DOI:
10.1039/D0EE01882A
(Minireview)
Energy Environ. Sci., 2021,
14, 320-352
Porous crystalline frameworks for thermocatalytic CO2 reduction: an emerging paradigm†
Received
17th December 2019
, Accepted 4th September 2020
First published on 4th September 2020
Abstract
Heterogeneous catalysts for CO2 reduction derived from porous, crystalline frameworks have emerged as efficient systems with comparable activity and superior selectivity to their inorganic counterparts. The spatial arrangement of active sites in such catalytically active frameworks is critical to their performance in CO2 reduction. This review presents a comprehensive and critical analysis of (thermal) CO2 reduction over catalysts derived from porous, crystalline frameworks, whose structural and chemical diversity offers unprecedented opportunities to regulate reactivity. Thermodyamic considerations and the impact of process parameters on reaction intermediates, governing mechanisms for CO2 reduction and catalyst stability are discussed. Strategies for leveraging the flexibility of porous, crystalline frameworks to improve their stability and promote CO2 reduction are presented which include: use as sacrificial precursors to an active phase; integration within composites; and as hosts for nanoparticle encapsulation. Finally, future challenges and research prospects are highlighted.
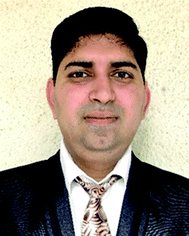
Sunil Mehla
| Sunil Mehla received his MSc in Chemistry from the Indian Institute of Technology Kanpur (2009) and MTech in Catalysis Technology, Chemical Engineering from the Indian Institute of Technology Madras (2012). He worked at HP Green R&D Centre of Hindustan Petroleum Corporation Limited for roughly 6 years (2018), researching and developing catalysts for isomerisation, cracking and diesel desulfurisation. He is currently pursuing his PhD at the Royal Melbourne Institute of Technology, Melbourne, under the supervision of Prof. Suresh Bhargava in the field of ordered noble metal nanoarrays for plasmonic sensing. |
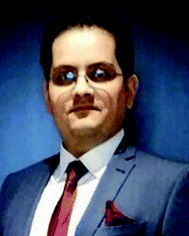
Ahmad E. Kandjani
| Dr Ahmad Kandjani received his PhD in Applied Chemistry from RMIT in 2014. Since then, he has worked as a Research Fellow with the Nanotechnology and Sensing group. In 2017, he was awarded the prestigious Vice-Chancellor's Fellowship at RMIT University. Dr Kandjani has a strong track record with over 100 publications (h-index 23), including 77 journal articles, 1 book chapter and 2 patents (1 PCT patent). The impact of his research has been highlighted on the front cover of nine prestigious journals 9 times. His research interests include novel semiconductor materials, long ranged colloidal crystal, nano-patterning, and optoelectronic devices and sensors. |
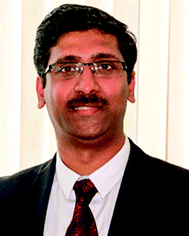
Ravichandar Babarao
| Dr Ravichandar Babarao is a Senior Lecturer at RMIT University and a visiting scientist at CSIRO. He received his PhD from National University of Singapore with the Best PhD Thesis Award in 2010 and the Prosper.net Scopus Young Scientist Award in 2015. He has also received the Alexander von Humboldt visiting scientist fellowship, 2016 RACI Rennie Memorial Medal, Victoria Fellowship in Physical Science and the 2019 Peter Schwerdtfeger Award. With >60 publications, his research interests are the chemistry of novel nanoporous materials for energy, environmental and biomedical applications including storage, separation and drug delivery. |
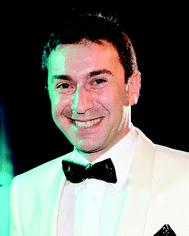
Adam F. Lee
| Professor Adam Lee is a Professor of Sustainable Chemistry at RMIT University and previously held Chair appointments at Cardiff, Warwick, Monash, and Aston universities, and a prestigious EPSRC Leadership Fellowship. His research addresses the rational design of nanoengineered materials for clean catalytic technologies, and the development of in situ/operando methods providing molecular insight into surface reactions. He has authored >260 articles (h-index 65, 14 722 cites), received the 2011 McBain Medal of the Royal Society of Chemistry and SCI, and 2012 Beilby Medal and Prize of the Royal Society of Chemistry, IOM3, and SCI, and is Editor-in-Chief of Materials Today Chemistry. |
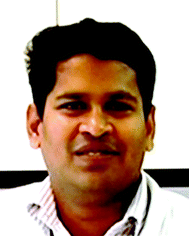
Selvakannan Periasamy
| Dr Selvakannan Periasamy has been working as a Research Fellow at RMIT University, since 2009. His major fields of interest are CO2 utilisation, methane activation, endothermic fuels for high speed flight vehicles, Surface Enhanced Raman Scattering and additive manufacturing in catalysis. He has 65 publications in international peer reviewed journals and a h-index of 33. Before joining RMIT, he worked as a Research Fellow at University of Paris-Sud, France (2007–2009) and as a Research Scientist at the Innovation Centre, Tata Chemicals Ltd, India (2005–2007). He received his PhD (2005) from the National Chemical Laboratory (University of Pune), India. He graduated from the American College, India with master's and bachelor's degrees in Chemistry (1996–2001). |
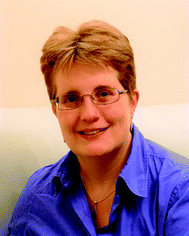
Karen Wilson
| Professor Karen Wilson is Professor of Catalysis at RMIT University and previously Chair of Catalysis and Research Director at the European Bioenergy Research Institute, Aston University, where she also held a prestigious Royal Society Industry Fellowship. Her research focuses on the development and scale-up of tunable, porous heterogeneous catalysts for green and sustainable chemistry and the utilisation of renewable resources in chemical processes. She has authored >260 journal papers (h-index 66, 14 819) and 20 book chapters, is Associate Editor of Sustainable Energy & Fuels, and serves on numerous Editorial Boards including Catalysis Today, Current Opinion in Green & Sustainable Chemistry and Energy & Environmental Materials. |
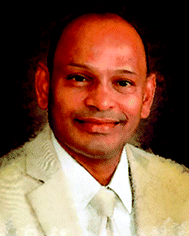
Seeram Ramakrishna
| Professor Seeram Ramakrishna, FREng is the Chair of Circular Economy Taskforce at the National University of Singapore (NUS). He is an editorial board member of Nature Scientific Reports. He is an elected Fellow of major professional societies and academies in Singapore, UK, India and USA. He is named among the World's Most Influential Minds and the Top 1% Highly Cited Researchers in Materials Science by Thomson Reuters and Clarivate Analytics. He has co-authored 1000 journal papers and 8 authored books received ∼110 000 citations and a h-index of 158. His research interests include innovations in sustainable materials and evaluation of circularity by life cycle assessment. |
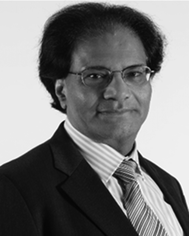
Suresh K. Bhargava
| Distinguished Professor Suresh Bhargava is a world-renowned interdisciplinary scientist at RMIT University who is recognised for delivering research excellence that underpins significant industrial applications. His journal articles that number over 520 have been cited over 16 210 times. Out of his seven patents, five have gone to industries or licensed to commercialisation. Quoted as being among the top 1% world scientists in the resource sector, Professor Bhargava provides consultancy and advisory services to many government and industrial bodies around the world including BHP Billiton, Alcoa World Alumina, Rio Tinto and Mobil Exxon. He has been recognised through many prestigious national and international awards. |
Broader context
The United Nations sustainable development goals have rallied environmental and energy scientists and engineers in pursuit of new technologies to initiate climate action and promote responsible consumption and production. Carbon dioxide (CO2) emissions are a major contributor in climate change, however CO2 capture and utilisation as a chemical feedstock could underpin a circular carbon economy. The transition from environmental pariah to abundant valuable feedstock requires new catalysts that can activate and selectively transform CO2 into chemicals and fuels, through atom and energy economical processes. This review explores the emergence of porous crystalline frameworks as heterogeneous catalysts for the thermochemical reduction of CO2 to small molecules. Elegant synthetic strategies enable structural diversity in porous crystalline frameworks, which in turn afford great control over the local chemical environment and reaction pathways available to CO2, although challenges remain in operational stability and product selectivity. Scale-up of such catalytic technologies, and their application in innovative reactors and integration with renewable hydrogen sources, will likely drive significant growth in commercial thermocatalytic CO2 reduction by 2030.
|
1.0 Introduction and background
The effects of rising anthropogenic CO2 emissions on climate change are well documented,1–3 and represent an imminent and existential threat to the biosphere requiring urgent and multipronged remediation strategies. The 21st Conference of the Parties (COP-21) to the United Nations Framework Convention on Climate Change, Paris 2015, tried to establish frameworks for national accountability and critical international comparisons to assist in meeting agreed targets, notably halving current CO2 emissions by 2050 and restricting the increase in global average air temperature to <2 °C.4,5 In addition, committees such as the Intergovernmental Panel on Climate Change, the Global Climate Change Initiative, the European Strategic Energy Technology Plan and the European Technology Platform for Zero Emission Fossil Fuel Power Plants have issued guidelines to direct progress in this field. Consequently, research into CO2 mitigation has rapidly expanded, and now bridges physical and social sciences with engineering.
CO2 removal from the atmosphere can be realised either using Carbon Capture and Sequestration (CCS), aimed at long-term geological storage or Carbon Capture and Utilisation (CCU) aimed at using CO2 as a feedstock for production of substances with higher value. CO2 Capture and Utilisation (CCU) has increasingly gained attention as a global warming remediation strategy due to potential applications in multiple sectors and the economic offset of investment costs by revenue from the sale of CO2 derived products. CCU is reportedly 20–40 times more efficient than CO2 Capture and Sequestration (CCS) over a 20 year timeframe.6 CCU can be applied to: (i) chemicals production; (ii) mineralisation; (iii) technological uses; (iv) enhanced biological use; and (v) fuels production.7 The first application is particularly appealing for the sustainable synthesis of commodity chemicals such as urea,8,9 salicylic acid,10 inorganic carbonates,11 formic acid,12 acetic acid,13,14 aromatic carboxylic acids,15 organic acyclic carbonates,16,17 cyclic carbonates,18,19 polycarbonates,20–22 carbamates,23–25 polyurethanes,26 acrylates,27 norbornane,28 lactones29,30 and pyrones,31,32 currently produced from fossil resources. Note that production of such (comparatively) low volume organic chemicals cannot significantly mitigate CO2 emissions,33,34 and hence focus is shifting towards the synthesis of high volume/high demand fuels and chemicals such as oxygenates (alcohols and ethers),35–37 alkanes,38 olefins39,40 and aromatics.41,42 A recent study43 identified 9 chemical products wherein a complete life cycle assessment suggested the use of CO2 as a feedstock could reduce the impact of short- and long-term global warming. These include CO from dry reforming of methane, CO from reverse water gas shift reaction, methanol, methane, formic acid by hydrogenation, ethanol, dimethyl ether, dimethyl carbonate, and polyols. In contrast, production of formic acid electrocatalytically, dimethoxymethane or Fischer–Tropsch (FT) products from CO2 was not beneficial for global warming, notably due to the high thermal energy demand for distillation of dilute formic acid solution (4.5 wt%) and associated cost of electricity and heat generation.43
Use of CO2 as a sustainable feedstock for chemicals and fuels is especially attractive as a process that can incorporate H2 from renewable energy. The integration of such ‘renewable H2’ permits the efficient synthesis of liquid transportation fuels with high combustion efficiencies and low pollutant emissions such as methanol, dimethyl ether, oxymethylene ethers and FT products.44 When obtained from CO2 reduction, CO and CH3OH are valuable building blocks to other fuels and chemicals by established FT, Methanol to Hydrocarbons (MTH) and Methanol to Olefins (MTO) technologies. CO2 conversion is energy intensive and often results in low product yields, hence initial research efforts focused on catalysts for CO and CH3OH synthesis as the most accessible products based on technology readiness level and conversion metrics.45 Subsequently, the direct reduction of CO2 to methane (termed methanation) has received significant attention, with high activity catalysts offering ∼100% selectivity at modest reaction temperatures (∼250 °C). This mirrors the emergence of abundant, low cost renewable H2, which offers an economically viable non-fossil route to hydrocarbons through the power-to-gas-concept; approximately 95 commercial power-to-gas projects are currently operational, generating ∼39 MW electricity worldwide.46 Nevertheless, CO2 methanation consumes large volumes of H2 and the generated methane has a low volumetric energy density hindering transport applications wherein on-board storage issues remain. Many countries also have abundant reserves of natural gas, and for these the emphasis is on the development of methane conversion (rather than production) technologies47 such as methane to ethane/ethylene, aromatics, methanol, or even methanesulfonic acid as a sustainable acidic solvent,48i.e. chemical rather than fuel production. It is important to recall that methane is also a potent greenhouse gas, with ∼5 to 10 times warming potential of CO2,49 emitted in large quantities from natural sources such as forests, bushlands, swamps and hence any anthropogenic emissions must be tightly controlled. In any of the above scenarios, access to low cost, concentrated CO2 and H2 is critical, and the need to capture the former, a subject of intensive research at different scales.50–52 Currently, the cost of renewable H2 obtained from water electrolysis ($2.91–10.92 per kg)53 still lags behind the cost of non-renewable H2 ($1.35–3.5 per kg),54 typically obtained from steam reforming of naphtha or natural gas. However, a recent report by the Hydrogen Council predicts the price of renewable H2 will significantly drop ($1–3 per kg) by 2030 due to economies of scales in its production and distribution, and advances in electrolysis technology and the falling costs of electrolysers.55 This review focuses on the emergence of porous crystalline framework catalysts for the thermocatalytic reduction of CO2; future technologies for such transformations will be underpinned by the availability of low cost and sustainably sourced H2.
Three principal conversion technologies exist for CO2 reduction, namely photocatalysis, electrocatalysis and thermocatalysis, although variants such as photothermal,56 electrothermal57 and photoelectrocatalysis58 have emerged. Each of these approaches has benefits and limitations, but they should not be viewed as competitors or alternatives since discoveries made in one often cross-fertilise advances in others. Thermocatalysis is suited for immediate adoption into existing CO2 emission-intensive industries where H2 is abundant and continuous product consumption guaranteed, as in petrochemical refining, and currently offers the highest reaction rates and product yields,59 and hence suitability for scale-up. In contrast, electrocatalytic and photocatalytic CO2 reduction are less energy intensive, typically operating at ambient temperature, and use water as a hydrogen source, but exhibit slower kinetics and usually produce a dilute aqueous/solvent product stream with high concomitant separation costs. Such technologies are therefore presently better suited to small scale, distributed CO2 reduction, and require significant research and development to progress to demonstration plants wherein new challenges are expected. The levelized cost of organic products by electrocatalysis is currently high due to electrolyser capital costs, with cost per unit electrode area in turn dependent on their current density and faradaic efficiency.60 Productivity is hampered by low gas solubility and poor diffusion (limited to a few tens of nm) through liquid electrolytes to the catalyst surface.61 Photocatalytic routes are currently hampered by inefficient light harvesting, charge separation and transfer to reactants, and slow multi-electron reduction kinetics resulting in low CO2 conversion and poor quantum efficiencies. Photocatalytic5,62–65 and electrocatalytic66,67 CO2 reduction over structured nanomaterials are the subject of comprehensive recent reviews, and hence this review focuses on the design, synthesis and application of solid catalysts derived from porous, crystalline frameworks for thermal CO2 conversion.
Porous, crystalline frameworks are a broad class of crystalline materials which possess well-defined, ordered and two-dimensional (2D) or three-dimensional (3D) framework structures comprising specific secondary building units (SBUs) made of cations (or clusters) and organic anions such as metal organic frameworks (MOFs),68 covalent organic frameworks (COFs)69 and zeolitic imidazolate frameworks (ZIFs).70 Their structures are usually visualised as nodes present at specific locations in 3D space, interconnected by linkers; a node is defined as a point in the framework where multiple linkers intersect. The diversity in composition, shape, size and nature of nodes, linkers and their connecting topology affords a plethora of possible crystalline frameworks. In 2017, the Cambridge Crystallographic Data Centre had catalogued 69
666 MOFs alone based on experimental crystal data.71
Research into crystalline frameworks has seen rapid growth, with numerous new applications of such materials as catalysts reported. Crystalline frameworks have received attention in photocatalysis56,66,72–78 electrocatalysis66,76,79,80 and catalysis using thermal energy.81,82 The rising interest in crystalline frameworks for thermocatalysis mirrors a deeper understanding of, and experimental improvements in, their stability at elevated temperatures and under reaction conditions. This is exemplified for MOFs, wherein the encapsulation of molecular catalysts and chemical fixation of CO2 by liquid phase reactions has been reviewed,82–87 along with some aspects of thermocatalytic CO2 reduction.81,88,89 However, the application of different crystalline framework classes, and their derivatives, as thermal catalysts for CO2 reduction has not been the subject of a comprehensive and critical analysis to date.
Thermocatalytic CO2 reduction is challenging in part due to the range of potential products, which may be thermodynamically favoured but with slow kinetics such as methanation (the Sabatier reaction) or methanol/dimethyl ether (DME) formation, or thermodynamically disfavoured as for partial reduction to CO. In general, a high CO2 activation barrier necessitates high reaction temperatures, whereas H2 activation requires high H2 pressures; both decrease energy efficiency. There are also materials design challenges: CO2 reduction requires molecular hydrogen to dissociate into H* adatoms proximate to an activated COx species, and hence the co-location of suitable active sites for each reactant. Porous, crystalline frameworks are an ideal platform to satisfy such spatial constraints due to their structural versatility. For example, metal nanoclusters encapsulated in a MOF can activate hydrogen, while linkers with appropriate functional groups can activate CO2. Fig. 1a and b illustrate how small (8 × 14 atoms) capped copper clusters (hydrogen activation sites) could be incorporated in the tetrahedral voids of PCN-426(Ni) (PCN, Porous Coordination Network), proximate to the linkers. The distance between Cu atoms and linkers could be tuned by placing larger (6 × 62 atoms) Cu clusters into the octahedral voids of PCN-426(Ni), see Fig. 1c and d, enabling control over the separation between H2 and CO2 activation sites. Porous crystalline frameworks also permit careful design of the local environment surrounding catalytically active sites, acting as macroligands able to tune their steric and electronic properties, and hence reactivity.90
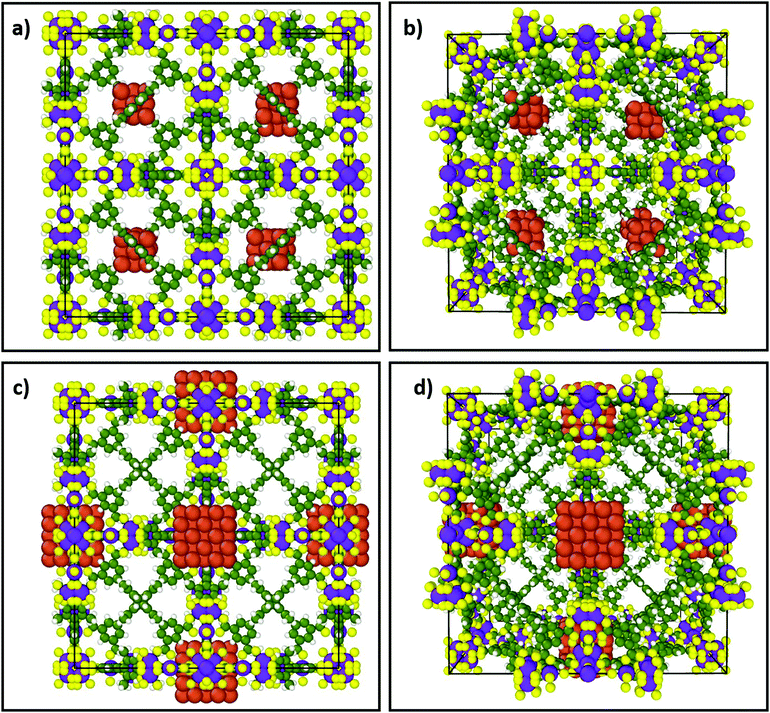 |
| Fig. 1 Two conceptual porous frameworks illustrating the encapsulation of different size (capped) copper nanoclusters within tetrahedral or octahedral cavities of PCN-426(Ni) with different Cu-linker separations. (a) Front view of 8 × 14 atom Cu cluster within tetrahedral voids of PCN-426(Ni). (b) Perspective view of structure in (a). (c) Front view of 6 × 62 atom Cu cluster within larger octahedral voids of PCN-426(Ni). (d) Perspective view of structure in (c). For illustrative purposes the capping agents are omitted. Orange: Cu; purple: Ni; yellow: O; green: C; white: H. These images were constructed using the open source molecular dynamics visualization software Ovito99 and structural data for PCN-426(Ni)100 and Cu nanoclusters;101 methodology is detailed in the ESI.† | |
Porous, crystalline frameworks are widely researched for selective gas sorption and molecular separations due to their high surface area, large pore volume, molecular sieving and tunable adsorbate–adsorbent interactions through surface functionalisation.91 Selective binding to framework metal atoms, differences in molecular packing efficiencies, framework flexibility and gate-opening mechanisms are effective strategies to facilitate molecular separation with enhanced selectivity.92 Many frameworks demonstrate a relatively high sorption capacity for CO2 and H2 arising from chemical interactions of these gases at nodes or linkers.93,94 For example, Demessence et al. reported a post-synthetically modified, pendant alkylamine containing MOF, 1-DMF, with an isosteric heat of adsorption for CO2 of 90 kJ mol−1.95 Lau et al. has shown that partial exchange of Zr ions with Ti ions in a UiO-66 framework (post-synthesis) doubles the CO2 sorption capacity, mainly due to decrease in pore size and higher adsorption enthalpy in the resulting heterometallic Zr–Ti UiO-66 MOF.96 Porous, crystalline frameworks offering enhanced H2 and CO2 sorption are highly desirable for thermocatalytic CO2 reduction; as a linear molecule with a large ionisation potential and small electron affinity, CO2 exhibits a high intrinsic activation barrier to reduction,97 however frameworks possessing electron-donating ligands can activate CO2 by charge transfer into its antibonding molecular orbitals.93 Framework basicity is therefore regarded a prerequisite structural feature of CO2 reduction catalysts. Using molecular simulations and comparison of a series of isoreticular MOFs (IRMOF1) differing in metal centre, organic linker, functional groups and framework topology, Babarao et al. have shown that CO2 adsorption in porous, crystalline frameworks depends on their free volume and accessible surface area, and the presence of functional groups and type of linkers.98 This review is organised as follows. Thermodynamic and process considerations of CO2 reduction are first discussed in Section 2, followed by a concise discussion of the key reaction intermediates and governing mechanisms in Section 3. Strategies to leverage the design flexibility of catalysts derived from porous crystalline frameworks for CO2 reduction are elaborated in Section 4. Critical consideration is given to the structural stability of crystalline frameworks under reaction conditions, often regarded as their greatest weakness in Section 5. Three broad approaches for the development of catalysts for thermal CO2 reduction are identified: (i) nanoparticle encapsulation in porous crystalline frameworks; (ii) the use of crystalline frameworks as sacrificial precursors; and (iii) the integration of crystalline frameworks into composite catalysts. These approaches are elaborated in Sections 6, 7 and 8 respectively. A concise analysis of porous crystalline framework-derived catalyst deactivation is presented in Section 9, before conclusions and a discussion of future outlooks.
