DOI:
10.1039/D2LC00220E
(Perspective)
Lab Chip, 2022,
22, 2403-2422
Frontiers in single cell analysis: multimodal technologies and their clinical perspectives
Received
8th March 2022
, Accepted 31st May 2022
First published on 9th June 2022
Abstract
Single cell multimodal analysis is at the frontier of single cell research: it defines the roles and functions of distinct cell types through simultaneous analysis to provide unprecedented insight into cellular processes. Current single cell approaches are rapidly moving toward multimodal characterizations. It replaces one-dimensional single cell analysis, for example by allowing for simultaneous measurement of transcription and post-transcriptional regulation, epigenetic modifications and/or surface protein expression. By providing deeper insights into single cell processes, multimodal single cell analyses paves the way to new understandings in various cellular processes such as cell fate decisions, physiological heterogeneity or genotype–phenotype linkages. At the forefront of this, microfluidics is key for high-throughput single cell analysis. Here, we present an overview of the recent multimodal microfluidic platforms having a potential in biomedical research, with a specific focus on their potential clinical applications.
1. Introduction
Untangling the complexity of cellular processes is a major challenge in biomedical research. This quest is fed by the technological development that have enabled analysis at the single cell level in fields including genomics,1–3 epigenomics,4 transcriptomics,5 metabolomics6 and proteomics.7,8 Collectively, these assays are capable of analysing all the major stages in the central dogma of molecular biology, from DNA and RNA sequencing to protein detection, thereby providing detailed insights into cellular processes. Over the past decade, further technical advances have allowed for the simultaneous measurement of multiple cellular parameters at the single cell level. Multimodal single cell analysis (MSCA) paves the way to gaining deep insights into cell heterogeneity to discover of new sub-categories of cells.9 Such analysis supports mechanistic understanding of genotype–phenotype relationships through a detailed descriptions of cell fitness and intermediate cell phenotypes.10 Large cellular atlases displaying cell lineage trajectories and molecular changes could thus be generated.
Microfluidics has recently been shown to be particularly promising as a technical platform for MSCA. Beyond the ability to miniaturize conventional assays, microfluidics offers advantages of precise automated liquid handling and high throughput capability. It is compatible with a diverse set of analytical measuring systems including fluorescence microscopy,11 imaging,12 Raman spectroscopy,13 chromatography and mass spectrometry.14 All these aspects are highly important for analysis of small sample volumes, handling scarce samples and achieving the necessary precision associated with such single cell measurements. Flow cytometry has long been considered the “gold standard” for cell-based analysis. Despite its widespread use, flow cytometry has several limitations, mainly its ability to measure only cellular proteins and the requirement for a relatively large starting number of cells.15 Therefore, the development of new microfluidic technologies is a crucial endeavor with great possibility to unlock the potential of MSCA.
In this review, we focus on the recent progress of microfluidics for MSCA, which represents the ability to simultaneously measure multiple parameters in one experiment. The examples discussed relate to the analysis of genotype–phenotype relationships, the development of chemotherapy resistance in cancer therapies, and cell heterogeneity in oncology, immunology and neurology. A specific focus is placed on the potential clinical applications of the technologies (Fig. 1) and potential technological developments.
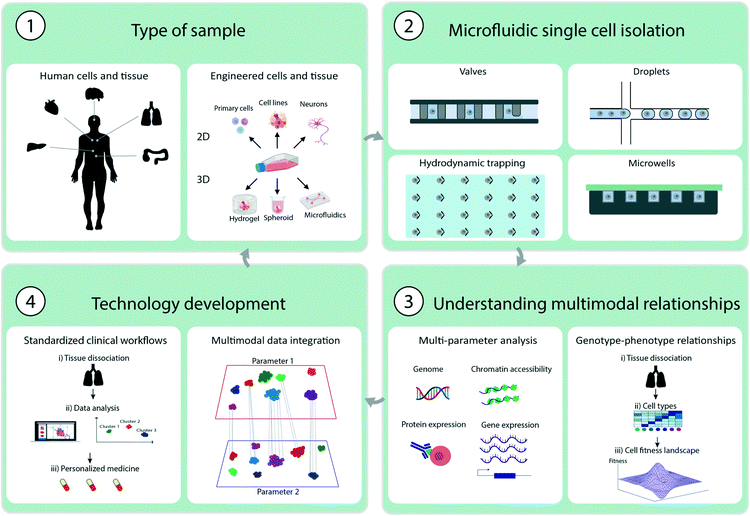 |
| Fig. 1 Illustration of multimodal single cell analysis methodology and technical advancements. 1. Illustration of sample types derived from primary tissue cells or engineered cells or tissues. 2. Different microfluidic single cell isolation techniques using valves, droplets, hydrodynamic trapping or microwells. 3. Understanding multimodal relationships by measure multiple single cell parameters for example genome, chromatin accessibility, protein or gene expression and understand genotype-phenotype relationships 4. Technological development by standardizing clinical workflows and data integration of multiple parameters to understand multimodal relationships. | |
2. Key strategies in multimodal analysis
Several key aspects should be considered when performing MSCA. In this section, we provide a short overview of experimental methods specifically designed for MSCA starting from sample preparation to microfluidic single cell isolation and manipulation techniques. We also provide an overview of strategies for multimodal analysis and commercial microfluidic platforms that are suitable for this analysis.
2.1 Sample preparation
Sample preparation is the first step of MSCA. Depending on the sample type and analytical method the preparation varies greatly. Enrichment of certain cell types may be necessary before the analysis: blood samples may need non-relevant cell types to be removed through density gradient separation or enrichment of the cells of interest with magnetic beads coupled to antibodies.15
For tissue samples, a key step is tissue dissociation. Primary aspects to consider include ensuring a homogeneous cell solution while maintaining high cell viability. Dissociation protocols must generally be optimized for each tissue type, because the composition of the extracellular matrix can substantially vary. Some cell types are sensitive to harsh dissociation protocols, and therefore gentle dissociation is required to maintain an accurate representation of the whole cell population. Furthermore, sample handling should be rapid, to avoid unwanted transcriptomic changes or overexpression of stress-response-related genes.16 Performing pertinent quality control at the bulk level is therefore highly recommended to ensure high quality of the samples for subsequent single cell analysis.17
Another concern is the presence of non-viable cells in the cell solution and leaching of cell components into the solution, which may result in compartmentalization of non-relevant cellular content together with single cells. To overcome these challenges, computational methods have recently been used to account for such biases, with a focus on transcriptomic analysis.18
2.2 Microfluidic single cell isolation
Several microfluidic approaches for single cell isolation have been developed in recent years. Because these techniques have been extensively reviewed elsewhere,19,20 they are summarized in Table 1, and only a brief overview is presented here.
Table 1 Comparison of different microfluidic single cell isolation techniques illustrating valves, microwells, droplets or hydrodynamic trapping
First, one approach relies on valve-based systems, as introduced by S. Quake and colleagues,21,22 who developed pressure controlled valves in a multilayer microfluidic channel to control and direct the flow. The valves create a chamber allowing for single cell isolation and precise control of reagent flow. Because of the high control over the pressure system, these units can be parallelized and can enable a fully integrated workflow. However, these systems tend to be fairly complex in fabrication and design.
Second, well-based systems rely on microwells for single cell isolation. Microwells are loaded with cells through cell sedimentation when a cell suspension is flowed through the device.23 The actual number of cells seeded in a microwell array is generally Poisson distributed. The Poisson statistics holds true as long as the assumption remains valid that the spatial position of a cell is not affected by other cells, i.e., cells are distributed in the initial sample with a uniform distribution. A low density cell suspension is used to ensure that one cell is present per well. The wells can be subsequently sealed for cell compartmentalization. This method enables high-throughput analysis and is attractive because of its simplicity,24 but it does not support complex workflows.
A third approach for single cell isolation relates to the field of droplet-based microfluidics. Using droplet-based microfluidics, nL to fL aqueous droplets can be generated in an inert oil phase25 with high throughput and reproducible sizes. Through emulsification of a cell suspension, droplets are loaded with single cells. Similarly to microcompartment based approaches, such single cell encapsulation/isolation is achieved by using low cell density suspensions so that most droplets contain at most one cell following Poisson distribution.26 Each droplet serves as a compartment for the analysis of a single cell, because the oil phase serves as a physical barrier and limits cross-contamination between droplets. Perfluorinated oils are usually used as continuous phase to create droplets for biological applications, because they have two interesting properties: (i) the solubility of organic molecules in these oils is considered low, thus restricting leakage of the droplet contents and restricting cross-talk between droplets,27 and (ii) the high solubility of respiratory gasses in perfluorinated oils which ensure cell survival in droplets.28 The use of perfluorinated oils has resulted in the commercial development of several fluorinated surfactants that allows for efficient droplet stabilization. Specific efforts have also be placed on ensuring biocompatibility of these compounds.29 Furthermore, after droplets are formed, the droplet content can be manipulated by injection of another aqueous phase in the droplet through picoinjection30 or droplet merging,31 or the droplet can be divided into smaller subunits with droplet splitting.32 Once the droplet is generated they can be sorted based on fluorescence.33 Advantages of such approaches thus rely both on its high throughput but also on the flexibility of operations that is given, permitting the efficient implementation of complex and multi-step workflows.
The approaches that have been introduced above compartmentalize single cells. In contrast, microfluidic cell trapping does not establish a physical barrier between single cells. Trapping-based systems form arrays of trapped cells in a microfluidic device when a cell suspension is flowed through the device. Individual cells have been passively trapped by means of a microfabricated physical geometry.34
However, in recent years, further integration of microfluidic devices has given rise to non-contact methods for active trapping of single cells. These methods rely on a physical contrast (e.g., permittivity, refraction index, density and compressibility) between the cells and their suspension medium. Trapping based on dielectrophoresis35 or optical methods36 has been developed. Both methods rely on the dielectric properties of the cell to generate a force, which holds the cell in place. For dielectrophoresis this is usually done by designing microelectrodes, while optical trapping relies on a highly focused laser beam with a steep intensity gradient. Furthermore, Collins et al. have illustrated the trapping of an array of single cells in acoustic traps.37 Through the use of standing surface acoustic waves, single cells can be trapped in pressure nodes and individually visualized.
2.3 Microfluidic single cell manipulation
After single cells are isolated, the cells are then incubated to enable long-term cell culture. Incubation in traps or wells is relatively straightforward, because these flow-through systems enable exchange of the medium and metabolites. However, cell incubation in droplets may be constrained by the limited amount of nutrients that the drop contains and the limited accessibility to oxygen. To circumvent these issues cells can instead be encapsulated in large droplets providing sufficient nutrients for long term cell culture38,39 or cultured in hydrogel droplets allowing for easy exchange of medium.40 Another aspect to consider is that dynamic droplet incubation may be required to provide homogeneous oxygen accessibility.41
Another critical step in multiomic cell analysis is cell lysis, because it is essential for efficient extraction and analysis of intracellular material. Lysis should not interfere with the rest of the microfluidic workflow, to ensure the pertinence of subsequent analysis. Most lysis methods have been developed for bulk suspensions. Therefore, transitioning these methods to microfluidic workflows might need adjustment and new methods dedicated to microfluidic single cell assays should also probably be developed. Most microfluidic platforms use chemical lysis, which can easily be integrated in microfluidic systems. Other options include electrical lysis42,43 or optical lysis.44 For chemical lysis, lytic agents can be added by controlling the microfluidic valves, or by flowing of the lytic agents through well-based systems. When droplets are used, the lytic agents can be encapsulated the cell when drops are produced, or afterward through droplet fusion or picoinjection.30 However, chemical cell lysis in trapping-based systems is more challenging. In general, extensive cell analysis, apart from microscopy, is challenging in trapped cells, because the traps do not provide a physical barrier against the diffusion of intracellular components. Marie et al. have, however, recently described the extraction of genetic information from single cells with hydrodynamic trapping in a narrow channel. Once the cell is trapped, the flow through the narrow channel is decreased to avoid inflow of other cells in the same channel and a chemical lysis agent is flushed to the cell. Each individual cell lysate is then extracted, with a parallelisation capability limited to 8 cells.45
2.4 Strategies for multimodal analysis
Most MSCA strategies have focused on either the combination of two omic methods or combining omic analysis and microscopy. The difficulty in combining omic analysis and microscopy relies on the efficient link of both measurements. By linking omic analysis with phenotypic analysis, such as cell shape, that can be observed in microscopy it is possible to highlight new genotype–phenotype relationships that has yet to be discovered. By barcoding cells with unique nucleic acid sequences different omic measurements can be analysed separately and linked together. Typically, cell imaging is performed first, and then a barcoded primer or bead is added to the cell, which can be identified during sequencing. The microfluidic chip can be prepared with different barcoded primers or oligonucleotides beforehand, so that sequencing can be associated with spatial coordinates on the microfluidic chip.46–48 Alternatively, in microwells, oligonucleotide barcodes can be added after single cell imaging, and the sequence of the barcodes can then be optically decoded through several rounds of fluorescent hybridization.49
A critical challenge in single cell omic analysis is performing high sensitivity analysis, given that single cells contain small amounts of protein and genomic material.50 Analysis is particularly challenging with MSCA, because preserving all information within a single cell is essential when more than one cellular parameter is detected. Common problems addressed include sample loss,51 incompatibility of protocols leading to molecular degradation,52 or insufficient sensitivity of available methods to ensure accurate detection of the analytes.
