DOI:
10.1039/D0RA06849G
(Paper)
RSC Adv., 2020,
10, 35856-35872
Design and optimization of a subunit vaccine targeting COVID-19 molecular shreds using an immunoinformatics framework†
Received
8th August 2020
, Accepted 7th September 2020
First published on 30th September 2020
Abstract
COVID-19 has been declared as a global health emergency and exposed the world to a deadly virus, which has dramatically changed the lives of humans for an unknown period of time. In the battleground with the virus, we have employed an immunoinformatics framework to design a robust vaccine as an insurance plan for the future. The pathogenic sequence with cryptic epitope taken from patients in Wuhan, China, was harnessed to design a promiscuous cytotoxic T-lymphocyte, helper T-lymphocyte, and B-cell epitope based subunit vaccine, engineered with adjuvants and conformational linkers. The reported vaccine has high antigenicity and immunogenicity profiles with potential TAP affinity, which ensures elevated antigen processing capability. It has strong binding with major histocompatibility complex (MHC) receptors (MHC-1 and MHC-2) and virus-specific membrane receptor TLR-2, with scores of −1010.7, −1035.7, and −1076.3 kcal mol−1, respectively. Molecular dynamics simulation analysis was used to assess the stable binding with TLR-2 with minimal atomic motions through a deformation plot, covariance matrix, and elastic network. Importantly, an in silico immunization assay showed the reliable elicitation of key players in terms of immune cells together with memory cells to evoke adaptive immune responses upon administration of the construct. In view of favorable outcomes, we also propose a plausible vaccine mechanism to elicit an immune response to fight coronavirus.
Introduction
A virus has emerged, which to date has infected over 25.5 million people in more than 180 countries/regions and caused fatalities of at least 850
000 and counting.1 Nothing screams like a pandemic2 than the current statistics of the infectious disease, COVID-19, which surfaced in Wuhan, China3 but is now the biggest concern around the globe. Such is the impact of Coronavirus, the cause of the global health emergency, that terms like lockdown, quarantine, and pandemic have become a part of the daily vernacular of people all around the world. Not only is COVID-19 easily transmitted, but it is also more dangerous (higher mortality rate) for the elderly population, or people with underlying health conditions like diabetes, heart disease, and hypertension.4,5 While the movement of people is restricted, health care systems are being pushed to their limit, and available treatments are succumbing to the virus, there is no bigger need of the hour than to develop a vaccine against the coronavirus.6
The initial reports of people presenting pneumonia-like symptoms in Wuhan, Central China, at the end of 2019 drew parallels to the SARS epidemic of 2003. Later, it was established that the cause of the infectious disease was a novel but related strain (SARS-CoV-2) to the SARS coronavirus (SARS-CoV).7,8 Coronaviruses are a group of closely linked viruses, which comprise the Coronaviridae, Roniviridae, and Arteriviridae families. Among these, coronavirus is a single-stranded RNA virus with the largest genome size of nearly 300 kb.9 They are enveloped positive-sense viruses with a size of 60–140 nm covered by crown-shaped spike projections towards their outer surface.10,11 SARS-CoV-2 comprises a minimum of four viral proteins, including a spike glycoprotein, envelope protein, nucleocapsid protein and membrane protein.12 These spike proteins, which are capable of recognizing membrane receptors present on the host cells, are also the reason for the name of the virus, because ‘corona’ is Latin for ‘crown’.13 The viral spike peplomers bind to membrane receptors, and consequent conformational changes allow their insertion into the cell membrane, resulting in an infection to the host.14,15 SARS-CoV-2 is of zoonotic origin, but it has spread worldwide because of human-to-human transmission. It has been reported to have been diagnosed using a real-time polymerase chain reaction (PCR) technique for the spike receptor protein domain.16–19 Also, in another report, spikes of the virus have been said to be critical for the tracking and destruction of coronaviruses20,21 which makes the spike glycoprotein a potential target for the design of antigenic determinants to engineer them into a subunit vaccine.22,23
This alarming situation with high mortality has made it urgent to develop potential antiviral drugs or a robust vaccine against COVID-19. The initial symptoms of Coronavirus infection are generally like those of the common cold and flu, with a dry cough leading to a fever, malfunctioning of the respiratory tract, lymphopenia, diarrhea, and many other symptoms.24 The first step to treat COVID-19 is social isolation to combat the transmission, however, this cannot be considered as a cure. The diagnosis and course of treatment for this type of coronavirus are not simplified by its symptoms, which are similar to those of common flu, but can prove fatal if left undetected. The pharmaceutical industry is on any given day more equipped to fight off a bacterial disease in comparison to a viral one.25 To date, no potent antiviral drugs have been reported. Many drugs have been studied using the concept of repurposing existing drugs via molecular modeling studies, and in vitro and in vivo studies, for drugs such as remdesivir, natural alkaloids, N-heterocycles etc.,26–29 although only a few of them have shown potential therapeutic efficiency employing molecular dynamics based drug design and virtual screening.30–33 Anti-viral treatments are already scarce, and the recent situation has highlighted the defectiveness in this area. Among the various routes that can be followed, one which seems most likely to succeed is prevention via vaccination.34 As per the Draft landscape of COVID-19 candidate vaccines by WHO, 143 vaccine candidates are in pre-clinical stages, while 33 of the vaccine candidates are in clinical evaluation. At least eight of these candidates have already advanced to phase III of clinical trials.35
In the COVID-19 pandemic, it is urgent to perform sequence analysis of the pathogenic strains of SARS-CoV-2 by employing high throughput technologies to design potential vaccine candidates (T-cell and B-cell epitopes) in a short time.36–38 Immunoinformatics approaches, along with structural biology approaches, have been widely used to design robust vaccine candidates with high specificity to target receptors present on the host. Immunoinformatics techniques can be used to assess the antigenicity, immunogenicity, and TAP affinities of epitopes39,40 and have also been widely employed to design and develop vaccines for many viral diseases, including the Chikungunya, Japanese encephalitis and Zika viruses.41–43
Hence, in the present study, we have designed a multi-epitope subunit vaccine for COVID-19. We used the pathogenic sequence of infection from the COVID-19 patient case reports from China.44 We used a reverse vaccinology strategy, which was executed by designing the potential epitopes of the pathogenic sequence using immunoinformatics approaches.45 The multi-epitope approach presents a significantly powerful therapeutic method for the formulation of a vaccine. A multi-epitope approach is an ideal and efficient methodology for the development of a subunit vaccine for therapeutic use to prevent and treat viral infections or tumors.46–48 A multi-epitope vaccine comprises multiple continuous HTL, CTL, and B-cell epitopes, alongside adjuvants. With the insertion of adjuvants, multi-epitope constructs become capable of improving immunogenicity and prolonged immune response.49,50 In the last decade, various multi-epitope vaccines have been reported under clinical trials and shown promising results. In comparative studies with single epitope vaccines, multi-epitope have shown potential advantages, with both cell-mediated and humoral immune response regulators. Multi-epitopes can be identified by diverse clones from different T-cell subsets,51 which consequently elicit a strong humoral and cellular immune response.52 This advanced approach makes for an ideal and efficient methodology for the development of a subunit vaccine for therapeutic use to prevent and treat viral infections.53
Moreover, to analyze the binding efficiency and specificity of the vaccine construct with the target receptors present on host cells, we performed a molecular interactions study with major histocompatibility complex (MHC) MHC-1 and MHC-2 receptors.54 Also, to validate our results, we performed binding analysis of the vaccine with virus-specific membrane receptor toll like receptors (TLRs).55 TLR-2 is a putative receptor that plays vital roles in the generation of innate and adaptive immune responses by identifying viral antigens. TLR-2 binding with the ligand triggers the regulatory network and stimulates the production of interferon IFN-β and interleukins to destruct viral pathogens.56
We employed molecular docking and molecular dynamics simulation assays to evaluate the potency and specificity of the vaccine candidate with target receptors.57,58 By using computational advancement avenues, we also performed an in silico immunization assay for the designed vaccine and assessed the strength of the vaccine construct to elicit the critical regulators of the immune system. Out of these significant results, we propose the mechanism of action of the vaccine construct to evoke strong immune responses. Moreover, the vaccine construct was well-characterized by physicochemical parameters, along with an in silico cloning expression assay. This study details a breakthrough multi-epitope subunit vaccine with high potential against COVID-19 via theoretical approaches.
Materials and methods
COVID-19 infectious sequence retrieval and assessment
The spike glycoprotein sequence of severe acute respiratory syndrome coronavirus 2, reported to be mainly responsible for coronavirus infection, according to multiple pieces of molecular evidence and reports in Wuhan China, was examined to determine the potential antigenic determinants to design the vaccine.44 The infectious glycoprotein protein sequence of COVID-19 was retrieved from the NCBI protein data bank with GenBank ID: QHR63290.2. We employed the most conserved SARS-coronavirus infectious sequence to design a potential epitope through BLAST analyses. The sequence alignment analysis, including the conservancy, domain, and homology analysis of the infectious sequence, was performed using different bioinformatics approaches.
Cytotoxic T-lymphocyte epitope identification
Cytotoxic T-lymphocyte (CTLs) epitopes were determined for the COVID-19 infectious sequence employing the CTLpred server. CTLpred works on a combined calculation using a machine learning method and artificial neural network to identify the potential major histocompatibility complex (MHC) binders.59 CTL epitopes with high antigenicity possess a high value to elicit a robust immune response and to design and develop potential subunit vaccines. The top CTL epitope outcomes were shortlisted for their high antigenicity values using the Vaxijen v2.0 autocross covariance transformation method.60
Putative helper T-lymphocyte epitope identification
Potential helper T-lymphocyte (HTL) epitopes were determined using the MHC2pred server. It identifies potential HTLs via combined machine learning and support vector machine approaches, and combinedly reports substantial HTLs through a vector of 180°.61 HTLs play a significant role in immunity derivation. HTLs act as vital regulators of the adaptive immune response and also help in the proliferation and generation of other interlinked immune cells.
