DOI:
10.1039/D2NR03727K
(Paper)
Nanoscale, 2022,
14, 13532-13541
Machine learning ensures rapid and precise selection of gold sea-urchin-like nanoparticles for desired light-to-plasmon resonance
Received
7th July 2022
, Accepted 6th August 2022
First published on 8th August 2022
Abstract
Sustainable energy strategies, particularly solar-to-hydrogen production, are anticipated to overcome the global reliance on fossil fuels. Thereby, materials enabling the production of green hydrogen from water and sunlight are continuously designed, e.g., ZnO nanostructures coated by gold sea-urchin-like nanoparticles, which employ the light-to-plasmon resonance to realize photoelectrochemical water splitting. But such light-to-plasmon resonance is strongly impacted by the size, the species, and the concentration of the metal nanoparticles coating on the ZnO nanoflower surfaces. Therefore, a precise prediction of the surface plasmon resonance is crucial to achieving an optimized nanoparticle fabrication of the desired light-to-plasmon resonance. To this end, we synthesized a substantial amount of metal (gold) nanoparticles of different sizes and species, which are further coated on ZnO nanoflowers. Subsequently, we utilized a genetic algorithm neural network (GANN) to obtain the synergistically trained model by considering the light-to-plasmon conversion efficiencies and fabrication parameters, such as multiple metal species, precursor concentrations, surfactant concentrations, linker concentrations, and coating times. In addition, we integrated into the model's training the data of nanoparticles due to their inherent complexity, which manifests the light-to-plasmon conversion efficiency far from the coupling state. Therefore, the trained model can guide us to obtain a rapid and automatic selection of fabrication parameters of the nanoparticles with the anticipated light-to-plasmon resonance, which is more efficient than an empirical selection. The capability of the method achieved in this work furthermore demonstrates a successful projection of the light-to-plasmon conversion efficiency and contributes to an efficient selection of the fabrication parameters leading to the anticipated properties.
Introduction
Global reliance on fossil fuels has led to an energy crisis and urges a sustainable energy strategy, especially solar-to-hydrogen production, thus stimulating substantial efforts in exploring nanoscale materials.1–7 In the nano-dimension, materials can alter their characteristics,8,57–59 such as, the reactivity to light, enabling electron polarization on the metal surface, or facilitating the electron excitation to the conduction band.9–16 Thereby, nano metals and metal-oxides display prominent functions, especially the light-to-plasmon resonance, which can be utilized to optimize the development of solar cells, optical sensors, and gas sensors.17–21 As a typical nano metal–oxide, zinc oxide (ZnO), a conventional n-type semiconductor with a wide bandgap of 3.37 eV at room temperature, has an absorbance peak of 340–370 nm within the ultraviolet range.22–28 In addition to its excellent conductivity, ZnO has outstanding biological compatibility and low toxicity, unlike most photosensitive materials (CdS, PdS, GaSe, and BiSe). Moreover, ZnO, owing to its hexagonal wurtzite crystallinity, exhibits a variety of anisotropic morphologies associated with the different synthesis methods, e.g., nanorods, nanosheets, nanoflower, and nanowires.29–32 However, ZnO still presents deficiencies in properties, i.e., the electron–hole recombination rate and the restricted response in the UV region. In this regard, many strategies have been proposed to increase the associated visible light absorption by coating ZnO substrates with various nanometals or narrowing the bandgap of ZnO substrates by introducing defects.33 These attempts have thus demonstrated that a coating of plasmonic nanoparticles on metal oxides can reduce the electron/hole recombination and consequently improves the light-to-electron conversion efficiency to improve the utilization of visible light.34–36 Moreover, the creation and separation of charge carriers can be enhanced when a Schottky barrier occurs on the heterojunction between metal oxides and noble nanoparticles.37–40 Therefore, noble nanoparticles are introduced to coat ZnO substrates by applying organic linkers (e.g., MPTMS or similar molecules). However, such coatings cannot subsequently improve the charge transport and thermal endurance of ZnO substrates, although some of their properties can be enhanced by exploiting hazardous organic linkers.41
To overcome such bottlenecks, machine learning (ML) methods have emerged as a powerful tool to discover an optimized process for material preparations.42,43,60 ML as a new tool has shown its uniqueness in materials sciences, e.g., deciphering crystallography44 and discovering superconducting materials.45 Moreover, the development of high-performance computing enables an extensive application of artificial neural networks (ANNs) and deep learning. ANNs have been used to construct models to establish the relationship between the physical properties of photonic materials and the associated electromagnetic responses. The established model can subsequently project the electromagnetic responses of a photonic material once their physical parameters are input.46 However, there are only a few published studies where ML is employed to discover an optimized method to prepare nanoparticles of an anticipated surface plasmon resonance.42,47
In this work, we successfully established a model trained by a genetic algorithm artificial network (GANN), a method applied in ML to train models to project the light-to-plasmon resonance. To do so, we empirically synthesized ZnO nanoflowers on the ITO substrates and subsequently coated the gold nanoparticles onto ZnO nanoflowers by ultraviolet treatment.48 Furthermore, a mixture of sodium citrate, hydroquinone, and HAuCl4, is loaded to generate the second growth of gold nanoparticles. The optical and morphological properties of Au/ZnO were subsequently analyzed by UV-visible spectroscopy, quantum efficiency (QE), scanning electron microscopy (SEM), transmission electron microscopy (TEM), and X-ray powder diffraction (XRD). Thereby, we trained the model through GANN by utilizing the parameters of nanoparticle fabrications and the yielded light-to-plasmon resonances. Consequently, we achieved a projection enabling us to select the fabrication parameters for the anticipated light-to-plasmon resonance.
