Tor, Perspect. Med. Chem., 2014, 6, PMC-S14459 CrossRef PubMed.
- J. W. Mayhew and S. L. Gorbach, J. Chromatogr. A, 1978, 151, 133–146 CrossRef CAS PubMed.
- C. Lecaroz, M. A. Campanero, C. Gamazo and M. J. Blanco-Prieto, J. Antimicrob. Chemother., 2006, 58, 557–563 CrossRef CAS PubMed.
- M. Y. Haller, S. R. Müller, C. S. McArdell, A. C. Alder and M. J.-F. Suter, J. Chromatogr. A, 2002, 952, 111–120 CrossRef CAS PubMed.
- T. Van den Meersche, E. Van Pamel, C. Van Poucke, L. Herman, M. Heyndrickx, G. Rasschaert and E. Daeseleire, J. Chromatogr. A, 2016, 1429, 248–257 CrossRef CAS PubMed.
- S. Yang, X. Zhu, J. Wang, X. Jin, Y. Liu, F. Qian, S. Zhang and J. Chen, Bioresour. Technol., 2015, 193, 156–163 CrossRef CAS PubMed.
- D. Moreno-González, F. J. Lara, N. Jurgovská, L. Gámiz-Gracia and A. M. García-Campaña, Anal. Chim. Acta, 2015, 891, 321–328 CrossRef.
- Y. Jin, J. W. Jang, C. H. Han and M. H. Lee, J. Agric. Food Chem., 2005, 53, 7639–7643 CrossRef CAS.
- Y. Luan, N. Wang, C. Li, X. Guo and A. Lu, Antibiotics, 2020, 9, 1–18 CrossRef PubMed.
- A. K. Yadav, D. Verma and P. R. Solanki, Mater. Today Chem., 2021, 22, 100567 CrossRef CAS.
- N. Chaudhary, A. K. Yadav, J. G. Sharma and P. R. Solanki, J. Environ. Chem. Eng., 2021, 9, 106771 CrossRef CAS.
- A. K. Yadav, T. K. Dhiman, G. Lakshmi, A. N. Berlina and P. R. Solanki, Int. J. Biol. Macromol, 2020, 151, 566–575 CrossRef CAS.
- K. Reder-Christ and G. Bendas, Sensors, 2011, 11, 9450–9466 CrossRef.
- A. S. Vasan, D. M. Mahadeo, R. Doraiswami, Y. Huang and M. Pecht, Front. Biosci., 2013, 5, 39–71 CrossRef PubMed.
- M. C. Morris and M. Blondel, Biotechnol. J., 2014, 9, 171–173 CrossRef CAS.
- M. Imran, S. Ahmed, A. Z. Abdullah, J. Hakami, A. A. Chaudhary, H. A. Rudayni, S. Khan, A. Khan and N. S. Basher, Luminescence, 2023, 38, 1064–1086 CrossRef CAS PubMed.
- J. Hong, M. Su, K. Zhao, Y. Zhou, J. Wang, S.-F. Zhou and X. Lin, Biosensors, 2023, 13, 327 CrossRef CAS.
- A. Chen and S. Chatterjee, Chem. Soc. Rev., 2013, 42, 5425–5438 RSC.
- L. Lan, Y. Yao, J. Ping and Y. Ying, Biosens. Bioelectron., 2017, 91, 504–514 CrossRef CAS.
- S. Nagatoishi, T. Nojima, E. Galezowska, A. Gluszynska, B. Juskowiak and S. Takenaka, Anal. Chim. Acta, 2007, 581, 125–131 CrossRef CAS PubMed.
- S. M. Ng and S. F. Chin, Anal. Lett., 2013, 46, 1278–1288 CrossRef CAS.
- X. Pang, J. Pan, L. Wang, W. Ren, P. Gao, Q. Wei and B. Du, Biosens. Bioelectron., 2015, 71, 88–97 CrossRef CAS PubMed.
- U. Resch-Genger, M. Grabolle, S. Cavaliere-Jaricot, R. Nitschke and T. Nann, Nat. Methods, 2008, 5, 763 CrossRef CAS.
- S. Yan, S. Yang, L. He, C. Ye, X. Song and F. Liao, Synth. Met., 2014, 198, 142–149 CrossRef CAS.
- H. W. Chu, B. Unnikrishnan, A. Anand, Y. W. Lin and C. C. Huang, J. Food Drug Anal., 2020, 28, 539 CAS.