Potential B-cell epitope prediction
Putative B-cell epitope were determined using an artificial neural network-based ABCpred server and Karplus and Kolskar algorithms using a BCpred server. The ABCpred server identifies potential MHC binders based on alpha, beta, and gamma weight from an extensive database of B-cell immunogenic epitopes. The Karplus algorithm is used to analyze epitopes according to the flexibility of binder at various temperatures, B factors, and alpha carbons of known structures from a database.62
Transporter associated protein affinity and toxicity profiling of shortlisted epitopes
The transporter associated with antigen processing (TAP) regulatory proteins is known for the transportation of cytosolic peptidic sequences to the endoplasmic reticulum and processing it to the cell membrane. The TAP affinity analysis of the shortlisted epitopes was performed using the TAPreg server. The TAPreg server examines the TAP efficiency of lead epitopes according to a quantitative structure–activity relationship. Also, the toxicity profiling of epitopes was carried out by a support vector machine-based Toxinpred server. The shortlisted epitopes with high antigenicity, strong TAP affinity, and non-toxic nature were employed to design a multi-epitope vaccine.63
Subunit vaccine construction and structural evaluation
Shortlisted epitopes with high antigenicity and TAP affinity were employed to design a multi-epitope subunit vaccine. A subunit vaccine was formed by combining the CTL, HTL, and B-cell epitopes.64 The epitopes were engineered and combined using various links and motifs, which also helped to maintain the conformation and flexibility of the vaccine construct.65 The vaccine construct was also adjoined with adjuvants to enhance the immunogenicity values of the vaccine. Adjuvants cholera toxin subunit-B (CTB) and tetanus toxin fragment fragment-C (TTFrC) were adjoined to the vaccine construct in order to evoke robust immune responses.66 After that, structural analysis of the designed multi-epitope subunit vaccine was carried out. The secondary structural analysis was done using Psipred and GOR4 software. The three-dimensional structure of the vaccine was optimized, and energy minimized using the Gromacs minimizer suite and analyzed for the phi–psi angle using a Ramachandran plot.
Molecular docking analysis of the vaccine candidate with MHC-1, MHC-2, and TLR-2
The strong binding ability of the vaccine construct (epitopes) with MHC molecules (MHC-1 and MHC-2) is essential to evoke the immune system and to develop a vaccine and immunotherapy directed against the infectious microorganisms. These interactions initiate an innate immune response and further elicit an adaptive immune response in response to the administrated specific epitopic antigens.67,68 Binding analyses of the designed multi-epitope vaccine with MHC receptors (MHC-1 and MHC-2) were performed using the Cluspro docking server.69 The Cluspro modeling tool works using fast Fourier transform (FFT) algorithms and identifies multiple binding energy clusters. It generates the 5000–15
000 binding modes in a defined box with an assessment of CHARMM energy.
Furthermore, it runs a semi-definite approach and computes the clusters using Monte-Carlo simulations. Also, we performed molecular docking with the TLR-2 receptor. Coronavirus is an RNA virus and has the specificity to bind with Toll-like receptors, as discussed in the introduction. TLRs are known as regulatory receptors for viral antigens, and their complex further initiates the cascade signalling pathways to evoke immune cells against the virus pathogens. We assessed the binding affinity of the engineered vaccine candidate with specific receptors, in reference to the infectious sequence (antigenic determinants) of SARS coronavirus. The antigenic determinants of the infectious sequence (spike glycoprotein) are reported to be majorly involved in the induction of the natural host immune response and in the regulatory network of virus infection and progression.70,71 Furthermore, we validated the results with other protein–protein docking tools, HEX 8.0 and PatchDock, towards an engineered vaccine candidate with specific membrane receptors. Resulting docked trajectories were analyzed through the energy minimization, conformational changes, and molecular dynamics to assess the binding of the vaccine construct.
Molecular dynamics simulation analysis of the interacting complex of the vaccine and TLR-2
The docked complex of the multi-epitope vaccine and TLR-2 (virus-specific receptor) was studied via molecular dynamics simulation using the i-Mod server. Stabilized binding and minimal deformation of the docked complex were examined using protein conformation dynamics via a normal mode algorithm (NMA). The NMA was used to determine the inherent motion of the complex coordinates72 and the stability, direction, and atomic motions (B-factors) on the interacting complex using deformation profiles, covariance matrices, and elastic network analyses. Deformability profiling of a docked trajectory depicts the binding stability and potency of the ligand to the receptor according to the atomic fluctuations.73 Moreover, we also performed theoretical calculations and determined the Eigen score of the interacting complex of the vaccine-TLR2. The Eigen score indicates the rigidity of the motion of the complex and is comparable to the deformation profiles. A low Eigen score indicates less stability and easy deformation of the atomics coordinates, as demonstrated using independent component algorithms.74
In silico immunization analysis of the vaccine construct
Immune response generation studies of the lead vaccine candidate were performed using an in silico immune simulation approach for 100 simulation steps with a 480 time steps for immune simulation prediction. Immunization experiments use the combined strategies of system biology along with the details given by data-driven prediction methods to analyze the immune response generation. C-ImmSim works using position-specific scoring matrix and machine learning approaches to elucidate the statics of antigen response at different time intervals. It is a potential approach that works based on Miyazawa and Jernigan protein–protein assessment to determine the molecular binding in the context of immune responses.75 We investigated the immune generation capability of the vaccine by simulation of the classical immunization approach and identified the immune system key player cells; cytotoxic T-cells, helper T-cells, B cells, and other immune cells.
Antigenicity, allergenicity, physicochemical characterization and in silico cloning assessment of the vaccine construct
The antigenicity and allergenicity profiles of the vaccine construct were also determined. The VaxiJen and AntigenPro servers were employed to assess the antigenicity of the vaccine construct, and the AllerTop server was used to evaluate the non-allergenic nature of the vaccine construct.76 In addition, an in silico cloning experiment was performed, and codon optimization was carried out to determine the optimized DNA sequence using the JCAT server. A restriction free cloning (RF) module was employed to execute and optimize the cloning experiment in E. coli (k12 strain).77,78
Results and discussion
COVID-19 infectious sequence retrieval and assessment
The coronavirus infectious sequence was retrieved from the molecular pieces of evidence of severe acute respiratory syndrome coronavirus 2. The latest version (March 2020) of the spike glycoprotein sequence of COVID-19 with a collection date of 30 December 2019 by Wuhan Jinyintan Hospital was availed from the protein database at the National Center for Biotechnology Information with GenBank ID QHR63290.2, which caused an epidemic in the people of Wuhan, China. The outbreak viral sequence was isolated from an early-stage patient and showed >95% genome level similarity with bat coronavirus. The retrieved sequence was analyzed using protein–protein BLAST and it was found that it (1273 amino acids sized) possesses high conservancy with the surface glycoprotein of SARS coronavirus-2, MERS coronavirus and spike protein of bat coronaviruses (Fig. S1†). Conserved protein domain analysis showed that it consisted of three domains, an N-terminal domain of the S1 subunit of spike protein (13–304 a.a.), the receptor binding domain of an S1 subunit (319–541 a.a.) and a corona S2 superfamily glycoprotein (662–1270 a.a.). Importantly, it consists of multiple features, including a highly conserved cryptic epitope, a target of neutralizing antibodies. This cryptic epitope has the ability to undergo cross-reactive binding with immunogenic antibodies and possesses high applicability to derive a vaccine for novel coronavirus. Moreover, pairwise sequence assessment using tree evaluation methods, neighbor-joining, and the fast minimum evolution method showed a high similarity with the infectious sequence of the Wuhan seafood market pneumonia virus (172 hits), with minimal variations of 0.0004–0.0005. With these shreds of evidence with encrypted epitope features, we employed the infectious sequence to design a multi-epitope subunit vaccine candidate and to evoke an immune response in humans against COVID-19.
Cytotoxic T-lymphocyte epitope identification
Cytotoxic T-lymphocytes (CTLs) play vital roles in cellular immunity and elimination of virus infections. Putative CTL epitopes (MHC-1 restricted CD8+) were determined for the COVID-19 infectious sequence using the CTLpred server. The CTLpred server works by using a direct algorithm to determine a high MHC-1 binder antigenic peptide sequence. It uses the combined approaches of artificial neural networks along with a support vector machine. Threshold values of 0.51 for artificial neural networks and 0.36 for the support vector machine were set to determine high binder epitopes with high specificity and sensitivity. The obtained results showed the potential epitopes, with high coverage of MHC-1 supertypes (>20) that covered 90 percent of the world population of MHC types. Obtained potential CTL epitopes (top-scored) were further analyzed for high antigenicity properties and shortlisted according to their high antigenic characteristics (Table 1).
