DOI:
10.1039/D3AY00460K
(Paper)
Anal. Methods, 2023,
15, 2925-2934
Advantages of using biologically generated 13C-labelled multiple internal standards for stable isotope-assisted LC-MS-based lipidomics†
Received
27th March 2023
, Accepted 31st May 2023
First published on 6th June 2023
Abstract
In comprehensive lipidomics studies, accurate quantification is essential but biological and/or clinical relevance is often hindered due to unwanted variations such as lipid degradation during sample preparation, matrix effects and non-linear responses of analytical instruments. In addition, the wide chemical diversity of lipids can complicate the accurate identification of individual lipids. These analytical limitations can potentially be corrected efficiently by the use of lipid-specific isotopically labelled internal standards (IS) but currently such IS mixtures have limited coverage of the mammalian lipidome. In this study, an in vivo13C labelling strategy was employed to explore four species (Escherichia coli, Arthrospira platensis, Saccharomyces cerevisiae and Pichia pastoris) as a source of 13C-labelled internal standards (13C-ISs) for more accurate and quantitative liquid chromatography (LC)-mass spectrometry (MS)-based lipidomics. Results showed that extracts from 13C-labelled P. pastoris and S. cerevisiae contain the highest percentage of uniformly labelled lipids (both 83% compared to 67% and 69% in A. platensis and E. coli, respectively) and 13C-labelled P. pastoris extract was identified as the optimum source of 13C-ISs for comprehensive data normalisation to correct unwanted variations during sample preparation and LC-MS analysis. Overall, use of a biologically generated 13C-IS lipid mixture of 357 identified lipid ions resulted in significant reduction in the lipid CV% of normalisation compared with other normalisation methods using total ion counts or a commercially available deuterated internal standard mixture. This improved normalisation using 13C-IS was confirmed in a typical lipidomics analysis using a large number of samples (>100+) and long analysis time (>70 h). This study highlights the benefit of an in vivo labelling strategy for reducing technical and analytical variations introduced during sample preparation and analysis in lipidomics studies.
Introduction
Perturbations in lipid turnover and metabolism are associated with several human diseases including diabetes,1 cancer,2 atherosclerosis,3 Alzheimer's,4 depression,5 and cardiovascular diseases.6 Monitoring changes in lipid profiles in biofluids or tissues can provide valuable information to improve our understanding of different pathological conditions and has the potential to guide the therapy more efficiently.7
Among the various analytical platforms that can be used for lipidomics analysis, liquid chromatography-mass spectrometry (LC-MS) has emerged as the most valuable tool to monitor and identify lipid changes8 due to its high sensitivity and resolving power, and excellent repeatability.9,10 However, the variable recovery of lipids from biological samples and unwanted ion suppression/enhancement of MS signals can impact on the quality of quantitative analyses.11 Systematic and random errors due to lipid degradation during sample preparation, and variations in instrumental response in large-batch analysis can also affect the quality of the data leading to high variability in important biological/clinical outcomes such as biomarker discovery.12 The development of a reliable normalisation method using isotope dilution mass spectrometry (IDMS) methodology, applicable to the structurally diverse lipid species in a biological sample, would therefore have significant advantages. The use of IDMS can also help with the identification of lipids where unambiguous characterisation can be achieved by matching retention time (RT) and fragmentation patterns of product ion spectra with co-extracted IDMS isotopically-labelled internal standards.13
Recently, several research groups have reported the use of isotopically labelled compounds as internal standard (IS) to minimise the matrix effects in lipidomics analysis.14,15 Furman et al.16 biosynthetically produced fifty-four uniformly 13C-labelled (U-13C) arachidonate oxidation products to evaluate the arachidonic acids and their oxidation products in patients with Alzheimer's disease. However, this approach was focused on only oxidised eicosanoids (C20) and hence is of limited value when considering the whole lipidome. Ideally, in IDMS, one isotopically labelled IS for each lipid or lipid species could be used to improve the reliability and accuracy of untargeted lipidomics analysis. However, most lipids are not readily available in isotopically labelled forms and, although chemical synthesis could potentially be used, it is laborious and costly to generate the required diverse and complex structures. To overcome this problem there has been significant recent interest in the biological synthesis of comprehensive mixtures of isotopically labelled IS in vivo for application in lipidomics using microorganisms.17 Potentially, organisms grown on 13C-labelled carbon sources can produce a wide range of isotopically labelled intracellular lipid compounds to be used as a source of IS. Ideally, to obtain a representative set of isotopically labelled compounds as a source of IS, the same organism being studied should also be used to generate the 13C-labelled IS mixture. For more complex metabolisms (e.g., mammalian cells), where it is difficult to produce high labelling efficacy due to slow growth and the need for multiple carbon sources required for survival,18 various microorganisms can be used as a source of IS.19 This approach has been used for quantitative analysis of intra- and extracellular metabolite concentrations in targeted lipidomics studies in which 13C-IS were generated from different organisms such as Escherichia coli,20Penicillium chrysogenum,21Pichia pastoris.22 A preliminary comparison of the E. coli Metabolome Database (ECMDB)23 and Human Metabolome Database (HMDB)24 revealed 376 common lipids between the two databases under the superclass “Lipids and lipid-like molecules” and “Organic compounds” Kingdoms, while 845 metabolites were found to be in common between the Yeast Metabolome Database (YMDB) of S. cerevisiae to the HMDB.24,25 This suggests that yeasts may be more suitable for generating the correct range of isotopically-labelled lipids for use in IDMS-based lipidomics applied to mammalian cells and tissues. To the best of our knowledge, only two lipidomics studies have explored the use of isotopically labelled extracts as IS in untargeted analysis of clinical biological samples.26 The LILY strategy (Lipidome Isotope Labelling of Yeast)17 revealed that in vivo labelling of P. pastoris enabled the production of 212 lipid standards from 13 lipid classes that can be applied for compound specific quantification and/or for class/RT specific quantification in yeast and human plasma samples by isotope dilution. However, the LILY strategy has not been extended yet to other microorganisms belonging to different kingdoms in order to determine which lipids can be covered for human samples. Also, the use of biosynthesized 13C-IS as quality control in untargeted lipidomics studies and their application for batch-to-batch corrections including normalisation of data sets obtained from extended analysis times have not been explored yet. The yeast Pichia pastoris has been widely used to produce biological compounds27 and has been shown to generate similar metabolite and lipid profiles to mammalian cells.17Escherichia coli has been previously used for small-scale production of uniformly 13C-labeled cell extract for quantitative metabolome analysis,28,29 but there are no examples of in vivo generation of 13C-IS to apply in lipidomics study of human samples. Similarly, the lipid profile of Arthrospira platensis and Saccharomyces cerevisiae have not been explored as a source of 13C-labelled lipids IS in complex human samples. Therefore, this work aims to (1) compare the lipidomes of E. coli, A. platensis, S. cerevisiae and P. pastoris; (2) select the optimal source of labelled IS mixture that can be employed in quantitative lipids analysis of human plasma samples by isotope dilution and (3) to evaluate the full capability of the biosynthesized 13C-ISs in correcting various sample processing and instrumental variations that could arise in typical untargeted lipidomics studies.