- S. Y. Lim, W. Shen and Z. Gao, Chem. Soc. Rev., 2015, 44, 362–381 RSC.
- X. Xu, R. Ray, Y. Gu, H. J. Ploehn, L. Gearheart, K. Raker and W. A. Scrivens, J. Am. Chem. Soc., 2004, 126, 12736–12737 CrossRef CAS.
- J. Zuo, T. Jiang, X. Zhao, X. Xiong, S. Xiao and Z. Zhu, J. Nanomater., 2015, 2015, 787862 Search PubMed.
- H. Li, X. He, Z. Kang, H. Huang, Y. Liu, J. Liu, S. Lian, C. H. A. Tsang, X. Yang and S. Lee, Angew. Chem., 2010, 122, 4532–4536 CrossRef.
- N. Sozer and J. L. Kokini, Trends Biotechnol., 2009, 27, 82–89 CrossRef CAS.
- K. M. Mayer and J. H. Hafner, Chem. Rev., 2011, 111, 3828–3857 CrossRef CAS PubMed.
- M. L. Anderson, C. A. Morris, R. M. Stroud, C. I. Merzbacher and D. R. Rolison, Langmuir, 1999, 15, 674–681 CrossRef CAS.
- K. M. Song, M. Cho, H. Jo, K. Min, S. H. Jeon, T. Kim, M. S. Han, J. K. Ku and C. Ban, Anal. Biochem., 2011, 415, 175–181 CrossRef CAS PubMed.
- Y. Wang, T. Ma, S. Ma, Y. Liu, Y. Tian, R. Wang, Y. Jiang, D. Hou and J. Wang, Microchim. Acta, 2017, 184, 203–210 CrossRef CAS.
- D. Chen, Y. Yu, F. Huang, A. Yang and Y. Wang, J. Mater. Chem., 2011, 21, 6186–6192 RSC.
- L. Wang, P. Li and Y. Li, Adv. Mater., 2007, 19, 3304–3307 CrossRef CAS.
- J. Zhang, F. Cheng, J. Li, J. J. Zhu and Y. Lu, Nano Today, 2016, 11, 309–329 CrossRef CAS.
- L. Mattsson, K. D. Wegner, N. Hildebrandt and T. Soukka, RSC Adv., 2015, 5, 13270–13277 RSC.
- T. Shi, H. Fu, L. Tan and J. Wang, Microchim. Acta, 2019, 186, 1–8 CrossRef CAS.
- M. I. Gaviria-Arroyave, J. B. Cano and G. A. Peñuela, Talanta Open, 2020, 2, 100006 CrossRef.
- X. Liu, Z. Zhou, T. Wang, P. Deng and Y. Yan, J. Mater. Sci., 2020, 55, 14153–14165 CrossRef CAS.
- Y. Wan, Y. Liu, C. Liu, H. Ma, H. Yu, J. Kang, C. Gao, Z. Wu, D. Zheng and B. Lu, J. Pharm. Biomed. Anal., 2018, 154, 75–84 CrossRef CAS.
- S. M. Yoo and S. Y. Lee, Trends Biotechnol., 2016, 34, 7–25 CrossRef CAS.
- J. Adrian, S. Pasche, G. Voirin, D. G. Pinacho, H. Font, F. Sánchez-Baeza, M.-P. Marco, J.-M. Diserens and B. Granier, TrAC, Trends Anal. Chem., 2009, 28, 769–777 CrossRef CAS.
- O. H. Shayesteh and A. G. Khosroshahi, Anal. Methods, 2020, 12, 1858–1867 RSC.
- V. V. Apyari, S. G. Dmitrienko, V. V. Arkhipova, A. G. Atnagulov, M. V. Gorbunova and Y. A. Zolotov, Spectrochim. Acta, Part A, 2013, 115, 416–420 CrossRef CAS.
- J. C. Gukowsky, C. Tan, Z. Han and L. He, J. Food Sci., 2018, 83, 1631–1638 CrossRef CAS.
- R. K. Sajwan and P. R. Solanki, Food Chem., 2022, 370, 131312 CrossRef CAS PubMed.
- R. K. Sajwan and P. R. Solanki, Food Chem., 2023, 415, 135590 CrossRef CAS.
- Y. Wang and R. R. Rando, Chem. Biol., 1995, 2, 281–290 CrossRef CAS.
- K. M. Song, S. Lee and C. Ban, Sensors, 2012, 12, 612–631 CrossRef PubMed.