Table 1 Top scoring cytotoxic T-lymphocyte epitopes for COVID-19
CTL rank |
Start position |
Epitope sequence |
ANN score (threshold 0.51) |
SVM score (threshold 0.36) |
Antigenicity values (threshold 0.4) |
Inferences |
1 |
1192 |
NLNESLIDL |
0.92 |
1.5879094 |
0.6827 |
Antigenic |
2 |
236 |
TRFQTLLAL |
0.99 |
1.4877641 |
0.3406 |
Less antigenic |
3 |
983 |
RLDKVEAEV |
0.71 |
1.6892505 |
0.0765![[thin space (1/6-em)]](https://www.rsc.org/images/entities/char_2009.gif) |
Very less antigenic |
4 |
679 |
NSPRRARSV |
0.87 |
1.0916413 |
0.1034![[thin space (1/6-em)]](https://www.rsc.org/images/entities/char_2009.gif) |
Less antigenic |
5 |
292 |
ALDPLSETK |
0.92 |
1.0117189 |
1.2241![[thin space (1/6-em)]](https://www.rsc.org/images/entities/b_char_2009.gif) |
Highly antigenic |
6 |
204 |
YSKHTPINL |
0.47 |
1.4169218 |
1.0547![[thin space (1/6-em)]](https://www.rsc.org/images/entities/char_2009.gif) |
Highly antigenic |
7 |
820 |
DLLFNKVTL |
0.73 |
1.1189541 |
0.6800 |
Antigenic |
8 |
972 |
AISSVLNDI |
0.99 |
0.84037452 |
0.0479 |
Less antigenic |
9 |
1125 |
NCDVVIGIV |
0.93 |
0.83215588 |
1.0608![[thin space (1/6-em)]](https://www.rsc.org/images/entities/b_char_2009.gif) |
Highly antigenic |
10 |
97 |
KSNIIRGWI |
0.89 |
0.86720593 |
−1.6883 |
Non antigenic |
Putative helper T-lymphocyte epitope identification
Helper T-lymphocyte peptides play essential roles in adaptive immunity. HTLs are known for eliminating virus infection by generating antibodies and macrophage cells. The MHC2pred server was employed to design the HTLs, using the condition that the high binder antigenic peptides possess an IC50 value of <500 nm and non-binder peptides possess an IC50 value of >500 nm. High binder HTLs (MHC-2 restricted) were determined using the MHC2pred server via a combination of machine learning and support vector machine approaches. We obtained the HTL epitope for a large class of MHC-2 receptor supertypes (ALLELE: HLA-DR1, ALLELE: HLA-DR4, ALLELE: HLA-DR9, ALLELE: HLA-DR11 and ALLELE: HLA-DR2). After that, the determined epitopes were shortlisted according to their antigenici characteristics, and highly antigenic epitopes were used to design a multi-epitope vaccine for COVID-19 (Table 2). To design and develop a robust vaccine adjuvant, tetanus toxin fragment-C (TTFrC) was engineered to the vaccine construct, hence HTLs for TTFrC adjuvant were determined using a position-specific scoring matrices based Rankpep tool (Table 3). TTFrC plays a vital role in internalization, transportation, and binding of a vaccine construct with target specific receptors.
Table 2 Data on potential helper T-lymphocyte epitopes in terms of their antigenic sequence, peptide score, and their computed antigenicity values
HTL epitope rank |
Antigenic sequence |
Residue number |
Peptide score |
Antigenicity values (threshold 0.4) |
Inferences |
1 |
RAAEIRASA |
1014 |
0.733 |
0.2752 |
Less antigenic |
2 |
RRARSVASQ |
682 |
0.727 |
0.5490 |
Moderately antigenic |
3 |
IRASANLAA |
1018 |
1.157 |
0.4455 |
Moderately antigenic |
4 |
SVTTEILPV |
721 |
1.153 |
0.8441 |
Antigenic |
5 |
GLTGTGVLT |
545 |
1.685 |
1.0556![[thin space (1/6-em)]](https://www.rsc.org/images/entities/b_char_2009.gif) |
Highly antigenic |
6 |
SFTVEKGIY |
305 |
1.643 |
0.3544 |
Less antigenic |
7 |
SNFGAISSV |
968 |
0.230 |
1.1296 |
Highly antigenic |
8 |
SAIGKIQDS |
929 |
0.217 |
0.5185 |
Moderately antigenic |
|
9 |
ECSNLLLQY |
748 |
1.214 |
0.3331 |
Less antigenic |
|
10 |
YQPYRVVVL |
505 |
1.206 |
0.5964![[thin space (1/6-em)]](https://www.rsc.org/images/entities/char_2009.gif) |
Moderately antigenic |
|
Table 3 Potential HTL epitopes for the adjuvant tetanus toxin fragment-C
Rank |
Epitope sequence |
Percentile score |
Starting residue position |
End residue position |
1 |
NDIISDISGFNSSVITYPDAQLVPGINGKAIHLVNNE |
22 |
40 |
66 |
2 |
IEYNDMFNNFTVSFWLRVPKVSASHLEQYGT |
28.12 |
78 |
108 |
3 |
LRVGYNAPGIPLYKKMEAVKLRDLK |
17.4 |
362 |
386 |
Potential B-cell epitope prediction using the Karplus method
B-cells are known to be involved in the derivation of humoral response by evoking class-switched antibodies. The Karplus method was employed, which is used to determine flexible B-cell epitopes using the BCpred 2.0 server. Epitopes with large scale flexibility were determined based on various parameters, including atomic fluctuations, temperature, and stability. Based on these parameters, we identified potential B-cell epitopes and further analyzed them for their antigenicity character, as we analyzed T-cell epitopes (Table 4). Moreover, shortlisted epitopes were screened according to their physicochemical properties (propensity, polarity, hydrophilicity, and accessibility) using the ABCpred server. We found that the shortlisted B-cell epitopes lie in the steady region within the optimal range (−3 to +3, as per the application algorithm) in terms of their physicochemical properties.79
Table 4 Promiscuous B-cell epitopes and their antigenic character profiling
Rank |
Epitope |
Position |
Score |
Antigenicity values (threshold 0.4) |
Inferences |
1 |
WIFGTTLDSKTQ |
104 |
0.86 |
0.6072 |
Antigenic |
2 |
SKRSFIEDLLFN |
813 |
0.85 |
−0.0939![[thin space (1/6-em)]](https://www.rsc.org/images/entities/char_2009.gif) |
Non-antigenic |
3 |
KTPPIKDFGGFN |
790 |
0.85 |
−0.2264![[thin space (1/6-em)]](https://www.rsc.org/images/entities/char_2009.gif) |
Non-antigenic |
4 |
QLPPAYTNSFTR |
23 |
0.85 |
−0.0689![[thin space (1/6-em)]](https://www.rsc.org/images/entities/char_2009.gif) |
Non-antigenic |
5 |
NYLYRLFRKSNL |
450 |
0.84 |
0.0104![[thin space (1/6-em)]](https://www.rsc.org/images/entities/char_2009.gif) |
Less antigenic |
6 |
GIGVTQNVLYEN |
908 |
0.82 |
0.7229 |
Highly antigenic |
7 |
QGNFKNLREFVF |
183 |
0.81 |
0.3002 |
Less antigenic |
8 |
SLLIVNNATNVV |
116 |
0.81 |
0.4935 |
Antigenic |
9 |
ADSFVIRGDEVR |
397 |
0.80 |
−0.1593 |
Less antigenic |
10 |
ALEPLVDLPIGI |
222 |
0.80 |
0.7479![[thin space (1/6-em)]](https://www.rsc.org/images/entities/b_char_2009.gif) |
Moderately antigenic |
TAP affinity and non-toxic character analysis of the shortlisted epitopes
Transporters associated with antigen procession (TAP) affinities of the lead epitopes were screened. Antigens must have a high TAP affinity to be processed through the endoplasmic reticulum to bind with MHC receptors and for consequent internalization and transfer to the cell membrane. The TAP efficiency of the shortlisted epitopes was examined in terms of their quantitative structure–activity relationship using the TAPreg server, and we determined the epitopes with high TAP affinities and large IC50 values (Table 5). Besides this, the epitopes were screened in terms of their physiological properties, including hydrophilicity, isoelectric point, hydrophobicity, and non-toxicity. We found that the epitopes lie in the optimal range with non-toxic characteristics. The screened epitopes were employed to construct a multi-epitope vaccine.
Table 5 TAP affinity and non-toxicity of the shortlisted epitopes
Type |
Antigenic sequence |
TAP affinity (IC50 (μM)) |
SVM score |
Hydrophobicity |
Hydropathicity |
Inference (nature) |
pI |
CTL |
ALDPLSETK |
19.64 |
−1.04 |
−0.18 |
−0.51 |
Non-toxin |
4.38 |
NCDVVIGIV |
60.33 |
−0.25 |
0.21 |
1.86 |
Non-toxin |
3.80 |
HTL |
SNFGAISSV |
6.96 |
−0.94 |
0.10 |
0.78 |
Non-toxin |
5.88 |
GLTGTGVLT |
29.77 |
−1.11 |
0.17 |
0.94 |
Non-toxin |
5.88 |
B-CELL |
ALEPLVDLPIGI |
26.82 |
−1.25 |
0.21 |
1.32 |
Non-toxin |
3.67 |
GIGVTQNVLYEN |
44.89 |
−1.49 |
−0.01 |
−0.01 |
Non-toxin |
4.0 |
Multi-epitope subunit vaccine construction and refinement
A multi-epitope vaccine was constructed using the criteria that it should be promiscuous, highly antigenic, and immunogenic, with overlapping CTLs, HTLs, and B-cell epitopes. In addition, a high binding affinity towards MHCs and conformational globularity of structure were also considered. Incorporating this functionality, we designed a multi-epitope subunit vaccine using the shortlisted, top-scoring 2CTL, 2HTL, and 2B-cell epitopes and combined them using linkers (Fig. 1). HTL epitopes were adjoined using a GPGPG linker, and TTFrC booster epitopes were also linked using the same linker. CTL epitopes were combined using an AAY linker. To boost the immune response of the designed vaccine, adjuvants were adjoined to the multi-epitope vaccine for prolonged strong immune responses. We used cholera toxin subunit-B (CTB) in the constructed vaccine, joined using EAAAK linkers. CTB is a strain of cholera toxin with no associated side effects and toxicity. Also, it has been used in many vaccines to boost their immune response. The overall formulation of the multi-epitope subunit vaccine is made up of four domains: adjuvant CTB at the N-terminal, TTFrC in the first domain of the construct, CTLs and HTLs in the center of the second and third domains, and B-cell epitopes at the C-terminal in the fourth domain, all joined together using various linkers. The linkers also play a potential role in maintaining the structural stability of the vaccine construct and provide flexibility and stabilize globular conformation.
