DOI:
10.1039/D2RE90011D
(Editorial)
React. Chem. Eng., 2022,
7, 792-794
Introduction to the themed collection on digitalization in reaction engineering
Received
24th February 2022
, Accepted 24th February 2022
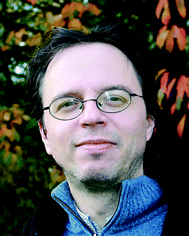 Federico Galvanin | Federico Galvanin is an associate professor of Chemical Engineering at University College London (UCL). He completed his MS and PhD in chemical engineering at the University of Padova (Italy). After a post-doctoral in Padova, he was appointed as a research associate at UCL where he became a lecturer in 2015. His research interests lie at the interface between mathematical modelling and experimentation and focus on the use of model-based design of experiments methods for the development of predictive models of complex systems. He has made significant literature contributions to the development of systems engineering methods and software tools for model identification using machine learning, optimal experimental design techniques and their use and integration in autonomous reactor platforms. |
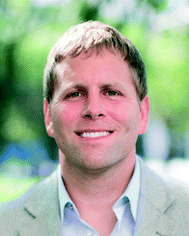 Ryan L. Hartman | Ryan L. Hartman is an associate professor in chemical and biomolecular engineering at New York University. He completed his BS at Michigan Technological University, a PhD at the University of Michigan, and his postdoctoral training at the Massachusetts Institute of Technology, all in chemical engineering. He has served as the Catalysis and Reaction Engineering Programming Chair of the American Institute of Chemical Engineers, an executive director of the International Symposia of Chemical Reaction Engineering, and as an advisory board member of Reaction Chemistry & Engineering. His research interests revolve around the design of artificial intelligence with continuous-flow microreactors for the discovery of reaction kinetics and mechanisms. |
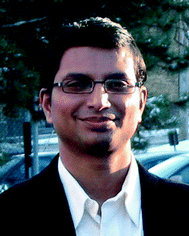 Amol A. Kulkarni | Amol A. Kulkarni is a scientist in the Chemical Engineering & Process Development Division at the CSIR-National Chemical Laboratory, Pune (Since 2005). He is a chemical engineer by training (B.E. and PhD, all from Institute of Chemical Technology, Mumbai). He was Humboldt Fellow at the Max Planck Institute-Magdeburg and later an IUSSTF research fellow at Massachusetts Institute of Technology. He works in the area of design and development of continuous flow reactors and their applications for flow syntheses of pharmaceutical intermediates, dyes, perfumery chemicals and nanomaterials, making notable contributions in the literature. He has developed several continuous processes and helped their implementation in large scale manufacturing. He also works on experimental and numerical analysis of interface dynamics of diffusion and reaction. |
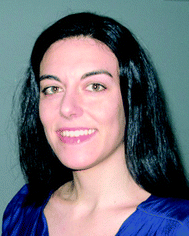 María José Nieves-Remacha | María José Nieves-Remacha is Senior Advisor at Lilly Research Laboratories at Eli Lilly and Company. She received her BS from Universidad Complutense de Madrid and PhD and MS in Chemical Engineering from Massachusetts Institute of Technology (2014). After that, she joined the Dow Chemical Company to work as a Senior Engineer in the Reaction Engineering group within the Core R&D Department at Freeport, TX. Her research interests are at the interface of computer science, engineering and chemistry including: computer-aided chemical synthesis and smart strategies for reaction optimization, through the combination of automation, flow technologies, inline monitoring and artificial intelligence/machine learning. |
A transformation of the chemicals industry driven by a need for the design of more efficient and sustainable chemical processes is underway.1,2 This fourth industrial revolution involves rethinking traditional approaches to wet chemistry laboratories, scale-ups, and production.3 Advancement in automation with artificial intelligence algorithms have created new opportunities for chemical reactors to work synchronously with digital twins,4–6 and although there has been an upswing in the number of papers on deep learning methods, few have explored their design and application in chemical reaction engineering. Featured in this themed issue, “Digitalization in Reaction Engineering”, are key studies centered around the convergence of deep learning methods, data science, automation, and spectroscopic analysis for reaction engineering.
