DOI:
10.1039/D2LC01177H
(Critical Review)
Lab Chip, 2023,
23, 1279-1299
3D printed microfluidics: advances in strategies, integration, and applications
Received
23rd December 2022
, Accepted 31st January 2023
First published on 8th February 2023
Abstract
The ability to construct multiplexed micro-systems for fluid regulation could substantially impact multiple fields, including chemistry, biology, biomedicine, tissue engineering, and soft robotics, among others. 3D printing is gaining traction as a compelling approach to fabricating microfluidic devices by providing unique capabilities, such as 1) rapid design iteration and prototyping, 2) the potential for automated manufacturing and alignment, 3) the incorporation of numerous classes of materials within a single platform, and 4) the integration of 3D microstructures with prefabricated devices, sensing arrays, and nonplanar substrates. However, to widely deploy 3D printed microfluidics at research and commercial scales, critical issues related to printing factors, device integration strategies, and incorporation of multiple functionalities require further development and optimization. In this review, we summarize important figures of merit of 3D printed microfluidics and inspect recent progress in the field, including ink properties, structural resolutions, and hierarchical levels of integration with functional platforms. Particularly, we highlight advances in microfluidic devices printed with thermosetting elastomers, printing methodologies with enhanced degrees of automation and resolution, and the direct printing of microfluidics on various 3D surfaces. The substantial progress in the performance and multifunctionality of 3D printed microfluidics suggests a rapidly approaching era in which these versatile devices could be untethered from microfabrication facilities and created on demand by users in arbitrary settings with minimal prior training.
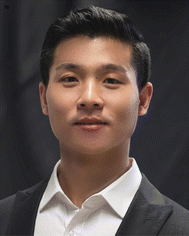 Ruitao Su | Ruitao Su is an assistant professor in the School of Mechanical and Power Engineering at Zhengzhou University. He received his B.S. degree (2013) in Mechanical Engineering from Huazhong University of Science and Technology, and his Ph.D. degree (2020) in Mechanical Engineering from the University of Minnesota. He completed his postdoctoral research in the Computational Design and Fabrication Group at MIT in 2022. His research focuses on additively and computationally deploying functional materials with applications in microfluidic and semiconducting devices. He received the Material Research Society (MRS) Graduate Student Silver Award, MRS Best Presentation Award, and the Pui Best Dissertation Award. |
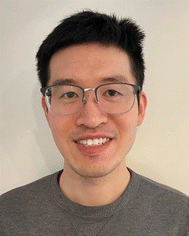 Fujun Wang | Fujun Wang is currently a bioengineer at Sartorius. His current research interests include microfluidics and automation instrumentation for bioprocessing applications. He obtained his PhD degree in Mechanical Engineering from the North Carolina State University with a research focus on fundamental droplet wetting and impacting dynamics. During his postdoctoral training at the University of Minnesota, he was involved in the research of 3D printing for developing microfluidic devices. |
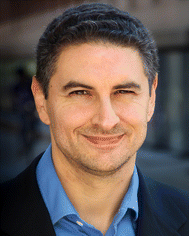 Michael C. McAlpine | Michael C. McAlpine is the Kuhrmeyer Family Chair Professor of Mechanical Engineering at the University of Minnesota. He received a B.S. (2000) in Chemistry with honors from Brown University, and a Ph.D. (2006) in Chemistry from Harvard University. His research interests are focused on 3D printing functional materials & devices for active electronic applications, with recent breakthroughs in 3D printed OLED displays and 3D printed bionic eyes (one of National Geographic's “12 innovations that will revolutionize the future of medicine”). He has received several awards for this work, including the Presidential Early Career Award for Scientists and Engineers (PECASE), and the National Institutes of Health Director's New Innovator Award. |
1. Introduction
Microfluidic devices enable the characterization and manipulation of fluidic flows in the sub-millimetre regime.1 Profound applications which have impacted multiple engineering and scientific research fields have been derived from these versatile tools.2 The functionalities and performances of microfluidic devices are highly dependent on the methods of fabrication, the skill of the engineer, and the materials used to construct the constituent components. Traditionally, microfluidic devices are fabricated by soft lithography, a process where features are moulded in elastomers such as polydimethylsiloxane (PDMS), leveraging the well-established microfabrication technologies that offer scale and are performed in cleanroom facilities.3 However, as the breadth of applications increases, the intrinsic limitations of two-dimensional (2D) structural patterning and assembly presented by soft lithography has hindered the realization of higher dimensional functionalities, such as three-dimensional (3D) microfluidic networks and devices conformal to nonplanar surfaces. Over the past decade, additive manufacturing, or 3D printing, has been actively explored to transcend the planarity of soft lithography and liberate the manufacturing to resource limited settings.4 Several 3D printing methods have demonstrated unique capabilities for the fabrication of microfluidic devices, each with comparative advantages in device resolution, configuration, or integration.5 In this review, we examine the recent progress in 3D printing strategies enabled by the adoption of new materials or design schemes, which have advanced the structural and functional integration of 3D printed microfluidics and relevant applications.
1.1. Characteristics of microfluidic flows
Several aspects of the behaviour of microscale flows are distinct from macroscale flows, such as the slip boundary conditions induced by molecular effects and substantially amplified continuum parameters.6 Closely relevant to most engineering applications are the laminar flow conditions and large surface-to-volume ratios, a result of the dimensional constraints imposed by the microscale fluid conduits. The Reynolds number (Re) is a widely used dimensionless number that weighs the effect of the inertial force against the viscous force in the fluid flow. With small channel sizes and moderate flow rates, the dominant viscous force typically results in a small Reynolds number (Re ≪ 1) and renders the microfluidic flow highly laminar (Fig. 1a-i). Hence, absent of any incorporated mixing mechanisms, molecular diffusion is the major driving force to mix different chemical species. The mixing is typically limited by the low diffusion coefficient at room temperature and the short time-scale of dwelling in the microfluidic devices.7 The highly laminar flow creates opportunities for unique applications such as the controlled generation of concentration gradients. For instance, laminar flows in the 3D printed branching microfluidic networks allow the concentration gradient to be controlled as the flows split and merge (Fig. 1a-ii).8 Streams carrying different chemical species (denoted by coloured dyes) were guided by the branching network, creating a linear superposition of the concentration gradients across the outlet region. The large surface-to-volume ratio at the microscale enables high rates of heat and mass transfer, resulting in high controllability over the microenvironment in the microfluidic devices, which is particularly useful for biochemical applications. For instance, in 3D printed perfusing hydrogel networks, thermofluidic heating could be introduced to cell-laden structures to facilitate heat-activated gene expression with a precise spatial patterning (Fig. 1b).9
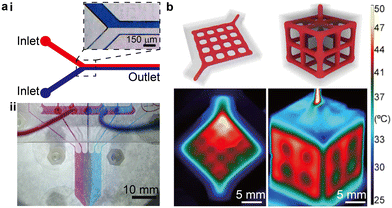 |
| Fig. 1 Examples demonstrating the highly laminar flow and large surface-to-volume ratio of microfluidics. (a) Laminar flow in microfluidics. i. Schematic and microscopic image showing that minimal mixing occurs in a plain microfluidic channel flow. ii. Image of a 3D printed gradient generator that exploits the microfluidic laminar flow. (b) High surface-to-volume ratio of microfluidics. Schematic of perfusing networks for thermofluidic activation and infrared images of the corresponding 3D printed structures perfused with a heated fluid. (a-ii) Was adapted with permission from ref. 8. (b) Was adapted with permission from ref. 9. | |
Recent studies have described the effects of different printing methods on the laminar flows and mixing in the 3D printed microfluidic devices. The surface roughness is the major factor that induces the variations,10 with extrusion printed surfaces being the coarsest (Ra ∼11 μm) and stereolithography (SL) printed surfaces being the smoothest (Ra ∼0.35 μm).11 With the same device design, there is evidence suggesting that within the laminar flow regime, chaotic advections are more prone to occur for extrusion and material jetting printed devices.12 Consequently, the mixing tends to be complete in the shortest distance in these devices.
1.2. Advances in 3D printing microfluidics
Driven by the need for multiplexed and high-throughput microfluidic biological and chemical experiments, the development of microfluidic devices calls for higher degrees of standardization and automation in device fabrication and alignment processes to enable seamless integration with multi-channel sensors and nonconventional substrates, such as soft, curved, or even dynamic surfaces.2,13–15 3D printing methods that have been routinely and successfully applied to the fabrication of microfluidic devices include extrusion-based printing, material jetting, and SL. The recent inclusion of direct laser writing (DLW) opens up a promising route for printing high-resolution (ca. 100 nm) microfluidic structures.16,17 Because the mechanism of each printing modality has been extensively discussed in previous reviews,5,18 we will focus on the recent progress of printing strategies and the potential impacts of each method in the microfluidic space.
Extrusion-based printing.
By creating a pressure gradient in the printing nozzle, extrusion-based printing forces inks in liquid or molten states to continuously flow out of the nozzles to deposit materials into layered structures according to pre-programmed toolpaths, enabling the direct assembly of spatial architectures and multifunctional materials in a straightforward manner. One approach, termed direct ink writing (DIW), extrudes inks at room temperature to build complex 3D structures.19 One early example demonstrated artificial chaotic advection via 3D printing of complex vascular geometries to promote the mixing of chemical species.20 A paraffin-based ink was extruded to build a 3D scaffold which was then removed to form interconnected microfluidic networks in the casted epoxy and photoresin matrix. Similar approaches were also applied to the printing of vascular tissue models that were perfused with solutions to sustain the biological viability for several weeks.21 Even though a high mixing efficiency and channel resolution (below 300 μm) could be achieved with this method, its reliance on fugitive materials limits its potential for wider applications. A more widespread approach involves extruding thermoplastics in a layer-by-layer manner, termed fused deposition modelling (FDM), to form microfluidic devices for applications that do not require high resolutions.22 Despite limitations in geometric accuracy, surface roughness, and mechanical flexibility, microfluidic devices fabricated via FDM have proven to be highly versatile in numerous chemical and biomedical applications.23–25 Trademarked by Stratasys Inc., FDM features heated building chambers to reduce the residual stress in the 3D printed parts, whereas a similar technology without the environmental temperature control, termed fused filament fabrication (FFF), is adopted by most home-friendly 3D printers targeting applications that are less demanding in terms of modelling accuracy.
Recently, advances in 3D printed silicone-based microfluidics have progressed considerably by expanding methods to print silicone thermosetting inks and exploiting their structural mechanics. Inks that are curable under ambient conditions, such as acetoxy silicone that vulcanizes via hydrolyzation-assisted condensation,26 represent ideal materials to construct microfluidic devices in open air environments. In one method, silicone filaments were printed on flat substrates to form the sidewalls of micro-channels and chambers, which were subsequently covered by poly(methyl methacrylate) (PMMA) plates to form enclosed microfluidic devices (Fig. 2a-i).8 Microfluidic channels that were printed by this method achieved resolutions of ca. 30 μm. 3D microfluidic ingredient generators were created by aligning and stacking multiple layers vertically, although this method was designed for planar and rigid microfluidic devices. Another method investigated the mechanism of printing enclosed silicone channels by exploiting the viscoelasticity of the ink (Fig. 2a-ii).13 An equilibrium angle range of the 3D printed inclined walls was determined by the yield-stress behaviour of the ink, which was reinforced by the real-time crosslinking of the ink in air.27 Not bounded by planar top covers, this method facilitated the direct writing of self-supporting microfluidic structures on 3D surfaces.
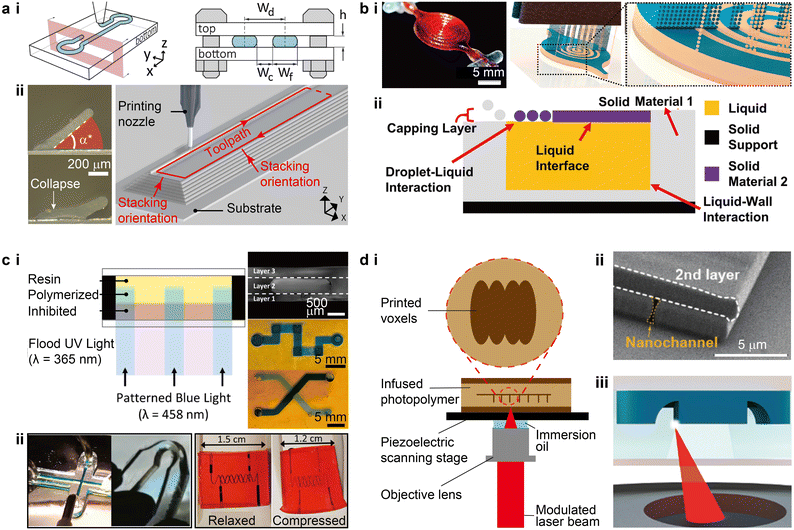 |
| Fig. 2 Advances in the strategies for 3D printing microfluidic devices. (a) Extrusion-based 3D printing. i. Schematic of extruding room temperature-curable silicone inks on flat substrates that are subsequently enclosed by a top cover. Wd, Wc, Wf, and h denote the design width, channel width, filament width, and channel height, respectively. ii. Images of printed silicone thin walls and a schematic of printing microfluidic channels. α* denotes the critical angle above which the printed walls could maintain their equilibrium states. (b) PolyJet printing. i. Conceptual schematic illustrating the PolyJet printing of a fluidic capacitor consisting of building material (blue) and temporary supporting material (beige). The image shows a completed capacitor. ii. Schematic of the solid–liquid co-printing process where liquid-wall and droplet-liquid interactions were involved. The printing heads and UV light source are not shown. (c) Stereolithography. i. Left: Schematic of a variation of dual wavelength SL. Right: Optical images of SL printed multi-layer microstructures, serpentine channels, and crossing channels. ii. Left: SL printed PDMS-based microfluidic channels with a width of 500 μm and a bent dog-bone specimen. Right: SL printed 2-phenoxyethyl acrylate-based soft microfluidic device containing helical channels with a width of 200 μm. (d) Direct laser writing. i. Typical configuration of a DLW printing stage. The magnified view illustrates a sequence of cured voxels. ii. SEM micrograph of a nanoscale channel printed by DLW. iii. Schematic of in situ direct laser writing, where the focused laser prints encased structures within a microfluidic channel. (a-i) Was adapted with permission from ref. 8. (a-ii) Was adapted with permission from ref. 13. (b-i) Was adapted with permission from ref. 29. (b-ii) Was adapted with permission from ref. 30. (c-i) Was adapted with permission from ref. 44. (c-ii) Was adapted with permission from ref. 53 (left) and ref. 54 (right). (d-ii) Was adapted with permission from ref. 60. (d-iii) Was adapted with permission from ref. 16. | |
Material jetting.
