DOI:
10.1039/D0RA06675C
(Paper)
RSC Adv., 2020,
10, 32856-32874
Pharmacoinformatics approaches to identify potential hits against tetraacyldisaccharide 4′-kinase (LpxK) of Pseudomonas aeruginosa
Received
2nd August 2020
, Accepted 24th August 2020
First published on 4th September 2020
Abstract
Pseudomonas aeruginosa infection can cause pneumonia and urinary tract infection and the management of Pseudomonas aeruginosa infection is critical in multidrug resistance, hospital-acquired bacteremia and ventilator-associated pneumonia. The key enzymes of lipid A biosynthesis in Pseudomonas aeruginosa are promising drug targets. However, the enzyme tetraacyldisaccharide 4′-kinase (LpxK) has not been explored as a drug target so far. Several pharmacoinformatics tools such as comparative metabolic pathway analysis (Metacyc), data mining from a database of essential genes (DEG), homology modeling, molecular docking, pharmacophore based virtual screening, ADMET prediction and molecular dynamics simulation were used in identifying novel lead compounds against this target. The top virtual hits STOCK6S-33288, 43621, 39892, 37164 and 35740 may serve as the templates for the design and synthesis of potent LpxK inhibitors in the management of serious Pseudomonas aeruginosa infection.
1. Introduction
Pseudomonas aeruginosa is a ubiquitous, Gram-negative, rod-shaped, single flagellated bacterium species. It is found in soil, water and air and can reside in a variety of environmental conditions and grows in the optimal temperature range from 25 °C to 37 °C. It can cause infection to humans, animals and plant species and particularly causes serious infections in humans with inherently low immunity or suffering from either cancer, TB, HIV or other any immune suppressed condition. The infections include pneumonia, urinary tract infection, skin and soft-tissue infections.1
Around 10–15 nosocomial infections worldwide in intensive care units in hospital setups are due to P. aeruginosa and the worrisome concern is the higher mortality in these infections. Further, the multi drug resistance the organism has acquired over the period of time is a major concern in its treatment.2 The systemic infection of P. aeruginosa is another challenging infectious condition which is a severe condition and needs timely intervention, quick clinical decisions and utilization of diagnostic advancement to get satisfactory results. It is still a major reason for deaths in hospital-acquired bacteremia and estimated as third leading causes of death due to Gram-negative systemic infection.3,4 P. aeruginosa is also responsible in causing ventilator-associated pneumonia (VAP) during prolonged mechanical ventilation and prior antibiotic therapy in developed and developing countries.5,6 Thus, in recent years much focus is seen to be given on various strategies in the management of P. aeruginosa infections.2 Another major concern in P. aeruginosa organism is its intrinsic and acquired resistance mechanisms which further limits the choices of antimicrobial therapy.7 In this context, understanding the mechanism of multi-drug resistance and identifying newer druggable targets in P. aeruginosa is better approach in drug design.8 The cell wall in Gram-negative organisms like P. aeruginosa maintains the shape, osmotic pressure and act as a barrier for transport of macromolecules.9 The cell wall is composed of an only one layer of peptidoglycan and surrounded by a membrane like structure which is also known as outer membrane in Gram-negative organisms. The outer membrane of Gram-negative bacteria contains a unique component, called as lipo-polysaccharide (LPS) which act as a barrier for the many external agents. LPS consist of three segments, a polysaccharide core, an O-antigen and lipid A. The polysaccharide core is made up of monosaccharide and an O-antigen segment is a linear 50–100 repeated units of monosaccharide. The lipid A segment, an endotoxin of bacteria, is a phosphorylated at polyacylated end of glucosamine containing long chain of statured fatty acids. The lipid A is responsible for the pathogenic responses to mammals where it induces the immune system of host cell.10–12 The enzymes involved in the biosynthetic pathway lipid A (Raetz pathway) can be the potential targets in drug design.13,14 Further, the recent report of Gupta et al. pointed out number of enzymes of lipid A biosynthetic pathway as potential drug targets in pathogenic species of Leptospira.15 In the present study, the key enzymes in lipid A biosynthesis pathway which are essential for the cell wall synthesis and pathogenesis of bacteria cell were explored as drug target.16 Lipid A biosynthesis pathway starts with acylation of UDP-N-acetylglucosamine (UDP-GlcNAc) with 3-hydroxymyristoate-acylcarrier-protein (3-OH-c14-ACP) to form UDP-3-O-acyl-N-acetylglucosamine (UDP-3-O-acylGlcNAc). This reaction is catalyzed by UDP-GlcNAc acyltransferase (LpxA) (Fig. 1).
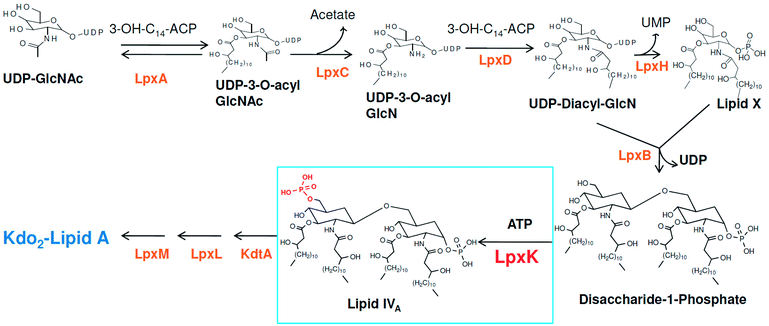 |
| Fig. 1 Lipid A biosynthesis pathway (Raetz pathway). | |
N-acetyl group undergoes deacylation in second step where UDP-3-O-acylGlcNAc is deacylated to UDP-3-O-acyl-N-glucosamine (UDP-3-O-acylGlcN) in presence of UDP-3-O-acyl-N-acetylglucosamine deacetylase (LpxC). The UDP-3-O-acyl-N-glucosamine so formed undergoes acylation at 2-OH group forming UDP-2,3-diacylglucosamine (UDP-2,3-diacyl-GlcN) in presence of another acetyltransferase (LpxD). It is converted into 2,3-diacylglucosamine-1-phosphate (lipid X) and a hydrolase enzyme (LpxH) catalyze this conversion. A tetraacyldisaccharide-1-phosphate is formed from condensation one unit of UDP-2,3-diacyl-GlcN and one unit of lipid X in presence of LpxB enzyme. The lipid A 4′-kinase (LpxK) transfers the gamma-phosphate of ATP to the 4′-position of a tetraacyldisaccharide 1-phosphate intermediate to form tetraacyldisaccharide 1,4′-bis-phosphate (lipid IVA). Further, through sequence of few biosynthetic steps catalyzed by enzymes such as KdtA, LpxL and LpxM the lipopolysaccharide KDO2-lipid A is formed, where KDO is a 3-deoxy-D-manno-oct-2-ulosonic acid unit.17–21 Some potent inhibitors of lipopolysaccharide biosynthesis have been reported against LpxA, LpxC, LpxD and LpXH of various bacterial species22–30 (Fig. 2).
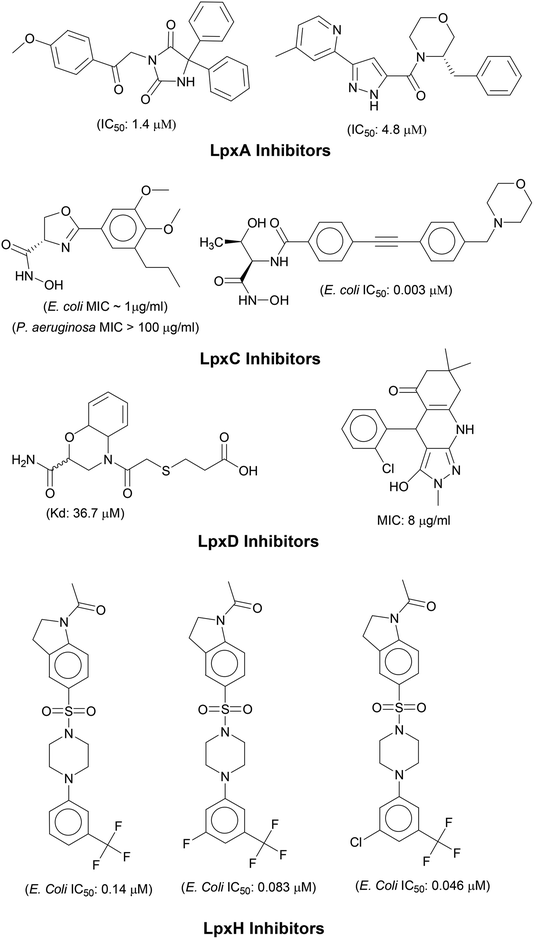 |
| Fig. 2 List of lipopolysaccharide biosynthesis Inhibitors. | |
The tetraacyldisaccharide 4′-kinase (LpxK) of P. aeruginosa is an important target in the biosynthesis of lipopolysaccharide. The structure of LpxK was modeled and used in the design novel natural compound analogues through docking based virtual screening. The sequence of P. aeruginosa tetraacyldisaccharide 4′-kinase gene (EMBL-EBIID QHF88678)31 was used and its profile which includes the interactions and protein features is available at UniProtKB database (Q9HZM3).32 Homology modeling is the best method to build the theoretical models of proteins if experimentally solved crystal structures are unavailable.33 These homology models can be used for further molecular modeling purpose such as docking studies. Here, the molecular docking method is one of the best methods to understand the binding mode and the affinities of inhibitors.34 Such molecular modeling approaches has been successful for identification of many potential anti-cancer and antibacterial agents.35–37 The results of the docking studies such as lowest binding free energies and the structural requirement to elicit the best binding affinity can be used in constructing the pharmacophore.38 This Pharmacophore was subjected to virtual screening to derive the promising hits.39 Further, the absorption, distribution, metabolism, elimination and toxicity (ADMET) characteristics were investigated to assess the effects and risk of the promising hits.40,41 Molecular docking studies results can be augmented well with molecular dynamics simulations wherein the detail investigation of binding modes and prediction of binding affinities is possible.
2 Materials and methods
2.1 Homology modeling
MetaCyc Metabolic Pathway Database (https://metacyc.org/) was explored to identify the P. aeruginosa specific metabolic pathways.42–44 The choke point finder tool implemented in this database was used to identify the key enzymes in these pathways. The essential genes and the corresponding metabolic enzymes of P. aeruginosa were explored in the database of essential genes (DEG) (http://www.essentialgene.org).45 The sequence of the key metabolic enzyme thus identified was retrieved from the Uniprot database (https://www.uniprot.org/).46 The sequence was subjected to the BLASTP search to identify the matching protein templates. These preliminary investigations lead to the identification of tetraacyldisaccharide 4′kinase (LpxK), the unique protein specific for the P. aeruginosa. The sequence of the P. aeruginosa LpxK was subjected to the similarity search in the Schrödinger Prime Module.47 Multiple template selection option with OPLS3e force filed was used to generate the homology models.48 The sitemap module of Schrödinger was used to identify the binding site. In this module the atoms on the protein's surface were identified and possible potential hydrophobic and hydrophilic regions were assigned. The hydrophilic regions are further categorized as hydrogen-bond donor, hydrogen-bond acceptor, and metal-binding regions. The resulting regions were clustered as potential binding sites on the protein's surface. Ten potential binding sites were identified and ranked on the basis of the binding site volume and the top ranked binding site was used for further studies.