- E. J. Cho, J. W. Lee and A. D. Ellington, Annu. Rev. Anal. Chem., 2009, 2, 241–264 CrossRef CAS PubMed.
- L. Li, B. Li, Y. Qi and Y. Jin, Anal. Bioanal. Chem., 2009, 393, 2051–2057 CrossRef CAS.
- K. M. Song, M. Cho, H. Jo, K. Min, S. H. Jeon, T. Kim, M. S. Han, J. K. Ku and C. Ban, Anal. Biochem., 2011, 415, 175–181 CrossRef CAS PubMed.
- V. Soheili, S. M. Taghdisi, M. Hassanzadeh Khayyat, B. S. Fazly Bazzaz, M. Ramezani and K. Abnous, Microchim. Acta, 2016, 183, 1687–1697 CrossRef CAS.
- Z. Liu, Y. Zhang, Y. Xie, Y. Sun, K. Bi, Z. Cui, L. Zhao and W. Fan, Chem. Res. Chin. Univ., 2017, 33, 714–720 CrossRef CAS.
- X. Han, Y. Zhang, J. Nie, S. Zhao, Y. Tian and N. Zhou, Microchim. Acta, 2018, 185, 4 CrossRef.
- Q. Ma, Y. Wang, J. Jia and Y. Xiang, Food Chem., 2018, 249, 98–103 CrossRef CAS PubMed.
- M. G. Caglayan and F. Onur, Spectrosc. Lett., 2014, 47, 771–780 CrossRef CAS.
- R. Y. Robati, A. Arab, M. Ramezani, F. A. Langroodi, K. Abnous and S. M. Taghdisi, Biosens. Bioelectron., 2016, 82, 162–172 CrossRef CAS.
- S. Ramalingam, C. M. Collier and A. Singh, Biosensors, 2021, 11, 29 CrossRef CAS PubMed.
- H. Han, J. Liu, J. Zhou, Y. Li, W. Wang and Z. Lu, Langmuir, 2020, 36, 11490–11498 CrossRef.
- R. Xu, Y. Cheng, X. Qi, X. Li, Z. Zhang, L. Chen, T. Sun, Z. Gao and M. Zhu, Anal. Chim. Acta, 2022, 1230, 340377 CrossRef CAS PubMed.
- J. Li, Y. Liu, H. Lin, Y. Chen, Z. Liu, X. Zhuang, C. Tian, X. Fu and L. Chen, Food Chem., 2021, 347, 128988 CrossRef CAS.
- Y. Tao, Y. Lin, J. Ren and X. Qu, Biosens. Bioelectron., 2013, 42, 41–46 CrossRef CAS.
- C. Wang, D. Chen, Q. Wang and R. Tan, Biosens. Bioelectron., 2017, 91, 262–267 CrossRef CAS.
- P. Tavakoli, S. M. Taghdisi, P. Maghami and K. Abnous, Spectrochim. Acta, Part A, 2022, 267, 120626 CrossRef CAS PubMed.
- T. Zhao, Q. Chen, Y. Wen, X. Bian, Q. Tao, G. Liu and J. Yan, Food Chem., 2022, 377, 132072 CrossRef CAS.
- Y. Xu, T. Han, X. Li, L. Sun, Y. Zhang and Y. Zhang, Anal. Chim. Acta, 2015, 891, 298–303 CrossRef CAS.
- C. Xu, Y. Ying and J. Ping, Microchim. Acta, 2019, 186, 1–7 CrossRef PubMed.
- Y. Ou, X. Jin, J. Liu, Y. Tian and N. Zhou, Anal. Biochem., 2019, 587, 113432 CrossRef CAS.
- J. Liu, J. Zeng, Y. Tian and N. Zhou, Analyst, 2018, 143, 182–189 RSC.
- R. K. Singh, B. Panigrahi, S. Mishra, B. Das, R. Jayabalan, P. K. Parhi and D. Mandal, J. Mol. Liq., 2018, 269, 269–277 CrossRef CAS.
- G. D. Saratale, R. G. Saratale, G. Ghodake, S. Shinde, D.-Y. Kim, A. A. Alyousef, M. Arshad, A. Syed, D. Pant and H.-S. Shin, Nanomaterials, 2020, 10, 997 CrossRef CAS.