Rather than creating continuous ink flows, material jetting deposits tiny material “bits” via a process resembling inkjet printing. This method has been commercialized along with a wide range of proprietary photopolymer-based inks, which are typically crosslinked via controlled optical irradiations.28 Depending on the number of printing heads that can be simultaneously operated, Polyjet printing and Multijet modelling are the most common methods of material jetting. The unique aspect of PolyJet printing lies in the compactly assembled printing nozzles that are synchronized to deliver a heterogeneous material system including supporting and multiple building inks (Fig. 2b-i).29 For the fabrication of microfluidic devices, one major drawback of material jetting is the need to remove supporting materials after the printing is completed in order to form hollow microstructures. The postprocessing step requiring removal of the solid support materials via dissolving or melting is labour-intensive, especially as the channel size decreases or when serpentine shapes and sharp turns are involved.30 This can affect resolution, throughput and automation. Thus, PolyJet printing has conventionally been used to print microfluidic channels with resolutions above 500 μm, providing a versatile approach to fabricating various microscale or mesoscale structures including fluidic logic elements.31 Recently, non-photocurable low viscosity liquid inks were printed as temporary supporting materials that simplified the postprocessing of microfluidic devices.30,32,33 For instance, Castiaux et al. demonstrated intricate planar microfluidic networks using glycerol/isopropanol mixtures as the supporting materials via a print-pause-print workflow, and a cross-sectional dimension as small as 125 μm × 54 μm was achieved with this method.32 Further, using one Stratasys cleaning solution as the supporting fluid, Hayes et al. systematically characterized the solid–liquid co-printing technique by examining the physics of drop-liquid interactions and the printing processes (Fig. 2b-ii).30 The adoption of multi-nozzle printing heads enabled automatic printing protocols that produced 3D microfluidic networks and valves, which were available to use within two hours after postprocessing.
Stereolithography.
SL prints objects by solidifying photopolymers that are contained in a vat, where the layer-by-layer photopolymerization occurs. The optical energy, delivered in the form of focused laser beams or digital images, is inputted to the vat by scanning the laser following pre-designed toolpaths or projecting the sliced patterns to the liquid–solid interface to execute the continuous solidifying process. Depending on the configuration of the SL printing system, light can be introduced from either the top or bottom of the vat.5,34 Depending on the dimensionality of concurrently polymerized entities or the development of the liquid–solid interface, printing strategies can be classified into four categories,35 including laser-scanning SL,36 projection SL,37,38 continuous SL,39,40 and volumetric SL.41–43 Among these technologies, volumetric SL, including tomographic SL41 and dual wavelength SL,43 is an emerging method that has the potential to produce geometrically complex objects with high throughput. Recently, one variation of the dual wavelength SL proposed by Smith et al. employed optical dose control methods to build objects layer-by-layer and reduce the viscosities of the printable inks, successfully demonstrating the fabrication of functional microfluidic devices (Fig. 2c-i).44 The dual wavelength chemistry adopts UV light (λ = 365 nm) as the inhibiting factor and blue light (λ = 458 nm) as the polymerizing factor.45 By coordinating the light intensities and exposure times of the two factors, the top and bottom boundary positions of the polymerization layer could be controlled to print the target devices that were simply supported by buoyancy, achieving multi-layered microfluidic devices with a channel size of 400 μm.
Ultraviolet (UV) light was initially utilized to cure the photopolymers during SL printing.46 Recent advances in polymer photochemistry have extended the available light source to the visible range to accommodate the safety concerns of using UV light in offices or homes.47,48 For instance, by identifying nontoxic photoabsorbers such as tartrazine, Grigoryan et al. discovered that aqueous pre-hydrogel solutions can be effectively crosslinked by a light source with a peak wavelength of 405 nm.49 The vascular structures printed via projection SL possessed good mechanical and optical properties, allowing for the creation of hydrogel-based sophisticated microfluidic mixers, bicuspid valves, and vascular networks that mimic the functions of organs. Photoresists such as acrylate- and epoxy-based polymers are conventionally used in SL, resulting in limited mechanical compliance and biocompatibility.50 Several ink recipes that promote the printing of elastomeric structures and devices have recently been reported, featuring either high mechanical flexibility or tuneable optical properties.51,52 Bhattacharjee et al. reported a new ink formulation consisting of PDMS-methacrylate macromers and a photoinitiator derived from phosphine oxide, which could be effectively cured by UV light at a wavelength of 385 nm.53 The printed elastomeric structures, including microfluidic devices, exhibited mechanical, optical, and chemical properties comparable to Sylgard-184 PDMS (Fig. 2c-ii). To overcome the issue of low structural resolution in SL printing of elastomeric microfluidic devices, Weigel et al. further developed a library of acrylic- and allyl-based inks that enabled the printing of devices with an elongation above 1000% and the smallest channel resolution below 100 μm (Fig. 2c-ii).54 The adoption of 2-phenoxyethyl acrylate (POEA) as the reactive monomer allowed for a high controllability over the cured layer thickness via the quantity of light exposure. The highly flexible and precise droplet generators printed with the optimized ink formulation produced emulsion droplets as small as 58 μm. The emergence and development of elastomeric inks for SL printing will likely catalyze the growth of this technique in fabricating highly flexible and biocompatible microfluidic devices. Yet, as with PolyJet printing, it remains a challenge to clear the 3D printed microchannels filled with uncured residual inks.
Direct laser writing.
DLW by two-photon or multiphoton polymerization is a type of volumetric stereolithography, which features a nonlinear correlation between the absorption rate and the light intensity.55 First utilized by Maruo et al. to print microstructures56 and later for the printing of nanoscale photonic crystal elements,57,58 DLW utilizes femtosecond lasers for rapid and precise crosslinking of photoresists at the focal point. Volumes surrounding the focal point that are crosslinked during successive printing steps create individual volumetric elements, i.e., the voxels.58,59 Continuous structures are printed by stitching the voxels in a predefined density and orientation (Fig. 2d-i). The sufficiently small diameter of the tightly focused laser beam coupled with a precisely controlled power payload can achieve submicron voxels and a feature resolution of ca. 100 nm (Fig. 2d-ii).60 Unexposed resins are removed in a developer bath after the printing is completed to create microfluidic structures.
Because of the low throughput when printing large components, such as the body structures and connecting ports, DLW is not typically used to print the entire microfluidic devices, but using multiple printing methods presents challenges in smoothly interfacing between the length scales. In one protocol termed in situ DLW (isDLW), PDMS moulding was first used to make the bulk components, and DLW was then dedicated to the printing of high-resolution structures within the microfluidic channels that were infused with inks (Fig. 2d-iii).16 PDMS-on-glass chips are typically used as the body structures because of the well-established methodology of PDMS moulding.61 However, the high gas permeability of PDMS induces a thin layer of O2 on the channel walls that inhibits the subsequent photopolymerization of photoresists during DLW printing.62 Alternatively, Alsharhan et al. used cyclic olefin polymer (COP) to form the body structures by hot embossing to effectively reduce O2 diffusion.16,63
Conventionally, DLW utilizes negative photoresists to print fine structures. Recently, positive photoresists such as AZ 4562 (MicroChemicals), were used to print monolithic microfluidic structures that could be integrated with porous membranes and potentially other functional components such as pumps and filters.73 Beyond rigid structures printed with photoresists, two-photon curable silicone69 and hydrogels such as polyethylene glycol diacrylate (PEGDA),68,70,74 represent ideal candidates for printing biocompatible and mechanically compliant microfluidic structures. Indeed, recent progress has demonstrated the printing of unique stimuli-responsive hydrogel microstructures with low-power lasers (as low as 0.1 Joule per laser pulse) in the visible spectrum.68
1.3. State-of-the-art characteristics of 3D printed microfluidic devices
Comparisons among various features of microfluidic devices which were 3D printed with different methods have been summarized previously.4,5 Recent progress in 3D printing technologies has altered the characteristic landscape of microfluidics (Table 1).
Table 1 Characteristics of 3D printed microfluidic devices
|
Extrusion |
Material jetting |
SL |
DLW |
Resolution |
>50 μm (ref. 13 and 64) |
∼50 μm (ref. 32) |
∼10 μm (ref. 65 and 66) |
∼100 nm with photoresist,67 ∼1 μm with hydrogel68 |
Elasticity (breaking strain) |
>400% when printed with silicone16 |
>200% when printed with Agilus30 (Stratasys) |
>100% when printed with PDMS-based inks53 |
Could potentially match PDMS69 |
Biocompatibility |
Biocompatible inks such as certain thermoplastics and silicone |
Biocompatible inks such as MED610 (Stratasys) |
Biocompatible inks such as PEGDA hydrogel and PDMS |
Biocompatible inks such as PEGDA hydrogel70 |
Multi-material printing |
Highly feasible13,71 |
Routinely executed |
Possible with volumetric additive manufacturing41 |
Possible when integrated with pre-fabricated bulk structures16,63 |
Valve size (overlapping area of flow and control channels) |
∼500 μm × 500 μm (ref. 13) |
Millimeter scale72 |
∼15 μm × 15 μm (ref. 65) |
∼10 μm × 10 μm (ref. 16) |
3D integration capability |
3D vascular networks20 or conformal microfluidics13 |
Arbitrary 3D architectures with supporting materials |
Arbitrary 3D architectures with voids filled with residual resins |
Arbitrary 3D architectures typically below 10 μm (ref. 67 and 68) |
One major advancement is the adoption of elastomeric materials, such as PDMS- and silicone-based inks, by SL and extrusion-based printing, which enabled enhanced mechanical compliance of microfluidic devices.13,53 Progress in the development of commercially available elastomeric inks, such as Agilus30 by Stratasys, also facilitated the printing of multi-material flexible devices such as soft robots.75 Improvements in the resolution of 3D printed structures, most notably in SL printed devices, has been enabled by innovations in control algorithms and optical modulations. For instance, Sanchez Noriega et al. introduced a generalized scheme of SL printing by disrupting the classic trade-off between the exposure time and the layer thickness throughout the printing process.65 The randomized and overlapping exposure algorithms for each layer expanded the parameter space for the x/y/z polymerization dose, resulting in valves as small as a few pixels with a cross-section of 15 μm × 15 μm, a resolution that thus far has only been demonstrated by soft lithography. By printing the channel and roof separately, which were then combined in a subsequent exposure, Xu et al. demonstrated a SL printing process that eliminated the over-exposure of residual resins in the channel.66 The new method, termed “in situ transfer vat photopolymerization”, disrupted the long-standing limitation to the z-resolution to realize a channel height below 10 μm. Important figures of merit such as autonomous microfluidic components and integration capabilities will be discussed in the following sections.
2. Advances in device integration and functionality
Numerous engineering applications require the integration of microfluidics with various non-fluidic sensing elements, autonomous components, and functional substrates that manifest in nonplanar or even dynamically modulating morphologies. 3D printing opens opportunities for innovation in these unconventional scenarios with its versatile adaptability to various materials and control algorithms. Highlighting the recent progress in device and functionality integration, this section reviews the advances in four important directions that benefit from the freeform fabrication capabilities of 3D printing and promises enhanced performance for next-generation microfluidic applications.
