DOI:
10.1039/D2LC00716A
(Perspective)
Lab Chip, 2023,
23, 1339-1348
Conformational-switch biosensors as novel tools to support continuous, real-time molecular monitoring in lab-on-a-chip devices
Received
1st August 2022
, Accepted 7th November 2022
First published on 19th January 2023
Abstract
Recent years have seen continued expansion of the functionality of lab on a chip (LOC) devices. Indeed LOCs now provide scientists and developers with useful and versatile platforms across a myriad of chemical and biological applications. The field still fails, however, to integrate an often important element of bench-top analytics: real-time molecular measurements that can be used to “guide” a chemical response. Here we describe the analytical techniques that could provide LOCs with such real-time molecular monitoring capabilities. It appears to us that, among the approaches that are general (i.e., that are independent of the reactive or optical properties of their targets), sensing strategies relying on binding–induced conformational change of bioreceptors are most likely to succeed in such applications.
Introduction
The past half century has seen enormous strides in the miniaturization of technologies ranging from computers and physical sensors to, more recently, multi-step, traditionally bench-top laboratory procedures.1–3 The advantages of miniaturization are obvious: lower costs, the power of parallelization, and, for miniature lab-on-a-chip (LOC) devices, minimal consumption of sample, an advance that opens up fundamentally new applications.4,5 Indeed, the progress we observed in this field is mesmerizing; in just a few years we passed from simple, single, linear microchannels on a chip used as “proof of concept”, to the recapitulation of multi-step (including functionalization, incubation, washing), benchtop laboratory assays used by researchers to study intricate biological events.6–12
The success of LOC approaches notwithstanding, there remains a broad category of bench-top procedures that have not yet seen significant implementation in automated, microfluidic processes: real-time chemical analysis (Fig. 1).13–16 That is, while a number of researchers have successfully and usefully integrated feedback control informed by physical measurements (e.g., of fluid height17 and speed,18 and bead position19) in LOC devices, to date no one has reported using the real-time measurement of the concentration of a specific molecular species to perform on-chip, feedback-controlled actuation.20–22 Here we discuss, as we see them, the challenges and opportunities associated with the ability to perform such real-time, high-frequency molecular analysis and use that to drive a closed-loop, feedback-controlled response.
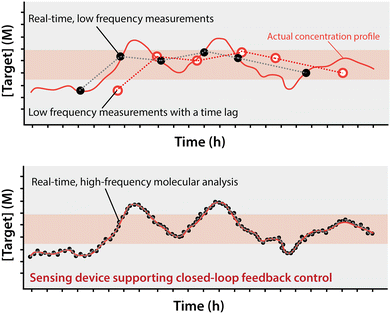 |
| Fig. 1 Here we review molecular monitoring approaches that are both real-time and continuous, or at least perform measurements at a frequency rapid relative to any significant fluctuations in the system. | |
To meet the vision we paint here, of closed-loop feedback control over molecular processes, will require measurement technologies that achieve a number of (often challenging) attributes simultaneously. Here we discuss each of these critical needs in turn. First, the measurements need to be molecular. That is, although the measurement of conductivity, temperature, and mechanical properties are already commonly performed in LoC devices (such as for the study of the biophysics of cells23–25), measurement of the concentration of specific molecules in LoC devices has seen far less exploration.26,27 Second, the measurements must be continuous and real time. That is, to support feedback control, the measurements must be performed at a frequency that is rapid relative to the timescale of any relevant change in target concentration and any lag between that change in target concentration, and the resulting change in sensor output must be shorter than the time scale of the event or process to be controlled (Fig. 1).28 Finally, the measurements must be quantitative. For example, a device aiming at keeping the concentration of a metabolite or drug constant can more precisely correct for variations if it receives a quantitative measurement of concentration rather than just a qualitative indication that the concentration has surpassed a predefined cut off.20–22,28,29
The above-described requirements preclude the use of many commonly employed analytical approaches in feedback control systems. Specifically, these attributes are likely limited to single-step, rapidly reversible devices, rather than multi-step processes.14,30,31 For example, the approach probably should not require the modification of the target (i.e., they should be “label-free” and should not be “sandwich assays”) as, while such approaches can be automated and made continuous (or near continuous) using LOC technologies,32 the time lag associated with multi-step processes reduces their applicability to closed-loop feedback control over LOC processes, which are typically quite rapid. Likewise, the time lags and often poor measurement frequency associated with sample “pre-conditioning”, and analytical approaches that require separation (e.g., chromatography) would also hinder application in feedback. In this light, we review here the strengths and weaknesses of the state-of-the art molecular measurement approaches that match these needs, in the hopes that such a discussion will pave the way towards the integration of measure-and-respond capabilities in LOC technologies. We will limit our consideration to the detection of molecules, rather than simple inorganic ions, as ion-selective electrodes are well established.33
Before we launch into our critical review of the available approaches to real-time, on-chip molecular monitoring we feel we should also note the metrics that we did not employ in judging them.
First, we do not discuss the “linear range” of the various approaches, as the very existence of such a range is a common misperception regarding sensors, such as biosensors, that are reliant on a target-recognizing receptor. Specifically, while the output of some assays is linear in target concentration, the output of receptor-based sensors does not change linearly with target concentration. This is because, while their output is often linear in receptor occupancy, occupancy itself obeys a hyperbolic, Langmuir isotherm dependence on target concentration.34,35 And while short segments of a hyperbola can be approximated as linear, how broad a range can be so approximated depends on an arbitrary decision regarding how large a deviation from linearity one is willing to accept as “close enough”.
Similarly, we do not discuss limits of detection, as these are impossible to compare in any general, “apples-to-apples” way.36 Specifically, limits of detection depend on both the affinity of the receptor for its target (which defines receptor occupancy at a given target concentration) and the signal-to-noise of the sensor (which defines the minimum receptor occupancy required to generate a statistically significant signal change).37 Limits of detection thus vary wildly between receptor/target pairs (due to differences in affinity), between sensor architectures (due to differences in the signal change produced at a given receptor occupancy), and even between different implementations of the same receptor and sensor architecture (depending on the noise associated with that implementation).
Likewise, we limit our discussion of temporal resolution as, here too, it is difficult to perform an apples-to-apples comparison. Specifically, for some sensors temporal resolution is defined by how many times per minute the sensor can be interrogated, but for others it is defined by how rapidly the sensor equilibrates in response to a change in target concentration. Indeed, the latter is dependent on target concentration and thus, as the target concentration falls, the time resolution of a sensor can switch between a regime in which it is defined by its interrogation frequency to one in which it is defined by equilibration time.
Finally, we do not discuss sensor stability in much detail as, in our experience, this varies enormously between sensor architectures and, indeed, even for a single sensor depending on the sample matrix. Moreover, different applications require different measurement durations and, with that, have different requirements regarding sensor stability. For example, the measurement of biomarkers associated to kidney failure may require a biosensor with hours- to days-long stability, while the measurement of the product of a chemical reactions may need only minutes-long stability.