2.2 Examination of substrate analogue and designing inhibitors
In order to design inhibitors of LpxK, active site analysis of template protein (PDB ID: 4EHX) LpxK was done.49 The bound substrate is piperazine based 4-(2-hydroxyethyl)-1-piperazine ethanesulfonic acid which suggested that sulfate group of piperazine core is bound to the side chains ARG72, ARG119 and HIS143 residue of template LpxK active site. The sitemap module of Schrödinger predicted the active site on modeled LpxK with residues TYR28, VAL31, ARG35, ASN58, VAL61, GLY62, THR64, LYS66, SER88, ARG89, GLY90, TYR91, GLU114, PRO115, ARG72, ARG132, ASP151, ASP152, GLN155, HIS156, LEU180, ARG186 and GLU187.50 The structural information of piperazine based analogue from template and physicochemical properties of active site residue were used to design the inhibitor compounds. The structural information of already known LpxA, LpxC, LpxD, and LpxH inhibitors (Fig. 2) and the isosteric replacement approach was also used to design the inhibitors as shown in Fig. 3. The core piperazine ring present in LpxK inhibitor was replaced by imidazole ring. This replacement was based on the isosteric similarity to the oxazoline ring in LpxC inhibitors. Further, the bioisosteric groups,51 either hydroxyl ethyl group as present in LpxK inhibitor or hydroxamic acid group as present in LpxC inhibitor, were substituted at 2nd position of the imidazole ring. The para substituted benzene sulfonyl substituent inspired from LpxH inhibitor was used as a substituent at 5th position of imidazole ring. Various substituents which offers hydrogen bond donor or hydrogen bond acceptor capabilities and which represent synthetically feasible groups from Topliss tree52 were substituted at the para position of benzene ring to arrive at the scaffold of designed LpxK inhibitors.
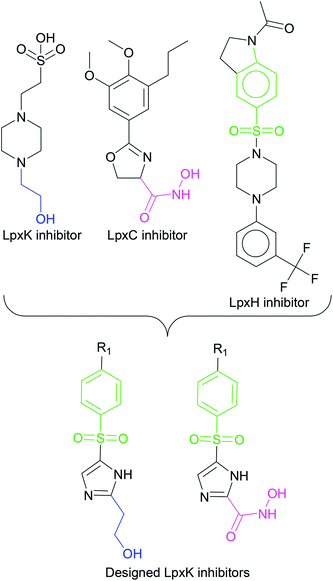 |
| Fig. 3 Design strategy of LpxK inhibitors. | |
2.3 Molecular docking
Molecular docking simulations were carried out on Schrödinger Glide.53 The validated homology model of LpxK was curetted through structure preparation wizard.54 The binding site predicted through sitemap module was used for generating the grid.50 The 2D structures of bound ligand 4-(2-hydroxyethyl)-1-piperazine ethanesulfonic acid and designed inhibitors were drawn with ligand preparation and library design wizard (Table 1).
Table 1 Designed Pseudomonas aeruginosa LpxK inhibitors
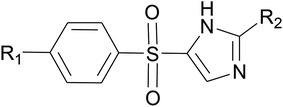
|
Compound no. |
R1 |
R2 |
IMMD01 |
–H |
–CH2CH2OH |
IMMD02 |
–OH |
–CH2CH2OH |
IMMD03 |
–NH2 |
–CH2CH2OH |
IMMD04 |
–SH |
–CH2CH2OH |
IMMD05 |
CH3 |
–CH2CH2OH |
IMMD06 |
–OCH3 |
–CH2CH2OH |
IMMD07 |
–CF3 |
–CH2CH2OH |
IMMD08 |
–NO2 |
–CH2CH2OH |
IMMD09 |
–COOH |
–CH2CH2OH |
IMMD10 |
–COCH3 |
–CH2CH2OH |
IMMD11 |
–COOCH3 |
–CH2CH2OH |
IMMD12 |
–Cl |
–CH2CH2OH |
IMMD13 |
–Br |
–CH2CH2OH |
IMMD14 |
–F |
–CH2CH2OH |
IMMD15 |
–CONH2 |
–CH2CH2OH |
IMMD16 |
–H |
–CONHOH |
IMMD17 |
–OH |
–CONHOH |
IMMD18 |
–NH2 |
–CONHOH |
IMMD19 |
–SH |
–CONHOH |
IMMD20 |
CH3 |
–CONHOH |
IMMD21 |
–OCH3 |
–CONHOH |
IMMD22 |
–CF3 |
–CONHOH |
IMMD23 |
–NO2 |
–CONHOH |
IMMD24 |
–COOH |
–CONHOH |
IMMD25 |
–COCH3 |
–CONHOH |
IMMD26 |
–COOCH3 |
–CONHOH |
IMMD27 |
–Cl |
–CONHOH |
IMMD28 |
–Br |
–CONHOH |
IMMD29 |
–F |
–CONHOH |
IMMD30 |
–CONH2 |
–CONHOH |
The 2D structures were transformed to most stable 3D conformers and subsequently energy minimized using OPLS3e force field.55 The ligand docking was carried out with Schrödinger GlideXP module.56 The docked ligands where scored and ranked as per their binding affinity score (G-score). The accuracy of docking protocol was ensured through the resulting root mean square deviations and deviations in docking scores.57
2.4 Pharmacophore modeling and virtual screening
The e-pharmacophore model was constructed from the top ranked docked conformers of the designed inhibitors.58 The structural and energy pharmacophoric features were obtained from phase module of Schrödinger suite.59 The virtual screening through phase module of the Schrödinger was carried out to developed 3D-pharmacophoric model.60 The InterBioScreen natural database (https://www.ibscreen.com/natural-compounds) consisting of 60
000 natural compounds and 485
000 synthetic compounds was screened against this pharmacophore.61
2.5 Enrichment calculation
The created hypothetic pharmacophore model was validated. In this validation step whether the hypothetic pharmacophore maps to the set of active ligands in a pool of active and inactive ligands was investigated. In this the enrichment calculation was carried out from a large decoy set and set of active ligands using enrichment calculator tool of Schrödinger suite.62 The decoy set of molecules were downloaded from Schrödinger decoy set database (https://www.Schrxf6dinger.com/glide) and molecular weight of average 400 kDa was used for the enrichment calculation.63 In total, 1030 compounds, consisting of 1000 decoys and 30 designed inhibitors were used to assess the selectivity and specificity of hypothesized model. The parameters like enrichment factor (EF) and receiver operating characteristic curve value (ROC) were calculated to check the accuracy of pharmacophore model to identify the known actives.64
2.6 Prime MM-GBSA
Molecular Mechanical/Generalized Born Surface Area calculations (MM-GBSA) are more accurate approximations of binding affinities and binding free energy estimates than the docking G-scores estimated through Glide XP module. Prime MM-GBSA (Molecular Mechanical/Generalized Born Surface Area) analysis module of Schrödinger suite was used to perform these calculations.65 The MM-GBSA calculations are based on the estimation of binding free energy in kcal mol−1 from the energy for protein–ligand complex, ligand, and solvated protein.66,67 The screened hits with best glide XP G-score and lowest binding free energies were subjected to ADMET and molecular dynamics simulation studies.
2.7 ADMET analysis
The ADMET screening involves the prediction of drug likeliness and the pharmacokinetic profile of screened hits. The QikProp module of Schrödinger suite was employed for the ADMET screening for the predication of drug like properties.68 The most informative properties such as partition coefficient (QP log
Po/w), water solubility (QP log
S), the number of hydrogen bond donor groups (HBD), the number of hydrogen bond acceptor groups (HBA), molecular weight (MW), percentage human oral absorption, predicted IC50 value for blockage of HERG K+ channels (QPlogHERG), Caco-2 cell permeability (QPPCaco),predicted brain/blood partition coefficient (QPlogBB), predicted apparent MDCK cell permeability (QPPMDCK), predicted skin permeability (QPlogKp) and number of violations of Lipinski's rule of five (rule of five) were determined.69,70 Among the top 20 screened compounds, those compounds complying the Lipinski rule of five for drug likeliness (molecular weight < 500, HBD < 5, HBA < 10, and QP log
Po/w < 5), and an acceptable pharmacokinetic parameters were further considered.
2.8 Molecular dynamics (MD) simulation and MM-PBSA calculations
MD simulations using Gromacs 4.5.6 (ref. 71 and 72) was carried out on the top ranked hits with lowest binding free energies and with acceptable ADMET. The production phase 25 nanosecond MD simulations were carried out on remote server of the Bioinformatics Resources and Applications Facility (BRAF), C-DAC, Pune.Gromos54a7 force field73 was chosen to generate the topology of the protein while the ligand topologies were generated from ATB server.74,75 The system was solvated using simple point charge (SPC216) model in a cubic unit cell the system and neutralized with addition of appropriate ions such as sodium and chloride. Steric clashes were removed through unrestrained energy minimization of the system with steepest descent criteria. Further, the system was equilibrated with constant volume and the pressure position restraint dynamics at constant temperature of 300 K for 100 picoseconds. The 25 ns production phase MD simulation was carried out with all covalent bonds restrained with the LINCS algorithm.76 Particle Mesh Ewald method (PME)77 was used to control the long range electrostatics such as Coulomb and Lennard Jones interactions with the cutoff value of 12 Å. The analysis of MD trajectories was carried out with respect to the root mean square deviations (RMSD) in the protein atoms and atoms in hit molecules. Other analysis such as root mean square fluctuations (RMSF) in the residues, the frequency of hydrogen bonds formed during progress of MD and the residues involved in hydrogen bond interactions at various time intervals was carried out. The molecular mechanics energies combined with the Poisson Boltzmann surface area continuum solvation (MM-PBSA) which uses trajectories at various time intervals and it is more accurate than the MM-GBSA calculations of single protein–ligand complex was also carried out to derive estimates of binding free energies of each hit molecule.
3 Results and discussion
3.1 Homology modeling
The analysis of Metacyc metabolic pathways of P. aeruginosa showed four unique metabolic pathways responsible for lipid synthesis of cell wall which can serve as promising targets in the drug design. Further analysis of these enzymes suggested that the tetraacyldisaccharide-1-phosphate 4′-kinase (LpxK) is an essential enzyme in the synthesis of bacterial cell wall and it is not expressed in Homo sapiens. This enzyme was also identified as the key genes essential for the survival of the P. aeruginosa at through the database of essential genes. The primary sequence of the LpxK has 333 amino acids. The BLAST results suggest that the template of the X-ray crystallographic structure of the LpxK (PDB ID: 4EHX) has the sequence identity of 30% and positives value of 49% with the best model. In order to build good homology model of LpxK, multiple templates of known X-ray crystallographic structure of Aquifex aeolicus (PDB ID: 4EHW, 4EHX and 4EHY) were used. The atomic resolution being the key parameter for the selection of the template structures, these template structures with atomic resolution 2.3, 1.9 & 2.2 Å were found appropriate in building homology model of P. aeruginosa specific LpxK. The similarity and identity was mapped with the sequence alignment of template and homology model in Schrödinger Prime STA Editor and the results are shown in Fig. 4. The modeled protein structure was further subjected to loop refinement and energy minimization using VSGB solvation model and OPLS3e force field.