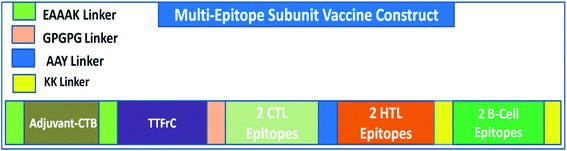 |
| Fig. 1 Scheme of the formulation of the multi-epitope vaccine against COVID-19. | |
Secondary and tertiary structural analyses of the vaccine construct
The secondary structure of the vaccine was analyzed using GOR4 and the Psipred server. The obtained results show that the vaccine construct has a stabilized structure, with 28.23% alpha-helix, 23.39% extended strands and 49.39% random coils. The substantial balance of the secondary structural parameters (helices, beta-strands, and random coils) suggested a flexible, stable, and globular conformation of the vaccine (Fig. 2). After that, the three-dimensional structure of the multi-epitope vaccine was designed using the 3Dpro server, which predicts a 3D structure using protein data bank (PDB) knowledge via statistical calculations and PDB structural silent features in terms of energy functions. Through threading methodology, multiple fragments templates were designed, and through an energy minimized simulated annealing process, various structure models were predicted. Out of them, the vaccine model with the lowest energy was retrieved.
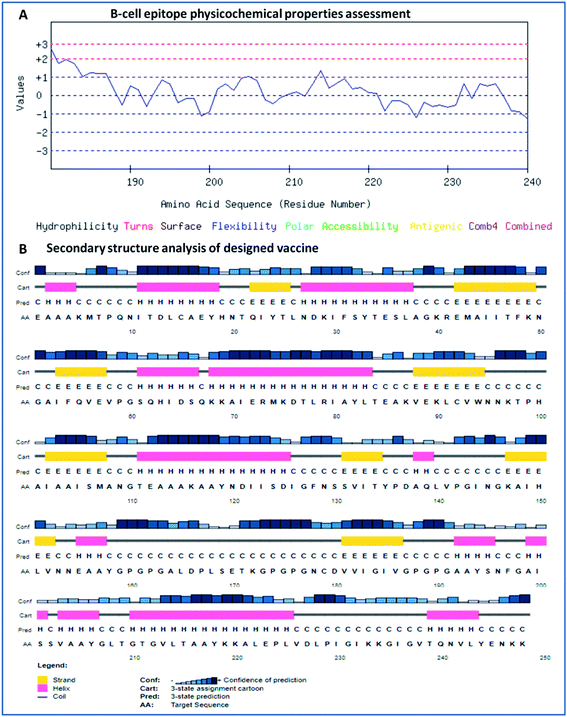 |
| Fig. 2 (A) Assessment of the physicochemical properties of the lead B-cell epitope and (B) secondary structural analysis of the vaccine construct. | |
The designed 3D structure of the vaccine was processed through energy minimization using a Gromacs-minimizer to confine the structural integrity and conformation. From the vaccine structure identified, 4000 unwanted atoms were removed. After that, the stereochemical and physicochemical properties of the vaccine construct were examined. Ramachandran plot assessment showed the phi-psi angles of the 3D structure and depicted 89.80% of residues that occurred in the favored region of the Ramachandran plot, in which 6.9% of residues are in the allowed region, and only 3.3% residues are in the outlier region (Fig. 3). Moreover, the 3D structure was analyzed for inherent atom mobility using B-factors. The determined atomic movement of the vaccine construct was compared with known structures determined by NMR, which showed high similarity to the known structures (Fig. S2†). The results signified the stable composition of the multi-epitope vaccine.
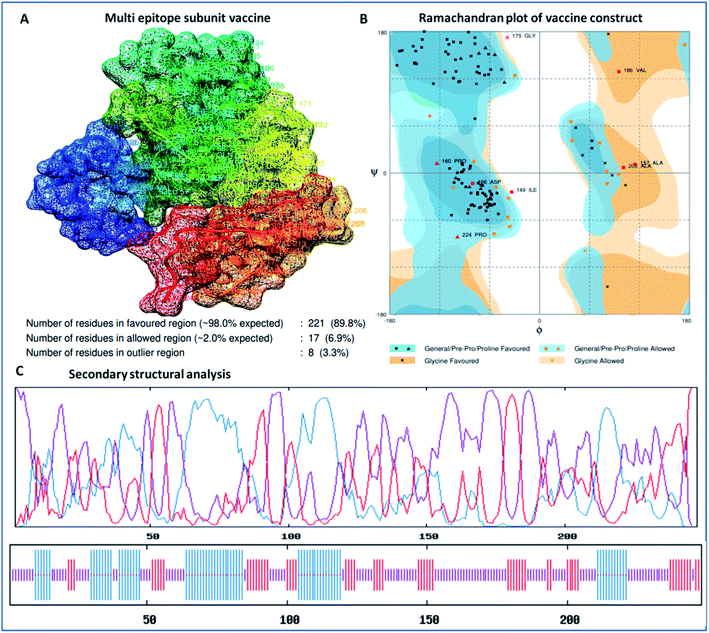 |
| Fig. 3 (A) 3D crystal structure of the formulated vaccine construct, (B) Ramachandran plot assessment of the multi-epitope vaccine construct and (C) secondary structural analysis of the designed vaccine, showing structural atomic fluctuation within a minimal range, signifying its structural stability. | |
Molecular docking analysis of the vaccine candidate with MHC-1, MHC-2, and TLR-2
Binding analyses of the vaccine construct with MHC-1 and MHC-2, and further with specific receptor TLR-2, were performed using a molecular docking assay. Receptors MHC-1 and MHC-2 were downloaded with PDB Ids 1I1Y and 1KG0, respectively, from the protein data bank. Both MHC receptors were processed and energy minimized to perform the molecular docking. Stereochemical analyses was carried out using Ramachandran plot assessment, which showed that both the receptors lie in favorable regions of the Ramachandran plot, with 97.4% residues of MHC-1 and 96.4% residues of MHC-2, respectively (Fig. 4). Cluspro docking software was employed to execute the molecular docking, and target receptors and the ligand vaccine construct were uploaded. Cluspro was used to run the docking algorithms using PIPER rigid body methods, and unstructured terminal residues were removed. Grids were formed with the points in an x, y, axis by rotating the receptors with a spacing 1.0 Å. From an outcome of 1000 docked complexes of target MHC receptors and ligand vaccine, the docked trajectory with the lowest energy was taken, and the results showed that the multi-epitope vaccine construct has strong binding with MHC-1 and MHC-2. The vaccine showed the closest binding with a lowest energy score of −1160.8 kcal mol−1 to the binding groove of MHC-1 and -1010.7 kcal mol−1 at the center of the receptor. And with MHC-2, a lowest energy score of −1035.7 kcal mol−1 and −969.3 kcal mol−1 to the center coordinates were recorded.
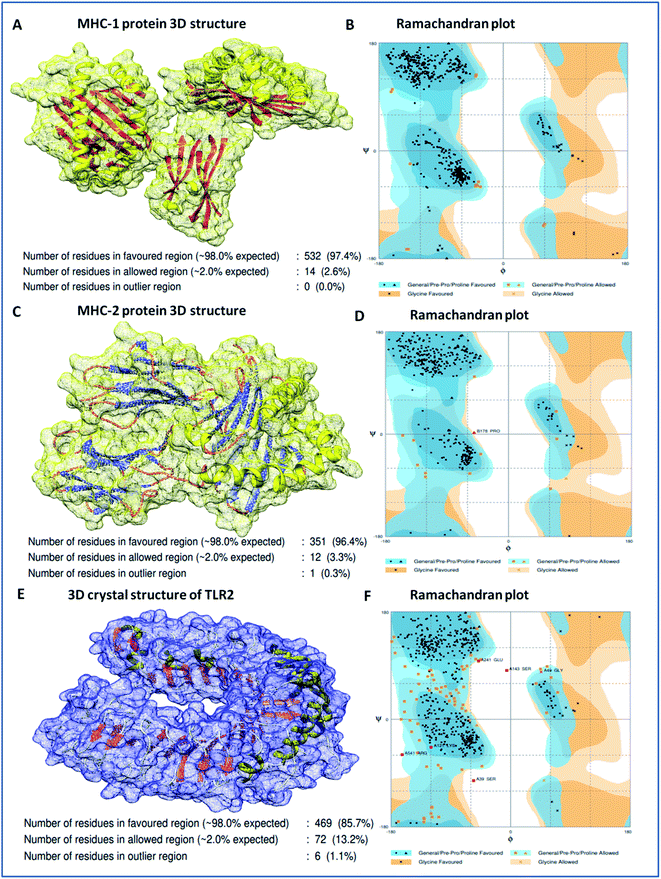 |
| Fig. 4 (A) 3D crystal structure of the MHC-1 receptor, (B) Ramachandran plot analysis of the MHC-1 receptor, (C) 3D crystal structure of the MHC-2 receptor, (D) Ramachandran plot analysis of the MHC-2 receptor, (E) 3D crystal structure of the TLR-2 receptor, and (F) Ramachandran plot analysis of the TLR-2 receptor. | |
Importantly, to validate and affirm our results, interaction analysis with the TLR-2 receptor (virus-specific membrane receptor) was performed. The TLR-2 receptor was availed with PDB ID 2Z7X from the protein data bank. The TLR-2 crystal structure was determined by X-ray diffraction with a high resolution of 2.1 Å. The structure is made up of chain-A of 549 amino acids in size and consists of three domains; extracellular, transmembrane, and cytoplasmic domains. The TLR-2 structure is conjugated with triactylated lipopeptide with N-acetyl-D-glucosamine, 2-(acetylamino)-2-deoxy-A-D-glucopyranose, and alpha, and beta-D-mannose for regulatory dimerization. The TLR-2 structural assessment showed its stable conformation, with 85.7% residues in the favored region and 13.2% residues in the allowed region of the Ramachandran plot (Fig. S3†). A molecular docking assay was performed using the methodology mentioned above. The obtained results showed the potential binding of the vaccine construct, with TLR-2 with energy scores of −1076.3 and −969.6 kcal mol−1 at the center of the membrane receptor (Fig. 5), whereas reference cumulative antigenic determinants of the infectious sequence of coronavirus showed a lower binding energy score of −695.8 kcal mol−1 for both in the center and lowest energy computations. Moreover, we validated the results using HEX 8.0 and PatchDock. HEX 8.0 affirmed the results, with a high binding energy score of −90.82 kJ mol−1, similarly, the PatchDock server showed a high molecular docking geometric score of 19
482.