Results and discussion
Crystalline characteristics
The crystallography of the fabricated ZnO nanoflowers was analyzed by XRD in Fig. 1. The peak at 34.44° corresponds to the plane (002), indicating that the hexagonal wurtzite structure was preferentially formed in the [001] direction. Furthermore, the measured lattice planes of (100) and (101) suggested the fabricated ZnO nanoflowers have a polycrystalline structure. We could moreover find a peak at 38.1° of low intensity, corresponding to the (111) plane of the metallic gold. The other measured lattice planes, such as (211), (222), (440), (611), (622), and (444), indicated the polycrystalline structure of the ITO thin film.
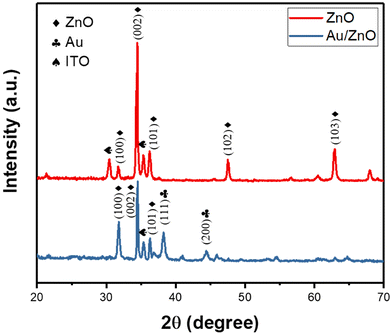 |
| Fig. 1 XRD patterns of the Au-decorated ZnO nanoflowers. The symbols depict the positions of the analyzed reflections from the Au, ZnO, and ITO planes. The Au-coated ZnO nanoflowers are synthesized using 10−4 M chloroauric acid, 0.2 wt% sodium citrate, and 3500 μL of hydroquinone. The phase analysis is executed by comparing to JCPD Standard (No. 75-576). | |
Decision flow
In order to obtain a well-trained model through GANN for the prediction, a delicate design of experiments is crucial to obtaining high-quality data and thus ensuring the trained model of high accuracy (Fig. 2). In selecting the inputs to train the model, we carefully considered the reaction mechanism, data analysis, and the collected data from previous experiments because a lucid comprehension of the reaction mechanism can lead to an appropriate input of the experimental parameters to train the model. Therefore, the trained model can yield a precise data projection in the range of interpolation and modulation of the activated-plasmon enhancement for the desired condition. In this regard, we systematically arranged the experimental parameters with a carefully designed distribution and could thus decrease the experimental amount. The achieved experimental results alongside the experimental parameters are exploited to initiate the model training with the necessary definitions, e.g., the length of data, the amount of data, the crossover ratio, the mutation ratio, and the calculation functions. However, the data varying beyond the average standard were eliminated to train the model. Subsequently, the residual data were further used and filtered according to their adaptability. However, a careful filtration is necessary as it can mislead the model training and cause mutation. After the preliminary model training, we can establish a premature model, which still has deficiencies to match the data. Hence, we need a mutation to process the derived crossover data. In addition, a feedback mechanism is required to guarantee an accurate prediction. Thereafter, we can gain a well-trained model once we accomplish a complete loop as shown in Fig. 2.
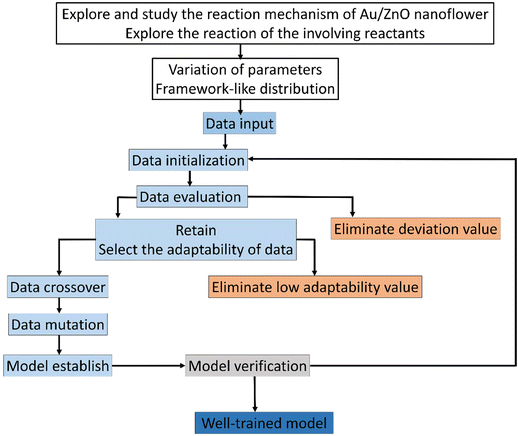 |
| Fig. 2 Machine learning framework of a closed learning loop based on GANN. The frame is separated into two sections. White represents the exploration and the parameter setting, and color represents the data input selection, the model establishment, and the feedback mechanism. | |
Model establishment
Three different algorithms, ANN, genetic algorithm (GA), and GANN, are usually applied to address nonlinear questions in conventional programming. We thereby employed these methods to derive a prediction of the position of surface plasmon resonance, which was verified by the empirical investigation (Fig. 2). We additionally defined a regression line (Y = X) as an ideal prediction. Namely, the closer the projected data are located to the regression line, the higher accuracy the trained model has. To maintain consistency, we utilized the same sets of parameters in training the model through the three algorithms aforementioned (Fig. 3). The generated results by applying ANN manifested a lower distribution condensation than GA and GANN, suggesting ANN in our study can lead to a more precise prediction (Fig. 3). Such precise prediction relies on the neuromorphic system of ANN, which can optimize the specific weights and generate a better classification. However, due to a lack of evolution, ANN is inefficient in handling complex questions. On the contrary, GA can generate a fitting function for our research by forming a crossover of the input parameters and mutations for multiple solutions. Therefore, a combination of ANN and GA can yield a more efficient model by considering the crossover and mutations. Moreover, this combination supports a feedback mechanism to modify and optimize the network structure, thus realizing deep learning on multiple levels.