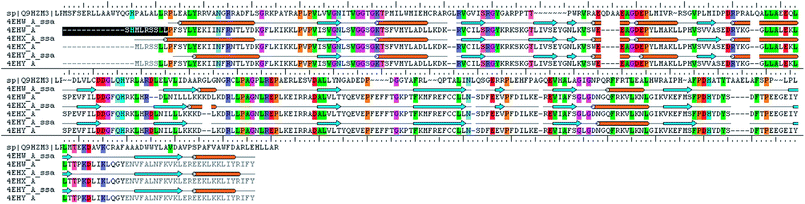 |
| Fig. 4 Sequence alignment of model and template. | |
The tools like Procheck, ProSA and SPDBV78–80 were used to validate the built homology models. The Cα deviation and all atom fit was calculated in SPDBV tool and the values 0.6 and 0.3 Å respectively for these measurements suggested that the model structure is reasonably good for further computational studies. The alignment of homology model and template structure is shown in Fig. 5.
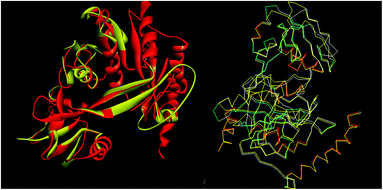 |
| Fig. 5 Structural alignment of homology model and template structure (A) all atom alignment (B) Cα backbone alignment. | |
Further, the homology model was checked for the structural integrity through the residue occupancies in Ramachandran plot. It was found that 98.1% residues are in allowed region (Fig. 6). ProSA-web server uses the atomic co-ordinates of each residue of the protein and provides the Z score which is the estimate of errors in experimental and theoretical models. This score also provides the impetus on overall quality of model protein which is determined on the basis of Z scores of all experimentally solved protein structures. The Z score of −5.48 suggest overall good quality of model protein.
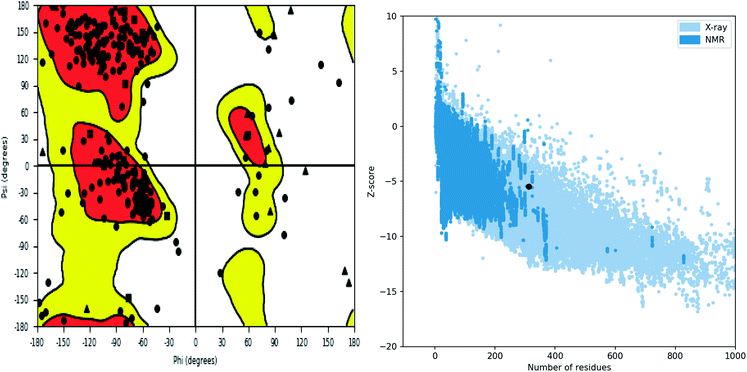 |
| Fig. 6 Structure validation parameter Ramachandran plot and ProSa Z score of model structure. | |
The residues TYR28, ASN58, THR64, SER88, ARG89, GLY90, TYR91, GLU114, PRO115, ARG132, ASP151, ASP152, GLN155, HIS156 and ARG186 were found as the key residues at the binding pocket contributing in various types of interactions (Fig. 7). The binding site of the model and template structures correlated well in terms of the presence of these residues.
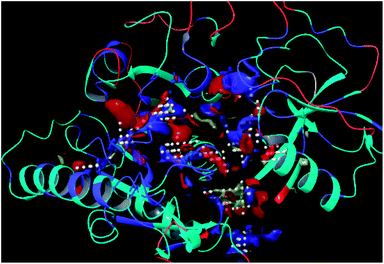 |
| Fig. 7 LpxK binding site residues predicted by sitemap finder module of Schrödinger. | |
3.2 Molecular docking
The residues producing interactions with the bound piperazine derivative at the binding site of LpxK were analyzed. All the designed inhibitors were docked at the binding site of LpxK. The results suggest that the residues TYR28, ASN58, THR64, SER88, ARG89, GLY90, TYR91, GLU114, PRO115, ARG132, ASP151, ASP152, GLN155, HIS156 and ARG186 are the key residues at the binding pocket. These residues form the interactions with the core scaffold of designed inhibitors. The details of interacting residues and the type of key interactions along with the structures of designed inhibitors are provided in the Table 2.
Table 2 Docking results
Comp. no. |
Glide_XP docking score (kcal mol−1) |
Interacting residues |
Kinds of interactions |
H-Bond |
vdW |
Pi |
IMMD01 |
−3.56 |
LYS66, SER88, ASP151, ASP152 |
2 |
2 |
2 |
IMMD02 |
−3.49 |
ASN58, THR60, SER88, ARG89, ASP151, ASP152 |
3 |
2 |
2 |
IMMD03 |
−3.39 |
ALA32, ARG35, ARG89, ASP152, GLN155, ARG186, GLU187 |
3 |
3 |
1 |
IMMD04 |
−2.80 |
ASN58, THR64, LYS66, ARG89, TYR91, ASP152 |
2 |
2 |
2 |
IMMD05 |
−3.49 |
LYS66, ARG89, GLU114, ASP151, ASP152 |
3 |
2 |
2 |
IMMD06 |
−3.65 |
ALA32, ARG35, ARG89, ASP152, ARG186, GLU187 |
3 |
3 |
2 |
IMMD07 |
−3.49 |
ARG89, GLY90, TYR91, GLY92, THR97, ILE128, PRO130 |
3 |
2 |
3 |
IMMD08 |
−3.83 |
ALA32, ARG35, ARG132, HIS156, ARG186, GLN187 |
3 |
3 |
1 |
IMMD09 |
−4.40 |
ASN58, THR64, SER88, ARG89, GLY90, ASP151, ARG186 |
3 |
4 |
1 |
IMMD10 |
−4.57 |
TYR28, ALA32, ARG35, ARG132, HIS156, ARG186 |
3 |
4 |
1 |
IMMD11 |
−3.78 |
ALA32, ARG35, ARG132, ASP152, HIS156, ARG186 |
3 |
4 |
1 |
IMMD12 |
−4.01 |
ALA32, ARG35, HIS156, ARG186, GLU187 |
2 |
3 |
2 |
IMMD13 |
−3.99 |
ALA32, ARG35, HIS156, ARG186, GLU187 |
2 |
4 |
2 |
IMMD14 |
−3.72 |
ARG89, TYR91, GLU114, ASP151 |
2 |
4 |
1 |
IMMD15 |
−3.69 |
ASN58, SER88, ARG89, GLY90, TYR91, ASP152, ARG189 |
3 |
2 |
2 |
IMMD16 |
−4.22 |
LYS66, SER88, ASP151, ASP152 |
2 |
2 |
2 |
IMMD17 |
−3.17 |
SER88, ARG89, GLY90, TYR91, ASP152 |
2 |
3 |
3 |
IMMD18 |
−3.48 |
HIS124, ASP150, ARG187, ARG269, LEU271 |
2 |
1 |
4 |
IMMD19 |
−4.09 |
ARG89, GLY90, TYR91, ASP151 |
2 |
2 |
3 |
IMMD20 |
−3.94 |
TYR28, ARG89, ARG132, ASP152, GLN155 |
3 |
3 |
2 |
IMMD21 |
−4.28 |
SER88, ARG89, GLY90, TYR91, ASP152 |
2 |
2 |
2 |
IMMD22 |
−4.40 |
VAL61, ARG89, GLY90, ASP151, ASP152, ARG186 |
2 |
2 |
2 |
IMMD23 |
−3.94 |
ASN58, THR64, LYS66, ARG89, TYR91, ASP152 |
2 |
4 |
3 |
IMMD24 |
−5.53 |
TYR28, ALA32, ARG35, ARG89, ARG132, HIS156, GLU187 |
2 |
4 |
2 |
IMMD25 |
−5.55 |
AGR89, GLY90, ASP152, ARG186 |
2 |
2 |
3 |
IMMD26 |
−4.62 |
ARG89, GLY90, TYR91, ASP151 |
3 |
3 |
1 |
IMMD27 |
−4.12 |
ARG35, ARG89, PRO130, ARG132, ASP152, HIS156 |
2 |
4 |
2 |
IMMD28 |
−4.21 |
ARG89, GLY90, TYR91, ASP151 |
2 |
2 |
1 |
IMMD29 |
−3.30 |
ARG35, ARG89, ASP152, GLN155, HIS156, ARG186 |
2 |
2 |
3 |
IMMD30 |
−6.63 |
SER88, AGR89, GLY90, ASP152, ARG186 |
4 |
4 |
3 |
Glide module uses an empirical scoring function called GlideScore which approximates the ligand binding free energy. In structure based drug design the choice of any tool used is based on its reliability in predicting the binding affinities and ligand binding free energies for ligand–receptor complexes.81,82 The imidazole analogue IMMD30 has the lowest binding free energy −6.634 kcal mol−1. The –CONH2 substituent on phenyl ring forms two hydrogen bond interactions, one between –NH2 of amide and SER88 and other between carbonyl oxygen of amide and GLY90 (Fig. 8). The hydroxamic acid hydroxyl group and amino group forms hydrogen bond with charged ASP152 residue. The residue ARG89 forms a salt bridge interaction with deprotonated imidazole nitrogen and also a π–π stacking interaction with imidazole ring. The residue Arg89 also forms π–π stacking interaction with imidazole ring. These interactions could be the key interactions necessary for LpxK inhibitory potential.
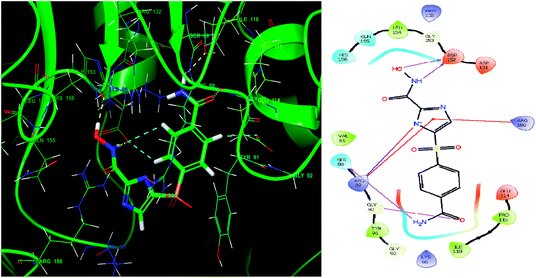 |
| Fig. 8 The binding pose and molecular interactions of inhibitor IMMD30 into the active site of the model structure of the LpxK. | |
3.3 Pharmacophore-model construction, enrichment analysis and virtual screening
The pharmacophoric features have some functionality which contributes in eliciting the activity of the inhibitors. The key pharmacophoric features (ADDRR) such as the hydrogen bond acceptor (A), hydrogen bond donor (D) and the aromatic ring (R) responsible for the inhibitory activity were identified. The key interactions the top ligands make at the binding site were exploited to construct the pharmacophoric model. The set of predefined features such as, hydrogen bond donor (D), hydrogen bond acceptor (A), aromatic ring (R) from the interactions of most active designed ligand IMMD30 were exploited (Fig. 9).
