DOI:
10.1039/D4RA01384K
(Paper)
RSC Adv., 2024,
14, 10481-10498
LC-MS/DIA-based strategy for comprehensive flavonoid profiling: an Ocotea spp. applicability case†
Received
23rd February 2024
, Accepted 22nd March 2024
First published on 2nd April 2024
Abstract
We introduce a liquid chromatography – mass spectrometry with data-independent acquisition (LC-MS/DIA)-based strategy, specifically tailored to achieve comprehensive and reliable glycosylated flavonoid profiling. This approach facilitates in-depth and simultaneous exploration of all detected precursors and fragments during data processing, employing the widely-used open-source MZmine 3 software. It was applied to a dataset of six Ocotea plant species. This framework suggested 49 flavonoids potentially newly described for these plant species, alongside 45 known features within the genus. Flavonols kaempferol and quercetin, both exhibiting O-glycosylation patterns, were particularly prevalent. Gas-phase fragmentation reactions further supported these findings. For the first time, the apigenin flavone backbone was also annotated in most of the examined Ocotea species. Apigenin derivatives were found mainly in the C-glycoside form, with O. porosa displaying the highest flavone
:
flavonol ratio. The approach also allowed an unprecedented detection of kaempferol and quercetin in O. porosa species, and it has underscored the untapped potential of LC-MS/DIA data for broad and reliable flavonoid profiling. Our study annotated more than 50 flavonoid backbones in each species, surpassing the current literature.
1 Introduction
In the dynamic field of natural product (NP) research, the quest for high coverage and accurate metabolite profiling is essential, particularly because of the presence of closely related compounds such as glycosylated flavonoids. These metabolites often present challenges in the automatic annotation process, thus there is a significant and current requirement for enhanced analytical characterization methods.1–5 Flavonoids, in general, are natural compounds widespread in plants, which are generally perceived as complex datasets in metabolomics studies. Plant species from the Ocotea genus are one of the richest in chemical diversity within the family of Lauraceae plants, while still considered a botanical challenge for precise identification and distinction.6–8 Lauraceae plants were highly recognized for their economic value in the past century, whereas several have demonstrated relevant medicinal potential, which is supported by traditional uses.9,10 Biological activities including anti-inflammatory, antimicrobial, and cytotoxic were reported for a range of Ocotea spp., which were attributed mainly to the presence of alkaloids, flavonoids, and lignoids.10 Recently, several benzylisoquinoline and aporphine alkaloids from 60 different Ocotea spp. were evidenced as potential biomarkers of anti-inflammatory activity.11 Moreover, flavonoids were found to be widespread in these species using advanced data-independent acquisition (DIA) molecular networking approaches.12
Recent phytochemical studies carried out by our group revealed the anti-inflammatory potential of O. diospyrifolia and O. odorifera, leading to the isolation of several NPs, including a novel aporphine alkaloid from the former, named diospirifoline.13,14 However, a gap in the complete chemical characterization of these plants persists, impeding comprehensive chemophenetic analyses.15 Chemophenetics is a recently proposed term to study the distribution and arrangement of NPs in a taxon, which is a crucial tool in the fields of chemosystematics and chemotaxonomy of plants.16 It enables the identification of biomarker distribution among species, which is invaluable, particularly for a genus such as Ocotea, in which morphological variability impacts species delimitation.15,17
In this context, most studies concerning Ocotea plants mainly focused on alkaloids,11,18,19 and lignoids,20–23 as this genus is recognized as a great producer of these NP classes.15,24 However, flavonoids were often underexploited, despite their renowned and extensive range of biological activities and health benefits. As part of the polyphenol family, flavonoids are characterized by their distinct 15-carbon skeleton, consisting of two phenyl rings (A and B) and a heterocyclic ring (C). This basic structure allows for the generation of a variety of subclasses, including flavones, flavonols, flavanones, flavan-3-ols, anthocyanidins, and isoflavones, each differing in the level of hydroxylation and other substitutions in their respective aromatic rings (Fig. 1a).25,26 In the context of glycosylated flavonoids, the presence of sugar moieties attached to the flavonoid backbone structure (Fig. 1b) often enhances their solubility, stability, and bioavailability, while still significantly altering their biological activities. However, the glycosylation in different backbone flavonoid positions also adds complexity to the profiling analyses and appropriate metabolite identification. The traditional analytical techniques often fall short in sensitivity, specificity, and throughput, underscoring the need for more robust and efficient methodologies.3,27 Modern analytical tools, combined with comprehensive annotation strategies can aid in addressing these drawbacks, also preventing redundant isolation and identification of already known metabolites.28,29
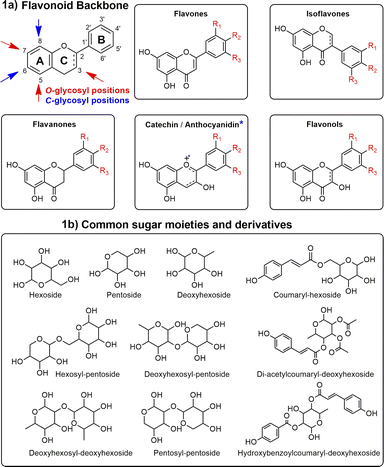 |
| Fig. 1 Main glycosylation types, aglycones, and sugars in flavonoids. This figure illustrates the diverse subclasses of flavonoids (1a) and the structural variations due to glycosylation (1b), highlighting the complexity and variety within the glycosylated flavonoid family. | |
Liquid chromatography coupled with mass spectrometry (LC-MS)-based untargeted metabolomics represents a modern approach in NP research, with great potential for field advancement by enhancing metabolite coverage.30,31 This approach typically employs data-dependent acquisition (DDA) and data-independent acquisition (DIA) techniques, which are pivotal for acquiring both precursor (MS1) and fragment (MS2) ion data.32,33 DDA selects precursor ions based on their measured MS1 scan abundance, to acquire their corresponding MS2 spectra.34 In contrast, DIA indiscriminately fragments all detectable precursor ions within a larger mass range.35 Although DIA's methods, spectral libraries, and software are better established in the proteomics field,36 DIA development and applications in metabolomics are starting to rise.37–39 The classic DIA methods are based on alternation between low and high-energy channels, to acquire both MS1 and MS2 scans, respectively.40,41 The terminology varies across MS platforms. For example, in Waters quadrupole-time of flight (QTOF) instruments it is referred to as MSE, while in Thermo Fisher Orbitrap™ instruments it is termed all-ion fragmentation (AIF).41
Modern mass spectrometers have been able to perform sophisticated DIA experiments with varied defined isolation windows in sequence. The data generated for them has fewer interferences from co-eluting species and it is, consequently, easier for the deconvolution step. The sequential window acquisition of all theoretical mass spectra (SWATH™), and the SONAR™, in AB SCIEX and Waters QTOF instruments, respectively, are examples of these modern DIA experiments.42,43 Thermo Orbitrap™ instruments includes the variable data-independent acquisition (vDIA),44 and most recently, the narrow-window data-independent acquisition (nDIA) in Orbitrap™ with the asymmetric track lossless (Astral™) analyzer.45
In addition, ion mobility spectrometry (IMS) is an ancillary technique to high-resolution mass spectrometry (HRMS) that has been demonstrated to improve the quality of DIA data and has been implemented in NP research.41,46 This technique is based on gas-phase electrophoretic separation of ions based on their size and conformations, resulting in an additional dimension to retention time and mass-to-charge ratio (m/z): the collision cross-section.47
DDA is considered to exhibit superior MS2 spectral quality, however often fails to cover low-abundance metabolites, leaving a significant number of metabolic features without MS2 spectra for metabolite annotation.39,41,48 This limitation is primarily due to the time allocation for each scan type during the acquisition process. In such top n DDA methodologies, approximately 95% of the instrument's time is devoted to MS2 acquisition. Conversely, in AIF or MSE/DIA methods, there is an approximately equal distribution of time between MS1 and MS2 scans.35 This balanced approach results in superior coverage of both metabolite and fragment spectra. Consequently, AIF or MSE/DIA generates much more complex spectral data, providing a more comprehensive profile of metabolites.49,50
Our group recently developed an integrative workflow for processing MSE/DIA data and conducting molecular networking. This workflow was applied to a dataset of 60 Ocotea plant species, resulting in the annotation of several NP's.12 Although advancements in automated data handling in metabolomics, manual inspection and critical analysis of LC-MS data remain an indispensable step for achieving reliable results.51,52 Herein, we present an innovative DIA-based strategy for straightforward flavonoid profiling of metabolomics plant datasets. It is a user-friendly way to manually explore the full DIA data. Once most previous studies focus on MS1 data for analysis, and MS2 just for further compound annotation, our approach uses the MS2 data directly to analyze the flavonoid aglycone distribution throughout the samples.
With this strategy, we could annotate a higher number of flavonoids compared to the same species included in the previous work.12 The strategy for flavonoid profiling employs the new MZmine 3 version software, one of the most popular for MS data processing and downstream analysis. As an applicability case, leaf extracts of six plant species of the Ocotea genus (O. diospyrifolia, O. guianensis, O. lancifolia, O. notata, O. odorifera, and O. porosa), already known for flavonoid content in literature, were analyzed in an LC-MS system with a high-resolution QTOF analyzer in MSE/DIA configuration. This dataset not only demonstrated the versatility of the LC-MS/DIA strategy but also highlighted its robustness in profiling a diverse range of glycosylated flavonoids from the Ocotea genus.
Despite the acknowledged importance of Ocotea spp. as a source of bioactive compounds, comprehensive metabolomic studies focusing on flavonoids in this genus are scarce, involving few studies related to metabolic profiling,12,53 extraction procedures optimization,54 biological activity,55–57 and chemophenetic analysis.58 Classical phytochemistry approaches showed interesting biological activities of Ocotea sp. flavonoid fractions, as antioxidant and antibacterial,59 antiherpertic,60 fungicide,61 and antimycobacterial.62 However, these approaches generally allow characterization of the isolated majoritarian compounds, resulting in an incomplete overview of the flavonoid content. Our research addresses this gap by employing an analytical framework that combines the high resolution and sensitivity of LC-MS with the coverage of DIA.
The primary goal of this study, beyond the relevance of chemical characterization of the Ocotea species, and corroboration for future chemosystematic studies within the genus, is to underscore the utility and effectiveness of the LC-MS/DIA method in NP research, particularly in accelerating and enhancing the confidence and coverage of targeted glycosylated flavonoid profiling. We introduce a strategy option for manually exploring MS2 DIA data (detailed in the video), addressing the need for more robust methods in data analysis, as DIA gains space in NP metabolomics studies.
2 Experimental
2.1 Chemicals
LC-MS acetonitrile and formic acid were purchased from Sigma Aldrich (St Louis, MO, USA). Water was obtained using an 18 MΩ cm Millipore Milli-Q™ water purification system (Millipore, Bedford, MA, USA). High-performance liquid chromatography (HPLC) grade solvents; including hexane, methanol, and ethanol, were acquired from Sigma Aldrich (St Louis, MO, USA).
2.2 Sample collection
For the ultra-performance liquid chromatography (UPLC) coupled to HRMS/DIA data analyses, a small amount of plant material (1–3 leaves) from O. diospyrifolia (Meisn.) Mez, O. guianensis Aubl., O. lancifolia (Schott) Mez, O. notata (Nees & Mart.) Mez, O. odorifera Vell. Rohwer, and O. porosa (Nees & Mart.) Barroso were provided by the Brazilian Herbarium Leopoldo Krieger (CESJ, Federal University of Juiz de Fora – UFJF, Minas Gerais). The plants were deposited with the voucher specimens #CESJ 62011, #CESJ 44460, #CESJ 45567, #CESJ 62057, #CESJ 31144, and #CESJ 51738, respectively, and they can be consulted at https://specieslink.net/col/CESJ/. The study of these plants was registered on the National System for Governance of Genetic Heritage and Associated Traditional Knowledge under the registration number #A5A8F67, and can be consulted at https://sisgen.gov.br/paginas/pubpesqatividade.aspx.