- L. Qin, G. Zeng, C. Lai, D. Huang, C. Zhang, P. Xu, T. Hu, X. Liu, M. Cheng and Y. Liu, Sens. Actuators, B, 2017, 243, 946–954 CrossRef CAS.
- J. Zhao, Y. Wu, H. Tao, H. Chen, W. Yang and S. Qiu, RSC Adv., 2017, 7, 38471–38478 RSC.
- Q. Luan, Y. Miao, N. Gan, Y. Cao, T. Li and Y. Chen, Sens. Actuators, B, 2017, 251, 349–358 CrossRef CAS.
- G. Ghodake, S. Shinde, R. G. Saratale, A. Kadam, G. D. Saratale, A. Syed, N. Marraiki, A. M. Elgorban and D. Kim, J. Sci. Food Agric., 2020, 100, 874–884 CrossRef CAS PubMed.
- Y. Ou, X. Jin, J. Fang, Y. Tian and N. Zhou, Microchem. J., 2020, 156, 104823 CrossRef CAS.
- S. E. Kim, K. Y. Ahn, J. S. Park, K. R. Kim, K. E. Lee, S. S. Han and J. Lee, Anal. Chem., 2011, 83, 5834–5843 CrossRef CAS PubMed.
- F. Zu, F. Yan, Z. Bai, J. Xu, Y. Wang, Y. Huang and X. Zhou, Microchim. Acta, 2017, 184, 1899–1914 CrossRef CAS.
-
S. A. Hussain, Fluorescence resonance energy transfer (FRET) sensor. arXiv, 2014, preprint, arXiv.1408.6559 DOI:10.48550/arXiv.1408.6559.
- B. Tan, H. Zhao, L. Du, X. Gan and X. Quan, Biosens. Bioelectron., 2016, 83, 267–273 CrossRef CAS PubMed.
- E. Song, M. Yu, Y. Wang, W. Hu, D. Cheng, M. T. Swihart and Y. Song, Biosens. Bioelectron., 2015, 72, 320–325 CrossRef CAS.
- H. Li, D. En Sun, Y. Liu and Z. Liu, Biosens. Bioelectron., 2014, 55, 149–156 CrossRef CAS.
- Y. Sun, T. Qi, Y. Jin, L. Liang and J. Zhao, RSC Adv., 2021, 11, 10054–10060 RSC.
- K. Ling, H. Jiang, L. Zhang, Y. Li, L. Yang, C. Qiu and F.-R. Li, Anal. Bioanal. Chem., 2016, 408, 3593–3600 CrossRef CAS PubMed.
- J. Wang, T. Lu, Y. Hu, X. Wang and Y. Wu, Spectrochim. Acta, Part A, 2020, 226, 117651 CrossRef CAS.
- S. Dehghani, N. M. Danesh, M. Ramezani, M. Alibolandi, P. Lavaee, M. Nejabat, K. Abnous and S. M. Taghdisi, Anal. Chim. Acta, 2018, 1030, 142–147 CrossRef CAS.
- X. Li, J. Ping and Y. Ying, TrAC, Trends Anal. Chem., 2019, 113, 1–12 CrossRef CAS.
- A. S. F. Belal, A. Ismail, M. M. Elnaggar and T. S. Belal, Spectrochim. Acta, Part A, 2018, 205, 48–54 CrossRef CAS.
- Y. Geng, M. Guo, J. Tan, S. Huang, Y. Tang, L. Tan and Y. Liang, Sens. Actuators, B, 2018, 268, 47–54 CrossRef CAS.
- Y. He, X. Wen, B. Zhang and Z. Fan, Sens. Actuators, B, 2018, 265, 20–26 CrossRef CAS.
- Y. Zhou, L. Zuo, Y. Wei and C. Dong, J. Lumin., 2020, 222, 117124 CrossRef CAS.
- Y. Tang, C. Gu, C. Wang, B. Song, X. Zhou, X. Lou and M. He, Biosens. Bioelectron., 2018, 102, 646–651 CrossRef CAS PubMed.
- N. Gan, C. Ou, H. Tang, Y. Zhou and J. Cao, RSC Adv., 2017, 7, 8381–8387 RSC.
- S. M. Taghdisi, N. M. Danesh, M. A. Nameghi, M. Ramezani and K. Abnous, Food Chem., 2016, 203, 145–149 CrossRef CAS PubMed.
- B. Lin, Y. Yu, Y. Cao, M. Guo, D. Zhu, J. Dai and M. Zheng, Biosens. Bioelectron., 2018, 100, 482–489 CrossRef CAS.