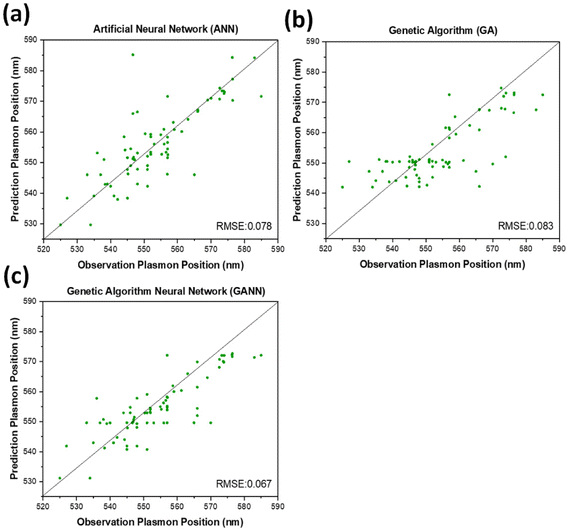 |
| Fig. 3 Leave-one-out cross-validation results for the three different algorithm models to predict the plasmon positions of the Au-coated ZnO nanoflowers: (a) the artificial neural network (RMSE = 0.078), (b) the genetic algorithm (RMSE = 0.083), and (c) the genetic algorithm neural network (RMSE = 0.067). The Y = X line is delineated as a representation of an ideal prediction. | |
After learning cycles, we achieved the prediction model through GANN, and we thereafter obtained the plasmon positions, the quantum efficiencies (QE), and the reciprocals of the full widths at half maximum (FWHMs) (Fig. 4). In training the model, we considered the experimental parameters, i.e., the times of the UV treatment and the hydroquinone amounts, as the dependent variables. On the contrary, we considered the anticipated parameters as independent variables, the positions of the surface plasmon resonance, the QEs, and the FWHMs. Therefore, the positions of the surface plasmon resonance can be projected concerning the times of the UV treatments and the hydroquinone amounts (Fig. 4a), where the color scale bar represents the plasmon shifting of a max wavelength of 585 nm (in red). The other colors in Fig. 4a represent decreasing plasmon positions from orange to blue (min: 525 nm). A larger wavelength of the surface plasmon resonance occurred at a hydroquinone amount in the range of 2200–2600 μL and the gold colloid amount of 7–22 μL. The lower wavelength of the surface plasmon resonance happened with a hydroquinone amount of less than 1100 μL or with a UV treatment over 20 min. Similarly, we obtained a QE prediction in Fig. 4b, where the red color is the maximum efficiency and the blue color is the minimum efficiency. The lower QE occurred when the hydroquinone amount was 1500–1900 μL or 2400–2800 μL. Interestingly, there was a small range displaying an impressive QE up to 66%, where the hydroquinone amount was around 1600 μL, and the UV treatment was roughly 4 min. We furthermore performed the FWHM prediction in Fig. 4c, providing information on the morphological peaks. We can thereby find a sharp peak for the UV treatment of roughly 32 min. Moreover, we can smoothen the absorption peak by decreasing the treatment time. This information is very beneficial in designing a material with the anticipated surface plasmon resonance.
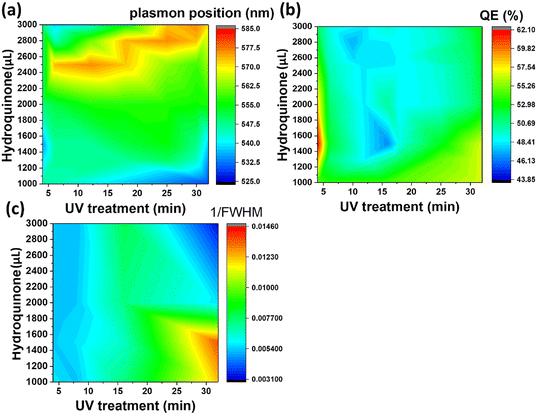 |
| Fig. 4 GANN was utilized to calculate (a) the plasmon positions, (b) the quantum efficiencies, and (c) the reciprocals of FWHMs of the Au-coated ZnO nanoflowers. | |
Model validation
In order to validate the model trained by GANN, we cross-checked the data projected by the model, regarding the positions of the surface plasmon resonances, the QEs, and the reciprocals of FWHMs (1/FWHM) (Fig. 5–7). In this regard, the concentration and the volume of the chloroauric acid solution were fixed at 10−4 M and 10 mL. We subsequently varied the treatment times of UV from 5 to 32 min, and the hydroquinone amounts from 1000 to 3000 μL. We observed a good correlation between the experimental data (Fig. 5) and the projected data (Fig. 4a): the high wavelength of the surface plasmon resonance appeared with the hydroquinone amount in the range of 2200 to 2600 μL; the low wavelength appears at the hydroquinone amount of 1000 μL and the UV treatment over 25 min. We thereby extracted the conditions to synthesize five samples of different wavelengths for the surface plasmon resonance. Additionally, we analyzed the sizes and the morphologies of the synthesized gold nanoparticles, suggesting that the bigger particles appeared at the surface plasmon resonance of a larger wavelength (Fig. 5). We furthermore observed that the thorns became shorter and more difficult to find when the surface plasmon resonance wavelength reached over 554 nm. Moreover, such particles became spherical when the wavelength was larger than 572 nm. Moreover, we noticed that the morphology of gold nanoparticles became random and began to aggregate, with a respective QE of 42% (Fig. 5e). Therefore, we selected 10−4 M chloroauric for further validation concerning QEs and 1/FWHMs (Fig. 6 and 7).