Experimental section
Materials
E. coli MG1655 was kindly provided by Dr Nigel Halliday, The Biodiscovery Institute, University of Nottingham (Nottingham, UK). Screened blood plasma (pooled normal, control plasma) was provided by the Nottingham University Hospitals Blood Bank, Queen's Medical Centre Campus, Nottingham University (Nottingham, UK). S. cerevisiae CEN.PK 113-7D and P. pastoris NCYC175 were supplied by EUROSCARF (Oberursel, Germany) and National Collection of Yeast Cultures (Norwich, UK), respectively. A. platensis (spirulina) whole cells (lyophilised powder) unlabelled and labelled (U-13C, 97%) and D-glucose (U-13C6, 99%) were purchased from Cambridge Isotope Laboratory (Massachusetts, USA). SPLASH LipidoMix standard solution was supplied by Avanti (Alabama, USA) and used as a deuterated internal standard (D-IS) mixture that includes all the major lipid classes at ratios similar to those found in human plasma. More details of chemicals and materials used in this study are given in the ESI.†
Growth conditions
To prepare the unlabelled (12C) extracts the studied species were grown as described below. A culture of E. coli was cultured overnight in M9 minimal media (prepared according to Cold Spring Harbor Protocols30). The cultures of S. cerevisiae and P. pastoris were prepared similarly in minimal media according to Verduyn et al.31 Overnight cultures were diluted with 100 mL of minimal media to an optical density (OD600) of 0.1 and grown at 122 rpm at 37 °C (E. coli) and 30 °C (S. cerevisiae and P. pastoris) until they reached the stationary phase. During the method development, filter-sterilised glucose solution was added as the only carbon source to a final concentration of 2% for growing all organisms. The growth rate of the studied species was monitored by measuring the OD600 every 2 h for 36 h (n = 3).
For the 13C uniformly labelled glucose experiments 99% U-13C-glucose was used in minimal media preparation instead of 12C-glucose, the culture conditions were otherwise identical to those used for 12C glucose. The effect of different concentrations (0.5, 1, 1.5%, 2%) of 13C glucose was evaluated for the selected species only.
Biosynthesized 13C-internal standard extraction
For each studied species, three biological replicates of cell culture at early stationary phase were extracted. The cellular lipids were extracted using a chloroform/methanol/water mixture according to the Bligh and Dyer method (details are given in the ESI†).32 The chloroform (lower) phase was dried under vacuum at room temperature and the dried sample was reconstituted using 200 μL of LC-MS grade isopropanol and stored at −80 °C until analysis.
Lipids extraction from plasma samples
Lipids were extracted from 50 μL of plasma with 500 μL of chloroform/methanol (1
:
2) and 100 μL of IS mixture according to the method described by Bligh and Dyer with small modifications given in the ESI.†32 The organic phase was dried under vacuum at room temperature and reconstituted using 100 μL LC-MS grade isopropanol and stored at −80 °C until further analysis.
LC-MS lipidomics analysis
Chromatographic separation was conducted on ACE Excel 2 μm super C18 column (50 × 2.1 mm, pore size 100 Å, Advanced Chromatography Technologies Ltd, Scotland, UK) using a Dionex Ultimate 3000 Series UHPLC system (Thermo Fisher Scientific, Hemel Hempstead, UK). The chromatographic conditions were set according to the method of Haoula et al.33 with some modifications (details of the LC experimental conditions are given in the ESI†).
Mass spectrometry was performed on a hybrid quadrupole Orbitrap Q-Exactive MS (Thermo Fisher Scientific, Hemel Hempstead, UK) acquiring data in full scan ion mode and tandem MS/MS in both positive and negative modes using an electrospray ionisation (ESI) source (full details of the MS experimental conditions are given in the ESI†). The data quality obtained from the LC-MS/MS analysis was assessed by determining the percentage coefficient of variation (CV%) in the mean peak intensities of all peaks present in the quality control (QC) samples using a metabolomics approach proposed by Want et al.34 The software LipidSearch 4.1.16 (MitsuiKnowledge Industry, Tokyo, Japan) was used for lipid identification. The parameters used for lipid identification and alignment across samples are listed in Tables S1 and S2 of the ESI.† Tox-ID software (Thermo Fisher Scientific, USA) was used to assess the labelling efficacy of the 13C labelling strategy.
Optimisation of the 13C IDMS internal standard source
The extracted lipids from different species (E. coli, S. cerevisiae and P. pastoris) were blended in different ratios to evaluate their suitability for use in normalisation studies where a uniform coverage across the major lipid classes is desirable. The effect of different concentrations (0.5–2.0%) of uniformly labelled 13C glucose was evaluated on the yield of labelled lipids. Three biological replicates of each condition were prepared and the OD600 were recorded each 2 h for 48 h. Samples at 36 h were taken at each 13C glucose concentration (n = 3) and their extracts were analysed using untargeted LC-MS to establish a comprehensive lipid profile and relative levels of lipids for each concentration of 13C glucose.
Normalisation methods applied to human plasma sample extracts
For normalisation by total ion current (TIC), ions detected in positive and negative modes were normalised by dividing their peak area by the TIC value corresponding to that mode. To normalise lipid peaks by 13C-IS, lipids ions were normalised by dividing their peak area by the peak area of the U-13C-labelled internal standard form. For normalisation by the deuterated internal standard (D-IS) mixture (SPLASH), 20 μL of the prepared solution was added and the detected peak area of the deuterated ions was used to normalise ions detected in the same class (D-IS masses used for both positive and negative mode are listed in Table S3†). When one lipid class was not detected or was not included in the SPLASH solution, a deuterated ion from other similar class was used for normalisation. The Friedman test was used to assess the difference between different normalisation methods.35 MetaboAnalyst was used to perform unsupervised principal component analysis (PCA) when applicable to evaluate the similarity between data sets and to assess the impact of different normalisation methods on the expected variation in the analysis.36
Initial investigation of lipidomics normalisation by 13C-IS mixture on human and murine plasma samples.