- P. Wu, Q. Du, Y. Sun, Z. Li and H. He, Anal. Methods, 2019, 11, 4501–4510 RSC.
- Y. Geng, M. Guo, J. Tan, S. Huang, Y. Tang, L. Tan and Y. Liang, Sens. Actuators, B, 2018, 268, 47–54 CrossRef CAS.
- J. Hassanzadeh, B. R. Moghadam, A. Sobhani-Nasab, F. Ahmadi and M. Rahimi-Nasrabadi, Spectrochim. Acta, Part A, 2019, 214, 451–458 CrossRef CAS PubMed.
- D. L. Giokas, A. G. Vlessidis, G. Z. Tsogas and N. P. Evmiridis, TrAC, Trends Anal. Chem., 2010, 29, 1113–1126 CrossRef CAS.
- Z. Gu, A. Fu, L. Ye, K. Kuerban, Y. Wang and Z. Cao, ACS Sens., 2019, 4, 2922–2929 CrossRef CAS.
- Z. F. Zhang, H. Cui, C. Z. Lai and L.-J. Liu, Anal. Chem., 2005, 77, 3324–3329 CrossRef CAS PubMed.
- S. Cho, L. Park, R. Chong, Y. T. Kim and J. H. Lee, Biosens. Bioelectron., 2014, 52, 310–316 CrossRef CAS.
- M. Deng, S. Xu and F. Chen, Anal. Methods, 2014, 6, 3117–3123 RSC.
- Y. Yao, X. Wang, W. Duan and F. Li, Analyst, 2018, 143, 709–714 RSC.
- Y. Sun, R. Han, Y. Dai, X. Zhu, H. Liu, D. Gao, C. Luo, X. Wang and Q. Wei, Sens. Actuators, B, 2019, 301, 127122 CrossRef CAS.
- S. Cheng, H. Liu, H. Zhang, G. Chu, Y. Guo and X. Sun, Sens. Actuators, B, 2020, 304, 127367 CrossRef CAS.
- A. H. Nguyen, X. Ma, H. G. Park and S. J. Sim, Sens. Actuators, B, 2019, 282, 765–773 CrossRef CAS.
- G. Wan, H. Cui, H. Zheng, Y. Pang, L. Liu and X. Yu, Luminescence, 2006, 21, 36–42 CrossRef CAS.
- K. Kim and K. S. Shin, Anal. Sci., 2011, 27, 775–783 CrossRef CAS PubMed.
- M. Knauer, N. P. Ivleva, R. Niessner and C. Haisch, Anal. Sci., 2010, 26, 761–766 CrossRef CAS.
- G. Wang, C. Lim, L. Chen, H. Chon, J. Choo, J. Hong and A. J. DeMello, Anal. Bioanal. Chem., 2009, 394, 1827–1832 CrossRef CAS.
- Q. Shi, J. Huang, Y. Sun, M. Yin, M. Hu, X. Hu, Z. Zhang and G. Zhang, Spectrochim. Acta, Part A, 2018, 197, 107–113 CrossRef CAS PubMed.
- Y. Jiang, D. W. Sun, H. Pu and Q. Wei, J. Food Meas. Charact., 2020, 14, 3184–3193 CrossRef.
- S. Bi, Y. Yuan, F. Zhang, Y. Wang, J. Liu, B. Yang and D. Song, Talanta, 2023, 260, 124635 CrossRef CAS PubMed.
- Y. Jiang, D. W. Sun, H. Pu and Q. Wei, Talanta, 2019, 197, 151–158 CrossRef CAS.
-
C. Karunakaran, R. Rajkumar and K. Bhargava, Introduction to biosensors, in Biosensors and bioelectronics, Elsevier, 2015, pp. 1–68 Search PubMed.
- A. Shanker and K. Lee, Synthetic Hybrid Biosensors, Reviews in Cell Biology and Molecular Medicine, 2014, 357–392 Search PubMed.
- D. Verma, D. Chauhan, M. Das Mukherjee, K. R. Ranjan, A. K. Yadav and P. R. Solanki, J. Appl. Electrochem., 2021, 51, 447–462 CrossRef CAS.
- D. Verma, A. K. Yadav, M. D. Mukherjee and P. R. Solanki, J. Environ. Chem. Eng., 2021, 9(4), 105504 CrossRef CAS.