2.0 Thermodynamic & process considerations
While the thermodynamics of a reaction establishes limits on the reactant conversion and product selectivity, process parameters can be adjusted to kinetically control catalyst activity and selectivity towards desired products. In this section the thermodynamics of major CO2 reduction reactions are analysed to identify ideal operating regimes with respect to process conditions. The choice of a specific porous, crystalline framework topology and composition for thermal catalysis should be guided by reaction parameters including temperature, pressure, space velocity, mechanical stress (on the catalyst), attrition resistance, and the physical/chemical properties of reactants, intermediates and products. After identifying a promising parameter set, a systematic search for a suitable crystalline framework is possible, based on its stability under the selected reaction environment. A reaction network for CO2 reduction and associated reaction enthalpies is shown in Scheme 1, based on data for the reverse water gas shift (RWGS) reaction102,103 and methanol synthesis5,6,104–108 wherein process conditions and catalyst performances have been compiled. RWGS being endothermic is favoured at high temperature, and hence CO2 conversion increases with an increase in temperature (Fig. 2). The RWGS has been studied under various conditions and promising results reported for diverse systems. Lu et al. obtained 100% selectivity to CO and 40% CO2 conversion over Ni/CeO2 at 700 °C, 1 bar pressure and a H2
:
CO ratio of 1,109 whereas Oshima et al. obtained the same conversion and selectivity at only 150 °C for a H2
:
CO ratio of 1 in the presence of an electric field.110 The former energy intensive conditions may render such a process uneconomical. Low conversion can be addressed by recycling the product stream (and unreacted CO2) back into the reactor, and hence catalysts which are highly selective to CO even at modest conversions, but under mild process conditions, are preferable. The energy efficiency of processes integrated with RWGS can be improved in a hybrid mode by coupling electrochemical CO2 reduction with downstream thermocatalytic process such as FT synthesis, although crystalline framework-derived catalysts are envisaged to offer promising low temperature RWGS activity due to the high dispersion of metal nanoparticles they facilitate. Selectivity control in RWGS is also attainable by varying the reactant space velocity, being favoured at high flow rates since CO formation involves fewer intermediates than for other products, with direct CO2 dissociation, formate and carboxylate mechanisms proposed. However, a high space velocity can also induce rapid heat removal and hence lower CO2 conversion, and such operation requires readily accessible active sites wherein rates are not constrained by reactant or product diffusion. Porous, crystalline frameworks usually satisfy these criteria due to their high surface area, pore volume and large pore apertures relative to the small molecules involved in RWGS (CO2, CO and H2O). Fixed bed, moving bed, fixed fluidised bed, fluidised bed and chemical looping reactors are all viable options for the RWGS reaction.
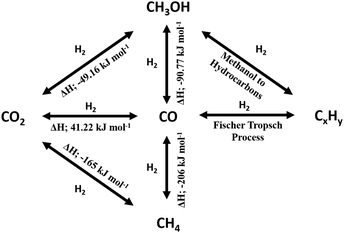 |
| Scheme 1 CO2 reduction reaction network and associated reaction enthalpies. | |
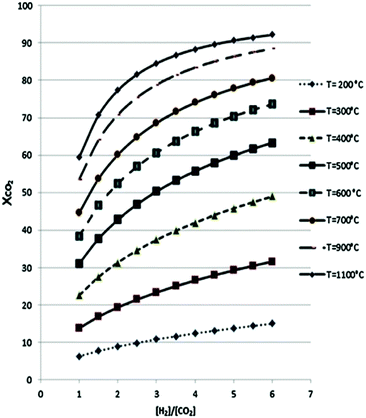 |
| Fig. 2 Reverse water–gas shift reaction of CO2 to CO as a function of H2 : CO2 ratio at different temperatures. Adapted from ref. 103 with permission from Elsevier, copyright 2017. | |
The enhanced dispersion and stabilisation of metal nanoparticles throughout framework-derived catalysts is also expected to promote activity for CO2 methanation. Indeed, for MIL-101, incorporation of a 20 wt% loading of 2.5 nm Ni nanoparticles resulted in 100% CO2 conversion with 100% selectivity to CH4 at 300 °C, 10 bar and H2
:
CO ratio of 3.111 Methanation is highly exothermic, and the thermodynamic conversion and selectivity both gradually decrease with increasing temperature, whereas higher pressures increase activity and selectivity (Fig. 3a and b). In exothermic reactions, heat management is critical for large-scale processes to limit catalyst deactivation by coking and/or sintering and to improve process economics through efficient use of waste heat.112 A study on the impact of space velocity and cooling rates for CO2 methanation using a transient mathematical model identified a negative impact of increasing reactant pressure due to faster catalyst coking induced by methane cracking.113 Methane yields improved with careful thermal management, with a complex, dynamic relationship observed between reactor performance, space velocity and heat removal rate. Heat exchanger type reactors are therefore best suited for large-scale methanation, although monolithic designs and certain scalable microreactors also enable efficient heat removal.114 At lab scale, fixed bed reactors have been favoured due to ease of operation. It is proposed that reactors and processing technologies developed for syngas (predominantly CO and H2, with some CO2) conversion could be adapted for CO2 methanation due to the similar feed streams, with only minor engineering barriers anticipated.59
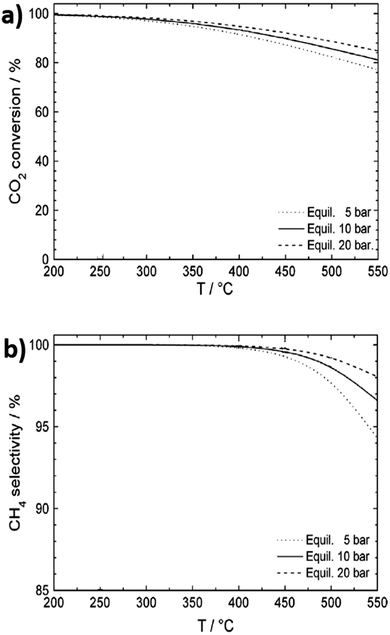 |
| Fig. 3 Thermodynamic equilibrium for CO2 conversion to methane. (a) CO2 conversion as a function of temperature and pressure. (b) CH4 selectivity as a function of temperature and pressure. Adapted from ref. 108 with permission from Elsevier, copyright 2013. | |
Methanol formation from CO2 is mildly exothermic reaction and favoured at low temperatures.104 Thermodynamic conversion limits for H2
:
CO2 ratios of 3 and 10 are shown in Fig. 4a and b, respectively (with corresponding methanol selectivities in Fig. 4c and d). For the former, at low (10 bar) pressure, CO2 conversion decreases from 20% at 150 °C to only 14% at 200 °C, before slowly increasing slowly thereafter to a maximum of 30% at 350 °C. Under the same conditions, methanol selectivity decreases continuously from 95% at 150 °C to almost zero at 350 °C. High methanol yields therefore require catalysts able to activate CO2 and H2 <200 °C due to the sharp fall in thermodynamic selectivity to methanol at higher temperature. Above 250 °C there is minimal difference in CO2 conversion (Fig. 4a) for 10 versus 30 bar, although significant improvement in methanol selectivity is obtained. However, relatively modest pressure increases do enhance CO2 conversion at 150 °C, and methanol yield can therefore be significantly augmented by a moderate increase in pressure for catalysts with high activity at low temperature. There are very few reports of CO2 reduction at such low temperatures. An increase in yield of CO or CH4 is typically obtained when CO2 reduction reaction takes place at temperatures higher than >250 °C.115 Since methanol synthesis from CO2 is mildly exothermic, heat management is expected to play an important role (albeit less so than for methanation) in governing catalyst selectivity and stability. As for many continuous flow reactions, the space velocity should be tuned to maximise contact time with the catalyst while promoting desorption and removal of products from the reactor bed.104
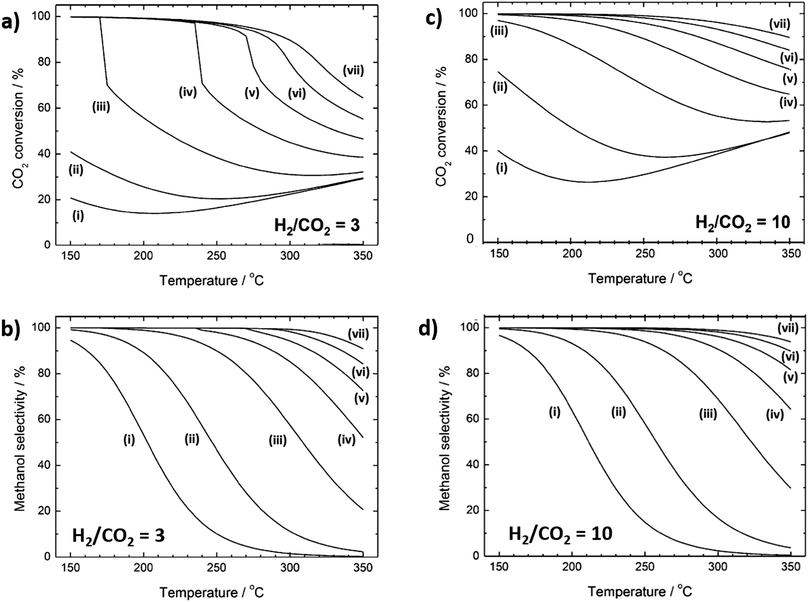 |
| Fig. 4 Thermodynamic equilibrium curves for CO2 hydrogenation to methanol. (a) CO2 conversion and (b) methanol selectivity for a H2 : CO2 ratio of 3. (c) CO2 conversion and (d) methanol selectivity for H2 : CO2 ratio of 10. Lines (i) to (vii) denote pressures of (i) 10, (ii) 30, (iii) 100, (iv) 200, (v) 300, (vi) 400 and (vii) 500 bar, respectively. Adapted from ref. 104 with permission from the American Chemical Society, copyright 2017. | |
Multi-tube fixed bed and fluidised bed reactors, microreactors and membrane reactors have all been utilised for methanol production from CO2 at the lab scale.116,117 Reactor and processing technology from syngas conversion to methanol can be adapted for CO2 reduction. At the industrial level, three reactor types have been employed for methanol synthesis: (i) the tubular boiling water reactor by Lurgi and Haldor-Topsoe which uses boiling water to remove heat from catalyst loaded tubes to achieve an isothermal reaction regime and low catalyst sintering rates; (ii) the quench reactor developed by ICI Synetix (now Johnson Matthey) using adiabatic catalyst beds and a cold feed gas for quenching which offers significant economic advantage; and (iii) series adiabatic reactors developed by Haldor-Topsoe and Kellogg with low pressure drops and high methanol productivity.118 Copper on metal oxide supports such as ZnO and ZrO2 are commonly employed for such engineering studies, however reaction parameter space should also be explored for new catalysts which exhibit high methanol selectivity and activity at low temperature, such as those derived from porous crystalline frameworks.
Recently, CO2 conversions reaching 34% with high selectively (90%) to hydrocarbons (olefins and gasoline) were reported for Fe catalysts, termed CO2-Fischer–Tropsch (CO2-FT).38 A tandem process for converting CO2 to hydrocarbons is feasible since the reaction conditions are essentially identical to those for CO-FT, and some CO-FT catalysts also exhibit significant RWGS activity for in situ conversion of CO2 to CO. Two challenges are identified for CO2-FT: CO2 can poison CO-FT catalysts; and water from the RWGS reaction can also deactivate CO-FT catalysts. Sustained production of hydrocarbons by CO2-FT is therefore problematic in regard of catalyst design and reaction engineering. CO-FT has been investigated in fixed bed, fluidised bed and three phase slurry reactors. The latter have been the reactor of choice for CO-FT since they offer unique advantages in respect of efficient heat removal, enhanced heat and mass transfer between catalyst and reactants, and continuous operation.119,120 For CO2-FT, the removal of water and recycling of undesired products (CO, CH4) back into the reactor must also be addressed. One solution is the use of independent modules, wherein a RWGS reactor feeds into a conventional CO-FT module, as this facilitates independent process optimisation for each reaction, which may prove intractable for a tandem process.59
3.0 Mechanistic insights
Mechanistic studies provide atomic scale insight into reactions, enabling key interdependencies between active sites, intermediates and products to be identified, rarely possible from a macroscopic analysis of reaction data, and thereby guiding catalyst design. Mechanistic studies of electrocatalytic CO2 reduction121–123 have received more attention than those for photocatalytic124,125 and thermocatalytic CO2 reduction.97,126,127 For thermocatalytic CO2 reduction, four distinct mechanisms are reported. Formate (HCOO*) and RWGS pathways are the most commonly proposed (Fig. 5), however other pathways such as the hydrocarboxyl (trans-COOH*) and revised formate mechanisms have also been advanced and experimentally verified. The former two pathways are supported by the experimental observation of formate and carboxylate (COO*) surface intermediates,128 although it is difficult to identify all reaction intermediates because of their transient lifetimes and limitations in the experimental techniques. The relative stabilisation of different reaction intermediates at catalyst surfaces dictates which reaction pathway, and hence product distribution, is favoured.
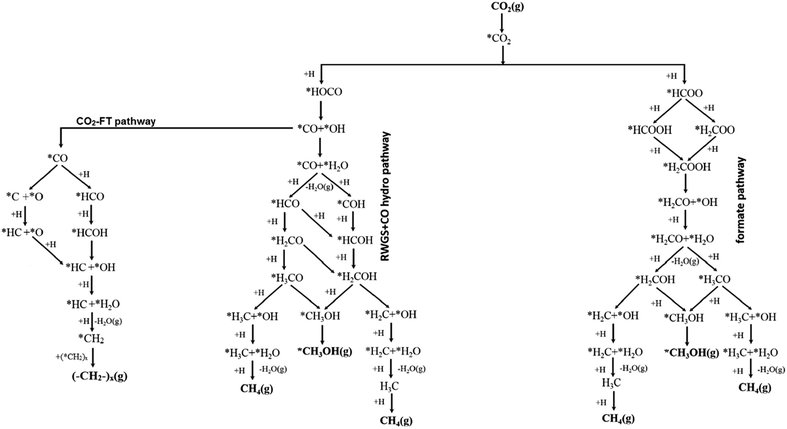 |
| Fig. 5 Reaction pathways in thermocatalytic CO2 reduction following formate, RWGS + CO and CO2-FT routes from a common carboxylate intermediate. Adapted with permission from ref. 140 with permission from the American Chemical Society, copyright 2017. | |
3.1 Formate mechanism
The formate mechanism involves an initial hydrogen transfer to CO2 to produce formate (HCOOH*) which can undergo sequential dissociation and reduction reactions to form dioxomethylene (H2COO*), formaldehyde (H2CO*), methoxy (CH3O*) and methanol.129 The detection of HCOO* species on Cu surfaces by XPS and TPD lends support to the formate mechanism.130 Other studies report that low-coordinate ionic Cu species (such as Cu+) stabilise formate (HCOO*) and dioxomethylene (H2COO*) intermediates promoting methanol formation,131,132 and may constitute the active sites in Cu/ZnO catalyst. In the formate mechanism, CO produced by the RWGS reaction accumulates as a by-product of the reaction. Hydrogenation of CO generates an unstable formyl intermediate (HCO*) which tends to dissociate to CO and H. Methanol synthesis via the formate pathway is widely reported for Cu surfaces (Cu(100)133), Cu clusters (Cu29),132 Cu nanoparticles on metal oxides (Cu/ZnO,132 Cu/SiO2,134 Cu/ZrO2134), alloy surfaces (Zn deposited Cu(111),135 Cu(100),136 Cu(110)136), metal carbides (Cu/β-Mo2C)137 and Pd based catalysts (Pd/β-Ga2O3,138 Pd4/In2O3139). For the formate route, hydrogenation of the formate or dioxomethylene intermediate and C–O bond cleavage are proposed as the rate determining steps.
3.2 Revised formate mechanism
Cu/ZnO is used for the industrial conversion of syngas to methanol and considered the benchmark catalyst for CO2 reduction to methanol. However, the origin of the high methanol selectivity for Cu/ZnO remains contentious. Recent studies over Cu(111) model catalysts suggest that HCOO* conversion to formic acid is more energetically favourable than to dioxomethylene,141 and a revised-formate (r-HCOO*) mechanism was consequently proposed wherein formic acid is the precursor to H2CO* and eventually methanol.97,141 Other studies support the revised-formate mechanism, and the high selectivity of Cu/ZnO and Cu/ZrO2 catalysts to methanol can be rationalised based on the oxophilicity of metal oxides and their ability to stabilise reactive intermediates.142–145 To date, the revised formate mechanism is proposed for Cu (ZnCu(211),146 ZnO/Cu(111),146 (Cu4/Al2O3)147) and Pd based systems ((Pd/CNT),148 PdCu(111)149).
3.3 RWGS + CO mechanism
The RWGS pathway involves CO2 reduction to a carboxylate species (COOH*) which dissociates into OH and CO surface species.140,150 Depending on the CO adsorption strength, it can either desorb or remain bound in an activated form (CO*). The catalyst and reaction conditions thereafter govern whether CO* is reduced to methane and/or methanol continuing on the RWGS pathway, or experiences C–O bond cleavage and subsequent reduction and C–C coupling following the CO2-FT pathway to higher hydrocarbons. In the RWGS + CO reaction pathway, formate is considered a spectator species in methanol formation with COOH* favoured as the key reactive intermediate.151 Catalysts which strongly adsorb CO will facilitate methanol and hydrocarbon formation, whereas those which bind CO more weakly will favour the RWGS reaction and CO desorption. The low selectivity of pure Cu catalysts for CO2 reduction to methanol is rationalised by the instability of formyl intermediate (HCO*) with respect to dissociation to CO.132 Dopants that stabilise formyl are therefore expected to increase selectivity to methanol, as predicted by density functional theory (DFT) calculations and Kinetic Monte Carlo (kMC) simulations for Ni/Cu(111) surface alloys.152 The RWGS + CO mechanism is proposed for Cu/CeOx,153 Cu/TiO2,154 Cu/ZrO2154 and Cu/ZnO/Al2O3155 at very high pressures (360 bar). DFT and kMC simulations suggest a more significant role for the RWGS + CO mechanism over Pt, Rh, Ni, Pt doped Cu(111) surfaces.152
3.4 The trans-COOH mechanism
Trace water, a by-product of the RWGS reaction, can influence activation barriers and the preferred reaction pathway,143 switching on the trans-COOH* mechanism, wherein DFT calculations suggest that trans-COOH* formation, rather than formate, is rate-limiting over Cu(111).156 Water lowers the energy barrier to trans-COOH* (and subsequent reactive intermediates) thereby improving selectivity to methanol. The trans-COOH mechanism proposes that hydrocarboxyl (COOH*) species are initially formed by reaction of adsorbed CO2 with H atoms (provided by H2O) to form COOH*. The COOH* is further hydrogenated to dihydroxycarbene (COHOH*), whose subsequent dissociation yields hydroxymethylidyne (COH*); hydrogenation of the latter then yields hydroxymethylene (HCOH) and hydroxymethyl (H2COH) intermediates, and methanol.118 CO formation from CO2 reduction may occur by either reduction of the COOH* intermediate and subsequent dissociation by the RWGS + CO pathway, or the direct dissociation of CO2 to CO and O* by a redox mechanism.143,157,158 The trans-COOH mechanism is suggested for metallic Cu,156 Au/Cu–ZnO–Al2O3159 and Ga3Ni5 surfaces.160
4.0 Leveraging flexibility and versatility in the design of catalysts from crystalline frameworks
Complete and predictable control over physicochemical properties across length scales is the Holy Grail of materials science. While substantial progress has been made in fabricating nanoparticles,161 thin films162 and 3D solids,163–165 generic multiscale materials design and synthesis remains beyond current modelling and fabrication capabilities. However, advances have been made in the development of single atom catalysts and nanoclusters stabilised on and within thin films,166 metal oxides167,168 and porous crystalline frameworks.169,170 Reticular chemistry, defined as the linking of molecular building blocks into predetermined structures by strong bonds, has brought material science a step closer to the design of multiscale functional materials. Our contributions in this field span nanoporous films and 3D porous architectures for adsorption,171,172 separation,173–175 sensing,176,177 reusable sensors,178,179 antibacterial films180–182 and catalysis.165,178,183–188
Allendorf and Stavila draw an analogy between the hierarchical structure of porous crystalline frameworks composed of several sub-components, characterised by different length scales and energetics, and interprotein assemblies (Fig. 6).189 Indeed such frameworks can be visualised and understood at several hierarchical levels, ranging from metal ion–ligand interactions, to host–guest interactions and interpenetration. In porous crystalline frameworks, nodes, typically comprising multivalent metal ions or metal clusters, are connected by organic ligands (linkers) to form 3D networks. The treatment of such frameworks as structurally ordered solids composed of secondary buildings units (SBU), i.e. nodes and linkers, aids their visualisation and the exploration of novel node and linker combinations, facilitating the design of new materials using a mix-and-match approach. The structural diversity achievable by this approach is evidenced by the vast number of such frameworks known, and accelerating discovery of new structures. Such diversity is enabled by composition independent, and charge density governed, interactions of nodes with linkers, allowing the exchange of nodes and linkers with similar charge densities.190 As previously noted, these porous crystalline frameworks provide high surface areas and pore volumes, selective gas adsorption, molecular confinement and excellent dispersion of active sites.191,192 Considering CO2 reduction, catalysts derived from porous crystalline frameworks should possess three key features: (i) high CO2 and H2 sorption capacities; (ii) a high density of active sites for efficient CO2 and H2 activation ideally co-located to promote transport of reactive intermediates; and (iii) a low affinity for the desired product (whether CO, CH3OH, CH4 and/or H2O). The rational design and synthesis of functional crystalline frameworks has been extensively reviewed.86,190–198 Their physicochemical properties can be modulated to a large extent through judicious selection of appropriate linkers, nodes and resulting cavity volumes.
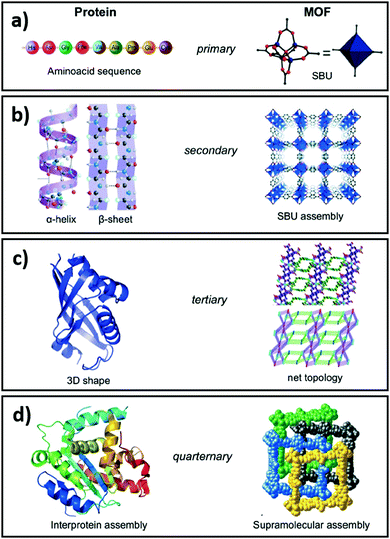 |
| Fig. 6 Comparison of the structural hierarchy of porous crystalline frameworks and interprotein assemblies. Reproduced from ref. 189 with permission from The Royal Society of Chemistry, copyright 2015. | |
Active sites can be incorporated in the form of coordinatively unsaturated metal sites, or the stabilisation of single atoms within the crystalline framework. Activities of individual catalytic sites in multifunctional catalysts derived from frameworks can also be modulated to tune overall activity and product selectivity. Finally, the shape, size and porosity of crystalline materials can be engineered to enhance molecular transport (overcoming potential molecular diffusion limitations199,200) and tune the hydrophobicity of active sites (to displace reaction products). These aspects of catalyst design are summarised below.
4.1 Linker and node modification in crystalline frameworks
Linkers in porous crystalline frameworks can be modified during synthesis or post-synthetically to incorporate metal or organic functions. Examples of metallated linkers (linkers containing metal moieties) used in framework synthesis201,202 and post-synthetic strategies203 are shown in Fig. 7a and b respectively. Linkers can also be modified to incorporate new organic functions204 (Fig. 7c).