To circumvent these issues two main approaches have been considered to perform multiomic analysis. In the first approach, a single cells are split in two, and separate analyses are performed on each aliquot.53 Droplet splitting in droplet-based microfluidics54 and the operation of valve-based systems are two microfluidic methods enabling such analysis. Before splitting the sample, a pre-amplification of the analytes can be performed when the sample remains intact, to improve the sensitivity of analyses for genetic measurements.55
The second approach is based on simultaneous detection of the analytes to link the cell parameters. This process generally uses beads that bind the different targeted analytes.56 A molecular barcode on the bead tags cellular material, thereby enabling the clustering of data originating from the same single cell after analysis in bulk. This method does not require any washing steps or removal of liquids, thus limiting the risk of sample loss. Efficient co-compartmentalization of single cells and beads is essential in this approach. As described previously, in droplet microfluidics, the compartmentalization process follows a Poisson distribution, because the sample is sufficiently diluted to avoid multiple cells or beads per compartment, thereby resulting in only a small fraction of the droplets containing both analytes, because the two loading processes are independent.25,55 Such issues could dramatically reduce experimental throughput. However it can be circumvented by different means such as close packing when deformable beads are encapsulated,57 loading the sample by inertial ordering of the particles at high flow velocity38 or hydrodynamic trapping of particles before droplet production.39
2.5 Commercial microfluidic platforms for single cell analysis
Commercial microfluidic platforms are an essential part of medical research and are available in clinical research settings (Table 2). Platforms available to date include the Fluidigm C1 (Fluidigm), Rhapsody platform (BD Biosciences), Chromium single cell platform (10× Genomics) and the Tapestri system (Mission Bio). The specific performances of these platforms for single cell isolation, throughput and cell parameters are compared in the following section.
Table 2 Comparison of commercial microfluidic platforms performing single cell multimodal analysis
Name |
Company |
Parameter 1 |
Parameter 2 |
Cell isolation method |
Advantages |
Disadvantages |
Throughput (per run) |
BD rhapsody |
BD biosciences |
Transcriptomics |
Surface proteins |
Nano/micro wells |
Easy data management and visualization, customize panels |
Requires fresh sample, high starting number of cells needed |
20 000 cells |
C1 REAP-seq |
Fluidigm |
Transcriptomics |
Surface proteins |
Valve-based system |
Multiple applications, flexible use, well established platform |
Limited throughput |
96 cells |
Chromium platform |
10× genomics |
Transcriptomics |
Surface proteins/chromatin accessibility |
Droplets |
High throughput, flexible use |
Requires fresh sample, high starting number of cells needed |
>10 000 cells |
Tapestri |
Mission bio |
Genomics |
Surface proteins |
Droplets |
Capable of DNA-sequencing, customize panels |
High starting number of cells needed |
∼5000 cells |
The fluidigm C1 Single-Cell Auto Prep system entered the market in 2013 and has since been applied in various single-cell omic studies. Fluidigm subsequently released another workflow called C1 REAP-seq, which is specifically designed for multiomic analysis of scRNA-seq and surface markers.58,59 The platform uses a valve-based system to execute a complex workflow. It uses a reservoir to capture, image, and perform cell lysis, reverse transcription and initial PCR reactions. The throughput of the platform is approximately 100 cells per run, thereby limiting the application scope of the workflow to low throughput analysis.60
The BD Rhapsody platform uses microwells. By pairing one cell with a barcoded bead in the well, scRNA-seq analysis can be combined with protein expression to achieve a throughput as high as 20
000 cells per experiment. The transparent microfluidic chip enables visualization and imaging during the workflow.
The 10× chromium single cell platform has been widely used for scRNA-seq experiments. This droplet-based platform encapsulates a cell and barcoded bead in a droplet and can achieve a throughput of approximately 10
000 cells per run. This platform has been used primarily for scRNA-seq analysis but now also offers a solution for measuring transcriptome and open chromatin regions with chromium single cell multiome ATAC.
Similarly, the Tapestri system (Mission Bio) uses droplet-based microfluidics to co-encapsulate cells and barcoded beads. The platform works by performing cell lysis, protease digestion, cell barcoding and targeted amplification with multiplex PCR within the droplets. scDNA-seq can be combined with surface protein detection with this platform.61 Information on copy number variations and single nucleotide variants can also be obtained.
3. Single cell multiomic analysis using microfluidics
Since the initial single cell transcriptomic study in 2009, many single cell RNA sequencing (scRNA-Seq) technologies have been developed.62 The throughput capability has significantly grown, and analysis of 2 million single cells has recently been reported.63 Transcriptomic analyses have paved the way to revealing cell heterogeneity, and unraveling previously unknown cell types and states in complex biological samples.64 scRNA-Seq assays have now been commercialized and are widely available.65 In combination with other molecular characterizations, scRNA-seq has been included in most single cell multiomics studies. Such measurements can provide data whose value exceeds that of the sum of its parts, particularly in revealing cell functions, discovering relationships across different omics and recording dynamic biological events. The multiomic single cell platforms available to date are introduced in the following sections and are compared in terms of throughput, analysis sensitivity and ability to detect multiple analytes (Table 3).
Table 3 Summary of multiomic single cell analysis technologies using microfluidic platforms
Parameter 1 |
Parameter 2 |
Method |
Microfluidic platform |
Biological model |
Throughput (number of cells) |
Max, number of analytes (per run) |
Applications |
Ref. |
Chromatin accessibility |
Transcriptomics |
ASTAR-Seq |
Fluidigm C1 microfluidic chip |
K562, JK1, BJ, and Jurkat human cell lines, mESCs, primary cord blood cells |
96 |
K562: 142886 chromatin accessibility sites and 4182 transcripts |
Identification of cell states and upstream regulatory networks |
58
|
Chromatin accessibility |
Transcriptomics |
SNARE-seq |
10× chromium droplet platform |
Mixtures of BJ, H1 hESC, K562 and GM12878 human cell lines, neonatal and adult mouse cerebral cortex |
∼10 000 |
1332 of transcripts and 2583 chromatin accessibility sites |
Identification of cell types, discovery of lineage-specific accessible sites, and connection of the dynamics of promoter accessibility with transcription level during neurogenesis |
56
|
Chromatin accessibility |
CRISPR perturbations |
Perturb-ATAC |
Fluidigm C1 microfluidic chip |
Human B lymphocytes GM12878 cells and primary human keratinocytes |
96 |
|
Analysing the role of different chromatin regultory factors to get insights in cell fate decisions |
68
|
Genomics |
Transcriptomics |
|
Valve based microfluidics |
Human myelogenous leukemia cell line K562 |
3 |
14 transcripts and 12 DNA target sequences |
Investigate the gene expression patterns and genomic alterations |
53
|
Genomics |
Transcriptomics |
|
Hydrodynamic trapping |
Mouse lymphocyte cell line A20 |
100 |
|
Simulatinious quantification of absolute amount of RNA and relative quantification of DNA |
43
|
Genomics |
Transcriptomics |
|
Hydrodynamic trapping |
Mouse lymphocyte cell line A20 |
12 cells per hour |
|
On chip single cell lysing, extraction, fractionation, and recovery of purified cytoplasmic RNA versus gDNA |
69
|
Nuclear RNA |
Cytoplasmic RNA |
SINC-seq |
Hydrodynamic trapping |
Human myelogenous leukemia cell line K562 |
12 cells per hour |
6200 and 5600 genes per cytRNA and nucRNA |
Detection of nuclear RNA and cytoplasmic RNA from single cells to analyse their correlation |
70
|
Gene expression |
DNA methylation |
sc-GEM |
Fluidigm C1 microfluidic chip |
Human BJ cells, iPSCs, ESCs and lung adenocarcinoma NSCLC sample |
96 |
50 methylated gene sites |
Assessment of primary lung adenocarcinomas and human fibroblasts reprogramming |
59
|
Enzymatic activities |
Transcriptomics |
sc-haircut |
10× chromium droplet platform |
PBMCs, haploid human UNGko and RNASEH2Cko cells |
1000–10 000 |
|
Identification of cell-type-specific DNA repair phenotypes in human lymphocytes |
71
|
Targeted genomic regions |
Transcriptomics |
CORTAD-seq |
Fluidigm C1 microfluidic chip |
Lung cancer cell line PC9 |
96 |
6000 transcripts, EGFR exons 19, 20, and 21 |
Study on lung cancer resistance to a targeted therapy |
72
|
Surface proteins |
Transcriptomics |
REAP-seq |
10× chromium droplet platform |
PBMCs |
1000–10 000 |
82 surface proteins and >20 000 RNA transcripts |
Assessment of immune response modulation, identification of unknown cell types |
73
|
Surface proteins |
Transcriptomics |
CITE-seq |
Drop-seq platform, 10× genomics droplet platform |
Human HeLa cells, mouse 4 T1 cells, PMBCs, and CBMCs |
1000–10 000 |
13 surface proteins and 400 RNA transcripts |
Integrated measurment of scRNA-seq along with surface proteins |
74
|
TCRs, surface proteins, sample identity by hashtags, and sgRNAs |
Transcriptomics |
ECCITE-seq |
10× chromium droplet platform |
A cell mixture of human PBMCs, T-cell lymphoma lines MyLa and Sez4, and mouse NIH-3 T3, PBMCs |
1000–10 000 |
49 surface proteins, ∼5000 ECCITE-seq genes, ∼4000 10× standard run, two TCRs (TCR α/β and TCR γ/δ), 7 hashing antibodies, CRISPR turbilations and 10 sgRNAs |
Multimodal CRISPR screens, clonotype-aware phenotyping of cancer samples |
76
|
Surface proteins |
Transcriptomics |
One-SENSE |
Nanowell-based, BD rhapsody platform |
PBMCs |
>20 000 cells |
>40 surface proteins and >400 transcripts |
Identification of T cell subsets |
77
|
Surface proteins |
Transcriptomics |
CITE-seq |
10× chromium droplet platform |
BMMCs and PBMCs from healthy and from patients with mixed-phenotype acute leukemia (MPAL) |
∼10 000 |
2370 transcripts and 4 surface protein markers |
Investigation of molecular features of MPAL from normal development |
78
|
Intracellular proteins |
Transcriptomics |
|
Valve-based microfluidics |
HEK and U87MG human cell lines |
9 cells |
∼2000 RNA transcripts and 3 proteins |
Identification of cell type specific signatures |
80
|
Cytokines |
Transcriptomics |
|
Nanowells-based splitable microchip |
Mouse macrophage cells |
20 |
5 cytokines and ∼4900 genes |
Study on inflammatory immune responses |
81
|
Protein |
Transcriptomics |
|
FACS and microfluidic devices |
Patient-derived glioblastoma cell line U3035MG |
210 cells |
∼96 proteins and transcripts |
Study on the effect of therapeutic agent BMP4 in glioblastoma |
83
|
Intracellular proteins |
Transcriptomics |
|
Fluidigm C1 microfluidic chip |
MCF7 human cell line |
96 |
38 intracellular proteins and 96 transcripts |
Study on the responses of breast adenocarcinoma to a chemical perturbation |
84
|
Protein |
Transcriptomics |
|
Fluidigm C1 microfluidic chip |
A549, SKBR3 and K562 human cell lines |
96 |
84 protein assays and 40 transcripts |
A comparison of mRNA and protein levels in single nanoliter reactions |
85
|
3.1 Combining transcriptomic and chromatin accessibility analysis
Many single cell assays are currently available to access open chromatin regions and subsequently reveal information on regulatory genes and gene activities. Most assays rely on enzyme-mediated modulation of chromatin, thus resulting in different degrees of openness to the chromatin landscape. Assay for transposase-accessible chromatin using sequencing (ATAC-seq) was developed to access open chromatin regions in single cells with the enzyme Tn5 transposase.66 Several studies have recently expanded on this analysis. Cao et al. have performed simultaneous profiling of RNA transcripts and accessible chromatin regions in the adult mouse kidney, and demonstrated the potential of this method by showing the transcriptional state of cells which gave new insights into the upstream regulatory landscape.67 Cell mixing experiments in combined murine and human cells have revealed that 99% of cells can be distinguished in terms of both scRNA-seq and sc-ATAC-seq profiles.