2.1. Integrating 3D printed microfluidics with micro-sensing arrays
One of the goals of the “lab-on-a-chip” concept is to seamlessly integrate microfluidic structures with electrodes that are functionalized with various sensing agents, which promises high throughput and multiplexing capabilities.76,77 3D printed microfluidic devices are uniquely compatible with prefabricated sensing arrays because of the potential for automated high precision alignment and structural conformality.78 Early research printed the microfluidic components as modularized parts which were subsequently assembled with separately prepared electrodes, making most components recyclable for rapid chemical and biological detection.79–81 This approach has proven to be highly versatile for integrating microfluidics to microfabricated sensing elements comprising nanostructures. For instance, Arshavsky-Graham et al. integrated 3D printed microfluidic devices with substrates that were patterned with porous silicon oxide (PSiO2), which were bonded via a UV-curable adhesive (Fig. 3a-i and ii).82 In this example, compared to the PDMS-based control group in which a high-temperature curing was applied to the integration process, the 3D printed counterpart avoided the elevated thermal conditions, which potentially contributed to an improved detection limit and sensitivity (Fig. 3a-iii). Directly printing the “lab portion” on electronics such as printed circuit boards has been of significant interest to the biochemical and 3D printing communities.8,83,84 The silicone-based self-supporting microfluidics introduced in section 1.2 provided a novel approach to directly align and print microfluidic channels and chambers on prefabricated sensing circuits (Fig. 3b-i).13 Instead of using a dedicated bonding step, the multi-channel salinity sensors were aligned in the 3D printing system via alignment marks. Then, printing toolpaths were specifically designed to accommodate the sensor layout and encapsulate the entire device during a single printing process. A strong bonding between the microfluidics and underlying substrate formed as the acetoxy silicone cured in air within several hours. The demonstrated salinity sensor sensitively differentiated NaCl solutions of varying concentrations (Fig. 3b-ii). Because no supporting materials or residual inks were involved in this method, this new ability to directly print hollow microchannels and chambers promises a higher degree of automation for integrating microfluidics with sensing arrays.
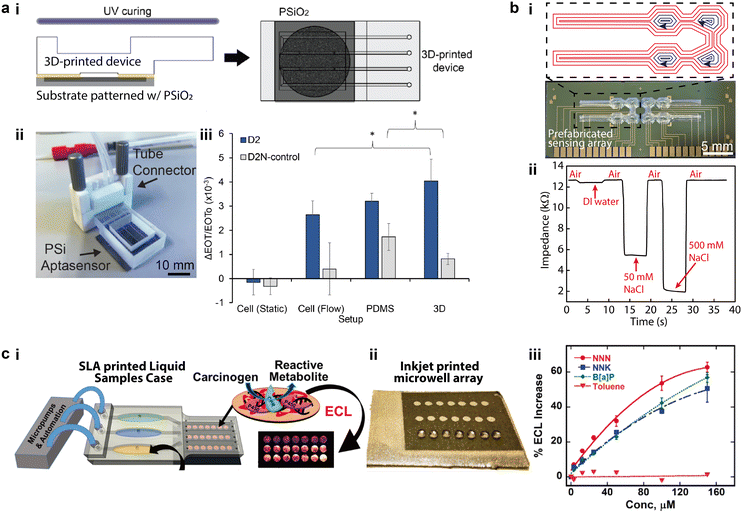 |
| Fig. 3 Integration of 3D printed microfluidics with micro-sensing arrays. (a) 3D printed microfluidic parts integrated with separately prepared sensing elements. i. Schematic of bonding 3D printed microfluidic devices to a porous silicon oxide (PSiO2) substrate with UV-curable adhesives. ii. Image of the assembled aptasensor connected to external tubes. iii. Relative changes of effective optical thickness (EOT) measured with two groups of 1 mM protein solutions on three different sensing platforms. (b) Microfluidic salinity sensor made by directly aligning and printing microfluidics on sensing arrays. i. Silicone-based microfluidics that were directly printed on prefabricated electronic sensors and the designed printing toolpaths. Red and blue toolpaths denote the printing of the lower channels and the top chamber covers, respectively. ii. Real-time impedance variation measured with the 3D printed salinity sensor for NaCl solutions of varying concentrations. (c) Microfluidic sensor made by assembling 3D printed microfluidic parts and sensing elements. i. Schematic of integrating SL printed microfluidic cases with inkjet printed sensing arrays to form an electrochemiluminescent (ECL) sensor for genotoxicity. ii. Image of sensing arrays patterned with inkjet printed microwell arrays. iii. Calibration curves of ECL magnitude for different concentration of standards versus toluene. (a) Was adapted with permission from ref. 82. (b) Was adapted with permission from ref. 13. (c) Was adapted with permission from ref. 88. | |
Recent integration schemes have adopted multimodal printing methods for preparing both microfluidic devices and micro sensing arrays in an additive manner.85–87 For instance, Kadimisetty et al. demonstrated an approach for 3D printing genotoxic detection arrays that employed SL to fabricate the microfluidic parts and inkjet printing to pattern the conductive pyrolytic graphite sensing arrays (Fig. 3c-i and ii).88 The two sets of components were then assembled with microprocessors and micropumps to enable automated sampling of genotoxic reactions by characterizing the electrochemiluminescence (ECL) intensity in a multiplexed manner. Covering a dynamic range from 3 μM to 150 μM, the spot-to-spot variability of ECL was below 10% for all standard concentrations (Fig. 3c-iii).
2.2. Modularized integration of 3D printed microfluidic devices
To avoid channel clogging and improve the device fabrication throughput, the community of 3D printed microfluidics has been actively studying an additive alternative that makes devices from separately printed components, which simplifies the postprocessing and circumvents the structural degradation during complex material removal. This modularized approach employs a “LEGO®-like” method for device construction and is capable of multiple printing modalities, realizing a wide range of functionalities such as autonomous components,89,90 logic circuitry components,91 spatial microfluidic networks,92,93 organs-on-a-chip,94,95 and sensors,96etc.
PolyJet printing supports the simultaneous printing of elastomeric and rigid structures to generate desired functions, but the nature of this printing modality often demands supporting materials. For instance, Hubbard et al. recently demonstrated a modularized design strategy to integrate complex fluidic circuitry consisting of multiple logic elements in fully 3D printed soft robots.75 Even though the entire device could be finished in one run, the need to remove the sacrificial supporting materials from the intricate microscale channels presented a challenge. Building upon the modularized printing concept, Childs et al. divided the microfluidic devices into subcomponents which were printed separately and had the channels open as printed.91 The subcomponents were cleared from the supporting materials and then assembled to form a functional microfluidic capacitor, reducing the postprocessing time by ca. 98% (Fig. 4a-i and ii). Bonded by pre-designed fasteners, the assembled capacitor displayed a burst pressure above 150 kPa, meeting the demand of most microfluidic applications.
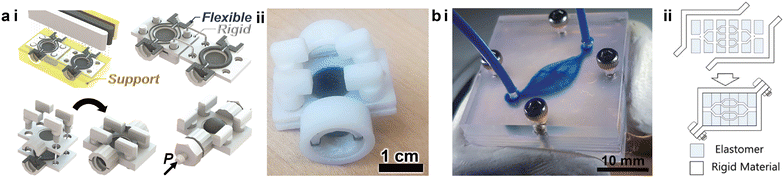 |
| Fig. 4 Modularized additive assembly of 3D printed microfluidic devices. (a) PolyJet printed microfluidic capacitor. i. Schematic of the assembly of a PolyJet printed microfluidic capacitor consisting of rigid (white), flexible (black), and supporting (yellow) materials. ii. Image of the assembled microfluidic capacitor. (b) SL printed complex vascular networks. i. Image of a 3D vascular network assembled from multiple 3D printed layers. ii. Schematic demonstrating the concept of the deconstruction and assembly of SL printed microfluidic networks. (a) Was adapted with permission from ref. 91. (b) Was adapted with permission from ref. 92. | |
SL printed microfluidic devices face the same dilemma of how to effectively remove the residual resins in complicated geometries. In particular, the trapped resins can adversely impact the channel resolution and accuracy due to inadvertent polymerization within the designed hollowed space. Instead of printing a monolithic entity as in conventional SL printing, Ching et al. proposed and verified a methodology of deconstructing 2D and 3D microfluidic networks into sub-units that did not involve enclosed channels, facilitating the fabrication of complex vascular networks (Fig. 4b-i).92 The functional sections were cut into layers or blocks that were printed with elastomers and bounded by rigid enclosures (Fig. 4b-ii). This approach proved to be compatible with hydrogels and photoresins, and realized a channel size below 100 μm, for promising applications in tissue engineering.
2.3. 3D printed autonomous microfluidic components
Autonomous microfluidic components are critical to overcoming several limitations of 3D printed microfluidic platforms, including the bulky and costly peripheral setups for controlling the fluid, challenges in scaling up the integration of the microfluidic systems, and difficulties in realizing multiplexed sensing applications. As the most basic autonomous element, microfluidic valves can be combined to form complex components such as pumps, mixers, and diluters.97–99 First reported by Unger et al. in 2000,100 the well-known Quake valve was made using soft lithography by stacking layers of patterned PDMS membranes. Building on this principle, the 3D printing community has gradually developed squeeze valves and membrane valves.101–103 Notable progress in different printing methods, device miniaturization, and integrated autonomous components has been achieved in recent years.
Among different printing methods, projection SL has been most actively explored for the fabrication of autonomous components.103,104 Considerable advances have been achieved in terms of structural design and device miniaturization. One major type of SL printed microfluidic valve, termed membrane valves, has dedicated air chambers larger than the flow channels to enhance the flexibility of the polymerized membrane in between. The air chambers have one additional outlet to rinse the residual resins before the chambers are sealed. Gong et al. introduced a method to enhance the durability of 3D printed membrane valves by adding thermal initiators in the resin and thermally baking the devices after printing, resulting in devices that could withstand more than 10
000 actuations (Fig. 5a-i and ii).101 By adding a displacement chamber, multiple valves were further integrated to form 3-to-2 multiplexers or mixers. With an open-at-rest design resembling the PDMS-based Quake valve, a variation of the membrane valve consists of one flow channel and one control channel, which is actuated by high pressure air to turn off the valve (Fig. 5a-iii and iv).102 Lee et al. demonstrated the Quake-style membrane valves that were printed with PEGDA and realized the integration of 64 valves in an area of ca. 1 cm2, promoting the autonomous operation of transparent and biocompatible microfluidic devices.102 Using the optically tailored 3D printing method, Sanchez Noriega et al. recently reduced the active area of the valves to as small as 46 μm × 46 μm, which were integrated into microfluidic pumps by layering three valves in parallel and operated following a set of on and off commands (Fig. 5a-v).65 They also demonstrated a new type of microfluidic valve, termed squeeze valves, along with corresponding pumps, by sandwiching the flow channel between two splitting control channels. The smallest squeeze valves possessed an active area of 16 μm × 16 μm and a dead volume of only a few picoliter.
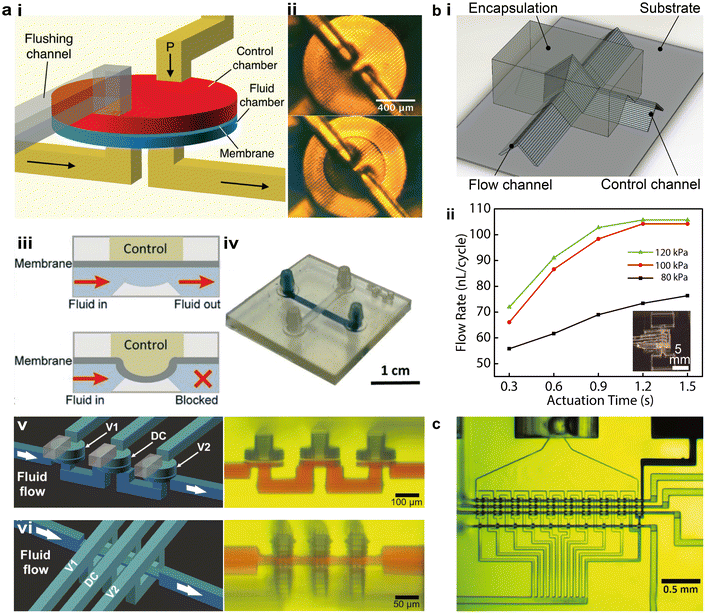 |
| Fig. 5 3D printed autonomous microfluidic components. (a) SL printed microfluidic valves and pumps. i. Schematic of the cross-sectional structure of a membrane valve in turn-on and turn-off states. ii. Image of an SL printed membrane valve. iii. Schematic showing the working principle of a membrane valve. iv. Images of an SL printed membrane valve in turn-on (top) and turn-off (bottom) states. v. Schematic and corresponding image of microfluidic pumps consisting of squeeze valves. vi. Schematic and corresponding image of microfluidic pumps consisting of membrane valves. (b) Microfluidic valves and pumps made by extrusion-based 3D printing. i. Schematic of a microfluidic valve built by extrusion-based 3D printing. ii. Flow rate tests of extrusion printed microfluidic pumps under varying actuation pressures and times. (c) Image of an integrated 10-stage 2-fold serial diluter consisting of SL printed squeeze valves within an overall footprint of 2.2 mm × 1.1 mm. (a-i and ii) Was adapted with permission from ref. 101. (a-iii and iv) Was adapted with permission from ref. 102. (a-v and vi) Was adapted with permission from ref. 65. (b) Was adapted with permission from ref. 13. (c) Was adapted with permission from ref. 65. | |
Extrusion-based printing has not been extensively studied to building microfluidic autonomous components. Leveraging the concept of elastomeric self-supporting structures, Su et al. established a methodology to construct microfluidic valves and pumps by stacking room-temperature vulcanizing silicone filaments under ambient conditions, without the need for sacrificial or supporting materials (Fig. 5b-i).13 By analogy to SL printed squeeze valves, the open-at-rest structure was realized by directly spanning the control channel across the flow channel, and the junction was encapsulated by UV-curable resins to form the valve.