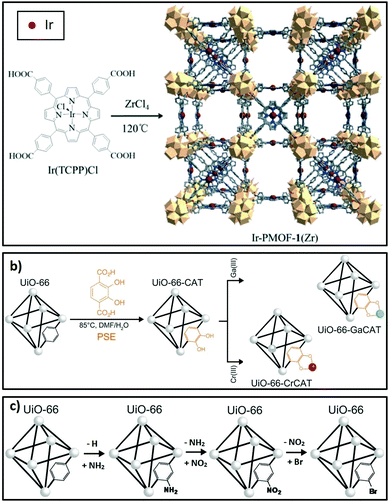 |
| Fig. 7 Strategies to modify linkers in porous crystalline frameworks. (a) Use of metallated moieties such as a metalloporphyrin tetracarboxylic ligand. (b) Post-synthetic metalation of linkers incorporated into the framework. (c) Post-synthetic functionalisation of linkers with organic moieties. (a) Reproduced from ref. 202 with permission from The Royal Society of Chemistry, copyright 2016; (b) reproduced from ref. 203 with permission from The Royal Society of Chemistry, copyright 2015; (c) adapted from ref. 203 and 204 with permission from The Royal Society of Chemistry, copyright 2015. | |
Covalent Organic Frameworks (COFs) are porous, organic two-dimensional or 3D solids composed of light elements (H, B, C, N, O) linked by strong covalent bonds. COFs have recently been synthesised with single active sites using porphyrin ligands containing Fe,205,206 Cu,207,208 Ni,207 Co201,202 and Mn206,209 centres. The high thermal stability of COFs, and their functionalisation by metallic moieties, has made them attractive potential catalysts. Metalloporphyrins can introduce Lewis acidity into frameworks through modulating the electron density.190 Phthalocyanines,205 bipyridine,210 phenylpyridine,211 N-heterocyclic carbine gold(I)212 and salen complexes213,214 are also reported as molecular linkers with which to incorporate metals into crystalline frameworks for catalytic applications. Nodes in porous crystalline frameworks can also be modified by either bottom-up approaches (using metal ions or clusters) or post-synthetic cation substitution or the attachment of single sites190,215,216 as shown in Fig. 8a, b, c and d, respectively.
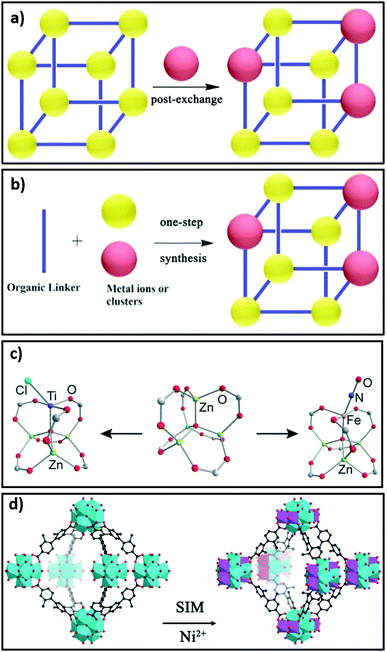 |
| Fig. 8 Strategies for node modification in crystalline frameworks. (a) Use of metal ions or clusters as nodes assembled using post-synthetic modification. (b) Bottom-up synthesis by cation substitution. (c) Post-synthetic attachment of single metal sites to nodes. (d) Node transformation in PCN-700(Zr) involving conversion of Zr6 clusters to Zr6Ni4 clusters by solution phase grafting referred to as solvent-assisted incorporation of metals (SIM). (a and b) Adapted from ref. 190 with permission from Wiley-VCH, copyright 2018; (c) adapted from ref. 215 with permission from the American Chemical Society, copyright 2013. (d) Adapted from ref. 216 with permission from Wiley-VCH, copyright 2015. | |
4.2 Cavity modification in crystalline frameworks
The chemical composition of the inner surface of framework cavities (pores) and cavity dimensions directly impact molecular adsorption and hence catalysis. The shape, size and chemical characteristics of cavities in crystalline frameworks can be varied by the modification of linkers or nodes, encapsulation of nanoclusters or novel method such as pore space partitioning. Pore space partitioning uses the shape of linkers to partition a large cavity into several smaller ones. Grafting of an appropriate molecular function to a partitioning linker enables repatterning of the parent framework (Fig. 9a and b). The pore space partitioning approach has been used to reduce the cavity of FJU-88 (12.0 Å × 9.4 Å) through introducing a triangular ligand, resulting in a new MOF FJU-90 with a cavity size of 5.4 Å × 5.1 Å offering improved separation of acetylene from CO2.217 Symmetry and size-matching pore partitioning agents such as 2,4,6-tri(4-pyridinyl)-1,3,5-triazine or a metal-complex cluster have also been introduced to the hexagonal channels of MIL-88/MOF-235 resulting in dramatically enhanced ammonia sorption.218 The size, shape and chemical characteristics of nanoporous cavities wherein catalysis predominantly occurs, can hence be readily tuned in crystalline frameworks.
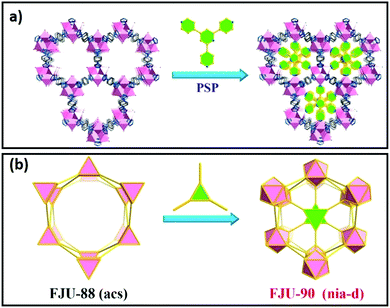 |
| Fig. 9 Pore space partitioning as a strategy for cavity modification. (a) Conversion of FJU-88 to FJU-90, seen along the crystallographic c-axis. (b) Polyhedral representation of FJU-88 conversion to FJU-90. Adapted with permission from ref. 217 with permission from the American Chemical Society, copyright 2019. | |
4.3 Single atom/site catalysts
Isolated metal sites at the corners, edges and facets of catalytic nanoparticles play an important role in governing their activity and selectivity. For example, a direct correlation between product selectivity in CO2 reduction and active site density is reported for Rh nanoparticles supported on TiO2.219 The densities of isolated (Rhiso) and total (RhNP) surface sites were estimated from diffuse reflectance infrared Fourier transform spectroscopy (DRIFTS) of adsorbed CO. Turnover frequencies (TOFs) for the RWGS reaction (producing CO from CO2) strongly correlated with Rhiso sites, whereas those for CH4 strongly correlated with RhNP sites. Reaction-induced restructuring altered the relative distribution of these sites resulting in changing product selectivity with time on stream.219 Engineering strategies such as varying the size (and size distribution) and morphology of nanoparticles/crystals have also been used to optimise the density and uniformity of isolated active sites. Homogeneous catalysis has drawn inspiration from biocatalysis to design molecular catalysts with single active sites; although this approach ensures extreme uniformity in catalysts it cannot circumvent shortcomings associated with product separation and continuous operation. The design of heterogeneous catalysts from porous crystalline framework containing single active sites (which may be individual atoms or ensembles thereof) has therefore been enthusiastically pursued.220,221 Single sites are defined as isolated, well-defined, spatially separated and, ideally, structurally identical species,220 and when present in heterogeneous catalysts promote shape selectivity and specific interactions.220 Single active sites can be incorporated into crystalline frameworks using coordinatively unsaturated nodes222 or metalation of linkers (as described in Section 4.1). Single atom catalysts (SACs) are a sub-class of single site catalysts in which individual site-isolated (usually metal) atoms are dispersed on a support.167,168,223 Single site (molecular) catalysts can be distinguished from SACs based on their coordination, since single sites have more coordination bonds with functional groups of the framework whereas single atoms are largely uncoordinated and dangling from a few anchoring bonds. SACs exhibit unique catalytic properties because of their low coordination, finding applications in hydrogenation, oxidation and organic synthesis. Crystalline frameworks have been used to create SACs by anchoring metal atoms at defect169 and coordinatively unsaturated sites,170 or pyrolysis of metal-functionalised framework to yield heteroatom-functionalised carbons with metal single atoms embedded.224
4.4 Diffusion limitations
Molecular diffusion of reactants and products is especially important for nanoporous framework-derived catalysts wherein mass-transport to/from active sites can be rate-limiting.81 For CO2 reduction, special attention must be given to the relative diffusion rates of H2 and CO2 reactants in the nanopores of crystalline framework derived catalysts. These rates may differ due to differences in molecular size and/or solubility in liquid-filled pores, resulting in exposure of active sites to a different local H2
:
CO2 molar ratio than in the bulk feed stream, impacting on reduction activity and selectivity. Experimental studies on CO versus H2 are well documented for the Fischer–Tropsch reaction. Post et al. identified that the limited mobility of reactant molecules, especially H2, in liquid-filled pores was a major limitation for synthesis gas conversion to fuels via the FT process over iron and cobalt catalysts in a fixed bed reactor.225 Catalyst activity may therefore be better optimised by considering the likely in-pore reactant ratios, rather than the bulk ratios typically considered for process control. Vervloet et al. attempted to identify favourable process conditions with respect to intra-particle diffusion limitations to maximise C5+ productivity for cobalt FT catalysts.226 They observed the H2
:
CO ratio increased towards the centre of catalyst particles, hindering chain growth probability due to intrinsically imbalanced H2 and CO diffusivities and consumption. Interestingly, C5+ space time yield could be increased 3–10 fold by lowering the bulk H2
:
CO ratio from 2 to 1 and increasing the reaction temperature by 30 K.226 Differential diffusion is expected to be even more significant for CO2 reduction due to its greater molecular size/mass relative to H2. Yao et al. investigated H2/CO/CO2 gas mixtures for the FT reaction over cobalt and iron catalysts and observed that CO2-rich reactant mixtures produced paraffin-rich products, whereas CO-rich reactant mixtures produced olefin-rich products.227
There is only limited literature on diffusion limitations for crystalline framework derived catalysts. Wang et al. compared the performance of Pt@ZIF-8 catalysts with different crystallite sizes for cylcooctene and hexene hydrogenation, observing that the product distribution depended on both pore and crystal sizes (Fig. 10).228 Larger cyclooctene molecules could not access framework pores due to their small openings, and hence hydrogenation was suppressed in all cases. In contrast, the n-hexane yield was inversely proportional to crystallite size, with the smallest (40 nm) particles offering the least diffusional resistance.
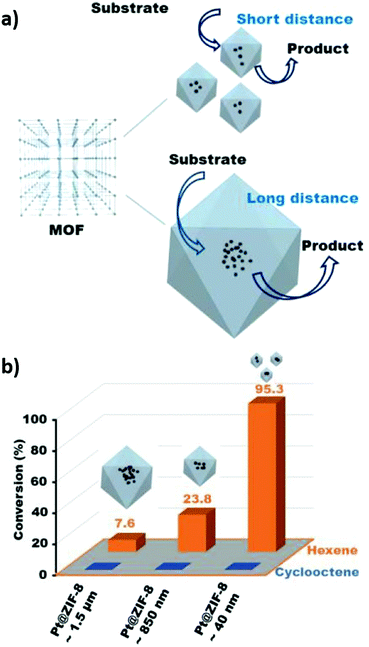 |
| Fig. 10 (a) Illustration of differences in path travelled by reactants in crystals of different sizes (b) Differences in product distribution obtained for cyclooctene and hexene hydrogenation with Pt@ZIF-8 catalysts of different particle size. Reproduced from ref. 228 with permission from Springer Nature, copyright 2017. | |
Compared to their porous inorganic analogues (e.g. mesoporous silicas and carbons), porous crystalline framework catalysts are expected to exhibit better mass transport properties arising from their:
(1) Higher surface areas and larger pore volumes. Crystalline frameworks can also be engineered to relieve diffusion constraints by introducing pore hierarchy (hollow crystals, three-dimensional ordered microcrystal (3DOM) and/or mesopore incorporation) and pore aperture engineering.229,230
(2) Availability of ordered sub-nanometer pore channels, which can facilitate molecular sieving to optimise the local concentration of reactant molecules at active sites. Framework flexibility and gate-opening mechanisms in crystalline frameworks as well as strategies like pore space partitioning also have potential for contribution to optimisation of the local environment.
(3) Tunable chemical functionality, which enables adsorbate–adsorbent interactions to be carefully optimised through pore surface, linker and node functionalisation and modification to retard or accelerate in-pore molecular transport.
4.5 Multifunctional catalysts based on crystalline frameworks
The interplay between different components of a multifunctional catalyst can also influence catalytic reactivity. For example, synergy between acid and metal functions is critical to optimise isomer production in bifunctional n-hexane hydroisomerisation231 and anisole hydrodeoxygenation.232 Similarly, the activity and density of hydrogen activation sites must be balanced against those of CO2 activation sites, with these being co-located to avoid diffusional barriers, for an efficient CO2 reduction catalyst. For example, high methanol selectivity (80%) and yields (297 mg gcat−1 h−1) were obtained over a Cu–ZnO–ZrO2 catalyst at 220 °C, 30 bar pressure and H2:CO2 3.1.233 ZnO–ZrO2 interfaces were identified as the active sites for CO2 adsorption and activation, whereas metallic Cu species were identified to be the active sites for hydrogen activation and dissociation. The density, activity and spatial proximity of active sites in the catalyst was controlled by systematic changes in catalyst composition and porosity (using colloidal crystal templating), which led to synergetic multifunctional catalysis.233 The design flexibility of porous crystalline framework presents new opportunities to regulate interdependent interactions between different active sites. For example, synergetic multifunctional catalysis between Cu, ZnO and ZrO2 components could be leveraged by dispersing ultrasmall Cu/ZnOx nanoparticles in UiO-66-bpy MOF, to obtain complete methanol selectivity and stable catalytic activity up to 100 hours, albeit at lower yields (136 mg gcat−1 h−1) to the above example, at 250 °C, 40 bar pressure and H2:CO2 of 3.234 High selectivity and catalyst stability are more desirable, since low CO2 conversion can be alleviated using product recycle.
4.6 Product release from catalysts based on crystalline frameworks
The release of products from CO2 reduction can be aided by the hydrophobisation of catalysts, or their construction as membranes. Methanol and water are major products of CO2 reduction (irrespective of the pathway followed), and their displacement from crystalline framework-derived catalysts can be assisted by the introduction of hydrophobic moieties adjacent to active sites; this can enhance catalyst stability and activity. Aguado et al. investigated a thin shell of Substituted Immidazolate Material (SIM) on alumina beads as a catalyst for Knoevenagel condensation, and measured intrinsic reaction rates free from external and internal diffusional limitations.235 A direct correlation was observed between the contact angle of water measured for a thin film of SIM on a flat surface and corresponding catalytic activity for Knoevenagel condensation of benzaldehyde and malononitrile wherein a seven-fold rate enhancement was reported. Recently, Li et al. demonstrated the use of a Na+-gated water conducting membrane, composed of a defect free film of zeolite NaA, grown on ceramic hollow-fibers, to efficiently separate water from the gaseous reactants and products of CO2 reduction; H2O:CO2, H2O:H2, H2O:CO and H2O:MeOH selectivities of 551, 190, 170 and 80 were respectively observed.236 CO2 reduction was subsequently investigated for a Cu/ZnO/Al2O3 catalyst loaded on the outer membrane surface, with the water permeate collected from the membrane core. Catalytic CO2 conversion and methanol yields were >2.6 times and >2.8 times higher respectively with than without the membrane at comparable selectivity (95% methanol in liquid products).236 Efficient molecular sieving between water and gas molecules >200 °C and >20 bar arose from the absence of defects and gating effect of Na+ located in 8-oxygen ring apertures of zeolite NaA, which regulated their effect size (Fig. 11). Such results for a commercial catalyst are unprecedented and highlight the importance of water removal for promoting CO2 reduction.
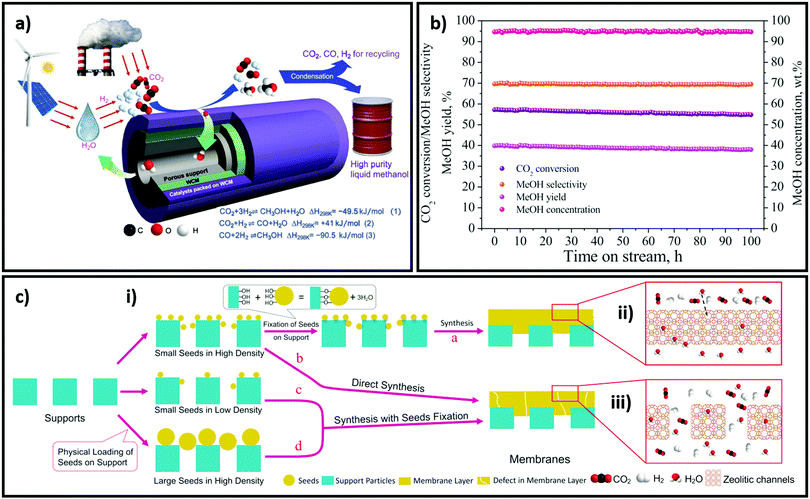 |
| Fig. 11 Application of a water-conducting membrane in CO2 reduction. (a) Schematic of water-conducting membrane dehydration reactor for high purity methanol production. (b) Catalyst performance for 100 h on-stream at 220 °C and 35 bar, H2 : CO2 ratio of 3 and GHSV of 4200 ml gcat−1 h−1 for a membrane length of 45 mm. (c) Different membrane preparation routes: (i) 50 to 200 nm seeds of NaA are attached to ceramic hollow-fibers by reaction with surface hydroxyls and annealing treatment to form a defect free membrane. Omitting the attachment step results in a defective membrane coating and poor selectivity. Decreasing the density of NaA seeds by a factor of 2 or 10, or using 300–400 nm and 400–700 nm NaA seeds also leads induces defects; (ii) a defect-free membrane effectively sieves water from gas molecules; (iii) defective membranes do not selectively transport water. Reproduced from ref. 236 with permission from Science, copyright 2020. | |
Incorporation of hydrophobic functions is also reported to stabilise crystalline frameworks under hydrous conditions during CO2 reduction. Omary et al. developed a superhydrophobic fluorine-based MOF which exhibited excellent stability in water.237 Methyl groups in ZIF-68, ZIF-69 and ZIF-70 also exhibit good stability in boiling water, methanol, benzene and even 8 M NaOH for up to 7 days, due to suppressed hydrolysis of [ZnN4] units associated with hydrophobic framework pores.238,239 Pyrazolate-based Ni8L6 MOF,240 BUT-12 and BUT-13241 likewise demonstrate good stability against water, acids and bases due to their hydrophobicity. Hydrophobisation can also be achieved by post-synthetic modification of crystalline frameworks using covalent,242 dative210 and click chemistry243 approaches.
5.0 Crystalline framework stability as a critical design parameter
The historical poor thermochemical stability of crystalline frameworks has previously limited their use in secondary processing and practical applications at industrial scale. For example, MOF-5 showed promise for several applications244,245 but could not be practically implemented due to gradual decomposition by moisture in air.246 However, due to the advent of new synthetic and modification strategies, and progress in theoretical understanding of bonding in crystalline frameworks,247 such limitations have been overcome. Consequently, crystalline frameworks now find application in areas such as the detection and removal of antibiotics (Nitrofurazone and Nitrofurantoin) and organic explosives (2,4,6-trinitrophenol and 4-nitrophenol) in water.241 The possibility of hydrothermal synthesis, in addition to solvothermal synthesis, has also improved the green credentials for crystalline framework production,197,248 and their resulting industrial scale-up.249 The stability of crystalline frameworks has been extensively reviewed,238,250,251 hence we focus here only on stability issues pertinent to thermocatalytic CO2 reduction. Current understanding suggests that the relatively labile nature of coordination bonds that underpin most crystalline framework is responsible for their poor thermochemical stability.250 In designing a stable crystalline framework, the first factor for consideration is the node–linker bond strength, which is governed by charge density matching of linkers and metal centres (nodes). Using Pearson's hard/soft, acid/base (HSAB) principle, Zhou et al. identified two types of stable crystalline frameworks: those based on hard carboxylate linkers coordinated to hard, high valent metal ions; and those based on soft azolate linkers coordinated to soft, low valent metal ions.238 MIL-53,252 MIL-100,253 MIL-101254 and Zr4+ based MOFs255 belong to the former hard base–hard acid type and are reportedly stable in water and even acidic conditions. However, crystalline frameworks of this type readily decomposed in basic media, as observed for PCN-222256 and MOF-545257 comprising Zr4+ and carboxylate linkers. Their poor stability in basic media was attributed to the competition of hard hydroxide anions with hard carboxylate linkers for coordination to metal cations. Carboxylate linkers have a relatively low affinity for protons due to their low pKa values and form strong coordination bonds with metal ions, leading to a good stability in acidic media.238 However, hydroxide anions have a stronger affinity for metal ions than carboxylate linkers, resulting in ligand exchange and framework degradation. Zeolite Immidazolate Frameworks (ZIF),239 PCN-601,258 triazole95 and pyrazolate259 based MOFs which belong to the latter soft acid–soft base group exhibit excellent stability in water and basic media, but readily decompose in acidic media. The high pKa values of azolate linkers make them particularly reactive with protons leading to degradation of metal–linker bonds. In addition to protons and hydroxide ions, other hard anions such as F−, CO32− and PO43− can also degrade crystalline frameworks,238 and their presence can be mitigated by synthesising frameworks based on soft metal ions and soft linkers. For example, PCN-602, comprising soft metal ions and linkers, exhibits excellent stability in 1 M KF, K3PO4 and Na2CO3 whereas PCN-224 (assembled from Zr6(OH)8 nodes and tetrakis(4-carboxyphenyl)porphyrin linkers) completely degraded under such conditions.260 Pearson's HSAB principle provides a useful rule of thumb for predicting the stability of crystalline frameworks.
Structural connectivity and rigidity also influence the stability of porous crystalline frameworks. High connectivity through multiple bonds can stabilise frameworks even if some bonds are weakened or degraded. Linker rigidity imparts stability to UiO MOFs (UiO-66, UiO-67 and UiO-68) constructed from a homologous series of linkers.257 Greater flexibility of the longer linkers in UiO-68 and UiO-67 reduced their stability compared to UiO-66.
For CO2 reduction to methanol, crystalline frameworks should be stable at temperatures up to 250 °C and in the presence of water vapour. Although crystalline frameworks are stable to water under ambient conditions, their stability under high partial pressures of water and methanol at reaction temperature has not been investigated. Heterogeneous catalysts containing metal functions typically require activation by reductive pretreatments at temperatures of 300–400 °C, and hence catalysts derived from crystalline frameworks should ideally by stable up to 400 °C under a reducing atmosphere. This is important for RWGS catalysts wherein frameworks must be stable towards both water vapour and CO (itself a reductant). Catalysts which favour formic261,262 or acetic acid263 formation are most likely to be stable when based on crystalline frameworks constructed from hard acids/hard bases.
6.0 Nanoparticle encapsulation in crystalline frameworks
Nanoparticle encapsulation in porous crystalline frameworks is an effective strategy for preparing multifunctional catalysts.264–266 Crystalline frameworks enable the encapsulation of extremely small nanoparticles which are stabilised by interactions with functional groups in linkers. Encapsulated nanoparticles may have higher catalytic activities than their naked counterparts, despite their confinement in framework cavities and coordination to linkers,267 attributed to strong metal support interactions and/or metal–ligand charge transfer. The surrounding framework can also induce shape and size selective catalysis for confined nanoparticles, wherein the geometric and electronic environment of reactants/products within cavities can be tuned by modifying the nodes and linkers,268 and where entropic considerations may become rate-controlling.269 Encapsulation of nanoparticles within crystalline frameworks also facilitates cooperative catalysis, wherein distinct active sites may participate in simultaneous (often simply termed bifunctional or one-pot) or sequential (termed tandem or cascade) catalytic reactions.270 This section describes the application of nanoparticles encapsulated within crystalline frameworks for CO2 reduction on the basis of the major product.