Another example of using ATAC-seq in combination with other cell parameters is a method called assay for single-cell transcriptome and accessibility regions (ASTAR-Seq) which labels open chromatin regions and performs reverse transcription of mRNA with the valve based fluidigm C1 platform. With this method, human B lymphocyte differentiation from umbilical cord blood has been analysed. By harvesting and analysing single cells at different time points of in vitro cell culturing, the authors have observed a correlation with time and cell differentiation were cells transition into a more mature cell state wherein they begin to express HBA2, a hemoglobin gene.58 Another droplet microfluidic platform allows for characterization of single-nucleus chromatin accessibility and mRNA expression sequencing (SNARE-seq) linking a cell's transcriptome with its accessible chromatin regions. In this method, ATAC-seq is used to analyse open chromatin regions, and RNA-seq is used for mRNA detection. Beads and nuclei are co-compartmentalized in droplets. The oligonucleotide split beads enable hybridization of DNA and mRNA to the same bead, thus linking the two parameters56 (Fig. 2). This method has provided new insights into the regulatory mechanisms between open chromatin regions and RNA transcripts. SNARE-seq has enabled reconstruction of the transcriptomic and epigenomic landscapes in rare cell types, thereby uncovering lineage specific accessible sites.
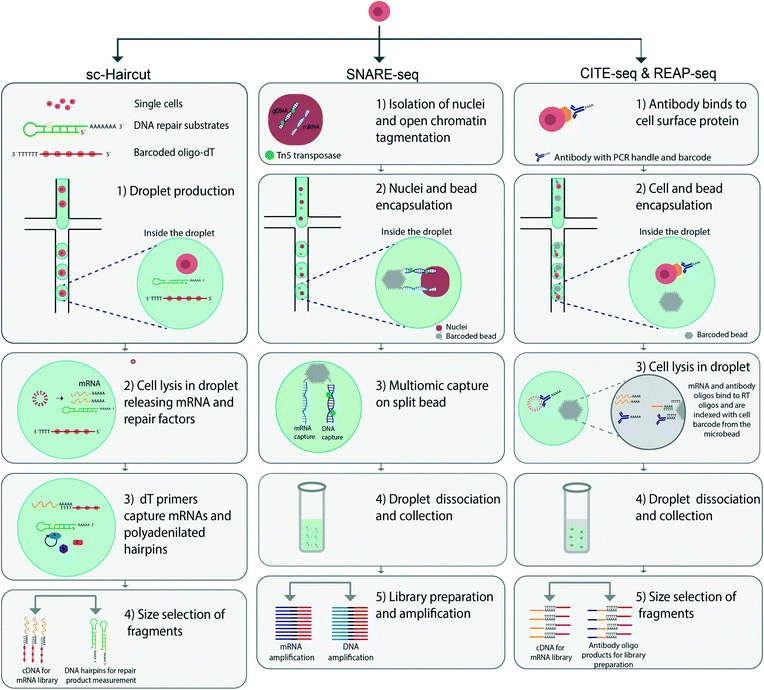 |
| Fig. 2 Comparison of different droplet microfluidic methods for MSCA analysis. The illustration comprises an overview of the workflow of Sc-haircut, SNARE-seq and CITE-seq/REAP-seq. Sc-haircut (left panel) performs parallel analysis of single cell DNA repair mechanisms and gene expression. 1) Droplet production and compartmentalisation of single cells, DNA repair substrates and barcoded oligo-DTs. 2) In drop cell lysis to release mRNA and repair factors. 3) dT primers bind to mRNA and polyadenylated hairpins. 4) Repair products and mRNAs are converted to cDNA with barcoded oligo-dT primers and separated based on size to prepare for library preparation. SNARE-seq (middle panel) 1) isolation of nuclei and DNA tagmentation using the enzyme Tn5 transposase to access the open chromatin regions 2) co-encapsulation of nuclei and beads 3) the split bead binds to both mRNA and DNA linking both modalities together. 4) Droplets are collected and merged, the beads can be harvested and washed 5) library preparation by PCR amplification of mRNA and DNA. CITE-seq /REAP-seq workflow (right panel): 1) preparing antibody-derived tags (ADTs) by oligonucleotide antibody binding to surface proteins on cells in bulk. 2) Co-compartmentalisation of single cells and barcoded beads in droplets using drop-seq 10× Genomics. 3) Cell lysis in droplets to release bound ADTs and mRNA. ADTs and mRNA are thereafter converted to cDNA and amplified using PCR. 4) Droplets are collected and merged 5) PCR for fragment amplification whereby fragments are separated by size. | |
Moreover, Rubin et al. developed Perturb-ATAC, a method in which sequencing of chromatin accessibility using ATAC-seq and CRISPR single guide RNA (sgRNA) are combined to study DNA regulatory factors and regulatory mechanisms affecting cell fate decisions in human B lymphocytes.68 Collectively, these methods provide novel strategies to study the epigenetic changes and dynamic gene expression in single cells.
3.2 Combining transcriptomic and genomic analysis
The use of microfluidics to physically separate aliquots from an initial droplet that contains a single cell, offers the possibility of measuring multiple cellular parameters simultaneously. One early example was presented in 2014 by Han et al., who used microfluidics to isolate and lyse single cells, whereby the cell content was aliquoted and analysis could be performed on the two cell aliquots. The team used a valve-based microfluidic platform for separating gDNA and cytosolic mRNA, thus enabling their simultaneous detection through separate amplification of the genome and transcriptome.53 However, the throughput was limited to three cells per microfluidic chip. Similar approaches have since been developed with automated cell handling capacity and increased throughput.43,69 Using the same underlying approach for separate analyses of cell aliquots, Abdelmoez et al. have developed a method called single-cell integrated nucRNA and cytRNA-sequencing (SINC-seq).70 The method relies on an electric field to separate the nucleus from the cytoplasm, thereby allowing for separate analysis. Through the use of this method, new insights into the regulatory relationship between nuclear RNA and cytoplasmic RNA have been discovered.
To characterize the heterogeneity of DNA repair cellular activities, Richer et al. have performed simultaneous measurement of mRNA transcripts and DNA repair mechanisms.71 They have developed a technique called single-cell haircut (sc-haircut), to compartmentalize single cells with barcoded oligo-dTs and enzymatic substrates for DNA repair using droplet microfluidics (Fig. 2). Enzymatic DNA repair mechanisms at defined positions are detected with synthetic damaged DNA hairpins. The repaired DNA hairpins and mRNAs are subsequently converted to cDNAs for separate analyses. Then, the amount of enzymatic DNA repair is quantified and associated with its gene expression. In this case, DNA lesions repaired by cellular enzymes produce a product that can be analysed by DNA sequencing.
To analyse cancer heterogeneity and drug resistance, a method called concurrent sequencing of the transcriptome and targeted genomic regions (CORTAD-seq) was developed by Kong et al.72 With the Fluidigm C1 platform, cells are first lysed, and both gDNA and cDNA are then subjected to PCR with primers specific to the regions of interest. The gDNA and cDNA are then physically separated for analyses. CORTAD-seq has been used to investigate acquired gefitinib drug resistance in PC9 cells, a lung cancer cell line, and observed that the transcriptome of the lung cancer cells undergoing a T790M mutation in the EGFR (epidermal growth factor receptor) commonly acquired in drug resistant lung epithelial cells.
3.3 Combining transcriptomic and protein analysis
The use of non-destructive assays before sequencing can link surface protein levels to cellular transcriptomes. Cellular Indexing of Transcriptomes and Epitopes by Sequencing (CITE-seq) and RNA Expression and Protein sequencing (REAP-seq) are two similar techniques that use droplet microfluidics for multimodal detection of surface proteins and mRNA transcripts.73,74 The first step in these techniques is attaching antibody-derived tags to cell surface proteins. Single cells are then co-encapsulated with barcoded beads with the commercial 10× Chromium platform. Thereafter cells are lysed inside the droplets to release both the antibody-derived tags and mRNAs, which then hybridize to the beads. The barcoded beads are composed of a PCR-handle, an antibody barcode and a poly A-tail, thus enabling the mRNA bound to beads to be transcribed and also linking the two parameters (Fig. 2). The difference between CITE-seq and REAP-seq is based on how the DNA barcode is conjugated with the antibody.73,74 The antibody used for CITE-seq is non-covalently conjugated to streptavidin attached to the DNA barcode, whereas the bonds are covalent in REAP-seq. In both methods, the number of proteins that can be simultaneously measured surpasses the number measurable by cytometry; this number is mainly limited by the number of barcode labeled antibodies as well as possible steric hindrance between the antibody and the cells. In REAP-seq, antibodies are conjugated with DNA barcodes of 66 bp divided into a unique sequence for each antibody (8 bp) and a primer sequence for amplification and subsequent sequencing. CITE-seq has been performed with 228 individual antibodies in a study constructing a multimodal reference atlas of the human PBMC.75
Mimitou et al. have expanded the CITE-seq platform to develop Expanded CRISPR compatible Cellular Indexing of Transcriptomes and Epitopes by sequencing (ECCITE-seq) in combination with the 10× Chromium platform, reaching a throughput of approximately 10
000 cells per run.76 ECCITE-seq can simultaneously detect at least five parameters in a single cell, including transcriptomics, surface proteins, sample identity by hashtags, T cell receptors (TCR α/β and TCR γ/δ) and sgRNA. Combining these parameters has enabled specific clonotype features of malignant tumor cells to be deciphered. This multiplex single-cell system can be combined in various ways and may be used in broad biomedical and clinical applications.
Mair et al. have presented a platform called one-dimensional soli expression by nonlinear stochastic embedding (One-SENSE) wherein the use of antibody-derived tags and mRNA-seq is similar to that in CITE-seq and REAP-seq.77 The major differences are that One-SENSE focuses on the selected targets at minimal read depth to minimize the cost, and it uses BD Rhapsody nanowells to achieve a higher throughput of more than 20
000 cells per run. The One-SENSE platform has been used to decipher immune cell heterogeneity in cells that were previously thought to be identical by simultaneously interrogating 492 immune-related genes and 41 surface proteins commonly used for immunophenotyping.
To analyse malignant features in acute leukemia, Granja et al. have performed integrated analyses combining CITE-seq for multiplexed protein quantification and transcriptomic profiling, and ATAC-seq for chromatin accessibility in single cells.78 Through analysis of blood and spinal fluid samples from diseased and healthy donors, specific malignant features have been detected despite widespread epigenetic heterogeneity in the patient cohort.