Feedback control
In the laboratory, scientists and technicians often employ single time point molecular measurements to guide sample processing, such as the adjustment of pH or ionic strength or the titration of a critical reagent. Given, however, that the real strength of LoC devices is their automated, no-human-intervention-required approach to sample processing, we believe that the equivalent ability in LoCs will be autonomous feedback control.38 Analogies in the macroscale world abound. Sensors capable of monitoring blood sugar levels in real time, for example, are now being used to control insulin dosing39–42 and have been applied in a commercially available product (e.g., the T:slim X2 Insulin Pump by Tandem Diabetes Care). Likewise, real-time, drug-monitoring biosensors have been shown to support feedback-controlled drug delivery, in which plasma drug concentrations are held constant to an unprecedented degree of precision.21,43 Here we argue that the same concept would profitably augment the power and scope of LOCs.
Feedback control systems are comprised of three critical elements (Fig. 2). One is a sensor that can monitor a time-varying property of interest (here the concentration of a specific molecule) in real time. Another is the actuator, the portion of the system that provides a response to the time varying property of interest in order to optimize it. Linking the two is the control algorithm that, taking input from the sensor, identifies the actuator response that will most optimally achieve the desired system behaviour.
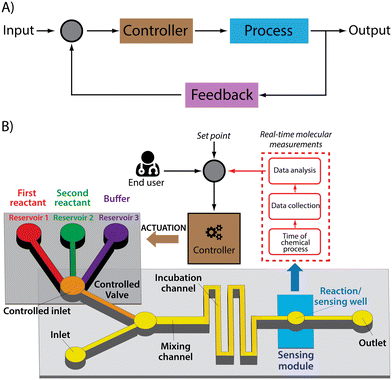 |
| Fig. 2 A) Closed loop feedback control employs real-time measurements of a desired output to inform a control algorithm that continuously adjust the system's inputs to sure the output remains with a desired bounds. B) The integration of real-time molecular measurements into LOC applications would provide opportunities for performing such feedback-control. By measuring molecular concentrations continuously (or at least at a frequency greater than the event of interest), LOCs can, instead of acting as inflexible reactors, optimize themselves in real-time to respond to changing molecular conditions. | |
While the sensing and actuating elements of feedback control systems are application-dependent (i.e., their characteristics cannot be generalized, but must be tailored to a given role), feedback control algorithms have several characteristics that are constant across feedback control systems. Specifically, the software employed in such devices must process the data and control the actuator with a time resolution that allows to change the system at a frequency meaningful for the specific application.28,44,45
Real-time molecular monitoring
In this perspective, we focus on generalizable sensor approaches. That is, even though they may support real-time continuous monitoring, we do not discuss sensing modalities based on the intrinsic physical properties (e.g., target fluorescence or absorbance), chemical reactivity (e.g., target redox chemistry), or enzymatic reactivity (e.g., the ability of an enzyme to convert the target into a coloured, fluorescent or redox active species) of the target. The reason we are ignoring these sometimes-important approaches is that they are only applicable to those (rare) molecular targets characterized by suitable physical properties or reactivity, or for which a suitable converting enzyme is available. For those readers interested in the topic we provide here some useful reference.46–53 Instead, in this perspective manuscript, we focus our discussion on sensing modalities that are independent of the physical properties and chemical and enzymatic reactivity of their targets. That is, we focus on sensors that employ target-binding receptors, such as antibodies, antibody mimics,54,55 or aptamers (antibody-like nucleic acids),56–58 that can be generated against effectively any water-soluble molecule.59 This potentially enormous advantage, however, is counterweighted by a potentially significant limitation: the binding of a target molecule to such a receptor does not usually produce any measurable signal (e.g., does not produce photons or electrons), leaving open the question of how to couple target recognition to an easily measurable output.
Many solutions to the above-described “signal transduction problem” have been described that support real-time molecular analysis. Broadly speaking, however, we can group these into two categories: (1) those that employ changes in mass, charge, or optical properties associated with the adsorption of a target to a receptor-coated surface, and (2) those that employ binding-induced changes in the physics (e.g., the conformation or dynamics) of a receptor to generate an output.
Receptor-based sensors relying on adsorption-linked physical changes
Adsorption-based sensors include such approaches as field effect transistors (FET), electrochemical impedance spectroscopy (EIS), quartz crystal microbalances (QCM), surface acoustic wave (SAW), surface plasmon resonance (SPR), and microcantilevers (Fig. 3). These approaches are label free (i.e., they do not require chemical modification of the target to generate a signal) and measure at high-frequency, thus supporting effectively continuous, real-time monitoring.
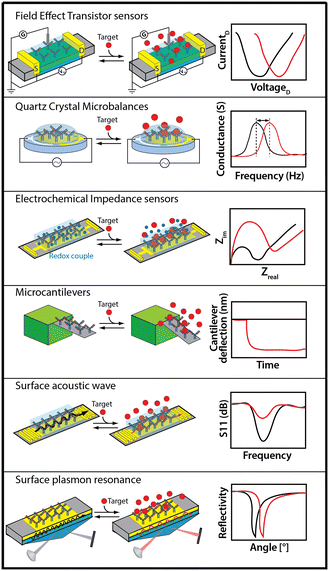 |
| Fig. 3 Approaches that monitor the adsorption of the target to a receptor-coated surface via the associated change in charge, mass, steric bulk, or optical properties may prove suitable for the development of measure-and-act LOC technologies. | |
Field effect transistors.
Monitor the change in electrostatic potential that often occurs when a target molecule adsorbs to a surface to gate a transistor, which in turn affects the drain current of the semiconductor channel.60–62 Using this approach, many groups have described sensors for the detection of charged macromolecules or small molecules (via binding-induced changes in the shape of a charged receptor) of remarkably low limits of detection (low-to sub-picomolar).61–65 And although existing examples are still limited, field effect transistors have been integrated into microfluidics in support of continuous target monitoring; including studies in which both protein biomarkers66 and general sweat composition67 were measured.
Electrochemical impedance spectroscopy.
Is an electrochemical technique that measures the effective resistance (impedance) of a system to passing an alternating current.68,69 The accumulation of the target of interest on the electrode surface generates a measurable change in this impedance, either by blocking the surface sterically (for macromolecular targets) or by causing the shape of a receptor to change (small molecule targets).70 In recent years, this approach has gained in popularity in bench-top laboratory experiments thanks to being label-free and low cost. This said, studies introducing this technique into lab-on-a-chip devices have primarily focused on the analysis of micrometre size analytes, such as whole cells, with few monitoring molecular targets.71–75
Quartz crystal microbalances and microcantilevers.
Are sensing techniques relying on modification of the vibration frequency of a resonator induced by the mass of an adsorbed target molecule.76–81 Although in principle they have the potential to carry out real time molecular sensing of macromolecular targets,82 they have not seen any realization in LOC applications that we are aware of. This is presumably due to their need for highly controlled environment (e.g., they are sensitive to vibrations, and require precise control of temperature and sample flow rates).83
Surface acoustic wave.