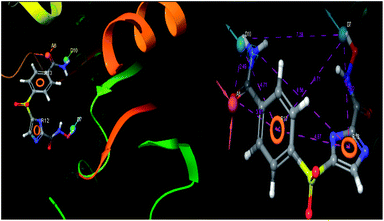 |
| Fig. 9 3D-pharmacophore models of the top docked hit IMMD30 into the active site of the homology model of the LpxH. | |
The results of the pharmacophore design suggest that the designed ligand IMMD30 has four key pharmacoporic features namely two aromatic ring sites (R12 & R13, designated as R), two hydrogen bond donor (D7& D10 designated as D) and one hydrogen bond acceptor (A, designated as A6). The two aromatic rings sites are phenyl ring and imidazole ring respectively. The hydrogen atoms of carboxamido –NH2 and hydroxamic acid –OH group are donor groups while the carbonyl oxygen of carboxamido group is acceptor site. This pharmacophore was subjected to virtual screening of InterBioScreen natural and synthetic compounds database as per the protocol shown in Fig. 10.
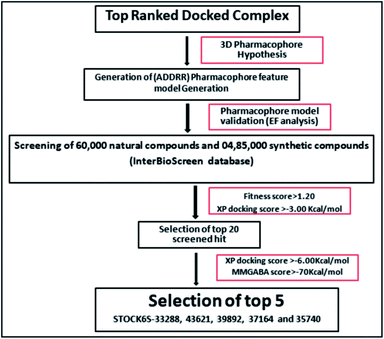 |
| Fig. 10 Schematic representation of the virtual screening protocol. | |
The enrichment calculator tool of Schrödinger suite was used to verify the effectiveness of a ligand database screening seeded with known actives. The enrichment factor score 98 suggests high predictive power of pharmacophore in predicting actives from decoy set with high recovery rate. The further validation of ROC parameter was done which focuses on correlation between the sensitivity (true positive) and specificity (false positive) of a test as shown in ROC plot in Fig. 11. The ROC value of 0.96 indicates that the pharmacophore model has a capacity to precisely predict the active molecules from diverse set of molecules with desired inhibitory activity. The ROC curve plot sharp progression indicates that model has the capacity to predict the actives in the start of the screening process. Moreover, enrichment analysis suggests that pharmacophore model is perfect for further virtual screening.
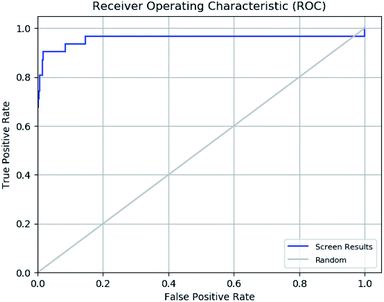 |
| Fig. 11 Receiver operating characteristic (ROC) curve of generated e-pharmacophore model (ADDRR). | |
The pharmacophoric features the hydrogen bond acceptor (A), two hydrogen bond donors (D) and two aromatic rings (AR) were chosen as the filtering criteria during virtual screening. The virtual screening experiment gave 102 hits with fitness score >0.999. These virtual hits were subjected to docking studies. The top 20 hits with docking score < −4.0 (kcal mol−1) were chosen for further studies (Table 3).
Table 3 Systematic representation of in silico docking data of the top ranked virtual hits
S. no. |
Hits_ID |
Glide_XP docking score (kcal mol−1) |
Interacting residues |
Kinds of interactions |
H-Bond |
vdW |
Pi |
1 |
STOCK6S-33288 |
−6.79 |
VAL61, SER88, ARG89, GLY90, TYR91, PRO115 ASP151, ASP152 |
7 |
2 |
2 |
2 |
STOCK6S-43621 |
−6.65 |
VAL61, SER88, ARG89, GLY90, TYR91, PRO115, ASP152 |
4 |
2 |
4 |
3 |
STOCK6S-39892 |
−6.63 |
SER88, ARG89, GLY90, ASP152, ARG186 |
3 |
2 |
3 |
4 |
STOCK6S-37164 |
−6.52 |
VAL61, SER88, ARG89, GLY90, TYR91, PRO115 ASP151, ASP152 |
4 |
2 |
2 |
5 |
STOCK6S-35740 |
−6.28 |
SER88, ARG89, GLY90, TYR91, ASP152 |
3 |
3 |
3 |
6 |
STOCK3S-95781 |
−5.791 |
TYR28, ARG35, ARG89, ARG132, HIS156, LEU180, PRO181, ARG186 |
3 |
2 |
4 |
7 |
STOCK4S-19314 |
−5.769 |
TYR28, ARG35, ARG89, ARG132, HIS156, LEU180, PRO181, ARG186 |
2 |
2 |
9 |
8 |
STOCK4S-13117 |
−5.697 |
VAL31, ARG35, ARG89, ARG132, GLN155, HIS156, ARG186 |
2 |
2 |
7 |
9 |
STOCK4S-16029 |
−5.644 |
TYR28, ARG35, ARG89, ARG132, HIS156, LEU180, PRO181, ARG186 |
2 |
2 |
7 |
10 |
STOCK4S-14901 |
−5.619 |
VAL31, ARG35, ARG89, ARG132, HIS156 |
2 |
2 |
4 |
11 |
STOCK4S-09064 |
−5.599 |
GLY62, THR64, ARG89, ARG186 |
3 |
1 |
3 |
12 |
STOCK4S-09935 |
−5.556 |
ALA32, ARG35, ARG89, ARG132, HIS156, GLU187 |
2 |
3 |
6 |
13 |
STOCK6S-33158 |
−5.555 |
LYS66, ARG89, GLY90, SER88, ASP151, ASP152 |
3 |
3 |
1 |
14 |
STOCK3S-87292 |
−5.553 |
GLY62, LYS66, THR64, SER88, ARG89, GLY90, TYR91, ASP152 |
3 |
4 |
2 |
15 |
STOCK4S-28410 |
−5.52 |
TYR28, ARG89, ARG132, HIS156, LEU180, ARG186 |
1 |
1 |
7 |
16 |
STOCK4S-00141 |
−5.478 |
TYR28, ARG89, ARG132, HIS156, LEU180, ARG186 |
1 |
1 |
8 |
17 |
STOCK3S-98611 |
−5.427 |
TYR28, ALA32, ARG35, ARG89, ARG132, HIS156, ARG186 |
2 |
2 |
4 |
18 |
STOCK1S-95046 |
−5.425 |
ARG35, ARG89, ARG132, HIS156, ARG186 |
1 |
2 |
3 |
19 |
STOCK4S-20723 |
−5.394 |
VAL61, GLY62, THR64, ARG89, ARG186 |
2 |
2 |
2 |
20 |
STOCK4S-09935 |
−4.791 |
TYR28, ALA32, ARG35, ARG89, ARG132, HIS156, ARG1861 |
2 |
2 |
3 |
The structures of five top virtual hits STOCK6S-33288, 43621, 39892, 37164 and 35740 are shown in Fig. 12. The virtual hits STOCK6S-33288, 43621, 39892, 37164 and 35740 showed low binding free energy of −6.79, −6.65, −6.63, −6.52 and −6.28 kcal mol−1 respectively. These virtual hits were found making hydrogen bond and hydrophobic π–π stacking interactions with the residues HIS35, LYS44, GLY62, THR64, HIS86, GLU87, SER88, ARG89, GLY90, TYR91, ARG132, ASP151, ASP152, HIS156 and ARG186. These interactions with the docked poses of the hit molecules at the binding site are shown in Fig. 13.
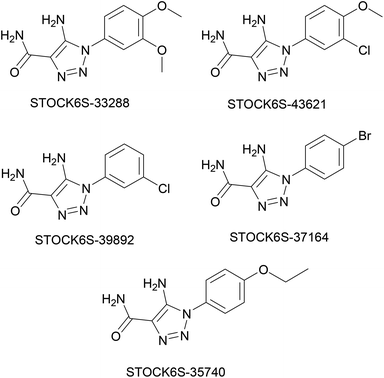 |
| Fig. 12 Structures of top five virtual hits. | |
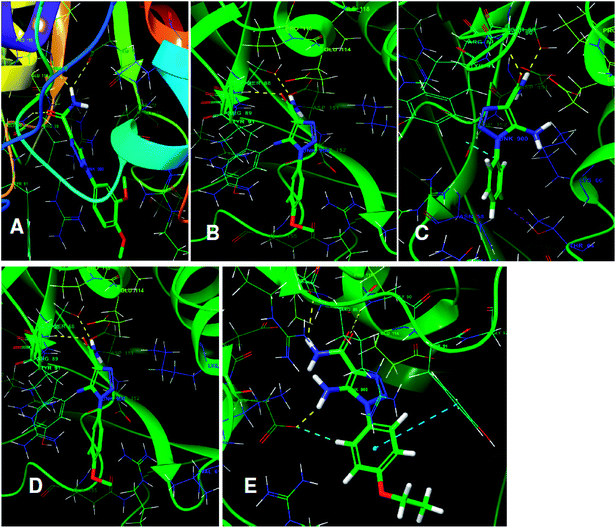 |
| Fig. 13 The binding pose of top hits at binding site, (A) STOCK6S-33288; (B) STOCK6S-43621; (C) STOCK6S-39892; and (D) STOCK6S-37164; (E) STOCK6S-35740. | |
The hits having the matching features of best docked ligands may have the highest potential to inhibit the LpxK of the P. aeruginosa. The docked conformer of the 5-(4-carbamoylbenzenesulfonyl)-N-hydroxy-1H-imidazole-2-carboxamideanalogue IMMD30 with lower binding free energy and the docked pose of the potential virtual hit STOCK6S-33288 at the binding site is shown in Fig. 14.
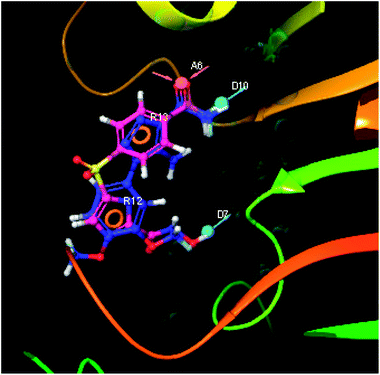 |
| Fig. 14 The docked conformers of ligand IMMD30 (magenta) and top virtual hit STOCK6S-33288 (blue) at the binding site. | |
3.4 Docking and MM-GBSA analysis
In order to predict binding mode and to calculate free energy of binding of the top hits, docking studies were carried out GLIDE module of the Schrödinger suite. Estimation of binding free energies of highly flexible protein–ligand complexes is very challenging due to insufficient sampling of the system.83 The methods such as molecular mechanics continuum solvent, the linear interaction energy (LIE), free-energy perturbation (FEP), and the thermodynamic integration (TI) approach are used in binding free energy calculations.84,85 Especially, the statistical mechanics and molecular dynamics based methods are known to predict the binding free energies accurately.86–88 The glide G-scores which is an approximation of binding free energy for top five hits in pharmacophore based virtual screening namely STOCK6S-33288, STOCK6S-43621, STOCK6S-39892, STOCK6S-37164, STOCK6S-35740 were −6.79, −6.65, −6.63, −6.52, −6.28 kcal mol−1 respectively and comparably very close to the reference compound IMMD30 with G-score −6.63 kcal mol−1. These top five hits were further subjected to MM-GBSA calculation to predict free energy of binging and the possible binding affinity. The MM-GBSA binding energy estimate for IMMD30 was found the lowest (−80.17 kcal mol−1) and all the top hits have the estimated binding free energies in the range −70.0 to −75.49 kcal mol−1 (Table 4). These estimated binding free energies suggest that the top hits may have good binding affinity to LpxK enzyme of P. aeruginosa.