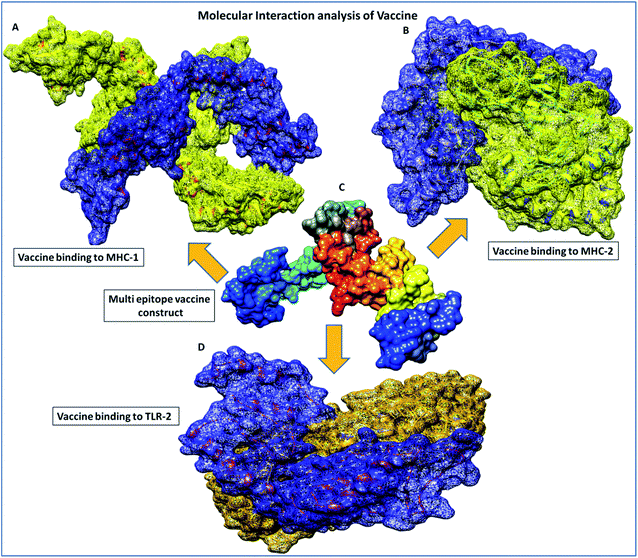 |
| Fig. 5 Molecular interaction analysis depiction of the multi-epitope vaccine construct. (A) Interacting complex of the vaccine construct (blue color) and MHC-1 (yellow color), (B) interacting complex of the vaccine construct (blue color), and MHC-2 (yellow color), (C) the multi-epitope subunit vaccine construct and depiction of the subunits of the domain in different colors, and (D) interacting complex of the vaccine construct (blue color) and TLR-2 (golden color). | |
Furthermore, in molecular interactions analysis, we observed strong binding of the designed vaccine with membrane receptors via the involvement of a large number of molecular contacts. We found that seven hydrogen bonds formed between the TLR2 and vaccine residues. Among them, five side-chain hydrogen bonds were formed between 321 ARG (NH1)- 35GLU (OE1) with a bond length of 2.91 Å, 321ARG (NH2)-35 GLU (OE2) with a bond length of 2.69 Å, 323 TYR (OH)- 35 GLU (OE1) with a bond length of 3.25 Å, 347 LYS (NZ)- 27 ASN (OD1) with a bond length of 2.65 Å, 41 ARG (NH1)-323 TYR (OH1) with a bond length of 2.72 Å and two main chain hydrogen bonds were formed between 322 PHE (O)-24 TYR (OH) with a bond length of 3.18 Å and 347 LYS (O)-24 TYR (OH) with a bond length of 2.84 Å and TLR2 receptor and vaccine residues, respectively. Also, we found the involvement of strong hydrophobic interactions of within a spatial distance of 5 Å, which were present in between 322 PHE-24 TYR, 324 LEU- 43 MET, 326 TYR- 45 ILE, 332 TYR-80 ILE, 349 PHE- 23 ILE, 349 PHE-24 TYR, 350 LEU- 45 ILE, 354 LEU- 102 ILE, 371 LEU-24 TYR, 373 VAL-23 ILE and 376 TYR- 23 ILE, along with ionic interactions of within 6 Å between the 296 ARG-76 ASP, 299 ASP-79 ARG, 321 ARG-35 GLU, 347 LYS-28 ASP and 384 ASP-90 LYS and TLR2 and vaccine residues, respectively. These significant molecular docking results suggest the strong binding of the vaccine with its specific receptors, meaning that it could be considered as a potential vaccine candidate.
Molecular dynamics simulation analysis of the vaccine construct with TLR-2
Molecular interaction analysis of the vaccine construct with the target TLR-2 receptor was performed to assess the stable binding using the i-Mod server. The docked complex of vaccine-TLR-2 was evaluated by NMA, which was used to conduct large scale flexibility and stability analysis. The i-Mod suite was used to execute the simulation by assessing the internal coordinates of the complex system. The trajectory was studied to determine the deformability. The outcome complex trajectory showed minimal deformation coordinates in the range of 0.1 to 0.9 Å through the chain hinge distortion assessment and indicated the steady binding of the vaccine with low deformation (Fig. 6). The NMA showed that there were few atomic fluctuations in the complex system trajectory of the vaccine and TLR-2. The Eigen score was calculated as 30.053311 × 10−6. Also, the covariance matrix analysis illustrated the atomic pairs of vaccine-TLR2 trajectory, correlated (red color), non-correlated (blue color), and uncorrelated (white color). It showed the coupling of the vaccine construct with TLR-2 protein residues and the conformational change in the TLR-2 binding groove. The elastic network of the model showed the pairing of atomic coordinates through distance dependent spring analysis. Every single dot in the elastic network plot represents one spring and is colored according to the stiffness of the complex with respective atomic pairs. The obtained spring models that are dark grey in color show the stability and compactness of the binding complex system. These significant results show the complex rigidity and stable binding of the vaccine with few atomic fluctuations and a low deformation index.
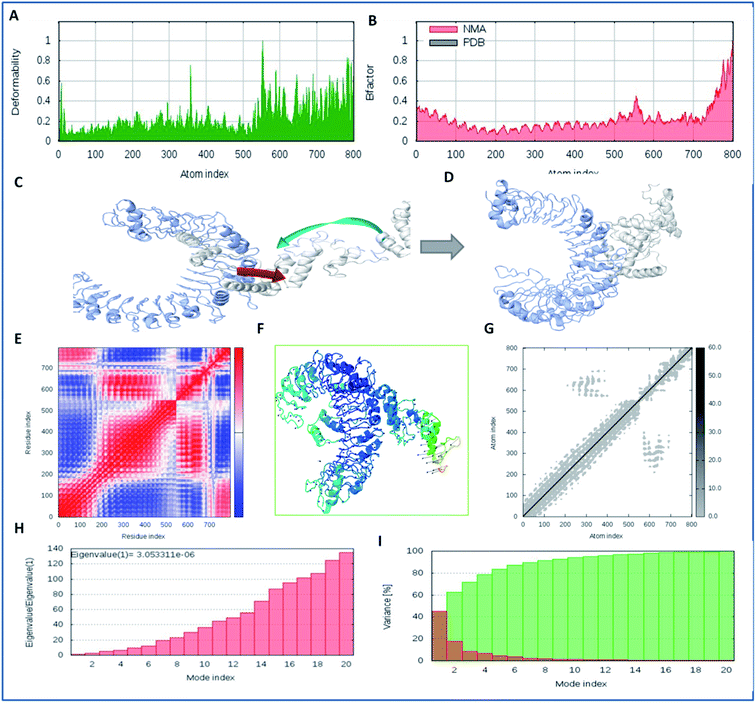 |
| Fig. 6 Molecular simulation dynamics analysis of the vaccine construct with TLR-2. (A) Deformation plot of the vaccine-TLR2 complex and depiction of the minimal deformations, (B) atomic fluctuation analysis of the vaccine-TLR2 complex using B factors, (C) trajectory of binding of the vaccine candidate to TLR2 in the initial frames, with the atomic movement of the ligand towards TLR2, (D) the closest and stabilized conformation of the binding frame of the ligand to the TLR2 receptor through NMA, (E) covariance matrix analysis of the complex system, (F) stabilized cluster conformation of binding of the lead vaccine candidate to TLR2 in 1000 frames, (G) elastic network analysis, and (H) Eigen value assessment of the complex trajectory of the vaccine construct with the TLR-2 membrane receptor. (I) Variance model index of binding complex of vaccine constrtuct with TLR-2. | |
In silico immunization assay of the vaccine
We examined the immune response elicitation of the vaccine construct by employing an in silico immune simulation method for 100 simulation steps. It is a novel approach that is used to analyze the immune response elicitation capability of the vaccine construct and antigens, combining a mesoscopic scale simulator with machine learning approaches. The vaccine candidate was exploited to assess the substantial immune response generation by T-cells, B-cells, memory cells, and other cells involved in combating viral infections. From the results, the in silico immunization experiment also presented the potency and novelty of the designed vaccine through mesoscopic based model cooperation and via molecular binding of the molecules. We found that the vaccine elicits high primary and secondary immune responses by evoking the crucial players of the immune system, including T-cell populations (cytotoxic T-cells and helper T-cells), sustainable memory cells, and other cells (elevated levels) (Fig. 7). A high level of IgM, IgG1, and IgG3 antibodies, as well as other immune cells were found after administration of the vaccine and intense and prolonged immune responses were observed against the virus by the high titers of IgG and IgM immunoglobulins (primary immune response). Moreover, by means of the amino acid sequence through simulation statics showed the elevation in cytotoxic T-cells, which reaches a maximum of 1155 cells per mm3 after 13 days of vaccine administration and after that decreases slowly up to 33 days, with an optimal level elevation of 1120 cells per mm3. The helper T-cells increased gradually on 5–6 days to a level of 5400–6000 cells per mm3, and the concentration remained elevated for a long time, up to 35 days. Elevated immune T-cells consequently evoked a high number of memory cells. Immunization assay also showed elevated levels of CTLs and HTLs in both the active and resting states in response to the vaccine and these played an essential role in strengthening the adaptive immunity against the virus infections. Also, the B-cell population (mainly responsible for innate immunity) was found to be increased, and the IgM and IgG isotype (IgG1 and IgG2) concentrations reached up to 460–480 cells per mm3 and remained at this level for a long time, simultaneously evoking memory cells. Memory cells play an essential role in the prevention of viral infection/re-infection via self-memorization upon encountering pathogens. Moreover, other central regulators of the immune system were found to be elevated (cytokines, interleukins, and natural killer cells) by the vaccine in silico immunization experimentation (Fig. 7). These results signify the potency of the designed vaccine to elicit a robust immune response to fight off the virus.