2.3 Sample preparation
The plant material was ground using pistil and liquid nitrogen. In 2 mL Eppendorf tubes, 20 mg of the ground material was extracted with 2 mL of ethanol
:
Milli-Q™ water solution in a 7
:
3 (V/V) ratio with the aid of an ultrasound bath for 15 minutes at 35 °C (170 W, 50 kHz, L100 Schuster, China). The extracts were then centrifuged at 22 °C and 112 rcf (G-force) to collect the supernatant. The samples were subjected to clean-up by partitioning with HPLC-grade hexane (2 × 200 μL) to remove non-polar substances, followed by filtration through polytetrafluoroethylene (PTFE) syringe filters (pore size 0.22 μm), and dried using an Eppendorf Speed-Vac Concentrator Plus 5305 (Hamburg, DEU) for 3 h at 40 °C. The samples were prepared at a concentration of 1 mg mL−1 in water
:
acetonitrile, 1
:
1, (V/V), and kept in a freezer (−20 °C) until analyses.63
2.4 UPLC-HRMS/DIA data acquisition
Data acquisition was performed using a UPLC system coupled to an electrospray (ESI) source and a Xevo™ G2-XS QTOF instrument (Waters Corp., Milford, MA, USA). Quality control measures of pooled samples, injection order, and solvent blanks were done as reported on our previous publication with several other Ocotea species.12 Chromatographic separation was achieved using a C18 column (100 × 2.1 mm, 1.8 μm particle size diameter; ACQUITY UPLC™ HSS T3), and a mobile phase gradient at a flow rate of 0.5 mL min−1, composed of acetonitrile (B) and water (A). Both phases are acidified with 0.1% of formic acid. The chromatographic gradient began with an initial composition of 1% of B, followed by a transition to 15% of B in 0.1 min. Further changes in solvent composition occurred at 7.5 min (80% of B), 8.5 min (99% of B), and 8.6 min (1% of B), which was held until 10 min. The total runtime was 10 min, with an injected sample volume of 5 μL. Oven and shelf temperatures were set at 40 °C and 10 °C, respectively. The mass spectrometer was operated in MSE acquisition mode with alternating high and low-energy scans, for positive and negative ionization modes. The ionization energy was set at 3 eV for low-energy scans and 25–40 eV for high-energy scans. ESI-MS at a resolution up to 40
000 with a full mass scan range set from 50 to 1000 m/z for functions 1 and 2 was applied. Instrument parameters, including cone voltage (40 V), capillary voltage (3.0 kV), cone gas flow (30 L h−1), auxiliary gas flow rate (10 L h−1), desolvation temperature (300 °C), source temperature (120 °C), and desolvation gas flow (600 L h−1), were optimized. High-purity nitrogen was employed for desolvation, collision, and cone gas. To ensure accuracy and reproducibility, a solution of leucine-encephalin was used as a lock mass with m/z 554.2622 (ESI−) and m/z 556.2768 (ESI+) for identification. MS data were continuously collected, and lock spray calibration was performed every 10 seconds.
2.5 Data processing
The obtained data in .raw format were converted to the standard format .mzML using the Waters2mzML 1.2.0, available on GitHub (https://github.com/AnP311/Waters2mzML).
Converted .mzML data were imported into MZmine v. 3.9.0 for data processing (mass detection, chromatogram building, deconvolution, isotope elimination, alignment, and gap filling). The following steps and main parameters were performed for MS1 level processing: mass detection (Mass detector: Centroid, noise level: 3 × 102), chromatogram builder using ADAP algorithm (Filters: MS level filter, MS1; minimum consecutive scans, 5; minimum intensity for consecutive scans, 8 × 102; minimum absolute height, 8 × 103; m/z tolerance, 0.005 m/z or 10 ppm), deconvolution using the local minimum resolver algorithm (dimension: retention time; chromatographic threshold, 0.85; minimum search range for retention time, 0.035; minimum absolute height, 8 × 103; minimum peak top/edge ratio, 1.8; peak duration range, 0–2 min; minimum scans, 5), isotope elimination using the 13C isotope filter (m/z tolerance, 0.003 m/z or 5 ppm; retention time tolerance, 0.05 min; monotonic shape, yes; maximum charge, 1; representative isotope, most intense). Alignment using the Join aligner algorithm (m/z tolerance, 0.007 m/z or 12 ppm; m/z weight, 3; retention time tolerance, 0.045 min; retention time weight, 2). Additionally, gap filling (intensity tolerance, 0.2; m/z tolerance, 0.005 m/z or 7 ppm; retention time tolerance, 0.04 min; minimum scans, 4) was performed to obtain the final feature lists (m/z, retention time, and intensity). Subsequently, for MS2 processing, the same steps and parameters were performed using the MS level filter set to MS2 in the ADAP chromatogram builder step, and adjusting the minimum absolute height to 4 × 103 in this step and also in resolving. Parameters (ESI 1 and 2†) and batch mode files from MZmine (ESI 3†) are available as ESI† at Zenodo's link described in data availability section; https://doi.org/10.5281/zenodo.10810967.
2.6 Databases construction
For compliance with this DIA strategy for flavonoid profiling, it is crucial to curate chemical databases for the subsequent automated annotation step. Thus, custom in-house databases, named Ocotea_flavDB (MS1), Br_flavDB (MS1), and FlavAglyDB (MS2) (available to download at ESI 4–6† at Zenodo's link https://doi.org/10.5281/zenodo.10810967) were previously prepared using SMILES as chemical structural data. To automate and speed up database construction, an .mol2 file based workflow was built on the Konstanz Information Miner (KNIME) platform (https://www.knime.com/) version 4.6.5 (University of Konstanz, Zurich, CHE). The workflow (ESI 7 – Fig. S1†) is online and available to download and utilize (https://hub.knime.com/-/spaces/-/%7EffDFj4alnx3YUYUt/).
Ocotea_flavDB consisted of 54 carefully curated flavonoid chemical structures previously isolated from plant species of the Ocotea genus. For that, we have utilized original published NP research articles in literature, and the Nuclei of Bioassays, Ecophysiology and Biosynthesis of Natural Products Database (NuBBEDB) (https://nubbe.iq.unesp.br/portal/nubbe-search.html) online database. NuBBEDB currently stores more than 2200 NPs from Brazilian plant species and allows users to download and extract useful data information, which has been manually curated to ensure reliability. Thus, the manual construction of specific plant species, genera, and family databases is more feasible and trustworthy.64,65
In addition, we have also constructed the Br_flavDB, encompassing 288 flavonoids isolated from Brazilian plant species, which are currently available on NuBBEDB (accessed January of 2024). These are MS1 databases and consist of comma-separated values (.csv) files containing the columns of the precursor flavonoid name, the high-resolution neutral mass, the molecular formula, the retention time (equal to the total time of the chromatographic method), and other information.
Another database, the MS2 database FlavAglyDB which also was built, comprised aglycone fragments of the main well-known flavonoids: kaempferol and isomers (datiscetin and luteolin), quercetin, catechin/epicatechin, apigenin, taxifolin, narigenin, and myricetin. These flavonoids were selected based on previous Ocotea biosynthetic chemical knowledge and can be adapted to any flavonoid aglycone, following the general fragments detailed in the ESI (ESI 8 at Zenodo's link https://doi.org/10.5281/zenodo.10810967).† The fragments were proposed based on the main gas-phase cleavage patterns of O- and C-glycosylated flavonoids in the literature.66–69 For the first type, heterolytic and homolytic cleavages were considered. For C-glycoconjugates, heterolytic cleavage resulting in modified aglycones with –C3H6O2 and –C2H3O residues derived from sugar moiety were proposed. Although some patterns occur in flavonoid substitution (e.g. flavonols O-substituted, and flavones C-substituted), we propose O- and C- cleavage types to all flavonoids of FlavAglyDB. These fragmentation reactions are shown in our results and discussion section. The MS2 database also consists of a .csv file, containing the columns of the aglycone fragments, the m/z, and the retention time (equal to the total time of the chromatographic method). In the case of FlavAglyDB, the m/z values are referred t to the [M − H]− ions.
2.7 Flavonoid profiling
For the annotation step, the local compound database search module of MZmine 3 was employed for both MS1 and MS2 feature lists. Only data acquired in the negative ionization mode was used for glycosylated flavonoid profiling. Whereas, positive mode data was explored for manual complementary aglycone analysis. The following parameters were set for both custom in-house DBs: m/z tolerance at 0.005 m/z or 5 ppm; retention time tolerance, equal to the total time of the chromatographic method (10 minutes); use of adducts (only for MS1 databases), MS mode negative, maximum charge equal 2, maximum molecules/cluster equal 2, and [M − H]−, [M + Cl]−, [M + Br]− and [M + FA]− adducts. The last option was set only for the MS1 databases because they contain the monoisotopic neutral masses of the compounds. Very important to point out, that for this MZmine module to be functional, the exact name of each column of the .csv database file has to be indicated on the parameter setting (see SV1†).
By these means, annotation was initially conducted by matching the monoisotopic mass with the entries of the databases, achieving the flavonoid candidates on MS1-level spectra, and aglycones annotation on MS2-level spectra. The intense annotated aglycones (MS2 features) without correspondent MS1 flavonoid annotation, were further manually inspected for their corresponding MS1 feature data, to search for neutral losses. This involved manually searching for precursor ions that matched in retention time and exhibited similar peak shapes. This method of scan-level correlation significantly streamlined the flavonoid annotation process.
Additionally, the FlavonoidSearch tool v1.2.0 (https://sakura-kagaku.com/komics/software/FlavonoidSearch/) was employed for isomeric distinctions between flavonols such as kaempferol, datiscetin, and luteolin. This tool encompasses a comprehensive database of probable mass fragments for known flavonoids and a computational tool for database searching. This ensures enhanced accuracy and a deeper understanding of flavonoid diversity. FlavonoidSearch operates by using mass spectra of metabolite peaks in positive ionization mode as queries, thus facilitating the automatic identification of flavonoids.70
2.8 Step-by-step of the strategy
For the application of the proposed strategy, MZmine 3 is applied for comprehensive data visualization, interpretation, and independent processing of MS1 and MS2 data, which can be replicated and applied for different data processing settings. This methodology, detailed here, is adaptable for any MSE or AIF LC-MS analysis, although herein was specifically for targeted analysis of glycosylated flavonoids. The following steps are shown in the protocol video attachment (Supplementary video 1†).
Step 1 starts with the generation of independent base peak chromatograms for both MS1 and MS2 levels.
Step 2 consists of a chromatogram comparison. Manually comparing MS1 and MS2 chromatograms allows researchers to directly compare high-intensity precursors with their fragments.
Step 3 is the overview of blank data, to identify non-significant peaks. Steps 1–3 are based on raw data and are depicted in the supplemental video.† This process is important for data first view and to further ensure accuracy for peak annotation.
Step 4 is the generation of feature lists. Following the initial data processing and parameters set up, which in our case is outlined in Section 2.5. Steps for feature list generation (mass detection, chromatogram building, resolving, alignment, gap filling, and annotation) can be done individually or in a batch. A batch mode file, including steps for feature list generation, is ready to adjust the parameters according to your sample acquisition and available on ESI (Supplementary video 1).† Two types of aligned feature lists are created: one for precursors (MS1) and another for fragments (MS2). These lists were refined by incorporating candidate flavonoids and aglycone hits from our in-house databases, corresponding to Sections 2.6 and 2.7.
Step 5 consists of the manual inspection of these features. It is conducted by feature lists and raw data analysis, focusing on retention time and peak shapes to ensure that fragments and their precursors exhibited co-elution with similar detection ratios. For instance, when examining fragment features at a retention time associated with a candidate precursor, it is anticipated that, for most cases, the MS2 features would display lower intensity than the MS1 precursor and share similar peak shapes.