Table 4 Docking scores, fitness scores, and energy involvement of IMMD30 and hit molecules against LpxH
Sr. no. |
Lead/hit Id |
XP G_score kcal mol−1 |
Glide energy kcal mol−1 |
Glide gscore kcal mol−1 |
Fitness score |
Docking score kcal mol−1 |
Binding energy/MM-GBSA kcal mol−1 |
1 |
IMMD30 |
−6.63 |
−39.624 |
−6.63 |
1.50 |
−6.63 |
−80.17 |
2 |
STOCK6S-33288 |
−6.79 |
−30.994 |
−6.79 |
1.321 |
−6.79 |
−75.49 |
3 |
STOCK6S-43621 |
−6.65 |
−34.436 |
−6.65 |
1.286 |
−6.65 |
−70.48 |
4 |
STOCK6S-39892 |
−6.63 |
−32.958 |
−6.63 |
1.255 |
−6.63 |
−70 |
5 |
STOCK6S-37164 |
−6.52 |
−32.918 |
−6.52 |
1.211 |
−6.52 |
−70.58 |
6 |
STOCK6S-35740 |
−6.28 |
−33.184 |
−6.28 |
1.200 |
−6.28 |
−70.03 |
3.5 In silico ADME predictions
The prediction of drug likeliness of possible hit molecules is an important step in drug discovery process. The physicochemical properties that affect absorption, distribution, metabolism, elimination and toxicity risk characteristics were studied and are given in Table 5. QikProp module of Schrödinger predicted 50 descriptors properties that affect the pharmacokinetic profile of the drug like molecules including the properties such as (molecular weight < 500, HBD < 5, HBA < 10, and log
P < 5, TPSA < 150). The hydrophobicity prediction (log
Po/w value) was the key parameter which is important aspect of absorption, solubility and membrane permeability requirement for a potential drug. For all the screened hits log
Po/w value is in the permissible range of −2.0 to 6.5. The availability of the drug to central nervous system is key requirement for many disease conditions and predicted CNS value for all screened hits lies in the range of −2 (inactive) and +2 (active). Predicted IC50 value for blockage of HERG K+ channels value is important in metabolism of the drug and it was found that all hits have blockage of HERG K+ channels value > −5. QPPCaco gives information about predicted apparent Caco-2 cell permeability in gut-blood barrier for non active drugs. The top screened hits were found having good QPPCaco value > 25. The QPlogBB, QPPMDCK and QPlogKp values give and information about lipophilic and lipophilic properties of the drug molecules which is crucial for the permeability of the drug across many cell membranes in the body and it was found that QPlogBB, QPPMDCK and QPlogKp values are within the permissible limits and hydrophilic nature (Table 5). The human oral absorption and percentage of human oral absorption values gives an idea about rate and amount of absorption and bioavailability of the drug in the blood and it was found that all the screened hits has medium to high rate of oral absorption and high percentage of oral absorption.
Table 5 Pharmacokinetic parameters of virtual hits for good oral bioavailability
Title |
QPlogPo/w |
QPlogHERG |
QPPCaco |
QPlogBB |
QPPMDCK |
QPlogKp |
#Metab |
QPlogKhsa |
HumanOralAbsp |
% HumanOralAbsp |
CNS |
RuleOfFive |
STOCK6S-33288 |
0.805 |
−4.139 |
114 |
−1.486 |
47.3 |
−4.55 |
3 |
−0.356 |
3 |
68.467 |
−2 |
0 |
STOCK6S-43621 |
0.926 |
−4.292 |
95.3 |
−1.363 |
91.9 |
−4.77 |
2 |
−0.295 |
3 |
67.791 |
−2 |
0 |
STOCK6S-39892 |
0.843 |
−4.322 |
101 |
−1.215 |
102 |
−4.64 |
1 |
−0.346 |
3 |
67.717 |
−2 |
0 |
STOCK6S-37164 |
0.871 |
−4.351 |
106 |
−1.187 |
116 |
−4.6 |
1 |
−0.329 |
3 |
68.283 |
−2 |
0 |
STOCK6S-35740 |
0.874 |
−4.52 |
104 |
−1.567 |
43 |
−4.47 |
2 |
−0.315 |
3 |
68.189 |
−2 |
0 |
STOCK3S-95781 |
3.302 |
−3.946 |
17.5 |
−2.032 |
7.92 |
−4.35 |
3 |
0.262 |
2 |
68.509 |
−2 |
0 |
STOCK4S-19314 |
3.013 |
−3.884 |
19.2 |
−1.938 |
8.76 |
−3.97 |
3 |
0.067 |
2 |
67.541 |
−2 |
0 |
STOCK4S-13117 |
2.371 |
−2.874 |
21.5 |
−1.862 |
9.92 |
−4.31 |
3 |
−0.174 |
2 |
64.677 |
−2 |
0 |
STOCK4S-16029 |
2.953 |
−3.841 |
15.4 |
−1.983 |
6.89 |
−4.53 |
3 |
0.176 |
2 |
65.466 |
−2 |
0 |
STOCK4S-14901 |
0.448 |
−4.699 |
59.2 |
−1.766 |
23.3 |
−4.73 |
1 |
−0.382 |
3 |
61.296 |
−2 |
0 |
STOCK4S-09064 |
0.814 |
−1.707 |
7.51 |
−1.88 |
3.18 |
−5.56 |
2 |
−0.492 |
2 |
47.384 |
−2 |
0 |
STOCK1S-49116 |
1.84 |
−2.884 |
24 |
−1.365 |
17.5 |
−4.53 |
2 |
−0.279 |
2 |
62.413 |
−2 |
0 |
STOCK6S-33158 |
0.601 |
−4.19 |
109 |
−1.321 |
45.3 |
−4.56 |
2 |
−0.319 |
3 |
66.963 |
−2 |
0 |
STOCK3S-87292 |
3.819 |
−5.242 |
525 |
−0.229 |
2668 |
−3.11 |
0 |
0.498 |
3 |
100 |
2 |
0 |
STOCK4S-28410 |
2.417 |
−2.982 |
18.6 |
−1.998 |
8.5 |
−4.53 |
2 |
−0.129 |
2 |
63.838 |
−2 |
0 |
STOCK4S-00141 |
3 |
−3.891 |
20.1 |
−1.837 |
9.2 |
−4.21 |
3 |
0.149 |
2 |
67.815 |
−2 |
0 |
STOCK3S-98611 |
1.445 |
−3.995 |
250 |
−1.198 |
111 |
−3.79 |
5 |
−0.192 |
3 |
78.337 |
−2 |
0 |
STOCK1S-95046 |
1.511 |
−3.702 |
481 |
−0.316 |
856 |
−3.67 |
1 |
−0.194 |
3 |
83.797 |
2 |
0 |
STOCK4S-20723 |
0.687 |
−4.416 |
62.3 |
−1.761 |
24.6 |
−4.94 |
2 |
−0.298 |
3 |
63.083 |
−2 |
0 |
3.6 Molecular dynamics simulation
Molecular dynamics simulation (MDS) is more accurate means of obtaining greater insight into binding of ligand molecules to the protein under investigation. Similar workflow where molecular docking and pharmacophore based virtual screening is integrated with molecular dynamics simulations and Molecular Mechanics Poisson-Boltzmann Surface Area (MM-PBSA) free energy calculations has been employed by us in some previous reports.57 The MDS studies provide the means of exploring conformational sampling over a long period during which the flexibility in ligand atom and protein backbone and side chain atoms can be explored. Further, this conformational sampling can be exploited in deriving the energy of protein, ligand and protein–ligand complex under solvated conditions which can be useful in estimating the binding free energy and binding affinity. In present study, a 25 ns MDS was performed to evaluate the conformational stability and binding affinity of the top five hits (STOCK6S-33288, STOCK6S-43621, STOCK6S-39892, STOCK6S-37164, STOCK6S-35740) to LpxK and results were compared with the best designed ligand (IMMD30). During MDS, the each complex was subjected to initial minimization step and subsequent equilibration steps at constant pressure and temperature conditions which ensured relieving the steric clashes and optimized conditions of bonds. After 25 ns production phase MDS, the binding modes, formation of hydrogen bond, π–π interactions and van der Waals interactions between ligands and protein atoms were analyzed. The parameters; root mean square deviation (RMSD), root mean square fluctuation (RMSF), hydrogen bond formation and MM-PBSA binding free energies were studied on the resulting trajectories of each complexes.
The measurement of protein-RMSD and ligand-RMSD is fairly good estimate of conformational stability of protein and ligands. It involves measurement of the deviations in mean positions of protein atoms or ligand atoms. Lower deviations indicate better conformational stability. The RMSD analysis showed that the complexes of LpxK with STOCK6S-33288 (Fig. 15, black) and STOCK6S-43621 (Fig. 15, magenta) are quickly stabilized at around 5 ns and almost remain stable throughout the entire 25 ns simulation with average RMSD of 0.49 nm for both the complexes. For the complexes with hits STOCK6S-35740 and STOCK6S-39892 the RMSD gets stabilized at around 15 ns simulation time then after remains stable and the average RMSD values were 0.53 and 0.55 nm respectively. Interestingly, the RMSD for the designed compound IMMD30 and STOCK6S-37164 was found higher with average of 0.60 and 0.61 nm respectively. These results of RMSD in LpxK protein atoms suggest that the compounds STOCK6S-33288 and STOCK6S-43621 promote the conformational stability and probably due to more favorable interactions.