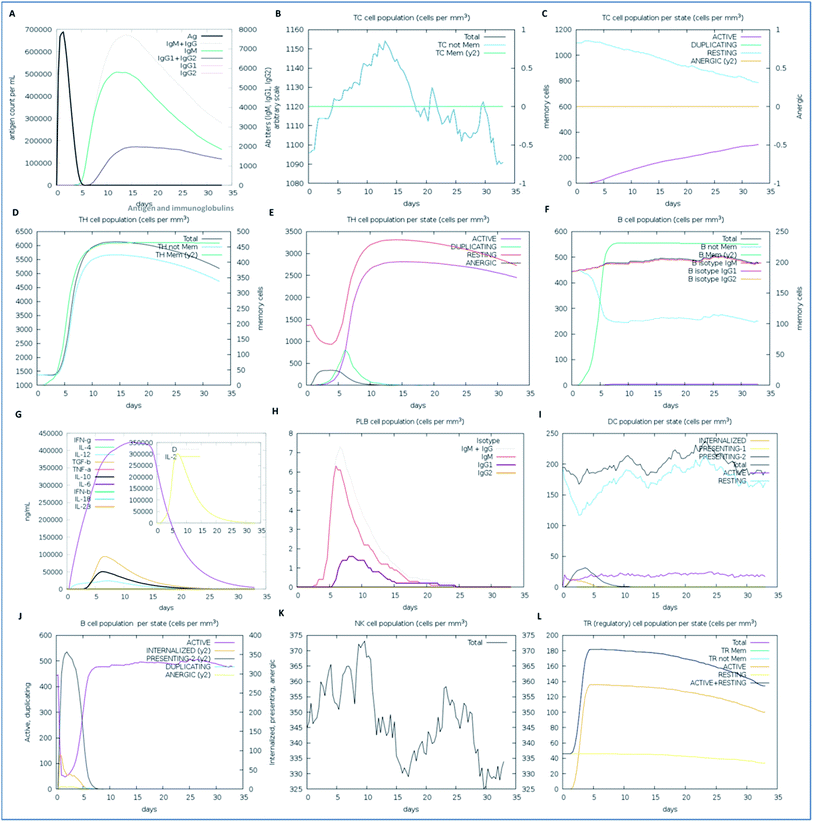 |
| Fig. 7 The immune response of the designed vaccine. (A) Elevation of immunoglobulins at different concentrations of antigen, (B) cytotoxic T-cell count, (C) T-cytotoxic cell populations in different states; resting and active, (D) helper T-cell population, (E) helper T-cell count in the resting and active states, (F) B-cell populations (IgM, IgG1, and IgG2) in response to the designed vaccine, (G) elevation of cytokines and interleukin cells, (H) B-cell populations (IgG and IgM) reached up to 7.2 cells per mm3, (I) dendritic cell population in the active and resting states, (J) B-cell populations in different states (active, internalized, and anergic), (K) natural killer cells throughout the 35 days, which reached a level of up to a maximum of 374 cells per mm3, and (L) T-regulatory cell population proliferation in the active and resting states. | |
Importantly, in light of these significant results, we also propose a plausible mechanism of action of the designed vaccine. The multi-epitope subunit vaccine (derived via the pathogenic sequence) binds to the respective MHCs and TLR receptor, which in turn activates the key players of the immune response against the viral infection (Fig. 8). Vaccine administration proliferates the CTLs, HTLs, and other regulatory immune cells, including interferons, cytokines, and natural killer cells, to destroy virus pathogens.
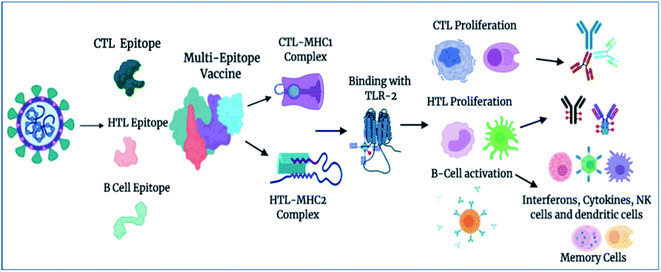 |
| Fig. 8 Proposed mechanism of action of the designed vaccine. The vaccine induces a robust immune response by elicitation of key players of immune system T-cells, B-cells, and other associated regulatory cells for the destruction of virus pathogens (created using BioRender.com Lic.). | |
Antigenicity, allergenicity and physicochemical property analysis of the vaccine
The antigenicity of the multi-epitope vaccine candidate was examined using the AntigenPro and Vaxijen servers. Both servers showed the high antigenicity of the vaccine, with scores of 0.8903 and 0.6083, respectively, which showed the high capacity of the vaccine to evoke a robust immune response. After that, the allergenicity of the vaccine was screened to determine its nature using the Allertop server. The obtained results showed that the vaccine construct is non-allergenic and has no toxicity or side effects associated with it. After that, the physicochemical behavior of the vaccine was determined using the Protparam server. The physicochemical characterization shows that the vaccine construct is of 248 amino acids in size and has a molecular weight of 26
139.8 Dalton. It has a theoretical isoelectric point (pI) of 6.5, meaning that it is slightly acidic in nature. The theoretical pI defines the pH, where the net charge of the peptide is zero and calculated according to the pK of all amino acids in the peptides. The theoretical pI is an essential parameter for peptide processing, including defining the approximate region of a 2D gel, where protein can be found.
Also, it was computed to be stable according to the characterization method, with an instability index with a score of 20.89. An instability score of less than 40 is considered to be steady and stable, as per the Protparam algorithm. Moreover, the vaccine was found to comprise a globular structure with an aliphatic index score of 91.77. It was also estimated to have prolonged existence for 1 h in mammalian reticulocytes by in vitro analysis and >10 h for Escherichia coli and >30 minutes in yeast through three consequent experimental studies, which signified the vaccine stability during the in vitro and in vivo states. Furthermore, the extinction coefficient was calculated and found to be 22
015 M−1 cm−1, with absorption values (0.1% (g l−1) 1.135 by including all cysteine pairs at 280 nm under aqueous conditions. The grand average of hydropathy (GRAVY) score was calculated to be −0.062, which shows the hydrophilic nature of the multi-epitope vaccine candidate, meaning that it can interact with water and blood and easily move to various targets. These physicochemical characterization analyses show the contents of the vaccine meet the standard criteria needed for the formulation of vaccines.
In silico cloning experiment of the vaccine construct
In silico cloning expression analysis of the vaccine construct was carried out via codon optimization of the vaccine sequence using the JCAT server. The JCAT server was optimized to perform cloning expression analysis of the multi-epitope vaccine in the k12 strain of E. coli as a host. Codon optimization showed that the vaccine has a DNA sequence of 743 base pairs in size and eminent expression of chimeric chains in response to the codon adaption index (CAI). The CAI index was calculated to be 1.0, with a high GC content of 50.13% of the improved DNA sequence of the vaccine construct and E. coli, with a GC content of 50.73%. The obtained results show that the chimeric form of E. coli is capable of expressing the multi-epitope vaccine and has high expressions for the restriction enzymes Ase I, Nde I, and SnaBI in plasmid vector pEGFP-N1 (Fig. 9).