Microfluidic pumps were fabricated by encapsulating three valves that were printed in parallel, demonstrating a pumping rate of over 100 nL per cycle when the valve was fully actuated (Fig. 5b-ii). PolyJet printing was investigated by Sochol et al. to demonstrate an array of microfluidic logic components such as fluidic capacitors, diodes, and transistors,29 which were recently integrated in 3D printed soft robots to realize fully automatic control and operation.75 Based on the method of a temporary liquid support introduced in section 1.2, PolyJet printed microfluidic valves and pumps were recently developed by Castiaux et al. to realize a channel width below 100 μm.72
One figure of merit closely related to 3D printed autonomous components is the integration density, i.e., the number of autonomous elements that could be integrated per unit area, which is largely determined by the miniaturization of the constituent elements. Soft lithography has held the record of high-density integration, approaching 1 million valves per cm2, leveraging the mature semiconductor fabrication techniques.105,106 With the spatially and optically tailored SL printing approach, Sanchez Noriega et al. realized a 10-stage 2-fold serial diluter by integrating miniaturized squeeze valves, which contained ca. 70 squeeze valves within an area of 2.2 mm × 1.1 mm (Fig. 5c).65 However, the trade-off between the structural resolution and X–Y footprint of the finished device limits the integration scale. Techniques such as micromirror arrays or image stitching can be applied to increase the overall size of the 3D printed devices.107 Theoretically, this promising approach can be applied to any existing SL printers with the appropriate projecting resolution.
2.4. 3D printed conformal microfluidic devices
The ability to directly print microfluidics and electronics on static or dynamic targets is an emerging area at the intersection of 3D printing, robotic control, and functional devices. This approach is key to novel applications, such as conformal biomedical sensing and in situ biofabrication.108,109 The challenges are to accurately collect the real-time topographical information, develop various biocompatible inks, and discover novel structural printing schemes.110 Combining functional materials, mathematical modeling, and artificial intelligence (AI), the adoption of additive manufacturing for conformal device printing represents an important research direction in the field of printing functional devices.
To fabricate microfluidic devices that conform to organs, Singh et al. employed 3D scanning with structured light to digitize the organ topography and design the printing toolpaths. The 3D printed microfluidic channel was applied to the renal cortex of a kidney with minimal geometric mismatch, providing a platform for organ health monitoring with potential advantages over organ-on-a-chip technologies (Fig. 6a).14 By pumping fluid through the microfluidic channel, biomarkers were injected into the channel and collected to acquire the molecular trajectories of the whole organ, demonstrating a minimally invasive “microfluidic biopsy” technique.
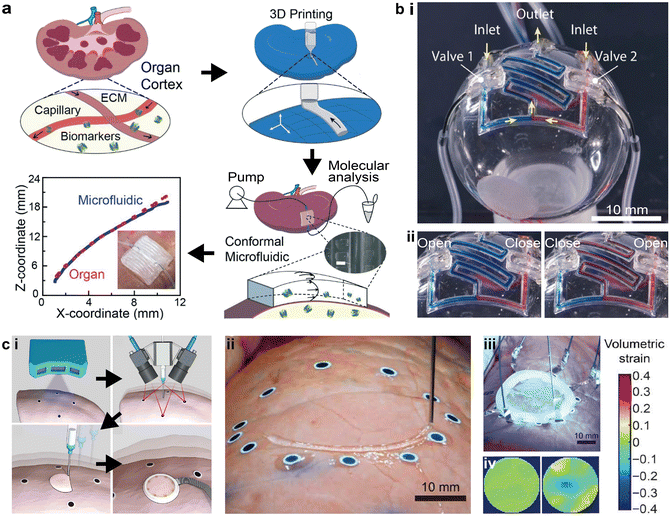 |
| Fig. 6 3D printed conformal microfluidic devices and adaptive printing strategy. (a) Schematic of printing conformal “microfluidic biopsy” devices on whole organs and the topographical fidelity of the printed device to the organ surface. Scale bar = 500 μm. (b) 3D printed conformal microfluidics on spherical surfaces. i. Image of serpentine microfluidic networks printed on spherical surfaces with integrated valves. ii. Images of the conformal microfluidic network in operation with different combinations of valve states. (c) Soft sensors that were conformally and adaptively 3D printed on deforming surfaces. i. Schematic of the major steps involved in the adaptive printing of sensors on deforming surfaces, including scanning the surface morphology, tracking the deforming surface, printing the device, and wiring to external circuitry. ii. Image of adaptively printing sensors on a deforming lung with attached fiducial markers. iii. Image of the finished deformable sensor being cured by UV light. iv. Acquired volumetric strain maps of the lung in resting and contractile states with the deformable sensor. (a) Was adapted with permission from ref. 14. (b) Was adapted with permission from ref. 13. (c) Was adapted with permission from ref. 15. | |
To precisely integrate autonomous microfluidic components such as valves in the conformal microfluidics, Su et al. demonstrated that the self-supporting elastomeric microfluidic structures could be printed on a 3D surface using known mathematical models.13 By designing a 2D projection approach, the filament stacking scheme was inspected filament-by-filament, and then converted into 3D printing toolpaths conformal to the nonplanar surface. Random channel routes such as serpentine mixers could be printed with this method (Fig. 6b-i). The demonstrated microfluidic mixing network also realized selective input sources via the integrated valves (Fig. 6b-ii).
To print microfluidics in more complex situations such as surfaces with large curvatures or deforming substrates, recent developments inthe in situ printing of soft electronics provide potential solutions.15,108 Additive manufacturing is a powerful platform for the automated fabrication of functional devices, yet more advanced control algorithms are necessary to guarantee both automation and accuracy. Zhu et al. demonstrated a closed-loop AI process that combined 3D scanning, real-time feature tracking, and motion prediction for 3D printing of biological sensors on deforming surfaces (Fig. 6c-i and ii). The 3D printed sensor was composed of ionic hydrogel inks which, after curing, can monitor the volumetric strain of the organ surface by mapping via electrical impedance tomography (Fig. 6c-iii and iv).
2.5. Discussion on future research
The seamless integration of 3D printed microfluidics with microsensing arrays is a promising avenue for the comprehensive manufacturing of packaged biochemical sensors. Yet, several technical issues remain towards achieving this goal, such as improving the resolution of printed microfluidic features to match those of the microsensing arrays, enhancing the alignment accuracy via the aid of computer vision, and optimizing the efficacy of functionalized sensing arrays with 3D printing. The modularized assembly approach may appear to reduce the degree of automation for 3D printed microfluidics, but the overall printing efficiency is improved when considering the geometrical precision and elimination of postprocessing steps such as the removal of supporting materials. The further development of photopolymerization processes for SL and PolyJet printing can help reduce or eliminate manual post-processing procedures. SL has been particularly suitable for device miniaturization and scalable integration. Other emerging methods utilizing extrusion-based printing or PolyJet provide new capabilities, such as the elimination of sacrificial inks and incorporation of multiple materials. Lastly, 3D printed conformal microfluidics benefit from state-of-the-art microfluidic structural design methods and printing algorithms, indicating an emerging prospect for advanced in situ biological sampling and analysis. Opportunities still exist in developing large-scale integrated microfluidic platforms and moving toward fully autonomous fabrication, pending further progress in developing inks, maximizing throughput, and programming control algorithms.
3. Advances in applications: droplets, point-of-care, and soft robotics
3D printed microfluidics provide an emerging capability to conformally and precisely integrate microfluidics with functional components and realize more advanced applications in biomedical research, the healthcare industry, bioprocessing (e.g., upstream cell cultivation and downstream purification),111–116 chemical synthesis and analysis, and fuel cells,117,118etc. Comprehensive review articles are available for each application based on either conventionally fabricated or 3D printed microfluidics.2,4,119 In this section, we highlight the progress of 3D printed microfluidic devices in advancing (a) droplet-based microfluidics, (b) point-of-care devices, and (c) soft robotics. Finally, an outlook is provided on the use of 3D printing technologies for further expanding on these critical microfluidic applications.
3.1. 3D printed droplet-based microfluidics
Droplet-based microfluidics are a powerful tool for advancing areas such as nanoparticle synthesis, high-throughput drug screening, and antibody discovery, etc.120–123 The most common method for passive droplet generation using microfluidics is to induce liquid instability, which originates from the adjacent immiscible fluids and the complex channel geometry.124 Conventional fabrication methods based on soft lithography require specialized equipment, well-trained personnel, and a cleanroom facility, which restricts the accessibility of this technology.121 By contrast, 3D printing provides an alternative method to fabricate customized droplet-based microfluidics under ambient conditions, anywhere and at any time. While PDMS-based microfluidics remains the prevailing method for droplet-related microfluidic applications, 3D printed counterparts are rapidly gaining interest and expanding to more complex and customized microfluidic operations. Typically, 3D printed droplet-based microfluidic devices generate droplets with sizes ranging from 50 μm to 500 μm, at a production rate of 100 Hz, with high monodispersity.125
3D printed droplet-based microfluidics manifest in three different types: the T-junction,126–133 flow-focusing,8,134,135 and co-flow.129,135–143 The co-flow configuration is typically non-planar and difficult to achieve in conventional PDMS-based microfluidics, whereas 3D printed non-planar droplet-based microfluidics can enable reliable emulsion droplet generation without the need for local surface modulation.129,135–144 One representative work increased the contact between the disperse and continuous phases, by fully surrounding the disperse phase with the continuous phase instead of wetting the channel walls. This was accomplished even when the cylindrical microfluidic channels were 3D printed using hydrophilic resins (Fig. 7a).137
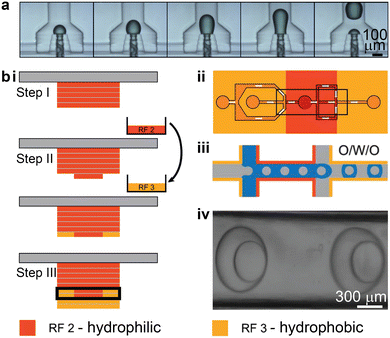 |
| Fig. 7 3D printing-enabled approaches for stable emulsion droplet generation. (a) 3D printed co-flow structures. Image sequences showing the dynamic process of droplet generation in a device with a 3D annular channel-in-channel design. (b) Local surface modulation enabled by multi-material 3D printing. i. Protocol for combining hydrophilic and hydrophobic resins in microfluidic fabrication. The exchange of resin is involved in step II. The microchannel is boxed in step III. ii. Schematic of a proposed design for generating O/W/O double emulsion droplets. iii. Illustration of how the double emulsion droplets can be stably formed through the hydrophobic–hydrophilic–hydrophobic locally modulated microfluidic channels. iv. Brightfield microscopy image showing the successful generation of two double emulsion droplets. (a) Was adapted with permission from ref. 137. (b) Was adapted with permission from ref. 145. | |
Multi-material printing has been employed to integrate both hydrophobic and hydrophilic materials into one microfluidic device (Fig. 7b-i).145 Mannel et al. demonstrated a fabrication process that started with printing the hydrophilic resin, and then the resin vat was switched to one containing hydrophobic resin to print the remaining part of the device. This enabled spatial control over the surface wettability, which is important for generating complex double-emulsion droplets. An oil-in-water droplet was first formed after travelling through the first hydrophobic region and the second hydrophilic region, after which the single emulsion droplet was encapsulated by oil in the third hydrophobic region (Fig. 7b-ii and iii). Even though this method adopted the planar junction design, the locally modulated surface wettability led to the stable generation of double emulsion droplets (Fig. 7b-iv).
3D printed droplet-based microfluidics present unique features or functionalities such as modular design,129,133,138,139,141,142,146 facile integration,8,126,130,138,147 and parallelization.148,149 Unlike the monolithic design of microfabrication, modular microfluidics provide a standard library of discrete microfluidic elements that can be assembled to rapidly construct droplet generators. For instance, by combining discrete microfluidic components, e.g., straight passes, L-joints, mixers, T-junctions, and X-junctions, emulsion droplet generators of the T-junction and flow-focusing types can be rapidly prototyped (Fig. 8a-i and ii).133 Moreover, the reversible transformation between the T-junction droplet-based microfluidics and the flow-focusing type can be rapidly realized by switching between the T-junction and X-junction elements.