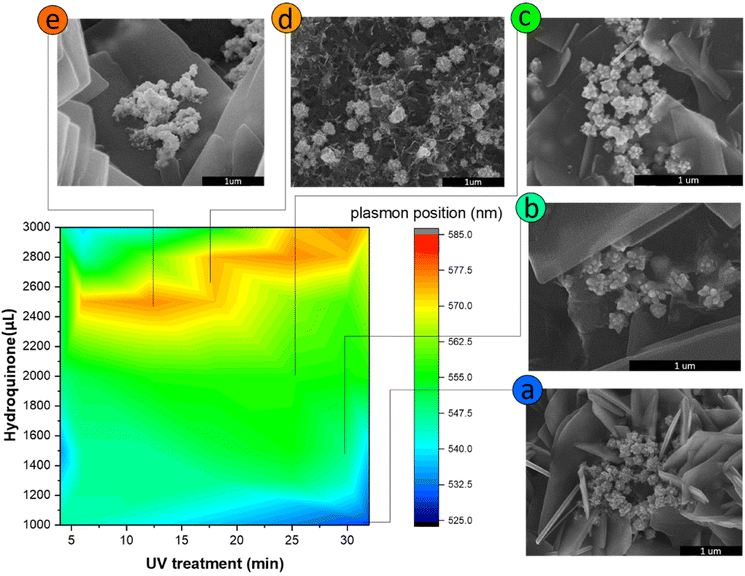 |
| Fig. 5 Configuration between the morphologies and the plasmon positions of the Au-coated ZnO nanoflowers. Size-dependent Au particles were coated on the ZnO nanoflowers under different conditions, displayed from (a) to (e). | |
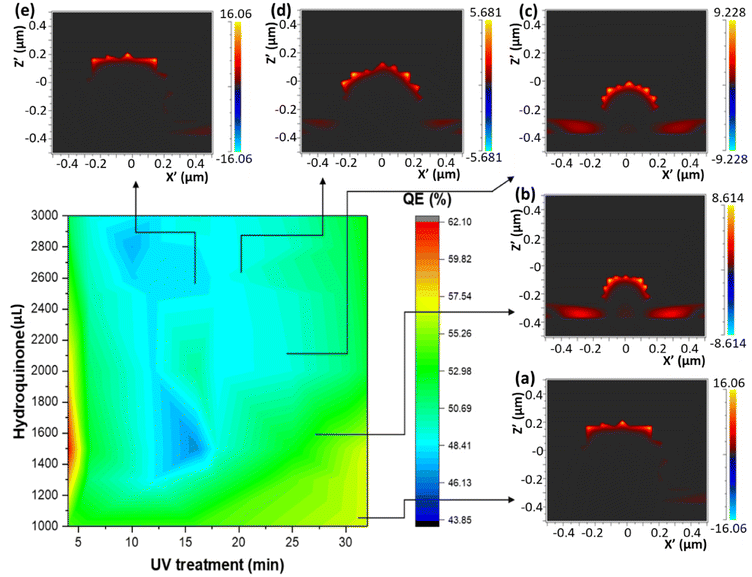 |
| Fig. 6 Configuration between the resonance simulation and the plasmon positions of the Au-coated ZnO nanoflowers. The FDTD simulation results are displayed from (a) to (e) concerning the urchin-like gold nanoparticles coated on the ZnO nanoflower surfaces of different sizes and morphologies by varying wavelengths of the incident light. The quantum efficiency (QE) indicates the conversion ratio of the photons of the incident light to the surface plasmon resonance. In order to calculate QE, we collected the scattering light of surface plasmon resonance by integrating the sphere, measured the energy of the scattering light, and then converted the scattering light energy into the quasi-particle number according to the Plank equation (E = nhυ). The quasi-particle number is the surface plasmon resonance number, which serves as a numerator. The power of incident light is also converted into the number of the incident photon by the Plank equation, which acts as a denominator. Thereby, the QE of light-to-plasmon is calculated. | |
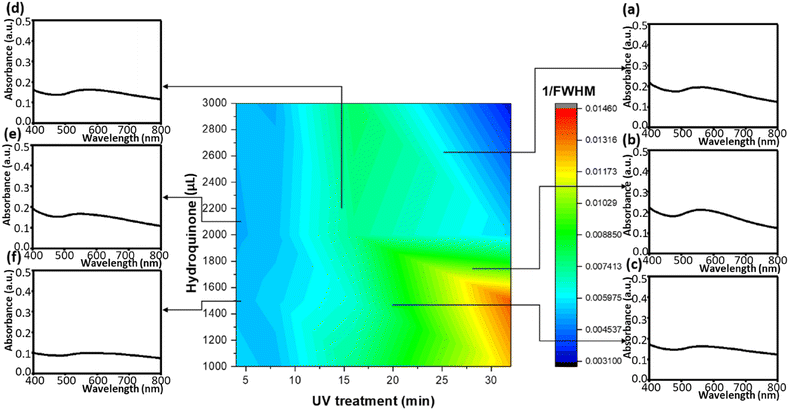 |
| Fig. 7 Configuration between the absorption spectra and the reciprocals of the FWHMs of the Au-coated ZnO nanoflowers. The absorption peaks of various morphologies at different conditions are displayed from (a) to (f). | |
We performed an FDTD simulation to study the generated electromagnetic field on the surfaces of the gold nanoparticles (Fig. 6). The strength of the electromagnetic field on the gold nanoparticles can consequently impact the resonant reaction of the surfaces.49,50 Subsequently, we utilized the FDTD models to simulate the particles with similar morphologies to those observed by SEM (Fig. 5a–e). The advantage of FDTD models considers the number of thorns, the size of particles, and the thickness of the ZnO. In our modeling, we fixed the thickness of the ZnO thin films to 30 nm and the incident light perpendicular to the ITO thin film. However, in the modeling, we introduced the incident light of two different wavelengths (400 nm and 800 nm) to the Au/ZnO system. Once the incident light of a specific wavelength induces an electron sea on the gold nanoparticle, a surface plasmon resonance can consequently occur (Fig. 6). Thereby, the strongest oscillation can subsequently take place on the surfaces of the gold nanoparticles, attributed to several factors. One of these factors is the interaction between the incident light and the electron sea, leading to the fluctuation in the system of the activated electromagnetic field.49,51 The fluctuation can further influence the electron coupling with the polar by the surface plasmon polariton. Another factor is the tip-enhancement due to the varying morphologies of the gold nanoparticles.52 Therefore, we used a second-growth method to synthesize the urchin-like gold nanoparticles on the ZnO nanoflower surfaces, yielding many thorns.53 These thorns of small tip radii manifest strong electric fields, inducing the electron polarization and thus leading to tip-enhancement. Therefore, it is probable to transfer electrons from gold nanoparticles to ZnO semiconductors.