Biosynthetic investigation. That is not a mandatory step and although it suits the hits. It can be performed to ensure confidence in level 3 annotations as per the Metabolomics Standards Initiative (MSI).71 To this, the Kyoto Encyclopedia of Genes and Genomes (KEGG) is a suitable online tool that can aid in mapping the flavonoid metabolic pathways.72
Critical structural analysis. The proposed structures from level 3 annotations guide the development of fragmentation hypotheses, aiding in the identification of candidate fragments. This approach allows researchers to explore and hypothesize about the structural components of NPs in respective studies. The strategy also enables the visualization of the distribution of the potential diagnostic ions of aglycone patterns observed across different samples in the dataset. Particularly, the manual search for diagnostic fragments is a feature that can be crucial in studies focused on complex NP profiling. In addition, new tools can be also integrated, such as the FlavonoidSearch, which employs structure- and fragmentation-related rules for flavonoid annotation, Section 2.7.
2.9 Further data analysis, figures, and graphs
Data analysis and visualization were also conducted using Python libraries. Pandas was utilized for data cleaning and manipulation. NumPy was used to support numerical calculations. Seaborn to perform and illustrate heatmap correlations, and Matplotlib for the creation of the graphs, including Venn diagrams using the Matplotlib-Venn extension. The Python libraries were accessed using the Google Colab online cloud service (https://colab.research.google.com/). All graphs were exported in .png format at 500 dpi resolution. Chemical structures were manipulated using the ChemDraw Ultra software version 12.0 (PerkinElmer Inc., Waltham, MA, USA). The high-resolution Figures were designed and processed using the open GIMP software version 2.10.34 (https://www.gimp.org/). The video was edited in CapCut applicative (https://www.capcut.com/).
3 Results and discussion
Data processing is one of the hardest and most important steps to achieve a reliable annotation process. However, there is a significant challenge in DIA data processing, in particular, the deconvolution of MS2 spectra and the reassociation of precursor ions with their corresponding fragments.49,73 While vendor-specific software typically offers robust algorithms for DIA processing, e.g. UNIFI from Waters, the contribution of Tsugawa et al. is particularly noteworthy. They developed MS-DIAL software, an open-source alternative that has enriched the field with the MS2Dec algorithm, adept at successfully deconvoluting the DIA data algorithm.73 The advent of MS-DIAL marked the beginning of a new era of open DIA tools and software development in the metabolomics field.74–76 Examples of recent software for DIA data processing are DecoID and MetaboAnnotatoR.77,78 Besides, MZmine 3,79 the most popular open tool for MS data processing, despite a major development in the implementation of sophisticated tools, currently still lacks robust automated modules for LC-MS/DIA data processing and export.
More recent alternatives to explore DIA data potential are based on the integration of DDA data acquired from the same samples, named DIAMetAnalyser,38 and data-dependent-assisted data-independent acquisition (DaDIA),80 as well as the combination of different collision energies in a single analytical run.48 In addition, constructing in-house MS2 spectral databases from chemical compounds and integrating them into data processing are also effective strategies for refining DIA analysis.81,82 However, these strategies depend on the availability of LC-MS systems and authentic chemical standards. Additionally, for the context of the above-mentioned DaDIA integrated strategies, for most DIA data available in public repositories, e.g. Metabolights, MassIVE, and MetabolomeXchange, the appropriate correspondent DDA data is not always available. Under these circumstances, for metabolomic studies, the classical approach to MS-DIAL or vendor software is still sought. Alternatively, as our results demonstrate, MZmine 3 can provide efficient manual handling and inspection of DIA data, as the current version offers optimal data visualization modules.79 Yet, the challenges in MZmine 3 remain in accurately relinking fragments to their respective precursors, crucial for downstream analysis and data export to platforms such as the Global Natural Product Social Molecular Networking (GNPS).83–85
Thus, to enhance our analytical capabilities, the primary aim was to refine the processing and management of MS2 data. This advancement facilitates the streamlined manual annotation of flavonoids by enabling the direct mapping of chromatogram points associated with the aglycones of interest. Although MS-DIAL automates data processing, reconstructing MS2 spectra and linking them to their MS1 counterparts, it falls short in offering a holistic view of fragment data due to its inability to generate comprehensive feature lists. In contrast, MZmine 3 stands out for its versatility, supporting the incorporation of MS2 filters and allowing for data processing similar to conventional MS1 methodologies. This approach, seemingly unexplored in the scientific community, holds significant potential for advancing analytical capacity for enhanced flavonoid profiling.
In this context, the use of LC-MS/DIA, coupled with the advanced features of MZmine 3, has enabled a comprehensive and detailed exploration of our datasets. This DIA-based approach has uncovered a broad spectrum of glycosylated flavonoid compounds in the Ocotea species, providing crucial information regarding the presence of different aglycone and glycan fragments.3,86 The software stands out for its effective data processing, intuitive and integrative learning curve about processing steps, and particularly for its user-friendly and advanced visualization capabilities. These features render MZmine 3 a valuable tool in metabolomics, offering robust solutions for navigating through LC-MS data complexities. Critical to our methodology were the meticulous phases of manual inspection and analysis. They were not just vital for accuracy, but also for ensuring the reliability of our metabolite annotations. The sophisticated combination of these methods was key in diving deep into the complex layers of these intricate NP extracts. In this way, our approach effectively showcased the immense potential of DIA in uncovering the hidden chemical diversity within the Ocotea genus. This includes annotating high and low-abundance flavonoids and often potentially non-reported ones. The results of our investigation strongly advocate for the merits of integrated automated and manual methodological approaches.
The reported hits in this study are based on the feature monoisotopic mass matching with the respective in-house databases. The generated MS1 and MS2 chromatograms (ESI 7 – Fig. S2–S13†) displayed distinct peaks at the retention time range of 1.5–6 min, indicating the presence of glycosylated flavonoids at MS1 and their respective potential fragmented aglycones at MS2 spectra. By applying the proposed strategy for DIA data processing, this can be further evidenced through overlaying MS1 and MS2 chromatograms (Fig. 2). That made it possible to examine continuously each one of the chromatograms regarding the distributions of these flavonoid ions, thus, highlighting several high-intensity precursors corresponding to fragment ions with similar peak shapes at the same retention times. The possibility of MS2 data processing and continuous visualisation is a particularity of AIF or MSE/DIA data, where the same instrument time is spent to acquire both MS1 and MS2 data.35 In the MZmine 3 workflow, the algorithms are usually applied to MS1 processing to further attribute MS2 spectra to processed features (precursors).79 By applying these same algorithms to the DIA-MS2 level spectra, we have achieved an MS2 feature table, which is a dataset of all fragments represented by resolved features. We could analyze the fragments from a holistic point of view, as they were uninterruptedly distributed over the DIA data in the same way that the precursors were.
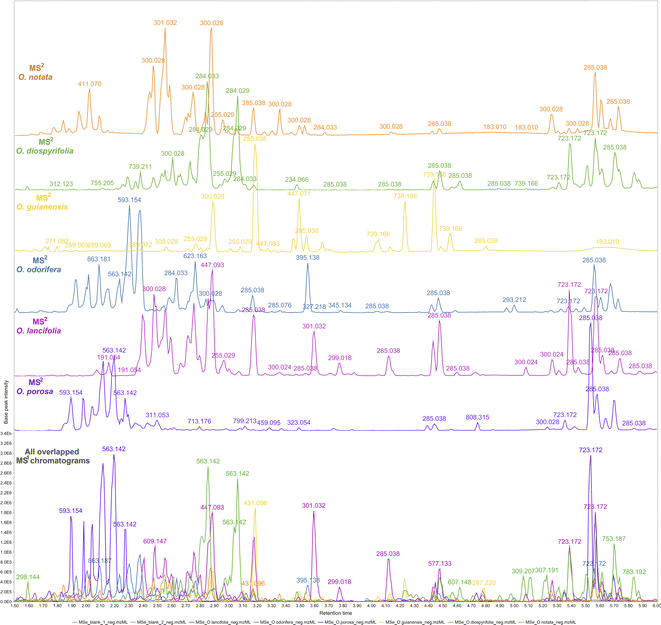 |
| Fig. 2 Overlapped and aligned LC-HRMS/DIA (MS1 and MS2) base peak intensity (BPI) chromatograms of Ocotea spp. leaf extracts in negative ionization mode in a 1.5–6.0 min retention time range. | |
The MS1 processing resulted in 1603 aligned features, with 189 hits from Ocotea_flavDB. According to the MSI, they represent annotations at level 3 of confidence.71 These annotations, even though level 3, gained reliability once they originated from a specific database containing related-to-genus NPs, thus accounting only for flavonoids previously isolated in Ocotea spp. Considering biosynthetic aspects, where specific genes and consequently enzymes are shared among families or genera,26 this strategy is useful to obtain more realistic annotations for the studied species, avoiding meaningless annotations.52
In addition, we achieved 193 hits from Br_flavDB, which also contributed to level 3 confidence annotations. These hits include several known flavonoids not previously reported in Ocotea spp., thus expanding the scope of flavonoid profiling as they originate from plant species present in the Brazilian flora. The details of each of those annotations are shown in the ESI (ESI 7 – Tables S1 and S2).†
The MS2 processing aligned all Ocotea samples, resulting in 2021 features with 216 hits from FlavAglyDB. These features represent the fragment chromatogram peaks extracted from the continuous data, as we have done for the MS1 features. This approach is possible due to the versatility of MZmine 3 filters to step-by-step processing, where it is possible to perform the common mass detection, chromatogram building, resolving (deconvolution), deisotoping, and alignment steps also to the MS2 data. This allowed us to explore all information about the fragments distributed over the chromatograms. Such an approach facilitates the manual annotation of the processing features, as it gives a clear idea of the most intense fragments at the same retention time. Furthermore, some fragments are considered diagnostic ions of certain compound classes, and thus their presence can reveal important information about the respective chemical structure.87,88 For example, in the case of flavonoids, aglycone fragments directly indicate the NP class, and ring substitution patterns, which can aid in discriminating among possible isomers and subclasses.89,90 This demonstrates the effectiveness of MZmine 3 versatile filters in step-by-step processing, facilitating manual annotation of features and revealing crucial information for targeted profiling. On the other hand, the MS2 hits, related to the putative identified aglycones, provide an upfront view of the flavonoid main backbone distribution among these species (Fig. 3). All this information can be crucial for identifying dominant flavonoid subclasses and understanding related biosynthetic pathways.
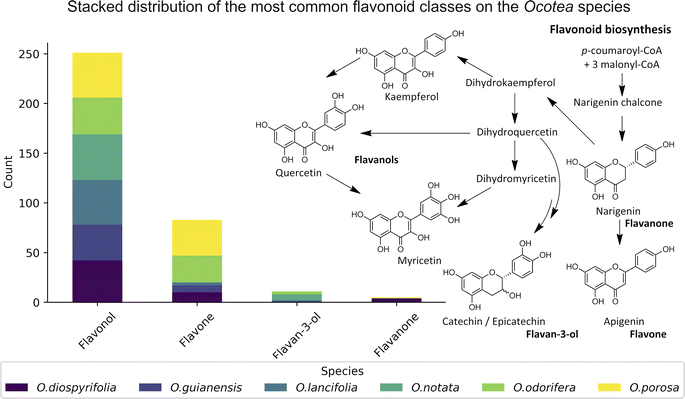 |
| Fig. 3 Stack bar graphs of flavonoid types. This figure displays the distribution of main flavonoid backbones among different Ocotea species, as annotated by MS2 processing. The stacked bars represent the various flavonoid subclasses and their respective biosynthetic pathways on the right side. | |
Additionally, we navigated through the complex isomeric landscape of kaempferol, datiscetin, and luteolin, which were all present in our in-house databases. Leveraging the advanced capabilities of the FlavonoidSearch tool, which utilizes the Jaccard index for spectra similarity scoring, thus focusing on fragmentation patterns comparison.70 This strategic approach, utilizing data garnered from positive ionization, was pivotal in inspecting distinct features at m/z 287.0550 across all Ocotea species under examination. This method allowed refined discrimination, enabling us to conclusively ascertain the presence of either kaempferol or datiscetin over luteolin, guided by the most compelling scores (ESI 7 – Fig. S14†). Further delving into the flavonoid profile, our decision to annotate kaempferol was informed by its prevalent role as a fundamental flavonoid backbone within the Ocotea genus, standing alongside other significant flavonoids that include the quercetin and the catechin/epicatechin.15 Therefore, the proposed strategy enables a comprehensive search for the aglycone fragments and could be complemented with other currently available tools.