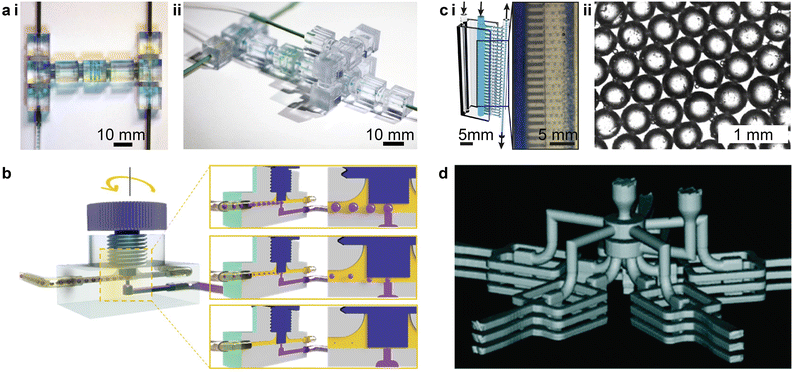 |
| Fig. 8 Unique features and functionalities enabled by 3D printed droplet-based microfluidics. (a) Modular 3D microfluidics for droplet generation. i. Assembly of a T-junction prototype based on prefabricated discrete microfluidic elements. ii. Assembly of a flow-focusing prototype. (b) Reconfigurable droplet microfluidics with integrated screw-and-nut mechanism. The droplet size is controlled by adjusting the gap between the channel surface and the screw nut front end around the junction area. (c) Rapid prototyped high-throughput emulsion droplet generator. i. Illustration of the vertical parallelization of 28 channels for high-throughput droplet generation. The inset shows a microscopic image for the production of numerous water-in-oil droplets. ii. Highly uniform droplets are generated as indicated by the hexagonal lattice structure. (d) Three-dimensional parallelized droplet generator. The 3D reconstructed microcomputed tomography (μCT) image shows that the device features five radially distributed stacks of flow-focusing type droplet generators, with three individual ones in each stack. (a) Was adapted with permission from ref. 133. (b) Was adapted with permission from ref. 126. (c) Was adapted with permission from ref. 149. (d) Was adapted with permission from ref. 148. | |
3D printed devices can also be integrated with commercial components for enhanced controllability and flexibility. For instance, by integrating a screw-and-nut mechanism into the T-junction droplet generator, the vertical junction gap can be finely tuned during operation (Fig. 8b).126 Though the characteristic size of the collection channel is on the order of 1 mm, the adjustable necking depth at the T-junction generates water-in-oil emulsion droplets with diameters ranging from 39 μm to 1404 μm. In another example, by integrating capillary tubing, tiny droplets smaller than 50 μm can be generated via the crossflow junction configuration while maintaining the characteristic size of the fluidic channels above 100 μm.130,147 Because the sizes of the generated droplets depend on the inner diameter of the outlet tubing, the generation of monodisperse droplets with sizes between 20 μm and 1 mm was successfully achieved by varying the tubing sizes.147
One important functionality enabled by 3D printed droplet microfluidics is large-scale parallelization for high-throughput production of droplets. Via the parallelization of 28 junction-type droplet generators along the vertical direction, the device demonstrated by Femmer et al. shared common inlets for the disperse and continuous phases, and a common outlet for the collection of emulsion droplets (Fig. 8c-i).149 An analysis of over 100 droplets collected from different parallel junctions indicated a standard deviation of less than 5% for the droplet diameters (Fig. 8c-ii). A 3D radial parallelization was also created by first stacking each single droplet generator vertically and then distributing branches of the stacked units in the radial direction (Fig. 8d).148 This 3D parallelized droplet-based microfluidic device has been reported to efficiently produce cell-laden microgels, resulting in a high viability (>95%) after cell encapsulation.
3.2. 3D printed microfluidics for point-of-care applications
Devices designed for point-of-care testing (POCT) and multiplexing diagnostics are important tools in modern healthcare, with the promise of reducing the need for laboratory personnel and facilities.150 Multiplexing the diagnostics further enables the simultaneous detection of multiple targets from a single sample. Portable and automated POCT platforms have been demonstrated for the rapid sensing of proteins and other molecules with comparable limits of detection (LOD) to their benchtop counterparts.151,152 Particularly, during the COVID-19 pandemic, numerous 3D printed POCT devices have emerged for detecting multiple pathogens including the coronavirus.152–154
The development of miniaturized and integrated fluid handling components for POCT applications can allow for compactness, simplicity, and on-site diagnostics.98,155–157 Different from the autonomous components introduced in section 2.3, microfluidic valves and pumps 3D printed for POCT devices are typically manually operated. For instance, 3D printed microfluidic components, including torque-actuated pumps and rotary valves, used manual actuation to deliver the fluid sample to the designated sites in the microfluidic networks for reacting and sensing, without the need for external pneumatic or hydraulic power sources (Fig. 9a-i and ii).98 Similar “finger-actuated” POCT devices have been developed as standalone microfluidic platforms for on-site water quality monitoring by detecting waterborne pathogens (Fig. 9a-iii).155 The 3D printed membrane can be actuated when manually squeezed to load the samples into the detection chamber, where enzyme-specific reagents and nutrient media were preloaded for bacteria culture and subsequent sensing. Recently, such “finger-actuated” membrane pumps have been integrated with “fluidic diodes” into more complex microfluidic circuits, such as a pulsatile two-fluid mixer, based on MultiJet 3D printing (Fig. 9b-i and ii).156 The membrane pump generated flow rates above 100 μL min−1, as controlled by the pumping frequency (Fig. 9b-iii). Though not evaluated for specific POCT applications, the work demonstrated a promising approach to fabricating versatile POCT devices for more complex fluid handling capabilities.
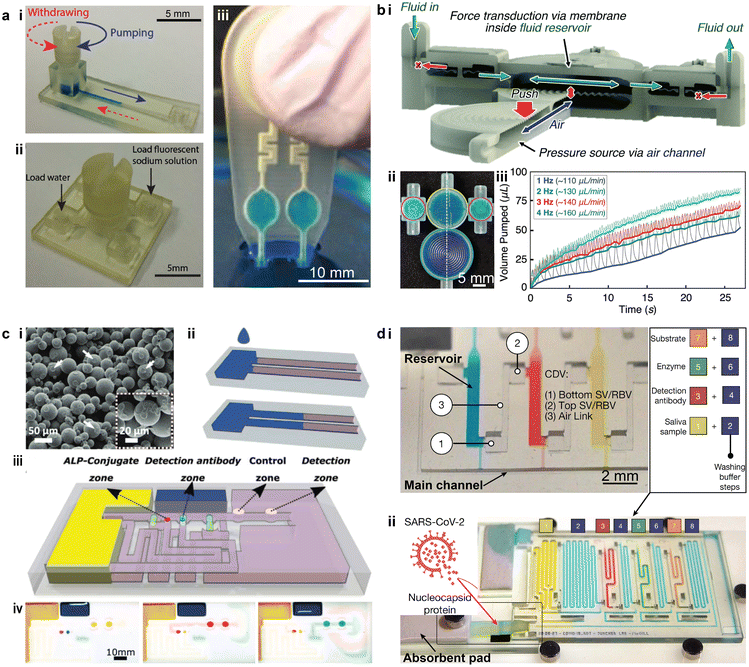 |
| Fig. 9 Examples of using 3D printed microfluidics for liquid handling in POCT devices. (a) Cost-effective 3D printed microfluidic components for manual liquid handling in POCT applications. i. 3D printed torque-actuated pumping unit for drawing liquid. ii. Rotary valve that switches among four individual ports. iii Finger-actuated 3D printed microfluidic platform for onsite water quality testing. (b) Finger-powered fluidic actuation and mixing for complex POCT applications. i. Cross-section of the device illustrating the actuation mechanism and flow direction. ii. Image of a 3D printed finger-powered two-fluid pulsatile mixer filled with blue dye fluid. iii. Relationship between pumped volume and time under different pumping frequencies. (c) 3D printed capillary microfluidic devices for POCT applications. i. SEM image of the bonded PMMA particles in a 3D printed porous part. ii. Illustration of liquid wicking (blue) in the porous solid constrained by the hydrophobic barriers. iii. Demonstration of a 3D printed capillary microfluidic device for enzyme-linked immunosorbent assays. The assay protocol is pre-programmed at the time of the device fabrication. iv. Optical images of the functional zones during the autonomous multistep assay. Coloured fluids were used to simulate different reagents. (d) 3D printed microfluidic chain reaction (MCR) system with structurally programmed capillary flow events. i. An MCR unit consisting of three reservoirs that were chained through capillary domino valves (CDVs). ii. An assembled MRC chip functionalized with SARS-CoV-2 nucleocapsid protein for assaying SARS-CoV-2 antibodies. (a-i and ii) Was adapted with permission from ref. 98. (a-iii) Was adapted with permission from ref. 155. (b) Was adapted with permission from ref. 156. (c) Was adapted with permission from ref. 162. (d) Was adapted with permission from ref. 163. | |
Capillary microfluidics is another approach to tackle the challenge of fluid handling and delivery in POCT devices, where passive transport of liquids is induced by the capillary action that occurs in either porous materials or fine capillary channels.158 Similar to paper microfluidics, the critical step in 3D printed devices is to create the hydrophobic barrier in the paper or other porous material so that the confined wicking channel could be formed.159 For instance, polypropylene (PP) filaments could be 3D printed on Whatman filter paper, and the bottom of the paper could be coated with wax pastel.160 For porous microfluidics, a common practice is to 3D print the base channel and then fill these open, hollow channels with cellulose powder that can enhance the capillary motion of liquids.161 Notably, a fully 3D printed porous capillary microfluidic device has been demonstrated based on poly(methyl methacrylate) (PMMA) powders (Fig. 9c-i).162 Hydrophobic walls in the hydrophilic porous material were formed by printing a binder that contains long-chain alkyl succinic anhydride at the designated locations. Consequently, the liquid wicking only occured in the nonfunctionalized PMMA strip and thus a well-confined liquid transport was created (Fig. 9c-ii). Moreover, this work presented a method to control the wicking flow in the porous channel via a third control channel which allowed the capillary flow to be pre-programmed, and analytical sequences could be designed for complex diagnostic tasks (Fig. 9c-iii). A proof-of-concept multi-step enzyme-linked immunosorbent assay (ELISA) was conducted using the device with precisely controlled capillary flows (Fig. 9c-iv).
To overcome the limited programmability of conventional capillary microfluidics, a recent study demonstrated the concept of a microfluidic chain reaction (MCR) by leveraging a structurally programmed capillary flow.163 Made on a projection SL printer, the monolithic microfluidic chips consisted of a series of reservoirs interconnected with capillary domino valves (CDVs). The confinement of liquid in the reservoir was enabled through a set of capillary valves based on a geometrical change in the capillary channels. The depletion of liquid in one reservoir triggered the movement of liquid in the next one, thereby controlling the propagation of a sequence of capillary flow events in serial, branching, or cascade configurations (Fig. 9d-i). Capillary pumps consisting of paper or absorbent pads were used to drain the liquid in each reservoir via the shared main channel, such that the subsequent one became exposed to the ambient via its air link. A large-scale capillary network integrated with 75 MCRs was demonstrated without disruption to the programmed propagation of capillary flow events. The automation of this system was confirmed via biochemical assays such as the automated detection of the SARS-CoV-2 antibody (Fig. 9d-ii).
3.3. 3D printed soft robotics embedded with microfluidic networks
3D printed microfluidics could also benefit the emerging field of soft robotics. A soft robotic entity typically comprises power sources, soft controllers, actuators, and necessary interconnecting networks to transmit the power.164 Early work utilized 3D printing to create the embedded micro-scale pneumatic network, which bridged the microfluidic control logic elements and the soft actuators.165 This work presented an entirely soft and autonomous robot which utilized the catalysed decomposition of monopropellant stored in the fuel reservoirs to generate gases to power the robot (Fig. 10a-i). An embedded 3D printing method was designed to fabricate the pneumatic microfluidic networks by extruding a fugitive ink (Pluronic® F-127 gel) within an uncured elastomer matrix (Fig. 10a-ii). To clear the microfluidic channels, the fugitive ink was auto-evacuated by heating the cured elastomer. The width of the ink filament and thus the size of the pneumatic channels could potentially be smaller than 100 μm, though the demonstrated prototype was regarded as a “mesofluidic” network.
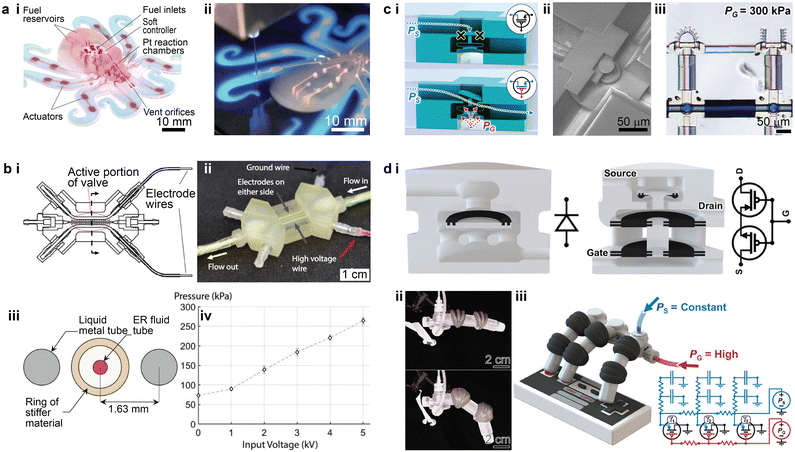 |
| Fig. 10 Examples of the integration of 3D printed microfluidics for soft robotic applications. (a) 3D printed interconnecting network for pneumatic power transmission. i. Photograph of a fully soft, autonomous robot assembly with an embedded microfluidic soft controller and 3D printed interconnecting network. ii. Fluidic interconnecting networks being embedded by extruding a fugitive ink. (b) 3D printed electroactive fluidic valve for power regulation of soft hydraulic robots. i. Top view cross-sectional schematic of the electroactive valve. ii. Image of a fully assembled electroactive valve. iii. Side view cross-sectional diagram showing the active portion of the valve with rings. iv. Plot of the average pressure held by the valve at steady state versus applied voltage. (c) 3D printed microrobotic actuators and controllers via in situ direct laser writing. i. Schematics showing the structure of the “normally closed” microfluidic transistor in closed and open states. ii. SEM image of a “normally closed” microfluidic transistor cross-section. iii. Image showing bending of one micro actuator triggered by the microfluidic transistor, while the other one is untriggered. (d) 3D printed soft robots with integrated fluidic circuitry. i. Conceptual illustration of the fluidic diode (left) and the normally closed fluidic transistor (right). ii. Images of a soft robotic finger with an integrated transistor after gate pressures of 0 kPa (top) and 20 kPa (bottom) were applied. iii. Conceptual illustration of a soft robotic hand after the maximum gate pressure was applied. Corresponding analogous circuit diagrams are on the lower right. (a) Was adapted with permission from ref. 165. (b) Was adapted with permission from ref. 166. (c) Was adapted with permission from ref. 17. (d) Was adapted with permission from ref. 75. | |
Beyond the interconnecting networks, a few recent studies have developed methods to integrate 3D printed microfluidics or millifluidics into the fluidic controller networks within soft robots.17,75,166 For instance, a microfluidic controller consisting of a 3D printed hydraulic valve was recently demonstrated (Fig. 10b-i and ii).166 The soft tentacle-like actuators were powered by the hydraulic pressure within the fluidic network, where an electrorheological (ER) fluid was utilized as the working liquid. The reversible response of the ER fluid under an electric field switches the hydraulic pressure in the soft actuators between the high and low states. Besides the working fluid channel, two parallel circular channels under 1 mm were printed and filled with a liquid metal, Galinstan (an alloy of gallium, indium, and tin), as the electrodes to apply the required electric field (Fig. 10b-iii). The 3D printed valve displayed a linear correlation between the pressure-holding capability and the applied voltage in the tested range (Fig. 10b-iv).