The effect of normalisation by 13C-IS mixture on the lipid profiles of extracted plasma samples was evaluated. Briefly, control (blank) plasma samples (50 μL, n = 6) (three sets of samples were used: human plasma, mouse plasma and pooled human plasma) were extracted in the presence of labelled yeast extract (13C-IS, 100 μL) (1
:
2 ratio) and of SPLASH® (20 μL). The pooled human plasma was used to reduce the effect of inter-sample variations so that effects of the normalisation could be assessed on sample extraction and instrumental variations (technical variations). Only lipid ions detected in three or more replicates from the same group were included in the analysis. These unlabelled identified ions were then normalised by TIC, 13C-IS or deuterated lipids from the SPLASH solution and compared to non-normalised data.
The effect of normalisation by 13C-IS on a large set of samples with long analysis time.
The effect of normalisation in reducing analytical and technical variations was evaluated. 101 aliquots from a well-mixed, pooled human plasma sample were extracted following addition of 13C-IS to each aliquot as previously described. To test the ability of biosynthesized 13C-IS in reducing variations that could be introduced during long samples analysis, 230 injections of a pooled extract of human plasma samples were analysed over a 77 h analysis time. The ability of biosynthesized 13C-IS in reducing instrumental day to day variations was assessed using 15 replicate injections of a pooled extract of human plasma analysed on three different days (day 0, 7 and 14). Three replicates from pooled plasma were extracted without 13C-IS and used for lipid identification. Only unlabelled ions whose 13C-labelled form was detected in all replicates were included in the analysis (compound-specific normalisation).
Results and discussion
Evaluation of 12C lipid profiles in different species as a potential source of 13C-IS for LC-HRMS based lipidomics
Table 1 represents the total number of lipids in common with HMDB for E. coli, A. platensis, S. cerevisiae and P. pastoris, identified by LipidSearch. Fig. S1 of ESI† represents examples of total ion current (TIC) chromatograms in ESI negative and positive modes and pie charts of the main classes of lipid molecules identified from the four microorganisms.
Table 1 Total number of lipids extracted from each strain and identified by LipidSearch in positive and negative mode that are in common to HMDB
Total identified lipids compared to HMDB |
P. pastoris
|
S. cerevisiae
|
A. platensis
|
E. coli
|
345 |
153 |
129 |
176 |
n (%) |
DG |
50 (14) |
26 (17) |
32 (25) |
73 (42) |
MG |
13 (4) |
9 (6) |
7 (6) |
27 (15) |
PC |
49 (14) |
26 (17) |
16 (13) |
— |
PE |
39 (11) |
37 (24) |
35 (27) |
44 (25) |
PS |
23 (7) |
11 (7) |
— |
4 (2) |
PG |
9 (3) |
3 (2) |
18 (14) |
9 (5) |
TG |
112 (32) |
15 (10) |
7 (5) |
6 (4) |
PI |
6 (2) |
5 (3) |
3 (2) |
— |
LPC |
10 (3) |
7 (5) |
— |
— |
Cer |
12 (4) |
2 (1) |
3 (2) |
4 (2) |
Other |
22 (6) |
12 (8) |
8 (6) |
9 (5) |
Table 1 shows that diacylglycerols (DG), phosphatidylethanolamines (PE) and monoacylglycerols (MG) represented the main classes of lipids detected in the four microorganisms. The two prokaryotic microorganisms E. coli and A. platensis had qualitative and quantitative differences in lipids profiles compared with the two eukaryotes, P. pastoris and S. cerevisiae. In terms of the total coverage of the human lipidome, P. pastoris was significantly better than the other microorganisms with over 300 human metabolome database (HMDB) lipids identified.
For E. coli, although preliminary comparison between the E. coli metabolome database (ECMDB) and the HMDB revealed 376 common lipids, only 176 were actually found and this could be due to different extraction solvents and analytical methods used for the lipid data in HMDB and ECMDB. The lower number of detected lipid ions in A. platensis could be attributed to the fact that the extracted sample was small (5 mg) but this number and its lack of coverage of the relevant mammalian classes of lipids make it less suitable for generating 13C lipids.
Both P. pastoris and S. cerevisiae revealed a wider range of lipid classes compared to E. coli and A. platensis with the presence of phosphatidylserines (PS), phosphatidylinositols (PI) and ceramides (Cer) increasing the relevance for mammalian lipid analysis. Although the database research indicated a high number of lipid-like molecules (845) in common between S. cerevisiae and human samples, experimental results showed that only 153 lipid ions were in common when HMDB data were compared to S. cerevisiae lipid profile (Table 1). However, in the P. pastoris extract, 345 lipids were found in common with the HMDB. Triacylglycerols (TG, 32%), DG (14%), PC (14%), PE (11%) and PS (7%) were the most predominant lipid ions in common between P. pastoris extract and human metabolome similar to previously reported data.17,26,37 TG represent more than one third of the identified lipids in P. pastoris extract, a much higher proportion than detected in S. cerevisiae, and this agrees with previously published data.37
Optimisation of biologically generated 13C-IS mixture to use for normalisation in lipidomics studies
Biologically generated 13C IS extracts from different species were mixed and compared. The results are summarised in Table 2 and Fig. S5.† The number of lipid ions identified using LipidSearch™ to analyse LC-MS data from the combined extracts of (a) P. pastoris and S. cerevisiae, and (b) E. coli, P. pastoris and S. cerevisiae increased compared to the single species extract. However, the number of the detected labelled ions in P. pastoris alone was comparable to other mixture samples (Table 2).