Thus, in the MS2 feature table, which was acquired in the negative mode, the presence of several glycosylated derivatives of kaempferol (286.0477 Da) and quercetin (302.0427 Da) were evidenced by the m/z values 285.0386 (−6.67 ppm) and 301.0337 (−5.65 ppm), along with the 284.0321 (−1.76 ppm) and 300.0272 (−1.33 ppm), corresponding respectively to the heterolytic and homolytic cleavage of O-glycosylated flavonoids.91 As per their structure, O-flavonoid glycosides are capable of undergoing both types of cleavage, a phenomenon well-established in ESI ionization tandem MS for this class of NPs.67,92,93 Additionally, apigenin C-glycosides were identified by the modified aglycones, exemplified by diagnostic ions at m/z's 311.0555 (−1.93 ppm), 341.0661 (−1.76 ppm), 353.0673 (1.69 ppm) and 383.0777 (1.04 ppm).68,69 Then, by subtracting the aglycone m/z value from the respective MS1 feature, with the same retention time and peak shape, it was possible to establish the neutral loss related to the aglycone moiety of each hit compound. The gas-phase fragmentation pathways involved in glycoside cleavage were proposed for the main O- and C-type glycosides observed (Fig. 4).
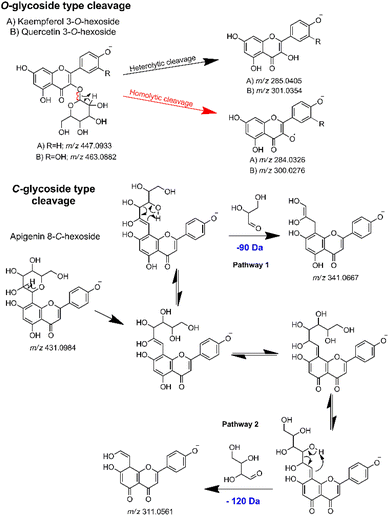 |
| Fig. 4 Key gas-phase fragmentation pathways for O- and C-type glycosides, highlighting the main glycoside cleavages. | |
Detailed information regarding the aglycones and sugars derived from heterolytic and homolytic O-cleavage are tabulated in the ESI (ESI 7 – Table S3; and ESI 8 at Zenodo's link https://doi.org/10.5281/zenodo.10810967).† The complete MS2 aglycone annotation lists are also included in the ESI (ESI 7 – Table S4).† Furthermore, determining the specific site at which sugar attaches to a flavonoid backbone without relying on highly controlled experiments and authentic standards, is not a simple task. Therefore, we have adopted a more reliable approach to annotate and report these NPs (Table 1).
Table 1 Representative examples of Ocotea flavonoids in LC-MS/DIA conducted in negative ionization mode, based on our custom in-house databases
RT (min) |
MS1 m/z |
MS1 precursor's annotation |
Error (ppm) |
MS2 m/z |
MS2 aglycone's annotation |
Error (ppm) |
Neutral loss (Da) |
Candidate bond type and sugar derivative moiety |
2.18 |
563.1420 |
Schaftoside or isoschaftoside (apigenin di-C-glycosides) |
2.43 |
353.0673 |
Apigenin-di-etenol [M − H]− |
1.76 |
210.075 (90 Da + 120 Da) |
Di-C-hexoside or C-hexoside-C-pentoside |
2.55 |
463.0869 |
Hyperin (quercetin 3-O-D-galactoside); isoquercitrin (quercetin 3-O-D-glucoside); quercimeritrin (quercetin 7-O-D-glucoside); 7-methoxyquercetin-3-O-xylopyranose; Quercetin-3-O-allopyranoside; myricetrin (myricetin 3-O-rhamnoside) |
−2.81 |
301.0319 |
Quercetin [M − H]− |
−1.17 |
162.055 |
O-Hexose |
2.75 |
433.0764 |
Reynoutrin (quercetin 3-O-xyloside); guajaverin (quercetin-3-O-arabinopyranoside) |
−2.77 |
300.0266 |
Quercetin [M − H]˙− |
−3.17 |
133.049 |
O-Pentose |
2.85 |
563.1426 |
Schaftoside or isoschaftoside (apigenin di-C-glycosides) |
3.50 |
284.0321 |
Kaempferol [M − H]˙− |
−1.90 |
279.111 |
O-Deoxyhexosyl-pentose |
2.87 |
447.0927 |
Astragalin (kaempferol 3-O-glucoside); quercitrin (quercetin 3-O-rhamnoside); orientin (luteolin 8-C-glucoside) or isoorientin (luteolin 6-C-glucoside) |
−1.34 |
300.0272 |
Quercetin [M − H]˙− |
−1.17 |
147.066 |
O-Deoxyhexose |
4.43 |
577.1346 |
Procyanidin B1; procyanidin B3; proanthocyanidin; kaempferol-3-O-(4′′-p-coumaroyl)-rhamnoside |
−0.87 |
285.0383 |
Kaempferol [M − H]− |
−7.58 |
292.097 |
O-Coumaroyl-deoxyhexose |
5.57 |
723.1727 |
Kaempferol 3-(2′′,4′′-di-p-coumaroylrhamnoside) |
1.11 |
285.0383 |
Kaempferol [M − H]− |
−7.58 |
438.134 |
O-Di-coumaroyl-deoxyhexose |
The reporting of glycosylated flavonoid compounds, as detailed in Table 1, is arguably more precise, particularly given the complexities associated with predicting sugar attachment sites. Under highly standardized and outlined conditions, and using mass spectrometry fragmentation rules, intensity ratios of flavonoid fragments can indicate the substitution position.3 However, simply automated spectral similarity approaches, often used in literature for annotating glycosylated flavonoids and specifying both the sugar type and its position (e.g. Astragalin = Kaempferol 3-O-β-D-glucose), may not always be accurate. Factors like collision type (e.g. CID, collision-induced dissociation, and HCD, higher energy collisional dissociation) and variations in collision energies can significantly influence the fragment distribution within MS2 spectra, potentially leading to misinterpretations.94,95
Given that public MS2 repositories, such as GNPS, pursue a large diversity of spectra acquired in different instruments under different conditions, it is crucial to manually inspect the automated hits concerning the instrument and collision energy. For instance, we compared MS2 spectra of astragalin from GNPS libraries, acquired under different conditions (two spectra acquired using an QTOF analyzer in negative mode, and two acquired using an Orbitrap analyzer in positive mode) (ESI 7 – Fig. S15†). Despite not specifying the collision energies of each data, the results showed quantitative and qualitative variance among the fragments. The qualitative variance was especially for Orbitrap MS data, demonstrating the potential for misinterpretation in the automated annotation of glycosylated flavonoids, once the sugar moiety position cannot be precisely assured based only on gas phase fragmentation reactions.
Furthermore, the lack of specified collision energies and standardization across the MS data repositories remains a challenge in metabolite annotation. Considering these findings, we advocate for reporting these NPs by indicating the aglycone, the glycosidic bond type, and the sugar type (e.g., Kaempferol O-hexoside) – information that is readily accessible through standard LC-MS methods. This approach ensures a more reliable and consistent annotation of glycosylated flavonoids, circumventing the uncertainties associated with automated spectral similarity techniques.
The annotation of compounds via MS2 spectral matching is typically classified as level 2 of confidence according to the MSI guidelines, denoting putatively annotated compounds (e.g. without chemical reference standards, based upon physicochemical properties and/or spectral similarity with spectral libraries).71 Herein, our annotations are reported with level 3 of confidence, indicating putatively characterized compound classes, however including precise flavonoid aglycone and sugar types. This is due to matching monoisotopic masses with phylogenetic-related databases could further yield more reliable level 3 annotations, minimizing the risk of unrelated and false positive hits.
In summary, this semi-automated approach for MS data analysis allows detailed DIA-based manual inspection. This was designed to overcome some of the primary barriers in confident metabolite annotation within plant metabolomics studies. This method provides an alternative approach in response to the current limitations, which include for instance the scarcity of sample-related MS2 spectral databases and recurrent annotation of unrelated hits. We underscore the critical role of the analyst's expertise in conjunction with the known semi-automated processes, enabling the extraction of maximal relevant information from the samples under the same analysis. Fully automated and not manually inspected analyses often contain errors that are overlooked in peer reviews.52 Therefore, the dual approach of manual inspection and automated processes ensures a more rigorous and accurate interpretation of MS data.
3.1 Ocotea spp. applicability case: literature comparison
Data on flavonoid profiles in Ocotea species remain scarce in existing literature, with only 3.4% of Ocotea species reported as flavonoid producers in the latest review from 2020.15 Despite that, biosynthesis investigation studies can provide valuable insights into plant evolution, linking botanical diversification to genetic alterations, which are associated with the evolutionary and adaptive processes of the Ocotea. In recognised Ocotea spp., flavonoids predominantly include glycosylated derivatives of catechin, epicatechin, quercetin, and kaempferol, with 45.5% being O-glycosylated. For the six Ocotea spp. present in this study, it was indicated an even larger proportion of the O-glycosylated derivatives of 60.1%. That suggests that these species may belong to a basal stage in Lauraceae's evolutionary lineage. Moreover, according to the literature, it is the genus's flavone-to-flavonol ratio of 0.05 that supports its ancestral role in the Lauraceae, as proposed by previous botanical and phylogenetic studies.15,96 Although not representative of the genus (n = 6), the flavonoid profiling in this research has revealed new aspects of this tendency, where the overall flavone-to-flavonol ratio of the studied species was 0.48 (60 hits from flavone, and 125 hits from flavonol). This ratio was not balanced among the species (O. odorifera = 0.76, O. diospyrifolia = 0.24, O. guianensis = 0.19, O. lancifolia = 0.04, O.notata = 0.0, and O. porosa = 0.80), suggesting that perhaps O. odorifera and O. porosa are not as evolutionarily close as the others in the Lauraceae family, when compared to previous research in the literature.15
The significant presence of flavones, which has led to a higher flavone-to-flavonol ratio, could suggest a more evolved phylogenetic position. This deviation in flavonoid composition across the species points towards a more complex evolutionary pathway within the Ocotea genus and could be indicative of the presence of diverse evolutionary paths. Even though, the less annotated O-alkylated flavonoids in Ocotea spp. in literature, and also in our results, might further support this antiquity proposal of the genus.15 However, as evolutionary analyses become more robust with larger sets of species, the precision of characterization analyses can also improve with advancements in analytical technology. Therefore, as these areas develop, the number of identified Ocotea flavonoid producers is likely to increase, offering a more comprehensive understanding of their evolutionary journey. Still, as an overview, the results suggest that flavonoid profiles might play a role in tracing the evolutionary position of Ocotea spp. within the Lauraceae family.
In this study, we significantly expanded the detection and annotation of glycosylated flavonoids in six Ocotea species, surpassing previous metabolomics and phytochemical counts in the literature. More specifically, only a few flavonoids were reported for these species: O. diospyrifolia (only 2),13 O. guianensis (6),54 O. lancifolia (9),61 O. notata (12),55,60,62 O. odorifera (11),57 and O. porosa (only 3),97 and our study annotated more than 50 flavonoid backbones for each species (ESI 7 – Table S5†). Except for O. notata, apigenin fragment backbones have been identified in the five other Ocotea species. This finding aligns with previous reports of apigenin flavonoids in O. odorifera. However, for the remaining four species, this is the first report identifying the presence of a flavone backbone. Additionally, this study also represents the first report of flavonol kaempferol and quercetin in O. porosa species.