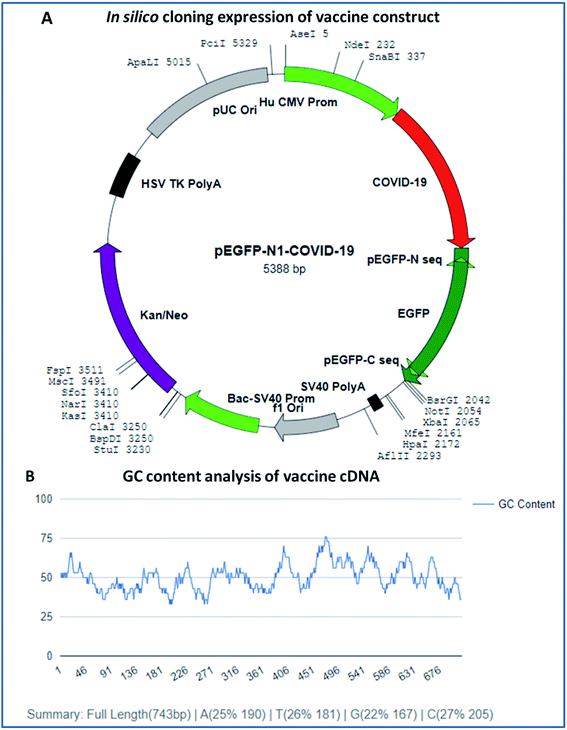 |
| Fig. 9 (A) In silico cloning experiment of the vaccine construct and (B) GC content analysis of the vaccine. | |
Conclusion
The COVID-19 pandemic has become a lethal situation in the world. Vaccination and prevention of viral spreading seem to be an assuring course of treatment for this highly contagious disease. To design and develop a vaccine against COVID-19, it has to be highly efficient, specific, and capable of evoking strong antiviral immune response. In this study, a multi-epitope subunit vaccine has been designed against coronavirus using the pathogenic sequence, retrieved from patients that have tested positive for COVID-19. Potential CTL, HTL, and B-cell epitopes were identified with high antigenicity, immunogenicity, and TAP affinities. The multi-epitope vaccine was found to bind in the proximity of MHC-1 and MHC-2 receptors. Also, it was found to interact closely with the virus-specific membrane receptor TLR-2. The docked complex trajectory analysis carried out via molecular dynamics simulations showed the stable binding of the vaccine with TLR-2 with low deformations and atomic fluctuations of the complex system. Importantly, the vaccine construct was also found to elicit a robust immune response with crucial regulators (T-cells, B-cells, and other immune cells) along with memory cells through in silico immunization assays. With these correlated findings, we propose the mechanism of action of the vaccine construct to elicit strong antiviral immune responses. Moreover, physicochemical properties and in silico cloning expression assays emphasized the high potency of the designed multi-epitope vaccine against the global threat of COVID-19.
Associated content
The improved DNA sequence of the designed vaccine construct, molecular shreds of evidence of the coronavirus infectious sequence (Fig. S1†), 3D structural assessments of the vaccine construct (Fig. S2†), atomic coordinate local quality estimation of membrane receptor TLR-2 (Fig. S3†), the energy minimized globular structure of the designed vaccine construct (Fig. S4†), and molecular dynamics simulation analyses of the vaccine construct (Fig. S5†).
Authors contribution
N. K., R. C., and D. S. designed the studies. N. K. and D. S. carried out the in silico experiments. N. K. and D. S. wrote the manuscript.
Abbreviations
SARS-CoV-2 | Severe acute respiratory syndrome coronavirus 2 |
CTLs | Cytotoxic T-lymphocytes |
MHC | Major histocompatibility complex |
HTL | Helper T-lymphocyte |
TLR | Toll-like receptors |
TAP | Transporter associated with antigen processing |
CTB | Cholera toxin subunit-B |
TTFrC | Tetanus toxin fragment-C |
NMA | Normal mode algorithm |
PDB | Protein data bank |
gB | Glycoprotein-B |
SVM | Support vector machine |
CAI | Codon adaption index |
Ig | Immunoglobulin |
FFT | Fast Fourier transform |
GRAVY | Grand average of hydropathy |
MD | Molecular dynamics |
RF | Restriction free |
Conflicts of interest
The authors declare no competing financial interests.
Acknowledgements
R. C. is grateful to CSIR, DST-Purse, and DST-SERB (EEQ/2016/000489) for providing financial assistance. N. K. thanks CSIR, New Delhi, India, for the Research Associateship (09/045(1706)2019 EMR-1) and the University of Delhi for Guest Assistant Professor Opportunity to conduct the research smoothly. D. S. thanks UGC for the SRF fellowship.
References
- WHO, Coronavirus Disease (COVID-19) Dashboard, https://covid19.who.int, 3 September 2020 Search PubMed
. - Coronavirus disease (COVID-19) Pandemic statics, World Health Organization (WHO), https://www.who.int/emergencies/diseases/novel-coronavirus-2019, March 2020 Search PubMed
. - C. Liu, Q. Zhou, Y. Li, L. V. Garner, S. P. Watkins, L. J. Carter, J. Smoot, A. C. Gregg, A. D. Daniels, S. Jervey and D. Albaiu, ACS Cent. Sci., 2020, 6, 315–331 CrossRef CAS
. - Coronavirus disease 2019 (COVID-19) Situation Report – 51. Data as reported by national authorities by 10 AM CET, 11 March 2020, https://apps.who.int/iris/bitstream/handle/10665/331475/nCoVsitrep11Mar2020-eng.pdf Search PubMed
. - Z. Wu and J. M. McGoogan, J. Am. Med. Assoc., 2020, 32091533, DOI:10.1001/jama.2020.2648
. - C. Chakraborty, A. R. Sharma, G. Sharma, M. Bhattacharya, M. R. P. Saha and S. S. Lee, Arch. Med. Res., 2020 DOI:10.1016/j.arcmed.2020.05.021
. - C. Chakraborty, A. R. Sharma, M. Bhattacharya, G. Sharma and S. S. Lee, Asian Pac. J. Trop. Med., 2020, 13, 242–246 CAS
. - S. R. Weiss and S. Navas-Martin, Microbiol. Mol. Biol. Rev., 2005, 69, 635–664 CrossRef CAS
. - S. Navas-Martin and S. R. Weiss, Viral Immunol., 2003, 16, 461–474 CrossRef CAS
. - T. Gallagher and M. Buchmeier, Virology, 2001, 279, 371–374 CrossRef CAS
. - C. Paules, H. Marston and A. Fauci, J. Am. Med. Assoc., 2020, 323, 707–709 CrossRef CAS
. - S. Shahinshavali, K. A. Hossain, A. V. D. N. Kumar, A. G. Reddy, D. Kolli, A. Nakhi, M. V. B. Rao and M. Pal, Tetrahedron Lett., 2020, 152336 CrossRef
. - D. R. Beniac, A. Andonov, E. Grudeski and T. F. Booth, Nat. Struct. Mol. Biol., 2006, 13, 751–752 CrossRef CAS
. - L. Zhang, D. Lin, X. Sun, U. Curth, C. Drosten, L. Sauerhering, S. Becker, K. Rox and R. Hilgenfeld, Science, 2020, 368, 409–412 CAS
. - P. S. Masters, Adv. Virus Res., 2006, 66, 193–292 CrossRef CAS
. - J. B. Mahony, A. Petrich and M. Smieja, Crit. Rev. Clin. Lab. Sci., 2011, 48, 217–249 CrossRef CAS
. - V. M. Corman, O. Landt, M. Kaiser, R. Molenkamp, A. Meijer, D. Chu, T. Bleicker, S. Brünink, J. Schneider, M. L. Schmidt, D. Mulders, B. L. Haagmans, B. van der Veer, S. van den Brink, L. Wijsman, L. G. Goderski, J. L. Romette, J. Ellis, M. Zambon, M. Peiris, H. Goossens, C. Reusken, M. P. G. Koopmans and C. Drosten, Eurosurveillance, 2020, 25, 2000045 Search PubMed
. - N. Zhang, L. Wang, X. Deng, R. Liang, M. Su, C. He, L. Hu, Y. Su, J. Ren, F. Yu, L. Du and S. Jiang, J. Med. Virol., 2020, 92, 408–417 CrossRef CAS
. - Y. Chen, Q. Liu and D. Guo, J. Med. Virol., 2020, 92, 418–423 CrossRef CAS
. - S. T. Ngo, N.