Table 2 Comparison between different mixtures of E. coli, S. cerevisiae and P. pastoris to find out the optimal source/sources of IS mixture (n = 3)a
Parameters |
E. coli
|
S. cerevisiae
|
P. pastoris
|
ES |
EP |
PS |
EPS |
ES = E. coli and S. cerevisiae extract mixture. EP = E. coli and P. pastoris extract mixture. PS = P. pastoris and S. cerevisiae extract mixture. EPS = E. coli, P. pastoris and S. cerevisiae extract mixture.
|
Number of identified lipid ions by LipidSearch™ (mean ± SD) |
187 ± 2 |
313 ± 8 |
401 ± 13 |
334 ± 3 |
379 ± 15 |
409 ± 7 |
418 ± 8 |
Numbers of detected ions by Tox-ID (mean ± SD) |
134 ± 2 |
253 ± 3 |
356 ± 3 |
268 ± 1 |
325 ± 4 |
383 ± 24 |
311 ± 29 |
Number of lipid ions with 13C-enrichment ≥99% (mean ± SD) |
121 ± 2 |
206 ± 6 |
313 ± 4 |
223 ± 2 |
288 ± 4 |
334 ± 16 |
279 ± 20 |
This could be due to ion suppression during analysis as highly abundant ions from different species could suppress co-eluted low abundant ions or because of the similarity of detected lipids between the samples.38 Although a mixture of the extracts from P. pastoris and S. cerevisiae could provide a small increase in the number of 13C labelled ions (Table 3), the high cost of growing and co-extracting S. cerevisiae and P. pastoris makes this procedure less worthwhile for a comprehensive source of 13C IS in the future quantitative lipidomics studies.
Table 3 The % of 13C-enrichment in detected lipid ions extracted from A. platensis, E. coli, P. pastoris, and S. cerevisiae. The detected lipids are divided in three sets based on the 13C-enrichment: unlabelled lipids (0% 13C-enrichment), partially labelled lipids (1–99% 13C-enrichment) and fully labelled lipids (>99% 13C-enrichment)
Species |
13C-enrichment in detected lipids |
0% |
1–99% |
>99% |
Arthrospira platensis
|
20% |
13% |
67% |
Escherichia coli
|
25% |
6% |
69% |
Pichia pastoris
|
10% |
7% |
83% |
Saccharomyces cerevisiae
|
9% |
8% |
83% |
The 13C enrichment detected (Table 3) shows that prokaryotic and eukaryotic microorganisms were again different in terms of the % incorporation of 13C during growth with 13C glucose as the sole carbon source. Both P. pastoris, and S. cerevisiae showed a notably higher level of highly enriched lipids than A. platensis and E. coli.
The higher percentage of unlabelled (0%) and partially labelled (1–99%) lipid ions in E. coli (31%) and A. platensis (33%) compared with P. pastoris and S. cerevisiae has potential to increase the complexity of the sample matrix. Additionally, the cost and limited isotopic purity (97%) of the commercially available 13C-labelled A. platensis powder limited its use as a source of 13C ISs. Partly 13C enriched lipids (1–99%, Table 3), despite increasing the complexity of the sample matrix, are unlikely to interfere with quantitation, since there will be no spectral overlays with the corresponding unlabelled analytes. This is because of the mass increment of the additional 1.00335 amu per 13C carbon present in the lipid backbone, introducing a significant mass shift when compared to the unlabelled analyte which can be readily detected by accurate mass spectrometry. The presence of unlabelled (12C) lipids in the 13C mixture presents a potential problem with quantification, and a correction factor might need to be applied depending on the type of study and quantification carried out. However, in a study where two or more groups are compared, the presence of unlabelled lipids will not hinder the relative quantification because an equal amount of 13C IS mixture will be added to all samples.
Since significantly more HMDB lipids were identified from P. pastoris compared to the other microorganisms, and more lipids were found to be fully 13C labelled in P. pastoris (83%) this single species was selected as the best source of biologically generated 13C-ISs. Another factor in favour of P. pastoris was that this species reached a much higher culture OD than E. coli and S. cerevisiae (Fig. S2–S4†) indicating that more cells can be grown and therefore potentially a higher concentration of 13C lipids/IS mixture can be extracted. Therefore, P. pastoris was selected as the optimal source of 13C ISs among the studied species.
For further analytical investigation and different uniformly labelled glucose concentrations were tested for cell growth with P. pastoris. Fig. 1 shows the growth rate of P. pastoris at four different concentrations of U-13C glucose (0.5, 1, 1.5 and 2%).
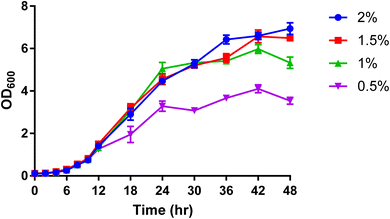 |
| Fig. 1 The effect of U-13C glucose concentration (0.5%, 1%, 1.5% and 2%) on the growth rate of P. pastoris based on the biomass production measured by the optical density at 600 nm (n = 3). The levels of 39 representative lipids identified from different lipid classes was monitored as shown in Fig. 2. Although data showed a significantly lower biomass production with 0.5 and 1% of 13C-glucose, the TIC, the number of detected features and the level of selected lipids from the extract of cells grown at 1% 13C-glucose were comparable to the extract of cells grown at 2% 13C-glucose. Given that a good coverage of 13C lipids was obtained with 1% glucose, the small advantage in 13C lipid generation using 1.5% or 2% 13C glucose (see Fig. 2) was not justified given the significant extra cost involved. Thus, optimal growth conditions for P. pastoris in minimal media was confirmed as 1% of the final 13C-labelled glucose concentration for 24 h and the 13C IS standards generated were used in all the subsequent plasma lipidomic analyses reported below. | |
 |
| Fig. 2 Heatmap representing the effect of glucose concentration on the level of 39 putatively-identified lipids detected in P. pastoris extract based on two-way ANOVA between different glucose concentration compared to 2% concentration. Equal samples from each of the four conditions were collected after 36 h of cultivation (early stationary phase) and the lipid contents of 39 lipids previously putatively identified were explored. *, **, ***, **** represent p-values of ≤0.05, ≤0.01, ≤0.001, and ≤0.0001, respectively. The numbers in the brackets on the right side of the figure represents the condition that is statistically different from 2% based on two-way ANOVA analysis per lipid. | |
Characterisation and isotopic purity of 13C-lipids generated by P. pastoris grown with U-13C glucose
Table S4† shows the 357 lipid ions identified in P. pastoris extract based on accurate fragmentation patterns by LipidSearch™. The detected lipids cover a wide range of lipid classes including fatty acyls (FA), (O-acyl)-ω-hydroxy fatty acids (OAHFA), glycerolipids (MG, DG, TG), glycerophospholipids (cardiolipins (CL), dimethylphosphatidylethanolamines (dMePE), lysophosphatidylcholines (LPC), lysophosphatidylethanolamines (LPE), phosphatidic acids (PA), PC, PE, PG, PI and PS), sphingolipids (Cer, glucosylceramides (CerG1), sphingomyelins (SM) and sphingosine (SO)) in accordance with previously reported P. pastoris studies.17,26 The MS/MS spectra obtained from unlabelled and labelled extracts (Fig. S6†) clearly indicate a mass shift equivalent to 1.00335 Da increment per carbon atom present in the backbone compared with identified unlabelled (12C) lipid ions. The retention times of 246 13C-labelled ions detected in P. pastoris extract matched (within ±15 s) with those of the equivalent 12C ions. Furthermore, their detected monoisotopic masses were in agreement with the calculated 13C-masses within a mass error of ±5 ppm, in agreement with Rampler et al.17 The labelling patterns of these ions revealed 13C labelling degree of ≥98% (Table S4 of ESI†). Exploring the labelling pattern of identified ions revealed that the other ions were either not labelled or partially labelled. Based on the information on the generated 13C labelled lipids, those with a mass error of <5 ppm of expected 13C-fully labelled mass detected within RT window of 30 s of unlabelled identified lipid were selected for use in the normalisation studies reported below.