We moreover found the variation of the UV treatment times and the hydroquinone amounts can impact the absorption peaks as well as the reciprocals of FWHMs (Fig. 7). In the configuration diagram, the morphology of the absorption peaks varied slightly, so it was necessary to apply a numerical analysis to investigate the trifling changes. However, the reciprocal FWHMs can directly inspect such variations. Thereby, 1/FWHMs of the Au/ZnO absorption system showed a resonant coherence due to the two influential factors. The first was the size, the morphology, the distribution, and the stacking of gold nanoparticles on ZnO nanoflowers. The second was whether the incident light could induce the electron sea on the gold nanoparticles or the tip-enhancement, dependent on the profile of the gold nanoparticles, the shape of thorns, the density of thorns, and the distribution of thorns.54 Overall, most of the above effects were directly related to the charge distribution and indirectly associated with the structure of the gold nanoparticles. A combination of material development and machine learning coupled with a genetic algorithm neural network can precisely project the material characteristics and optimize the fabrication process of the material composite. Moreover, the developed projection model can effectively and accurately simulate the empirical results.
Conclusion
In this work, we coated the urchin-like gold nanoparticles onto ZnO nanoflower surfaces and successfully established a precise prediction model trained by GANN. There were four factors significantly impacting the precision of the trained model. Moreover, these four factors can be observed from the decrease of RMSE and the convergence of the tested and trained data. The first was the amount of the hidden layer, separating data into different districts in order to clarify the whole database. The second was the neural number in the hidden layer. An increased number of neurons can enlarge the complexity of the training process. Furthermore, the number of neurons was double the number of the independent variables. The third was the mating ratio, the adjustment of which was crucial to establishing a well-training model. Moreover, an appropriate mating ratio can optimize the solution. The last was the learning cycle, whose prolongation can reduce the RMSE and yield a better projection. Consequently, the utilization of the model obtained by machine learning can finally generate predictable results in line with an empirical fabrication, facilitating a tuning of the wavelength of the surface plasmon resonance and the quantum efficiencies. We can thereby produce gold nanoparticles of the desired light-to-plasmon resonance, guiding the design of high-performance water-splitting cells.
Materials and methods
Materials
Zinc acetate (Zn(CH3COO)2·2H2O), chloroauric acid (HAuCl4), sodium citrate (Na3C6H5O7·2H2O), and hydroquinone (C6H4(OH)2) were purchased from Sigma Aldrich. The applied chemical reagents were of analytical grade. Deionized water (DI) was used throughout the experiment.
Preparation of pure ZnO
The ITO substrate was cleaned with ethanol and acetone by ultrasonication for 10 minutes, rinsed with DI water, and dried with N2. The precursors of the ZnO seed layer were 0.05 M zinc acetate in 0.02 M hexamethylenetetramine (HMTA). After coating the precursors on the ITO substrates, samples were then annealed at 350 °C for 10 minutes. Subsequently, the samples were immersed in the precursor solution of ZnO nanorods at 95 °C for 3 hours, which were prepared by dissolving the 0.02M zinc acetate solution in HMTA (0.02 M, 0.01 M, and 0.005 M). Afterward, the samples were annealed again at 350 °C for 30 minutes. The annealed samples were then immersed into the precursors of ZnO nanoflowers containing 0.15 M zinc acetate in methanol at 60 °C for a given time (16, 18, 20, 22, and 24 hours).
Preparation of Au-modified ZnO
ZnO nanoflower substrates were immersed in a 10−4 M HAuCl4 solution, and then an ultraviolet (UV) light was applied for an appropriate time (4 min, 8 min, 16 min, and 32 min) to obtain the reduced AuIII on the ZnO nanoflower surfaces for the generation of spherical gold nanoparticles. The samples were afterward rinsed twice with DI water. The 0.2 wt% sodium citrate solution in 3 mL of hydroquinone was subsequently added to the samples, and HAuCl4 was then added.
Characterization
The surface morphology of the gold sea-urchin-like nanoparticles was determined by field emission scanning electron microscopy (FESEM, Hitachi). The transmission electron microscopy (TEM) images were recorded with a JEOL 3010 TEM (Netherlands, Philips).55,56 The X-ray powder diffraction (XRD) patterns were obtained from a D8 Advance diffractometer with Cu K alpha radiation (wavelength = 0.111 nm). The absorption spectra of the samples were measured using a Lambda 950 UV-vis spectrophotometer (PerkinElmer, New York, US).
Machine learning
Predictable results in materials science are the sought-after research goals that can guide controllable properties, characteristics, and functions of the fabricated materials. Artificial neural networks are well-known predictive models containing functional algorithms for different situations and complicated problems. In addition, a genetic algorithm neural network (GANN) has been successfully applied to model and tune the ample variables for practical processes. Such machine-learning studies were conducted with a commercial software “Super PCNeueron”.
A genetic algorithm neural network (GANN) consists of two main calculation parts: a genetic algorithm for optimal process and an artificial neural network for establishing a nonlinear model. The genetic algorithm contains selection, crossover, and mutation, each based on the natural reproduction rule. An artificial neural network is based on the operation of the human brain containing neurons and synapses. Thereby data can be better classified and evaluated.
The network contains a single hidden layer and 6 neurons as shown in Fig. 8. The input data comprise experiment parameters: various UV treatment times and hydroquinone amounts. After a matrix calculation, the accuracy of the trained model is inspected. If the trained model shows a low accuracy or does not fit, the model will be refined until the GA process is optimized.
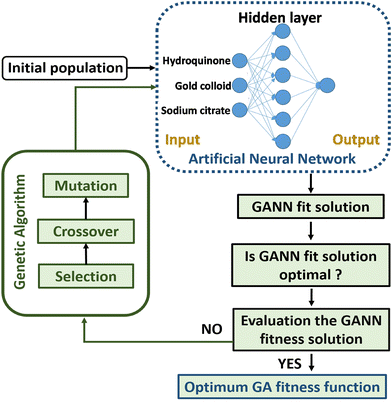 |
| Fig. 8 Flow chart of the empirical parameters in the artificial neural network coupling with the genetic algorithm of the Au-coated ZnO nanoflowers. The framework consists of two sections: the blue section (the structure of the artificial neural network) and the green section (the genetic algorithm process). | |
Conflicts of interest
There are no conflicts to declare.