Sensors transform an electrical signal into a mechanical wave, which is highly sensitive to perturbation provoked by physical phenomena (such as the binding of a target molecule to a receptor on the sensor surface) with target binding being detected via changes in the acoustic wave's amplitude, phase or frequency.84–86 As needed to support on-chip feedback control, the technique supports effectively continuous measurements87 and is easily integrated in microfluidic devices.84 We are not aware, however, of any examples in which such molecular sensors have been implemented in a LOC format. This despite the fact that surface acoustic wave sensors are able not only to detect targets, but also to “act” on the system in response to such measurements by, for example, generating an aerosol88 or bubbles,89 or driving mixing.90 Indeed, using real-time image analysis to placement, surface acoustic waves have been used to position beads in LOC devices in a feedback-controlled format.19
Surface plasmon resonance.
Sensors measure perturbations on the resonant oscillation of conduction electrons at the sensor surface generated by the adsorption of target molecules.91–93 Bench-top examples of this technology, such as the widely used Biacore®, are routinely employed to characterize biomolecular binding. Their integration into LOCs has also found many applications, especially for the development of point-of-care diagnostic devices.94 This said, however, we have not yet seen examples of on-chip SPR being used for closed-loop monitoring and actuation on chips.95,96
The pros and cons of relying on adsorption.
When coupled with the generality of antibodies and aptamers, adsorption-based sensing strategies are extremely versatile, and many have proven amenable to successful integration into LOC architectures. The transduction mechanisms underlying these approaches, however, fail when challenged with complex sample matrices. For example, variations in sample viscosity affect the output of surface acoustic wave sensors,97 and changes in the ionic strength can perturb the output of impedimetric measurements.70 Worse, the non-specific adsorption of interferents from complex samples (such as whole blood) generates significant, false signals in all adsorption-based approaches as these, too, produce significant changes in mass, electric field, etc.98 Undoubtedly their integration in dedicated LOC may solve some of these limitations by, for example, supporting automated sample preparation.87,99 But the delays inherent in such preparation likely preclude the sort of real-time sensing and responding that is the focus of our thinking here.
Techniques relying on binding-induced changes in receptor physics
An alternative to adsorption-based biosensor approaches are biosensor architectures that employ the same mechanism that nature employs to achieve real-time molecular monitoring in the cell: binding-induced changes in the physics of a receptor.100 That is, instead of monitoring binding-linked changes in mass, charge, etc., nature employs binding-induced changes in a receptor's conformation, oligomerization state, or dynamics to convert a binding event into an easily detectable output (Fig. 4).
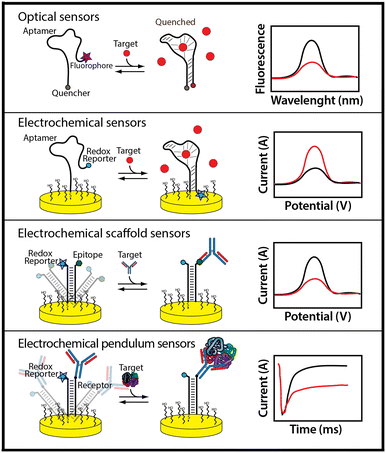 |
| Fig. 4 Employing binding-induced conformational change to produce an optical or electrochemical output appears an excellent option for performing continuous, real-time molecular monitoring in support of feedback control functionalities. | |
The use of binding-induced conformational changes in biosensing is a fairly recent advance.100 To achieve it requires the availability of a receptor that undergoes a large-scale shape change upon target binding. Fortunately, systematic ways of engineering this property into single-domain proteins and nucleic acids are now well established. When these are coupled with in vitro selection schemes it is now possible to generate aptamers (artificially selected receptors comprised of DNA or RNA),58 polypeptides, and single domain proteins101,102 that bind any of a wide range of specific macromolecular and small-molecule targets and, in doing so, undergo a binding-induced conformational change that can be used to generate an optical or electrochemical output.
Optical beacons.
Employ fluorescent read-outs to report on a binding-induced conformational change.103,104 The first reported of these, molecular beacons, are stem-loop DNA structures modified at their ends with a fluorophore and a quencher. In the absence of their target the stem-loop structure holds the fluorophore/quencher pair in proximity, reducing fluorescence. The binding of a nucleic acid target to the loop opens the stem, separating the fluorophore/quencher pair and enhancing fluorescence. A similar approach can be employed using aptamers by introducing the aptamer sequence into the loop such that its binding-induced conformational change opens or closes the stem, producing a change in fluorescence.105 Historically, the primary limitation of the aptamer beacon approach was the limited availability of sufficiently high-performance aptamers.106 Fortunately, however, recent years have seen significant advances in aptamer selection, which should facilitate their translation into such applications.107,108
Electrochemical DNA (E-DNA)109,110 and electrochemical aptamer-based (EAB) sensors.
111
Are beacon-like sensors in which the optical output of beacons is replaced with an electrochemical read-out. These sensors are comprised of a target-recognizing stem-loop or aptamer attached via one end to an electrode surface via formation of a mixed monolayer and modified on the other end with a redox reporter (e.g., methylene blue) that provides an electrochemical signal. The binding-induced conformational change of this receptor in turn alters the electron transfer rate of the redox reporter, producing an easily measurable output when the sensor is interrogated via, for example, square wave voltammetry.106,112 Of relevance to our theme, the reagentless, reversible nature of their signalling renders these sensors able to monitor molecular concentrations in real time and with high frequency (a few times a second to every few seconds).113,114 And the selectivity of this signal transduction mechanism ensures that they work well even when deployed directly in complex sample streams, including undiluted whole blood and even in situ in the bodies of live animals.20,29,115–122 Given that electrochemical aptamer-based sensors are (1) reversible, reagentless, real-time, selective enough to deploy directly in complex sample streams, (2) generalizable to a wide range of targets, and (3) can be fabricated on micron-scale electrodes123 the approach results easily integration into LOC applications (Fig. 3).43
Molecular pendulums.
Are a still more recent approach to coupling target recognition to a change in receptor physics, one that employs binding-induced changes in the dynamics of a receptor attached to a rigid lever arm. In this, a short, double-stranded DNA is used as “scaffold” that attached at one end to an electrode surface via a flexible linker and modified on the other with a receptor and either an optical124 or electrochemical109,125 reporter. Upon the binding of a macromolecular target to the receptor, steric blocking126 or changes in the hydrodynamic radius of the complex124,125 alter the dynamics with which the reporter approaches the surface, causing a change in electrochemical or optical output. Using an electrochemical output, Kelley and co-worker have demonstrated the continuous, real-time measurement of troponin in saliva using a sensor in this class.125
The advantages of using binding-induced changes in receptor physics.
Techniques relying on binding-induced conformational change meet the criteria required to support feedback control: they are quantitative molecular sensors that do not require reagent addition or wash steps and respond rapidly and in real time to changing target concentrations. In addition, their use of this biomimetic signal transduction mechanism renders this class of sensor surprisingly impervious to false positives associated with non-specific adsorption, meaning that they achieve these attributes even when challenged with complex sample matrices.106
Example systems
With a deeper understanding in hand regarding potential approaches to performing real-time molecular analysis in complex sample streams, we now briefly present several example systems and applications (Fig. 5). Our examples illustrate the breadth of the sensor landscape and reveal the impact of sensor choice on system complexity. However, while they highlight the ability to integrate continuous, real-time monitoring in microfluidic devices, none of our examples couples these measurements with closed-loop feedback-controlled actuation, which we believe remains a valuable, untapped advance in LOC applications.