The heatmap correlation analysis showed that O. lancifolia and O. notata have very similar feature profiles, whereas O. porosa and O. guianensis demonstrated to be quite distinct (Fig. 5). In addition, the comparative analysis between Br_flavDB and Ocotea_flavDB hits was concisely visualized through a Venn diagram, revealing the intersection and uniqueness of the feature hits across the datasets. The diagram delineated 49 m/z hits that were exclusive to Br_flavDB, illustrated by the distinct left circle. These hits suggest the presence of new flavonoids in these plants, not yet isolated for species from the Ocotea genus, although they are present in other plant genera from Brazilian flora. Conversely, Ocotea_flavDB exhibited exclusivity in 45 unique m/z hits, as represented by the right circle in the Venn diagram. This supports the fact that manual curation is essential for enhanced in-house database constructions, as Ocotea_flavDB contains online database entries but also highly curated literature data, including all published articles in the literature for the Ocotea genus. In addition, a significant overlap between the two databases hits, with 144 unique m/z is depicted in the intersecting zone (Fig. 6), which was expected to be the largest section, as the online NuBBEDB data is present in both in-house databases.
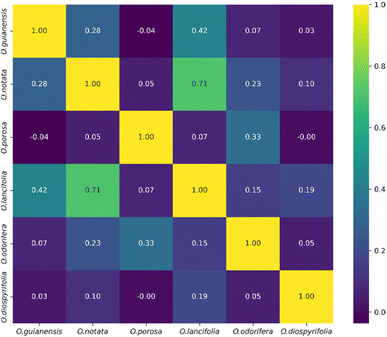 |
| Fig. 5 Heatmap correlation of feature profiles from the 6 Ocotea species. | |
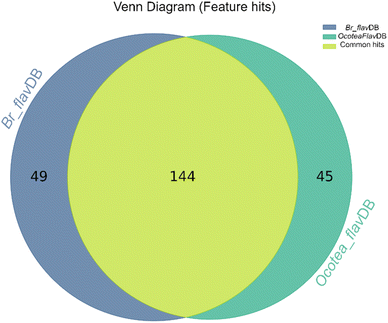 |
| Fig. 6 Venn Diagram of Br_flavDB and Ocotea_flavDB hits. This diagram illustrates the unique and shared feature hits between these two in-house databases. | |
We annotated common flavonoid backbones such as apigenin, kaempferol, and quercetin across most species. Notably, myricetin was exclusive to O. lancifolia, O. notata, and O. odorifera, while narigenin was unique to O. diospyrifolia and O. porosa. We observed that the flavonol backbones, kaempferol, and quercetin, predominantly exhibited O-glycosylation patterns, while apigenin derivatives were found mainly as C-glycosides. Interestingly, myricetin backbones were detected for the first time in these mentioned species. However, we highlight that as myricetin backbone was not annotated in its pure aglycone form (m/z [M − H]− = 317.0303), unlike kaempferol, quercetin, and apigenin. Also, it was only annotated as C-glycoside fragments. This is particularly intriguing because the genus Ocotea is not known in the literature as a producer of myricetin derivatives, in corroboration with our study, which only recorded a few myricetin MS1 hits among the species. In addition, the C-glycoside myricetin derivatives are not widespread in plants. Consequently, while this suggests the possibility of new flavonoids in these species, there is also a potential for false positives. Further in-depth chemical investigation is therefore essential to resolve this issue and potentially confirm the presence of these flavonoids. Regarding the primary flavonoid types: flavone, flavonol, and flavanonol were commonly found across most species. Flavan-3-ol is found in all except O. diospyrifolia and O. porosa. Thus, our comparative analysis (Fig. 7) has been designed to illustrate the distribution of these chemical components across different species, offering more explicit insights into the chemical diversity of backbones and flavonoid types within the Ocotea genus.
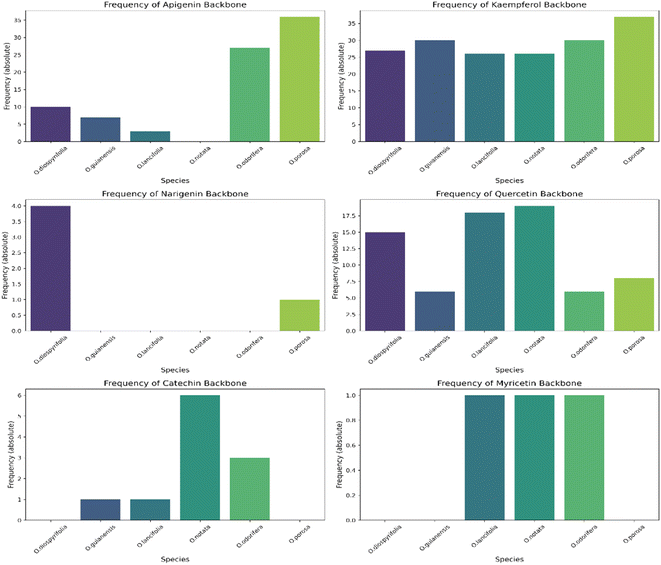 |
| Fig. 7 Distribution of flavonoid types across Ocotea species. This figure presents individual bar graphs showing the distribution of flavonoid types: flavone, flavonol, flavanonol, and flavan-3-ol, among each examined species within the Ocotea genus. The bars represent the absolute frequency distribution of each flavonoid type, illustrating their prevalence or absence among the species. | |
Despite the high complexity of LC-HRMS/DIA data, our strategy enabled a comprehensive yet straightforward analysis of Ocotea plant extracts, bypassing exhaustive isolation and characterization processes. DIA-MS, though less commonly used than DDA, is highly valuable for metabolomics studies, particularly for annotation-based metabolic profiling due to its extensive metabolite and fragment coverage.35,39,48 Our results were revealing, particularly in discerning the distinct aglycone patterns across different plant species, further enhanced by analyses of gas-phase fragmentation reactions. Moreover, with the development of specialized in-house databases, and the use of advanced computational tools, we have achieved reliable annotation and comprehensive flavonoid profiling. These tools can predict possible glycosylation patterns, aiding in the interpretation of MS flavonoid data. In summary, while traditional techniques face challenges in the analysis of glycosylated flavonoids, ongoing advancements in analytical methods and computational tools are continually improving the detection and annotation of these complex and widespread molecules in complex NP sources.
Therefore, rather than attempting precise annotations of complex glycosylated flavonoids, a more generalized method would involve identifying the aglycone, the type of glycosidic bond, and the sugar type (such as “Kaempferol-O-hexoside”). These elements are more easily reproduced using standard LC-MS techniques. Thus, it offers a pragmatic and reliable way to report glycosylated flavonoids in NP research. Adopting this strategy could enhance the accuracy and utility of profiling flavonoid data in the field of metabolomics.
4 Conclusion
This study not only speeds up the way for the annotation process but also significantly improves the accuracy and coverage for identifying the correct aglycones, sugar moieties, and low-abundance glycosylated flavonoid types. Despite certain challenges in MS2 spectra deconvolution in MZmine 3, our findings underscore the immense potential of LC-MS/DIA in revolutionizing metabolite profiling in NP research. By combining cutting-edge technological platforms with expert manual scrutiny, we have demonstrated a powerful strategy that can be readily implemented in NP research. This approach is crucial for advancing our understanding and annotation of complex and varied glycosylated flavonoids. As our applicability case has shown, the Ocotea plants might produce an extensive profile of glycosylated flavonoids, which could be potentially useful in chemosystematics studies due to their distinct patterns. Still, in the present study, the apigenin flavone backbone was reported for most of the investigated Ocotea species, an unprecedented finding in the literature. The flavonols kaempferol and quercetin were mainly found in the O-glycosides form, while flavone apigenin derivatives were in the C-glycosides form. Interestingly, O. porosa displayed the highest flavone
:
flavonol ratio of 0.8, and thus distinct from the normally expected ratio for the Ocotea plant species. Besides those interesting results, our study also sheds light on the best practices for reporting such compounds, prompting a revaluation of reporting standards of specific classes, such as glycosylated flavonoids. Ultimately, this integrated approach paves the way for more comprehensive and reliable metabolite annotation in complex NP sources using LC-MS/DIA data.
Future research should aim to extend this profiling approach to a wider range of species within the Ocotea genus and other complex plant matrices. Further development of more inclusive and detailed metabolite databases would also enhance the applicability of this methodology across different fields of NP research. Moreover, exploring the biological activities of the newly identified flavonoids could provide valuable insights into their potential health benefits and pharmacological applications. This study sets a new benchmark for flavonoid profiling in complex natural matrices, offering valuable methodologies and insights for researchers in the fields of metabolomics, analytical chemistry, and NP chemistry at large.
Data availability
The Ocotea datasets of the current study were deposited to the Mass Spectrometry Interactive Virtual Environment (MassIVE) repository. The data that support the findings of this study are available under the registry code MSV000094030. Guidelines for files submitted to MassIVE for public access can be found online (https://massive.ucsd.edu/ProteoSAFe/static/massive.jsp). The MZmine processing parameters, and the in-house databases (.csv files), MZmine batch file (.xml), and the structures of aglycone fragments and sugar neutral losses (.cdx file) are available at Zenodo open repository (https://doi.org/10.5281/zenodo.10810967). Accompanying information (ESI 7 and video†) supplements this paper online. Additional files to download are available at Zenodo's link https://doi.org/10.5281/zenodo.10810967.
Author contributions
A. K. N., A. C. C. P. L., D. A. C. P., M. F. A., and P. C. P. B. conceived the idea. A. K. N. and M. F. A. wrote the draft paper. M. M. performed the high-resolution DIA-MSE experiments for data acquisition. M. F. A. performed MS data conversion and data processing. A. K. N. and M. S. F. constructed the KNIME workflows. A. K. N., D. A. C. P., F. C. N., M. F. A., P. C. P. B., R. C. contributed to data interpretation. A. K. N., D. F. D., F. C. N., M. F. A., M. G. S. aided in gas-phase fragmentation reactions elucidation. All authors participated in the discussion and revision of the manuscript.
Conflicts of interest
There are no known conflicts to declare.
Acknowledgements
This work received financial support from the Coordenação de Aperfeiçoamento de Pessoal de Nível Superior (CAPES Foundation, Brazil), finance code 001, from the Fundação de Amparo à Pesquisa do Estado de Minas Gerais (FAPEMIG, Brazil), finances codes: APQ-05218-23, APQ-00544-23, APQ-02353-17, APQ-00207-18 and BPD-00760-22, and the Conselho Nacional de Desenvolvimento Científico e Tecnológico (CNPq, Brazil) finances code 408115/2023-8, 316204/2021-8, and 406837/2021-0. The authors extend their thankfulness to the Leopoldo Krieger CESJ herbarium (UFJF- Federal University of Juiz de Fora – Minas Gerais, MG, Brazil) for generously providing the Ocotea spp. material. Their contributions were invaluable to the applicability case of this research.
References
- L. Abrankó and B. Szilvássy, Mass spectrometric profiling of flavonoid glycoconjugates possessing isomeric aglycones, J. Mass Spectrom., 2015, 50, 71–80, DOI:10.1002/jms.3474.
- Z. Yan, G. Lin, Y. Ye, Y. Wang and R. Yan, A Generic Multiple Reaction Monitoring Based Approach for Plant Flavonoids Profiling Using a Triple Quadrupole Linear Ion Trap Mass Spectrometry, J. Am. Soc. Mass Spectrom., 2014, 25, 955–965, DOI:10.1007/s13361-014-0863-6.
- A. C. Pilon, H. Gu, D. Raftery, V. da S. Bolzani, N. P. Lopes, I. Castro-Gamboa and F. Carnevale Neto, Mass Spectral Similarity Networking and Gas-Phase Fragmentation Reactions in the Structural Analysis of Flavonoid Glycoconjugates, Anal. Chem., 2019, 91, 10413–10423, DOI:10.1021/acs.analchem.8b05479.
- Y. Song, Q. Song, W. Liu, J. Li and P. Tu, High-confidence structural identification of metabolites relying on tandem mass spectrometry through isomeric identification: A tutorial, TrAC, Trends Anal. Chem., 2023, 160, 116982, DOI:10.1016/j.trac.2023.116982.
- T. Li, K. Zhang, X. Niu, W. Chen, X. Yang, X. Gong, P. Tu, Y. Wang, W. Liu and Y. Song, MS/MS fingerprint comparison between adjacent generations enables substructure identification: Flavonoid glycosides as cases, J. Pharm. Biomed. Anal., 2023, 234, 115559, DOI:10.1016/j.jpba.2023.115559.