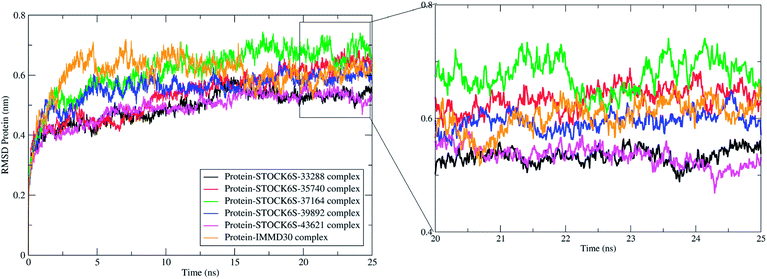 |
| Fig. 15 Root mean square deviation (RMSD) in LpxK protein atoms with bound five top hits and IMMD30. | |
The measurement of RMSD in ligand atoms is also important in judging the overall stability of the protein–ligand complexes. The hit molecules STOCK6S-33288, STOCK6S-39892, STOCK6S-37164, STOCK6S-35740 were found having small deviation of around 0.005 to 0.1 nm, where as the hit molecule STOCK8S-43621 and IMMD30 were having slightly higher RMSD in the range 0.1 to 0.2 (Fig. 16). These results suggest that the hit molecules produce favorable interactions at the binding site of LpxK.
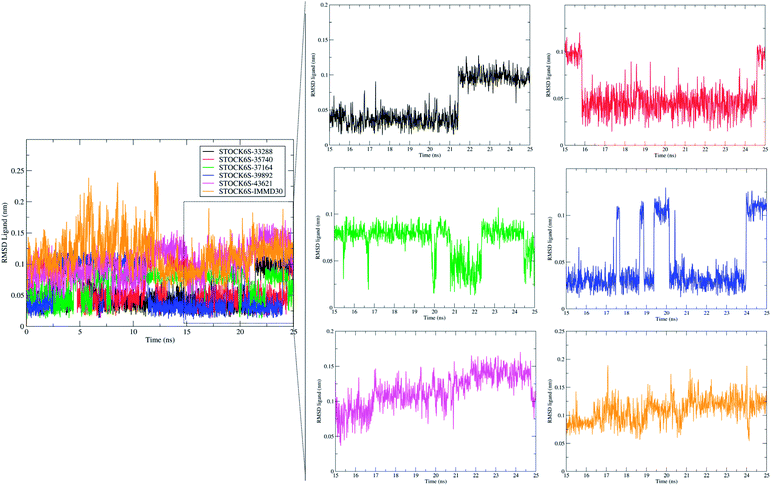 |
| Fig. 16 Root mean square deviation (RMSD) in atoms of hit molecules and IMMD30. | |
The elasticity of the protein residues from their center of mass is estimated through RMSF measurement. The RMSF for individual amino acids correlates with the trend observed in RMSD of complexes (Fig. 17). It is evident that there are fluctuations in the residues 96 to 116, 196 to 216 and 236 to 296. These residues are present in the binding site of LpxK and the fluctuations in these amino acid residues suggest the critical interactions between these residues and the atoms of hit compounds.
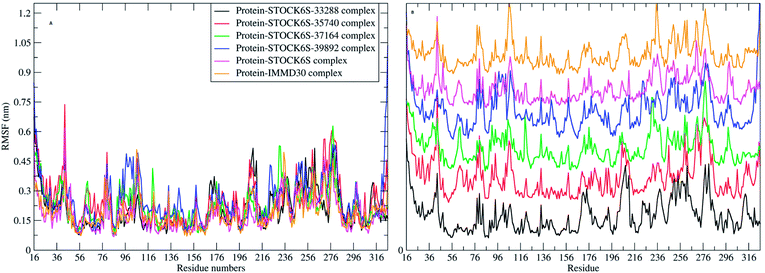 |
| Fig. 17 RMSF in residues (A) combined plot and (B) the plot isolated for each complex. | |
The non bonded interactions such as hydrogen bond interactions between the hit compounds and the residues at the binding site of the LpxK is another important parameter in the judging and estimating the binding affinity and activity of the ligand. More the number of hydrogen bonds formed during MDS and longer the life time of such hydrogen bonds more will be the binding affinity and consequent stability of the resulting protein–ligand complex. The hydrogen bond formation phenomenon was critically evaluated for all the complexes. Maximum number of hydrogen bonds formed during the progress of production phase MDS were 5, 3, 6 and 9 in case of STOCK6S-33288, STOCK6S-37164, STOCK6S-43621 and IMMD30 respectively (Fig. 18). In case of complex with STOCK6S-39892 and STOCK6S-35740 the maximum hydrogen bonds were 3 and 2 respectively.
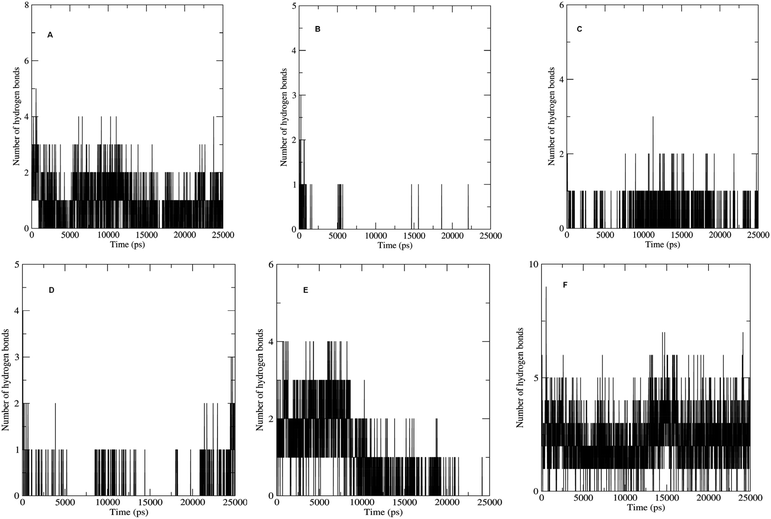 |
| Fig. 18 Number of hydrogen bonds formed. (A) STOCK6S-33288, (B) STOCK6S-35740, (C) STOCK6S-37164, (D) STOCK6S-39892, (E) STOCK6S-43621 and (F) IMMD30. | |
Which residues are important in hydrogen bond formation was investigated further with PyContact program.89 The residues HIS140, TYR75, ASP136, GLN139, ARG116, PRO165, TYR12, ASP135, TYR75, ARG73, ARG170 were found making the hydrogen bond interactions with the atoms of hit molecules and IMMD30 (Fig. 19). These results are supporting the docking studies where the same residues were predicted to be the key residues.
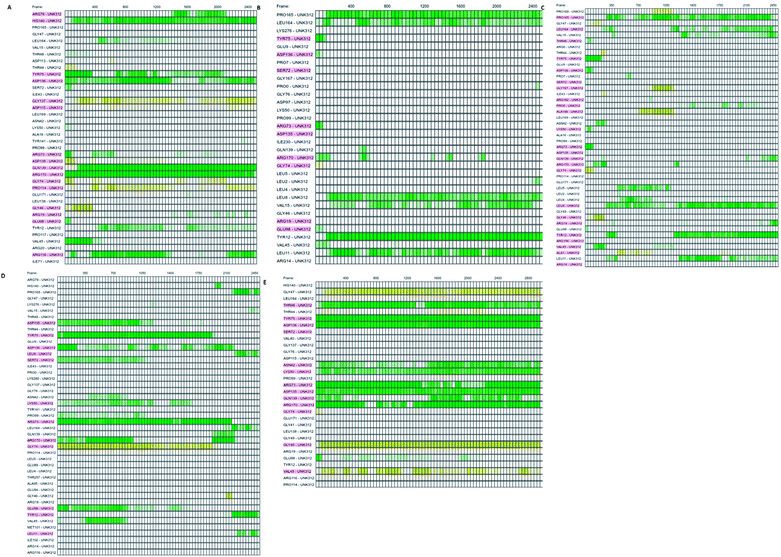 |
| Fig. 19 The residues involved in hydrogen bond formation. (A) STOCK6S-33288, (B) STOCK6S-35740, (C) STOCK6S-39892, (D) STOCK6S-43621 and (E) IMMD30. | |
Further investigation of the percentage of hydrogen bond formation reveled that in case of STOCK6S-33288 the residue PRO114 is the main residue making the key contact; while in all other compounds the residues TYR75, GLU94, GLY46, ASP135 are the key residues (Fig. 20).
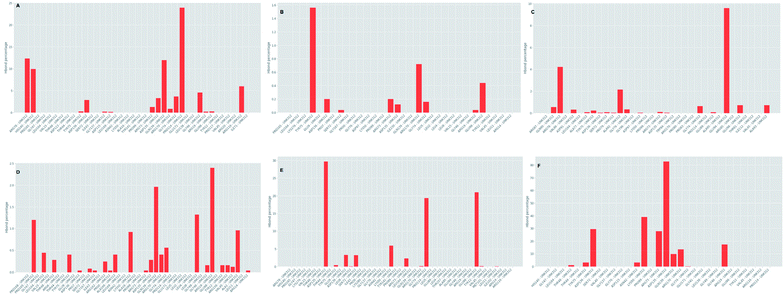 |
| Fig. 20 Per residue hydrogen bond percentage. | |
MM-PBSA calculations based on extracted MDS trajectories is another important measurement in analyzing the binding free energy and the binding affinity of ligands to proteins. The g_mmpbsa program was used to calculate the van der Waal energy, electrostatic energy, polar solvation energy, SASA energy and binding energy (Table 6). The results show that compound STOCK6S-35740 has lowest binding free energy (−61.9 kJ mol−1). The lowest binding free energy may be due to in part it has lowest polar solvation energy (20.6 kJ mol−1) as compared to other compounds. Polar solvation energy being greatest for compound IMMD30 (216.1 kJ mol−1), it has higher binding free energy (18.0 kJ mol−1). All other hit compounds have lower binding free energy and favorable affinity in terms of negative magnitude of binding free energy. The plot of binding energy versus the time steps in MDS (from the trajectories extracted at various time intervals) is shown in Fig. 21. The results of MM-PBSA calculation suggest that the all the hit compounds have favorable binding energy and in turn binding affinity and are predicted to be better inhibitors of LpxK.