Higher levels of integration between the microfluidics and soft robotics were recently reported (Fig. 10c), where the entire soft robotic entity was fabricated inside the microfluidic channel (40 μm in height and 60 μm in width) via the isDLW technique.17 The microfluidic channels connected the hydraulic pressure source and the microfluidic controller. The bellow-structured controller acted as a normally closed transistor, in which a certain gate pressure was required to expand the bellow to displace the disk such that the flow can travel through the structure (from left to right) to inflate the bellow actuator (Fig. 10c-i and ii). The integrated soft robotic circuitry consisting of 3D printed microfluidic transistors and microgrippers demonstrated successful operation modulated by the source and gate pressures (Fig. 10c-iii).
Based on the integration and miniaturization of the 3D printed microfluidic logic elements, follow-up work was conducted to increase the complexity of the microfluidic logic tasks (Fig. 10d-i).75 Multi-material PolyJet 3D printing was employed to fabricate the soft robotic system in a single run with integrated fluidic circuitry. For the 3D printed microfluidic logic elements, an elastic material was used for the diaphragm and O-rings, while a rigid material was used to construct other parts of the body. This approach allowed one to fabricate a variety of fluidic logic elements, from simple fluidic diodes to complicated logic arrays. The 3D printed complex fluidic logic elements enabled periodic motion of a soft robotic finger and aperiodic motion of a soft robotic hand (Fig. 10d-ii and iii).
3.4. Discussion on future research
Though 3D printed microfluidic devices provide unique advantages, such as rapid prototyping, 3D integration, and multi-material fabrication for the reviewed applications, there is room for performance improvement. Specifically, 3D printed droplet microfluidics capable of generating tiny droplets (diameter <50 μm) at high frequency (>1 kHz) will be valuable for improving the throughput of the particle generation. For POCT applications, 3D printing has proven to be a cost-effective method for the fabrication of microfluidic-based devices. The next step is to realize mass production to establish 3D printing as a cost-effective POCT choice for diagnostics in the developing world. This might involve developing new printing strategies, such as multi-nozzle and multi-head printing, and optimizing printing parameters for high throughput and quality control. Mass production might also be achievable via the miniaturisation and widespread distribution of 3D printers, effectively “crowd-sourcing” the production, especially in resource limited environments. Finally, the integration of 3D printed microfluidics with soft robotics is a compelling direction rich with potential applications, such as microfluidics-based wearable devices for rehabilitation, haptic technologies, and distributed autonomous systems.
4. Conclusions and outlook
We inspected the progress in various 3D printing methodologies for microfluidic devices, including the recently employed DLW, by highlighting innovations in printing strategies, process control algorithms, material properties, and integration innovations. Notably, extrusion-based printing allows for the printing of thermosetting polymers such as silicone on curvilinear surfaces without requiring sacrificial supporting materials. SL printing has witnessed an improvement in resolution to the sub-100 μm regime, by tailoring the optical exposure and in situ assembly of the 3D printed parts during fabrication. Novel printing principles such as volumetric SL or AI-assisted toolpath predictions suggest that 3D printing could potentially achieve higher throughput and dynamic adaptability. The ink palette for 3D printed microfluidics has also witnessed a substantial leap by incorporating elastomeric materials with mechanical, optical, and chemical properties comparable to those of PDMS. These synergistic advances have expanded the range of applications beyond proof-of-concept demonstrations, such as the utilization of 3D printed droplet generators in drug screening and disease modelling, biological replication, point-of-care devices, soft robotics, and elsewhere. The emerging trend indicates that unique features of 3D printing such as freeform construction of 3D micro architectures and seamless integration with sensing arrays are becoming more feasible. Indeed, 3D printing has been established as a viable and promising rapid prototyping technology in the microfluidics community for fundamental research and engineering applications.
Moving forward, we expect that a few challenges will have to be overcome for 3D printing microfluidic devices, including, but not limited to, multimaterial and multifunctional printing, high resolution printing, and scalable fabrication. Existing methods rely on unitary polymerization or deposition modalities, which typically yield monolithic structures. Yet, progress in integrating printing heads that house multiple materials of distinct properties, along with novel methods such as embedded 3D printing (particularly for Polyjet printing and extrusion-based printing) have enabled initial demonstrations of multimaterial functional devices.167–169 We expect that transferring these insights to the fabrication of microfluidic devices will significantly expand the composition and functionality of microfluidics. Further, as suggested by the isDLW technique,16 another promising strategy to meet this demand is to integrate the features of different printing methods by developing multimodal and multimaterial platforms where devices possessing multiscale features or functionalities can be printed in a high-throughput manner. Our discussion also reveals that research into the fundamental physics, such as microscale material mechanics and the precise control of photopolymerization dose, will contribute as a driving force to improve figures of merit such as structural resolution, fluidic control and integration scales. As the complexity of microfluidic devices and requirements for material tunability increase, geometric optimization and material discovery will demand new research insights. Computational methods such as machine learning coupled with limited experimental input have proven to be a compelling approach to enhance the automation of 3D printed microfluidics.170–172 In terms of applications, we foresee that biological and chemical applications will continue to be key fields of interest where 3D printed microfluidics will provide a versatile role. Promisingly, examples of multifunctional integrated devices such as wearable sensors and soft robotics will thrive as the freeform fabrication capabilities of 3D printing continue to develop.
Author contributions
RS, FW, and MCM conceived the framework of the paper. RS organized the review paper and wrote section 1 and section 2. FW drafted section 3. MCM provided a comprehensive review and edits to the manuscript.
Conflicts of interest
There are no conflicts to declare.
Acknowledgements
Effort for this review article was supported by the National Science Foundation under grant PHY-2020695. The authors would like to thank Dr. Ghazaleh Haghiashtiani for input related to material development and comments during the preparation of the manuscript.
References
- D. J. Harrison, K. Fluri, K. Seiler, Z. Fan, S. Effenhauser Carlo and A. Manz, Science, 1993, 261, 895–897 CrossRef CAS PubMed.
- P. N. Nge, C. I. Rogers and A. T. Woolley, Chem. Rev., 2013, 113, 2550–2583 CrossRef CAS PubMed.
- Y. Xia and G. M. Whitesides, Annu. Rev. Mater. Sci., 1998, 28, 153–184 CrossRef CAS.
- S. Waheed, J. M. Cabot, N. P. Macdonald, T. Lewis, R. M. Guijt, B. Paull and M. C. Breadmore, Lab Chip, 2016, 16, 1993–2013 RSC.
- N. Bhattacharjee, A. Urrios, S. Kang and A. Folch, Lab Chip, 2016, 16, 1720–1742 RSC.
-
K. Sharp, R. Adrian, J. Santiago and J. I. Molho, in MEMS: Introduction and Fundamentals, ed. M. Gad-el-Hak, 2 edn, 2005, ch. 10 Search PubMed.
- J. B. You, K. Kang, T. T. Tran, H. Park, W. R. Hwang, J. M. Kim and S. G. Im, Lab Chip, 2015, 15, 1727–1735 RSC.
- T. Ching, Y. Li, R. Karyappa, A. Ohno, Y.-C. Toh and M. Hashimoto, Sens. Actuators, B, 2019, 297, 126609 CrossRef CAS.
- C. Corbett Daniel, B. Fabyan Wesley, B. Grigoryan, E. Connor Colleen, F. Johansson, I. Batalov, C. Regier Mary, A. DeForest Cole, S. Miller Jordan and R. Stevens Kelly, Sci. Adv., 2020, 6, eabb9062 CrossRef PubMed.
- J. Jia, Q. Song, Z. Liu and B. Wang, Microsyst. Technol., 2018, 25, 2385–2397 CrossRef.
- N. P. Macdonald, J. M. Cabot, P. Smejkal, R. M. Guijt, B. Paull and M. C. Breadmore, Anal. Chem., 2017, 89, 3858–3866 CrossRef CAS PubMed.
- M. Zeraatkar, D. Filippini and G. Percoco, Micromachines, 2019, 10(5), 298 CrossRef PubMed.
- R. Su, J. Wen, Q. Su, M. S. Wiederoder, S. J. Koester, J. R. Uzarski and M. C. McAlpine, Sci. Adv., 2020, 6, eabc9846 CrossRef CAS PubMed.
- M. Singh, Y. Tong, K. Webster, E. Cesewski, A. P. Haring, S. Laheri, B. Carswell, T. J. O'Brien, C. H. Aardema, R. S. Senger, J. L. Robertson and B. N. Johnson, Lab Chip, 2017, 17, 2561–2571 RSC.
- Z. Zhu, H. S. Park and M. C. McAlpine, Sci. Adv., 2020, 6, eaba5575 CrossRef PubMed.
- A. T. Alsharhan, R. Acevedo, R. Warren and R. D. Sochol, Lab Chip, 2019, 19, 2799–2810 RSC.
- A. T. Alsharhan, O. M. Young, X. Xu, A. J. Stair and R. D. Sochol, J. Micromech. Microeng., 2021, 31, 044001 CrossRef CAS.
- G. Weisgrab, A. Ovsianikov and P. F. Costa, Adv. Mater. Technol., 2019, 4, 1900275 CrossRef CAS.
- J. A. Lewis and G. M. Gratson, Mater. Today, 2004, 7, 32–39 CrossRef CAS.
- D. Therriault, S. R. White and J. A. Lewis, Nat. Mater., 2003, 2, 265–271 CrossRef CAS PubMed.
- B. K. David, A. H. Kimberly, A. S.-S. Mark and A. L. Jennifer, Proc. Natl. Acad. Sci. U. S. A., 2016, 113, 3179–3184 CrossRef PubMed.
- D. Pranzo, P. Larizza, D. Filippini and G. Percoco, Micromachines, 2018, 9, 374 CrossRef PubMed.
- G. W. Bishop, J. E. Satterwhite, S. Bhakta, K. Kadimisetty, K. M. Gillette, E. Chen and J. F. Rusling, Anal. Chem., 2015, 87, 5437–5443 CrossRef CAS PubMed.
- M. G. Guerra, C. Volpone, L. M. Galantucci and G. Percoco, Addit. Manuf., 2018, 21, 53–62 CAS.
- M. D. Symes, P. J. Kitson, J. Yan, C. J. Richmond, G. J. T. Cooper, R. W. Bowman, T. Vilbrandt and L. Cronin, Nat. Chem., 2012, 4, 349–354 CrossRef CAS PubMed.
-
B. Müller and W. Rath, Formulating adhesives and sealants : chemistry, physics and applications, Vincentz Network, Hannover, Germany, 2010 Search PubMed.
- H. A. Barnes, J. Non-Newtonian Fluid Mech., 1999, 81, 133–178 CrossRef CAS.
- S. Keshan Balavandy, F. Li, N. P. Macdonald, F. Maya, A. T. Townsend, K. Frederick, R. M. Guijt and M. C. Breadmore, Anal. Chim. Acta, 2021, 1151, 238101 CrossRef CAS PubMed.
- R. D. Sochol, E. Sweet, C. C. Glick, S. Venkatesh, A. Avetisyan, K. F. Ekman, A. Raulinaitis, A. Tsai, A. Wienkers, K. Korner, K. Hanson, A. Long, B. J. Hightower, G. Slatton, D. C. Burnett, T. L. Massey, K. Iwai, L. P. Lee, K. S. Pister and L. Lin, Lab Chip, 2016, 16, 668–678 RSC.
- B. Hayes, T. Hainsworth and R. MacCurdy, Addit. Manuf., 2022, 55, 102785 CAS.
- J. M. Lee, M. Zhang and W. Y. Yeong, Microfluid. Nanofluid., 2016, 20, 5 CrossRef.
- A. D. Castiaux, C. W. Pinger, E. A. Hayter, M. E. Bunn, R. S. Martin and D. M. Spence, Anal. Chem., 2019, 91, 6910–6917 CrossRef CAS PubMed.