- A. S. Chanderbali, H. van der Werff and S. S. Renner, Phylogeny and Historical Biogeography of Lauraceae: Evidence from the Chloroplast and Nuclear Genomes, Ann. Mo. Bot. Gard., 2001, 88, 104, DOI:10.2307/2666133.
- Y. Song, W.-B. Yu, Y.-H. Tan, J.-J. Jin, B. Wang, J.-B. Yang, B. Liu and R. T. Corlett, Plastid phylogenomics improve phylogenetic resolution in the Lauraceae, J. Syst. Evol., 2020, 58(4), 423–439, DOI:10.1111/jse.12536.
- T.-W. Xiao, Y. Xu, L. Jin, T.-J. Liu, H.-F. Yan and X.-J. Ge, Conflicting phylogenetic signals in plastomes of the tribe Laureae (Lauraceae), PeerJ, 2020, 8, e10155, DOI:10.7717/peerj.10155.
- D. L. Custódio and V. Florêncio da Veiga Junior, Lauraceae alkaloids, RSC Adv., 2014, 4, 21864–21890, 10.1039/C4RA01904K.
- W. M. N. H. W. Salleh and F. Ahmad, Phytochemistry and Biological Activities of the Genus Ocotea (Lauraceae): A Review on Recent Research Results (2000-2016), J. Appl. Pharm. Sci., 2017, 7(5), 204–218, DOI:10.7324/JAPS.2017.70534.
- A. Katchborian-Neto, K. J. de Nicácio, J. C. Cruz, P. C. P. Bueno, M. Murgu, D. F. Dias, M. G. Soares, A. C. C. Paula and D. A. Chagas-Paula, Bioprospecting-based untargeted metabolomics identifies alkaloids as potential anti-inflammatory bioactive markers of Ocotea species (Lauraceae), Phytomedicine, 2023, 120, 155060, DOI:10.1016/j.phymed.2023.155060.
- A. Katchborian-Neto, M. F. Alves, P. C. P. Bueno, K. J. de Nicácio, M. S. Ferreira, T. B. Oliveira, H. Barbosa, M. Murgu, A. C. C. de Paula Ladvocat, D. F. Dias, M. G. Soares, J. H. G. Lago and D. A. Chagas-Paula, Integrative open workflow for confident annotation and molecular networking of metabolomics MSE/DIA data, Briefings Bioinf., 2024, 25(2), bbae013, DOI:10.1093/bib/bbae013.
- A. F. Silva, M. F. C. Santos, T. S. C. Maiolini, P. P. O. Salem, M. Murgu, A. C. C. Paula, E. O. Silva, K. J. Nicácio, A. G. Ferreira, D. F. Dias, M. G. Soares and D. A. Chagas-Paula, Chemistry of leaves, bark, and essential oils from Ocotea diospyrifolia and anti-inflammatory activity – Dual inhibition of edema and neutrophil recruitment, Phytochem Lett., 2021, 42, 52–60, DOI:10.1016/j.phytol.2021.02.002.
- B. G. V. de Alcântara, F. P. de Oliveira, A. Katchborian-Neto, R. Casoti, O. S. Domingos, M. F. C. Santos, R. B. de Oliveira, A. C. C. de Paula, D. F. Dias, M. G. Soares and D. A. Chagas-Paula, Confirmation of ethnopharmacological anti-inflammatory properties of Ocotea odorifera and determination of its main active compounds, J. Ethnopharmacol., 2021, 264, 113378, DOI:10.1016/j.jep.2020.113378.
- A. S. Antonio, V. F. Veiga-Junior and L. S. M. Wiedemann, Ocotea complex: A metabolomic analysis of a Lauraceae genus, Phytochemistry, 2020, 173, 112314, DOI:10.1016/j.phytochem.2020.112314.
- C. Zidorn, Plant chemophenetics − A new term for plant chemosystematics/plant chemotaxonomy in the macro-molecular era, Phytochemistry, 2019, 163, 147–148, DOI:10.1016/j.phytochem.2019.02.013.
- D. Trofimov, P. L. R. de Moraes and J. G. Rohwer, Towards a phylogenetic classification of the Ocotea complex (Lauraceae): classification principles and reinstatement of Mespilodaphne, Bot. J. Linn. Soc., 2019, 190, 25–50, DOI:10.1093/botlinnean/boz010.
- J. H. Yariwake Vilegas, O. R. Gottlieb, M. A. C. Kaplan and H. E. Gottlieb, Aporphine alkaloids from Ocotea caesia, Phytochemistry, 1989, 28, 3577–3578, DOI:10.1016/0031-9422(89)80403-0.
- N. C. Franca, A. M. Giesbrecht, O. R. Gottlieb, A. F. Magalhães, E. G. Magalhães and J. G. S. Maia, Benzylisoquinolines from Ocotea species, Phytochemistry, 1975, 14, 1671–1672, DOI:10.1016/0031-9422(75)85390-8.
- M. C. C. P. Gomes, M. Yoshida, O. R. Gottlieb, C. Juan, C. Martinez and H. E. Gottlieb, Bicyclo(3.2.1)octane neolignans from an Ocotea species, Phytochemistry, 1983, 22, 269–273, DOI:10.1016/S0031-9422(00)80104-1.
- J. M. David, M. Yoshida and O. R. Gottlieb, Neolignans from bark and leaves of Ocotea porosa, Phytochemistry, 1994, 36, 491–499, DOI:10.1016/S0031-9422(00)97102-4.
- A. L. Lacava Lordello and M. Yoshida, Neolignans from leaves of Ocotea catharinensis, Phytochemistry, 1997, 46, 741–744, DOI:10.1016/S0031-9422(97)00343-9.
- M. O. M. Marques, M. C. C. P. Gomes, M. Yoshida and O. R. Gottlieb, Bicyclo [3.2.1] octanoid neolignans from Ocotea porosa, Phytochemistry, 1992, 31, 275–277, DOI:10.1016/0031-9422(91)83053-N.
- M. M. R. Silva Teles, A. A. Vieira Pinheiro, C. Da Silva Dias, J. Fechine Tavares, J. M. Barbosa Filho and E. V. Leitão Da Cunha, Alkaloids of the Lauraceae, The Alkaloids: Chemistry and Biology, 2019, vol. 82, pp. 147–304, DOI:10.1016/bs.alkal.2018.11.002.
- K. Yonekura-Sakakibara, Y. Higashi and R. Nakabayashi, The origin and evolution of plant flavonoid metabolism, Front. Plant Sci., 2019, 10, 943, DOI:10.3389/fpls.2019.00943.
- P. M. Dewick, Medicinal Natural Products, John Wiley & Sons, Ltd, Chichester, UK, 2009, DOI:10.1002/9780470742761.
- Z. Lei, B. W. Sumner, A. Bhatia, S. J. Sarma and L. W. Sumner, UHPLC-MS analyses of plant flavonoids, Curr. Protoc. Plant Biol., 2019, 4, e20085, DOI:10.1002/cppb.20085.
- D. Chagas-Paula, T. Zhang, F. Da Costa and R. Edrada-Ebel, A metabolomic approach to target compounds from the Asteraceae family for dual COX and LOX inhibition, Metabolites, 2015, 5, 404–430, DOI:10.3390/metabo5030404.
- J.-L. Wolfender, J.-M. Nuzillard, J. J. J. van der Hooft, J.-H. Renault and S. Bertrand, Accelerating metabolite identification in natural product research: Toward an ideal combination of Liquid Chromatography–High-Resolution Tandem Mass Spectrometry and NMR profiling, in silico databases, and chemometrics, Anal. Chem., 2019, 91, 704–742, DOI:10.1021/acs.analchem.8b05112.
- L. Chen, W. Lu, L. Wang, X. Xing, Z. Chen, X. Teng, X. Zeng, A. D. Muscarella, Y. Shen, A. Cowan, M. R. McReynolds, B. J. Kennedy, A. M. Lato, S. R. Campagna, M. Singh and J. D. Rabinowitz, Metabolite discovery through global annotation of untargeted metabolomics data, Nat. Methods, 2021, 18, 1377–1385, DOI:10.1038/s41592-021-01303-3.
- L. Cui, H. Lu and Y. H. Lee, Challenges and emergent solutions for LC-MS/MS based untargeted metabolomics in diseases, Mass Spectrom. Rev., 2018, 37, 772–792, DOI:10.1002/mas.21562.
- C. Chen, D. Lee, J. Yu, Y. Lin and T. Lin, Recent advances in LC-MS-based metabolomics for clinical biomarker discovery, Mass Spectrom. Rev., 2023, 42, 2349–2378, DOI:10.1002/mas.21785.
- T. M. D. Ebbels, J. J. J. van der Hooft, H. Chatelaine, C. Broeckling, N. Zamboni, S. Hassoun and E. A. Mathé, Recent advances in mass spectrometry-based computational metabolomics, Curr. Opin. Chem. Biol., 2023, 74, 102288, DOI:10.1016/j.cbpa.2023.102288.
- V. Davies, J. Wandy, S. Weidt, J. J. J. van der Hooft, A. Miller, R. Daly and S. Rogers, Rapid development of improved data-dependent acquisition strategies, Anal. Chem., 2021, 93, 5676–5683, DOI:10.1021/acs.analchem.0c03895.
- J. Guo and T. Huan, Comparison of full-scan, data-dependent, and data-independent acquisition modes in Liquid Chromatography–Mass Spectrometry based untargeted metabolomics, Anal. Chem., 2020, 92, 8072–8080, DOI:10.1021/acs.analchem.9b05135.
- R. Lou and W. Shui, Acquisition and analysis of DIA-based proteomic data: A comprehensive survey in 2023, Mol. Cell. Proteomics, 2024, 23(2), 100712, DOI:10.1016/j.mcpro.2024.100712.
- F. Fenaille, P. Barbier Saint-Hilaire, K. Rousseau and C. Junot, Data acquisition workflows in liquid chromatography coupled to high resolution mass spectrometry-based metabolomics: Where do we stand?, J. Chromatogr. A, 2017, 1526, 1–12, DOI:10.1016/j.chroma.2017.10.043.
- O. Alka, P. Shanthamoorthy, M. Witting, K. Kleigrewe, O. Kohlbacher and H. L. Röst, DIAMetAlyzer allows automated false-discovery rate-controlled analysis for data-independent acquisition in metabolomics, Nat. Commun., 2022, 13, 1347, DOI:10.1038/s41467-022-29006-z.
- R. Wang, Y. Yin and Z.-J. Zhu, Advancing untargeted metabolomics using data-independent acquisition mass spectrometry technology, Anal. Bioanal. Chem., 2019, 411, 4349–4357, DOI:10.1007/s00216-019-01709-1.
- D. Amodei, J. Egertson, B. X. MacLean, R. Johnson, G. E. Merrihew, A. Keller, D. Marsh, O. Vitek, P. Mallick and M. J. MacCoss, Improving precursor selectivity in data-independent acquisition using overlapping windows, J. Am. Soc. Mass Spectrom., 2019, 30, 669–684, DOI:10.1007/s13361-018-2122-8.
- F. Carnevale Neto, T. N. Clark, N. P. Lopes and R. G. Linington, Evaluation of ion mobility spectrometry for improving constitutional assignment in natural product mixtures, J. Nat. Prod., 2022, 85, 519–529, DOI:10.1021/acs.jnatprod.1c01048.
- L. C. Gillet, P. Navarro, S. Tate, H. Ro, N. Selevsek, L. Reiter, R. Bonner and R. Aebersold, Targeted data extraction of the MS/MS spectra generated by data-independent acquisition: a new concept for consistent and accurate proteome analysis, Mol. Cell. Proteomics, 2012, 11(6), O111.016717, DOI:10.1074/mcp.O111.016717–1.