6.1 Methanol selective CO2 reduction catalysts
An early use of crystalline frameworks as catalysts can be traced back to 2008 when Müller et al. investigated Cu/ZnO supported on MOF-5 for CO2 reduction.271 Volatile precursors [CpCuL] (L = PMe3, CNtBu) and ZnEt2 were incorporated in the MOF matrix by solventless, gas phase adsorption and decomposed by hydrogenolysis or photoassisted thermolysis (Cu) and annealing (ZnO) to obtain Cu/CuO/MOF-5, ZnO/MOF-5 and Cu/ZnO/MOF-5 catalysts. The Cu/ZnO/MOF-5 (1.4% Cu, 40.1% ZnO) catalyst achieved a methanol yield 60% that of the industrial catalyst (60% Cu/30% ZnO) and productivity of 212 μmol gcat−1 h−1 despite the far lower Cu loading. This excellent performance was attributed to a high dispersion of Cu nanoparticles on the MOF support, and Cu/ZnO interfacial interaction. However, the catalyst degraded after few hours on-stream due to MOF-5 deactivation by water vapour. In 2016, Rungtaweevoranit et al. achieved an eight-fold enhancement in methanol yield (at 100% selectivity), compared to a commercial Cu/ZnO/Al2O3 catalyst, using 1% Cu/UiO-66 which comprised 18 nm copper nanoparticles individually encapsulated within UiO-66 crystallites (note these particles are larger than the framework cavities).267 Polyvinylpyrrolidone (PVP) protected copper nanoparticles were added to MOF precursors during the catalyst synthesis. Of the controls examined (Cu/MCF-26, Cu/ZrO2, Cu/Al2O3, Cu/MIL-101, Cu/ZIF-8, and Cu/ZnO/Al2O3), methanol formation was only observed for Cu/ZrO2, Cu/ZnO/Al2O3 and Cu/UiO-66, highlighting the importance of Cu–Zn and Cu–Zr interactions in methanol formation. The activity of encapsulated Cu nanoparticles was twice that of Cu nanoparticles impregnated post-synthesis of the MOF, and exhibited superior stability, highlighting the benefits of encapsulation. Cu/UiO-66 catalyst outperformed Cu/ZnO/Al2O3 at all temperatures, and was completely selective to CH3OH whereas the commercial catalyst formed CO >200 °C. XPS evidenced partial reduction of Zr for the impregnated Cu/UiO-66 catalyst, likened to the strong metal support interaction (SMSI) observed for metal nanoparticles on reducible oxides.272 An et al. also investigated CuO/ZnO nanoparticles on a UiO-bpy MOF, reporting 100% methanol selectivity and a space time yield of 2.59 gMeOH kgCu−1 h−1 for 100 hours at 250 °C and 40 bar.234 CuCl2 and ZnEt2 were used to introduce Cu and Zn (Fig. 12) to the MOF. Cu binding to bpy ligands was verified by UV-VIS-NIR and the observation of a metal–ligand charge-transfer (MLCT) band at 470–530 nm. This MCLT band disappeared after catalyst reduction at 250 °C and 40 bar H2, coincident with a copper reduction peak (Cu2+–Cu0) in temperature programmed reduction (TPR) studies, confirming a strong interaction between Cu nanoparticles and the MOF framework. XPS evidenced Cu0, Zn2+, Zn0, Zr4+ and Zr3+ species in the as-prepared catalyst. A related Cu/UiO-bpy catalyst was more active (6%) than CuZn/UiO-bpy catalyst (3%) but showed worse methanol selectivity (52%). Increasing the Zn
:
Cu ratio from 0.26 to 0.91 increased the methanol space time yield from 1.05 to 2.61 gMeOH kgCu−1 h−1, emphasising the importance of Cu–Zn interactions in methanol formation as reported for conventional bimetallic catalysts.5,104,105 The bpy ligands and Zr oxy nodes were also important for good catalyst activity, since a poorer performance was obtained for a related Zr-based MOFs with a different linker (UiO-67) and an Al-based MOF-253. Mechanistically, it was proposed that H2 was first activated on Cu sites and subsequently spills over to O-deficient ZnOx and Zr sites at which CO2 is activated (to form carbonate and bicarbonate) and then reduced to methanol (Fig. 13). Kobayashi et al. compared the performance of Cu/γ-Al2O3 (Al3+), Cu/ZIF-8 (Zn2+), Cu/MIL-100 (Cr3+), Cu/UiO-66 (Zr4+), Cu/UiO-66-COOH (Zr4+), Cu/UiO-66-NH2 (Zr4+) and Cu/Hf-UiO-66 (Hf4+) for CO2 reduction at 220 °C, 2 atm and a H2
:
CO2 ratio of 5 (Fig. 14a). They also proposed an interfacial charge-transfer between Cu atoms and the UiO-66 framework, correlating Zr/Hf binding energy shifts and methanol productivity (Fig. 14b).273 Methanol formation over Cu/UiO-66 was 70 times higher than for Cu/γ-Al2O3, with a further three-fold enhancement observed for hafnium analogues (Cu/Hf-UiO-66). Amine functionalisation of Cu/UiO-66 (Zr4+) led to marginal decrease in catalytic performance, however functionalisation with electron-withdrawing carboxylic acid groups increased methanol productivity three-fold, further evidencing that charge-transfer from Cu nanoparticles to the MOF framework was responsible for promoting CO2 reduction to methanol versus Cu/γ-Al2O3.
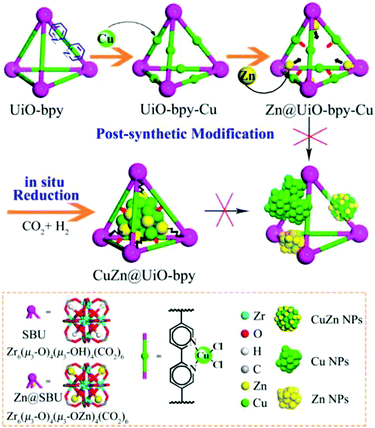 |
| Fig. 12 Synthesis of a CuZn@UiO-bpy catalyst for selective CO2 reduction to methanol. Adapted from ref. 234 with permission from the American Chemical Society, copyright 2017. | |
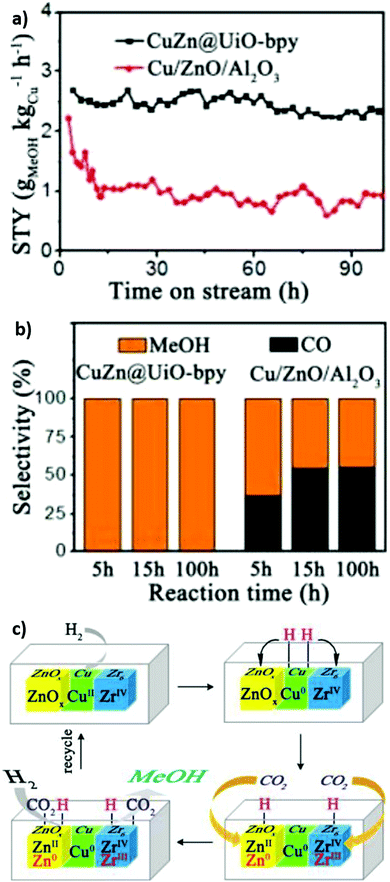 |
| Fig. 13 Cooperative catalysis using crystalline frameworks. (a) Methanol space-time yield versus reaction time. (b) Methanol selectivity versus time on-stream (c) Proposed mechanism for CO2 hydrogenation at Cu/Zn/Zr interfacial sites. Adapted from ref. 234 with permission from the American Chemical Society, copyright 2017. | |
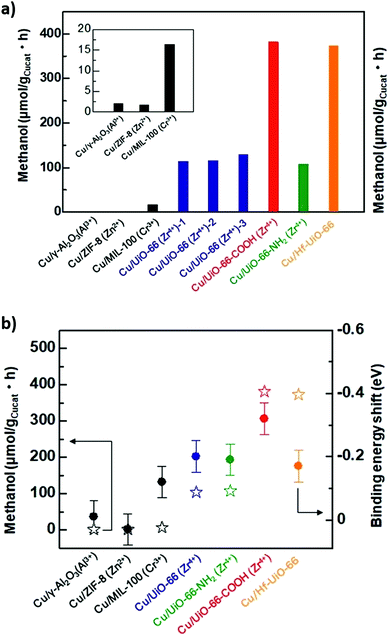 |
| Fig. 14 (a) Methanol productivity for crystalline framework encapsulated Cu nanoparticles. (b) Correlation between methanol productivity and binding energy shift of support metal component following Cu encapsulation. Adapted from ref. 273 with permission from The Royal Society of Chemistry, copyright 2019. | |
The preceding examples highlight the importance of active sites arising from electronic interactions between metallic Cu and ZnO or ZrOx nodes in crystalline frameworks (UiO-66 and UiO-66-bpy). Although a variety of other crystalline frameworks have been investigated for methanol formation, these have proved unsuccessful. Note that such electronic perturbation of Cu appears a consequence of framework confinement rather than direct bond formation, and that electron-donating and withdrawing framework linkers offer fine control over the electronic state of Cu nanoparticles. This contrasts with conventional supported catalysts which only offer limited control over the local geometric and electronic environment around metal active sites. Although reports of methanol selective catalysts remain limited, spatial confinement and localised electronic perturbation of metal clusters/nanoparticles to modulate their environment and reactivity is an emerging paradigm. A performance comparison of porous crystalline framework derived methanol, ethanol and formic acid selective catalysts prepared by various routes appears in Table S2 (ESI†).
6.2 Methane selective CO2 reduction catalysts
A range of metals have been studied for the Sabatier reaction, including Pt, Pd, Rh, Ru, Fe, Co and Cu, however Ni nanoparticles supported on inorganic metal oxides such as Al2O3, TiO2, SiO2, ZrO2, CeO2, MgO and CaO have emerged as the most promising methanation catalysts274 due to their relatively high activity and Earth-abundance/low cost. Ni catalysts for CO2 methanation based on encapsulated nanoparticles within crystalline frameworks (MIL-101) have been developed which achieve complete conversion and 100% methane selectivity for up to 200 hours.111 Nanoparticle size and reducibility were identified as critical parameters that control activity and selectivity, being a function of the catalyst preparation and nature of the encapsulating framework (and resulting Ni interactions).
Zhen et al. investigated Ni-MOF-5 for the Sabatier reaction at 1 bar and a H2
:
CO2 ratio of 4.275 CO2 conversion increased with Ni loading, reaching a plateau ∼10 wt%, and was completely selective to methane at all conversions. A stable conversion of 75% was obtained at 320 °C for 80 hours, after which CO production was also observed. The high activity of this catalyst was ascribed to small Ni particles (9 nm) and a corresponding high metal dispersion (42%) and the facile reduction of Ni at 295 °C observed by TPR; a 10 wt% Ni/SiO2 catalyst with larger particles (42 nm) showed little reduction at this temperature. Readily reducible Ni species, associated with a SMSI, are likely active species for CO2 methanation. CO2 reduction was postulated to occur by initial reduction to CO and subsequent reduction to methane on Ni(111) facets. Ni/UiO-66 was also explored for this reaction.276 Ultra-dispersed Ni nanoparticles (2 nm) throughout the high surface area UiO-66 framework, and associated SMSI, increased the TOF to 155 h−1 at 320 °C and 68% conversion compared to 94 h−1 for Ni-MOF-5 at the same temperature and 75% conversion; both 100% selective to methane. Ni/UiO-66 was stable for 100 hours on-stream. However, the best results for CO2 methanation were obtained using 20 wt% Ni/MIL-101 prepared by double solvent impregnation.111 At 10 bar and a H2
:
CO2 ratio of 4, this delivered complete CO2 conversion and 100% methane selectivity at 300 °C, with a TOF of 188 h−1 stable for up to 200 hours. The high CH4 productivity was attributed to a high Ni dispersion (2.5 nm particles) and concomitant strong SMSI, which lowered the Ni(Ox) reduction temperature to only 252 °C, and a high proportion of Ni(111) facets from the double solvent synthesis. DFT calculations suggest that the activation barrier for CO2 dissociation over Ni(111) facets of 10 kcal mol−1 is half that over Ni(200) facets. Mihet et al. compared the performance of MIL-101 and UiO-66 encapsulated Ni nanoparticles of comparable size (<4 nm) and 10 wt% loading, prepared by impregnation versus the double solvent methods and subsequent NaBH4 reduction.274 Similar catalytic performance was observed for both syntheses with Ni/UiO-66, whereas impregnation was superior to the double solvent method for Ni encapsulated in MIL-101, in contradiction to the results of Zhen et al. The best performing Ni/MIL-101 catalyst prepared by impregnation gave 56% CO2 conversion and 92% methane selectivity at 320 °C, a CO2
:
H2 ratio of 8 and GHSV of 4650 h−1. However, it is difficult to draw comparisons between these two studies due to their differing reaction conditions, Ni loading, particle sizes and synthetic details. Pd nanoparticles have also been dispersed in UiO-66 framework by a sol–gel method, and the resulting 6 wt% Pd/UiO-66 catalyst achieved 56% CO2 conversion and 97% methane selectivity at 340 °C, 40 bar and a H2
:
CO2 ratio of 4.277
Encapsulation of Ni nanoparticles in porous crystalline frameworks appears an effective strategy for CO2 methanation, with 100% selectivity achieved over several catalysts, with highest yield (100% between 300–340 °C) reported for 20% Ni/MIL-101.111 Deactivation mechanisms remain to be established, necessary to improve catalyst lifetimes for large-scale application. Synthetic methods able to maximise the fraction of Ni(111) facets in encapsulated nanoparticles should also be leveraged to promote low temperature CO2 activation. To date, co-promoters have not been investigated for methanation catalysts derived from crystalline frameworks, and strategies to engineer e.g. hollow, core–shell, shape-controlled and bimetallic nanoparticles, or SACs have significant scope for exploitation in this field. The performance of porous crystalline framework derived methane selective catalysts prepared by various routes is compared in Table S3 (ESI†).
6.3 Carbon monoxide selective CO2 reduction catalysts
The Reverse Water Gas Shift (RWGS) reaction, being endothermic, is favoured at high temperatures and hence is an energy intensive process. Catalysts are therefore sought for low temperature RWGS with high CO selectivity. Nanoparticle catalysts using noble metals such as Au, Pt and Rh show high activity for RWGS but offer poor CO selectivity at low temperature.278 In contrast, catalysts based on Earth-abundant metals such as Zn, Cu, Fe and In show poor activity and selectivity for the RWGS reaction.278 Attention has therefore focused on supported noble metal catalysts, with a recent shift to the use of crystalline frameworks as a matrix for their dispersion and activation.
Gutterod et al. investigated the performance of Pt nanoparticles encapsulated in a UiO-67 framework for RWGS. Although CO2 conversion was only 6%, the selectivity to CO was >90% between 220 to 280 °C (along with a small amount of methane) for a H2
:
CO2 ratio of 6 at 1 bar, however CO selectivity reached 100% under H2 deficient conditions.279 For a loading of 1.5 wt%, ∼60% of Pt atoms were reduced at 350 °C, with a metal dispersion of 14% and 2.5 nm average particle size. Bimetallic Pt–Pd nanoparticles and Pt–Pd hollow nanocages encapsulated in UiO-67 were also studied, with Pt–Pd nanocages more active and selective, achieving 81% CO selectivity and 18% CO2 conversion at 400 °C, 20 bar and a H2
:
CO2 ratio of 3.280 CO was proposed to form by a redox mechanism, evidenced by a change in Pt2+/Pt0 speciation post-reaction. This performance was superior to Pt/mullite, Pt–Co/MCF-17 and Pt–Co/CeO2 catalysts.280 Other bimetallic catalysts, comprising Au core–Pd shell (Au@Pd) nanoparticles encapsulated in different MOFs, namely UiO-66,281 [Co2(oba)4(3-bpdh)2]·4H2O,282 MOF-74278 and UiO-67280 have also been investigated for the RWGS reaction. The Au@Pd functionalised UiO-67 was also further functionalised with PVP-stabilised Pt nanoparticles. Catalysts were evaluated between 200 and 400 °C, at 20 bar and H2
:
CO2 ratios ranging from 1 to 4. At 400 °C and a H2
:
CO2 ratio of 4, CO2 conversion decreased in the following order MOF-74 (35%) ∼ UiO-67 (35%) > Co-MOF (16%) > UiO-66 (7%), while CO selectivity decreased in the order MOF-74 (99%) > Co-MOF (88%) > UiO-67 (35%) > UiO-66 (72%). Pt nanoparticles on the external surface of the framework crystals were believed responsible for CO2 activation while Au@Pd nanoparticles encapsulated within the MOFs conferred high CO selectivity. In all cases higher temperatures favoured methane production rather than CO. A redox mechanism for CO formation was proposed with synergy between the two types of metallic nanoparticles mediated by hydrogen spillover.
Noble metal nanoparticles, particularly bimetallics, when encapsulated in crystalline frameworks can effectively promote RWGS between 300–400 °C. Epitaxial growth of MOF shells around bimetallic nanoparticles, and promotion of the resulting materials by metal (Pt) nanoparticles, is a complex but creative design strategy to independently tune different steps of the reaction pathway. Judicious choice of an appropriate crystalline framework, such as MOF-74, facilitates CO2 conversions reaching 35%, with CO selectivities exceeding 98%. Further investigations are required to elucidate the precise nature of the synergy between metal components within core–shell bimetallic, and between spatially separated metal nanoparticles, and how reactivity would be affected in the different metals were interchanged. A performance comparison of porous crystalline framework derived CO selective catalysts prepared by various routes appears in Table S4 (ESI†).
6.4 Formic acid selective CO2 reduction catalysts
Formic acid (FA) is actively pursued as a renewable, liquid energy storage and transport medium due to its high effective gravimetric H2 density (4.4 wt%) and low toxicity. FA decomposition can be controlled to achieve room temperature H2 release over a suitable catalyst. Although metal complexes are reported for FA synthesis and decomposition, their practical application is hindered by their poor stability, requirement for solvents as a reaction medium, and problematic catalyst/product recovery and separation. Heterogeneous counterparts are therefore desirable. Pd mono-, bi- and trimetallic nanoparticles, such as PdAg, PdAu, PdCu, PdAuCo and PdCuCr, show high activity for CO2 reduction to FA. Wen et al. reported CO2 reduction to FA and subsequent FA decomposition using the same ZIF-8@Pd1Ag2@ZIF-8 catalyst in a liquid phase batch process.283 This appears the only example of thermocatalytic CO2 reduction to FA using a catalyst derived from a crystalline framework. A “ship-in-a-bottle” approach was adopted to fabricate the catalyst; bimetallic Pd1Ag2 nanoparticles were added to solution during ZIF-8 synthesis, which was then allowed to proceed. This resulted in a layer of bimetallic Pd1Ag2 nanoparticles sandwiched between a ZIF-8 core and shell. The resulting FA productivity was 17 mmolFA gcat−1 over 24 h at 100 °C, 20 bar and a H2
:
CO2 ratio of 1. FA productivity decreased in the order ZIF-8@Pd1Ag2@ZIF-8 (17) > ZIF-8@Pd2Ag1@ZIF-8 (11) > ZIF-8@Pd0.5Ag2.5@ZIF-8 (10) > ZIF-8@Pd3@ZIF-8 (8.5). The activity of the optimum Pd1Ag2 catalyst was attributed to the small size (2.8 nm) of bimetallic nanoparticles, enhanced CO2 sorption in the ZIF-8 framework and charge-transfer to Pd from both Ag and proximate basic linkers. This catalyst was stable and reusable due to the protective ZIF-8 shell which hindered nanoparticles sintering.
6.5 Ethanol selective CO2 reduction catalysts
Copper CO2 reduction catalysts show poor selectivity towards ethanol and noble metals are required to enhance selectivity towards ethanol.284 An et al. designed an ethanol selective CO2 reduction catalyst by supporting CuI sites on Zr12 clusters, via deprotonation of hydroxyl groups on the clusters followed by metal–oxygen coordination, in a MOF assembled from Zr12 SBUs and organic linkers. The 14 negative charges on the [Zr12O8(μ3-O−)8(μ2-O−)6(carboxylate)18]14− SBUs were balanced by 11 CuI species at SBU binding sites, and three neighbouring Li+ ions. The Li+ ions were subsequently ion-exchanged with Cs+ to obtain the active catalyst. The Zr12-bpdc-CuCs catalyst was >99% selective to ethanol for 96% CO2 conversion at 100 °C, 20 bar and a H2
:
CO2 ratio of 3 in THF solvent. Activity rapidly dropped with increasing pressure, but under supercritical CO2 (300 bar) a turnover number (TON) of 4080 was achieved in 10 hours (an order of magnitude higher than at 20 bar). Catalysis was ascribed to cooperativity between proximate dinuclear CuI2 sites, which activated hydrogen and promoted C–C coupling of reactively-formed methanol and formyl species. Alkali cations provided an electron-rich environment for the Cu centre and helped stabilise the formyl intermediate. This study again highlights the importance of metal cluster-assembled crystalline frameworks as flexible architectures whose properties can be further refined to enable cooperative catalysis between well-defined active sites.
7.0 Crystalline frameworks as sacrificial precursors
Porous crystalline frameworks have also been explored as sacrificial precursors for the synthesis of catalysts with controlled composition, and unique nanostructure and morphology.285–287 Pyrolysis (heating under an inert atmosphere) of frameworks is usually employed to obtain carbon derivatives, however annealing in the presence of sulphur, selenium or sodium hypophosphite has also been used to create sulphide, selenide and phosphide derivatives.285 The advantages of crystalline frameworks as sacrificial precursors are that: (i) they typically offer high surface areas, pore volumes and unique crystal morphologies, some of these properties being retained in their pyrolysed derivatives since decomposition of framework components occurs simultaneously throughout crystals; (ii) their composition, topology and morphology are tunable, which imparts a similar versatility in their derivatives; and (iii) guest molecules, nanoparticles and species incorporated in framework cavities may be retained in their derivatives. Core–shell morphologies are most commonly obtained by such approaches, wherein pyrolysis of organic linkers results in carbon encapsulation of node/guest species. This approach has also proved successful for fabricating carbon supported SACs.224
In the context of CO2 reduction, framework-derived catalysts usually comprise porous functionalised carbon matrices encapsulating small (<5 nm) metal nanoparticles.288 Such carbon coated nanoparticles are resistant to sintering, phase change, and/or coking by virtue of the surrounding thin protective carbon layer. Chemical functionalities within the carbon shell can also influence nanoparticle reactivity,288 with CO2 reduction to methanol, methane, CO and hydrocarbons all reported. A mind map of catalysts derived from crystalline frameworks for CO2 reduction is presented in Fig. 15, with corresponding research reports compiled in Table S1 (ESI†).