-
R. MacCurdy, R. Katzschmann, K. Youbin and D. Rus, 2016 IEEE International Conference on Robotics and Automation (ICRA), Stockholm, Sweden, 2016, pp. 3878–3885, DOI:10.1109/ICRA.2016.7487576.
- B. C. Gross, J. L. Erkal, S. Y. Lockwood, C. Chen and D. M. Spence, Anal. Chem., 2014, 86, 3240–3253 CrossRef CAS PubMed.
- J. Huang, Q. Qin and J. Wang, Processes, 2020, 8(9), 1138 CrossRef CAS.
-
C. W. Hull, US Pat., 4,575,330, 1986 Search PubMed.
- A. Bertsch, J. Y. Jezequel and J. C. Andre, J. Photochem. Photobiol., A, 1997, 107, 275–281 CrossRef CAS.
- A. Bertsch, S. Zissi, J. Y. Jezequel, S. Corbel and J. C. Andre, Microsyst. Technol., 1997, 3, 42–47 CrossRef.
- D. A. Walker, J. L. Hedrick and C. A. Mirkin, Science, 2019, 366, 360–364 CrossRef CAS PubMed.
- J. R. Tumbleston, D. Shirvanyants, N. Ermoshkin, R. Janusziewicz, A. R. Johnson, D. Kelly, K. Chen, R. Pinschmidt, J. P. Rolland, A. Ermoshkin, E. T. Samulski and J. M. DeSimone, Science, 2015, 347, 1349–1352 CrossRef CAS PubMed.
- B. E. Kelly, I. Bhattacharya, H. Heidari, M. Shusteff, C. M. Spadaccini and H. K. Taylor, Science, 2019, 363, 1075 CrossRef CAS PubMed.
- D. Loterie, P. Delrot and C. Moser, Nat. Commun., 2020, 11, 852 CrossRef CAS PubMed.
- M. Regehly, Y. Garmshausen, M. Reuter, N. F. König, E. Israel, D. P. Kelly, C.-Y. Chou, K. Koch, B. Asfari and S. Hecht, Nature, 2020, 588, 620–624 CrossRef CAS PubMed.
- K. A. Smith, S. Habibi, M. P. de Beer, Z. D. Pritchard and M. A. Burns, Biomicrofluidics, 2022, 16, 044106 CrossRef CAS PubMed.
- M. P. de Beer, H. L. van der Laan, M. A. Cole, R. J. Whelan, M. A. Burns and T. F. Scott, Sci. Adv., 2019, 5, eaau8723 CrossRef PubMed.
-
P. P. Jouni, Lasers as Tools for Manufacturing of Durable Goods and Microelectronics, San Jose, CA, United States, 1996, vol. 2703, DOI:10.1117/12.237759.
- H. Lin, D. Zhang, P. G. Alexander, G. Yang, J. Tan, A. W.-M. Cheng and R. S. Tuan, Biomaterials, 2013, 34, 331–339 CrossRef CAS PubMed.
- H. K. Park, M. Shin, B. Kim, J. W. Park and H. Lee, NPG Asia Mater., 2018, 10, 82–89 CrossRef CAS.
- B. Grigoryan, S. J. Paulsen, D. C. Corbett, D. W. Sazer, C. L. Fortin, A. J. Zaita, P. T. Greenfield, N. J. Calafat, J. P. Gounley, A. H. Ta, F. Johansson, A. Randles, J. E. Rosenkrantz, J. D. Louis-Rosenberg, P. A. Galie, K. R. Stevens and J. S. Miller, Science, 2019, 364, 458–464 CrossRef CAS PubMed.
-
S. Corbel, O. Dufaud and T. Roques-Carmes, in Stereolithography: Materials, Processes and Applications, ed. P. J. Bartolo, Springer US, Boston, MA, 2011, DOI:10.1007/978-0-387-92904-0_6, pp. 141–159.
- D. K. Patel, A. H. Sakhaei, M. Layani, B. Zhang, Q. Ge and S. Magdassi, Adv. Mater., 2017, 29, 1606000 CrossRef PubMed.
- C. J. Thrasher, J. J. Schwartz and A. J. Boydston, ACS Appl. Mater. Interfaces, 2017, 9, 39708–39716 CrossRef CAS PubMed.
- N. Bhattacharjee, C. Parra-Cabrera, Y. T. Kim, A. P. Kuo and A. Folch, Adv. Mater., 2018, 30, 1800001 CrossRef PubMed.
- N. Weigel, M. J. Männel and J. Thiele, ACS Appl. Mater. Interfaces, 2021, 13, 31086–31101 CrossRef CAS PubMed.
-
J. Fourkas, in Laser Growth and Processing of Photonic Devices, ed. N. A. Vainos, Woodhead Publishing, 2012, DOI:10.1533/9780857096227.2.137, pp. 139–161.
- S. Maruo, O. Nakamura and S. Kawata, Opt. Lett., 1997, 22, 132–134 CrossRef CAS PubMed.
- M. Deubel, G. von Freymann, M. Wegener, S. Pereira, K. Busch and C. M. Soukoulis, Nat. Mater., 2004, 3, 444–447 CrossRef CAS PubMed.
- N. Anscombe, Nat. Photonics, 2010, 4, 22–23 CrossRef CAS.
- D. Nishiguchi, I. S. Aranson, A. Snezhko and A. Sokolov, Nat. Commun., 2018, 9, 4486 CrossRef PubMed.
-
Y. J. Heo, S. Iwanaga and S. Takeuchi, presented in part at the 2012 IEEE 25th International Conference on Micro Electro Mechanical Systems (MEMS), Paris, France, 29 Jan.-2 Feb. 2012, 2012.
- O. Vanderpoorten, Q. Peter, P. K. Challa, U. F. Keyser, J. Baumberg, C. F. Kaminski and T. P. J. Knowles, Microsyst. Nanoeng., 2019, 5, 40 CrossRef PubMed.
- D. A. Markov, E. M. Lillie, S. P. Garbett and L. J. McCawley, Biomed. Microdevices, 2014, 16, 91–96 CrossRef CAS PubMed.
- J. Y. Han, S. Warshawsky and D. L. DeVoe, Sci. Rep., 2021, 11, 10980 CrossRef CAS PubMed.
- R. F. Quero, G. S. D. da Silveira, J. A. F. da Silva and D. P. D. Jesus, Lab Chip, 2021, 21, 3715–3729 RSC.
- J. L. Sanchez Noriega, N. A. Chartrand, J. C. Valdoz, C. G. Cribbs, D. A. Jacobs, D. Poulson, M. S. Viglione, A. T. Woolley, P. M. Van Ry, K. A. Christensen and G. P. Nordin, Nat. Commun., 2021, 12, 5509 CrossRef CAS PubMed.
- Y. Xu, F. Qi, H. Mao, S. Li, Y. Zhu, J. Gong, L. Wang, N. Malmstadt and Y. Chen, Nat. Commun., 2022, 13, 918 CrossRef CAS PubMed.
- A. E. Goodling, S. Nagelberg, B. Kaehr, C. H. Meredith, S. I. Cheon, A. P. Saunders, M. Kolle and L. D. Zarzar, Nature, 2019, 566, 523–527 CrossRef CAS PubMed.
- Y. Haoyi, D. Haibo, Z. Qiming, G. Zhongze and G. Min, Adv. Manuf., 2020, 2, 31–38 Search PubMed.
- S. Rekstyte, M. Malinauskas and S. Juodkazis, Opt. Express, 2013, 21, 17028–17041 CrossRef PubMed.
- Y. Tao, C. Lu, C. Deng, J. Long, Y. Ren, Z. Dai, Z. Tong, X. Wang, S. Meng, W. Zhang, Y. Xu and L. Zhou, Micromachines, 2022, 13(1), 32 CrossRef PubMed.
- M. A. Skylar-Scott, J. Mueller, C. W. Visser and J. A. Lewis, Nature, 2019, 575, 330–335 CrossRef CAS PubMed.
- A. D. Castiaux, M. A. Selemani, M. A. Ward and R. S. Martin, Anal. Methods, 2021, 13, 5017–5024 RSC.
- G. van der Velden, D. Fan and U. Staufer, Micro Nano Eng., 2020, 7, 100054 CrossRef.
- M. Hippler, E. Blasco, J. Qu, M. Tanaka, C. Barner-Kowollik, M. Wegener and M. Bastmeyer, Nat. Commun., 2019, 10, 232 CrossRef PubMed.
- J. D. Hubbard, R. Acevedo, K. M. Edwards, A. T. Alsharhan, Z. Wen, J. Landry, K. Wang, S. Schaffer and R. D. Sochol, Sci. Adv., 2021, 7, eabe5257 CrossRef PubMed.
- M. C. McAlpine, H. D. Agnew, R. D. Rohde, M. Blanco, H. Ahmad, A. D. Stuparu, W. A. Goddard and J. R. Heath, J. Am. Chem. Soc., 2008, 130, 9583–9589 CrossRef CAS PubMed.
- A. T. Woolley, K. Lao, A. N. Glazer and R. A. Mathies, Anal. Chem., 1998, 70, 684–688 CrossRef CAS PubMed.
- Y. Yang, Y. Ohtake, T. Yatagawa and H. Suzuki, Virtual Phys. Prototyp., 2022, 17, 33–51 CrossRef.
- J. L. Erkal, A. Selimovic, B. C. Gross, S. Y. Lockwood, E. L. Walton, S. McNamara, R. S. Martin and D. M. Spence, Lab Chip, 2014, 14, 2023–2032 RSC.
- C. K. Tang, A. Vaze and J. F. Rusling, Lab Chip, 2017, 17, 484–489 RSC.
- S. A. N. Gowers, V. F. Curto, C. A. Seneci, C. Wang, S. Anastasova, P. Vadgama, G.-Z. Yang and M. G. Boutelle, Anal. Chem., 2015, 87, 7763–7770 CrossRef CAS PubMed.
- S. Arshavsky-Graham, A. Enders, S. Ackerman, J. Bahnemann and E. Segal, Microchim. Acta, 2021, 188, 67 CrossRef CAS PubMed.
- G. Fei, L. Nie, L. Zhong, Q. Shi, K. Hu, C. Parra-Cabrera, H. Oprins, R. Ameloot and S. Yang, Mater. Today Commun., 2022, 31, 103482 CrossRef CAS.
- R. B. Oueslati, D. Therriault and S. Martel, IEEE Trans. Compon. Packag. Technol., 2008, 31, 869–874 CAS.
- J. F. Rusling, ACS Sens., 2018, 3, 522–526 CrossRef CAS PubMed.
- B. M. C. Costa, A. G. Coelho, M. J. Beauchamp, J. B. Nielsen, G. P. Nordin, A. T. Woolley and J. A. F. da Silva, Anal. Bioanal. Chem., 2022, 414, 545–550 CrossRef CAS PubMed.
- Y. Shen, S. Modha, H. Tsutsui and A. Mulchandani, Biosens. Bioelectron., 2021, 171, 112721 CrossRef CAS PubMed.
- K. Kadimisetty, S. Malla and J. F. Rusling, ACS Sens., 2017, 2, 670–678 CrossRef CAS PubMed.
- Y. Alvarez-Brana, J. Etxebarria-Elezgarai, L. Ruiz de Larrinaga-Vicente, F. Benito-Lopez and L. Basabe-Desmonts, Sens. Actuators, B, 2021, 342, 129991 CrossRef CAS.
- Q. Ji, J. M. Zhang, Y. Liu, X. Li, P. Lv, D. Jin and H. Duan, Sci. Rep., 2018, 8, 4791 CrossRef PubMed.
- E. H. Childs, A. V. Latchman, A. C. Lamont, J. D. Hubbard and R. D. Sochol, J. Microelectromech. Syst., 2020, 29, 1094–1096 CAS.
- T. Ching, Y.-C. Toh and M. Hashimoto, Adv. Eng. Mater., 2020, 22, 1901109 CrossRef CAS.
- X. Chen, D. Mo and M. Gong, Micromachines, 2020, 11, 224 CrossRef PubMed.
- R. Rauti, A. Ess, B. Le Roi, Y. Kreinin, M. Epshtein, N. Korin and B. M. Maoz, APL Bioeng., 2021, 5, 026103 CrossRef CAS PubMed.
- J. Nie, Q. Gao, J.-J. Qiu, M. Sun, A. Liu, L. Shao, J.-Z. Fu, P. Zhao and Y. He, Biofabrication, 2018, 10, 035001 CrossRef PubMed.
- K. G. Lee, K. J. Park, S. Seok, S. Shin, D. H. Kim, J. Y. Park, Y. S. Heo, S. J. Lee and T. J. Lee, RSC Adv., 2014, 4, 32876–32880 RSC.
- M. R. Behrens, H. C. Fuller, E. R. Swist, J. Wu, M. M. Islam, Z. Long, W. C. Ruder and R. Steward, Sci. Rep., 2020, 10, 1543 CrossRef CAS PubMed.
- H. N. Chan, Y. Shu, B. Xiong, Y. Chen, Y. Chen, Q. Tian, S. A. Michael, B. Shen and H. Wu, ACS Sens., 2016, 1, 227–234 CrossRef CAS.
- H. Gong, A. T. Woolley and G. P. Nordin, Biomicrofluidics, 2019, 13, 014106 CrossRef PubMed.
- M. A. Unger, H.-P. Chou, T. Thorsen, A. Scherer and S. R. Quake, Science, 2000, 288, 113 CrossRef CAS PubMed.