Measurements of both transcriptomic and intracellular proteins are necessary to obtain a complete description of the cell state. This information can shed light on the complex relationships among various cell types or states, and different disease conditions. Technologies for single cell proteomic analysis remain in their infancy. Commonly used methods for bulk analysis, such liquid chromatography-mass spectrometry, require a relatively large amount of input material, thereby making these analytes difficult to detect at the single cell level.79 Furthermore, proteins cannot be amplified similarly to RNA or DNA; therefore, the number of proteins that can be measured with single cell proteomics is limited. In the following sections, we present methods that have been used to circumvent these issues.
Xu et al. have developed a microfluidic system for investigating cell specific transcripts and proteins. Cells and sequencing beads are loaded onto a chip with a series of microchambers.80 Intracellular proteins are then subjected to a fluorescence sandwich immunoassay inside the microchambers while the barcoded beads are isolated for cDNA library generation before sequencing. The fluorescence sandwich immunoassay requires a series of washing steps; thus, the use of microfluidic valves plays a key role in adding and removing liquids. However, the throughput is limited to the number of available microchambers.
To understand and analyse single cell features of heterogeneous immune cells, George and Wang have performed cytokine profiling of murine macrophages.81 Their system is based on multiplex protein and transcriptomic analysis from the same cell with a Splittable Microchip. The Splittable Microchip consists of a polydimethylsiloxane nano-well array coated with cytokine specific antibodies. Secreted cytokines then bind the antibody array; this is followed by incubation with a fluorescent secondary antibody that can be detected with a fluorescence scanner. RNA sequences have been simultaneously analysed, thereby linking the secretome with the transcriptome. Another method for simultaneous detection of proteins and transcriptomes in single cells is proximity extension assays (PEA)83 together with RNA detection.50 PEA is an immunoassay in which proteins are quantified with oligonucleotides associated with antibodies. The antibodies bind different epitopes and form a DNA duplex, which is quantified by real-time qPCR. One such method has been presented by Genshaft et al., who have focused on the correlation and expression of proteins and the transcriptome over time by using the Fluidigm C1 platform.84 The authors have detected as many as 27 different intracellular proteins in MCF7 cells, a breast cancer cell line. Similarly, Gong et al. have used PEA for simultaneous detection of 31 intracellular proteins and transcriptome profiling with the Fluidigm C1 platform.85 Their scatter plot analysis has shown a significant correlation between protein and mRNA levels for several genes.
3.4 Combining genomic and chromatin accessibility
Satpathy et al. have reported combined targeted genomic sequencing and chromatin expression. Using ATAC-seq (ATAC-seq) for chromatin accessibility combined with sequencing of genes encoding for the T-cell receptor, the authors have identified T cell heterogeneity in in populations that may appear identical according to surface markers.82
In another study, Cheow et al. have used the fluidigm C1 platform for single-cell genotype, expression and methylation (sc-GEM) analysis at different stages of primary lung adenocarcinomas and human fibroblast reprogramming. The authors have measured gene expression profiles with single cell RT-qPCR, and DNA methylation changes with the single cell restriction analysis of methylation (SCRAM) assay.59
4. Single cell multimodal analysis using microfluidics
Recently, the wave of multimodal measurements has further propagated beyond the omics view. The field is moving toward combining phenotypic analysis and omic measurements to obtain a more comprehensive picture of cell states and cell types (Fig. 3). In this section, we present currently available multimodal single cell platforms and compare them in terms of throughput, detection capability and field of application in Table 4.
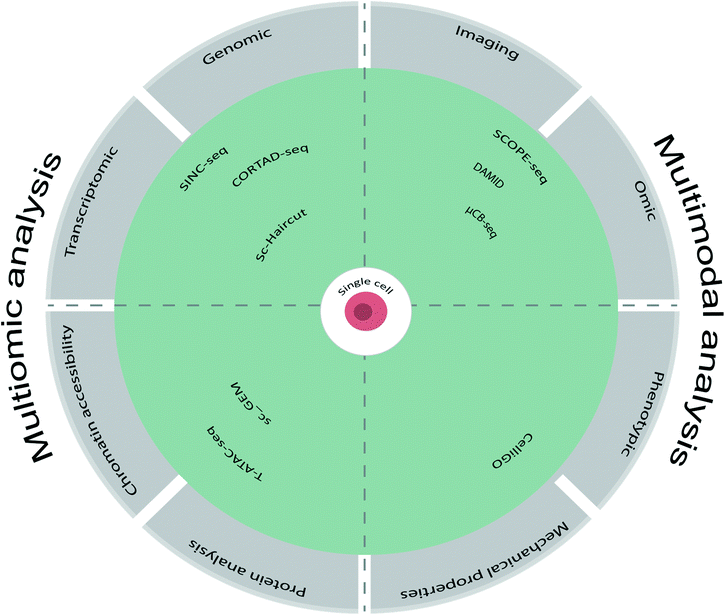 |
| Fig. 3 Schematic overview of multiomic analysis encompassing protein analysis, chromatin accessibility, transcriptomic and genomic analysis. Multimodal analysis has a broader meaning, encompassing other cell characteristics such as cell phenotypic analysis, imaging and cell mechanical properties. Specific examples of the techniques using such analysis are described below each section. | |
Table 4 Summary of multimodal single cell analysis technologies using microfluidic platforms
Parameter 1 |
Parameter 2 |
Method |
Microfluidic platform |
Biological model |
Throughput (number of cells) |
Max, number of analytes (per run) |
Applications |
Ref. |
Optical phenotype |
Transcriptomics |
SCOPE-Seq |
Microwell-based microfluidics |
A mix of human cell U87 line and mouse cell line 3 T3 |
30 500 |
∼3600 transcripts, 19 imaging features |
Simultaneous detection of live cell imaging and scRNA-seq |
49
|
Optical phenotype |
Transcriptomics |
|
Droplet and nanowell-based microfluidics |
Human PBMCs, Jurkat and HEK293 human cell lines and 3 T3 mouse cell line |
∼10 000 |
17 500 and 15 200 transcripts from mouse and human cells |
Identification of cell states and subsets |
46
|
Optical phenotype |
Transcriptomics |
μCB-seq |
Valve-based microfluidics |
Human embryonic kidney cells HEK293T and human adipocyte precursor cells (preadipocytes) |
10 |
3008 transcripts |
High-resolution imaging and scRNA-seq |
47
|
Live-cell imaging |
Transcriptomics |
|
Fluidigm C1 microfluidic chip |
Macrophage-like cell line RAW264.7 |
96 |
∼400 000 reads per cell |
Live cell imaging combined with scRNA-seq to correlate NF-κB activation with the transcriptome |
48
|
Live-cell imaging |
Genomics |
DamID |
Valve-based microfluidics |
Embryonic kidney cells Hek293t |
10 |
|
Analyse protein and DNA interactions |
86
|
Cell imaging |
Proteomics |
Chip-DIA |
Hydrodynamic trapping |
Non-small-cell lung cancer PC-9 cells and chronic B cell leukemia cells |
10–100 |
∼1500 protein groups |
Streamlined platform for sensitive single cell protein detection |
87
|
Calcium imaging |
Transcriptomics |
|
Fluidigm C1 microfluidic chip |
Human neocortex sample and mouse embryonic cortical cells |
∼500 |
∼3118 transcripts |
Study on the cell type specificity and neurotransmitter signaling in the developing human neocortex |
88
|
Cellular mass, growth rate |
Transcriptomics |
sSMR |
Micochannel-based microfluidics |
Primary T cells, L1210, FL5.12 human cell lines and a patient-derived glioblastoma cell line BT159 |
120 cells per hour |
>4000 transcripts |
Identification of cancer cell heterogeneity in response to drugs |
89
|
CD47 immunofluorescence imaging |
Transcriptomics |
DISCO |
Electrode- and droplet- based digital microfluidics |
Murine melanoma B16 cell line, human glioblastoma U87 cell line, CRISPR-modified HAP1 cells |
Hundreds of cell contents per hour |
Immunofluorescence of CD47 expression, NGS and sanger sequencing |
Identification of genetic mutations in CD47 gene |
44
|
IgG activity |
Paired antibody V gene sequencing |
CelliGO |
Droplet-based microfluidics |
Primary mouse spleen B cells |
Hundreds of thousands of cells per hour |
|
Investigation of IgG repertoire by screening IgG-secreting cells in terms of antibody activity and sequence in response to both soluble and membrane-bound antigens |
91
|
Electrical |
Mechanical |
|
|
SUM159PT breast cancer cell lines |
Hundreds of thousands of cells per hour |
|
Real time measurements on biophysical parameters of single cells. |
92
|
4.1 Combining omics and imaging
Fluorescence imaging is a widely used method for phenotypic analysis of cell morphology and cell specific molecules. One approach to obtaining single cell multimodal data involves coupling live-cell imaging to transcriptomic data. Yuan et al. demonstrated this by using dual-barcoded beads. After single-cell imaging in microwells, the dual-barcoded beads are added (Fig. 4). The dual barcode consists of commercially available drop-seq beads for scRNA-seq, to which additional oligonucleotides are added to serve as a second “optical” barcode. The sequence of the additional oligonucleotides can be optically decoded through several rounds of hybridization and fluorescence imaging of the beads. This process allows for spatially locating the beads and cells in the microwell array; thereby single cell imaging can be associated with sequencing data.49
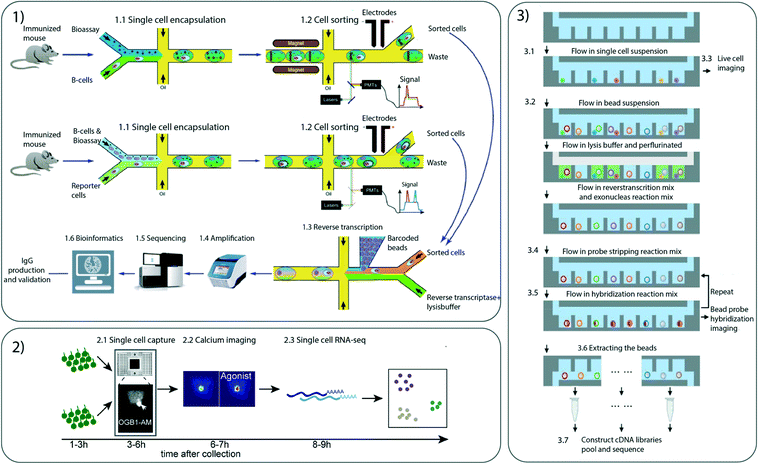 |
| Fig. 4 Different microfluidic multimodal single cell techniques 1) Droplet microfluidic platform for screening of IgG-secreting single cells and to characterize antibody binding to both membrane-bound and secreted antigens. 1.1) Droplet production 1.2) cell sorting 1.3) reverse transcription 1.4) amplification 1.5) sequencing 1.6) bioinformatics. 2) Microfluidic integrated chip for separating single cells and perform calcium imaging 2.1) single cell capture 2.2) calcium imaging 2.3) scRNA-seq. 3) SCOPE-seq platform developed to analyse phenotype and scRNA-seq using a microwell approach. 3.1) Loading of the cell suspension placing a single cell in a well 3.2) bead solution is added 3.3) cell imaging 3.4) Adding of probe stripping reaction mix 3.5) adding of probe hybridization reaction mix 3.6) beads are extracted and cDNA libraries are constructed. Images taken with permission from Gérard et al., Mayer et al. and Yuan et al. | |
In another approach to study genotype–phenotype relationships, Zhang et al. have performed parallel optical phenotyping and transcriptomic analysis.46 The platform combines droplet microfluidics with a nano-well array and has achieved a throughput of 10
000 cells. Cells and beads are co-encapsulated in droplets for RNA-seq. However, the fluorescence intensity of the droplet is interrogated beforehand. Droplets of interest are then dispensed at indexed locations on a nanowell array. The array is indexed by an oligobarcode that allows downstream RNA-seq to be linked to the fluorescence measurements.