Acknowledgements
The authors gratefully acknowledge the support of National Cheng Kung University and National Science and Technology Council, Taiwan, ROC for projects 109-2221-E-006-024-MY3, 111-2224-E-006-005-, 111-2622-8-006-027, 110-2224-E-006-007-, and 111-2923-E-006-008-MY3.
References
- E. W. Edwards, M. Chanana, D. Wang and H. Mohwald, Stimuli-responsive reversible transport of nanoparticles across water/oil interfaces, Angew. Chem., Int. Ed., 2008, 47(2), 320–323 CrossRef CAS PubMed.
- C. W. Cheng, E. J. Sie, B. Liu, C. H. A. Huan, T. C. Sum, H. D. Sun and H. J. Fan, Surface plasmon enhanced band edge luminescence of ZnO nanorods by capping Au nanoparticles, Appl. Phys. Lett., 2010, 96(7), 071107 CrossRef.
- Y. K. Mishra, S. Mohapatra, R. Singhal, D. K. Avasthi, D. C. Agarwal and S. B. Ogale, Au–ZnO: A tunable localized surface plasmonic nanocompositem, Appl. Phys. Lett., 2008, 92(4), 043107 CrossRef.
- P. Fageria, S. Gangopadhyay and S. Pande, Synthesis of ZnO/Au and ZnO/Ag nanoparticles and their photocatalytic application using UV and visible light, RSC Adv., 2014, 4(48), 24962–24972 RSC.
- Y. Wang, X. Li, N. Wang, X. Quan and Y. Chen, Controllable synthesis of ZnO nanoflowers and their morphology-dependent photocatalytic activities, Sep. Purif. Technol., 2008, 62(3), 727–732 CrossRef CAS.
- F. Pan, Y.-H. Su, J. Augusto, W.-S. Hwang and H.-L. Chen, Optical inclusion transformation with different amount of cerium addition during solidification of SS400 steel, Opt. Quantum Electron., 2016, 48(12), 536 CrossRef.
- P.-Y. Kung, F. Pan and Y.-H. Su, Spintronic hydrogen evolution induced by surface plasmon of silver nanoparticles loaded on Fe- and Co-doped ZnO nanorods, J. Mater. Chem. A, 2021, 9(44), 24863–24873 RSC.
- F. Pan, M. Liu, S. Altenried, M. Lei, J. Yang, H. Straub, W. W. Schmahl, K. Maniura-Weber, O. Guillaume-Gentil and Q. Ren, Uncoupling bacterial attachment on and detachment from polydimethylsiloxane surfaces through empirical and simulation studies, J. Colloid Interface Sci., 2022, 622, 419–430 CrossRef CAS PubMed.
- R. A. Wahyuono, C. Schmidt, A. Dellith, J. Dellith, M. Schulz, M. Seyring, M. Rettenmayr, J. Plentz and B. Dietzek, ZnO nanoflowers-based photoanodes: aqueous chemical synthesis, microstructure and optical properties, Open Chem., 2016, 14, 158 CAS.
- P.-Y. Kung, S.-L. Cai, F. Pan, T.-W. Shen and Y.-H. Su, Photonic fano resonance of multishaped Cu2O nanoparticles on ZnO nanowires modulating efficiency of hydrogen generation in water splitting cell, ACS Sustainable Chem. Eng., 2018, 6(5), 6590–6598 CrossRef CAS.
- P.-Y. Kung, F. Pan and Y.-H. Su, Gold nanoparticles on TM:ZnO (TM: Fe, Co) as spinplasmon-assisted electro-optic reaction modulator in solar-to-hydrogen water splitting cell, ACS Sustainable Chem. Eng., 2020, 8(39), 14743–14751 CrossRef CAS.
- Y.-S. Lai, M. A. J. V. G. Del Rosario, W.-F. Chen, S.-C. Yen, F. Pan, Q. Ren and Y.-H. Su, Energy-yielding mini heat thermocells with WS2 Water-splitting dual system to recycle wasted heat, ACS Appl. Energy Mater., 2019, 2(10), 7092–7103 CrossRef CAS.
- Y.-S. Lai, F. Pan and Y.-H. Su, Firefly-like water splitting cells based on FRET phenomena with ultrahigh performance over 12%, ACS Appl. Mater. Interfaces, 2018, 10(5), 5007–5013 CrossRef CAS PubMed.
- M.-Y. Tseng, Y.-H. Su, Y.-S. Lai, F. Pan and P.-Y. Kung, Cobalt–citrate metal–organic-framework UTSA-16 on TiO2 nanoparticles, IOP Conf. Ser.: Mater. Sci. Eng., 2020, 720, 012008 CAS.
- R. Yu, F. Pan, C. Schreine, X. Wang, D. M. Bell, G. Qiu and J. Wang, Quantitative determination of airborne redox-active compounds based on heating-induced reduction of gold nanoparticles, Anal. Chem., 2021, 93(44), 14859–14868 CrossRef CAS PubMed.
- F. Pan, S. Altenried, F. Zuber, R. S. Wagner, Y.-H. Su, M. Rottmar, K. Maniura-Weber and Q. Ren, Photo-activated titanium surface confers time dependent bactericidal activity towards Gram positive and negative bacteria, Colloids Surf., B, 2021, 206, 111940 CrossRef CAS PubMed.
- T.-S. Pan, J. Sharma, C.-C. Chu and Y. Tai, Evidences of plasmonic effect in an organic–inorganic hybrid photovoltaic device using flower-like ZnO@Au nanoparticles, J. Nanopart. Res., 2014, 16(10), 2637 CrossRef.