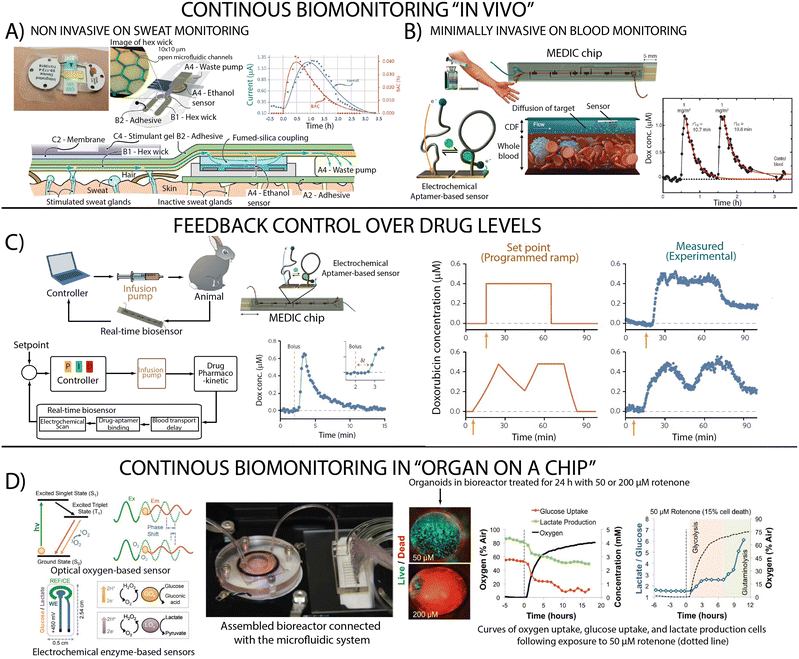 |
| Fig. 5 Example systems and applications including (A) in vivo continuous sweat monitoring with an enzymatic sensor (reproduced from ref. 127 with permission from the Royal Society of Chemistry), (B) continuous blood monitoring system that employs electrochemical aptamer-based sensors (from ref. 43. Reprinted with permission from AAAS) and (C) their integration to support feedback control over drug levels in live animals (reprinted by permission from Springer-Nature: ref. 128 copyright 2017). (D) Real-time measurements in organ-on-a-chip to achieve the monitoring of oxygen, glucose and lactate using optical and enzymatic sensors (reproduced from ref. 129 copyright 2017 National Academy of Sciences). | |
Continuous biomonitoring
The continuous monitoring of biomarkers (e.g., metabolites, proteins indicative of disease) has seen extensive development of technologies for sampling biofluids and continuous measurement of analytes in those biofluids, with interstitial fluid and sweat being particularly viable.130 A recently described sweat biosensor (Fig. 5A),127 for example, utilizes an enzymatic sensor to continuously measure sweat ethanol, which strongly correlates with blood ethanol. Continuous measurement of circulating therapeutic concentrations of drugs in blood has also been demonstrated using electrochemical aptamer-based sensors (Fig. 5B) and even integrated with “off-chip” feedback control over drug levels in live animals (Fig. 5C).43
Continuous monitoring in “organ on chip” devices
Monitoring the behaviour of tissues and organoids in highly controlled environments is advancing our understanding of diseases and their treatments, an area in which we believe real-time, on-chip molecular monitoring could contribute significantly. For example, organs in the body benefit from the fact that the composition of the blood that bathes them is regulated via feedback control by, for example, the liver. Performing similar monitoring of –and control over– the nutrient bath that keeps the organoid healthy would likely also be of value. Similarly, with the appropriate input, optogenetics can be used to perform feedback control of cellular processes.131,132 Briefly these systems harness the response of various light-activated proteins (channels, pumps and enzymes) to generate an actuation at the molecular scale. Using optogenetics, researchers developed feedback control system that regulate cellular growth,133 bacterial co-culture composition,134 gene expression,135 intracellular signalling dynamics.136 To date, however, real-time measurements in organ-on-a-chip applications are limited to the monitoring of oxygen137,138 or cell integrity (Fig. 5D).139
Future perspective
While we did not discuss enzymatic sensors (due to their limited generalizability), it is worth considering the most successful individual molecular sensor, the enzymatic “continuous glucose monitor”, as an example of the potential that real-time molecular monitoring can achieve. This commercially available sensor uses glucose oxidase to oxidize glucose (using endogenous oxygen as a reagent), producing hydrogen peroxide, which, in turn can be detected electrochemically.140 Many groups have demonstrated the real-time measuring of glucose, with much of this work focusing on the development of wearable and implantable diagnostic devices.141–145 The impact of those devices in the life of diabetic patient is unvaluable. Now the question is, why are similar strategies not widespread and mostly confined to glucose sensing? In this perspective manuscript we gave our answer: the lack of biosensors that can actually support real-time continuous monitoring in a generalizable way. However, this manuscript also shows how we and other research groups are actively trying to solve this limitation with the development of biosensors relying on the use of binding-induced conformational change bioreceptors.
Conclusions
During the last ten years researchers in the field of LOCs succeeded in developing devices capable to carry out important functions. But as yet demonstrations of real-time molecular monitoring on LOCs have been rare, and the integration of these in closed-loop, feedback control “sense-and-actuate” systems has been non-existent. Looking forward, however, we believe the combination of the increasingly mature field of LOC with the likewise maturing field of real time molecular sensing offers the promise of unprecedented functionalities. On one side, we expect new LOCs to provide solutions for the low specificity and delicate operation of adsorption-based techniques, generating new ultra-sensitive, real-time molecular monitoring devices. On the other side, we expect that the integration of real-time monitoring capabilities into microfluidic devices will allow developer to focus on the development of new actuating functionalities into LOCs.
Conflicts of interest
KWP serves on the scientific advisory boards of several companies that are commercializing electrochemical biosensors, albeit not in LOC applications.
Acknowledgements
UCSB author acknowledges partial support from the NIH through award R01EB022015. The Univ. of Cincinnati author acknowledge partial support from the National Science Foundation ECCS Award #1608275, and ECCS Award #2025720, and Air Force Research Labs (USAF Contract No. FA9550-20-1-0117). C. P. (ISGlobal) acknowledges support from the Spanish Ministry of Science and Innovation and State Research Agency through the program PID2020-116770RJ-I00 and the “Centro de Excelencia Severo Ochoa 2019-2023” Program (CEX2018-000806-S), support from the Generalitat de Catalunya through the CERCA Program, and support from the Hospital Clinic of Barcelona.
Notes and references
- B. Derkus, Biosens. Bioelectron., 2016, 79, 901–913 CrossRef CAS PubMed.
- R. Costa, Crit. Rev. Anal. Chem., 2014, 44, 299–310 CrossRef CAS PubMed.
- S. Lin, E. K. Lee, N. Nguyen and M. Khine, Lab Chip, 2014, 14, 3475–3488 RSC.