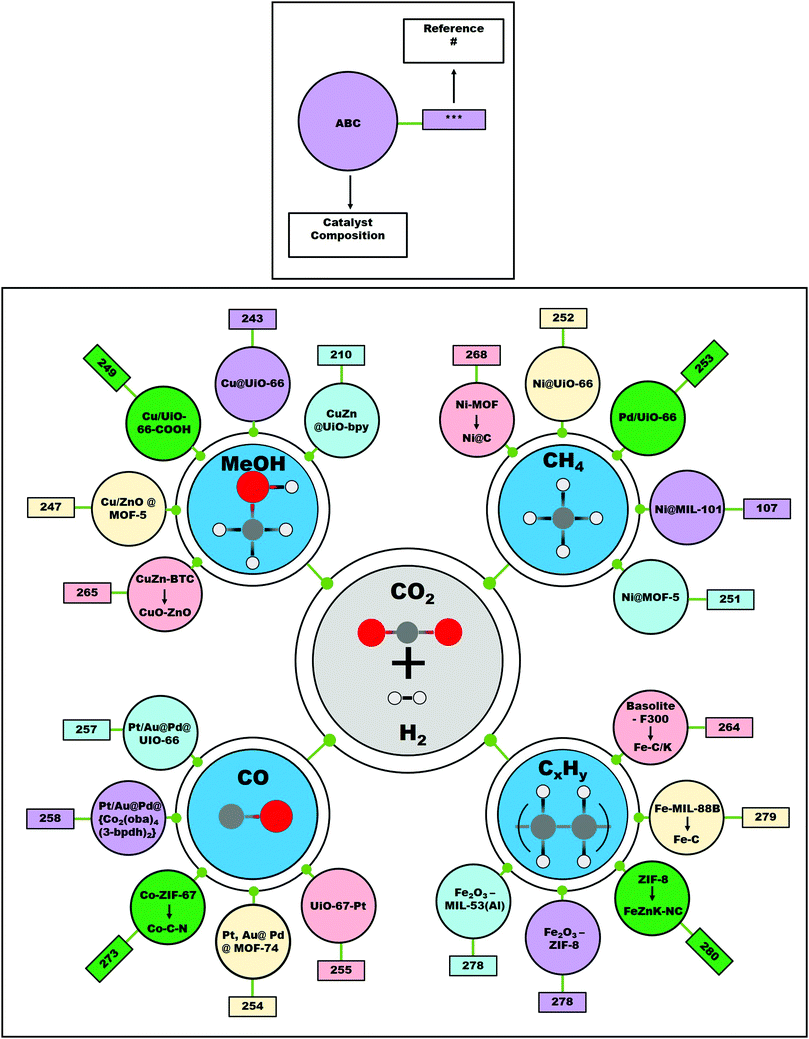 |
| Fig. 15 A mind map capturing representative catalysts derived from crystalline framework for CO2 reduction to CH3OH, CO, CH4 and hydrocarbons. | |
7.1 Methanol selective CO2 reduction catalysts
Decomposition of crystalline frameworks has been used to prepare carbon, metal oxide and alloy catalysts for the selective reduction of CO2 to methanol. Zhang et al. synthesised a bimetallic CuO/ZnO catalyst dispersed in N-doped carbon by the pyrolysis of a Cu/Zn-BTC coordination polymer; this afforded a uniform dispersion of Cu and Zn in the pyrolysed catalyst and strong interfacial interactions.289 The catalytic activity was higher than a Cu/ZnO/Al2O3 reference for up to 275 hours on-stream, with comparable methanol selectivity obtained at 220–250 °C and 40 bar. The high catalyst stability was attributed to the high metal dispersion and Cu–Zn interactions. A Pd–ZnO catalyst derived from pyrolysis of ZIF-8 framework containing encapsulated Pd nanoparticles achieved a methanol productivity of 0.65 gMeOH gcat−1 h−1 at 270 °C, 45 bar and a H2
:
CO2 ratio of 3.290 The encapsulated Pd nanoparticles spanned 2–4 nm, and evidence of PdZn alloy formation was apparent in the pyrolysed catalyst. Modest but stable CO2 conversion (20%) and methanol selectivity (55%) were observed for 50 hours on-stream, in contrast to the parent Pd/ZIF-8 for which CO was the major product. The higher methanol selectivity observed for the MOF-derived Pd–ZnO catalyst likely reflects electronic perturbation of Pd upon alloying with Zn. Intermediate formate (HCOO*) species were detected over PdZn alloy particles, with ZnO species responsible for CO2 activation and the stabilisation of oxygenate intermediates (H2CO*, CH3OH*).290
An In2O3@Co3O4 core–shell oxide composite, derived from the oxidative decomposition of In-impregnated ZIF-67(Co) achieved a similar methanol productivity of 0.65 gMeOH gcat−1 h−1 but with higher selectivity (87%) for 100 hours on-stream at 250–300 °C, 50 bar and a H2
:
CO2 ratio of 4.115 During reaction, the core–shell oxide composite converted to a mixed carbide phase (Co3InC0.75) decorated by discrete cobalt and indium oxides. An induction period was observed for the onset of methanol production, associated with formation of the mixed carbide phase, although the oxide nanoparticles were considered the catalytically active species. The induction period could be altered by changing the synthesis and ZIF-67 decomposition protocol, decreasing its duration to only 10 hours (versus 30 hours for a co-precipitated catalyst). Methanol formation over In2O3 may follow a Mars–van Krevelen mechanism involving the generation of oxygen vacancies by H2 and their quenching by CO2 activation (promoted by Co3O4). A conventional In2O3/Co3O4 catalyst prepared by co-precipitation demonstrated 0.65 gMeOH gcat−1 h−1 and 80% selectivity, supporting the proposal that these oxides are the active species.
Liu et al. investigated a Cu@ZrOx catalyst with 3D porosity, derived by in situ reconstruction of Cu nanoclusters encapsulated in defective UiO-66, obtaining a methanol productivity of 0.80 gMeOH gcat−1 h−1 at 13% CO2 conversion and 79% selectivity, 260 °C, 45 bar and a H2
:
CO2 ratio of 3, stable for 105 hours.291 During reaction, defective Zr clusters of the UiO-66 framework rearranged into a stable tetragonal zirconia phase, while retaining strong Cu–ZrOx metal support interactions through electron-transfer from Cu to Zr, which promoted the formation of Cu+ at the ZrO2 interface. DRIFT studies suggest that Cu+ are responsible for the high methanol selectivity by virtue of stabilising reactively-formed formate (HCOO*) and methoxy (CH3O*) intermediates.
ZrO2–MOx solid solutions comprising ZnO (ZnO–ZrO2), CuO (CuO–ZrO2) and Co3O4 (Co3O4–ZrO2) obtained from metal-functionalised, Schiff base grafted UiO-66 precursors, were tested for CO2 reduction at 320 °C, 30 bar and a H2
:
CO2 ratio of 3 for 80 hours on-stream. The UiO-66 framework was post-synthetically modified to incorporate a Schiff base, with Zn, Cu or Co ions subsequently chelated to the base prior to pyrolysis to obtain the mixed oxide solid solutions. CuO–ZrO2 showed the highest CO2 conversion (23%) but was 87% selective to CO. DRIFTS showed the presence of a CO* intermediate on the catalyst surface, indicating poor stabilisation of desired oxygenates. Co3O4–ZrO2 showed a modest CO2 conversion of 11% but with 93% selectivity to methane, although only methoxy (CH3O*) intermediates were observed by DRIFTS despite evident C–O bond cleavage. In contrast, ZnO–ZrO2 showed low (6%) CO2 conversion but 70% methanol selectivity, with formate and methoxy intermediates observed by DRIFTS; ZnO appears most effective in stabilising oxygenate intermediates.
Selective CO2 reduction to methanol is possible over various mixed metal oxides derived from the decomposition of crystalline frameworks, including Pd–ZnO, In2O3–CO3O4–Co3InC0.75, Cu–ZrOx and ZnO–ZrO2 (though not CuO–ZrOx). However, these catalysts appear to operate by diverse mechanisms involving alloys (Pd–ZnO), oxygen vacancy formation over redox active surfaces (In2O3–Co3O4) and selective stabilisation of methoxy intermediates (ZnO–ZrOx). Although high methanol selectivity and good stability was demonstrated for some catalysts, comparable to conventionally prepared inorganic catalysts, improving their modest activity remains an ongoing challenge as evident from Table S2 provided in ESI.†
7.2 Methane selective CO2 reduction catalysts
Porous crystalline frameworks have also been used as precursors to carbon and ZrO2 catalysts selective for methane production. Lin et al. prepared a carbon catalyst containing a Ni nanoparticle core with a carbon shell, Ni@C, by pyrolysis of a Ni-MOF (of undisclosed structure but based on trimesic acid linkers) at 500 °C for 2 hours.292 The resulting Ni@C catalyst was 100% selective to methane with a CO2 conversion (25%) three times that of a conventional Ni/C catalyst at 250 °C. Higher reaction temperatures increased conversion to 92% with stable operation at 325 °C for 24 hours. The high methanation activity of Ru has been exploited through doping of UiO-66 and subsequent in-operando activation to form a 1 wt% Ru/ZrO2 catalyst.293In situ XRD studies, supported by XAS, XPS and TEM, revealed that H2 promotes the formation of Ru0 nanoparticles, while CO2 acts as a mild oxidant to combust framework carbon and promote crystallisation of a (carbon-free) tetragonal ZrO2 phase able to stabilise the highly dispersed Ru nanoparticles.294
MOF-derived Ru/ZrO2 was completely selective to CH4 at high H2 (93%) and CO2 (96%) conversions, and stable for 160 hours, outperforming a commercial methaniser catalyst and 1 wt% Ru/SBA-15, 1 wt% Ru/C and 1 wt% Ru/ZrO2 controls under all reaction conditions. Zeng et al. investigated the performance of Ni supported on porous hydrous zirconia (obtained by UiO-66 decomposition in a basic medium) for CO2 reduction in a fixed-bed reactor at 300 °C and 20 bar, achieving >99% selectivity and a methane productivity of 5851 mmol gNi−1 h−1.295 The parent framework morphology was retained in the porous ZrO2, with surface hydroxyls reported to facilitate CO2 activation, however no significant performance advantage was observed compared to conventional Ni catalysts. The performance of porous crystalline framework derived methane selective catalysts is compared in Table S3 (ESI†).
7.3 Carbon monoxide selective CO2 reduction catalysts
Carbon catalysts derived from porous crystalline frameworks incorporating Cu, Zn and Co exhibit comparable RWGS performance to noble metal nanoparticles encapsulated within intact frameworks (Section 6.3). Zhang et al. investigated Cu/C and Cu/Zn/C catalysts prepared by pyrolysis of Cu-BTC frameworks as a strategy to hinder Cu nanoparticle sintering (held responsible for the deactivation of commercial Cu/Zn/Al2O3 catalysts).296 High metal (Cu and/or Zn) loadings of 70–75 wt% were achieved, albeit associated with large 15 to 25 nm particles. The pyrolysed carbon shell was porous, conferring surface areas spanning 96–125 m2 g−1 and protecting Cu nanoparticles against oxidation by air or N2O. At 500 °C, a H2
:
CO2 ratio of 3 and 1 bar, framework-derived Cu/C was 100% selective to CO with a stable, low activity for 20 hours. Catalyst stability was superior to a Cu/Zn catalyst prepared using an activated carbon support which deactivated after 11 hours on-stream. Lu et al. synthesized highly dispersed Co nanoparticles encapsulated in carbon matrices by pyrolysis of Co3(BTC)3·12H2O or ZIF-67.297 The 700 °C pyrolyzed ZIF-67 derived catalyst (Co–C–N-700) contained Co nanoparticles in a N-doped carbon matrix, and was eight times more active (25% CO2 conversion) for the RWGS reaction than a conventional Cu/Zn/Al2O3 catalyst, while achieving 90% CO selectivity at 450 °C, 1 bar and a H2
:
CO2 ratio of 2. Co–C–N-700 also proved superior to a Co–C-700 analogue derived from Co3(BTC)3·12H2O. The high activity of Co–C–N-700 catalyst was attributed to the formation of a Mott–Schottky junction and concomitant electron-transfer across the Co-support interface, which DFT calculations suggest lowers the barrier for CO2 reduction to CO by a redox mechanism and formate intermediates.297 Although such reports are scarce, they highlight possible routes to noble metal-free catalysts for atmospheric pressure CO2 reduction. A performance comparison of different porous crystalline framework derived CO selective catalysts prepared by various routes appears in Table S4 (ESI†).
7.4 CO2 Fischer–Tropsch catalysts
CO2 Fischer–Tropsch (CO2-FT) synthesis has gained attention for CO2 valorisation, with a Na-Fe3O4/HZSM-5 catalyst recently reported delivering up to 34% conversion and a high selectivity (up to 90%) to olefins and gasoline at 320 °C and 30 bar.38 The use of crystalline frameworks as sacrificial precursors was initially reported for CO-FT synthesis to prepare catalysts based on iron and cobalt carbides encapsulated in a carbon matrix.298–301 Guo et al. adopted a similar approach to disperse α-Fe2O3 nanoparticles in MIL-53(Al) and ZIF-8 by a solid grinding synthesis, resulting in a CO2 conversion up to 30% to CO and higher hydrocarbons at 300 °C and 30 bar.302 A higher olefin selectivity was observed for α-Fe2O3-ZIF-8 versus α-Fe2O3-MIL-53(Al). Decomposition of Fe-MIL-88b was also performed to obtain a high metal loading (34–51 wt%) CO2-FT catalyst offering CO2 conversion up to 46 wt% and good selectivity to hydrocarbons.303 Control over metal–support interactions is critical in CO2 FT catalysis, since reduction and carbonation of must be balanced against the desire for a high dispersion (and hence active site density).288 The latter is aided by a classical SMSI which however negatively impacts on metal reducibility and CO2 adsorption. Catalyst doping by K+ strongly promoted olefin formation relative to paraffins. During reaction, Fe nanoparticles were first reduced to a low valent/metallic state and subsequently a carbide phase whose composition was dependent on the nature of reaction intermediates and products.303 Pyrolysis at higher temperature resulted in metallic iron species which carbonized to a more graphitic, and less active, θ-Fe3C phase during reaction, while pyrolysis at 500–600 °C predominantly generated magnetite and the in-operando formation of Haag Carbide (χ-Fe5C2) which was held responsible for FT activity. This carbide was favoured over the K-doped catalyst. Wezendonk et al. reached similar conclusions while investigating the impact of MOF topology on resulting Fe/C phases and their performance in CO-FT synthesis.301 Fe/C catalysts derived by pyrolysis of MIL-68, MIL-88A, MIL-100, MIL-101, MIL-127, and Fe-BTC at 500 °C differed in their porosity, Fe content and nanoparticle size, crystallite density and thermal stability. Short nearest neighbour distances in parent frameworks led to larger Fe nanoparticles post pyrolysis, and a low pyrolysis temperature led to lower CO-FT activity. Iron catalysts (and K-promoted variants) derived from ZIF-8 for CO2 reduction304 exhibited slightly lower conversion (36%) at 320 °C, 30 bar and a H2
:
CO2 ratio of 3 than those obtained by MIL-88b pyrolysis,303 but achieved >60% hydrocarbon selectivity and a high olefin
:
paraffin ratio ≥5 due to the presence of pyridinic N.
Heteroatom promotion of iron carbide catalysts for CO2-FT, derived by pyrolysis of Basolite F300 MOF, is also reported.288 A CO2 conversion of 40% and selectivity to light olefins of 40% (24% selectivity to C1+ hydrocarbons) was obtained at 320 °C, 30 bar and a H2
:
CO ratio of 3 following promotion by 1 wt% K. Heteroatoms were introduced to the pyrolysed catalysts by incipient wetness impregnation. Despite the high (41 wt%) Fe loading, the average nanoparticle size was 4.4 nm with very few particles >20 nm. Potassium was the best promoter (being superior to Li and Na), although Cu and Pt also increased CO2 conversion, CH4 and light saturated hydrocarbons selectivity, whereas Mn, Mg and Mo enhanced CO selectivity and Ni, Rh and Co enhanced CH4 selectivity. XRD and XPS showed the presence of sintered Fe3O4 and a complex mix of metallic iron, Fe5C2, Fe7C3 and Fe3C in the K-promoted catalyst, whereas only less crystalline Fe3O4 and Fe3C were observed in Pt and Mo promoted catalysts. CO2-FT activity appears related to the RWGS activity of Fe3O4 and FT activity of FexCy phases. Potassium was largely present as the carbonate. CO2 and H2 chemisorption on the K-promoted catalyst were increased by 258% and decreased by 61% respectively compared to the unpromoted pyrolyzed catalyst. It was proposed that K charge-transfer to Fe strengthened the Fe–C interaction and rendered it less electrophilic, stabilising Fe against reduction by chemisorbed hydrogen. In contrast, Pt increased the H2 uptake, likely by weakening Fe–C interactions and resulting in CH4 formation due to a coverage of hydrogen adatoms. Mo-doping catalyst supressed CO2 and H2 adsorption, thereby promoting the RWGS reaction. In general, CO2-FT generates a complex mixture of Fe metal, oxide and carbide phases, and greater control over iron speciation could result in further improvements in activity and selectivity to hydrocarbons. A performance comparison of porous crystalline framework derived CO2 Fischer–Tropsch catalysts appears in Table S5 (ESI†).
8.0 Composite catalysts based on crystalline frameworks
Novel composites incorporating crystalline frameworks have appeared in the recent literature for niche applications, exploiting functional groups in the framework cavity or at their surface. Such composites may retain the physicochemical properties of their individual components, but gain additional properties such as liquid phase porosity,305 framework flexibility,306 and framework conductivity.307 Multifunctional and tandem reactions, including CO2 reduction, can benefit from the presence of compositionally distinct entities. Only a few reports exist to date on composite catalysts derived from crystalline frameworks for CO2 reduction, but significant advances are anticipated in this field.
8.1 Methanol selective CO2 reduction catalysts
Zhao et al. investigated the performance of Cu–ZnO–Al2O3 catalysts prepared by the pyrolysis of a composite comprising a Cu/Al layered double hydroxide (stacked sheet morphology) and Zn-BTC MOF (nanoflower morphology). The resulting material was 90% selective to methanol at 200 °C, 30 bar and a H2
:
CO2 ratio of 3, but only exhibited 4% CO2 conversion.308 Conversion increased with reaction temperature, but at the expense of methanol selectivity. In situ DRIFTS identified HCOO*, CO* and CH3O* intermediates, with CH3O* stabilised over ZnO accounting for methanol formation. Pd/ZnO@ZIF-8 composites have also been prepared by encapsulation of Pd/ZnO microspheres309 or nanorods310 with a ZIF-8 shell. CO2 reduction over the nanorod composites achieved 80% methanol selectivity, albeit for a low (∼6%) conversion, at 250 °C, 45 bar and a H2
:
CO2 ratio of 3 with stable activity over 25 hours. The ZIF-8 shell was grown by etching Zn2+ cations from the ZnO core to form the MOF precursor, which also introduced oxygen defects into the nanorods, with the ZIF-8 shell thickness controlled by the etching time. PdZn alloy nanoparticles were formed at the ZnO–ZIF-8 interface by subsequent wet impregnation and reduction. Methanol production was attributed to a synergy between the alloy nanoparticles and oxygen defects in ZnO. Increasing the ZIF-8 shell thickness created more defects, but disfavoured PdZn alloying at the expense of pure Pd nanoparticles. Metal-oxide@MOF core shell constructs are an attractive strategy for multistep reactions such as CO2 reduction since active sites at the oxide core surface remain accessible through the porous (and potentially catalytically active) MOF shell.
8.2 Carbon monoxide selective CO2 reduction catalysts
A Pd/ZSM-5@UiO-66-NH2 composite catalyst, comprised of Pd/ZSM-5 and Pd/UiO-66-NH2 is reported for RWGS at 320 °C, 20 bar and a H2
:
CO2 ratio of 3 for 40 hours on-stream.311 CO2 conversion decreased in the order Pd/UiO-66-NH2 (25%) > Pd/ZSM-5@UiO-66-NH2 (17%) > Pd/ZSM-5 (4%) > ZSM-5@UiO-66-NH2 (0%), while CO selectivity decreased from Pd/ZSM-5@UiO-66-NH2 (92%) > Pd/ZSM-5 (91%) > Pd/UiO-66-NH2 (31%) > ZSM-5@UiO-66-NH2 (0%). The Pd containing composite offered the optimal balance of modest conversion and high CO selectivity. The amine group in UiO-66-NH2 helped to stabilise smaller Pd nanoparticles (∼2 nm) than could be obtained on ZSM-5 alone (5 nm); CO2 conversion was proportional to Pd particle size. The high CO selectivity of Pd/ZSM-5 and Pd/ZSM-5@UiO-66-NH2 compared to Pd/UiO-66-NH2 indicated that ZSM-5 also participated in the reaction, possibly stabilising important reaction intermediates. CO2 reduction was proposed to occur by a redox mechanism, evidenced by Pd2+ species on the catalyst post-reaction. Stabilisation of Pd nanoparticles by the UiO-66-NH2 shell, and the high thermal stability of the ZSM-5 core, supressed catalyst deactivation, contrasting with Pd/UiO-66-NH2 which decomposed to ZrO2 during reaction. This study highlights the importance of crystalline framework linkers in stabilising metal nanoparticles.
8.3 Formic acid selective CO2 reduction catalysts
Mori et al. investigated the performance of a PdAg/TiO2@ZIF-8 composite catalyst for CO2 reduction to formic acid in a batch reactor using 1 M NaHCO3 as the CO2 source at 100 °C, 20 bar and a H2
:
CO2 ratio of 1 for 6 hours reaction, observing a TON of 913 and >99% formic acid selectivity.312 This TON was twice that of a standalone PdAg/TiO2 catalyst, and 29 times higher than that of PdAg–ZIF-8. The nanocomposite was synthesised by encapsulating rutile crystallites, pre-functionalised with PdAg alloy nanoparticles, with a 1.6 nm thick ZIF-8 shell. DFT calculations suggest that alloy nanoparticles and Zn2+ ions in the ZIF-8 framework promote HCO3− adsorption, with the former also influencing the electronic density of carbon in the bound HCO3−. Electronic-rich Pd was identified by XPS and DFT: the average atomic charge from DFT for Pd19Ag18 and Pd11Ag26 clusters in the presence of ZIF-8 was more negative that without the MOF shell. The high activity of PdAg/TiO2@ZIF-8 versus PdAg/TiO2 may in part reflect the presence of electronic-rich Pd in the composite, which reportedly promotes CO2 reduction to formic acid.
9.0 Catalyst lifetime and deactivation
Catalyst lifetime is critical to the commercial implementation of a catalytic process, influencing overall sustainability, reactor selection, process design/integration, and of course economic viability.313 Rapid catalyst deactivation and attendant short lifetimes can sometimes be overcome using recirculating fluidised bed reactors, coupled with a catalyst regeneration step, however this places additional constraints on catalyst formulation such as the need for binders, the choice of particle shape, mechanical strength, attrition resistance and so on. Catalysts derived from porous crystalline frameworks must therefore demonstrate sufficient stability and operational lifetime. Deactivation may arise from: (i) degradation of the metallic component due to sintering, coking, poisoning and/or oxidation;314 (ii) physicochemical changes in the supporting or encapsulating crystalline framework.315
9.1 Deactivation of metal component
Sintering is a general term used to describe the growth of metal particles under a reactive environment, and may occur through either particle migration and agglomeration or Ostwald ripening in which some particles grow at the expense of others.316 It is a universal phenomenon in thermocatalysis processes, and while the extent may vary, it is almost always detrimental due to a loss of active surface area. Metal atom migration and/or particle agglomeration reflect the mobility of a material upon heating and are governed by the Tamman and Huttig temperatures.317 Metal–support interactions can mitigate sintering, but may compromise catalytic activity and/or selectivity. For CO hydrogenation, monodispersed Ni nanoparticles over SiO2 were transformed during reaction to a bimodal particle distribution comprising small spherical and large faceted crystals (with a high density of Ni(111) facets).318 Particle sintering was attributed to the formation and migration of mobile Ni subcarbonyl species in the presence of CO.318 The same phenomenon is potentially problematic for certain CO2 reduction catalysts containing transition metals able to form volatile carbonyl complexes which facilitate support migration, or even loss to the vapor phase. In the case of Ni, improved nanoparticle stabilisation can be achieved using mixed oxides with a redox capacity, such as Ce0.72Zr0.28O2,319 and strongly interacting supports such as NiAl layered double hydroxides which allow for a topotactic transition of the Ni2+ ions,320 and can stabilise catalysts for hundreds of hour on-stream. Small (111) faceted Ni nanoparticles on MIL-101 also exhibit excellent stability for CO2 reduction to methane for up to 200 hours at 300 °C.111 Crystalline frameworks offer tunable, 3D framework–linker interactions for encapsulated metal particles, and hence better sinter-resistance than conventional low area, mesoporous or planar supports in which the metal local environment is less constrained.