Q. A. Pham, L. T. Le, D. H. Pham and V. V. Vu, Computational determination of potential inhibitors of SARS-CoV-2 main protease, J. Chem. Inf. Model., 2020 DOI:10.1021/acs.jcim.0c00491
. - L. Enjuanes, S. Zuñiga, C. Castaño-Rodriguez, J. Gutierrez-Alvarez, J. Canton and I. Sola, Adv. Virus Res., 2016, 96, 245–286 CAS
. - D. Gentile, V. Patamia, A. Scala, M. T. Sciortino, A. Piperno and A. Rescifina, Mar. Drugs, 2020, 18, 225 CrossRef CAS
. - O. O. Olubiyi, M. Olagunju, M. Keutmann, J. Loschwitz and B. Strodel, Molecules, 2020, 25, 3193 CrossRef CAS
. - C. Huang, Y. Wang, X. Li, L. Ren, J. Zhao, Y. Hu, L. Zhang, G. Fan, J. Xu, X. Gu, Z. Cheng, T. Yu, J. Xia, Y. Wei, W. Wu, X. Xie, W. Yin, H. Li, M. Liu, Y. Xiao, H. Gao, L. Guo, J. Xie, G. Wang, R. Jiang, Z. Gao, Q. Jin, J. Wang and B. Cao, Lancet, 2020, 395, 497–506 CrossRef CAS
. - A. K. Ghosh, M. Brindisi, D. Shahabi, M. E. Chapman and A. D. Mesecar, ChemMedChem, 2020, 15, 907–932 CrossRef CAS
. - M. Hagar, H. A. Ahmed, G. Aljohani and O. A. Alhaddad, Int. J. Mol. Sci., 2020, 21, 3922 CrossRef CAS
. - N. Kumar, A. Awasthi, A. Kumari, D. Sood, P. Jain, T. Singh, N. Sharma, A. Grover and R. Chandra, J. Biomol. Struct. Dyn., 2020, 1–16 Search PubMed
. - A. Saha, A. R. Sharma, M. Bhattacharya, G. Sharma, S. S. Lee and C. Chakraborty, Arch. Med. Res., 2020, 51, 585–586 CrossRef CAS
. - A. Saha, A. R. Sharma, M. Bhattacharya, G. Sharma, S. S. Lee and C. Chakraborty, Arch. Med. Res., 2020, 51, 595–597 CrossRef CAS
. - R. Alexpandi, J. F. De Mesquita, S. K. Pandian and A. V. Ravi, Front. Microbiol., 2020, 11, 1796 CrossRef
. - W. R. Ferraz, R. A. Gomes, A. L. S Novaes and G. H. Goulart Trossini, Future Med. Chem., 2020 DOI:10.4155/fmc-2020-0165
. - N. Kumar, D. Sood, P. J. van der Spek, H. S. Sharma and R. Chandra, J. Proteome Res., 2020 DOI:10.1021/acs.jproteome.0c00367
. - A. R. Fehr and S. Perlman, Methods Mol. Biol., 2015, 1282, 1–23 CrossRef CAS
. - T. Singhal, Indian J. Pediatr., 2020, 87, 281–286 CrossRef
. - Draft landscape of COVID-19 candidate vaccines, https://www.who.int/publications/m/item/draft-landscape-of-covid-19-candidate-vaccines, 28 August 2020 Search PubMed
. - G. Li, Y. Fan, Y. Lai, T. Han, Z. Li, P. Zhou, P. Pan, W. Wang, D. Hu, X. Liu, Q. Zhang and J. Wu, J. Med. Virol., 2020, 92, 424–432 CrossRef CAS
. - L. Gralinski and R. Baric, J. Pathol., 2014, 235, 185–195 CrossRef
. - S. Su, G. Wong, W. Shi, J. Liu, A. Lai, J. Zhou, W. Liu, Y. Bi and G. F. Gao, Trends Microbiol., 2016, 24, 490–502 CrossRef CAS
. - M. T. Qamar, A. Bari, M. M. Adeel, A. Maryam, U. A. Ashfaq, X. Du, I. Muneer, H. I. Ahmad and J. Wang, J. Transl. Med., 2018, 16, 298 CrossRef
. - B. Ahmad, U. A. Ashfaq, M. U. Rahman, M. S. Masoud and M. Z. Yousaf, Microb. Pathog., 2019, 132, 243–253 CrossRef CAS
. - M. U. Mirza, S. Rafique, A. Ali, M. Munir, N. Ikram, A. Manan, O. M. Salo-Ahen and M. Idrees, Sci. Rep., 2016, 6, 37313 CrossRef
. - U. Tahir, M. Qamar, A. Bari, M. M. Adeel, A. Maryam, U. A. Ashfaq, X. Du, I. Muneer, H. I. Ahmad and J. Wang, J. Transl. Med., 2018, 16, 298 CrossRef
. - N. Kumar, D. Sood, N. Sharma and R. Chandra, J. Chem. Inf. Model., 2020, 60, 421–433 CrossRef CAS
. - P. Zhou, X. Yang, X. Wang, B. Hu, L. Zhang, W. Zhang, H. R. Si, Y. Zhu, B. Li, C. L. Huang, H. D. Chen, J. Chen, Y. Luo, H. Guo, R. D. Jiang, M. Q. Liu, Y. Chen, X. R. Shen, X. Wang, X. S. Zheng, K. Zhao, Q. J. Chen, F. Deng, L. L. Liu, B. Yan, F. X. Zhan, Y. Y. Wang, G. F. Xiao and Z. L. Shi, Nature, 2020, 579, 270–273 CrossRef CAS
. - R. Dong, Z. Chu, F. Yu and Y. Zha, Front. Immunol., 2020, 11, 1784 CrossRef
. - A. Naz, F. Shahid, T. T. Butt, F. M. Awan, A. Ali and A. Malik, Front. Immunol., 2020, 11, 1663 CrossRef
. - M. Khan, S. Khan, A. Ali, H. Akbar, A. M. Sayaf, A. Khan and D. Q. Wei, Sci. Rep., 2019, 9, 1–13 CrossRef
. - Y. Lei, F. Zhao, J. Shao, Y. Li, S. Li, H. Chang and Y. Zhang, PeerJ, 2019, 6, e6185 CrossRef
. - M. Bhattacharya, A. R. Sharma, P. Patra, P. Ghosh, G. Sharma, B. C. Patra, S. S. Lee and C. Chakraborty, J. Med. Virol., 2020, 92, 618–631 CrossRef CAS
. - M. Kaurav, J. Madan, M. S. Sudheesh and R. S. Pandey, Artif. Cells, Nanomed., Biotechnol., 2018, 46, S818–S831 CrossRef CAS
. - S. Khan, A. Khan, A. U. Rehman, I. Ahmad, S. Ullah, A. A. Khan, S. S. Ali, S. G. Afridi and D. Q. Wei, Infect., Genet. Evol., 2019, 73, 390–400 CrossRef CAS
. - L. Li and N. Petrovsky, Expert Rev. Vaccines, 2016, 15, 313–329 CrossRef CAS
. - S. Kaliamurthi, G. Selvaraj, S. Chinnasamy, Q. Wang, A. S. Nangraj, W. C. Cho, K. Gu and D. Q. Wei, Viruses, 2019, 11, 63 CrossRef CAS
. - N. Kumar, A. Singh, S. Grover, A. Kumari, P. K. Dhar, R. Chandra and A. Grover, J. Biomol. Struct. Dyn., 2019, 37, 2098–2109 CrossRef CAS
. - C. Chakraborty, A. R. Sharma, M. Bhattacharya, G. Sharma, S. S. Lee and G. Agoramoorthy, J. Med. Virol., 2020 DOI:10.1002/jmv.25997
. - L. Oliveira-Nascimento, P. Massari and L. M. Wetzler, Front. Immunol., 2012, 3, 79 Search PubMed
. - N. Kumar, D. Sood, R. Tomar and R. Chandra, ACS Omega, 2019, 4, 21370–21380 CrossRef CAS
. - D. Sood, N. Kumar, G. Rathee, A. Singh, V. Tomar and R. Chandra, Sci. Rep., 2018, 8, 16964 CrossRef
. - M. Bhasin and G. P. Raghava, Vaccine, 2004, 22, 3195–3204 CrossRef CAS
. - I. A. Doytchinova and D. R. Flower, BMC Bioinf., 2007, 8, 4 CrossRef
. - P. Guan, I. A. Doytchinova, C. Zygouri and D. R. Flower, Nucleic Acids Res., 2003, 31, 3621–3624 CrossRef CAS
. - J. Chen, H. Liu, J. Yang and K. C. Chou, Amino Acids, 2007, 33, 423–428 CrossRef CAS
. - S. Gupta, P. Kapoor, K. Chaudhary, A. Gautam, R. Kumar, Open Source Drug Discovery Consortium and G. P. Raghava, PLoS One, 2013, 8, e73957 CrossRef CAS
. - Y. Feng, M. Qiu, S. Zou, Y. Li, K. Luo, R. Chen, Y. Sun, K. Wang, X. Zhuang, S. Zhang, S. Chen and F. Mo, bioRxiv, 2020 DOI:10.1101/2020.03.03.962332
. - B. Sarkar, M. A. Ullah, F. T. Johora, M. A. Taniya and Y. Araf, Immunobiology, 2020, 225, 151955 CrossRef CAS
. - T. Stratmann, Vaccines, 2015, 3, 579–596 CrossRef CAS
. - C. Chakraborty, A. R. Sharma, M. Bhattacharya, G. Sharma, S. S. Lee and G. Agoramoorthy, J. Med. Virol., 2020 DOI:10.1002/jmv.26078
. - A. W. Purcell, J. McCluskey and J. Rossjohn, Nat. Rev. Drug Discovery, 2007, 6, 404–414 CrossRef CAS
. - D. Kozakov, D. R. Hall, B. Xia, K. A. Porter, D. Padhorny, C. Yueh, D. Dmitri Beglov and S. Vajda, Nat. Protoc., 2017, 12, 255–278 CrossRef CAS
. - C. Chakraborty, A. R. Sharma, G. Sharma, M. Bhattacharya and S. S. Lee, Eur. Rev. Med. Pharmacol. Sci., 2020, 24, 4016–4026 CAS
. - M. I. Abdelmageed, A. H. Abdelmoneim, M. I. Mustafa, N. M. Elfadol, N. S. Murshed, S. W. Shantier and A. M. Makhawi, BioMed Res. Int., 2020, 2020, 2683286 Search PubMed
. - J. A. Kovacs, P. Chacón and R. Abagyan, Proteins: Struct., Funct., Bioinf., 2004, 56, 661–668 CrossRef CAS
. - J. R. López-Blanco, J. I. Garzón and P. Chacón, Bioinformatics, 2011, 27, 2843–2850 CrossRef
. - J. R. López-Blanco, J. I Aliaga, E. S. Quintana-Ortí and P. Chacón, Nucleic Acids Res., 2014, 42, W271–W276 CrossRef
. - N. Rapin, O. Lund, M. Bernaschi and F. Castiglione, PLoS One, 2010, 5, e9862 CrossRef
. - I. Dimitrov, I. Bangov, D. R. Flower and I. Doytchinova, J. Mol. Model., 2014, 20, 2278 CrossRef
. - M. Bhattacharya, A. R. Sharma, P. Patra, P. Ghosh, G. Sharma, B. C. Patra, R. P. Saha, S. S. Lee and C. Chakraborty, Inform. Med., 2020, 20, 100394 Search PubMed
. - A. Grote, K. Hiller, M. Scheer, R. Münch, B. Nörtemann, D. C. Hempel and D. Jahn, Nucleic Acids Res., 2005, 33, W526–W531 CrossRef CAS
. - S. Saha and G. P. S. Raghava, Proteins, 2006, 65, 40–48 CrossRef CAS
.
Footnote |
† Electronic supplementary information (ESI) available. See DOI: 10.1039/d0ra06849g |
|
This journal is © The Royal Society of Chemistry 2020 |
Click here to see how this site uses Cookies. View our privacy policy here.