- S. Vyawahare, A. D. Griffiths and C. A. Merten, Chem. Biol., 2010, 17, 1052–1065 CrossRef CAS PubMed.
- J. Park, D. H. Han and J. K. Park, Lab Chip, 2020, 20, 1191–1203 RSC.
- J. Park, I. Wetzel, D. Dréau and H. Cho, Adv. Healthcare Mater., 2018, 7, 1700551 CrossRef PubMed.
- H. Montón, M. Medina-Sánchez, J. A. Soler, A. Chałupniak, C. Nogués and A. Merkoçi, Biosens. Bioelectron., 2017, 94, 408–414 CrossRef PubMed.
- J. Nawroth, J. Rogal, M. Weiss, S. Y. Brucker and P. Loskill, Adv. Healthcare Mater., 2018, 7, 1700550 CrossRef PubMed.
- S. H. Lee and J. H. Sung, Adv. Healthcare Mater., 2018, 7, 1700419 CrossRef.
- P. Tseng, C. Murray, D. Kim and D. Di Carlo, Lab Chip, 2014, 14, 1491 RSC.
- Q. Zhou, D. Patel, T. Kwa, A. Haque, Z. Matharu, G. Stybayeva, Y. Gao, A. M. Diehl and A. Revzin, Lab Chip, 2015, 15, 4467–4478 RSC.
- H. Azizgolshani, J. R. Coppeta, E. M. Vedula, E. E. Marr, B. P. Cain, R. J. Luu, M. P. Lech, S. H. Kann, T. J. Mulhern, V. Tandon, K. Tan, N. J. Haroutunian, P. Keegan, M. Rogers, A. L. Gard, K. B. Baldwin, J. C. de Souza, B. C. Hoefler, S. S. Bale, L. B. Kratchman, A. Zorn, A. Patterson, E. S. Kim, T. A. Petrie, E. L. Wiellette, C. Williams, B. C. Isenberg and J. L. Charest, Lab Chip, 2021, 21, 1454–1474 RSC.
- V. Chan, H. H. Asada and R. Bashir, Lab Chip, 2014, 14, 653–670 RSC.
- M. C. Brothers, M. Debrosse, C. C. Grigsby, R. R. Naik, S. M. Hussain, J. Heikenfeld and S. S. Kim, Acc. Chem. Res., 2019, 52, 297–306 CrossRef CAS PubMed.
- J. Li, J. Y. Liang, S. J. Laken, R. Langer and G. Traverso, Trends Chem., 2020, 2, 319–340 CrossRef CAS.
- S. Campuzano, M. Pedrero, M. Gamella, V. Serafín, P. Yáñez-Sedeño and J. M. Pingarrón, Sensors, 2020, 20, 1–22 Search PubMed.
- L. R. Soenksen, T. Kassis, M. Noh, L. G. Griffith and D. L. Trumper, Lab Chip, 2018, 18, 902–914 RSC.
- N. Wang, R. Liu, N. Asmare, C.-H. Chu, O. Civelekoglu and A. F. Sarioglu, Lab Chip, 2021, 21, 1916–1928 RSC.
- T. D. Nguyen, Y. Q. Fu, V. T. Tran, A. Gautam, S. Pudasaini and H. Du, Sens. Actuators, B, 2020, 318, 128143 CrossRef CAS.
- P. Dauphin-Ducharme, K. Yang, N. Arroyo-Currás, K. L. Ploense, Y. Zhang, J. Gerson, M. Kurnik, T. E. Kippin, M. N. Stojanovic and K. W. Plaxco, ACS Sens., 2019, 4, 2832–2837 CrossRef CAS PubMed.
- N. Arroyo-Currás, G. Ortega, D. A. Copp, K. L. Ploense, Z. A. Plaxco, T. E. Kippin, J. P. Hespanha and K. W. Plaxco, ACS Pharmacol. Transl. Sci., 2018, 1, 110–118 CrossRef PubMed.
- P. L. Mage, B. S. Ferguson, D. Maliniak, K. L. Ploense, T. E. Kippin and H. T. Soh, Nat. Biomed. Eng., 2017, 1, 1–10 CrossRef CAS.
- H. Kavand, R. Nasiri and A. Herland, Adv. Mater., 2022, 34, 2107876 CrossRef CAS.
- G. Caluori, J. Pribyl, M. Pesl, S. Jelinkova, V. Rotrekl, P. Skladal and R. Raiteri, Biosens. Bioelectron., 2019, 124–125, 129–135 CrossRef CAS PubMed.
- J. R. Soucy, A. J. Bindas, A. N. Koppes and R. A. Koppes, iScience, 2019, 21, 521–548 CrossRef PubMed.
- A. Weltin, S. Hammer, F. Noor, Y. Kaminski, J. Kieninger and G. A. Urban, Biosens. Bioelectron., 2017, 87, 941–948 CrossRef CAS PubMed.
- A. D. Simmons, C. Williams, A. Degoix and V. I. Sikavitsas, Biosens. Bioelectron., 2017, 90, 443–449 CrossRef CAS PubMed.
- S. D. Curtis, K. L. Ploense, M. Kurnik, G. Ortega, C. Parolo, T. E. Kippin, K. W. Plaxco and N. Arroyo-Currás, Anal. Chem., 2019, 91, 12321–12328 CrossRef CAS PubMed.
- A. Idili, N. Arroyo-Currás, K. L. Ploense, A. T. Csordas, M. Kuwahara, T. E. Kippin and K. W. Plaxco, Chem. Sci., 2019, 10, 8164–8170 RSC.
- O. Simoska and K. J. Stevenson, Analyst, 2019, 144, 6461–6478 RSC.
- S. Campuzano, M. Pedrero, M. Gamella, V. Serafín, P. Yáñez-Sedeño and J. M. Pingarrón, Sensors, 2020, 20, 1–22 Search PubMed.
- M. Poudineh, C. L. Maikawa, E. Y. Ma, J. Pan, D. Mamerow, Y. Hang, S. W. Baker, A. Beirami, A. Yoshikawa, M. Eisenstein, S. Kim, J. Vučković, E. A. Appel and H. T. Soh, Nat. Biomed. Eng., 2020, 5, 53–63 CrossRef PubMed.
- Y. Shao, Y. Ying and J. Ping, Chem. Soc. Rev., 2020, 49, 4405–4465 RSC.
- F. Ricci, A. Vallée-Bélisle, A. J. Simon, A. Porchetta and K. W. Plaxco, Acc. Chem. Res., 2016, 49, 1884–1892 CrossRef CAS PubMed.
- E. C. Hulme and M. A. Trevethick, Br. J. Pharmacol., 2010, 161, 1219–1237 CrossRef CAS PubMed.
- G. Gauglitz, Anal. Bioanal. Chem., 2017, 410, 5–13 CrossRef PubMed.
- B. D. Wilson and H. T. Soh, Trends Biochem. Sci., 2020, 45, 639–649 CrossRef CAS PubMed.