- H. Gong, A. T. Woolley and G. P. Nordin, Lab Chip, 2016, 16, 2450–2458 RSC.
- Y. S. Lee, N. Bhattacharjee and A. Folch, Lab Chip, 2018, 18, 1207–1214 RSC.
- A. K. Au, N. Bhattacharjee, L. F. Horowitz, T. C. Chang and A. Folch, Lab Chip, 2015, 15, 1934–1941 RSC.
- C. I. Rogers, K. Qaderi, A. T. Woolley and G. P. Nordin, Biomicrofluidics, 2015, 9, 016501 CrossRef PubMed.
- T. Thorsen, S. J. Maerkl and S. R. Quake, Science, 2002, 298, 580 CrossRef CAS PubMed.
- I. E. Araci and S. R. Quake, Lab Chip, 2012, 12, 2803–2806 RSC.
- X. Zheng, J. Deotte, M. P. Alonso, G. R. Farquar, T. H. Weisgraber, S. Gemberling, H. Lee, N. Fang and C. M. Spadaccini, Rev. Sci. Instrum., 2012, 83, 125001 CrossRef PubMed.
- E. Kucukdeger, Y. Tong, M. Singh, J. Zhang, L. K. Harding, A. Salado, S. W. Ellingson and B. N. Johnson, Flexible Printed Electron., 2021, 6, 044002 CrossRef CAS.
- W. Zhang, L. Zhang, Y. Liao and H. Cheng, Int. J. Extreme Manuf., 2021, 3, 042001 CrossRef CAS.
- Z. Zhu, S.-Z. Guo, T. Hirdler, C. Eide, X. Fan, J. Tolar and M. C. McAlpine, Adv. Mater., 2018, 30, 1707495 CrossRef PubMed.
- T. Habib, C. Brämer, C. Heuer, J. Ebbecke, S. Beutel and J. Bahnemann, Lab Chip, 2022, 22, 986–993 RSC.
- S. Garcia-Rey, J. B. Nielsen, G. P. Nordin, A. T. Woolley, L. Basabe-Desmonts and F. Benito-Lopez, Polymer, 2022, 14, 2537 CAS.
- S. R. Bazaz, A. Mihandust, R. Salomon, H. A. N. Joushani, W. Li, H. A. Amiri, F. Mirakhorli, S. Zhand, J. Shrestha and M. Miansari, Lab Chip, 2022, 4093–4109 RSC.
- S. Razavi Bazaz, O. Rouhi, M. A. Raoufi, F. Ejeian, M. Asadnia, D. Jin and M. Ebrahimi Warkiani, Sci. Rep., 2020, 10, 1–14 CrossRef PubMed.
- J. Chen, C.-Y. Liu, X. Wang, E. Sweet, N. Liu, X. Gong and L. Lin, Biosens. Bioelectron., 2020, 150, 111900 CrossRef CAS PubMed.
- A. Enders, J.-A. Preuss and J. Bahnemann, Micromachines, 2021, 12, 1060 CrossRef PubMed.
- K.-E. Guima, L. E. Gomes, J. Alves Fernandes, H. Wender and C. A. Martins, ACS Appl. Mater. Interfaces, 2020, 12, 54563–54572 CrossRef CAS PubMed.
- K.-E. Guima, P.-H. L. Coelho, M. A. Trindade and C. A. Martins, Lab Chip, 2020, 20, 2057–2061 RSC.
- C. M. Ho, S. H. Ng, K. H. Li and Y. J. Yoon, Lab Chip, 2015, 15, 3627–3637 RSC.
- W. Li, L. Zhang, X. Ge, B. Xu, W. Zhang, L. Qu, C.-H. Choi, J. Xu, A. Zhang and H. Lee, Chem. Soc. Rev., 2018, 47, 5646–5683 RSC.
- N. Shembekar, C. Chaipan, R. Utharala and C. A. Merten, Lab Chip, 2016, 16, 1314–1331 RSC.
- B. Amoyav, Y. Goldstein, E. Steinberg and O. Benny, Pharmaceutics, 2020, 13, 13 CrossRef PubMed.
- F. Tian, L. Cai, C. Liu and J. Sun, Lab Chip, 2022, 512–529 RSC.
- P. Zhu and L. Wang, Lab Chip, 2017, 17, 34–75 RSC.
- N. Gyimah, O. Scheler, T. Rang and T. Pardy, Micromachines, 2021, 12, 339 CrossRef PubMed.
- H. Van Nguyen, H. Q. Nguyen, V. D. Nguyen and T. S. Seo, Sens. Actuators, B, 2019, 296, 126676 CrossRef.
- Z. Jiao, L. Zhao, C. Tang, H. Shi, F. Wang and B. Hu, Anal. Methods, 2019, 11, 3286–3293 RSC.
- V. Romanov, R. Samuel, M. Chaharlang, A. R. Jafek, A. Frost and B. K. Gale, Anal. Chem., 2018, 90, 10450–10456 CrossRef CAS PubMed.
- Q. Ji, J. M. Zhang, Y. Liu, X. Li, P. Lv, D. Jin and H. Duan, Sci. Rep., 2018, 8, 1–11 CAS.
- J. M. Zhang, A. A. Aguirre-Pablo, E. Q. Li, U. Buttner and S. T. Thoroddsen, RSC Adv., 2016, 6, 81120–81129 RSC.
- S. Tsuda, H. Jaffery, D. Doran, M. Hezwani, P. J. Robbins, M. Yoshida and L. Cronin, PLoS One, 2015, 10, e0141640 CrossRef PubMed.
- L. Donvito, L. Galluccio, A. Lombardo, G. Morabito, A. Nicolosi and M. Reno, J. Micromech. Microeng., 2015, 25, 035013 CrossRef.
- K. C. Bhargava, B. Thompson and N. Malmstadt, Proc. Natl. Acad. Sci. U. S. A., 2014, 111, 15013–15018 CrossRef CAS PubMed.
- Z. Chen, J. Y. Han, L. Shumate, R. Fedak and D. L. DeVoe, Adv. Mater. Technol., 2019, 4, 1800511 CrossRef.
- J. M. Zhang, Q. Ji, Y. Liu, J. Huang and H. Duan, Lab Chip, 2018, 18, 3393–3404 RSC.
- J. Zhang, W. Xu, F. Xu, W. Lu, L. Hu, J. Zhou, C. Zhang and Z. Jiang, J. Food Eng., 2021, 290, 110212 CrossRef CAS.
- C. A. Warr, H. S. Hinnen, S. Avery, R. J. Cate, G. P. Nordin and W. G. Pitt, Micromachines, 2021, 12, 91 CrossRef PubMed.
- S. Vijayan and M. Hashimoto, RSC Adv., 2019, 9, 2822–2828 RSC.
- R. Song, M. S. Abbasi and J. Lee, Microfluid. Nanofluid., 2019, 23, 1–11 CrossRef.
- M. J. Männel, L. Selzer, R. Bernhardt and J. Thiele, Adv. Mater. Technol., 2019, 4, 1800408 CrossRef.
- Z. Zhou, T. Kong, H. Mkaouar, K. N. Salama and J. M. Zhang, Sens. Actuators, A, 2018, 280, 422–428 CrossRef CAS.
- Y. Morimoto, M. Kiyosawa and S. Takeuchi, Sens. Actuators, B, 2018, 274, 491–500 CrossRef CAS.
- K. Alessandri, M. Feyeux, B. Gurchenkov, C. Delgado, A. Trushko, K.-H. Krause, D. Vignjević, P. Nassoy and A. Roux, Lab Chip, 2016, 16, 1593–1604 RSC.
- A. Rotem, A. R. Abate, A. S. Utada, V. Van Steijn and D. A. Weitz, Lab Chip, 2012, 12, 4263–4268 RSC.
- M. J. Männel, N. Weigel, N. Hauck, T. Heida and J. Thiele, Adv. Mater. Technol., 2021, 6, 2100094 CrossRef.
- A. J. Morgan, L. H. S. Jose, W. D. Jamieson, J. M. Wymant, B. Song, P. Stephens, D. A. Barrow and O. K. Castell, PLoS One, 2016, 11, e0152023 CrossRef PubMed.
- C. T. Riche, E. J. Roberts, M. Gupta, R. L. Brutchey and N. Malmstadt, Nat. Commun., 2016, 7, 1–7 CrossRef PubMed.
- T. Kamperman, L. M. Teixeira, S. S. Salehi, G. Kerckhofs, Y. Guyot, M. Geven, L. Geris, D. Grijpma, S. Blanquer and J. Leijten, Lab Chip, 2020, 20, 490–495 RSC.
- T. Femmer, A. Jans, R. Eswein, N. Anwar, M. Moeller, M. Wessling and A. J. Kuehne, ACS Appl. Mater. Interfaces, 2015, 7, 12635–12638 CrossRef CAS PubMed.
- V. Gubala, L. F. Harris, A. J. Ricco, M. X. Tan and D. E. Williams, Anal. Chem., 2012, 84, 487–515 CrossRef CAS PubMed.
- K. Kadimisetty, S. Malla, K. S. Bhalerao, I. M. Mosa, S. Bhakta, N. H. Lee and J. F. Rusling, Anal. Chem., 2018, 90, 7569–7577 CrossRef CAS PubMed.
- M. El-Tholoth, H. Bai, M. G. Mauk, L. Saif and H. H. Bau, Lab Chip, 2021, 21, 1118–1130 RSC.
- K. Yin, X. Ding, Z. Xu, Z. Li, X. Wang, H. Zhao, C. Otis, B. Li and C. Liu, Sens. Actuators, B, 2021, 344, 130242 CrossRef CAS PubMed.
- X. Ding, Z. Li and C. Liu, Sens. Actuators, B, 2022, 351, 130998 CrossRef CAS PubMed.
-
E. C. Sweet, N. Liu, J. Chen and L. Lin, 2019 IEEE 32nd International Conference on Micro Electro Mechanical Systems (MEMS), Seoul, Korea (South), 2019 Search PubMed.
- E. Sweet, R. Mehta, Y. Xu, R. Jew, R. Lin and L. Lin, Lab Chip, 2020, 20, 3375–3385 RSC.
- M. R. Behrens, H. C. Fuller, E. R. Swist, J. Wu, M. Islam, Z. Long, W. C. Ruder and R. Steward, Sci. Rep., 2020, 10, 1–10 CrossRef PubMed.
- L. Xu, A. Wang, X. Li and K. W. Oh, Biomicrofluidics, 2020, 14, 031503 CrossRef CAS PubMed.
- E. Carrilho, A. W. Martinez and G. M. Whitesides, Anal. Chem., 2009, 81, 7091–7095 CrossRef CAS PubMed.
- A. Zargaryan, N. Farhoudi, G. Haworth, J. F. Ashby and S. H. Au, Sci. Rep., 2020, 10, 1–9 CrossRef PubMed.
- Y. He, Q. Gao, W.-B. Wu, J. Nie and J.-Z. Fu, Micromachines, 2016, 7, 108 CrossRef PubMed.
- C. Achille, C. Parra-Cabrera, R. Dochy, H. Ordutowski, A. Piovesan, P. Piron, L. Van Looy, S. Kushwaha, D. Reynaerts and P. Verboven, Adv. Mater., 2021, 33, 2008712 CrossRef CAS PubMed.
- M. Yafia, O. Ymbern, A. O. Olanrewaju, A. Parandakh, A. Sohrabi Kashani, J. Renault, Z. Jin, G. Kim, A. Ng and D. Juncker, Nature, 2022, 605, 464–469 CrossRef CAS PubMed.
- C. Lee, M. Kim, Y. J. Kim, N. Hong, S. Ryu, H. J. Kim and S. Kim, Int. J. Control Autom. Syst., 2017, 15, 3–15 CrossRef.
- M. Wehner, R. L. Truby, D. J. Fitzgerald, B. Mosadegh, G. M. Whitesides, J. A. Lewis and R. J. Wood, Nature, 2016, 536, 451–455 CrossRef CAS PubMed.
- A. Zatopa, S. Walker and Y. Menguc, Soft Robot., 2018, 5, 258–271 CrossRef PubMed.
- T. Hanemann, D. Syperek and D. Notzel, Materials, 2020, 13(6), 1481 CrossRef CAS PubMed.
- R. L. Truby, M. Wehner, A. K. Grosskopf, D. M. Vogt, S. G. M. Uzel, R. J. Wood and J. A. Lewis, Adv. Mater., 2018, 30, 1706383 CrossRef PubMed.
- R. D. Weeks, R. L. Truby, S. G. M. Uzel and J. A. Lewis, Adv. Mater., 2022, 2206958 Search PubMed.
- C. Yu and J. Jiang, Int. J. Bioprint., 2020, 6, 253 Search PubMed.
- T. Erps, M. Foshey, K. Lukovic Mina, W. Shou, H. Goetzke Hanns, H. Dietsch, K. Stoll, B. von Vacano and W. Matusik, Sci. Adv., 2021, 7, eabf7435 CrossRef CAS PubMed.
- A. Lashkaripour, C. Rodriguez, N. Mehdipour, R. Mardian, D. McIntyre, L. Ortiz, J. Campbell and D. Densmore, Nat. Commun., 2021, 12, 25 CrossRef CAS PubMed.
|
This journal is © The Royal Society of Chemistry 2023 |
Click here to see how this site uses Cookies. View our privacy policy here.