Metal coking (surface poisoning by strongly-bound carbon) is another undesirable yet common mechanism of deactivation in thermal catalysts involving carbon-containing reactants,321 and typically a consequence of cracking C–C and/or C–H bonds. Severe coking is typically observed at temperatures >300 °C, and its impact is especially severe for microporous catalysts, such as zeolites, and some MOFs and COFs, wherein small pores are rapidly blocked by carbon residues, and consequently any unique activity or e.g. shape selectivity, associated with micropores is lost.322 Coking can be drastically reduced by low temperature operation, and or the introduction or pore hierarchy.323,324 The high activity of catalysts derived from crystalline frameworks in some instances permits low(er) temperature CO2 reduction, which may alleviate coking. The larger pore apertures and higher degree of pore connectivity in crystalline frameworks relative to zeolites is also expected to be beneficial in limiting the impact of coking, particularly for the CO2-FT reaction wherein the production of higher carbons is often accompanied by coke formation. Iron-based CO2-FT are also susceptible to phase changes on contact with carbonaceous species. The more stable, but less active, Fe3C phase can form by carburisation of the more active, but less stable, Fe5C2 phase.322 A better understanding of the stability and interconversion of iron carbide phases and their respective activities is important to advance CO2-FT technologies.
Metal poisoning by reversibly-bound reactants, surface intermediates or products is difficult to control. Water and CO, common to almost all CO2 reduction pathways, present a major challenge, reacting with transition and alkali/alkaline-earth metals. Water is a strong poison of Fe CO2-FT catalysts due to its rapid reaction with FexC phases, which suppresses hydrocarbon production.325 High pressure CO2 feedstocks may also contain significant SOx and NOx impurities which can strongly coordinate metals within framework nodes and/or encapsulated or supported nanoparticles. Framework hydrophobisation is one strategy to improve the water tolerance of such catalysts. Further opportunities exist to construct multifunctional catalysts which combine the molecular sieving and selective binding capabilities of crystalline frameworks to remove SOx and NOx poisons, with catalytic sites for CO2 reduction.
9.2 Reduction in activity due to change in physical and chemical characteristics of the crystalline framework
Different strategies have been proposed to utilise crystalline frameworks as catalyst components, where they serve the function of acting as a support, encapsulation materials or are present as a composite material for a specific purpose. Prolonged exposure to reaction environment can lead to changes in the physical and chemical characteristics of the framework components such as modification of framework–linker functional groups, modification of framework-nodes, partial destruction of framework structure due to breaking of weak bonds and permanent fixation of a rotating framework linker. Such changes may lead to temporary or permanent reduction in the activity and/or lifetime of crystalline frameworks-based catalysts. For example, a (Cu/ZnO)@MOF-5 catalyst completely collapsed during CO2 reduction, with resulting methanol yields falling 17-fold (from 212 to 12 μmolMeOH gcat−1 h−1) due to this structural collapse.271 A Ru/UiO-66 catalyst also collapsed during CO2 reduction to form a Ru0/ZrO2 phase, but retained 99.9% selectivity to methane and 96% CO2 conversion for 160 hours.293 Such structural changes can impact the interaction of framework and metal components, potentially hindering the requisite synergy for cooperative catalysis in CO2 reduction. For example, a study of condensation reactions in Fe(BTC) and MIL-100(Fe) MOFs revealed that reaction intermediates such as bisarylmethylcation, and by-products such as benzoic acid, can poison catalysis by strong adsorption and blockage of pores.326
10.0 Summary and outlook
The versatility of crystalline frameworks, which possess unique structural, physical and chemical properties has led to their rapid exploration as functional nanomaterials. CO2 reduction presents a host of scientific and technical challenges, requiring multifunctional catalysts able to adsorb and activate CO2 and H2 at active sites within close proximity of each other, and which facilitate rapid molecular diffusion327,328 and are stable under moderate temperatures and high pressures. The versatility of crystalline frameworks arises from the innumerable combinations of nodes and linkers (and ease of their chemical modification), and resulting diversity of cavity shape, size, topology and chemical composition.
Three promising design strategies have been exploited to develop CO2 reduction catalysts derived from crystalline frameworks, namely the encapsulation (and/or dispersion) of metal, oxide or carbide nanoparticles, use of frameworks as sacrificial precursors, and the incorporation of frameworks into nanocomposites. The first of these may disrupt existing technologies for CO2 reduction, evident from the high activity and tunable selectivity to either methanol, methane or CO. Encapsulation can increase the activity and stability of metal nanoparticles by SMSI and or metal–ligand charge-transfer with crystalline framework components. Confinement also ensures proximity between metal sites active for H2 dissociation with those for CO2 activation in framework cavities. For example, modulation of the electronic properties of Cu nanoparticles encapsulated in UiO-66 and UiO-66-bpy (Zr-based) frameworks,234 enabled methanol synthesis with high selectivity due to co-location of Zr/ZnO sites for CO2 activation and Cu sites for H2 activation. However, further improvements in activity (CO2 conversion) are required for such framework-derived catalysts, while maintaining high methanol selectivity. This will necessitate precise control over the location and uniformity of encapsulated metal nanoparticles, and extended lifetime testing and synthetic scale-up. The exquisite structural control offered by porous crystalline frameworks is exemplified by the Zr12-bpdc-CuCs catalyst for the selective reduction of CO2 to ethanol,284 while CO2 reduction to methane over a 20% Ni/MIL-101 catalyst (preferentially exposing Ni(111) facets) can deliver a 100% methane yield for 100 hours operation. The use of crystalline frameworks as sacrificial precursors to CO2 reduction catalysts enables the preparation of metal core–porous carbon shell architectures that permit facile reactant/product transport to/from active sites while suppressing metal sintering and coking. Such materials are ideally suited for CO2-FT to hydrocarbons (notably olefins).303 Controlled decomposition of crystalline frameworks has also allowed the synthesis of well-defined metal oxide catalysts with high activities for methanol115 or methane293 formation from CO2. When carefully designed, porous crystalline frameworks can also be combined with other discrete catalyst entities such as layered double hydroxides308 or oxide nanocrystals312e.g. or to form nanocomposite catalysts which retain the properties of their parent components but afford unique synergies, such as the promotion of CO2 reduction to formic acid.
In light of the vast array of potential crystalline frameworks and functionalisation strategies, judicious selection of the appropriate CO2 reduction catalyst should proceed through careful consideration of the precise reaction conditions (and hence product desired) under which it will operate. High temperatures and high H2
:
CO2 ratios generally favour hydrocarbon products (CO2-FT), but also increase the likelihood of coking. Low temperatures and low H2
:
CO2 ratios (and the presence of basicity) will favour oxygenate products such as methanol and formic acid but result in poor activity.
As catalysts approach commercialisation, technoeconomic life cycle assessments will be necessary to assess the use of non-renewable H2 for CO2 reduction. New thermocatalytic technologies for CO2 reduction may initially be exploited within the chemicals sector, which is responsible for significant CO2 emissions (and wherein non-renewable H2 production is already available) and is a major user of methanol, formic acid, hydrocarbons and syngas. CO2 capture and use at such sources could drastically reduce the cost of feedstock/product supply and logistics. Technological progress in greenhouse gas capture and mitigation is likely to improve faster than solar, wind and battery advances.329 Such improvements will be driven in part by membrane separation, condensation/rectification and adsorption strategies.329 It remains to be seen whether scientific, engineering and regulatory agencies can facilitate adoption of such CO2 capture strategies by emission intensive industries such as cement, iron, steel and ammonia production, petrochemical refining and fermentation. A supportive environment created by regulatory policies and appropriate carbon taxes or subsidies would promote the immediate adoption of thermocatalytic CO2 reduction from non-renewable H2 (grey H2), offering partial mitigation of CO2 emissions.
Advances in renewable H2 (green H2) production will significantly lower its price relative to existing grey H2 sources (which are energy intensive with a large carbon footprint), driving the transition to cleaner CO2 reduction processes using the former. A critical assessment of methanol production from CO2 and green H2 identified the cost of so-called green methanol to be 1.3 to 2.6 times higher than of fossil-derived methanol, with 73% of the green methanol costs associated with obtaining renewable H2.330 Several innovative strategies have been proposed to improve the technoeconomic basis for green H2.331–333 For example, concentrated solar-thermal routes could yield H2 at $3–17 per kg.334,335 H2 produced by water electrolysis using renewable electricity from wind, geothermal, tidal or hydro sources is generally considered the most sustainable and clean option, with costs between $2.91–10.92 per kg.53 Current costs for grey H2 are significantly lower ($1.35–3.5 per kg54), hence the uptake of CO2 reduction technologies is strongly dependent on lowering the cost of green H2. However, the global landscape for green H2 is set to drastically change in the near future. Expected strong reductions in capital expenditure costs for electrolysers of 70–80%, and falling levelized cost of renewable energy, are predicted to drive the cost of green H2 to only $1–1.5 per kg in optimal locations (competitive with grey H2) and $2–3 per kg under average conditions by 2030.55 Worldwide governmental initiatives promoting large scale H2 production will likely prove the biggest drivers to reduce the total cost of ownership for H2 (production and distribution).55 The development and implementation of large-scale thermocatalytic CO2 reduction technologies, employing some form of crystalline framework catalysts, is therefore anticipated over the next two decades, when green H2 is expected to be cost-competitive with alternative fossil and renewable energy resources.
A complete mechanistic understanding of thermocatalytic CO2 reduction remains elusive. There is a wide gap between experimental studies and theoretical predictions. Although experiments have focused on composition–activity and structure–activity relationships, led by an empirical trial-and-error approach, few are sufficiently detailed and rigorous to inform theoretical chemists. Conversely, computational studies on simple model catalysts have limited application in rationalising experimental results from more complex nanoparticle and multifunctional catalysts in practical use. Therefore, it is vital for further progress that experimental and theoretical studies are conducted together in the same group or via collaborative efforts to further advance this exciting field.
Conflicts of interest
There are no conflicts of interest to declare.
Acknowledgements
Authors sincerely acknowledge the support from Australian Research Council (ARC) under the Discovery project (DP180104076) on “Novel conversion process for carbon dioxide to chemicals”. R. B. acknowledges the Australian Research Council (DECRA fellowship DE160100987) for financial support.
References
- J. R. Petit, J. Jouzel, D. Raynaud, N. I. Barkov, J. M. Barnola, I. Basile, M. Bender, J. Chappellaz, M. Davisk, G. Delaygue, M. Delmotte, V. M. Kotlyakov, M. Legrand, V. Y. Lipenkov, C. Lorius, L. P. Pin, C. Ritz, E. Saltzmank and M. Stievenard, Nature, 1999, 399, 429–436 CrossRef CAS.
- P. Lionello and L. Scarascia, Reg. Environ. Change, 2018, 18, 1481–1493 CrossRef.
- R. Yu, P. Zhai and Y. Chen, Curr. Opin. Environ. Sust., 2018, 30, 75–81 CrossRef.
- R. Falkner, Int. Aff., 2016, 92, 1107–1125 CrossRef.
- S. Roy, A. Cherevotan and S. C. Peter, ACS Energy Lett., 2018, 3, 1938–1966 CrossRef CAS.
- M. D. Porosoff, B. Yan and J. G. Chen, Energy Environ. Sci., 2016, 9, 62–73 RSC.
- M. Aresta, A. Dibenedetto and A. Angelini, Chem. Rev., 2014, 114, 1709–1742 CrossRef CAS PubMed.
-
G. Pagani, US Pat., 4,208,347, 1980.
-
F. Zardi and S. Debernardi, US Pat., 8,367,867 B2, 2013 Search PubMed.
- H. Kolbe and E. Lautemann, Annalen, 1869, 113, 125 CrossRef.
-
E. Solvay, US Pat., 263981A, 1882.
- M. W. Farlow and H. Adkins, J. Am. Chem. Soc., 1935, 57, 2222–2223 CrossRef CAS.
- B. Eliasson, C.-j. Liu and U. Kogelschatz, Ind. Eng. Chem. Res., 2000, 39, 1221–1227 CrossRef CAS.
- P. J. Chantry, J. Chem. Phys., 1972, 57, 3180–3186 CrossRef CAS.
- J. F. Walker and N. D. Scott, J. Am. Chem. Soc., 1938, 60, 951–955 CrossRef CAS.
- W. McGhee and D. Riley, J. Org. Chem., 1995, 60, 6205–6207 CrossRef CAS.
- Y. R. Jorapur and D. Y. Chi, J. Org. Chem., 2005, 70, 10774–10777 CrossRef CAS PubMed.
- M. A. Pacheco and C. L. Marshall, Energy Fuels, 1997, 11, 2–29 CrossRef CAS.
- T. Sakai, Y. Tsutsumi and T. Ema, Green Chem., 2008, 10, 337–341 RSC.
- A. Rokicki and W. Kuran, J. Macromol. Sci., Rev. Macromol. Chem. Phys., 1981, 100, 135–186 Search PubMed.
- D. J. Darensbourg, Chem. Rev., 2007, 107, 2388–2410 CrossRef CAS PubMed.
- G.-P. Wu, S.-H. Wei, W.-M. Ren, X.-B. Lu, T.-Q. Xu and D. J. Darensbourg, J. Am. Chem. Soc., 2011, 133, 15191–15199 CrossRef CAS PubMed.
- M. Aresta, D. Ballivet-Tkatchenko, D. B. Dell'Amico, M. C. Bonnet, D. Boschi, F. Calderazzo, R. E. Faure, L. Labella and F. Marchetti, Chem. Commun., 2000, 1099–1100 RSC.
- M. H. Jamróz, J. C. Dobrowolski, J. E. Rode and M. A. Borowiak, THEOCHEM, 2002, 618, 101–108 CrossRef.
- A. Dibenedetto, M. Aresta, C. Fragale and M. Narracci, Green Chem., 2002, 4, 439–443 RSC.
- O. Ihata, Y. Kayaki and T. Ikariya, Macromolecules, 2005, 38, 6429–6434 CrossRef CAS.
- I. Pápai, G. Schubert, I. Mayer, G. Besenyei and M. Aresta, Organometallics, 2004, 23, 5252–5259 CrossRef.
- D. del Moral, A. M. Banet Osuna, A. Córdoba, J. M. Moretó, J. Veciana, S. Ricart and N. Ventosa, Chem. Commun., 2009, 4723–4725 RSC.
- K. Buchmüller, N. Dahmen, E. Dinjus, D. Neumann, B. Powietzka, S. Pitter and J. Schön, Green Chem., 2003, 5, 218–223 RSC.
- M. Aresta, A. Dibenedetto, I. Papai and G. Schubert, Inorg. Chim. Acta, 2002, 334, 294–300 CrossRef CAS.
- I. Yoshio, I. Yoshio and H. Harukichi, Chem. Lett., 1977, 855–856 Search PubMed.
- T. Tsuda, S. Morikawa, R. Sumiya and T. Saegusa, J. Org. Chem., 1988, 53, 3140–3145 CrossRef CAS.
- K. Armstrong and P. Styring, Front. Energy Res., 2015, 3, 8 Search PubMed.
- N. Mac Dowell, P. S. Fennell, N. Shah and G. C. Maitland, Nat. Clim. Change, 2017, 7, 243 CrossRef CAS.
- T. Witoon, P. Kidkhunthod, M. Chareonpanich and J. Limtrakul, Chem. Eng. J., 2018, 348, 713–722 CrossRef CAS.
- S. Bai, Q. Shao, P. Wang, Q. Dai, X. Wang and X. Huang, J. Am. Chem. Soc., 2017, 139, 6827–6830 CrossRef CAS PubMed.
- J. M. Thomas and K. D. M. Harris, Energy Environ. Sci., 2016, 9, 687–708 RSC.
- J. Wei, Q. Ge, R. Yao, Z. Wen, C. Fang, L. Guo, H. Xu and J. Sun, Nat. Commun., 2017, 8, 15174 CrossRef PubMed.
- C. Xie, C. Chen, Y. Yu, J. Su, Y. Li, G. A. Somorjai and P. Yang, Nano Lett., 2017, 17, 3798–3802 CrossRef CAS PubMed.
- Z. Li, J. Wang, Y. Qu, H. Liu, C. Tang, S. Miao, Z. Feng, H. An and C. Li, ACS Catal., 2017, 7, 8544–8548 CrossRef CAS.
- X. Zhang, A. Zhang, X. Jiang, J. Zhu, J. Liu, J. Li, G. Zhang, C. Song and X. Guo, J. CO2 Util., 2019, 29, 140–145 CrossRef CAS.
- Y. Wang, L. Tan, M. Tan, P. Zhang, Y. Fang, Y. Yoneyama, G. Yang and N. Tsubaki, ACS Catal., 2019, 9(2), 895–901 CrossRef CAS.
- N. Thonemann and M. Pizzol, Energy Environ. Sci., 2019, 12, 2253–2263 RSC.
- S. Deutz, D. Bongartz, B. Heuser, A. Kätelhön, L. Schulze Langenhorst, A. Omari, M. Walters, J. Klankermayer, W. Leitner, A. Mitsos, S. Pischinger and A. Bardow, Energy Environ. Sci., 2018, 11, 331–343 RSC.
- R. G. Grim, Z. Huang, M. T. Guarnieri, J. R. Ferrell, L. Tao and J. A. Schaidle, Energy Environ. Sci., 2020, 13, 472–494 RSC.
- M. Thema, F. Bauer and M. Sterner, Renewable Sustainable Energy Rev., 2019, 112, 775–787 CrossRef CAS.
- F. Schuth, Science, 2019, 363, 1282–1283 CrossRef PubMed.
- T. Palden, B. Onghena, M. Regadío and K. Binnemans, Green Chem., 2019, 21, 5394–5404 RSC.
- H. Rodhe, Science, 1990, 248, 1217–1219 CrossRef CAS PubMed.
- P. Bains, P. Psarras and J. Wilcox, Prog. Energy Combust. Sci., 2017, 63, 146–172 CrossRef.
- E. I. Koytsoumpa, C. Bergins and E. Kakaras, J. Supercrit. Fluids, 2018, 132, 3–16 CrossRef CAS.
- C. Wang, G. Wang, R. Yang, X. Sun, H. Ma and S. Sun, Langmuir, 2017, 33, 503–509 CrossRef CAS PubMed.
- K. Schoots, F. Ferioli, G. Kramer and B. Vanderzwaan, Int. J. Hydrogen Energy, 2008, 33, 2630–2645 CrossRef CAS.
-
H. Dagdougui, R. Sacile, C. Bersani and A. Ouammi, in Hydrogen Infrastructure for Energy Applications, ed. H. Dagdougui, R. Sacile, C. Bersani and A. Ouammi, Academic Press, 2018, pp. 7–21 DOI:10.1016/B978-0-12-812036-1.00002-0.
-
H. Council, Path to hydrogen competitiveness A cost perspective, 2020 Search PubMed.
- M. Ghoussoub, M. Xia, P. N. Duchesne, D. Segal and G. Ozin, Energy Environ. Sci., 2019, 12, 1122–1142 RSC.
- Y. Song, X. Zhang, K. Xie, G. Wang and X. Bao, Adv. Mater., 2019, 31, 1902033 CrossRef CAS PubMed.
- L. Zhang, Z.-J. Zhao, T. Wang and J. Gong, Chem. Soc. Rev., 2018, 47, 5423–5443 RSC.
- F. Marques Mota and D. H. Kim, Chem. Soc. Rev., 2019, 48, 205–259 RSC.
- J. M. Spurgeon and B. Kumar, Energy Environ. Sci., 2018, 11, 1536–1551 RSC.
- F. P. García de Arquer, C.-T. Dinh, A. Ozden, J. Wicks, C. McCallum, A. R. Kirmani, D.-H. Nam, C. Gabardo, A. Seifitokaldani, X. Wang, Y. C. Li, F. Li, J. Edwards, L. J. Richter, S. J. Thorpe, D. Sinton and E. H. Sargent, Science, 2020, 367, 661–666 CrossRef PubMed.
- U. Ulmer, T. Dingle, P. N. Duchesne, R. H. Morris, A. Tavasoli, T. Wood and G. A. Ozin, Nat. Commun., 2019, 10, 3169 CrossRef PubMed.
- Y. Ma, Z. Wang, X. Xu and J. Wang, Chin. J. Catal., 2017, 38, 1956–1969 CrossRef CAS.
- Y. Chen, D. Wang, X. Deng and Z. Li, Catal. Sci. Technol., 2017, 7, 4893–4904 RSC.
- D. Chen, X. Zhang and A. F. Lee, J. Mater. Chem. A, 2015, 3, 14487–14516 RSC.
- W. Wang, X. M. Xu, W. Zhou and Z. P. Shao, Adv. Sci., 2017, 4, 1600371 CrossRef PubMed.
- A. Liu, M. Gao, X. Ren, F. Meng, Y. Yang, L. Gao, Q. Yang and T. Ma, J. Mater. Chem. A, 2020, 8, 3541–3562 RSC.
- S. L. James, Chem. Soc. Rev., 2003, 32, 276–288 RSC.
- X. Feng, X. Ding and D. Jiang, Chem. Soc. Rev., 2012, 41, 6010–6022 RSC.
- J. Yao and H. Wang, Chem. Soc. Rev., 2014, 43, 4470–4493 RSC.
- P. Z. Moghadam, A. Li, S. B. Wiggin, A. Tao, A. G. P. Maloney, P. A. Wood, S. C. Ward and D. Fairen-Jimenez, Chem. Mater., 2017, 29, 2618–2625 CrossRef CAS.
- J. L. Wang, C. Wang and W. B. Lin, ACS Catal., 2012, 2, 2630–2640 CrossRef CAS.
- S. B. Wang and X. C. Wang, Small, 2015, 11, 3097–3112 CrossRef CAS PubMed.
- A. Dhakshinamoorthy, A. M. Asiri and H. Garcia, Angew. Chem., Int. Ed., 2016, 55, 5414–5445 CrossRef CAS PubMed.
- L. Zeng, X. Y. Guo, C. He and C. Y. Duan, ACS Catal., 2016, 6, 7935–7947 CrossRef CAS.
- Z. B. Liang, C. Qu, D. G. Xia, R. Q. Zou and Q. Xu, Angew. Chem., Int. Ed., 2018, 57, 9604–9633 CrossRef CAS PubMed.
- J. D. Xiao, L. L. Han, J. Luo, S. H. Yu and H. L. Jiang, Angew. Chem., Int. Ed., 2018, 57, 1103–1107 CrossRef CAS PubMed.
- X. Chang, T. Wang and J. Gong, Energy Environ. Sci., 2016, 9, 2177–2196 RSC.
- A. Mahmood, W. H. Guo, H. Tabassum and R. Q. Zou, Adv. Energy Mater., 2016, 6, 1600423 CrossRef.
- B. A. Johnson, A. Bhunia, H. H. Fei, S. M. Cohen and S. Ott, J. Am. Chem. Soc., 2018, 140, 2985–2994 CrossRef CAS PubMed.
- D. Yang and B. C. Gates, ACS Catal., 2019, 9, 1779–1798 CrossRef CAS.
- L. Jiao, Y. Wang, H. L. Jiang and Q. Xu, Adv. Mater., 2018, 30, 1703663 CrossRef PubMed.
- H. He, J. A. Perman, G. Zhu and S. Ma, Small, 2016, 12, 6309–6324 CrossRef CAS PubMed.
- Y. B. Huang, J. Liang, X. S. Wang and R. Cao, Chem. Soc. Rev., 2017, 46, 126–157 RSC.
- A. Dhakshinamoorthy, A. M. Asiri and H. Garcia, ACS Catal., 2019, 9(2), 1081–1102 CrossRef CAS.