- A. D. Mickle, S. M. Won, K. N. Noh, J. Yoon, K. W. Meacham, Y. Xue, L. A. McIlvried, B. A. Copits, V. K. Samineni, K. E. Crawford, D. H. Kim, P. Srivastava, B. H. Kim, S. Min, Y. Shiuan, Y. Yun, M. A. Payne, J. Zhang, H. Jang, Y. Li, H. H. Lai, Y. Huang, S. Il Park, R. W. Gereau and J. A. Rogers, Nature, 2019, 565, 361–365 CrossRef CAS PubMed.
- H. Teymourian, A. Barfidokht and J. Wang, Chem. Soc. Rev., 2020, 49, 7671–7709 RSC.
- A. Heller and B. Feldman, Chem. Rev., 2008, 108, 2482–2505 CrossRef CAS PubMed.
- J. D. Zahn, Y. C. Hsieh and M. Yang, Diabetes Technol. Ther., 2005, 7, 536–545 CrossRef CAS PubMed.
- J. Li, J. Y. Liang, S. J. Laken, R. Langer and G. Traverso, Trends Chem., 2020, 2, 319–340 CrossRef CAS.
- B. S. Ferguson, D. A. Hoggarth, D. Maliniak, K. Ploense, R. J. White, N. Woodward, K. Hsieh, A. J. Bonham, M. Eisenstein, T. E. Kippin, K. W. Plaxco and H. T. Soh, Sci. Transl. Med., 2013, 5, 213ra165 Search PubMed.
- S. Kumar and M. Khammash, Front. Bioeng. Biotechnol., 2022, 10, 918917 CrossRef PubMed.
- L. S. Kumari and A. Z. Kouzani, IEEE Rev. Biomed. Eng., 2022, 16, 91–108 Search PubMed.
- L. C. Tai, C. H. Ahn, H. Y. Y. Nyein, W. Ji, M. Bariya, Y. Lin, L. Li and A. Javey, ACS Sens., 2020, 5, 1831–1837 CrossRef CAS PubMed.
- A. J. Bandodkar, W. J. Jeang, R. Ghaffari and J. A. Rogers, Annu. Rev. Anal. Chem., 2019, 12, 1–22 CrossRef PubMed.
- J. Tu, R. M. Torrente-Rodríguez, M. Wang and W. Gao, Adv. Funct. Mater., 2020, 30, 1906713 CrossRef CAS.
- J. R. Sempionatto, V. R. V. Montiel, E. Vargas, H. Teymourian and J. Wang, ACS Sens., 2021, 6, 1745–1760 CrossRef CAS PubMed.
- A. Clifford, J. Das, H. Yousefi, A. Mahmud, J. B. Chen and S. O. Kelley, J. Am. Chem. Soc., 2021, 143, 5281–5294 CrossRef CAS PubMed.
- Y. Yang and W. Gao, Chem. Soc. Rev., 2019, 48, 1465–1491 RSC.
- S. M. Khor, J. Choi, P. Won and S. H. Ko, J. Nanomater., 2022, 12, 221 CrossRef CAS PubMed.
- Y. J. Heo and S. Takeuchi, Adv. Healthcare Mater., 2013, 2, 43–56 CrossRef CAS PubMed.
- O. S. Oliinyk, M. Baloban, C. L. Clark, E. Carey, S. Pletnev, A. Nimmerjahn and V. V. Verkhusha, Nat. Methods, 2022, 19, 740–750 CrossRef CAS PubMed.
- A. Idili, A. Bonini, C. Parolo, R. Alvarez-Diduk, F. Di Francesco and A. Merkoçi, Adv. Funct. Mater., 2022, 2201881 CrossRef CAS.
- R. Micura and C. Höbartner, Chem. Soc. Rev., 2020, 49, 7331–7353 RSC.
- M. Debiais, A. Lelievre, M. Smietana and S. Müller, Nucleic Acids Res., 2020, 48, 3400–3422 CrossRef CAS PubMed.
- T. Mairal, V. C. Özalp, P. L. Sánchez, M. Mir, I. Katakis and C. K. O'Sullivan, Anal. Bioanal. Chem., 2008, 390, 989–1007 CrossRef CAS PubMed.
- S. Sang, Y. Wang, Q. Feng, Y. Wei, J. Ji and W. Zhang, Crit. Rev. Biotechnol., 2016, 36, 465–481 CAS.
- M. Magliulo, K. Manoli, E. Macchia, G. Palazzo and L. Torsi, Adv. Mater., 2015, 27, 7528–7551 CrossRef CAS PubMed.
- S. Mao, J. Chang, H. Pu, G. Lu, Q. He, H. Zhang and J. Chen, Chem. Soc. Rev., 2017, 46, 6872–6904 RSC.
- I. M. Bhattacharyya, S. Cohen, A. Shalabny, M. Bashouti, B. Akabayov and G. Shalev, Biosens. Bioelectron., 2019, 132, 143–161 CrossRef CAS PubMed.
- K. Shoorideh and C. O. Chui, Proc. Natl. Acad. Sci. U. S. A., 2014, 111, 5111–5116 CrossRef CAS PubMed.
- V. Parkula, M. Berto, C. Diacci, B. Patrahau, M. Di Lauro, A. Kovtun, A. Liscio, M. Sensi, P. Samorì, P. Greco, C. A. Bortolotti and F. Biscarini, Anal. Chem., 2020, 92, 9330–9337 CrossRef CAS PubMed.
- N. Nakatsuka, K. A. Yang, J. M. Abendroth, K. M. Cheung, X. Xu, H. Yang, C. Zhao, B. Zhu, Y. S. Rim, Y. Yang, P. S. Weiss, M. N. Stojanović and A. M. Andrews, Science, 2018, 362, 319–324 CrossRef CAS PubMed.
- N. I. Khan, M. Mousazadehkasin, S. Ghosh, J. G. Tsavalas and E. Song, Analyst, 2020, 145, 4494–4503 RSC.
- E. Garcia-Cordero, F. Bellando, J. Zhang, F. Wildhaber, J. Longo, H. Guérin and A. M. Ionescu, ACS Nano, 2018, 12, 12646–12656 CrossRef CAS PubMed.
- A. Hedayatipour, S. Aslanzadeh and N. McFarlane, Biosens. Bioelectron., 2019, 143, 111600 CrossRef CAS PubMed.
- M. Varshney and Y. Li, Biosens. Bioelectron., 2009, 24, 2951–2960 CrossRef CAS PubMed.
- B. Y. Chang and S. M. Park, Annu. Rev. Anal. Chem., 2010, 3, 207–229 CrossRef CAS.
- M. W. van der Helm, O. Y. F. Henry, A. Bein, T. Hamkins-Indik, M. J. Cronce, W. D. Leineweber, M. Odijk, A. D. van der Meer, J. C. T. Eijkel, D. E. Ingber, A. van den Berg and L. I. Segerink, Lab Chip, 2019, 19, 452–463 RSC.
- Y. Xu, X. Xie, Y. Duan, L. Wang, Z. Cheng and J. Cheng, Biosens. Bioelectron., 2016, 77, 824–836 CrossRef CAS PubMed.
- P. Capaldo, S. R. Alfarano, L. Ianeselli, S. D. Zilio, A. Bosco, P. Parisse and L. Casalis, ACS Sens., 2016, 1, 1003–1010 CrossRef CAS.