- D. Verma, K. R. Ranjan, M. Das Mukherjee and P. R. Solanki, Biosens. Bioelectron.: X, 2022, 100217 CAS.
- D. Verma, T. K. Dhiman, M. Das Mukherjee and P. R. Solanki, J. Electrochem. Soc., 2021, 168(9), 097504 CrossRef CAS.
-
N. Chaudhry, D. Verma, A. K. Yadav, J. G. Sharma and P. Solanki, Determination of ciprofloxacin using the Ziziphus mauritania derived fluorescent carbon dots based optical sensor, 2022 DOI:10.21203/rs.3.rs-1775535/v1.
- V. Naresh and N. Lee, Sensors, 2021, 21(4), 1109 CrossRef CAS.
- J. Radhakrishnan, S. Wang, I. M. Ayoub, J. D. Kolarova, R. F. Levine and R. J. Gazmuri, Am. J. Physiol. Heart Circ. Physiol., 2007, 292, H767–75 CrossRef CAS PubMed.
- F. Zhou, D. Xing, B. Wu, S. Wu, Z. Ou and W. R. Chen, Nano Lett., 2010, 10, 1677–1681 CrossRef CAS PubMed.
-
G. Inzelt, A. Lewenstam and F. Scholz, Handbook of reference electrodes, Springer, 2013, vol. 541 Search PubMed.
-
F. G. Thomas and G. Henze, Introduction to Voltammetric Analysis: Theory and Practice, Csiro Publishing, 2001, pp. 1–247 Search PubMed.
- D. Grieshaber, R. MacKenzie, J. Vörös and E. Reimhult, Sensors, 2008, 8, 1400–1458 CrossRef CAS.
- M. Chaudhary, A. Kumar, A. Devi, B. P. Singh, B. D. Malhotra, K. Singhal, S. Shukla, S. Ponnada, R. K. Sharma and C. A. Vega-Olivencia, Mater. Adv., 2023, 4, 432–457 RSC.
- Q. Wei, Y. Zhao, B. Du, D. Wu, H. Li and M. Yang, Food Chem., 2012, 134, 1601–1606 CrossRef CAS PubMed.
- C. Wardak, K. Pietrzak, K. Morawska and M. Grabarczyk, Sensors, 2023, 23, 5839 CrossRef PubMed.
- S. A. Abdel-Gawad, H. H. Arab and A. A. Albassam, Chemosensors, 2022, 10, 146 CrossRef CAS.
- J. Wang, R. Liang and W. Qin, TrAC, Trends Anal. Chem., 2020, 130, 115980 CrossRef CAS.
- J. Yu, W. Tang, F. Wang, F. Zhang, Q. Wang and P. He, Sens. Actuators, B, 2020, 311, 127857 CrossRef CAS.
- Z. Khoshbin, A. Verdian, M. R. Housaindokht, M. Izadyar and Z. Rouhbakhsh, Biosens. Bioelectron., 2018, 122, 263–283 CrossRef CAS.
- S. Pilehvar, C. Reinemann, F. Bottari, E. Vanderleyden, S. Van Vlierberghe, R. Blust, B. Strehlitz and K. De Wael, Sens. Actuators, B, 2017, 240, 1024–1035 CrossRef CAS.
- C. Zhou, H. Zou, C. Sun and Y. Li, Food Chem., 2021, 361, 130109 CrossRef CAS PubMed.
- X. Qin, Y. Yin, H. Yu, W. Guo and M. Pei, Biosens. Bioelectron., 2016, 77, 752–758 CrossRef CAS.
- L. Yang and R. Bashir, Biotechnol. Adv., 2008, 26, 135–150 CrossRef CAS.
- H. S. Magar, R. Y. A. Hassan and A. Mulchandani, Sensors, 2021, 21 Search PubMed.
- C. Slouka, D. J. Wurm, G. Brunauer, A. Welzl-Wachter, O. Spadiut, J. Fleig and C. Herwig, Sensors, 2016, 16 Search PubMed.
- T. Anusha, K. S. Bhavani, J. V. Shanmukha Kumar, P. K. Brahman and R. Y. A. Hassan, Bioelectrochemistry, 2022, 143, 107935 CrossRef CAS.
- Y. Zhu, P. Chandra, K.-M. Song, C. Ban and Y.-B. Shim, Biosens. Bioelectron., 2012, 36, 29–34 CrossRef CAS PubMed.