- Y.-S. Kang, Y. Lu, K. Chen, Y. Zhao, P. Wang and W.-Y. Sun, Coord. Chem. Rev., 2019, 378, 262–280 CrossRef CAS.
- J. Gascon, A. Corma, F. Kapteijn and F. X. Llabrés i Xamena, ACS Catal., 2014, 4, 361–378 CrossRef CAS.
- W.-G. Cui, G.-Y. Zhang, T.-L. Hu and X.-H. Bu, Coord. Chem. Rev., 2019, 387, 79–120 CrossRef CAS.
- C. Wang, B. An and W. Lin, ACS Catal., 2019, 9, 130–146 CrossRef CAS.
- F. M. Wisser, Y. Mohr, E. A. Quadrelli and J. Canivet, ChemCatChem, 2020, 12, 1270–1275 CrossRef CAS.
- M. Oschatz and M. Antonietti, Energy Environ. Sci., 2018, 11, 57–70 RSC.
- Z. Bao, G. Chang, H. Xing, R. Krishna, Q. Ren and B. Chen, Energy Environ. Sci., 2016, 9, 3612–3641 RSC.
- A. R. Millward and O. M. Yaghi, J. Am. Chem. Soc., 2005, 127, 17998–17999 CrossRef CAS PubMed.
- L. Xia, Q. Liu, F. Wang and J. Lu, J. Mol. Model., 2016, 22, 254 CrossRef PubMed.
- A. Demessence, D. M. D'Alessandro, M. L. Foo and J. R. Long, J. Am. Chem. Soc., 2009, 131, 8784–8786 CrossRef CAS PubMed.
- C. Hon Lau, R. Babarao and M. R. Hill, Chem. Commun., 2013, 49, 3634–3636 RSC.
- Y. Li, S. H. Chan and Q. Sun, Nanoscale, 2015, 7, 8663–8683 RSC.
- R. Babarao and J. Jiang, Langmuir, 2008, 24, 6270–6278 CrossRef CAS PubMed.
- A. Stukowski, Modell. Simul. Mater. Sci. Eng., 2009, 18, 015012 CrossRef.
- Y.-P. Chen, T.-F. Liu, S. Fordham and H.-C. Zhou, Acta Crystallogr., Sect. B: Struct. Sci., Cryst. Eng. Mater., 2015, 71, 613–618 CrossRef CAS PubMed.
- A. Jain, S. P. Ong, G. Hautier, W. Chen, W. D. Richards, S. Dacek, S. Cholia, D. Gunter, D. Skinner, G. Ceder and K. A. Persson, APL Mater., 2013, 1, 011002 CrossRef.
- Y. A. Daza and J. N. Kuhn, RSC Adv., 2016, 6, 49675–49691 RSC.
- X. Su, X. Yang, B. Zhao and Y. Huang, J. Energy Chem., 2017, 26, 854–867 CrossRef.
- A. Álvarez, A. Bansode, A. Urakawa, A. V. Bavykina, T. A. Wezendonk, M. Makkee, J. Gascon and F. Kapteijn, Chem. Rev., 2017, 117, 9804–9838 CrossRef PubMed.
- M. M.-J. Li and S. C. E. Tsang, Catal. Sci. Technol., 2018, 8, 3450–3464 RSC.
- S. Dang, H. Yang, P. Gao, H. Wang, X. Li, W. Wei and Y. Sun, Catal. Today, 2019, 330, 61–75 CrossRef CAS.
- A. Dokania, A. Ramirez, A. Bavykina and J. Gascon, ACS Energy Lett., 2019, 4, 167–176 CrossRef CAS.
- S. Abelló, C. Berrueco and D. Montané, Fuel, 2013, 113, 598–609 CrossRef.
- B. Lu and K. Kawamoto, Mater. Res. Bull., 2014, 53, 70 CrossRef CAS.
- K. Oshima, T. Shinagawa, Y. Nogami, R. Manabe, S. Ogo and Y. Sekine, Catal. Today, 2014, 232, 27–32 CrossRef CAS.
- W. Zhen, F. Gao, B. Tian, P. Ding, Y. Deng, Z. Li, H. Gao and G. Lu, J. Catal., 2017, 348, 200–211 CrossRef CAS.
- C. Janke, M. S. Duyar, M. Hoskins and R. Farrauto, Appl. Catal., B, 2014, 152–153, 184–191 CrossRef CAS.
- D. Sun, F. M. Khan and D. S. A. Simakov, Chem. Eng. J., 2017, 329, 165–177 CrossRef CAS.
- S. Rönsch, J. Schneider, S. Matthischke, M. Schlüter, M. Götz, J. Lefebvre, P. Prabhakaran and S. Bajohr, Fuel, 2016, 166, 276–296 CrossRef.
- A. Pustovarenko, A. Dikhtiarenko, A. Bavykina, L. Gevers, A. Ramírez, A. Russkikh, S. Telalovic, A. Aguilar, J.-L. Hazemann, S. Ould-Chikh and J. Gascon, ACS Catal., 2020, 10, 5064–5076 CrossRef CAS.
- M. R. Rahimpour and M. Lotfinejad, Chem. Eng. Technol., 2007, 30, 1062–1076 CrossRef CAS.
- M. R. Rahimpour, M. Bayat and F. Rahmani, Chem. Eng. J., 2010, 157, 520–529 CrossRef CAS.
- J. Zhong, X. Yang, Z. Wu, B. Liang, Y. Huang and T. Zhang, Chem. Soc. Rev., 2020, 49, 1385–1413 RSC.
- S. T. Sie and R. Krishna, Appl. Catal., A, 1999, 186, 55–70 CrossRef CAS.
- J.-S. Kim, S. Lee, S.-B. Lee, M.-J. Choi and K.-W. Lee, Catal. Today, 2006, 115, 228–234 CrossRef CAS.
- S. Ringe, E. L. Clark, J. Resasco, A. Walton, B. Seger, A. T. Bell and K. Chan, Energy Environ. Sci., 2019, 12, 3001–3014 RSC.
- A. Bagger, W. Ju, A. S. Varela, P. Strasser and J. Rossmeisl, ACS Catal., 2019, 9, 7894–7899 CrossRef CAS.
- S. Xu and E. A. Carter, Chem. Rev., 2019, 119, 6631–6669 CrossRef CAS PubMed.
- S. S. Bhosale, A. K. Kharade, E. Jokar, A. Fathi, S.-m. Chang and E. W.-G. Diau, J. Am. Chem. Soc., 2019, 141, 20434–20442 CrossRef CAS PubMed.
- J. Fu, K. Jiang, X. Qiu, J. Yu and M. Liu, Mater. Today, 2020, 32, 222–243 CrossRef CAS.
- A. Alvarez, M. Borges, J. J. Corral-Perez, J. G. Olcina, L. Hu, D. Cornu, R. Huang, D. Stoian and A. Urakawa, ChemPhysChem, 2017, 18, 3135–3141 CrossRef CAS PubMed.
- X. Chen, X. Su, H.-Y. Su, X. Liu, S. Miao, Y. Zhao, K. Sun, Y. Huang and T. Zhang, ACS Catal., 2017, 7, 4613–4620 CrossRef CAS.
- J. Yoshihara and C. T. Campbell, J. Catal., 1996, 161, 776–782 CrossRef CAS.
- D. Cheng, F. R. Negreiros, E. Aprà and A. Fortunelli, ChemSusChem, 2013, 6, 944–965 CrossRef CAS PubMed.
- J. Yoshihara, S. C. Parker, A. Schafer and C. T. Campbell, Catal. Lett., 1995, 31, 313–324 CrossRef CAS.
- T. Kakumoto, Energy Convers. Manage., 1995, 36, 661–664 CrossRef CAS.
- Y. Yang, J. Evans, J. A. Rodriguez, M. G. White and P. Liu, Phys. Chem. Chem. Phys., 2010, 12, 9909–9917 RSC.
- P. B. Rasmussen, P. M. Holmblad, T. Askgaard, C. V. Ovesen, P. Stoltze, J. K. Norskov and I. Chorkendorff, Catal. Lett., 1994, 26, 373–381 CrossRef CAS.
- I. A. Fisher and A. T. Bell, J. Catal., 1997, 172, 222–237 CrossRef CAS.
- T. Fujitani, I. Nakamura, T. Uchijima and J. Nakamura, Surf. Sci., 1997, 383, 285–298 CrossRef CAS.
- I. Nakamura, T. Fujitani, T. Uchijima and J. Nakamura, Surf. Sci., 1998, 400, 387–400 CrossRef CAS.
- S. Posada-Pérez, P. J. Ramírez, R. A. Gutiérrez, D. J. Stacchiola, F. Viñes, P. Liu, F. Illas and J. A. Rodriguez, Catal. Sci. Technol., 2016, 6, 6766–6777 RSC.
- S. Collins, M. Baltanas and A. Bonivardi, J. Catal., 2004, 226, 410–421 CrossRef CAS.
- J. Ye, C.-j. Liu, D. Mei and Q. Ge, J. Catal., 2014, 317, 44–53 CrossRef CAS.
- S. Kattel, P. Liu and J. G. Chen, J. Am. Chem. Soc., 2017, 139, 9739–9754 CrossRef CAS PubMed.
- L. C. Grabow and M. Mavrikakis, ACS Catal., 2011, 1, 365–384 CrossRef CAS.
- M. Behrens, F. Studt, I. Kasatkin, S. Kuhl, M. Havecker, F. Abild-Pedersen, S. Zander, F. Girgsdies, P. Kurr, B.-L. Kniep, M. Tovar, R. W. Fischer, J. K. Norskov and R. Schlogl, Science, 2012, 336, 893–897 CrossRef CAS PubMed.
- Q.-L. Tang, Q.-J. Hong and Z.-P. Liu, J. Catal., 2009, 263, 114–122 CrossRef CAS.
- Q.-J. Hong and Z.-P. Liu, Surf. Sci., 2010, 604, 1869–1876 CrossRef CAS.
- Q. Sun and Z. Liu, Front. Chem., 2011, 6, 164 Search PubMed.
- S. Kattel, P. J. Ramirez, J. G. Chen, J. A. Rodriguez and P. Liu, Science, 2017, 355, 1296–1299 CrossRef CAS PubMed.
- C. Liu, B. Yang, E. Tyo, S. Seifert, J. DeBartolo, B. von Issendorff, P. Zapol, S. Vajda and L. A. Curtiss, J. Am. Chem. Soc., 2015, 137, 8676–8679 CrossRef CAS PubMed.
- J. Wang, S.-m. Lu, J. Li and C. Li, Chem. Commun., 2015, 51, 17615–17618 RSC.
- X. Nie, X. Jiang, H. Wang, W. Luo, M. J. Janik, Y. Chen, X. Guo and C. Song, ACS Catal., 2018, 8, 4873–4892 CrossRef CAS.
- A. A. Gokhale, J. A. Dumesic and M. Mavrikakis, J. Am. Chem. Soc., 2008, 130, 1402–1414 CrossRef CAS PubMed.
- L. C. Grabow, A. A. Gokhale, S. T. Evans, J. A. Dumesic and M. Mavrikakis, J. Phys. Chem. C, 2008, 112, 4608–4617 CrossRef CAS.
- Y. Yang, M. G. White and P. Liu, J. Phys. Chem. C, 2012, 116, 248–256 CrossRef CAS.
- J. Graciani, K. Mudiyanselage, F. Xu, A. E. Baber, J. Evans, S. D. Senanayake, D. J. Stacchiola, P. Liu, J. Hrbek, J. F. Sanz and J. A. Rodriguez, Science, 2014, 345, 546–550 CrossRef CAS PubMed.
- S. Kattel, B. Yan, Y. Yang, J. G. Chen and P. Liu, J. Am. Chem. Soc., 2016, 138, 12440–12450 CrossRef CAS PubMed.
- R. Gaikwad, A. Bansode and A. Urakawa, J. Catal., 2016, 343, 127–132 CrossRef CAS.
- Y.-F. Zhao, Y. Yang, C. Mims, C. H. F. Peden, J. Li and D. Mei, J. Catal., 2011, 281, 199–211 CrossRef CAS.
- R. J. Madon, D. Braden, S. Kandoi, P. Nagel, M. Mavrikakis and J. A. Dumesic, J. Catal., 2011, 281, 1–11 CrossRef CAS.
- A. J. Elliott, R. A. Hadden, J. Tabatabaei, K. C. Waugh and F. W. Zemicael, J. Catal., 1995, 157, 153–161 CrossRef CAS.
- N. Pasupulety, H. Driss, Y. A. Alhamed, A. A. Alzahrani, M. A. Daous and L. Petrov, Appl. Catal., A, 2015, 504, 308–318 CrossRef CAS.
- Q. Tang, Z. Shen, L. Huang, T. He, H. Adidharma, A. G. Russell and M. Fan, Phys. Chem. Chem. Phys., 2017, 19, 18539–18555 RSC.
- Y. Xia, Y. Xiong, B. Lim and S. E. Skrabalak, Angew. Chem., Int. Ed., 2009, 48, 60–103 CrossRef CAS PubMed.
- B. Anasori, M. R. Lukatskaya and Y. Gogotsi, Nat. Rev. Mater., 2017, 2, 16098 CrossRef CAS.
- X. W. Lou, L. A. Archer and Z. C. Yang, Adv. Mater., 2008, 20, 3987–4019 CrossRef CAS.
- S. C. Glotzer and M. J. Solomon, Nat. Mater., 2007, 6, 557–562 CrossRef PubMed.
- C. M. A. Parlett, K. Wilson and A. F. Lee, Chem. Soc. Rev., 2013, 42, 3876–3893 RSC.
- S. Zhou, L. Shang, Y. Zhao, R. Shi, G. I. N. Waterhouse, Y.-C. Huang, L. Zheng and T. Zhang, Adv. Mater., 2019, 0, 1900509 CrossRef PubMed.
- S. F. J. Hackett, R. M. Brydson, M. H. Gass, I. Harvey, A. D. Newman, K. Wilson and A. F. Lee, Angew. Chem., Int. Ed., 2007, 46, 8593–8596 CrossRef CAS PubMed.
- K. Liu, X. Zhao, G. Ren, T. Yang, Y. Ren, A. F. Lee, Y. Su, X. Pan, J. Zhang, Z. Chen, J. Yang, X. Liu, T. Zhou, W. Xi, J. Luo, C. Zeng, H. Matsumoto, W. Liu, Q. Jiang, K. Wilson, A. Wang, B. Qiao, W. Li and T. Zhang, Nat. Commun., 2020, 11, 1263 CrossRef CAS PubMed.
- A. M. Abdel-Mageed, B. Rungtaweevoranit, M. Parlinska-Wojtan, X. Pei, O. M. Yaghi and R. J. Behm, J. Am. Chem. Soc., 2019, 141, 5201–5210 CrossRef CAS.
- K.-i. Otake, Y. Cui, C. T. Buru, Z. Li, J. T. Hupp and O. K. Farha, J. Am. Chem. Soc., 2018, 140, 8652–8656 CrossRef CAS PubMed.
- A. Nalaparaju, R. Babarao, X. S. Zhao and J. W. Jiang, ACS Nano, 2009, 3, 2563–2572 CrossRef CAS PubMed.
- W. Liang, R. Babarao, T. L. Church and D. M. D'Alessandro, Chem. Commun., 2015, 51, 11286–11289 RSC.
- W. M. Bloch, R. Babarao, M. R. Hill, C. J. Doonan and C. J. Sumby, J. Am. Chem. Soc., 2013, 135, 10441–10448 CrossRef CAS.
- O. T. Qazvini, R. Babarao and S. G. Telfer, Chem. Mater., 2019, 31, 4919–4926 CrossRef CAS.
- L. K. Macreadie, E. J. Mensforth, R. Babarao, K. Konstas, S. G. Telfer, C. M. Doherty, J. Tsanaktsidis, S. R. Batten and M. R. Hill, J. Am. Chem. Soc., 2019, 141, 3828–3832 CrossRef CAS.
- V. E. Coyle, A. E. Kandjani, M. R. Field, P. Hartley, M. Chen, Y. M. Sabri and S. K. Bhargava, Biosens. Bioelectron., 2019, 141, 111479 CrossRef CAS PubMed.
- P. Makam, R. Shilpa, A. E. Kandjani, S. R. Periasamy, Y. M. Sabri, C. Madhu, S. K. Bhargava and T. Govindaraju, Biosens. Bioelectron., 2018, 100, 556–564 CrossRef CAS PubMed.
- A. Esmaielzadeh Kandjani, Y. M. Sabri, M. Mohammad-Taheri, V. Bansal and S. K. Bhargava, Environ. Sci. Technol., 2015, 49, 1578–1584 CrossRef CAS PubMed.
- A. E. Kandjani, M. Mohammadtaheri, A. Thakkar, S. K. Bhargava and V. Bansal, J. Colloid Interface Sci., 2014, 436, 251–257 CrossRef CAS PubMed.
- H. K. Daima, P. R. Selvakannan, A. E. Kandjani, R. Shukla, S. K. Bhargava and V. Bansal, Nanoscale, 2014, 6, 758–765 RSC.
- Z. M. Davoudi, A. E. Kandjani, A. I. Bhatt, I. L. Kyratzis, A. P. O'Mullane and V. Bansal, Adv. Funct. Mater., 2014, 24, 1047–1053 CrossRef CAS.
- A. Elbourne, V. E. Coyle, V. K. Truong, Y. M. Sabri, A. E. Kandjani, S. K. Bhargava, E. P. Ivanova and R. J. Crawford, Nanoscale Adv., 2019, 1, 203–212 RSC.
- V. Bansal, H. Jani, J. Du
Plessis, P. J. Coloe and S. K. Bhargava, Adv. Mater., 2008, 20, 717–723 CrossRef CAS.
- S. Mehla, J. Das, D. Jampaiah, S. Periasamy, A. Nafady and S. K. Bhargava, Catal. Sci. Technol., 2019, 9, 3582–3602 RSC.
- A. E. Kandjani, Y. M. Sabri, M. R. Field, V. E. Coyle, R. Smith and S. K. Bhargava, Chem. Mater., 2016, 28, 7919–7927 CrossRef CAS.
- Z. Hu, Y. Peng, Y. Gao, Y. Qian, S. Ying, D. Yuan, S. Horike, N. Ogiwara, R. Babarao, Y. Wang, N. Yan and D. Zhao, Chem. Mater., 2016, 28, 2659–2667 CrossRef CAS.
- S. Mehla, S. Kukade, P. Kumar, P. V. C. Rao, G. Sriganesh and R. Ravishankar, Fuel, 2019, 242, 487–495 CrossRef CAS.
- Y. Wang, H. Arandiyan, S. A. Bartlett, A. Trunschke, H. Sun, J. Scott, A. F. Lee, K. Wilson, T. Maschmeyer, R. Schlögl and R. Amal, Appl. Catal., B, 2020, 277, 119029 CrossRef CAS.
- M. D. Allendorf and V. Stavila, CrystEngComm, 2015, 17, 229–246 RSC.
- W. Tu, Y. Xu, S. Yin and R. Xu, Adv. Mater., 2018, 30, 1707582 CrossRef PubMed.
- O. K. Farha and J. T. Hupp, Acc. Chem. Res., 2010, 43, 1166–1175 CrossRef CAS PubMed.
- H. Furukawa, K. E. Cordova, M. O'Keeffe and O. M. Yaghi, Science, 2013, 341, 1230444 CrossRef PubMed.
- S. Mehla, V. Krishsna, G. Sriganesh and R. Ravishankar, RSC Adv., 2018, 8, 33702–33709 RSC.
- A. A. Olajire, Renewable Sustainable Energy Rev., 2018, 92, 570–607 CrossRef CAS.
- N. A. Khan, Z. Hasan and S. H. Jhung, Coord. Chem. Rev., 2018, 376, 20–45 CrossRef CAS.
- P. Silva, S. M. F. Vilela, J. P. C. Tome and F. A. A. Paz, Chem. Soc. Rev., 2015, 44, 6774–6803 RSC.
- J. Chen, K. Shen and Y. Li, ChemSusChem, 2017, 10, 3165–3187 CrossRef CAS.
- C. Wang, D. M. Liu and W. B. Lin, J. Am. Chem. Soc., 2013, 135, 13222–13234 CrossRef CAS.
- R. Krishna, Chem. Soc. Rev., 2012, 41, 3099–3118 RSC.
- W.-Y. Gao, A. D. Cardenal, C.-H. Wang and D. C. Powers, Chem. – Eur. J., 2019, 25, 3465–3476 CrossRef CAS PubMed.
- S. Lin, C. S. Diercks, Y.-B. Zhang, N. Kornienko, E. M. Nichols, Y. Zhao, A. R. Paris, D. Kim, P. Yang, O. M. Yaghi and C. J. Chang, Science, 2015, 349, 1208–1213 CrossRef CAS PubMed.
- H. Cui, Y. Wang, Y. Wang, Y.-Z. Fan, L. Zhang and C.-Y. Su, CrystEngComm, 2016, 18, 2203–2209 RSC.
- Y. Lee, S. Kim, H. Fei, J. K. Kang and S. M. Cohen, Chem. Commun., 2015, 51, 16549–16552 RSC.
- L. Shen, R. Liang, M. Luo, F. Jing and L. Wu, Phys. Chem. Chem. Phys., 2015, 17, 117–121 RSC.
- H. J. Mackintosh, P. M. Budd and N. B. McKeown, J. Mater. Chem., 2008, 18, 573–578 RSC.
- A. M. Shultz, O. K. Farha, J. T. Hupp and S. T. Nguyen, Chem. Sci., 2011, 2, 686–689 RSC.
- X. Chen, M. Addicoat, E. Jin, L. Zhai, H. Xu, N. Huang, Z. Guo, L. Liu, S. Irle and D. Jiang, J. Am. Chem. Soc., 2015, 137, 3241–3247 CrossRef CAS PubMed.
- A. Nagai, X. Chen, X. Feng, X. Ding, Z. Guo and D. Jiang, Angew. Chem., Int. Ed., 2013, 52, 3770–3774 CrossRef CAS PubMed.
- W. Zhang, P. Jiang, Y. Wang, J. Zhang and P. Zhang, Catal. Sci. Technol., 2015, 5, 101–104 RSC.
- E. D. Bloch, D. Britt, C. Lee, C. J. Doonan, F. J. Uribe-Romo, H. Furukawa, J. R. Long and O. M. Yaghi, J. Am. Chem. Soc., 2010, 132, 14382–14384 CrossRef CAS.
- J. X. Jiang, C. Wang, A. Laybourn, T. Hasell, R. Clowes, Y. Z. Khimyak, J. Xiao, S. J. Higgins, D. J. Adams and A. I. Cooper, Angew. Chem., Int. Ed., 2011, 50, 1072–1075 CrossRef CAS PubMed.
- W. Wang, A. Zheng, P. Zhao, C. Xia and F. Li, ACS Catal., 2014, 4, 321–327 CrossRef CAS.
- X. Han, Q. Xia, J. Huang, Y. Liu, C. Tan and Y. Cui, J. Am. Chem. Soc., 2017, 139, 8693–8697 CrossRef CAS PubMed.
- L.-H. Li, X.-L. Feng, X.-H. Cui, Y.-X. Ma, S.-Y. Ding and W. Wang, J. Am. Chem. Soc., 2017, 139, 6042–6045 CrossRef CAS PubMed.
- C. K. Brozek and M. Dincă, J. Am. Chem. Soc., 2013, 135, 12886–12891 CrossRef CAS PubMed.
- S. Yuan, Y.-P. Chen, J. Qin, W. Lu, X. Wang, Q. Zhang, M. Bosch, T.-F. Liu, X. Lian and H.-C. Zhou, Angew. Chem., Int. Ed., 2015, 54, 14696–14700 CrossRef CAS.