- C. Russell, A. C. Ward, V. Vezza, P. Hoskisson, D. Alcorn, D. P. Steenson and D. K. Corrigan, Biosens. Bioelectron., 2019, 126, 806–814 CrossRef CAS PubMed.
- G. Rosati, M. Urban, L. Zhao, Q. Yang, C. de Carvalho Castro e Silva, S. Bonaldo, C. Parolo, E. P. Nguyen, G. Ortega, P. Fornasiero, A. Paccagnella and A. Merkoçi, Biosens. Bioelectron., 2022, 196, 113737 CrossRef CAS PubMed.
- J. Y. Chen, L. S. Penn and J. Xi, Biosens. Bioelectron., 2018, 99, 593–602 CrossRef CAS PubMed.
- C. Zheng, X. Wang, Y. Lu and Y. Liu, Food Control, 2012, 26, 446–452 CrossRef CAS.
- S. Cotrone, D. Cafagna, S. Cometa, E. De Giglio, M. Magliulo, L. Torsi and L. Sabbatini, Anal. Bioanal. Chem., 2012, 402, 1799–1811 CrossRef CAS PubMed.
- M. Alvarez and L. M. Lechuga, Analyst, 2010, 135, 827 RSC.
- K. S. Hwang, S.-M. Lee, S. K. Kim, J. H. Lee and T. S. Kim, Annu. Rev. Anal. Chem., 2009, 2, 77–98 CrossRef CAS.
- T. Wang, H. Yang, D. Qi, Z. Liu, P. Cai, H. Zhang and X. Chen, Small, 2018, 14, 1702933 CrossRef PubMed.
- R. McKendry, J. Zhang, Y. Arntz, T. Strunz, M. Hegner, H. P. Lang, M. K. Baller, U. Certa, E. Meyer, H. J. Güntherodt and C. Gerber, Proc. Natl. Acad. Sci. U. S. A., 2002, 99, 9783–9788 CrossRef CAS PubMed.
- H. F. Ji and B. D. Armon, Anal. Chem., 2010, 82, 1634–1642 CrossRef CAS.
- D. B. Go, M. Z. Atashbar, Z. Ramshani and H. C. Chang, Anal. Methods, 2017, 9, 4112–4134 RSC.
- G. Greco, M. Agostini and M. Cecchini, IEEE Access, 2020, 8, 112507–112514 Search PubMed.
- K. Länge, Sensors, 2019, 19, 5382 CrossRef.
- E. R. Gray, V. Turbé, V. E. Lawson, R. H. Page, Z. C. Cook, R. B. Ferns, E. Nastouli, D. Pillay, H. Yatsuda, D. Athey and R. A. McKendry, NPJ Digit. Med., 2018, 1, 35 CrossRef.
- K. S. Wong, W. T. H. Lim, C. W. Ooi, L. Y. Yeo and M. K. Tan, Lab Chip, 2020, 20, 1856–1868 RSC.
- S. Jin, X. Wei, Z. Yu, J. Ren, Z. Meng and Z. Jiang, ACS Appl. Mater. Interfaces, 2020, 12, 22318–22326 CrossRef CAS PubMed.
- H. Lim, S. M. Back, H. Choi and J. Nam, Lab Chip, 2020, 20, 120–125 RSC.
- P. D. Howes, S. Rana and M. M. Stevens, Chem. Soc. Rev., 2014, 43, 3835–3853 RSC.
- C. Liu, T. Lei, K. Ino, T. Matsue, N. Tao and C. Z. Li, Chem. Commun., 2012, 48, 10389 RSC.
- S. S. Hinman, K. S. McKeating and Q. Cheng, Anal. Chem., 2018, 90, 19–39 CrossRef CAS.
- J. M. Haber, P. R. C. Gascoyne and K. Sokolov, Lab Chip, 2017, 17, 2821–2830 RSC.
- E. Mauriz, P. Dey and L. M. Lechuga, Analyst, 2019, 144, 7105–7129 RSC.
- B. Chocarro-Ruiz, A. Fernández-Gavela, S. Herranz and L. M. Lechuga, Curr. Opin. Biotechnol., 2017, 45, 175–183 CrossRef CAS PubMed.
- T. M. A. Gronewold, Anal. Chim. Acta, 2007, 603, 119–128 CrossRef CAS PubMed.
- B. Liu, X. Liu, S. Shi, R. Huang, R. Su, W. Qi and Z. He, Acta Biomater., 2016, 40, 100–118 CrossRef CAS PubMed.
- H. J. Lee, K. Namkoong, E. C. Cho, C. Ko, J. C. Park and S. S. Lee, Biosens. Bioelectron., 2009, 24, 3120–3125 CrossRef CAS PubMed.
- A. Vallée-Bélisle and K. W. Plaxco, Curr. Opin. Struct. Biol., 2010, 20, 518–526 CrossRef PubMed.
- M. E. Iezzi, L. Policastro, S. Werbajh, O. Podhajcer and G. A. Canziani, Front. Immunol., 2018, 9 Search PubMed.
- T. De Meyer, S. Muyldermans and A. Depicker, Trends Biotechnol., 2014, 32, 263–270 CrossRef CAS PubMed.
- S. Tyagi and F. R. Kramer, Nat. Biotechnol., 1996, 14, 303–308 CrossRef CAS PubMed.
- S. A. E. Marras, S. Tyagi and F. R. Kramer, Clin. Chim. Acta, 2006, 363, 48–60 CrossRef CAS PubMed.
- T. A. Feagin, N. Maganzini and H. T. Soh, ACS Sens., 2018, 3, 1611–1615 CrossRef CAS PubMed.
- N. Arroyo-Currás, P. Dauphin-Ducharme, K. Scida and J. L. Chávez, Anal. Methods, 2020, 12, 1288–1310 RSC.
- S. K. Dembowski and M. T. Bowser, Analyst, 2018, 143, 21–32 RSC.
- F. Pfeiffer, M. Rosenthal, J. Siegl, J. Ewers and G. Mayer, Curr. Opin. Biotechnol., 2017, 48, 111–118 CrossRef CAS PubMed.
- K. J. Cash, F. Ricci and K. W. Plaxco, J. Am. Chem. Soc., 2009, 131, 6955–6957 CrossRef CAS PubMed.
- C. Parolo, A. S. Greenwood, N. E. Ogden, D. Kang, C. Hawes, G. Ortega, N. Arroyo-Currás and K. W. Plaxco, Microsyst. Nanoeng., 2020, 6, 13 CrossRef CAS PubMed.
- B. R. Baker, R. Y. Lai, M. S. Wood, E. H. Doctor, A. J. Heeger and K. W. Plaxco, J. Am. Chem. Soc., 2006, 128, 3138–3139 CrossRef CAS PubMed.
- L. R. Schoukroun-Barnes, F. C. Macazo, B. Gutierrez, J. Lottermoser, J. Liu and R. J. White, Annu. Rev. Anal. Chem., 2016, 9, 163–181 CrossRef CAS PubMed.
- N. Arroyo-Currás, P. Dauphin-Ducharme, G. Ortega, K. L. Ploense, T. E. Kippin and K. W. Plaxco, ACS Sens., 2018, 3, 360–366 CrossRef PubMed.