- P. Li, Z. Wei, T. Wu, Q. Peng and Y. Li, Au−ZnO hybrid nanopyramids and their photocatalytic properties, J. Am. Chem. Soc., 2011, 133(15), 5660–5663 CrossRef CAS PubMed.
- C. Zhang, M. Shao, F. Ning, S. Xu, Z. Li, M. Wei, D. G. Evans and X. Duan, Au nanoparticles sensitized ZnO nanorod@nanoplatelet core–shell arrays for enhanced photoelectrochemical water splitting, Nano Energy, 2015, 12, 231–239 CrossRef.
- L. Wang, S. Wang, M. Xu, X. Hu, H. Zhang, Y. Wang and W. Huang, A Au-functionalized ZnO nanowire gas sensor for detection of benzene and toluene, Phys. Chem. Chem. Phys., 2013, 15(40), 17179–17186 RSC.
- X.-j. Wang, W. Wang and Y.-L. Liu, Enhanced acetone sensing performance of Au nanoparticles functionalized flower-like ZnO, Sens. Actuators, B, 2012, 168, 39–45 CrossRef CAS.
- P. Chang and J. G. Lu, ZnO nanowire field-effect transistors, IEEE Trans. Electron Devices, 2008, 55(11), 2977–2987 CAS.
- M. Ahmad, S. Yingying, A. Nisar, H. Sun, W. Shen, M. Wei and J. Zhu, Synthesis of hierarchical flower-like ZnO nanostructures and their functionalization by Au nanoparticles for improved photocatalytic and high performance Li-ion battery anodes, J. Mater. Chem., 2011, 21(21), 7723–7729 RSC.
- D. A. Wheeler, R. J. Newhouse, H. N. Wang, S. L. Zou and J. Z. Zhang, Optical properties and persistent spectral hole burning of near infrared-absorbing hollow gold nanospheres, J. Phys. Chem. C, 2010, 114(42), 18126–18133 CrossRef CAS.
- W. He, H.-K. Kim, W. G. Wamer, D. Melka, J. H. Callahan and J.-J. Yin, Photogenerated charge carriers and reactive oxygen species in ZnO/Au hybrid nanostructures with enhanced photocatalytic and antibacterial activity, J. Am. Chem. Soc., 2014, 136(2), 750–757 CrossRef CAS PubMed.
- J. Yuan, E. S. G. Choo, X. Tang, Y. Sheng, J. Ding and J. Xue, Synthesis of ZnO–Pt nanoflowers and their photocatalytic applications, Nanotechnology, 2010, 21(18), 185606 CrossRef PubMed.
- Z. L. Wang and J. Song, Piezoelectric nanogenerators based on zinc oxide nanowire arrays, Science, 2006, 312(5771), 242 CrossRef CAS PubMed.
- F. Boccuzzi, A. Chiorino, S. Tsubota and M. Haruta, An IR study of CO-sensing mechanism on Au/ZnO, Sens. Actuators, B, 1995, 25(1), 540–543 CrossRef CAS.
- Ş. Aydoğan, K. Çınar, H. Asıl, C. Coşkun and A. Türüt, Electrical characterization of Au/n-ZnO Schottky contacts on n-Si, J. Alloys Compd., 2009, 476(1), 913–918 CrossRef.
- H. Zhang, G. Chen and D. W. Bahnemann, Photoelectrocatalytic materials for environmental applications, J. Mater. Chem., 2009, 19(29), 5089–5121 RSC.
- Y. Chen, D. Zeng, K. Zhang, A. Lu, L. Wang and D.-L. Peng, Au–ZnO hybrid nanoflowers, nanomultipods and nanopyramids: one-pot reaction synthesis and photocatalytic properties, Nanoscale, 2014, 6(2), 874–881 RSC.
- J. Zhang, X. Liu, S. Wu, B. Cao and S. Zheng, One-pot synthesis of Au-supported ZnO nanoplates with enhanced gas sensor performance, Sens. Actuators, B, 2012, 169, 61–66 CrossRef CAS.
- J. Guo, J. Zhang, M. Zhu, D. Ju, H. Xu and B. Cao, High-performance gas sensor based on ZnO nanowires functionalized by Au nanoparticles, Sens. Actuators, B, 2014, 199, 339–345 CrossRef CAS.
- P. Pawinrat, O. Mekasuwandumrong and J. Panpranot, Synthesis of Au–ZnO and Pt–ZnO nanocomposites by one-step flame spray pyrolysis and its application for photocatalytic degradation of dyes, Catal. Commun., 2009, 10(10), 1380–1385 CrossRef CAS.
- Q. Wang, B. Geng and S. Wang, ZnO/Au hybrid nanoarchitectures: wet-chemical synthesis and structurally enhanced photocatalytic performance, Environ. Sci. Technol., 2009, 43(23), 8968–8973 CrossRef CAS PubMed.
- X. Liu, J. Zhang, X. Guo, S. Wu and S. Wang, Amino acid-assisted one-pot assembly of Au, Pt nanoparticles onto one-dimensional ZnO microrods, Nanoscale, 2010, 2(7), 1178–1184 RSC.
- W. I. Park, G.-C. Yi, J. W. Kim and S. M. Park, Schottky nanocontacts on ZnO nanorod arrays, Appl. Phys. Lett., 2003, 82(24), 4358–4360 CrossRef CAS.
- A. S. K. Hashmi, Gold-catalyzed organic reactions, Chem. Rev., 2007, 107(7), 3180–3211 CrossRef CAS PubMed.
- P. Nordlander and E. Prodan, Plasmon hybridization in nanoparticles near metallic surfaces, Nano Lett., 2004, 4(11), 2209–2213 CrossRef CAS.