Comparison of three lipidomics normalisation methods using biosynthesized 13C-lipids, total ion count or SPLASH applied to small sample sets of human and mouse plasma
Compound-specific normalisation using 13C labelled IS mixture from labelled P. pastoris extract was performed on three sets of plasma samples: six biologically different fasting human plasma samples, six biologically different fasting mice plasma samples and a pooled fasting human plasma sample. The results of the 13C IS normalisation were compared to normalisation by TIC and SPLASH deuterated IS mixture.
Fig. S7† represents the result of compound-specific normalisation of ions (n = 112) detected in human plasma samples (Fig. S7A†) and ions (n = 132) detected in mouse plasma samples (Fig. S7B†) extracted in the presence of biosynthesized 13C-IS. It appears that normalisation by TIC improves the overall response and an improved CV% was obtained for most of the selected ions. However, we observed that normalisation by 13C-IS or by SPLASH increased the CV% of the selected ions. This was an unexpected result since the introduced 13C-IS mixture was expected to correct analytical and technical variation. To further investigate the efficacy of the introduced normalisation methods we used replicate analysis of a well-mixed pooled human plasma, where the biological variability between samples has been eliminated. Fig. S7C† represents the result of compound-specific normalisation detected in pooled plasma samples extracted in the presence of 13C-IS. Statistical analysis showed a significant increase in the CV% of selected ions after normalisation by all the methods used, suggesting that normalisation had increased rather than decreased inter-sample variation. These unexpected results can be explained by the relatively small set of samples used (n = 6) compared to a typical lipidomics experiment which would have a much larger set of samples. Therefore, in the light of these results, the effect of 13C-IS normalisation was further evaluated in an experiment with a larger number of samples and higher inter-individual differences between samples where the level of random error is expected to increase and affect the quality of the data.
Comparison of lipidomics normalisation using biosynthesized 13C-lipids and total ion count applied to a large set of samples and 77 h analysis time
The effect of normalisation by 13C-IS in reducing variations introduced during the extraction of a large set of human plasma samples (n = 101) was evaluated. A total of 446 ions were identified in the pooled human QC sample and 142 labelled ions were present in all samples and used for compound-specific normalisation. Fig. 3(A) represents the normalisation results of these ions. As shown, ions normalised by TIC or by 13C-IS showed a significant reduction in CV% compared to that in the raw data. Ions normalised by 13C-IS gave an average CV% of 6.36% compared with those non-normalised data which gave an average of 11.01%. This highlights the efficacy of normalisation by 13C-IS mixture in reducing variations caused during samples preparation especially when a typical size of sample set (101 in this case) is analysed.
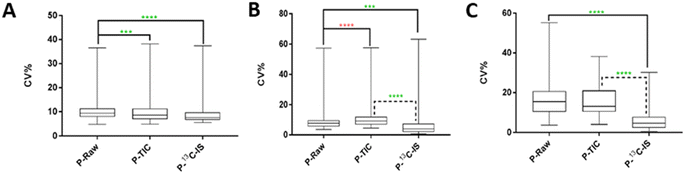 |
| Fig. 3 Coefficient of variance distributions for normalization of (A) pooled human plasma sample repeatably extracted (n = 101) to study the effect of normalization by 13C-IS in reducing variations introduced during extraction of large set of samples; (B) pooled human plasma sample repeatably injected and analyzed (n = 230) to study the effect of normalization by 13C-IS in reducing variations introduced during sample analysis over a long analysis time; (C) pooled human plasma sample extracted and analyzed (n = 15) at different days (day 0, day 7 and day 14) to study the effect of normalization by 13C-IS in reducing variations introduced during sample analysis on three separate days (batch to batch variations). (****p < 0.0001, ***p < 0.001, where the green color indicates a reduction in overall CV% while the red color indicates an increase in overall CV%, while the lack of comparison between the raw and normalized data indicates no significance). | |
The effect of normalisation by 13C-IS was further investigated for its ability to correct unwanted variations introduced during long LC-MS analysis (n = 230, total analysis time 77 hours). Using the same procedure as for the previous experiment. 100 labelled ions identified in all samples were used for compound-specific normalisation. In this experiment, the calculated CV% shown in Fig. 3(B) reflects variations introduced during sample analytical variations over 77 hours. In metabolomics the analysis of a large set of samples, for example in cohort studies or samples measured at different time points, is a challenge due to analytical drift caused by metabolite degradation (oxidation or hydrolysis) and/or inconsistency in LC and MS instrumentation (RT, peak shape or variation in MS detector response). Although randomisation of the sample run order is used to reduce the effect of these variations, it cannot be completely removed or compensated without the use of IS. As can be seen in Fig. 3(B), ions normalised by 13C-IS revealed a significantly lower overall CV%, compared to those in raw data (p < 0.001) and normalised by TIC (p < 0.0001). In Fig. 3(A) and (B), analytical drifts in instrumental responses were observed over time, leading to a clear separation between injections analysed over 77 hours in PCA scores plot. Similar drifts in QC samples were observed in a large-scale analysis of serum and plasma samples,39 and in urine samples40 due to sample instability or unexpected instrumental hardware or software errors. Normalisation by TIC still showed significant variations in instrument responses as can be seen in Fig. 3(A) and (B), whereas normalisation by 13C-IS was able to correct these drifts and remove any difference between injections (Fig. 3(C)).