Table 6 MM-PBSA calculations for hit molecules
Compound ID |
van der Waals energy (kJ mol−1) |
Electrostatic energy (kJ mol−1) |
Polar solvation energy (kJ mol−1) |
SASA energy (kJ mol−1) |
Binding energy (kJ mol−1) |
STOCK6S-33288 |
−109.858 (±9.752) |
−14.372 (±15.368) |
95.093 (±16.497) |
−11.825 (±0.881) |
−40.962 (±17.371) |
STOCK6S-35740 |
−75.878 (±11.490) |
2.104 (±5.147) |
20.367 (±23.140) |
−8.529 (±1.408) |
−61.936 (±20.454) |
STOCK6S-37164 |
−73.245 (±16.895) |
−20.606 (±17.471) |
69.414 (±35.946) |
−8.121 (±2.357) |
−32.558 (±13.881) |
STOCK6S-39892 |
−64.993 (±14.371) |
−8.080 (±13.821) |
32.983 (±26.411) |
−7.887 (±1.645) |
−47.977 (±22.513) |
STOCK6S-43621 |
−88.704 (±19.117) |
−16.240 (±9.461) |
88.795 (±40.120) |
−9.596 (±1.906) |
−25.746 (±23.271) |
IMMD30 |
−110.532 (±16.625) |
−73.436 (±13.572) |
216.123 (±29.385) |
−14.098 (±1.517) |
18.056 (±14.767) |
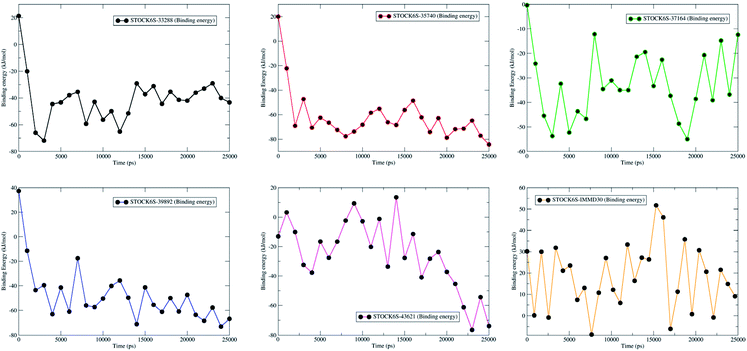 |
| Fig. 21 Contribution of binding free energy for each hit compounds and IMMD30 during MDS. | |
4. Conclusion
The comparative pathway analysis of metabolic pathway of P. aeruginosa helped in finding the unique metabolic pathway, lipid A biosynthesis pathway, which is responsible for cell wall synthesis in the P. aeruginosa. Careful analysis of the reported inhibitors of some enzymes of this pathway prompted us to implement a novel pharmacoinformatic approach on a key enzyme LpxK, which is not still explored to best of our knowledge. Tetraacyldisaccharide 4′-kinase (LpxK) is essential for bacterial survival and inhibitors of this enzyme can give potential antibacterial compounds effective against P. aeruginosa. In pursuit of finding such inhibitors, the variety of rational drug design approaches were carried out. The homology model of LpxK specific to P. aeruginosa was modeled and validated. From the currently known inhibitors of LpxA, LpxC, LpxD and LpxD, new inhibitors specific for LpxK were designed and their inhibitory potential was validated through docking studies. The pharmacophore model based the most active designed compounds was built and validated for the inhibitory potential. This pharmacophore was subsequently used to screen the InterBioScience database of natural and synthetic compounds, which gave the 102 top hits. Amongst these, top 5 hits and the most active designed compound was subjected to molecular dynamics studies. The results of MDS suggested that all the potential hits, STOCK6S-33288, STOCK6S-35740, STOCK6S-37164, STOCK6S-39892, STOCK6S-43621 have the better binding affinity towards LpxK and have the lowest binding free energy in MM-PBSA energy estimates. The virtual hits STOCK6S-33288 and STOCK6S-35740, which are the triazole derivatives could serve as a lead for further development of potential inhibitors of the P. aeruginosa specific LpxK. These virtual hit could be potentially beneficial in multidrug resistance, hospital-acquired bacteremia and ventilator-associated pneumonia due to underlying P. aeruginosa infections. However, this requires further experimental studies to support the molecular modeling results.
Conflicts of interest
There are no conflicts of interest to declare.
Acknowledgements
The authors thankful to the Mrs Fatima Rafiq Zakaria Chairman, Maulana Azad Educational Trust and Principal, Y. B. Chavan College of Pharmacy, Dr Rafiq Zakaria Campus, Aurangabad 431 001 (M.S.), India for providing the facility and support.
References
- S. Wagner, R. Sommer, S. Hinsberger, C. Lu, R. W. Hartmann, M. Empting and A. Titz, J. Med. Chem., 2016, 59, 5929–5969 CrossRef CAS.
- M. Bassetti, A. Vena, A. Croxatto, E. Righi and B. Guery, Drugs Context, 2018, 7, 1–18, DOI:10.7573/dic.212527.
- C. Kang, S. Kim, H. Kim, S. Park, Y. Choe, M. Oh, E. Kim and K. Choe, Clin. Infect. Dis., 2003, 37, 745–751 CrossRef.
- J. T. Thaden, L. P. Park, S. A. Maskarinec, F. Ruffin, V. G. Fowler and D. Van Duin, Antimicrob. Agents Chemother., 2017, 61, e02671, DOI:10.1128/AAC.02671-16.
- L. Fernández-Barat, M. Ferrer, F. De Rosa, A. Gabarrús, M. Esperatti, S. Terraneo, M. Rinaudo, G. Li Bassi and A. Torres, J. Infect., 2017, 74, 142–152 CrossRef.
- D. J. Weber, W. A. Rutala, E. E. Sickbert-Bennett, G. P. Samsa, V. Brown and M. S. Niederman, Infect. Control Hosp. Epidemiol., 2007, 28, 825–831 CrossRef.
- L. Nguyen, J. Garcia, K. Gruenberg and C. MacDougall, Curr. Infect. Dis. Rep., 2018, 20, 23, DOI:10.1007/s11908-018-0629-6.
- K. Tomono, T. Sawai and S. Kohno, Ryoikibetsu Shokogun Shirizu, 1999, 23, 198–201 Search PubMed.
- S. M. Huszczynski, J. S. Lam and C. M. Khursigara, Pathogens, 2020, 9, 6, DOI:10.3390/pathogens9010006.
- K. Hoshino, O. Takeuchi, T. Kawai, H. Sanjo, T. Ogawa, Y. Takeda, K. Takeda and S. Akira, J. Immunol., 1999, 162, 3749–3752 CAS.
- S. Akira, S. Uematsu and O. Takeuchi, Cell, 2006, 124, 783–801 CrossRef CAS.
- C. A. Janeway Jr and R. Medzhitov, Annu. Rev. Immunol., 2002, 20, 197–216, DOI:10.1146/Annurev.Immunol.20.083001.084359.
- B. S. Park, D. H. Song, H. M. Kim, B. S. Choi, H. Lee and J. O. Lee, Nature, 2009, 458, 1191–1195 CrossRef CAS.
- A. Poltorak, X. He, I. Smirnova, M. Y. Liu, C. Van Huffel, X. Du, D. Birdwell, E. Alejos, M. Silva, C. Galanos, M. Freudenberg, P. Ricciardi-Castagnoli, B. Layton and B. Beutler, Science, 1998, 282, 2085–2088 CrossRef CAS.
- R. Gupta, R. Verma, D. Pradhan, A. K. Jain, A. Umamaheswari and C. S. Rai, PLoS One, 2019, 14, e0221446, DOI:10.1371/journal.pone.0221446.
- C. González-Bello, Adv. Ther., 2019, 2, 1800117, DOI:10.1002/adtp.201800117.
- K. J. Babinski, A. A. Ribeiro and C. R. H. Raetz, J. Biol. Chem., 2002, 277, 25937–25946 CrossRef CAS.
- M. S. Anderson, H. G. Bull, S. M. Galloway, T. M. Kelly, S. Mohan, K. Radika and C. R. H. Raetz, J. Biol. Chem., 1993, 268, 19858–19865 CAS.
- H. E. Young, J. Zhao, J.
R. Barker, Z. Guan, R. H. Valdivia and P. Zhou, mBio, 2016, 7, e00090, DOI:10.1128/mBio.00090-16.
- L. E. Metzger and C. R. H. Raetz, Biochemistry, 2010, 49, 6715–6726 CrossRef CAS.
- J. D. King, D. Kocíncová, E. L. Westman and J. S. Lam, Innate Immun., 2009, 15, 261–312 CrossRef CAS.
- W. Han, X. Ma, C. J. Balibar, C. M. Baxter Rath, B. Benton, A. Bermingham, F. Casey, B. Chie-Leon, M. K. Cho, A. O. Frank, A. Frommlet, C. M. Ho, P. S. Lee, M. Li, A. Lingel, S. Ma, H. Merritt, E. Ornelas, G. De Pascale, R. Prathapam, K. R. Prosen, D. Rasper, A. Ruzin, W. S. Sawyer, J. Shaul, X. Shen, S. Shia, M. Steffek, S. Subramanian, J. Vo, F. Wang, C. Wartchow and T. Uehara, J. Am. Chem. Soc., 2020, 142, 4445–4455, DOI:10.1021/jacs.9b13530.
- K. G. Kroeck, M. D. Sacco, E. W. Smith, X. Zhang, D. Shoun, A. Akhtar, S. E. Darch, F. Cohen, L. D. Andrews, J. E. Knox and Y. Chen, Sci. Rep., 2019, 9, 1–12 CrossRef CAS.
- T. E. Bohl, K. Shi, J. K. Lee and H. Aihara, Nat. Commun., 2018, 9, 1–13 CrossRef CAS.
- D. L. Richie, K. T. Takeoka, J. Bojkovic, L. E. Metzger, C. M. Rath, W. S. Sawyer, J. R. Wei and C. R. Dean, PLoS One, 2016, 11, 1–22 CrossRef.
- M. Lee, J. Zhao, S. H. Kwak, J. Cho, M. Lee, R. A. Gillespie, D. Y. Kwon, H. Lee, H. J. Park, Q. Wu, P. Zhou and J. Hong, ACS Infect. Dis., 2019, 5, 641–651 CrossRef CAS.
- P. Zhou and J. Zhao, Biochim. Biophys. Acta, Mol. Cell Biol. Lipids, 2017, 1862, 1424–1438 CrossRef CAS.
- J. Cho, M. Lee, C. S. Cochrane, C. G. Webster, B. A. Fenton, J. Zhao, J. Hong and P. Zhou, Proc. Natl. Acad. Sci. U. S. A., 2020, 117, 4109–4116 CrossRef CAS.
- I. Mochalkin, J. D. Knafels and S. Lightle, Protein Sci., 2008, 17, 450–457 CrossRef CAS.
- H. Kurasaki, K. Tsuda, M. Shinoyama, N. Takaya, Y. Yamaguchi, R. Kishii, K. Iwase, N. Ando, M. Nomura and Y. Kohno, ACS Med. Chem. Lett., 2016, 7, 623–628 CrossRef CAS.
- D. M. Krzyzanowska, A. Ossowicki, M. Rajewska, T. Maciag, M. Jablonska, M. Obuchowski, S. Heeb and S. Jafra, Front. Microbiol., 2016, 7, 1–18 Search PubMed.
- C. K. Stover, X. Q. Pham, A. L. Erwin, S. D. Mizoguchi, P. Warrener, M. J. Hickey, F. S. L. Brinkman, W. O. Hufnagle, D. J. Kowallk, M. Lagrou, R. L. Garber, L. Goltry, E. Tolentino, S. Westbrock-Wadman, Y. Yuan, L. L. Brody, S. N. Coulter, K. R. Folger, A. Kas, K. Larbig, R. Lim, K. Smith, D. Spencer, G. K. S. Wong, Z. Wu, I. T. Paulsen, J. Relzer, M. H. Saler, R. E. W. Hancock, S. Lory and M. V. Olson, Nature, 2000, 406, 959–964 CrossRef CAS.