Similarly, Chen et al. have reported microfluidic cell barcoding and sequencing (μCB-seq) to pair high-resolution imaging and scRNA-seq. μCB-seq consists of parallel valves fabricated by combining multilayer soft lithography and DNA array printing. The valves can be pre-loaded with barcoded primers for capturing reverse-transcribed cDNA. When the fluidic valves are closed, the cells are imaged and sorted to enable the enrichment of cells of interest.47
In another study, parallel measurements of transcriptome and proteome have been analysed using live cell confocal imaging to display cell size and shape as well as NF-κB expression levels after LPS stimulation in RAW 264-7 cells.48
Because of the vast use of scRNA-seq technologies in recent years, many multimodal approaches have involved this technique. However, several studies have instead focused on other analyses to branch out from this context. For example, Altemose et al. have investigated protein and DNA interactions by combining image analysis with sequencing. By using a DNA adenine methyltransferase enzyme, the authors have spatially localized histone modifications and obtained insights into the regulatory relationship between the DNA and specific histone modifications.86 In another recent approach Gebreyesus et al. have developed a platform for single cell counting, imaging and proteomic processing.87 This platform is capable of distinguishing different cell types based on size as well as sensitive protein detection using all-in-one proteomic sample preparation and data- independent acquisition (DIA) mass spectrometry (MS) for proteomic analysis.
4.2 Combining omics and phenotypic analysis
Cells from various tissues within an organism display phenotypic heterogeneity, owing to both genetic and epigenetic factors. Mayer et al. have reported cell diversity in the developing human neocortex with a microfluidic integrated fluidic circuit consisting of flow and control channels, valves, multiplexors and logic devices. This method detects physiological responsiveness by using intracellular calcium as a stimulus to analyse series of neurotransmitter receptor agonists that are later correlated with scRNA-seq data. Physiological reactions to calcium stimuli associate with the molecular identity, thereby allowing new sub-populations of cells to be detected that could otherwise not be identified (Fig. 4).88
Kimmerling et al. have presented a method for analysing cellular mass and growth in single cells to investigate the link between cell fitness and responses to a drug treatment.89 By using small fluidic channels, cells are separated with respect to buoyancy, and the cell mass is measured with a sensor array. The cells can be analysed gradually by simply turning on and off the flow. The phenotypic data are later associated with scRNA-seq from the same cell.
Microfluidic chips can be integrated with electronic circuits to allow for easy manipulation of liquids.90 Recently, Lamanna et al. have performed phenotypic and omic analyses by using digital microfluidics of single cells for -omics (DISCO) capture. Cells can be easily manipulated and lysed by laser capture microdissection inside the digital microfluidic chip. CD47 CRISPR-modified HAP1 cells have been analyse for phenotypic changes in CD47 expression, combined with genomics, transcriptomics or proteomics.44
4.3 Other multimodal analysis
Other studies have analysed cells for multiple parameters simultaneously without including omic analysis. Gérard et al. have presented an efficient and flexible system that combines screening for IgG activity with sequencing of paired antibody V genes through droplet-based microfluidic techniques.91 The droplets containing antibodies of interest are sorted with a sandwich immune assay based on fluorescent and magnetic beads. The sorted droplets are then barcoded with hydrogel beads bearing primers for IgHV and VL genes tagged with unique barcodes, and the barcoded V genes are sequenced and analysed (Fig. 4). The analyses have been applied in a murine setting but could easily be adapted to humans. For example, by enriching human circulating B cells from peripheral blood, the platform can facilitate analysis of the human immune V gene repertoire in diseased and healthy humans and thus may have diagnostic potential.
Another recent approach for detecting biophysical properties of single cells was developed by Rezard et al.92 By using micro-electro mechanical systems (MEMS) cells could be trapped and analysed for mechanical and electrical properties in real time during continuous flow.
5. Technological developments and clinical transitions
Despite its numerous achievements, MSCA is still in its infancy, with many challenges to overcome. The improvement needed for MSCA include both assay development as well as technological development. Challenges in assay development include reaching limit of detection due to the limited sample volume, low genomic coverage and low sensitivity of analytical methods.
Technological development faces challenges such as correct data alignment of multiple cellular parameters, accounting for high degrees of missing data and inherent assay noise. Recently developed techniques, such as Cobolt,93 MOFA+94 and weighted-nearest neighbour,75 are capable of analysing multimodal datasets. Another recent method, bridge integration, offers supervised mapping that utilize well-known cellular atlases such as the human cell atlas as a reference.95 By mapping datasets to these reference data points, potential errors can easily be adjusted for and datasets across different cell parameters can easily be compared.
5.1 Technological developments for MSCA
With new technologies being constantly developed, multimodal analysis is exploding. However, there are multiple different cellular properties that are yet to be integrated with multimodal measurements. As a perspective, we highlight here the analysis of biophysical properties of the cells, yet to be better implemented with other techniques for multimodal analysis.96 Those biophysical properties are of relevance as they have been associated with the progression of cell differentiation and diseases.
Mechanical measurements of cell stiffness and physical resistance to external forcing have been demonstrated using various actuation modes. Pipette aspiration97 with a low throughput capacity or parallel arrays of constrictions in microchannels98,99 with a larger throughput capacity have been used to explore the mechanics of the cell and even the response of the nucleus. The use of soft compartments also proved a powerful tool to relate mechanical stresses on cells to increased motility, of relevance to study the dynamics of tumor evolution,100 and new microfluidic devices have been developed to investigate the effect of compressive stress on cell growth.101
Optical and magnetic tweezers have been developed for mechanical phenotyping.102,103 Both approaches require a dielectric or magnetic bead to be attached to the cell. By applying a controlled oscillating field, by the use of a tightly focused laser or a magnetic field, the bead is moved in response to the field. The oscillatory motion of the bead causes cell deformation. The resistance against this deformation is determined by the mechanical properties of the cell: the viscoelastic moduli of entangled actin networks were measured,104 and cytoskeletal changes for prostate cancer cells under hypoxic stress were observed.105 A more recent development based on the magnetic tweezers is a method named magnetic twisting cytometry.106 Here the applied magnetic field move and rotate the bead around different axes to determine the mechanical properties of the cell. Another mechanical single cell study was performed by precise microfabrication of microactuators. A muscle cell was attached to the micropillar and it was illustrated that the extension and contraction of this cell, induced by chemical stimuli, was able to bend the micropillar.107 These methods are in general limited in throughput and are invasive for the cell as the binding to a bead might limit its further use in a multimodal approach.
Non-contact methods offer interesting potential. Acoustic methods for mechanical phenotyping use standing acoustic waves to generate an acoustic radiation force on the cell that moves it to the pressure nodes.108 The velocity at which the cell moves is used to extract its compressibility. A novel development in this method utilizes standing acoustic waves together with an acoustic impedance gradient in a microchannel.109 Due to the acoustic radiation force, cells migrate to the equilibrium point in the microchannel, the point where its acoustic impedance matches the surroundings. This method works similar as density gradient centrifugation and is size insensitive. Overall, the combination of label free and high throughput methods for mechanical characterizations of the cells have a potential for implementation in a multimodal approach.
5.2 Perspective techniques for MSCA
Another approach to MSCA is to perform single cell analysis on one cell parameter and later integrated other cellular parameters from different experiments. However, the correspondence between individual cells is absent when data from multiple experiments derived from different cell populations are incorporated. The methods presented for these analyses have great potential to be transformed into multimodal analysis. For example, Zhong et al. have investigated various cell types, cell linages, molecular features and transcriptional regulation of the developing human hippocampus.110 To understand the molecular features of developing hippocampal cells, the authors performed scRNA-seq and chromatin analysis with the 10× chromium platform and ATAC-seq, respectively. They identified spatially specific gene expression in both the pre-frontal cortex and the hippocampus, which could be used as a tool for investigating other short-term or long-term memory related diseases. In another study exploring neural cell heterogeneity, Lake et al. have analysed single-cell transcriptional and epigenetic states in the human adult brain with droplet microfluidic methods.111 Through scRNA-seq and epigenetic states on separate samples, the data sets can be computationally integrated.
To reveal additional spatial relationships between cells multimodal measurements have been performed on tissue samples. Using microfluidic methods for adding barcode beads to the sample, simultaneous mapping of mRNAs and proteins has been performed directly on the tissue sample.112
5.3 Clinical perspectives: current and future applications
As described above, single cell multimodal analysis allows for biological differences between individual cells to be inferred, and has thus broadened knowledge of cellular heterogeneity and its implications in cell function. Single cell multimodal analysis could substantially advance clinical research and applications, particularly regarding early diagnostics and disease monitoring, by tracking the precise composition and distribution of specific cell populations with the cellular changes. Several projects have integrated MSCA platforms to analyse patient-derived samples, an overview of the recent progress is in oncology, immunology and neurology presented below.
5.3.1 Oncology.
Tumors are composed of a large variety of cell types and cell states. Because of both inter- and intratumor heterogeneity, therapy resistance and tumor relapse remain the greatest challenges in cancer treatments.113 At the single cell level, cells have different drug treatment responses, and even a small number of resistant cells in a tumor can affect treatment outcomes. Because therapy resistant cells may be rare in tumors,114 highly sensitive methods are urgently needed for detecting these cells. Single cell multimodal analysis has the unique potential to reveal the biological variations within the tumor115 and to relate specific acquired mutations to molecular outcomes, thereby enabling the detection of therapy resistant cells at an early stage and subsequent rapid diagnosis of medical outcomes.116
Multimodal analysis can also be used to create detailed lineage trees of cancer cell evolution.117 By reconstructing cell lineage trees according to the extent to which cells share somatic variations, the understanding of how tumors evolve over time and the prediction of treatment outcomes can be improved. As a result, more specific and efficient treatments will be possible for patients. Projects such as The Human Tumor Atlas Network118 and CancerSEA119 aim at identifying cancer cell heterogeneity to create single-cell longitudinal atlases. The Human Tumor Atlas Network will use omic analyses and spatial multiplex in situ methods on patient derived tumors to create accessible three-dimensional atlases of multiple different sets of cancers. By studying genetic differences and integrating them with clinical outcomes, these projects aim to identify new biomarkers or therapeutically relevant cell types. The CancerSEA database focuses on decoding functional states of cancer cells at the single-cell level by using single cell transcriptomic data. These methods may serve as key stepping-stones for the ambitious goal of mapping multimodal data in cancer research.
5.3.2 Immunology.
The immune system is highly complex and consists of many types of cells, which have previously been defined primarily through cell surface markers and scRNA-seq.120 Although cell surface markers provide a good indication of the protein expression of a cell, a complete picture with multiple cellular parameters is desirable to understand the immune system in depth.121 For example, lymphoid cells communicate with their environment in a variety of ways, including cell–cell interactions and recruitment of new cells through chemokine secretion. A variety of cell types are known by their specific functions in this ecosystem, but only bulk data on the secretome are available, whereas cell–cell variations and their effects are underexplored to date. By culturing cells in individual compartments in multimodal analysis platforms, not only RNA and intracellular proteins but also individual secretomes, then transcriptomes or genomes can be analysed.
Unterman et al. have analysed the peripheral lymphocyte population in patients with COVID-19. Using CITE-seq surface proteins on lymphocytes, B cell and T cells receptors have been analysed together with scRNA-seq. The authors concluded that both B cells and T cells have an altered distribution in the primary response to COVID-19.122
5.3.3 Neurology.
MSCA have the potential to unravel complex nervous system interactions and discover new cell-signaling mechanisms controlling functions within the central nervous system. Furthermore, studying cellular development and differentiation will also be possible by tracking different cell trajectories, to predict cell lineages and visualize how these diverse cells are organized within neural tissues.123 Another possible parameter that may be integrated in neurology is the analysis of cell electrophysiological properties to identify new subclasses of cells and establish cellular heterogeneity.124 As described above, Mayer et al. have analysed cell diversity in the developing human neocortex and have found new sub-populations of cells.88 These types of approaches are key stepping-stones in assessing the full complexity of the nervous system.