- Y. Ye, Z. Ma, R.-B. Lin, R. Krishna, W. Zhou, Q. Lin, Z. Zhang, S. Xiang and B. Chen, J. Am. Chem. Soc., 2019, 141, 4130–4136 CrossRef CAS PubMed.
- Y. Wang, X. Zhao, H. Yang, X. Bu, Y. Wang, X. Jia, J. Li and P. Feng, Angew. Chem., Int. Ed., 2019, 58, 6316 CrossRef CAS PubMed.
- J. C. Matsubu, V. N. Yang and P. Christopher, J. Am. Chem. Soc., 2015, 137, 3076–3084 CrossRef CAS PubMed.
- S. M. J. Rogge, A. Bavykina, J. Hajek, H. Garcia, A. I. Olivos-Suarez, A. Sepúlveda-Escribano, A. Vimont, G. Clet, P. Bazin, F. Kapteijn, M. Daturi, E. V. Ramos-Fernandez, F. X. Llabrés i Xamena, V. Van Speybroeck and J. Gascon, Chem. Soc. Rev., 2017, 46, 3134–3184 RSC.
- Y.-S. Wei, M. Zhang, R. Zou and Q. Xu, Chem. Rev., 2020 DOI:10.1021/acs.chemrev.9b00757.
- Ü. Kökçam-Demir, A. Goldman, L. Esrafili, M. Gharib, A. Morsali, O. Weingart and C. Janiak, Chem. Soc. Rev., 2020, 49, 2751–2798 RSC.
- X. Li, X. Yang, Y. Huang, T. Zhang and B. Liu, Adv. Mater., 2019, 31, 1902031 CrossRef CAS.
- A. Han, B. Wang, A. Kumar, Y. Qin, J. Jin, X. Wang, C. Yang, B. Dong, Y. Jia, J. Liu and X. Sun, Small Methods, 2019, 3, 1800471 CrossRef.
- M. F. M. Post, A. C. v. t. Hoog, J. K. Minderhoud and S. T. Sie, AIChE J., 1989, 35, 1107–1114 CrossRef CAS.
- D. Vervloet, F. Kapteijn, J. Nijenhuis and J. R. van Ommen, Catal. Sci. Technol., 2012, 2, 1221–1233 RSC.
- Y. Yao, X. Liu, D. Hildebrandt and D. Glasser, Appl. Catal., A, 2012, 433–434, 58–68 CrossRef CAS.
- B. Wang, W. Liu, W. Zhang and J. Liu, Nano Res., 2017, 10, 3826–3835 CrossRef CAS.
- D. Liu, J. Wan, G. Pang and Z. Tang, Adv. Mater., 2019, 31, 1803291 CrossRef PubMed.
- Z.-X. Cai, Z.-L. Wang, J. Kim and Y. Yamauchi, Adv. Mater., 2019, 31, 1804903 CrossRef PubMed.
- N. Musselwhite, K. Na, K. Sabyrov, S. Alayoglu and G. A. Somorjai, J. Am. Chem. Soc., 2015, 137, 10231–10237 CrossRef CAS PubMed.
- A. Shivhare, J. A. Hunns, L. J. Durndell, C. M. A. Parlett, M. A. Isaacs, A. F. Lee and K. Wilson, ChemSusChem DOI:10.1002/cssc.202000764.
- Y. Wang, S. Kattel, W. Gao, K. Li, P. Liu, J. G. Chen and H. Wang, Nat. Commun., 2019, 10, 1166 CrossRef.
- B. An, J. Zhang, K. Cheng, P. Ji, C. Wang and W. Lin, J. Am. Chem. Soc., 2017, 139, 3834–3840 CrossRef CAS PubMed.
- S. Aguado, J. Canivet, Y. Schuurman and D. Farrusseng, J. Catal., 2011, 284, 207–214 CrossRef CAS.
- H. Li, C. Qiu, S. Ren, Q. Dong, S. Zhang, F. Zhou, X. Liang, J. Wang, S. Li and M. Yu, Science, 2020, 367, 667–671 CrossRef CAS PubMed.
- C. Yang, U. Kaipa, Q. Z. Mather, X. Wang, V. Nesterov, A. F. Venero and M. A. Omary, J. Am. Chem. Soc., 2011, 133, 18094–18097 CrossRef CAS PubMed.
- S. Yuan, L. Feng, K. C. Wang, J. D. Pang, M. Bosch, C. Lollar, Y. J. Sun, J. S. Qin, X. Y. Yang, P. Zhang, Q. Wang, L. F. Zou, Y. M. Zhang, L. L. Zhang, Y. Fang, J. L. Li and H. C. Zhou, Adv. Mater., 2018, 30, 1704303 CrossRef PubMed.
- K. S. Park, Z. Ni, A. P. Cote, J. Y. Choi, R. Huang, F. J. Uribe-Romo, H. K. Chae, M. O'Keeffe and O. M. Yaghi, Proc. Natl. Acad. Sci. U. S. A., 2006, 103, 10186–10191 CrossRef CAS PubMed.
- N. M. Padial, E. Quartapelle Procopio, C. Montoro, E. Lopez, J. E. Oltra, V. Colombo, A. Maspero, N. Masciocchi, S. Galli, I. Senkovska, S. Kaskel, E. Barea and J. A. Navarro, Angew. Chem., Int. Ed., 2013, 52, 8290–8294 CrossRef CAS.
- B. Wang, X.-L. Lv, D. Feng, L.-H. Xie, J. Zhang, M. Li, Y. Xie, J.-R. Li and H.-C. Zhou, J. Am. Chem. Soc., 2016, 138, 6204–6216 CrossRef CAS PubMed.
- S. M. Cohen, Chem. Rev., 2012, 112, 970–1000 CrossRef CAS PubMed.
- S. M. Cohen, Chem. Sci., 2010, 1, 32–36 RSC.
- H. Li, M. Eddaoudi, M. O'Keeffe and O. M. Yaghi, Nature, 1999, 402, 276–279 CrossRef CAS.
- M. Eddaoudi, J. Kim, N. Rosi, D. Vodak, J. Wachter, M. O'Keeffe and O. M. Yaghi, Science, 2002, 295, 469–472 CrossRef CAS PubMed.
- J. A. Greathouse and M. D. Allendorf, J. Am. Chem. Soc., 2006, 128, 10678–10679 CrossRef CAS PubMed.
- S. Zuluaga, E. M. A. Fuentes-Fernandez, K. Tan, F. Xu, J. Li, Y. J. Chabal and T. Thonhauser, J. Mater. Chem. A, 2016, 4, 5176–5183 RSC.
- I. Pakamorė, J. Rousseau, C. Rousseau, E. Monflier and P. Á. Szilágyi, Green Chem., 2018, 20, 5292–5298 RSC.
- M. Rubio-Martinez, C. Avci-Camur, A. W. Thornton, I. Imaz, D. Maspoch and M. R. Hill, Chem. Soc. Rev., 2017, 46, 3453–3480 RSC.
- A. J. Howarth, Y. Liu, P. Li, Z. Li, T. C. Wang, J. T. Hupp and O. K. Farha, Nat. Rev. Mater., 2016, 1, 15018 CrossRef CAS.
- J. S. Qin, S. Yuan, C. Lollar, J. D. Pang, A. Alsalme and H. C. Zhou, Chem. Commun., 2018, 54, 4231–4249 RSC.
- C. Serre, F. Millange, C. Thouvenot, M. Nogues, G. Marsolier, D. Louer and G. Ferey, J. Am. Chem. Soc., 2002, 124, 13519–13526 CrossRef CAS PubMed.
- G. Férey, C. Serre, C. Mellot-Draznieks, F. Millange, S. Surblé, J. Dutour and I. Margiolaki, Angew. Chem., 2004, 116, 6456–6461 CrossRef.
- G. Ferey, C. Mellot-Draznieks, C. Serre, F. Millange, J. Dutour, S. Surble and I. Margiolaki, Science, 2005, 309, 2040–2042 CrossRef CAS PubMed.
- J. H. Cavka, S. Jakobsen, U. Olsbye, N. Guillou, C. Lamberti, S. Bordiga and K. P. Lillerud, J. Am. Chem. Soc., 2008, 130, 13850–13851 CrossRef PubMed.
- D. Feng, Z.-Y. Gu, J.-R. Li, H.-L. Jiang, Z. Wei and H.-C. Zhou, Angew. Chem., Int. Ed., 2012, 51, 10307–10310 CrossRef CAS.
- W. Morris, B. Volosskiy, S. Demir, F. Gándara, P. L. McGrier, H. Furukawa, D. Cascio, J. F. Stoddart and O. M. Yaghi, Inorg. Chem., 2012, 51, 6443–6445 CrossRef CAS PubMed.
- K. Wang, X.-L. Lv, D. Feng, J. Li, S. Chen, J. Sun, L. Song, Y. Xie, J.-R. Li and H.-C. Zhou, J. Am. Chem. Soc., 2016, 138, 914–919 CrossRef CAS PubMed.
- H. J. Choi, M. Dinca and J. R. Long, J. Am. Chem. Soc., 2008, 130, 7848–7850 CrossRef CAS.
- X.-L. Lv, K. Wang, B. Wang, J. Su, X. Zou, Y. Xie, J.-R. Li and H.-C. Zhou, J. Am. Chem. Soc., 2017, 139, 211–217 CrossRef CAS PubMed.
- S. Wang, S. Hou, C. Wu, Y. Zhao and X. Ma, Chin. Chem. Lett., 2019, 30, 398 CrossRef CAS.
- C. Wu, F. Irshad, M. Luo, Y. Zhao, X. Ma and S. Wang, ChemCatChem, 2019, 11, 1256–1263 CrossRef CAS.
- C. D. Wu and M. Zhao, Adv. Mater., 2017, 29, 1605446 CrossRef PubMed.
- M. Singh, D. Jampaiah, A. E. Kandjani, Y. M. Sabri, E. Della Gaspera, P. Reineck, M. Judd, J. Langley, N. Cox, J. van Embden, E. L. H. Mayes, B. C. Gibson, S. K. Bhargava, R. Ramanathan and V. Bansal, Nanoscale, 2018, 10, 6039–6050 RSC.
- G. Li, S. Zhao, Y. Zhang and Z. Tang, Adv. Mater., 2018, 30, 1800702 CrossRef PubMed.
- S. Luo, Z. Zeng, G. Zeng, Z. Liu, R. Xiao, M. Chen, L. Tang, W. Tang, C. Lai, M. Cheng, B. Shao, Q. Liang, H. Wang and D. Jiang, ACS Appl. Mater. Interfaces, 2019, 11, 32579–32598 CrossRef CAS PubMed.
- B. Rungtaweevoranit, J. Baek, J. R. Araujo, B. S. Archanjo, K. M. Choi, O. M. Yaghi and G. A. Somorjai, Nano Lett., 2016, 16, 7645–7649 CrossRef CAS PubMed.
- V. Mouarrawis, R. Plessius, J. I. van der Vlugt and J. N. H. Reek, Front. Chem., 2018, 6, 623 CrossRef CAS PubMed.
- P. J. Dauenhauer and O. A. Abdelrahman, ACS Cent. Sci., 2018, 4, 1235–1243 CrossRef CAS PubMed.
- T. L. Lohr and T. J. Marks, Nat. Chem., 2015, 7, 477–482 CrossRef CAS PubMed.
- M. Mueller, S. Hermes, K. Kaehler, M. W. E. van den Berg, M. Muhler and R. A. Fischer, Chem. Mater., 2008, 20, 4576–4587 CrossRef CAS.
- H. Tang, Y. Su, B. Zhang, A. F. Lee, M. A. Isaacs, K. Wilson, L. Li, Y. Ren, J. Huang, M. Haruta, B. Qiao, X. Liu, C. Jin, D. Su, J. Wang and T. Zhang, Sci. Adv., 2017, 3, e1700231 CrossRef.
- H. Kobayashi, J. M. Taylor, Y. Mitsuka, N. Ogiwara, T. Yamamoto, T. Toriyama, S. Matsumura and H. Kitagawa, Chem. Sci., 2019, 10, 3289–3294 RSC.
- M. Mihet, O. Grad, G. Blanita, T. Radu and M. D. Lazar, Int. J. Hydrogen Energy, 2019, 44, 13383–13396 CrossRef CAS.
- W. L. Zhen, B. Li, G. X. Lu and J. T. Ma, Chem. Commun., 2015, 51, 1728–1731 RSC.
- Z.-W. Zhao, X. Zhou, Y.-N. Liu, C.-C. Shen, C.-Z. Yuan, Y.-F. Jiang, S.-J. Zhao, L.-B. Ma, T.-Y. Cheang and A.-W. Xu, Catal. Sci. Technol., 2018, 8, 3160–3165 RSC.
- H. Jiang, Q. Gao, S. Wang, Y. Chen and M. Zhang, J. CO2 Util., 2019, 31, 167–172 CrossRef CAS.
- Y. Han, H. Xu, Y. Su, Z.-l. Xu, K. Wang and W. Wang, J. Catal., 2019, 370, 70–78 CrossRef CAS.
- E. S. Gutterod, S. Oien-Odegaard, K. Bossers, A. E. Nieuwelink, M. Manzoli, L. Braglia, A. Lazzarini, E. Borfecchia, S. Ahmadigoltapeh, B. Bouchevreau, B. T. Lonstad-Bleken, R. Henry, C. Lamberti, S. Bordiga, B. M. Weckhuysen, K. P. Lillerud and U. Olsbye, Ind. Eng. Chem. Res., 2017, 56, 13207–13219 CrossRef.
- H. Zhang, H. Xu, Y. Li and Y. Su, Appl. Mater. Today, 2020, 19, 100609 CrossRef.
- Z. Zheng, H. Xu, Z. Xu and J. Ge, Small, 2018, 14, 1702812 CrossRef PubMed.
- X. Zhao, H. Xu, X. Wang, Z. Zheng, Z. Xu and J. Ge, ACS Appl. Mater. Interfaces, 2018, 10, 15096–15103 CrossRef CAS PubMed.
- M. Wen, K. Mori, Y. Futamura, Y. Kuwahara, M. Navlani-García, T. An and H. Yamashita, Sci. Rep., 2019, 9, 15675 CrossRef PubMed.
- B. An, Z. Li, Y. Song, J. Zhang, L. Zeng, C. Wang and W. Lin, Nat. Catal., 2019, 2, 709–717 CrossRef CAS.
- A. Indra, T. Song and U. Paik, Adv. Mater., 2018, 30, 1705146 CrossRef.
- Q. Wang and D. Astruc, Chem. Rev., 2020, 120, 1438–1511 CrossRef CAS PubMed.
- K. O. Otun, X. Liu and D. Hildebrandt, J. Energy Chem., 2020, 51, 230–245 CrossRef.
- A. Ramirez, L. Gevers, A. Bavykina, S. Ould-Chikh and J. Gascon, ACS Catal., 2018, 8, 9174–9182 CrossRef CAS.
- C. Zhang, P. Y. Liao, H. Wang, J. Sun and P. Gao, Mater. Chem. Phys., 2018, 215, 211–220 CrossRef CAS.
- Y. Yin, B. Hu, X. Li, X. Zhou, X. Hong and G. Liu, Appl. Catal., B, 2018, 234, 143–152 CrossRef CAS.
- T. Liu, X. Hong and G. Liu, ACS Catal., 2020, 10, 93–102 CrossRef CAS.
- X. Lin, S. Wang, W. Tu, Z. Hu, Z. Ding, Y. Hou, R. Xu and W. Dai, Catal. Sci. Technol., 2019, 9, 731–738 RSC.
- R. Lippi, S. C. Howard, H. Barron, C. D. Easton, I. C. Madsen, L. J. Waddington, C. Vogt, M. R. Hill, C. J. Sumby, C. J. Doonan and D. F. Kennedy, J. Mater. Chem. A, 2017, 5, 12990–12997 RSC.
- R. Lippi, A. M. D’Angelo, C. Li, S. C. Howard, I. C. Madsen, K. Wilson, A. F. Lee, C. J. Sumby, C. J. Doonan, J. Patel and D. F. Kennedy, Catal. Today, 2020 DOI:10.1016/j.cattod.2020.04.043.
- L. Zeng, Y. Wang, Z. Li, Y. Song, J. Zhang, J. Wang, X. He, C. Wang and W. Lin, ACS Appl. Mater. Interfaces, 2020, 12, 17436–17442 CrossRef CAS PubMed.
- J. Zhang, B. An, Y. Hong, Y. Meng, X. Hu, C. Wang, J. Lin, W. Lin and Y. Wang, Mater. Chem. Front., 2017, 1, 2405–2409 RSC.
- X. Lu, Y. Liu, Y. He, A. N. Kuhn, P.-C. Shih, C.-J. Sun, X. Wen, C. Shi and H. Yang, ACS Appl. Mater. Interfaces, 2019, 11, 27717–27726 CrossRef CAS.
- B. An, K. Cheng, C. Wang, Y. Wang and W. Lin, ACS Catal., 2016, 6, 3610–3618 CrossRef CAS.
- B. Qiu, C. Yang, W. Guo, Y. Xu, Z. Liang, D. Ma and R. Zou, J. Mater. Chem. A, 2017, 5, 8081–8086 RSC.
- V. P. Santos, T. A. Wezendonk, J. J. D. Jaén, A. I. Dugulan, M. A. Nasalevich, H.-U. Islam, A. Chojecki, S. Sartipi, X. Sun, A. A. Hakeem, A. C. J. Koeken, M. Ruitenbeek, T. Davidian, G. R. Meima, G. Sankar, F. Kapteijn, M. Makkee and J. Gascon, Nat. Commun., 2015, 6, 6451 CrossRef CAS PubMed.
- T. A. Wezendonk, Q. S. E. Warringa, V. P. Santos, A. Chojecki, M. Ruitenbeek, G. Meima, M. Makkee, F. Kapteijn and J. Gascon, Faraday Discuss., 2017, 197, 225–242 RSC.
- S. Hu, M. Liu, F. S. Ding, C. S. Song, G. L. Zhang and X. W. Guo, J. CO2 Util., 2016, 15, 89–95 CrossRef CAS.
- J. H. Liu, A. F. Zhang, M. Liu, S. Hu, F. S. Ding, C. S. Song and X. W. Guo, J. CO2 Util., 2017, 21, 100–107 CrossRef CAS.
- J. Liu, Y. Sun, X. Jiang, A. Zhang, C. Song and X. Guo, J. CO2 Util., 2018, 25, 120–127 CrossRef CAS.
- M. Costa Gomes, L. Pison, C. Cervinka and A. Padua, Angew. Chem., Int. Ed., 2018, 57, 11909–11912 CrossRef CAS PubMed.
- M. L. Pinto, S. Dias and J. Pires, ACS Appl. Mater. Interfaces, 2013, 5, 2360–2363 CrossRef CAS PubMed.
- S. Chandra, T. Kundu, S. Kandambeth, R. BabaRao, Y. Marathe, S. M. Kunjir and R. Banerjee, J. Am. Chem. Soc., 2014, 136, 6570–6573 CrossRef CAS PubMed.
- F. Zhao, L. Fan, K. Xu, D. Hua, G. Zhan and S.-F. Zhou, J. CO2 Util., 2019, 33, 222–232 CrossRef CAS.
- L. Lin, T. Zhang, H. Liu, J. Qiu and X. Zhang, Nanoscale, 2015, 7, 7615–7623 RSC.
- X. Li, G. Liu, D. Xu, X. Hong and S. C. Edman Tsang, J. Mater. Chem. A, 2019, 7, 23878–23885 RSC.
- X. Pan, H. Xu, X. Zhao and H. Zhang, ACS Sustainable Chem. Eng., 2020, 8, 1087–1094 CrossRef CAS.
- K. Mori, A. Konishi and H. Yamashita, J. Phys. Chem. C, 2020, 124(21), 11499–11505 CrossRef CAS.
- P. Forzatti and L. Lietti, Catal. Today, 1999, 52, 165–181 CrossRef CAS.
- A. Cao, R. Lu and G. Veser, Phys. Chem. Chem. Phys., 2010, 12, 13499–13510 RSC.
- M. Opanasenko, A. Dhakshinamoorthy, J. Čejka and H. Garcia, ChemCatChem, 2013, 5, 1553–1561 CrossRef CAS.
- T. W. Hansen, A. T. DeLaRiva, S. R. Challa and A. K. Datye, Acc. Chem. Res., 2013, 46, 1720–1730 CrossRef CAS PubMed.
- A. J. Grano, F. M. Sayler, J.-H. Smått and M. G. Bakker, Mater. Lett., 2013, 111, 154–157 CrossRef CAS.
- M. Agnelli, M. Kolb and C. Mirodatos, J. Catal., 1994, 148, 9–21 CrossRef CAS.
- F. Ocampo, B. Louis and A.-C. Roger, Appl. Catal., A, 2009, 369, 90–96 CrossRef CAS.
- S. He, C. Li, H. Chen, D. Su, B. Zhang, X. Cao, B. Wang, M. Wei, D. G. Evans and X. Duan, Chem. Mater., 2013, 25, 1040–1046 CrossRef CAS.
- E. E. Wolf and F. Alfani, Catal. Rev., 1982, 24, 329–371 CrossRef CAS.
- S.-C. Lee, J.-S. Kim, W. C. Shin, M.-J. Choi and S.-J. Choung, J. Mol. Catal. A: Chem., 2009, 301, 98–105 CrossRef CAS.
- W. Khan, X. Jia, Z. Wu, J. Choi and A. C. Yip, Catalysts, 2019, 9, 127 CrossRef.
- X. Shen, J. Kang, W. Niu, M. Wang, Q. Zhang and Y. Wang, Catal. Sci. Technol., 2017, 7, 3598–3612 RSC.
- R. Satthawong, N. Koizumi, C. Song and P. Prasassarakich, Catal. Today, 2015, 251, 34–40 CrossRef CAS.
- M. Opanasenko, A. Dhakshinamoorthy, J. Čejka and H. Garcia, ChemCatChem, 2013, 5, 1553–1561 CrossRef CAS.
- D. Saha, Z. Bao, F. Jia and S. Deng, Environ. Sci. Technol., 2010, 44, 1820–1826 CrossRef CAS PubMed.
- T. M. Tovar, J. Zhao, W. T. Nunn, H. F. Barton, G. W. Peterson, G. N. Parsons and M. D. LeVan, J. Am. Chem. Soc., 2016, 138, 11449–11452 CrossRef CAS PubMed.
- M. Sharifzadeh, G. Triulzi and C. L. Magee, Energy Environ. Sci., 2019, 12, 2789–2805 RSC.
- A. González-Garay, M. S. Frei, A. Al-Qahtani, C. Mondelli, G. Guillén-Gosálbez and J. Pérez-Ramírez, Energy Environ. Sci., 2019, 12, 3425–3436 RSC.
- M. Qolipour, A. Mostafaeipour and O. M. Tousi, Renewable Sustainable Energy Rev., 2017, 78, 113–123 CrossRef.
- R. Boudries, Int. J. Hydrogen Energy, 2018, 43, 3406–3417 CrossRef CAS.
- Y. Khojasteh Salkuyeh, B. A. Saville and H. L. MacLean, Int. J. Hydrogen Energy, 2017, 42, 18894–18909 CrossRef CAS.
- T. Pregger, D. Graf, W. Krewitt, C. Sattler, M. Roeb and S. Möller, Int. J. Hydrogen Energy, 2009, 34, 4256–4267 CrossRef CAS.
- R. J. Detz, J. N. H. Reek and B. C. C. van der Zwaan, Energy Environ. Sci., 2018, 11, 1653–1669 RSC.
Footnote |
† Electronic supplementary information (ESI) available. See DOI: 10.1039/d0ee01882a |
|
This journal is © The Royal Society of Chemistry 2021 |