The effect of normalisation by 13C-IS on data acquired on three separate days was also evaluated. 105 labelled ions were present in all samples and used for compound-specific normalisation. The calculated CV% in raw data shown in Fig. 3(C) reflects variations introduced due to batch-to-batch variations. This type of variation arises from sample handling, preparation and storage, MS performance and column stability over time that collectively affect the results of large-scale studies. It appears that ions normalised by TIC had similar overall CV% compared to that in raw data, whereas ions normalised by 13C-IS had a significantly improved overall CV% (Fig. 3(C)).
Normalisation by 13C-IS can effectively reduce day-to-day variations introduced by the instrument during samples analysis significantly, and this is confirmed by the PCA scores plot where there are clear separations between different batches without normalisation or normalised by TIC (Fig. 4(A), (B), (D) and (E)) but no clusters were observed after normalisation by 13C-IS as shown in Fig. 4(C) and (F).
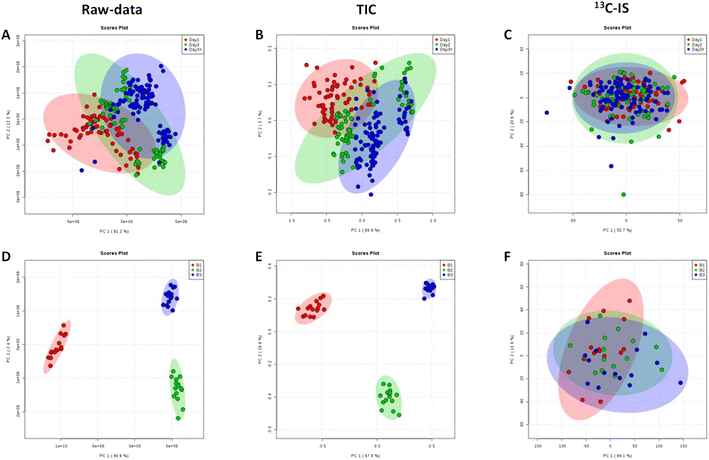 |
| Fig. 4 (A–C) PCA score plots of pooled human plasma sample from blood bank repeatably injected (n = 230) and analysed over 77 hours (red, green and blue colours represent samples run in the first day, the second day and the third day respectively). (D and E) PCA score plots of pooled human plasma samples from blood bank extracted and analysed (n = 15) at different days (red, green and blue colours represent samples analysed at day 0, day 7 and day 14 respectively). The PCA show the effect of TIC normalisation (B and E) and compound-specific normalisation by 13C-IS (C and F) compared to raw un-normalised data (A and D). Only common ions between plasma samples and 13C-yeast extract were included in the analysis. | |
Furthermore, in order to identify the main lipid species that contributed to the distinctive PCA groupings in Fig. 4 partial least squares discriminant analysis (PLS-DA) was performed, and a variable importance in projection (VIP) value > 1 was selected as a cut off for lipids that were significantly contributing to the grouping. The 15 lipid species that contributed to additional variability in the raw data were decreased to 10 lipids after normalization with TIC and after 13C-IS normalization only 2 lipid species had a VIP greater than 1 as presented in Table S5.† This confirmed that the 13C-IS normalisation had a direct and specific effect in reducing the excess variation of the dataset.
Conclusion
We confirm that a wide range of 13C-labelled lipids can be biosynthesized using the yeast P. pastoris using straightforward and simple culture conditions. Compared with E. coli, A. platensis, S. cerevisiae, the P. pastoris lipid profile provided the best match to the lipid profile of human plasma. Using the 13C-labelled IS mixture from P. pastoris in typical lipidomics experiments resulted in a significant reduction of unwanted variations due to sample processing and LC-MS analysis, especially where samples are analysed over several days or in separate batches. Furthermore, this study demonstrates that the in vivo labelling strategy provides a cost-effective and practical solution to perform reliable untargeted lipidomics, avoiding expensive or difficult-to-obtain commercial lipid standards. In our work we used a single batch of the biosynthesized 13C-labelled IS mixture, which provided enough material for many hundreds of individual samples. Clearly, there may be batch-to-batch variability in any biosynthesized lipid mixture, so it is important to generate sufficient material to cover the full requirements of any extended lipidomics study. Finally, this normalisation technique could be readily transferred to a variety of biological sample types such as mammalian tissue or other biofluid samples.
Conflicts of interest
The authors declare no competing financial interest.
Acknowledgements
We are grateful to Prof. Simon Avery, Faculty of Medicine & Health Sciences (University of Nottingham), for his valuable input in the selection of yeast species for investigation. MAJ thanks the University of Petra for scholarship funding of her PhD at the University of Nottingham.
References
- S. Eid, K. M. Sas, S. F. Abcouwer, E. L. Feldman, T. W. Gardner, S. Pennathur and P. E. Fort, New insights into the mechanisms of diabetic complications: role of lipids and lipid metabolism, Diabetologia, 2019, 62(9), 1539–1549 CrossRef PubMed.
- A. Pakiet, J. Kobiela, P. Stepnowski, T. Sledzinski and A. Mika, Changes in lipids composition and metabolism in colorectal cancer: a review, Lipids Health Dis., 2019, 18(1), 29 CrossRef PubMed.
- A. Pirillo, F. Bonacina, G. D. Norata and A. L. Catapano, The Interplay of Lipids, Lipoproteins, and Immunity in Atherosclerosis, Curr. Atheroscler. Rep., 2018, 20(3), 12 CrossRef PubMed.
- M. W. Wong, N. Braidy, A. Poljak, R. Pickford, M. Thambisetty and P. S. Sachdev, Dysregulation of lipids in Alzheimer's disease and their role as potential biomarkers, Alzheimer's & Dementia, 2017, 13(7), 810–827 Search PubMed.