- M. Santos-Cancel, R. A. Lazenby and R. J. White, ACS Sens., 2018, 3, 1203–1209 CrossRef CAS PubMed.
- N. Arroyo-Currás, J. Somerson, P. A. Vieira, K. L. Ploense, T. E. Kippin and K. W. Plaxco, Proc. Natl. Acad. Sci. U. S. A., 2017, 114, 645–650 CrossRef PubMed.
- A. Idili, C. Parolo, G. Ortega and K. W. Plaxco, ACS Sens., 2019, 4, 3227–3233 CrossRef CAS PubMed.
- H. Li, J. Somerson, F. Xia and K. W. Plaxco, Anal. Chem., 2018, 90, 10641–10645 CrossRef CAS PubMed.
- C. Parolo, A. Idili, G. Ortega, A. Csordas, A. Hsu, Q. Yang, B. S. Ferguson, J. Wang, K. W. Plaxco, N. N. Arroyo-Currás, Q. Yang, B. S. Ferguson, J. Wang and K. W. Plaxco, ACS Sens., 2020, 5, 1877–1881 CrossRef CAS PubMed.
- Y. Wu, B. Midinov and R. J. White, ACS Sens., 2019, 4, 498–503 CrossRef CAS PubMed.
- A. Idili, J. Gerson, C. Parolo, T. Kippin and K. W. Plaxco, Anal. Bioanal. Chem., 2019, 411, 4629–4635 CrossRef CAS PubMed.
- A. Idili, J. Gerson, T. Kippin and K. W. Plaxco, Anal. Chem., 2021, 93, 4023–4032 CrossRef CAS PubMed.
- A. Idili, C. Parolo, R. Alvarez-Diduk and A. Merkoçi, ACS Sens., 2021, 6, 3093–3101 CrossRef CAS PubMed.
- J. Liu, S. Wagan, M. Dávila Morris, J. Taylor and R. J. White, Anal. Chem., 2014, 86, 11417–11424 CrossRef CAS PubMed.
- A. Langer, P. A. Hampel, W. Kaiser, J. Knezevic, T. Welte, V. Villa, M. Maruyama, M. Svejda, S. Jähner, F. Fischer, R. Strasser and U. Rant, Nat. Commun., 2013, 4, 1–8 Search PubMed.
- J. Das, S. Gomis, J. B. Chen, H. Yousefi, S. Ahmed, A. Mahmud, W. Zhou, E. H. Sargent and S. O. Kelley, Nat. Chem., 2021, 13, 428–434 CrossRef CAS PubMed.
- D. Kang, C. Parolo, S. Sun, N. E. Ogden, F. W. Dahlquist and K. W. Plaxco, ACS Sens., 2018, 3, 1271–1275 CrossRef CAS PubMed.
- A. Hauke, P. Simmers, Y. R. Ojha, B. D. Cameron, R. Ballweg, T. Zhang, N. Twine, M. Brothers, E. Gomez and J. Heikenfeld, Lab Chip, 2018, 18, 3750–3759 RSC.
- P. L. Mage, B. S. Ferguson, D. Maliniak, K. L. Ploense, T. E. Kippin and H. T. Soh, Nat. Biomed. Eng., 2017, 1, 1–10 CrossRef CAS.
- D. Bavli, S. Prill, E. Ezra, G. Levy, M. Cohen, M. Vinken, J. Vanfleteren, M. Jaeger and Y. Nahmias, Proc. Natl. Acad. Sci. U. S. A., 2016, 113, E2231–E2240 CrossRef CAS PubMed.
- J. Heikenfeld, A. Jajack, B. Feldman, S. W. Granger, S. Gaitonde, G. Begtrup and B. A. Katchman, Nat. Biotechnol., 2019, 37, 407–419 CrossRef CAS PubMed.
- S. Kumar and M. Khammash, Front. Bioeng. Biotechnol., 2022, 10, 1–15 Search PubMed.
- S. Pouzet, A. Banderas, M. Le Bec, T. Lautier, G. Truan and P. Hersen, Bioengineering, 2020, 7, 1–17 CrossRef PubMed.
- A. Milias-Argeitis, M. Rullan, S. K. Aoki, P. Buchmann and M. Khammash, Nat. Commun., 2016, 7, 1–11 Search PubMed.
- J. Gutiérrez Mena, S. Kumar and M. Khammash, Nat. Commun., 2022, 13, 1–16 Search PubMed.
- E. J. Olson, L. A. Hartsough, B. P. Landry, R. Shroff and J. J. Tabor, Nat. Methods, 2014, 11, 449–455 CrossRef CAS PubMed.
- J. E. Toettcher, D. Gong, W. A. Lim and O. D. Weiner, Nat. Methods, 2011, 8, 837–839 CrossRef CAS PubMed.
- D. Bavli, S. Prill, E. Ezra, G. Levy, M. Cohen, M. Vinken, J. Vanfleteren, M. Jaeger and Y. Nahmias, Proc. Natl. Acad. Sci. U. S. A., 2016, 113, E2231–E2240 CrossRef CAS.
- A. Moya, M. Ortega-Ribera, X. Guimerà, E. Sowade, M. Zea, X. Illa, E. Ramon, R. Villa, J. Gracia-Sancho and G. Gabriel, Lab Chip, 2018, 18, 2023–2035 RSC.
- S. J. Trietsch, E. Naumovska, D. Kurek, M. C. Setyawati, M. K. Vormann, K. J. Wilschut, H. L. Lanz, A. Nicolas, C. P. Ng, J. Joore, S. Kustermann, A. Roth, T. Hankemeier, A. Moisan and P. Vulto, Nat. Commun., 2017, 8, 1–7 CrossRef CAS.
- N. Oliver, M. Reddy, C. Marriott, T. Walker and L. Heinemann, NPJ Digit. Med., 2019, 2, 1–5 CrossRef PubMed.
- M. Adeel, M. M. Rahman, I. Caligiuri, V. Canzonieri, F. Rizzolio and S. Daniele, Biosens. Bioelectron., 2020, 165, 112331 CrossRef CAS PubMed.
- E. Sehit and Z. Altintas, Biosens. Bioelectron., 2020, 159, 112165 CrossRef CAS PubMed.
- Y. Liu, A. Wu, J. Hu, M. Lin, M. Wen, X. Zhang, C. Xu, X. Hu, J. Zhong, L. Jiao, Y. Xie, C. Zhang, X. Yu, Y. Liang and X. Liu, Anal. Biochem., 2015, 483, 7–11 CrossRef CAS PubMed.
- B. J. Van Enter and E. Von Hauff, Chem. Commun., 2018, 54, 5032–5045 RSC.
- J. Kim, A. S. Campbell, B. Esteban-Fernández de Ávila and J. Wang, Nat. Biotechnol., 2019, 37, 389–406 CrossRef CAS PubMed.
Footnote |
† These authors contributed equally to the preparation of the manuscript. |
|
This journal is © The Royal Society of Chemistry 2023 |
Click here to see how this site uses Cookies. View our privacy policy here.