5.4 Challenges for transitioning MSCA to clinical research
Several challenges must be addressed before single cell multimodal analyses can be used in clinical applications. First, an emerging need exists to develop guidelines and workflows specifically for clinical settings, and to minimize variations in sample quality due to sample collection and preservation procedures, for which standardized protocols and specific sample clean-up steps are required. Other key aspects in translating single cell multimodal analysis to clinical research include achieving high analysis sensitivity and high throughput while minimizing costs. Crucially, multimodal data must be properly analysed, and computational methods specifically tailored for analysing multiplexed data must be developed.125
6. Conclusion
Single cell analysis have recently benefited from the technologies developed to simultaneously determine multiple parameters of individual cells. These developments are leading to unprecedented insight into cell fate decisions, genotype–phenotype relationships and cell heterogeneity. However, simultaneously evaluating multiple aspects of single cells remains difficult with several limitations still to be overcome: low genomic coverage, limited sample volume and low sensitivity of analytical methods. Microfluidic technologies combine advantages such as precise manipulation of fluids, automated liquid handling, high throughput and the potential for coupling to different analytical measuring systems. The development of microfluidic devices and their applications currently provides and will continue to provide new research methodologies with unprecedented accuracy for single cell analysis. These technologies are therefore likely to shape the future direction of multimodal analysis with a potential for translation into clinical research in years to come.
Conflicts of interest
V. Taly and J.-C. Baret are co-founders and scientific advisors of emulseo.
Acknowledgements
This work is part of a project that has received funding from the European Union's Horizon 2020 research and innovation programme under the Marie Skłodowska-Curie grant agreement No 813786. W. Xiao received funding from the SIRIC CARPEM (CAncer Research and Personalized Medicine) and the ITMO CANCER through the program single cell COCAHEMISCLE. The work was conducted with the support of the Ligue Nationale Contre le Cancer (LNCC, Program “Equipe labelisée LIGUE”; no. EL2016. LNCC). This work was also supported by the Ministère de l'Enseignement Supérieur et de la Recherche, the Université Paris-Descartes, the Centre National de la Recherche Scientifique (CNRS), the Institut National de la Santé et de la Recherche Médicale (INSERM) and Canceropole Ile-de-France.
References
- E. Z. Macosko, Highly Parallel Genome-wide Expression Profiling of Individual Cells Using Nanoliter Droplets, Cell, 2015, 161(5), 1202–1214 CrossRef CAS PubMed.
- C. Gawad, W. Koh and S. R. Quake, Dissecting the clonal origins of childhood acute lymphoblastic leukemia by single-cell genomics, Proc. Natl. Acad. Sci. U. S. A., 2014, 111(50), 17947–17952 CrossRef CAS PubMed.
- N. Navin, J. Kendall, J. Troge, P. Andrews, L. Rodgers and J. McIndoo,
et al., Tumour evolution inferred by single-cell sequencing, Nature, 2011, 472(7341), 90–94 CrossRef CAS PubMed.
- T. Nagano,
et al., Cell-cycle dynamics of chromosomal organization at single-cell resolution, Nature, 2017, 547(7661), 61–67 CrossRef CAS PubMed.
- A. M. Klein, L. Mazutis, I. Akartuna, N. Tallapragada, A. Veres and V. Li,
et al., Droplet Barcoding for Single-Cell Transcriptomics Applied to Embryonic Stem Cells, Cell, 2015, 161(5), 1187–1201 CrossRef CAS PubMed.
- S. Guo, C. Zhang and A. Le, Curr. Opin. Biotechnol., 2021, 71, 115–122 CrossRef CAS PubMed.
- A. J. Hughes, D. P. Spelke, Z. Xu, C. C. Kang, D. V. Schaffer and A. E. Herr, Single-cell western blotting, Nat. Methods, 2014, 11(7), 749–755 CrossRef CAS PubMed.
- L. Qiu, F. Wimmers, J. Weiden, H. A. Heus, J. Tel and C. G. Figdor, A membrane-anchored aptamer sensor for probing IFNc secretion by single cells, Chem. Commun., 2017, 53(57), 8066–8069 RSC.
- G. Chen, B. Ning and T. Shi, Single-Cell RNA-Seq Technologies and Related Computational Data Analysis, Front. Genet., 2019, 10, 317 CrossRef CAS PubMed.
- J. Lee, D. Y. Hyeon and D. Hwang, Single-cell multiomics: technologies and data analysis methods, Exp. Mol. Med., 2020, 52(9), 1428–1442 CrossRef CAS PubMed.
- S. W. Lin, C. H. Chang and C. H. Lin, High-throughput Fluorescence Detections in Microfluidic Systems, Genomic Med., Biomarkers, Health Sci., 2011, 3(1), 27–38 CrossRef.
- M. Sesen and G. Whyte, Image-Based Single Cell Sorting Automation in Droplet Microfluidics, Sci. Rep., 2020, 10(1), 8736 CrossRef CAS PubMed.
- Q. Xiong, C. Y. Lim, J. Ren, J. Zhou, K. Pu and M. B. Chan-Park,
et al., Magnetic nanochain integrated microfluidic biochips, Nat. Commun., 2018, 9(1), 1743 CrossRef PubMed.
- R. R. Svejdal, D. Sticker, C. Sønderby, J. P. Kutter and K. D. Rand, Thiol-ene microfluidic chip for fast on-chip sample clean-up, separation and ESI mass spectrometry of peptides and proteins, Anal. Chim. Acta, 2020, 1140, 168–177 CrossRef CAS PubMed.
- J. Sandberg, B. Werne, M. Dessing and J. Lundeberg, Rapid flow-sorting to simultaneously resolve multiplex massively parallel sequencing products, Sci. Rep., 2011, 1, 108 CrossRef CAS PubMed.
- E. Denisenko, B. B. Guo, M. Jones, R. Hou, L. de Kock and T. Lassmann,
et al., Systematic assessment of tissue dissociation and storage biases in single-cell and single-nucleus RNA-seq workflows, Genome Biol., 2020, 21(1), 130 CrossRef CAS PubMed.
- R. Hanamsagar, T. Reizis, M. Chamberlain, R. Marcus, F. O. Nestle and E. de Rinaldis,
et al., An optimized workflow for single-cell transcriptomics and repertoire profiling of purified lymphocytes from clinical samples, Sci. Rep., 2020, 10(1), 2219 CrossRef CAS PubMed.
- M. D. Young and S. Behjati, SoupX removes ambient RNA contamination from droplet-based single-cell RNA sequencing data, Gigascience, 2020, 9(12), giaa151 CrossRef PubMed.
- H. Tavakoli, W. Zhou, L. Ma, S. Perez, A. Ibarra and F. Xu,
et al., Recent advances in microfluidic platforms for single-cell analysis in cancer biology, diagnosis and therapy, TrAC, Trends Anal. Chem., 2019, 117, 13–26 CrossRef CAS PubMed.
- P. Shinde, L. Mohan, A. Kumar, K. Dey, A. Maddi and A. Patananan,
et al., Current Trends of Microfluidic Single-Cell Technologies, Int. J. Mol. Sci., 2018, 19(10), 3143 CrossRef PubMed.
- J. Wang, H. C. Fan, B. Behr and S. R. Quake, Genome-wide Single-Cell Analysis of Recombination Activity and De Novo Mutation Rates in Human Sperm, Cell, 2012, 150(2), 402–412 CrossRef CAS PubMed.
- M. A. Unger, Monolithic Microfabricated Valves and Pumps by Multilayer Soft Lithography, Science, 2000, 288(5463), 113–116 CrossRef CAS PubMed.
- J. Yuan and P. A. Sims, An Automated Microwell Platform for Large-Scale Single Cell RNA-Seq, Sci. Rep., 2016, 6(1), 33883 CrossRef CAS PubMed.
- T. M. Gierahn, M. H. Wadsworth, T. K. Hughes, B. D. Bryson, A. Butler and R. Satija,
et al., Seq-Well: portable, low-cost RNA sequencing of single cells at high throughput, Nat. Methods, 2017, 14(4), 395–398 CrossRef CAS PubMed.
- R. Zilionis, J. Nainys, A. Veres, V. Savova, D. Zemmour and A. M. Klein,
et al., Single-cell barcoding and sequencing using droplet microfluidics, Nat. Protoc., 2017, 12(1), 44–73 CrossRef CAS PubMed.
- D. J. Collins, A. Neild, A. deMello, A. Q. Liu and Y. Ai, The Poisson distribution and beyond: methods for microfluidic droplet production and single cell encapsulation, Lab Chip, 2015, 15(17), 3439–3459 RSC.
- Y. Bai, X. He, D. Liu, S. N. Patil, D. Bratton and A. Huebner,
et al., A double droplet trap system for studying mass transport across a droplet-droplet interface, Lab Chip, 2010, 10(10), 1281 RSC.
- J. G. Riess and M. P. Krafft, Fluorinated materials for in vivo oxygen transport (blood substitutes), diagnosis and drug delivery, Biomaterials, 1998, 19(16), 1529–1539 CrossRef CAS PubMed.
- P. Gruner, B. Riechers, L. A. Chacòn Orellana, Q. Brosseau, F. Maes and T. Beneyton,
et al., Stabilisers for water-in-fluorinated-oil dispersions: Key properties for microfluidic applications, Curr. Opin. Colloid Interface Sci., 2015, 20(3), 183–191 CrossRef CAS.
- A. R. Abate, T. Hung, P. Mary, J. J. Agresti and D. A. Weitz, High-throughput injection with microfluidics using picoinjectors, Proc. Natl. Acad. Sci. U. S. A., 2010, 107(45), 19163–19166 CrossRef CAS PubMed.
- C. Priest, S. Herminghaus and R. Seemann, Controlled electrocoalescence in microfluidics: Targeting a single lamella, Appl. Phys. Lett., 2006, 89(13), 134101 CrossRef.
- D. R. Link, S. L. Anna, D. A. Weitz and H. A. Stone, Geometrically Mediated Breakup of Drops in Microfluidic Devices, Phys. Rev. Lett., 2004, 92(5), 054503 CrossRef CAS PubMed.
- L. Mazutis, J. Gilbert, W. L. Ung, D. A. Weitz, A. D. Griffiths and J. A. Heyman, Single-cell analysis and sorting using droplet-based microfluidics, Nat. Protoc., 2013, 8(5), 870–891 CrossRef CAS PubMed.
- D. Di Carlo, N. Aghdam and L. P. Lee, Single-Cell Enzyme Concentrations, Kinetics, and Inhibition Analysis Using High-Density Hydrodynamic Cell Isolation Arrays, Anal. Chem., 2006, 78(14), 4925–4930 CrossRef CAS PubMed.
- S. Bhattacharya, T. C. Chao, N. Ariyasinghe, Y. Ruiz, D. Lake and R. Ros,
et al., Selective trapping of single mammalian breast cancer cells by insulator-based dielectrophoresis, Anal. Bioanal. Chem., 2014, 406(7), 1855–1865 CrossRef CAS PubMed.
- C. Liberale, G. Cojoc, F. Bragheri, P. Minzioni, G. Perozziello and R. La Rocca,
et al., Integrated microfluidic device for single-cell trapping and spectroscopy, Sci. Rep., 2013, 3(1), 1258 CrossRef CAS PubMed.
- D. J. Collins, B. Morahan, J. Garcia-Bustos, C. Doerig, M. Plebanski and A. Neild, Two-dimensional single-cell patterning with one cell per well driven by surface acoustic waves, Nat. Commun., 2015, 6(1), 8686 CrossRef CAS PubMed.
- J. Clausell-Tormos, D. Lieber, J. C. Baret, A. El-Harrak, O. J. Miller and L. Frenz,
et al., Droplet-Based Microfluidic Platforms for the Encapsulation and Screening of Mammalian Cells and Multicellular Organisms, Chem. Biol., 2008, 15(5), 427–437 CrossRef CAS PubMed.