- V. K. Vyas, R. D. Ukawala, M. Ghate and C. Chintha, Indian J. Pharm. Sci., 2012, 74, 1 CrossRef CAS.
- X.-Y. Meng, H.-X. Zhang, M. Mezei and M. Cui, Curr. Comput.-Aided Drug Des., 2011, 7, 146–157 CrossRef CAS.
- S. Rampogu, M. Son, A. Baek, C. Park, R. M. Rana, A. Zeb, S. Parameswaran and K. W. Lee, Comput. Biol. Chem., 2018, 74, 327–338 CrossRef CAS.
- K. J. Simmons, I. Chopra and C. W. G. Fishwick, Nat. Rev. Microbiol., 2010, 8, 501–510 CrossRef CAS.
- D. K. Yadav, R. Rai, N. Kumar, S. Singh, S. Misra, P. Sharma, P. Shaw, H. Pérez-Sánchez, R. L. Mancera and E. H. Choi, Sci. Rep., 2016, 6, 38128 CrossRef CAS.
- S. Khedkar, A. Malde, E. Coutinho and S. Srivastava, Med. Chem., 2007, 3, 187–197 CrossRef CAS.
- E. Lionta, G. Spyrou, D. Vassilatis and Z. Cournia, Curr. Top. Med. Chem., 2014, 14, 1923–1938 CrossRef CAS.
- F. Cheng, W. Li, G. Liu and Y. Tang, Curr. Top. Med. Chem., 2013, 13, 1273–1289 CrossRef CAS.
- D. K. Yadav, S. Kumar, S. Saloni, H. Singh, M. Kim, P. Sharma, S. Misra and F. Khan, Drug Des., Dev. Ther., 2017, 11, 1859–1870 CrossRef CAS.
- R. Caspi, T. Altman, J. M. Dale, K. Dreher, C. A. Fulcher, F. Gilham, P. Kaipa, A. S. Karthikeyan, A. Kothari, M. Krummenacker, M. Latendresse, L. A. Mueller, S. Paley, L. Popescu, A. Pujar, A. G. Shearer, P. Zhang and P. D. Karp, Nucleic Acids Res., 2010, 38, D473–D479 CrossRef CAS.
- R. Caspi, H. Foerster, C. A. Fulcher, P. Kaipa, M. Krummenacker, M. Latendresse, S. Paley, S. Y. Rhee, A. G. Shearer, C. Tissier, T. C. Walk, P. Zhang and P. D. Karp, Nucleic Acids Res., 2007, 36, D623–D631 CrossRef.
- R. Caspi, R. Billington, C. A. Fulcher, I. M. Keseler, A. Kothari, M. Krummenacker, M. Latendresse, P. E. Midford, Q. Ong, W. K. Ong, S. Paley, P. Subhraveti and P. D. Karp, Nucleic Acids Res., 2018, 46, D633–D639 CrossRef CAS.
- R. Zhang, Nucleic Acids Res., 2004, 32, 271D–272D CrossRef.
- S. Pundir, M. J. Martin and C. O'Donovan, Protein Bioinformatics, Springer, 2017 Search PubMed.
- D. Cappel, M. L. Hall, E. B. Lenselink, T. Beuming, J. Qi, J. Bradner and W. Sherman, J. Chem. Inf. Model., 2016, 56, 2388–2400 CrossRef CAS.
- R. Abel, L. Wang, E. D. Harder, B. J. Berne and R. A. Friesner, Acc. Chem. Res., 2017, 50, 1625–1632 CrossRef CAS.
- R. P. Emptage, K. D. Daughtry, C. W. Pemble and C. R. H. Raetz, Proc. Natl. Acad. Sci. U. S. A., 2012, 109, 12956–12961 CrossRef CAS.
- T. Halgren, Chem. Biol. Drug Des., 2007, 69, 146–148 CrossRef CAS.
- L. Lima and E. Barreiro, Curr. Med. Chem., 2005, 12, 23–49 CrossRef CAS.
- J. G. Topliss, J. Med. Chem., 1972, 15, 1006–1011, DOI:10.1021/jm00280a002.
- T. A. Halgren, R. B. Murphy, R. A. Friesner, H. S. Beard, L. L. Frye, W. T. Pollard and J. L. Banks, J. Med. Chem., 2004, 47, 1750–1759 CrossRef CAS.
- G. Madhavi Sastry, M. Adzhigirey, T. Day, R. Annabhimoju and W. Sherman, J. Comput.-Aided Mol. Des., 2013, 27, 221–234 CrossRef CAS.
- K. Roos, C. Wu, W. Damm, M. Reboul, J. M. Stevenson, C. Lu, M. K. Dahlgren, S. Mondal, W. Chen, L. Wang, R. Abel, R. A. Friesner and E. D. Harder, J. Chem. Theory Comput., 2019, 15, 1863–1874 CrossRef CAS.
- R. A. Friesner, R. B. Murphy, M. P. Repasky, L. L. Frye, J. R. Greenwood, T. A. Halgren, P. C. Sanschagrin and D. T. Mainz, J. Med. Chem., 2006, 49, 6177–6196 CrossRef CAS.
- M. G. Damale, R. B. Patil, S. A. Ansari, H. M. Alkahtani, A. A. Almehizia, D. B. Shinde, R. Arote and J. Sangshetti, RSC Adv., 2019, 9, 26176–26208 RSC.
- B. Vyas, O. Silakari, M. Singh Bahia and B. Singh, SAR QSAR Environ. Res., 2013, 24, 733–752 CrossRef CAS.
- K. Rohini, P. Agarwal, B. Preethi, V. Shanthi and K. Ramanathan, Appl. Biochem. Biotechnol., 2019, 187, 194–210 CrossRef CAS.
- S. L. Dixon, A. M. Smondyrev and S. N. Rao, Chem. Biol. Drug Des., 2006, 67, 370–372 CrossRef CAS.
- P. Ambure, J. Bhat, T. Puzyn and K. Roy, J. Biomol. Struct. Dyn., 2019, 37, 1282–1306 CrossRef CAS.
- S. Rajamanikandan, J. Jeyakanthan and P. Srinivasan, Appl. Biochem. Biotechnol., 2017, 181, 192–218 CrossRef CAS.
- J.-F. Truchon and C. I. Bayly, J. Chem. Inf. Model., 2007, 47, 488–508 CrossRef CAS.
- C. Empereur-mot, H. Guillemain, A. Latouche, J.-F. Zagury, V. Viallon and M. Montes, J. Cheminf., 2015, 7, 52, DOI:10.1186/s13321-015-0100-8.
- P. D. Lyne, M. L. Lamb and J. C. Saeh, J. Med. Chem., 2006, 49, 4805–4808 CrossRef CAS.
- A. R. Muralidharan, C. Selvaraj, S. K. Singh, C. A. Nelson Jesudasan, P. Geraldine and P. A. Thomas, Med. Chem. Res., 2014, 23, 2445–2455 CrossRef CAS.
- D. L. Mobley and K. A. Dill, Structure, 2009, 17, 489–498 CrossRef CAS.
- C. A. Lipinski, Drug Discovery Today: Technol., 2004, 1, 337–341 CrossRef CAS.
- D. Sengupta, D. Verma and P. K. Naik, J. Biosci., 2007, 32, 1307–1316 CrossRef CAS.
- S. Shukla, R. S. Srivastava, S. K. Shrivastava, A. Sodhi and P. Kumar, Appl. Biochem. Biotechnol., 2012, 167, 1430–1445 CrossRef CAS.
- J. Gelpi, A. Hospital, R. Goñi and M. Orozco, Adv. Appl. Bioinf. Chem., 2015, 8, 37–47 Search PubMed.
- H. J. C. Berendsen, D. van der Spoel and R. van Drunen, Comput. Phys. Commun., 1995, 91, 43–56 CrossRef CAS.
- J. Huang, S. Rauscher, G. Nawrocki, T. Ran, M. Feig, B. L. de Groot, H. Grubmüller and A. D. MacKerell, Nat. Methods, 2017, 14, 71–73 CrossRef CAS.
- W. Huang, Z. Lin and W. F. van Gunsteren, J. Chem. Theory Comput., 2011, 7, 1237–1243 CrossRef CAS.
- A. K. Malde, L. Zuo, M. Breeze, M. Stroet, D. Poger, P. C. Nair, C. Oostenbrink and A. E. Mark, J. Chem. Theory Comput., 2011, 7, 4026–4037 CrossRef CAS.
- B. Hess, C. Kutzner, D. van der Spoel and E. Lindahl, J. Chem. Theory Comput., 2008, 4, 435–447 CrossRef CAS.
- H. G. Petersen, J. Chem. Phys., 1995, 103, 3668–3679 CrossRef CAS.
- R. A. Laskowski, M. W. MacArthur, D. S. Moss and J. M. Thornton, J. Appl. Crystallogr., 1993, 26, 283–291 CrossRef CAS.
- M. Wiederstein and M. J. Sippl, Nucleic Acids Res., 2007, 35, W407–W410 CrossRef.
- M. U. Johansson, V. Zoete, O. Michielin and N. Guex, BMC Bioinf., 2012, 13, 173 CrossRef.
- Z. Cournia, B. Allen and W. Sherman, J. Chem. Inf. Model., 2017, 57, 2911–2937 CrossRef CAS.
- D. Mondal, J. Florian and A. Warshel, J. Phys. Chem. B, 2019, 123, 8910–8915 CrossRef CAS.
- N. Singh and W. Li, Int. J. Mol. Sci., 2020, 21, 4765 CrossRef.
- N. Homeyer, F. Stoll, A. Hillisch and H. Gohlke, J. Chem. Theory Comput., 2014, 10, 3331–3344 CrossRef CAS.
- L. Wang, Y. Wu, Y. Deng, B. Kim, L. Pierce, G. Krilov, D. Lupyan, S. Robinson, M. K. Dahlgren, J. Greenwood, D. L. Romero, C. Masse, J. L. Knight, T. Steinbrecher, T. Beuming, W. Damm, E. Harder, W. Sherman, M. Brewer, R. Wester, M. Murcko, L. Frye, R. Farid, T. Lin, D. L. Mobley, W. L. Jorgensen, B. J. Berne, R. A. Friesner and R. Abel, J. Am. Chem. Soc., 2015, 137, 2695–2703 CrossRef CAS.
- J. Wereszczynski and J. A. McCammon, Q. Rev. Biophys., 2012, 45, 1–25 CrossRef CAS.
- D. L. Mobley and M. K. Gilson, Annu. Rev. Biophys., 2017, 46, 531–558 CrossRef CAS.
- J. Michel and J. W. Essex, J. Comput.-Aided Mol. Des., 2010, 24, 639–658 CrossRef CAS.
- M. Scheurer, P. Rodenkirch, M. Siggel, R. C. Bernardi, K. Schulten, E. Tajkhorshid and T. Rudack, Biophys. J., 2018, 114, 577–583 CrossRef CAS.
|
This journal is © The Royal Society of Chemistry 2020 |
Click here to see how this site uses Cookies. View our privacy policy here.