- M. A. Moseley, C. J. Hughes, P. R. Juvvadi, E. J. Soderblom, S. Lennon, S. R. Perkins, J. W. Thompson, W. J. Steinbach, S. J. Geromanos, J. Wildgoose, J. I. Langridge, K. Richardson and J. P. C. Vissers, Scanning quadrupole
data-independent acquisition, part A: Qualitative and quantitative characterization, J. Proteome Res., 2018, 17(2), 770–779, DOI:10.1021/acs.jproteome.7b00464.
- A. Goon, Z. Khan, D. Oulkar, R. Shinde, S. Gaikwad and K. Banerjee, A simultaneous screening and quantitative method for the multiresidue analysis of pesticides in spices using ultra-high performance liquid chromatography-high resolution (Orbitrap) mass spectrometry, J. Chromatogr. A, 2018, 1532, 105–111, DOI:10.1016/j.chroma.2017.11.066.
- U. H. Guzman, A. Martinez-Val, Z. Ye, E. Damoc, T. N. Arrey, A. Pashkova, S. Renuse, E. Denisov, J. Petzoldt, A. C. Peterson, F. Harking, O. Østergaard, R. Rydbirk, S. Aznar, H. Stewart, Y. Xuan, D. Hermanson, S. Horning, C. Hock, A. Makarov, V. Zabrouskov and J. V. Olsen, Ultra-fast label-free quantification and comprehensive proteome coverage with narrow-window data-independent acquisition, Nat. Biotechnol., 2024 DOI:10.1038/s41587-023-02099-7.
- Y. Wang, N. Vorsa, P. B. Harrington and P. Chen, Nontargeted metabolomic study on variation of phenolics in different cranberry cultivars using UPLC-IM–HRMS, J. Agric. Food Chem., 2018, 66(46), 12206–12216, DOI:10.1021/acs.jafc.8b05029.
- T. Radchenko, C. J. Kochansky, M. Cancilla, M. D. Wrona, R. J. M.-Smith, J. Kirk, G. Murray, F. Fontaine and I. Zamora, Metabolite identification using an ion mobility enhanced data-independent acquisition strategy and automated data processing, Rapid Commun. Mass Spectrom., 2020, 34(12), e8792, DOI:10.1002/rcm.8792.
- C. A. Ledesma-Escobar, F. Priego-Capote and M. Calderón-Santiago, MetaboMSDIA: A tool for implementing data-independent acquisition in metabolomic-based mass spectrometry analysis, Anal. Chim. Acta, 2023, 1266, 341308, DOI:10.1016/j.aca.2023.341308.
- J. Guo, H. Yu, S. Xing and T. Huan, Addressing big data challenges in mass spectrometry-based metabolomics, Chem. Commun., 2022, 58, 9979–9990, 10.1039/D2CC03598G.
- J. Zhou, Y. Li, X. Chen, L. Zhong and Y. Yin, Development of data-independent acquisition workflows for metabolomic analysis on a quadrupole-orbitrap platform, Talanta, 2017, 164, 128–136, DOI:10.1016/j.talanta.2016.11.048.
- S. An, R. Wang, M. Lu, C. Zhang, H. Liu, J. Wang, C. Xie and C. Yu, MetaPro: a web-based metabolomics application for LC-MS data batch inspection and library curation, Metabolomics, 2023, 19, 57, DOI:10.1007/s11306-023-02018-6.
- G. Theodoridis, H. Gika, D. Raftery, R. Goodacre, R. S. Plumb and I. D. Wilson, Ensuring fact-based metabolite identification in Liquid Chromatography–Mass Spectrometry-based metabolomics, Anal. Chem., 2023, 95, 3909–3916, DOI:10.1021/acs.analchem.2c05192.
- E. J. Llorent-Martínez, V. Spínola and P. C. Castilho, Phenolic profiles of Lauraceae plant species endemic to Laurisilva forest: A chemotaxonomic survey, Ind. Crops Prod., 2017, 107, 1–12, DOI:10.1016/j.indcrop.2017.05.023.
- A. S. Antonio, A. T. C. Aguiar, G. R. C. dos Santos, H. M. G. Pereira, V. F. da Veiga-Junior and L. S. M. Wiedemann, Phytochemistry by design: a case study of the chemical composition of Ocotea guianensis optimized extracts focused on untargeted metabolomics analysis, RSC Adv., 2020, 10, 3459–3471, 10.1039/C9RA10436D.
- R. V. Pereira, A. S. Mecenas, C. R. A. Malafaia, A. Claudia, F. Amaral, M. F. Muzitano, N. K. Simas and I. Correa Ramos Leal, Evaluation of the chemical composition and antioxidant activity of extracts and fractions of Ocotea notata (Ness) Mez (Lauraceae), Nat. Prod. Res., 2020, 34, 3004–3007, DOI:10.1080/14786419.2019.1602828.
- I. M. A. Reis, D. S. A. Cassiano, R. S. Conceição, H. F. Freitas, S. S. R. Pita, J. M. David and A. Branco, Acetylcholinesterase inhibitory activity of Ocotea pomaderroides extracts: HPLC-MS/MS characterization and molecular modeling studies, Nat. Prod. Res., 2022, 36(4), 999–1003, DOI:10.1080/14786419.2020.1839453.
- D. C. Gontijo, G. C. Brandão, P. C. Gontijo, A. B. de Oliveira, M. A. N. Diaz, L. G. Fietto and J. P. V. Leite, Identification of phenolic compounds and biologically related activities from Ocotea odorifera aqueous extract leaves, Food Chem., 2017, 230, 618–626, DOI:10.1016/j.foodchem.2017.03.087.
- A. S. Antonio, G. R. C. dos Santos, H. M. G. Pereira, V. F. Veiga-Junior and L. S. M. Wiedemann, Chemophenetic study of Ocotea canaliculata (Lauraceae) by UHPLC–HRMS and GNPS, Nat. Prod. Res., 2022, 36(4), 984–988, DOI:10.1080/14786419.2020.1837823.
- F. C. M. Betim, C. F. de Oliveira, K. S. Rech, A. M. Souza, O. G. Miguel, M. D. Miguel, D. P. Montrucchio, J. B. B. Maurer and J. F. G. de Dias, Ocotea nutans (Nees) Mez: structural elucidation of C-hetorosides flavonoids and evaluation of their antioxidant and antibacterial properties from ethyl acetate extract, Nat. Prod. Res., 2022, 36(11), 2902–2906, DOI:10.1080/14786419.2021.1931184.
- R. Garrett, M. T. V. Romanos, R. M. Borges, M. G. Santos, L. Rocha and A. J. R. da Silva, Antiherpetic activity of a flavonoid fraction from Ocotea notata leaves, Rev. Bras. Farmacogn., 2012, 22, 306–313, DOI:10.1590/S0102-695X2012005000003.
- D. T. Silva, R. Herrera, B. F. Batista, B. M. Heinzmann and J. Labidi, Physicochemical characterization of leaf extracts from Ocotea lancifolia and its effect against wood-rot fungi, Int. Biodeterior. Biodegrad., 2017, 117, 158–170, DOI:10.1016/j.ibiod.2016.12.007.
- I. F. B. Costa, S. D. Calixto, M. Heggdorne de Araujo, T. U. P. Konno, L. W. Tinoco, D. O. Guimarães, E. B. Lasunskaia, I. R. C. Leal and M. F. Muzitano, Antimycobacterial and nitric oxide production inhibitory activities of Ocotea notata from Brazilian Restinga, Sci. World J., 2015, 2015, 1–9, DOI:10.1155/2015/947248.
- R. C. De Vos, S. Moco, A. Lommen, J. J. Keurentjes, R. J. Bino and R. D. Hall, Untargeted large-scale plant metabolomics using liquid chromatography coupled to mass spectrometry, Nat. Protoc., 2007, 2, 778–791, DOI:10.1038/nprot.2007.95.
- M. Valli, R. N. dos Santos, L. D. Figueira, C. H. Nakajima, I. Castro-Gamboa, A. D. Andricopulo and V. S. Bolzani, Development of a Natural Products Database from the Biodiversity of Brazil, J. Nat. Prod., 2013, 76(3), 439–444, DOI:10.1021/np3006875.
- A. C. Pilon, M. Valli, A. C. Dametto, M. E. F. Pinto, R. T. Freire, I. Castro-Gamboa, A. D. Andricopulo and V. S. Bolzani, NuBBEDB: an updated database to uncover chemical and biological information from Brazilian biodiversity, Sci. Rep., 2017, 7(1), 7215, DOI:10.1038/s41598-017-07451-x.
- E. Hvattum and D. Ekeberg, Study of the collision-induced radical cleavage of flavonoid glycosides using negative electrospray ionization tandem quadrupole mass spectrometry, J. Mass Spectrom., 2003, 38, 43–49, DOI:10.1002/jms.398.
- L. Abrankó, J. F. García-Reyes and A. Molina-Díaz, In-source fragmentation and accurate mass analysis of multiclass flavonoid conjugates by electrospray ionization time-of-flight mass spectrometry, J. Mass Spectrom., 2011, 46, 478–488, DOI:10.1002/jms.1914.
- J. Cao, C. Yin, Y. Qin, Z. Cheng and D. Chen, Approach to the study of flavone di-C-glycosides by high performance liquid chromatography-tandem ion trap mass spectrometry and its application to characterization of flavonoid composition in Viola yedoensis, J. Mass Spectrom., 2014, 49, 1010–1024, DOI:10.1002/jms.3413.
- V. Vukics and A. Guttman, Structural characterization of flavonoid glycosides by multi-stage mass spectrometry, Mass Spectrom. Rev., 2010, 29, 1–16, DOI:10.1002/mas.20212.
- N. Akimoto, T. Ara, D. Nakajima, K. Suda, C. Ikeda, S. Takahashi, R. Muneto, M. Yamada, H. Suzuki, D. Shibata and N. Sakurai, FlavonoidSearch: A system for comprehensive flavonoid annotation by mass spectrometry, Sci. Rep., 2017, 7, 1243, DOI:10.1038/s41598-017-01390-3.
- L. W. Sumner, A. Amberg, D. Barrett, M. H. Beale, R. Beger, C. A. Daykin, T. W.-M. Fan, O. Fiehn, R. Goodacre, J. L. Griffin, T. Hankemeier, N. Hardy, J. Harnly, R. Higashi, J. Kopka, A. N. Lane, J. C. Lindon, P. Marriott, A. W. Nicholls, M. D. Reily, J. J. Thaden and M. R. Viant, Proposed minimum reporting standards for chemical analysis, Metabolomics, 2007, 3, 211–221, DOI:10.1007/s11306-007-0082-2.
- M. Kanehisa, KEGG: Kyoto Encyclopedia of Genes and Genomes, Nucleic Acids Res., 2000, 28, 27–30, DOI:10.1093/nar/28.1.27.
- H. Tsugawa, T. Cajka, T. Kind, Y. Ma, B. Higgins, K. Ikeda, M. Kanazawa, J. VanderGheynst, O. Fiehn and M. Arita, MS-DIAL: data-independent MS/MS deconvolution for comprehensive metabolome analysis, Nat. Methods, 2015, 12, 523–526, DOI:10.1038/nmeth.3393.
- S. Rakusanova, O. Fiehn and T. Cajka, Toward building mass spectrometry-based metabolomics and lipidomics atlases for biological and clinical research, TrAC, Trends Anal. Chem., 2023, 158, 116825, DOI:10.1016/j.trac.2022.116825.
- H. Tsugawa, K. Ikeda, M. Takahashi, A. Satoh, Y. Mori, H. Uchino, N. Okahashi, Y. Yamada, I. Tada, P. Bonini, Y. Higashi, Y. Okazaki, Z. Zhou, Z.-J. Zhu, J. Koelmel, T. Cajka, O. Fiehn, K. Saito, M. Arita and M. Arita, A lipidome atlas in MS-DIAL 4, Nat. Biotechnol., 2020, 38, 1159–1163, DOI:10.1038/s41587-020-0531-2.
- L. Perez de Souza and A. R. Fernie, Computational methods for processing and interpreting mass spectrometry-based metabolomics, Essays Biochem., 2023, EBC20230019, DOI:10.1042/EBC20230019.