- A. Parekh, D. Smeeth, Y. Milner and S. Thure, The Role of Lipid Biomarkers in Major Depression, Healthcare, 2017, 5(1), 5 CrossRef PubMed.
- A. D. Watson, Thematic review series: systems biology approaches to metabolic and cardiovascular disorders. Lipidomics: a global approach to lipid analysis in biological systems, J. Lipid Res., 2006, 47(10), 2101–2111 CrossRef CAS PubMed.
- G. Hussain, H. Anwar, A. Rasul, A. Imran, M. Qasim, S. Zafar, M. Imran, S. K. S. Kamran, N. Aziz, A. Razzaq, W. Ahmad, A. Shabbir, J. Iqbal, S. M. Baig, M. Ali, J. L. Gonzalez de Aguilar, T. Sun, A. Muhammad and A. Muhammad Umair, Lipids as biomarkers of brain disorders, Crit. Rev. Food Sci. Nutr., 2020, 60(3), 351–374 CrossRef CAS PubMed.
- M. Lange, Z. Ni, A. Criscuolo and M. Fedorova, Liquid chromatography techniques in lipidomics research, Chromatographia, 2019, 82(1), 77–100 CrossRef CAS.
- Y. Chen, Z. Ma, X. Shen, L. Li, J. Zhong, L. S. Min, L. Xu, H. Li, J. Zhang and L. Dai, Serum Lipidomics Profiling to Identify Biomarkers for Non-Small Cell Lung Cancer, BioMed Res. Int., 2018, 2018, 5276240 Search PubMed.
- K. Huynh, C. K. Barlow, K. S. Jayawardana, J. M. Weir, N. A. Mellett, M. Cinel, D. J. Magliano, J. E. Shaw, B. G. Drew and P. J. Meikle, High-Throughput Plasma Lipidomics: Detailed Mapping of the Associations with Cardiometabolic Risk Factors, Cell Chem. Biol., 2019, 26(1), 71–84 CrossRef CAS PubMed.
- F. Gosetti, E. Mazzucco, D. Zampieri and M. C. Gennaro, Signal suppression/enhancement in high-performance liquid chromatography tandem mass spectrometry, J. Chromatogr. A, 2010, 1217(25), 3929–3937 CrossRef CAS PubMed.
- D. Broadhurst, R. Goodacre, S. N. Reinke, J. Kuligowski, I. D. Wilson, M. R. Lewis and W. B. Dunn, Guidelines and considerations for the use of system suitability and quality control samples in mass spectrometry assays applied in untargeted clinical metabolomic studies, Metabolomics, 2018, 14(6), 72 CrossRef PubMed.
- H. G. Gika, G. A. Theodoridis, R. S. Plumb and I. D. Wilson, Current practice of liquid chromatography-mass spectrometry in metabolomics and metabonomics, J. Pharm. Biomed. Anal., 2014, 87, 12–25 CrossRef CAS PubMed.
- W. Zhou, S. Yang and P. G. Wang, Matrix effects and application of matrix effect factor, Bioanalysis, 2017, 9(23), 1839–1844 CrossRef CAS PubMed.
- D. Chen, W. Han, X. Su, L. Li and L. Li, Overcoming Sample Matrix Effect in Quantitative Blood Metabolomics Using Chemical Isotope Labeling Liquid Chromatography Mass Spectrometry, Anal. Chem., 2017, 89(17), 9424–9431 CrossRef CAS PubMed.
- R. Furman, J. V. Lee and P. H. Axelsen, Analysis of eicosanoid oxidation products in Alzheimer brain by LC-MS with uniformly 13C-labeled internal standards, Free Radical Biol. Med., 2018, 118, 108–118 CrossRef CAS PubMed.
- E. Rampler, C. Coman, G. Hermann, A. Sickmann, R. Ahrends and G. Koellensperger, LILY-lipidome isotope labeling of yeast: in vivo synthesis of (13)C labeled reference lipids for quantification by mass spectrometry, Analyst, 2017, 142(11), 1891–1899 RSC.
- N. Grankvist, J. D. Watrous, K. A. Lagerborg, Y. Lyutvinskiy, M. Jain and R. Nilsson, Profiling the Metabolism of Human Cells by Deep (13)C Labeling, Cell Chem. Biol., 2018, 25(11), 1419–1427 CrossRef CAS PubMed.
- S. Schatschneider, S. Abdelrazig, L. Safo, A. M. Henstra, T. Millat, D. H. Kim, K. Winzer, N. P. Minton and D. A. Barrett, Quantitative Isotope-Dilution High-Resolution-Mass-Spectrometry Analysis of Multiple Intracellular Metabolites in Clostridium autoethanogenum with Uniformly (13)C-Labeled Standards Derived from Spirulina, Anal. Chem., 2018, 90(7), 4470–4477 CrossRef CAS PubMed.
- D. H. Kim, F. Achcar, R. Breitling, K. E. Burgess and M. P. Barrett, LC-MS-based absolute metabolite quantification: application to metabolic flux measurement in trypanosomes, Metabolomics, 2015, 11(6), 1721–1732 CrossRef CAS PubMed.
- G. Wang, J. Chu, Y. Zhuang, W. van Gulik and H. Noorman, A dynamic model-based preparation of uniformly-(13)C-labeled internal standards facilitates quantitative metabolomics analysis of Penicillium chrysogenum, J. Biotechnol., 2019, 299, 21–31 CrossRef CAS PubMed.
- E. Rampler, G. Hermann, G. Grabmann, Y. El Abiead, H. Schoeny, C. Baumgartinger, T. Köcher and G. Koellensperger, Benchmarking Non-Targeted Metabolomics Using Yeast-Derived Libraries, Metabolites, 2021, 11(3), 160 CrossRef CAS PubMed.
- A. C. Guo, T. Jewison, M. Wilson, Y. Liu, C. Knox, Y. Djoumbou, P. Lo, R. Mandal, R. Krishnamurthy and D. S. Wishart, ECMDB: the E. coli Metabolome Database, Nucleic Acids Res., 2013, 41(database issue), D625–D630 CAS.