- P. K. Periyannan Rajeswari, H. N. Joensson and H. Andersson-Svahn, Droplet size influences division of mammalian cell factories in droplet microfluidic cultivation: Microfluidics and Miniaturization, Electrophoresis, 2017, 38(2), 305–310 CrossRef CAS PubMed.
- T. Zhang, H. Zhang, W. Zhou, K. Jiang, C. Liu and R. Wang,
et al., One-Step Generation and Purification of Cell-Encapsulated Hydrogel Microsphere With an Easily Assembled Microfluidic Device, Front. Bioeng. Biotechnol., 2022, 9, 816089 CrossRef PubMed.
- L. Mahler, M. Tovar, T. Weber, S. Brandes, M. M. Rudolph and J. Ehgartner,
et al., Enhanced and homogeneous oxygen availability during incubation of microfluidic droplets, RSC Adv., 2015, 5(123), 101871–101878 RSC.
- N. de Lange, T. M. Tran and A. R. Abate, Electrical lysis of cells for detergent-free droplet assays, Biomicrofluidics, 2016, 10(2), 024114 CrossRef CAS PubMed.
- H. Shintaku, H. Nishikii, L. A. Marshall, H. Kotera and J. G. Santiago, On-Chip Separation and Analysis of RNA and DNA from Single Cells, Anal. Chem., 2014, 86(4), 1953–1957 CrossRef CAS PubMed.
- J. Lamanna, E. Y. Scott, H. S. Edwards, M. D. Chamberlain, M. D. M. Dryden and J. Peng,
et al., Digital microfluidic isolation of single cells for -Omics, Nat. Commun., 2020, 11(1), 5632 CrossRef CAS PubMed.
- R. Marie, M. Pødenphant, K. Koprowska, R. C. M. Vulders, J. Wilding and N. Ashley,
et al., Sequencing of human genomes extracted from single cancer cells isolated in a valveless microfluidic device, Lab Chip, 2018, 12, 1891–1902 RSC.
- J. Q. Zhang, C. A. Siltanen, L. Liu, K. C. Chang, Z. J. Gartner and A. R. Abate, Linked optical and gene expression profiling of single cells at high-throughput, Genome Biol., 2020, 21(1), 49 CrossRef CAS PubMed.
- T. N. Chen, A. Gupta, M. D. Zalavadia and A. Streets, μCB-seq: microfluidic cell barcoding and sequencing for high-resolution imaging and sequencing of single cells, Lab Chip, 2020, 20(21), 3899–3913 RSC.
- K. Lane, D. Van Valen, M. M. DeFelice, D. N. Macklin, T. Kudo and A. Jaimovich,
et al., Measuring Signaling and RNA-Seq in the Same Cell Links Gene Expression to Dynamic Patterns of NF-κB Activation, Cell Syst., 2017, 4(4), 458–469.e5 CrossRef CAS PubMed.
- J. Yuan, J. Sheng and P. A. Sims, SCOPE-Seq: a scalable technology for linking live cell imaging and single-cell RNA sequencing, Genome Biol., 2018, 19(1), 227 CrossRef CAS PubMed.
- K. Taniguchi, T. Kajiyama and H. Kambara, Quantitative analysis of gene expression in a single cell by qPCR, Nat. Methods, 2009, 6(7), 503–506 CrossRef CAS PubMed.
- Y. Hou, H. Guo, C. Cao, X. Li, B. Hu and P. Zhu,
et al., Single-cell triple omics sequencing reveals genetic, epigenetic, and transcriptomic heterogeneity in hepatocellular carcinomas, Cell Res., 2016, 26(3), 304–319 CrossRef CAS PubMed.
- Q. R. Xing, N. O. Cipta, K. Hamashima, Y. C. Liou, C. G. Koh and Y. H. Loh, Unraveling Heterogeneity in Transcriptome and Its Regulation Through Single-Cell Multi-Omics Technologies, Front. Genet., 2020, 11, 662 CrossRef CAS PubMed.
- L. Han, X. Zi, L. X. Garmire, Y. Wu, S. M. Weissman and X. Pan,
et al., Co-detection and sequencing of genes and transcripts from the same single cells facilitated by a microfluidics platform, Sci. Rep., 2015, 4, 6485 CrossRef PubMed.
- S. Sohrabi, N. Kassir and M. K. Moraveji, Droplet microfluidics: fundamentals and its advanced applications, RSC Adv., 2020, 10(46), 27560–27574 RSC.
- S. M. Prakadan, A. K. Shalek and D. A. Weitz, Scaling by shrinking: empowering single-cell “omics” with microfluidic devices, Nat. Rev. Genet., 2017, 18(6), 345–361 CrossRef CAS PubMed.
- S. Chen, B. B. Lake and K. Zhang, High-throughput sequencing of the transcriptome and chromatin accessibility in the same cell, Nat. Biotechnol., 2019, 37(12), 1452–1457 CrossRef CAS PubMed.
- A. R. Abate, C. H. Chen, J. J. Agresti and D. A. Weitz, Beating Poisson encapsulation statistics using close-packed ordering, Lab Chip, 2009, 9(18), 2628 RSC.
- Q. R. Xing, C. A. E. Farran, Y. Y. Zeng, Y. Yi, T. Warrier and P. Gautam,
et al., Parallel bimodal single-cell sequencing of transcriptome and chromatin accessibility, Genome Res., 2020, 30(7), 1027–1039 CrossRef CAS PubMed.
- L. F. Cheow, E. T. Courtois, Y. Tan, R. Viswanathan, Q. Xing and R. Z. Tan,
et al., Single-cell multimodal profiling reveals cellular epigenetic heterogeneity, Nat. Methods, 2016, 13(10), 833–836 CrossRef CAS PubMed.
- I. Hajji, M. Serra, L. Geremie, I. Ferrante, R. Renault and J. L. Viovy,
et al., Droplet microfluidic platform for fast and continuous-flow RT-qPCR analysis devoted to cancer diagnosis application, Sens. Actuators, B, 2020, 303, 127171 CrossRef CAS.
- D. W. Ruff, D. M. Dhingra, K. Thompson, J. A. Marin and A. T. Ooi, High-Throughput Multimodal Single-Cell Targeted DNA and Surface Using the Mission Bio Tapestri Platform, in: Single-Cell Protein Analysis, Methods Mol. Biol., 2022, 2386, 171–188 CrossRef PubMed.
- F. Tang, C. Barbacioru, Y. Wang, E. Nordman, C. Lee and N. Xu,
et al., mRNA-Seq whole-transcriptome analysis of a single cell, Nat. Methods, 2009, 6(5), 377–382 CrossRef CAS PubMed.
- J. Cao, The single cell transcriptional landscape of mammalian organogenesis, Nature, 2019, 566(7745), 496–502 CrossRef CAS PubMed.
- D. Hebenstreit, Methods, Challenges and Potentials of Single Cell RNA-seq, Biology., 2012, 1(3), 658–667 CrossRef CAS PubMed.
- V. Svensson, R. Vento-Tormo and S. A. Teichmann, Exponential scaling of single-cell RNA-seq in the past decade, Nat. Protoc., 2018, 13(4), 599–604 CrossRef CAS PubMed.
- J. D. Buenrostro, B. Wu, H. Y. Chang and W. J. Greenleaf, ATAC-seq: A Method for Assaying Chromatin Accessibility Genome-Wide, Curr. Protoc. Mol. Biol., 2015, 109, 21.29.1 Search PubMed.
- J. Cao, D. A. Cusanovich, V. Ramani, D. Aghamirzaie, H. A. Pliner and A. J. Hill,
et al., Joint profiling of chromatin accessibility and gene expression in thousands of single cells, Science, 2018, 361(6409), 1380–1385 CrossRef CAS PubMed.
- A. J. Rubin, K. R. Parker, A. T. Satpathy, Y. Qi, B. Wu and A. J. Ong,
et al., Coupled Single-Cell CRISPR Screening and Epigenomic Profiling Reveals Causal Gene Regulatory Networks, Cell, 2019, 176(1–2), 361–376.e17 CrossRef CAS PubMed.
- K. Kuriyama, H. Shintaku and J. G. Santiago, Isotachophoresis for fractionation and recovery of cytoplasmic RNA and nucleus from single cells, Electrophoresis, 2015, 36(14), 1658–1662 CrossRef CAS PubMed.
- M. N. Abdelmoez, K. Iida, Y. Oguchi, H. Nishikii, R. Yokokawa and H. Kotera,
et al., SINC-seq: correlation of transient gene expressions between nucleus and cytoplasm reflects single-cell physiology, Genome Biol., 2018, 19(1), 66 CrossRef PubMed.
- A. L. Richer, K. A. Riemondy, L. Hardie and J. R. Hesselberth, Simultaneous measurement of biochemical phenotypes and gene expression in single cells, Nucleic Acids Res., 2020, 48(10), e59 CrossRef CAS PubMed.
- S. L. Kong, H. Li, J. A. Tai, E. T. Courtois, H. M. Poh and D. P. Lau,
et al., Concurrent Single-Cell RNA and Targeted DNA Sequencing on an Automated Platform for Comeasurement of Genomic and Transcriptomic Signatures, Clin. Chem., 2019, 65(2), 272–281 CrossRef CAS PubMed.
- V. M. Peterson, K. X. Zhang, N. Kumar, J. Wong, L. Li and D. C. Wilson,
et al., Multiplexed quantification of proteins and transcripts in single cells, Nat. Biotechnol., 2017, 35(10), 936–939 CrossRef CAS PubMed.
- M. Stoeckius, C. Hafemeister, W. Stephenson, B. Houck-Loomis, P. K. Chattopadhyay and H. Swerdlow,
et al., Simultaneous epitope and transcriptome measurement in single cells, Nat. Methods, 2017, 14(9), 865–868 CrossRef CAS PubMed.
- Y. Hao, S. Hao, E. Andersen-Nissen, W. M. Mauck, S. Zheng and A. Butler,
et al., Integrated analysis of multimodal single-cell data, Cell, 2021, 184(13), 3573–3587.e29 CrossRef CAS PubMed.
- E. P. Mimitou, A. Cheng, A. Montalbano, S. Hao, M. Stoeckius and M. Legut,
et al., Multiplexed detection of proteins, transcriptomes, clonotypes and CRISPR perturbations in single cells, Nat. Methods, 2019, 16(5), 409–412 CrossRef CAS PubMed.
- F. Mair, J. R. Erickson, V. Voillet, Y. Simoni, T. Bi and A. J. Tyznik,
et al., A Targeted Multi-omic Analysis Approach Measures Protein Expression and Low-Abundance Transcripts on the Single-Cell Level, Cell Rep., 2020, 31(1), 107499 CrossRef CAS PubMed.
- J. M. Granja, S. Klemm, L. M. McGinnis, A. S. Kathiria, A. Mezger and M. R. Corces,
et al., Single-cell multiomic analysis identifies regulatory programs in mixed-phenotype acute leukemia, Nat. Biotechnol., 2019, 37(12), 1458–1465 CrossRef CAS PubMed.
- B. Budnik, E. Levy, G. Harmange and N. Slavov, SCoPE-MS: mass spectrometry of single mammalian cells quantifies proteome heterogeneity during cell differentiation, Genome Biol., 2018, 19(1), 161 CrossRef PubMed.
- A. M. Xu, Q. Liu, K. L. Takata, S. Jeoung, Y. Su and I. Antoshechkin,
et al., Integrated measurement of intracellular proteins and transcripts in single cells, Lab Chip, 2018, 18(21), 3251–3262 RSC.
- J. George and J. Wang, Assay of Genome-Wide Transcriptome and Secreted Proteins on the Same Single Immune Cells by Microfluidics and RNA Sequencing, Anal. Chem., 2016, 88(20), 10309–10315 CrossRef CAS PubMed.