- G. Graça, Y. Cai, C.-H. E. Lau, P. A. Vorkas, M. R. Lewis, E. J. Want, D. Herrington and T. M. D. Ebbels, Automated annotation of untargeted all-ion fragmentation LC–MS metabolomics data with MetaboAnnotatoR, Anal. Chem., 2022, 94, 3446–3455, DOI:10.1021/acs.analchem.1c03032.
- E. Stancliffe, M. Schwaiger-Haber, M. Sindelar and G. J. Patti, DecoID improves identification rates in metabolomics through database-assisted MS/MS deconvolution, Nat. Methods, 2021, 18, 779–787, DOI:10.1038/s41592-021-01195-3.
- R. Schmid, S. Heuckeroth, A. Korf, A. Smirnov, O. Myers, T. S. Dyrlund, R. Bushuiev, K. J. Murray, N. Hoffmann, M. Lu, A. Sarvepalli, Z. Zhang, M. Fleischauer, K. Dührkop, M. Wesner, S. J. Hoogstra, E. Rudt, O. Mokshyna, C. Brungs, K. Ponomarov, L. Mutabdžija, T. Damiani, C. J. Pudney, M. Earll, P. O. Helmer, T. R. Fallon, T. Schulze, A. Rivas-Ubach, A. Bilbao, H. Richter, L.-F. Nothias, M. Wang, M. Orešič, J.-K. Weng, S. Böcker, A. Jeibmann, H. Hayen, U. Karst, P. C. Dorrestein, D. Petras, X. Du and T. Pluskal, Integrative analysis of multimodal mass spectrometry data in MZmine 3, Nat. Biotechnol., 2023, 4, 447–449, DOI:10.1038/s41587-023-01690-2.
- J. Guo, S. Shen, S. Xing and T. Huan, DaDIA: Hybridizing data-dependent and data-independent acquisition modes for generating high-quality metabolomic data, Anal. Chem., 2021, 93, 2669–2677, DOI:10.1021/acs.analchem.0c05022.
- S. Naz, H. Gallart-Ayala, S. N. Reinke, C. Mathon, R. Blankley, R. Chaleckis and C. E. Wheelock, Development of a Liquid Chromatography–High Resolution Mass Spectrometry metabolomics method with high specificity for metabolite identification using all ion fragmentation acquisition, Anal. Chem., 2017, 89, 7933–7942, DOI:10.1021/acs.analchem.7b00925.
- P. Barbier Saint Hilaire, K. Rousseau, A. Seyer, S. Dechaumet, A. Damont, C. Junot and F. Fenaille, Comparative evaluation of data dependent and data independent acquisition workflows implemented on an Orbitrap fusion for untargeted metabolomics, Metabolites, 2020, 10, 158, DOI:10.3390/metabo10040158.
- L.-F. Nothias, D. Petras, R. Schmid, K. Dührkop, J. Rainer, A. Sarvepalli, I. Protsyuk, M. Ernst, H. Tsugawa, M. Fleischauer, F. Aicheler, A. A. Aksenov, O. Alka, P.-M. Allard, A. Barsch, X. Cachet, A. M. Caraballo-Rodriguez, R. R. Da Silva, T. Dang, N. Garg, J. M. Gauglitz, A. Gurevich, G. Isaac, A. K. Jarmusch, Z. Kameník, K. Bin Kang, N. Kessler, I. Koester, A. Korf, A. Le Gouellec, M. Ludwig, C. Martin H., L.-I. McCall, J. McSayles, S. W. Meyer, H. Mohimani, M. Morsy, O. Moyne, S. Neumann, H. Neuweger, N. H. Nguyen, M. Nothias-Esposito, J. Paolini, V. V. Phelan, T. Pluskal, R. A. Quinn, S. Rogers, B. Shrestha, A. Tripathi, J. J. J. van der Hooft, F. Vargas, K. C. Weldon, M. Witting, H. Yang, Z. Zhang, F. Zubeil, O. Kohlbacher, S. Böcker, T. Alexandrov, N. Bandeira, M. Wang and P. C. Dorrestein, Feature-based molecular networking in the GNPS analysis environment, Nat. Methods, 2020, 17, 905–908, DOI:10.1038/s41592-020-0933-6.
- M. Wang, J. J. Carver, V. V Phelan, L. M. Sanchez, N. Garg, Y. Peng, D. D. Nguyen, J. Watrous, C. A. Kapono, T. Luzzatto-Knaan, C. Porto, A. Bouslimani, A. V Melnik, M. J. Meehan, W.-T. Liu, M. Crüsemann, P. D. Boudreau, E. Esquenazi, M. Sandoval-Calderón, R. D. Kersten, L. A. Pace, R. A. Quinn, K. R. Duncan, C.-C. Hsu, D. J. Floros, R. G. Gavilan, K. Kleigrewe, T. Northen, R. J. Dutton, D. Parrot, E. E. Carlson, B. Aigle, C. F. Michelsen, L. Jelsbak, C. Sohlenkamp, P. Pevzner, A. Edlund, J. McLean, J. Piel, B. T. Murphy, L. Gerwick, C.-C. Liaw, Y.-L. Yang, H.-U. Humpf, M. Maansson, R. A. Keyzers, A. C. Sims, A. R. Johnson, A. M. Sidebottom, B. E. Sedio, A. Klitgaard, C. B. Larson, C. A. Boya P, D. Torres-Mendoza, D. J. Gonzalez, D. B. Silva, L. M. Marques, D. P. Demarque, E. Pociute, E. C. O'Neill, E. Briand, E. J. N. Helfrich, E. A. Granatosky, E. Glukhov, F. Ryffel, H. Houson, H. Mohimani, J. J. Kharbush, Y. Zeng, J. A. Vorholt, K. L. Kurita, P. Charusanti, K. L. McPhail, K. F. Nielsen, L. Vuong, M. Elfeki, M. F. Traxler, N. Engene, N. Koyama, O. B. Vining, R. Baric, R. R. Silva, S. J. Mascuch, S. Tomasi, S. Jenkins, V. Macherla, T. Hoffman, V. Agarwal, P. G. Williams, J. Dai, R. Neupane, J. Gurr, A. M. C. Rodríguez, A. Lamsa, C. Zhang, K. Dorrestein, B. M. Duggan, J. Almaliti, P.-M. Allard, P. Phapale, L.-F. Nothias, T. Alexandrov, M. Litaudon, J.-L. Wolfender, J. E. Kyle, T. O. Metz, T. Peryea, D.-T. Nguyen, D. VanLeer, P. Shinn, A. Jadhav, R. Müller, K. M. Waters, W. Shi, X. Liu, L. Zhang, R. Knight, P. R. Jensen, B. Ø. Palsson, K. Pogliano, R. G. Linington, M. Gutiérrez, N. P. Lopes, W. H. Gerwick, B. S. Moore, P. C. Dorrestein and N. Bandeira, Sharing and community curation of mass spectrometry data with Global Natural Products Social Molecular Networking, Nat. Biotechnol., 2016, 34, 828–837, DOI:10.1038/nbt.3597.
- A. T. Aron, E. C. Gentry, K. L. McPhail, L.-F. Nothias, M. Nothias-Esposito, A. Bouslimani, D. Petras, J. M. Gauglitz, N. Sikora, F. Vargas, J. J. J. van der Hooft, M. Ernst, K. Bin Kang, C. M. Aceves, A. M. Caraballo-Rodríguez, I. Koester, K. C. Weldon, S. Bertrand, C. Roullier, K. Sun, R. M. Tehan, C. A. Boya P., M. H. Christian, M. Gutiérrez, A. M. Ulloa, J. A. Tejeda Mora, R. Mojica-Flores, J. Lakey-Beitia, V. Vásquez-Chaves, Y. Zhang, A. I. Calderón, N. Tayler, R. A. Keyzers, F. Tugizimana, N. Ndlovu, A. A. Aksenov, A. K. Jarmusch, R. Schmid, A. W. Truman, N. Bandeira, M. Wang and P. C. Dorrestein, Reproducible molecular networking of untargeted mass spectrometry data using GNPS, Nat. Protoc., 2020, 15, 1954–1991, DOI:10.1038/s41596-020-0317-5.
- F. Hadacek, Secondary or specialized metabolites, or natural products: A case study of untargeted LC–QTOF auto-MS/MS analysis, Cells, 2022, 11, 1025, DOI:10.3390/cells11061025.
- D. P. Demarque, A. E. M. Crotti, R. Vessecchi, J. L. C. Lopes and N. P. Lopes, Fragmentation reactions using electrospray ionization mass spectrometry: an important tool for the structural elucidation and characterization of synthetic and natural products, Nat. Prod. Rep., 2016, 33, 432–455, 10.1039/C5NP00073D.
- B. de Lima, F. da Silva, E. Soares, R. de Almeida, F. da Silva-Filho, A. Barison, E. Costa, H. Koolen, A. de Souza and M. L. Pinheiro, Integrative approach based on leaf spray mass spectrometry, HPLC-DAD-MS/MS, and NMR for comprehensive characterization of isoquinoline-derived alkaloids in leaves of Onychopetalum amazonicum R. E. Fr, J. Braz. Chem. Soc., 2020, 31, 79–89, DOI:10.21577/0103-5053.20190125.
- N. Fabre, I. Rustan, E. de Hoffmann and J. Quetin-Leclercq, Determination of flavone, flavonol, and flavanone aglycones by negative ion liquid chromatography electrospray ion trap mass spectrometry, J. Am. Soc. Mass Spectrom., 2001, 12, 707–715, DOI:10.1016/S1044-0305(01)00226-4.
- D. Tsimogiannis, M. Samiotaki, G. Panayotou and V. Oreopoulou, Characterization of flavonoid subgroups and hydroxy substitution by HPLC-MS/MS, Molecules, 2007, 12, 593–606, DOI:10.3390/12030593.
- W.-Z. Yang, W.-Y. Wu, M. Yang and D.-A. Guo, Elucidation of the fragmentation pathways of a complex 3,7- O -glycosyl flavonol by CID, HCD, and PQD on an LTQ-Orbitrap Velos Pro hybrid mass spectrometer, Chin. J. Nat. Med., 2015, 13, 867–872, DOI:10.1016/S1875-5364(15)30091-1.
- W. Yang, X. Qiao, T. Bo, Q. Wang, D. Guo and M. Ye, Low energy induced homolytic fragmentation of flavonol 3-O-glycosides by negative electrospray ionization tandem mass spectrometry, Rapid Commun. Mass Spectrom., 2014, 28, 385–395, DOI:10.1002/rcm.6794.
- B. D. Davis and J. S. Brodbelt, An investigation of the homolytic saccharide cleavage of deprotonated flavonol 3-O-glycosides in a quadrupole ion trap mass spectrometer, J. Mass Spectrom., 2008, 43, 1045–1052, DOI:10.1002/jms.1381.
- C. Przybylski and V. Bonnet, Discrimination of cyclic and linear oligosaccharides by tandem mass spectrometry using collision-induced dissociation (CID), pulsed-Q-dissociation (PQD) and the higher-energy C-trap dissociation modes, Rapid Commun. Mass Spectrom., 2013, 27, 75–87, DOI:10.1002/rcm.6422.
- S. Mörlein, C. Schuster, M. Paal and M. Vogeser, Collision energy-breakdown curves – An additional tool to characterize MS/MS methods, Clin. Mass Spectrom., 2020, 18, 48–53, DOI:10.1016/j.clinms.2020.10.001.
- Y. Murai, G. Kokubugata, M. Yokota, J. Kitajima and T. Iwashina, Flavonoids and anthocyanins from six Cassytha taxa (Lauraceae) as taxonomic markers, Biochem. Syst. Ecol., 2008, 36, 745–748, DOI:10.1016/j.bse.2008.06.007.
- J. M. David, M. Yoshida and O. R. Gottlieb, Phenylpropanoid-catechins from bark of Ocotea porosa, Phytochemistry, 1994, 35, 545–546, DOI:10.1016/S0031-9422(00)94800-3.
|
This journal is © The Royal Society of Chemistry 2024 |
Click here to see how this site uses Cookies. View our privacy policy here.