- N. Gogurla, A. K. Sinha, S. Santra, S. Manna and S. K. Ray, Multifunctional Au-ZnO plasmonic nanostructures for enhanced UV photodetector and room temperature NO sensing devices, Sci. Rep., 2014, 4(1), 6483 CrossRef CAS PubMed.
- N. Udawatte, M. Lee, J. Kim and D. Lee, Well-defined Au/ZnO nanoparticle composites exhibiting enhanced photocatalytic activities, ACS Appl. Mater. Interfaces, 2011, 3(11), 4531–4538 CrossRef CAS PubMed.
- J. A. Arzola-Flores and A. L. González, Machine learning for predicting the surface plasmon resonance of perfect and concave gold nanocubes, J. Phys. Chem. C, 2020, 124(46), 25447–25454 CrossRef CAS.
- C.-C. Wu, F. Pan and Y.-H. Su, Surface plasmon resonance of gold nano-sea-urchins controlled by machine-learning-based regulation in seed-mediated growth, Adv. Photonics Res., 2021, 2(9), 2100052 CrossRef.
- S. P. Ong, Accelerating materials science with high-throughput computations and machine learning, Comput. Mater. Sci., 2019, 161, 143–150 CrossRef CAS.
- V. Stanev, C. Oses, A. G. Kusne, E. Rodriguez, J. Paglione, S. Curtarolo and I. Takeuchi, Machine learning modeling of superconducting critical temperature, npj Comput. Mater., 2018, 4(1), 29 CrossRef.
- T. Zhang, Q. Liu, Y. Dan, S. Yu, X. Han, J. Dai and K. Xu, Machine learning and evolutionary algorithm studies of graphene metamaterials for optimized plasmon-induced transparency, Opt. Express, 2020, 28(13), 18899–18916 CrossRef CAS PubMed.
- J. C. M. Gomes, L. C. Souza and L. C. Oliveira, SmartSPR sensor: Machine learning approaches to create intelligent surface plasmon based sensors, Biosens. Bioelectron., 2021, 172, 112760 CrossRef CAS PubMed.
- J.-J. Wu and C.-H. Tseng, Photocatalytic properties of nc-Au/ZnO nanorod composites, Appl. Catal., B, 2006, 66(1), 51–57 CrossRef CAS.
- N. Kumar, B. M. Weckhuysen, A. J. Wain and A. J. Pollard, Nanoscale chemical imaging using tip-enhanced Raman spectroscopy, Nat. Protoc., 2019, 14(4), 1169–1193 CrossRef CAS PubMed.
- L. Meng, T. Huang, X. Wang, S. Chen, Z. Yang and B. Ren, Gold-coated AFM tips for tip-enhanced Raman spectroscopy: theoretical calculation and experimental demonstration, Opt. Express, 2015, 23(11), 13804–13813 CrossRef CAS PubMed.
- N. Behr and M. B. Raschke, Optical antenna properties of scanning probe tips: plasmonic light scattering, tip−sample coupling, and near-field enhancement, J. Phys. Chem. C, 2008, 112(10), 3766–3773 CrossRef CAS.
- J. Li, J. Zhou, T. Jiang, B. Wang, M. Gu, L. Petti and P. Mormile, Controllable synthesis and SERS characteristics of hollow sea-urchin gold nanoparticles, Phys. Chem. Chem. Phys., 2014, 16(46), 25601–25608 RSC.
- L. Yang, G. Li, J. Chang, J. Ge, C. Liu, F. Vladimir, G. Wang, Z. Jin and W. Xing, Sea urchin-like Aucore@Pdshell electrocatalysts with high FAOR performance: coefficient of lattice strain and electrochemical surface area, Appl. Catal., B, 2020, 260, 118200 CrossRef CAS.
- K. L. Kelly, E. Coronado, L. L. Zhao and G. C. Schatz, The optical properties of metal nanoparticles: the influence of size, shape, and dielectric environment, J. Phys. Chem. B, 2003, 107(3), 668–677 CrossRef CAS.
- F. Pan, H.-L. Chen, Y.-H. Su, Y.-H. Su and W.-S. Hwang, Inclusions properties at 1673 K and room temperature with Ce addition in SS400 steel, Sci. Rep., 2017, 7, 2564 CrossRef PubMed.
- F. Pan, G. Giovannini, S. Zhang, S. Altenried, F. Zuber, Q. Chen, L. F. Boesel and Q. Ren, pH-responsive silica nanoparticles for triggered treatment of skin wound infections, Acta Biomater., 2022, 145, 172–184 CrossRef CAS PubMed.
- J. Wei, R. Wang, F. Pan and Z. Fu, Polyvinyl alcohol/graphene oxide conductive hydrogels via the synergy of freezing and salting out for strain sensors, Sensors, 2022, 22(8), 3015 CrossRef CAS PubMed.
- Y. Yang, N. Wu, B. Li, W. Liu, F. Pan, Z. Zeng and J. Liu, Biomimetic porous MXene sediment-based hydrogel for high-performance and multifunctional electromagnetic interference shielding, ACS Nano, 2022 DOI:10.1021/acsnano.2c06164.
- J. Wei, C. Zhu, Z. Zeng, F. Pan, F. Wan, L. Lei, G. Nyström and Z. Fu, Bioinspired cellulose-integrated MXene-based hydrogels for multifunctional sensing and electromagnetic interference shielding, Interdiscip Mater., 2022, 1–12 Search PubMed.
- Y.-S. Lai and Y.-H. Su, Over 9% water splitting nature dyes solar cells via artificial intelligent selected combination, ACS Agric. Sci. Technol., 2022, 2, 615–624 CrossRef CAS.
Footnotes |
† Contributed equally. |
‡ Present address: Department of Chemistry, University of Basel, Mattenstrasse 24a, BPR 1096, Basel 4058, Switzerland. |
|
This journal is © The Royal Society of Chemistry 2022 |
Click here to see how this site uses Cookies. View our privacy policy here.