- A. T. Satpathy, N. Saligrama, J. D. Buenrostro, Y. Wei, B. Wu and A. J. Rubin,
et al., Transcript-indexed ATAC-seq for precision immune profiling, Nat. Med., 2018, 24(5), 580–590 CrossRef CAS PubMed.
- S. Darmanis, C. J. Gallant, V. D. Marinescu, M. Niklasson, A. Segerman and G. Flamourakis,
et al., Simultaneous Multiplexed Measurement of RNA and Proteins in Single Cells, Cell Rep., 2016, 14(2), 380–389 CrossRef CAS PubMed.
- A. S. Genshaft, S. Li, C. J. Gallant, S. Darmanis, S. M. Prakadan and C. G. K. Ziegler,
et al., Multiplexed, targeted profiling of single-cell proteomes and transcriptomes in a single reaction, Genome Biol., 2016, 17(1), 188 CrossRef PubMed.
- H. Gong, X. Wang, B. Liu, S. Boutet, I. Holcomb and G. Dakshinamoorthy,
et al., Single-cell protein-mRNA correlation analysis enabled by multiplexed dual-analyte co-detection, Sci. Rep., 2017, 7(1), 2776 CrossRef PubMed.
- N. Altemose, A. Maslan, C. Rios-Martinez, A. Lai, J. A. White and A. Streets, μDamID: A Microfluidic Approach for Joint Imaging and Sequencing of Protein-DNA Interactions in Single Cells, Cell Syst., 2020, 11(4), 354–366.e9 CrossRef CAS PubMed.
- S. T. Gebreyesus, A. A. Siyal, R. B. Kitata, E. S. W. Chen, B. Enkhbayar and T. Angata,
et al., Streamlined single-cell proteomics by an integrated microfluidic chip and data-independent acquisition mass spectrometry, Nat. Commun., 2022, 13(1), 37 CrossRef CAS PubMed.
- S. Mayer, J. Chen, D. Velmeshev, A. Mayer, U. C. Eze and A. Bhaduri,
et al., Multimodal Single-Cell Analysis Reveals Physiological Maturation in the Developing Human Neocortex, Neuron, 2019, 102(1), 143–158.e7 CrossRef CAS PubMed.
- R. J. Kimmerling, S. M. Prakadan, A. J. Gupta, N. L. Calistri, M. M. Stevens and S. Olcum,
et al., Linking single-cell measurements of mass, growth rate, and gene expression, Genome Biol., 2018, 19(1), 207 CrossRef CAS PubMed.
- E. Samiei, M. Tabrizian and M. Hoorfar, A review of digital microfluidics as portable platforms for lab-on a-chip applications, Lab Chip, 2016, 16(13), 2376–2396 RSC.
- A. Gérard, A. Woolfe, G. Mottet, M. Reichen, C. Castrillon and V. Menrath,
et al., High-throughput single-cell activity-based screening and sequencing of antibodies using droplet microfluidics, Nat. Biotechnol., 2020, 8(6), 715–721 CrossRef PubMed.
- Q. Rezard, G. Perret, J. C. Gerbedoen, D. Pekin, F. Cleri and D. Collard,
et al., Developing A Mems Device for High-Throughput Multi-Parameter Single Cell Biophysical Analysis, in 2021 IEEE 34th International Conference on Micro Electro Mechanical Systems (MEMS). Gainesville, FL, USA, IEEE, 2021, 494–497 Search PubMed.
- B. Gong, Y. Zhou and E. Purdom, Cobolt: integrative analysis of multimodal single-cell sequencing data, Genome Biol., 2021, 22(1), 351 CrossRef CAS PubMed.
- R. Argelaguet, D. Arnol, D. Bredikhin, Y. Deloro, B. Velten and J. C. Marioni,
et al., MOFA+: a statistical framework for comprehensive integration of multi-modal single-cell data, Genome Biol., 2020, 21(1), 111 CrossRef PubMed.
-
Y. Hao, T. Stuart, M. Kowalski, S. Choudhary, P. Hoffman, A. Hartman, A. Srivastava, G. Molla, S. Madad, C. Fernandez-Granda and R. Satija, Dictionary learning for integrative, multimodal, and scalable single-cell analysis, bioRxiv, 2022.
- A. Ravetto, P. D. A. Anderson, C. V. C. Bouten and J. M. J. den Toonder, Microfluidics for Single Cell Mechanical Characterization: A Review, SM Journal of Biomedical Engineering, 2017, 3(2), 1016 Search PubMed.
- A. C. Rowat, J. Lammerding and J. H. Ipsen, Mechanical Properties of the Cell Nucleus and the Effect of Emerin Deficiency, Biophys. J., 2006, 91(12), 4649–4664 CrossRef CAS PubMed.
- K. D. Nyberg, M. B. Scott, S. L. Bruce, A. B. Gopinath, D. Bikos and T. G. Mason,
et al., The physical origins of transit time measurements for rapid, single cell mechanotyping, Lab Chip, 2016, 16(17), 3330–3339 RSC.
- L. A. Lautscham, Migration in Confined 3D Environments Is Determined by a Combination of Adhesiveness, Nuclear Volume, Contractility, and Cell Stiffness, Biophys. J., 2015, 109(5), 900–913 CrossRef CAS PubMed.
- K. Alessandri, B. R. Sarangi, V. V. Gurchenkov, B. Sinha, T. R. Kießling and L. Fetler,
et al., Cellular capsules as a tool for multicellular spheroid production and for investigating the mechanics of tumor progression in vitro, Proc.
Natl. Acad. Sci., 2014, 110(37), 14843–14848 CrossRef PubMed.
- L. J. Holt, O. Hallatschek and M. Delarue, Methods Cell Biol., 2018, 147, 215–231 CAS.
- I. De Vlaminck and C. Dekker, Recent Advances in Magnetic Tweezers, Annu. Rev. Biophys., 2012, 41(1), 453–472 CrossRef CAS PubMed.
- S. Khakshour, T. V. Beischlag, C. Sparrey and E. J. Park, Probing Mechanical Properties of Jurkat Cells under the Effect of ART Using Oscillating Optical Tweezers, PLoS One, 2015, 10(4), e0126548 CrossRef PubMed.
- F. Ziemann, J. Rädler and E. Sackmann, Local measurements of viscoelastic moduli of entangled actin networks using an oscillating magnetic bead micro-rheometer, Biophys. J., 1994, 66(6), 2210–2216 CrossRef CAS PubMed.
- S. Khakshour, M. P. Labrecque, H. Esmaeilsabzali, F. J. S. Lee, M. E. Cox and E. J. Park,
et al., Retinoblastoma protein (Rb) links hypoxia to altered mechanical properties in cancer cells as measured by an optical tweezer, Sci. Rep., 2017, 7(1), 7833 CrossRef CAS PubMed.
- L. Chen, V. Maybeck, A. Offenhäusser and H. J. Krause, Implementation and application of a novel 2D magnetic twisting cytometry based on multi-pole electromagnet, Rev. Sci. Instrum., 2016, 87(6), 064301 CrossRef PubMed.
- Y. Tanaka, K. Sato, T. Shimizu, M. Yamato, T. Okano and I. Manabe,
et al., Demonstration of a bio-microactuator powered by vascular smooth muscle cells coupled to polymer micropillars, Lab Chip, 2008, 8(1), 58–61 RSC.
- D. Hartono, Y. Liu, P. L. Tan, X. Y. S. Then, L. Y. L. Yung and K. M. Lim, On-chip measurements of cell compressibility via acoustic radiation, Lab Chip, 2011, 11(23), 4072 RSC.
- P. Augustsson, J. T. Karlsen, H. W. Su, H. Bruus and J. Voldman, Iso-acoustic focusing of cells for size-insensitive acousto-mechanical phenotyping, Nat. Commun., 2016, 7(1), 11556 CrossRef CAS PubMed.
- S. Zhong, W. Ding, L. Sun, Y. Lu, H. Dong and X. Fan,
et al., Decoding the development of the human hippocampus, Nature, 2020, 577(7791), 531–536 CrossRef CAS PubMed.
- B. B. Lake, S. Chen, B. C. Sos, J. Fan, G. E. Kaeser and Y. C. Yung,
et al., Integrative single-cell analysis of transcriptional and epigenetic states in the human adult brain, Nat. Biotechnol., 2018, 36(1), 70–80 CrossRef CAS PubMed.
- Y. Liu, High-Spatial-Resolution Multi-Omics Sequencing via Deterministic Barcoding in Tissue, Cell, 2020, 183(6), 1665 CrossRef CAS PubMed.
- D. P. Tabassum and K. Polyak, Tumorigenesis: it takes a village, Nat. Rev. Cancer, 2015, 15(8), 473–483 CrossRef CAS PubMed.
- S. V. Sharma, D. Y. Lee, B. Li, M. P. Quinlan, F. Takahashi and S. Maheswaran,
et al., A Chromatin-Mediated Reversible Drug-Tolerant State in Cancer Cell Subpopulations, Cell, 2010, 141(1), 69–80 CrossRef CAS PubMed.
- D. Ramazzotti, A. Lal, B. Wang, S. Batzoglou and A. Sidow, Multi-omic tumor data reveal diversity of molecular mechanisms that correlate with survival, Nat. Commun., 2018, 9(1), 4453 CrossRef PubMed.
- M. A. Ortega, O. Poirion, X. Zhu, S. Huang, T. K. Wolfgruber and R. Sebra,
et al., Using single-cell multiple omics approaches to resolve tumor heterogeneity, Clin. Transl. Med., 2017, 6(1), 46 Search PubMed.
- I. C. Macaulay, C. P. Ponting and T. Voet, Single-Cell Multiomics: Multiple Measurements from Single Cells, Trends Genet., 2017, 33(2), 155–168 CrossRef CAS PubMed.
- O. Rozenblatt-Rosen, A. Regev, P. Oberdoerffer, T. Nawy, A. Hupalowska and J. E. Rood,
et al., The Human Tumor Atlas Network: Charting Tumor Transitions across Space and Time at Single-Cell Resolution, Cell, 2020, 181(2), 236–249 CrossRef CAS PubMed.
- H. Yuan, M. Yan, G. Zhang, W. Liu, C. Deng and G. Liao,
et al., CancerSEA: a cancer single-cell state atlas, Nucleic Acids Res., 2019, 8(47), D900–D908 CrossRef PubMed.
- M. J. T. Stubbington, O. Rozenblatt-Rosen, A. Regev and S. A. Teichmann, Single-cell transcriptomics to explore the immune system in health and disease, Science, 2017, 358(6359), 58–63 CrossRef CAS PubMed.
- E. Papalexi and R. Satija, Single-cell RNA sequencing to explore immune cell heterogeneity, Nat. Rev. Immunol., 2018, 18(1), 35–45 CrossRef CAS PubMed.
- A. Unterman, T. S. Sumida, N. Nouri, X. Yan, A. Y. Zhao and V. Gasque,
et al., Single-Cell Omics Reveals Dyssynchrony of the Innate and Adaptive Immune System in Progressive COVID-19, Nat. Commun., 2022, 13(1), 440 CrossRef CAS PubMed.
- J. Fuzik, A. Zeisel, Z. Máté, D. Calvigioni, Y. Yanagawa and G. Szabó,
et al., Integration of electrophysiological recordings with single-cell RNA-seq data identifies neuronal subtypes, Nat. Biotechnol., 2016, 34(2), 175–183 CrossRef CAS PubMed.
- M. van den Hurk and C. Bardy, Single-cell multimodal transcriptomics to study neuronal diversity in human stem cell-derived brain tissue and organoid models, J. Neurosci. Methods, 2019, 325, 108350 CrossRef CAS PubMed.
- K. Leonavicius, J. Nainys, D. Kuciauskas and L. Mazutis, Multi-omics at single-cell resolution: comparison of experimental and data fusion approaches, Curr. Opin. Biotechnol., 2019, 55, 159–166 CrossRef CAS PubMed.
|
This journal is © The Royal Society of Chemistry 2022 |
Click here to see how this site uses Cookies. View our privacy policy here.