- D. S. Wishart, C. Knox, A. C. Guo, R. Eisner, N. Young, B. Gautam, D. D. Hau, N. Psychogios, E. Dong, S. Bouatra, R. Mandal, I. Sinelnikov, J. Xia, L. Jia, J. A. Cruz, E. Lim, C. A. Sobsey, S. Shrivastava, P. Huang, P. Liu, L. Fang, J. Peng, R. Fradette, D. Cheng, D. Tzur, M. Clements, A. Lewis, A. De Souza, A. Zuniga, M. Dawe, Y. Xiong, D. Clive, R. Greiner, A. Nazyrova, R. Shaykhutdinov, L. Li, H. J. Vogel and I. Forsythe, HMDB: a knowledgebase for the human metabolome, Nucleic Acids Res., 2009, 37(database issue), D603–D610 CrossRef CAS PubMed.
- T. Jewison, C. Knox, V. Neveu, Y. Djoumbou, A. C. Guo, J. Lee, P. Liu, R. Mandal, R. Krishnamurthy, I. Sinelnikov, M. Wilson and D. S. Wishart, YMDB: The Yeast Metabolome Database, Nucleic Acids Res., 2012, 40(database issue), D815–D820 CrossRef CAS PubMed.
- E. Rampler, A. Criscuolo, M. Zeller, Y. El Abiead, H. Schoeny, G. Hermann, E. Sokol, K. Cook, D. A. Peake, B. Delanghe and G. Koellensperger, A Novel Lipidomics Workflow for Improved Human Plasma Identification and Quantification Using RPLC-MSn Methods and Isotope Dilution Strategies, Anal. Chem., 2018, 90(11), 6494–6501 CrossRef CAS PubMed.
- M. Tomas-Gamisans, P. Ferrer and J. Albiol, Integration and Validation of the Genome-Scale Metabolic Models of Pichia pastoris: A Comprehensive Update of Protein Glycosylation Pathways, Lipid and Energy Metabolism, PLoS One, 2016, 11(1), e0148031 CrossRef PubMed.
- M. Weiner, J. Trondle, A. Schmideder, C. Albermann, K. Binder, G. A. Sprenger and D. Weuster-Botz, Parallelized small-scale production of uniformly (13)C-labeled cell extract for quantitative metabolome analysis, Anal. Biochem., 2015, 478, 134–140 CrossRef CAS PubMed.
- B. D. Bennett, E. H. Kimball, M. Gao, R. Osterhout, S. J. Van Dien and J. D. Rabinowitz, Absolute metabolite concentrations and implied enzyme active site occupancy in Escherichia coli, Nat. Chem. Biol., 2009, 5(8), 593–599 CrossRef CAS PubMed.
-
CSH, M9 recipe, in Cold Spring Harbor Protocols, 2006, DOI:10.1101/pdb.rec8146.
- C. Verduyn, E. Postma, W. A. Scheffers and J. P. Van Dijken, Effect of benzoic acid on metabolic fluxes in yeasts: a continuous-culture study on the regulation of respiration and alcoholic fermentation, Yeast, 1992, 8(7), 501–517 CrossRef CAS PubMed.
- E. G. Bligh and W. J. Dyer, A rapid method of total lipid extraction and purification, Can. J. Biochem. Physiol., 1959, 37(8), 911–917 CrossRef CAS PubMed.
- Z. Haoula, S. Ravipati, D. J. Stekel, C. A. Ortori, C. Hodgman, C. Daykin, N. Raine-Fenning, D. A. Barrett and W. Atiomo, Lipidomic analysis of plasma samples from women with polycystic ovary syndrome, Metabolomics, 2015, 11(3), 657–666 CrossRef CAS PubMed.
- E. J. Want, I. D. Wilson, H. Gika, G. Theodoridis, R. S. Plumb, J. Shockcor, E. Holmes and J. K. Nicholson, Global metabolic profiling procedures for urine using UPLC-MS, Nat. Protoc., 2010, 5(6), 1005–1018 CrossRef CAS PubMed.
- M. Vinaixa, S. Samino, I. Saez, J. Duran, J. J. Guinovart and O. Yanes, A Guideline to Univariate Statistical Analysis for LC/MS-Based Untargeted Metabolomics-Derived Data, Metabolites, 2012, 2(4), 775–795 CrossRef CAS PubMed.
- J. Chong, O. Soufan, C. Li, I. Caraus, S. Li, G. Bourque, D. S. Wishart and J. Xia, MetaboAnalyst 4.0: towards more transparent and integrative metabolomics analysis, Nucleic Acids Res., 2018, 46(W1), W486–W494 CrossRef CAS PubMed.
- V. A. Ivashov, K. Grillitsch, H. Koefeler, E. Leitner, D. Baeumlisberger, M. Karas and G. Daum, Lipidome and proteome of lipid droplets from the methylotrophic yeast Pichia pastoris, Biochim. Biophys. Acta, 2013, 1831(2), 282–290 CrossRef CAS PubMed.
- C. G. Enke, A predictive model for matrix and analyte effects in electrospray ionization of singly-charged ionic analytes, Anal. Chem., 1997, 69(23), 4885–4893 CrossRef CAS PubMed.
- W. B. Dunn, D. Broadhurst, P. Begley, E. Zelena, S. Francis-McIntyre, N. Anderson, M. Brown, J. D. Knowles, A. Halsall, J. N. Haselden, A. W. Nicholls, I. D. Wilson, D. B. Kell and R. Goodacre, Human serum metabolome, C., procedures for large-scale metabolic profiling of serum and plasma using gas chromatography and liquid chromatography coupled to mass spectrometry, Nat. Protoc., 2011, 6(7), 1060–1083 CrossRef CAS PubMed.
- M. R. Lewis, J. T. Pearce, K. Spagou, M. Green, A. C. Dona, A. H. Yuen, M. David, D. J. Berry, K. Chappell, V. Horneffer-van der Sluis, R. Shaw, S. Lovestone, P. Elliott, J. Shockcor, J. C. Lindon, O. Cloarec, Z. Takats, E. Holmes and J. K. Nicholson, Development and Application of Ultra-Performance Liquid Chromatography-TOF MS for Precision Large Scale Urinary Metabolic Phenotyping, Anal. Chem., 2016, 88(18), 9004–9013 CrossRef CAS PubMed.
Footnotes |
† Electronic supplementary information (ESI) available: Additional experimental details, materials, and methods, including tables and figures. See DOI: https://doi.org/10.1039/d3ay00460k |
‡ Shared first authorship. |
|
This journal is © The Royal Society of Chemistry 2023 |
Click here to see how this site uses Cookies. View our privacy policy here.