-
M. Stoytcheva and R. Zlatev, Electrochemical Sensors for Environmental Analysis, in Encyclopedia of Applied Electrochemistry, ed. G. Kreysa, K. Ota and R. F. Savinell, SpringerNew York, New York, NY, 2014, pp. 613–616 Search PubMed.
- K. P. Wang, Y. C. Zhang, X. Zhang and L. Shen, SN Appl. Sci., 2019, 1, 157 CrossRef CAS.
- A. Bagheri Hashkavayi, J. B. Raoof, R. Azimi and R. Ojani, Anal. Bioanal. Chem., 2016, 408, 2557–2565 CrossRef CAS PubMed.
- J. Yin, W. Guo, X. Qin, J. Zhao, M. Pei and F. Ding, Sens. Actuators, B, 2017, 241, 151–159 CrossRef CAS.
- J. Yin, W. Guo, X. Qin, M. Pei, L. Wang and F. Ding, New J. Chem., 2016, 40, 9711–9718 RSC.
- Y. Yin, X. Qin, Q. Wang and Y. Yin, RSC Adv., 2016, 6, 39401–39408 RSC.
- K. Ghanbari and M. Roushani, Bioelectrochemistry, 2018, 120, 43–48 CrossRef CAS PubMed.
- W. Lian, S. Liu, J. Yu, J. Li, M. Cui, W. Xu and J. Huang, Biosens. Bioelectron., 2013, 44, 70–76 CrossRef CAS.
- X. Wu, H. Kuang, C. Hao, C. Xing, L. Wang and C. Xu, Biosens. Bioelectron., 2012, 33, 309–312 CrossRef CAS.
- R. Campanile, E. Scardapane, A. Forente, C. Granata, R. Germano, R. Di Girolamo, A. Minopoli, R. Velotta, B. Della Ventura and V. Iannotti, Nanomaterials, 2020, 10, 1526 CrossRef CAS.
- B. Y. Zhao, Q. Wei, C. Xu, H. Li, D. Wu, Y. Cai, K. Mao, Z. Cui and B. Du, Sens. Actuators, B, 2011, 155, 618–625 CrossRef.
- S. Yu, Q. Wei, B. Du, D. Wu, H. Li, L. Yan, H. Ma and Y. Zhang, Biosens. Bioelectron., 2013, 48, 224–229 CrossRef CAS.
- L. Tian, Y. Zhang, L. Wang, Q. Geng, D. Liu, L. Duan, Y. Wang and J. Cui, ACS Appl. Mater. Interfaces, 2020, 12, 52713–52720 CrossRef CAS.
- L. Wang, X. Peng, H. Fu, C. Huang, Y. Li and Z. Liu, Biosens. Bioelectron., 2020, 147, 111777 CrossRef CAS.
-
M. Singh, R. Kumar, D. Tandon, P. Sood and M. Sharma, 2020 IEEE International Conference on Communication, Networks and Satellite (Comnetsat), IEEE, 2020, pp. 50–54.
- J. Lv, S. Deng and L. Zhang, Biosaf. Health, 2021, 3, 22–31 CrossRef.
- H. J. Lau, C. H. Lim, S. C. Foo and H. S. Tan, Curr. Genet., 2021, 67, 421–429 CrossRef CAS.
- S. Raisch and S. Krakowski, Acad. Manag. Rev., 2021, 46, 192–210 CrossRef.
- N. Ahmed, Z. Ali, M. Riaz, B. Zeshan, J. I. Wattoo and M. N. Aslam, Asian Pac. J. Cancer Prev., 2020, 21, 1333 CrossRef CAS.
- A. Zafar, R. Hasan, S. Q. Nizami, L. von Seidlein, S. Soofi, T. Ahsan, S. Chandio, A. Habib, N. Bhutto and F. J. Siddiqui, Int. J. Infect. Dis., 2009, 13, 668–672 CrossRef PubMed.
- Y. Sun, J. Zhao and L. Liang, Microchim. Acta, 2021, 188, 21 CrossRef CAS.
- X. Liu, T. Wang, Y. Lu, W. Wang, Z. Zhou and Y. Yan, Sens. Actuators, B, 2019, 289, 242–251 CrossRef CAS.
- J. Wen, D. Jiang, X. Shan, W. Wang, F. Xu and Z. Chen, Analyst, 2021, 146, 3493–3499 RSC.
|
This journal is © The Royal Society of Chemistry 2024 |
Click here to see how this site uses Cookies. View our privacy policy here.