The development of predictive reaction kinetic models, critical for the design of chemical reactors, historically depends on laborious experimentation often generating significant quantities of chemical waste that results in models limited to the range of tested conditions. The vast number of chemical reactions used in industrial applications7,8 further motivate versatility in the computational methodology, and ideally, one should be able to validate their models from a limited amount of data. An automated, computational approach to reaction network identification could accelerate kinetics development and catalyst selection, while requiring only a handful of experiments for validations (10.1039/d1re00098e). New approaches to the design of experiments for selecting a “fit for purpose” kinetic model could improve the efficient use of limited data and the design of the next set of experiments to advance early understanding of kinetics (10.1039/d1re00222h). Catalyst stability, its dependence on the composition and reaction conditions, yielding optimal kinetics are fundamental challenges that computer-aided design of experiments can help to discover (10.1039/d1re00441g).
Chemical reactions are without a doubt complex, which only adds to the arduous endeavour of designing chemical reactors. Reactor dynamics and phase behaviors have traditionally complicated industrial-scale reactor designs and operations,9 but with recent developments in deep learning methods their transient interplay can be exploited in the laboratory for a faster mapping of the reaction space topology. For instance, synchronous analysis during unsteady-state operation can enable quicker, data-rich exploration by comparison to steady-state experimentation (10.1039/d1re00350j). On the other hand, Bayesian based self-optimization of gas–liquid–solid multiphase reactions can help decipher the influence of multivariate conditions for exceptionally complicated reactions, and with improved computational performance over conventional optimization algorithms (10.1039/d1re00397f). For multiphase gas–liquid or liquid–liquid reactions, where the mass transfer rate influences the process, often it is difficult to have an estimate of intrinsic rates and using lumped models tend to bring more empiricism than accuracy as the scale of production increases. Digitalizing such processes with an accurate estimate of all limiting parameters helps reduce the downtime or test time to wait for the desired steady state operations even for sensitive cases where multiple steady states are expected.
This themed issue highlights investigations that have the potential to impact applications across the reaction engineering discipline. Reactions of longstanding industrial significance, such as the Fischer–Tropsch (10.1039/d1re00351h), or multiphase nanomaterials syntheses and their designs (10.1039/d1re00247c) can be explored for improved manufacturing efficiencies. Generally speaking, benchtop digitalization integrated with chemical process design, e.g., manufacturing with renewable feedstocks such as biomass (10.1039/d1re00560j), is a step towards more sustainable chemicals manufacturing and better life-cycle assessments. We would like to thank all authors for their remarkable work, with each contributing insightful concepts that are foundations to the emerging field of digitalization in reaction engineering.
References
-
IBM, What is Industry 4.0?, 2022, Available from: https://www.ibm.com/topics/industry-4-0 Search PubMed.
- V. Venkatasubramanian, The promise of artificial intelligence in chemical engineering: Is it here, finally?, AIChE J., 2019, 65(2), 466–478 CrossRef CAS.
- S. Yang,
et al., Hybrid Modeling in the Era of Smart Manufacturing, Comput. Chem. Eng., 2020, 140, 106874 CrossRef CAS.
- A. Thakkar,
et al., Artificial intelligence and automation in computer aided synthesis planning, React. Chem. Eng., 2021, 6(1), 27–51 RSC.
- S. J. Qin and L. H. Chiang, Advances and opportunities in machine learning for process data analytics, Comput. Chem. Eng., 2019, 126, 465–473 CrossRef CAS.
- A. Bamberg,
et al., The Digital Twin - Your Ingenious Companion for Process Engineering and Smart Production, Chem. Eng. Technol., 2021, 44(6), 954–961 CrossRef CAS.
- C&EN Staff, C&EN's World Chemical Outlook 2022, Chem. Eng. News, 2022, 100(2) Search PubMed
https://cen.acs.org/magazine/100/10002.html
.
-
C. Council
Chemical Industry, 2022, Available from: https://ukchemistrygrowth.com/chemistry/ Search PubMed.
- G. Donati and R. Paludetto, Scale up of chemical reactors, Catal. Today, 1997, 34(3), 483–533 CrossRef CAS.
|
This journal is © The Royal Society of Chemistry 2022 |
Click here to see how this site uses Cookies. View our privacy policy here.