DOI:
10.1039/D4VA00112E
(Perspective)
Environ. Sci.: Adv., 2024,
3, 1181-1196
Solving freshwater conservation challenges through next-generation sequencing approaches
Received
11th April 2024
, Accepted 11th June 2024
First published on 11th June 2024
Abstract
Freshwater ecosystems face numerous conservation challenges due to anthropogenic pressures and environmental changes, necessitating advanced monitoring methods for effective conservation strategies. Traditional monitoring approaches have limitations, including low resolution and the inability to address emerging threats or understand the structure–function relationship within ecosystems. This paper explores how Next-Generation Sequencing (NGS) approaches can revolutionize freshwater conservation efforts by integrating unbiased molecular insights into biomonitoring. By leveraging NGS methods a comprehensive understanding of ecosystem dynamics can be achieved. The paper emphasizes the critical link between microbial community composition and ecosystem functioning, highlighting the assessment of functional diversity and activity as key metrics in evaluating ecosystem health. The significant advancements NGS brings to the field enable a proactive approach to conservation strategies and informed management decisions. This paper provides a comprehensive overview of the importance and advancements in integrating NGS methods, marking a paradigm shift in conservation practices and leveraging cutting-edge technologies to safeguard the integrity and resilience of freshwater ecosystems for future generations.
Environmental significance
Freshwater ecosystems are under increasing threat from anthropogenic pressures and environmental changes, necessitating innovative monitoring approaches for effective conservation. In this perspective paper, we delve into the transformative potential of Next-Generation Sequencing (NGS) in freshwater conservation while critically examining the limitations of traditional biomonitoring methods. NGS, by offering comprehensive molecular insights into ecosystem dynamics, addresses key constraints of conventional biomonitoring and enhances our understanding of community composition and ecosystem functioning. These advancements empower us to adopt proactive conservation measures and make informed decisions regarding ecosystem management. This paper underscores the importance of integrating NGS methods in freshwater biomonitoring and elucidates how this integration revolutionizes conservation practices, ensuring the sustainability of freshwater ecosystems for future generations.
|
1. Introduction
Although freshwater systems occupy only 2.3% of the global land surface and comprise only 0.01% of the water on Earth,1 these systems harbor a disproportionately high fraction of global biodiversity with nearly 9.5% of Earth's described animal species, including one-third of all described vertebrates.2 This biodiversity is of significant relevance in maintaining and supporting ecological health while providing valuable and essential goods and services to society.3 Between 1970 and 2018, an average decline of 69% was recorded for wildlife populations (based on the Living Planet Index (LPI); average change in the relative abundance of 31
821 populations, representing 5230 species monitored across the globe). These losses are particularly pronounced in freshwater ecosystems,4–7 and recent estimates suggest that at least 10
000 to 20
000 freshwater species have gone extinct in the last century or are at risk of extinction,2,8 with loss rates about five times greater than the corresponding rate for terrestrial fauna.8,9
The very high risk of biodiversity losses in freshwater ecosystems is due to the convergences of high biodiversity and the plethora of hazards posed by human exploitation, including water supplies, irrigation, electricity generation, waste disposal, and transportation.9–12 Examples of significant global pressures on freshwater ecosystems include non-chemical stress factors such as habitat degradation, altered flows, invasive species, and climate change.5,9 In addition, chemical pollution has been shown to cause detrimental effects on freshwater communities and their functions.9,13 While the main drivers and their interactions leading to biodiversity loss are complex and multifaceted, it is crucial to discern the primary causes to identify and implement effective mitigation strategies.5
Protecting these valuable ecosystems and maintaining the goods and services they provide to humans necessitates understanding how human activities disrupt natural processes and implementing conservation and legislative actions to halt their increasing degradation.14,15 While classical methods in biomonitoring, based on the presence or absence of specific taxonomic groups, have been useful for assessing the general health status of ecosystems, their interpretation can be challenging when deriving appropriate restoration measures due to high variability and technical difficulties. Recent scientific progress has introduced novel genomics approaches and technologies with high potential to revolutionize ecosystem health assessments, such as eDNA barcoding (i.e., eDNA used for individual species identification/detection; e.g., Helmer et al., 2023 (ref. 16)), eDNA metabarcoding (i.e., simultaneous detection of multiple species in an environmental sample using amplicon sequencing; e.g., Sales et al., 2020 (ref. 17)), metagenomics (i.e., study of genetic material directly recovered from environmental samples through shotgun sequencing that involves the direct sequencing of total eDNA; e.g., Rehman et al., 2020 (ref. 18)), or metatranscriptomics (i.e., analysis of RNA transcripts from communities to assess their gene expression and metabolic activities; e.g., Bergsveinson et al., 2020 (ref. 19)). Addressing the question of how recent technological advances can better elucidate the effects of environmental pollution on biodiversity in freshwater ecosystems and facilitate the identification of stressors to aid in determining appropriate management measures is the focus of this paper. The subsequent sections (1) outline conventional methods employed in biomonitoring and biodiversity assessments, emphasizing their constraints, (2) introduce the field of molecular biological investigation of taxonomic and functional biodiversity, and (3) explore how these emerging techniques can contribute to the assessment of the ecological status of freshwater systems.
2. Monitoring of freshwater ecosystems: traditional approaches & their limitations
The severe threats numerous freshwater ecosystems face worldwide have prompted increased recognition of the urgent need for their protection and restoration by policymakers and managers. Consequently, various regional, national, and international environmental policies have been developed, such as the Clean Water Act in the United States (CWA, 33 U.S.C. §1251 et seq. 1972), the Canadian Environmental Protection Act (S.C. 1999, c. 33), the Urban Wastewater Treatment Directive (91/271/EEC), and the European Union (EU) Water Framework Directive (WFD, 2000/60/EC).20 However, to effectively enforce legislation through appropriate management measures, it is crucial to efficiently and objectively assess the health status of freshwater ecosystems and quantify the impacts that natural and anthropogenic stressors have on these systems.
For aquatic ecosystems, the assessment of their status as well as their rate of change commonly involves physical, chemical, and biological measurements, as these parameters are intended to provide the full range of information needed to enable decision-making for improving ecological status and ensuring long-term sustainable use.21,22 The chemical status of freshwater ecosystems can be assessed by monitoring the presence and concentrations of chemical constituents. However, the sole quantification of chemical constituents does not necessarily allow to predict biological hazards or risks due to the complex dynamics of exposure in natural systems. Hence, an assessment of the biological status (i.e., through biomonitoring) is required.23 Consequently, the assessment of bioindicators plays a pivotal role in environmental protection strategies. Bioindicators, characterized as organisms or biological responses that mirror the health of an ecosystem and its reactions to environmental shifts, serve as integrative indicators of a given ecosystem's physical and chemical attributes across temporal and spatial scales.24
The incorporation of ecosystem integrity to guide management decisions (e.g., biological quality elements under the EU WFD, or valued components (VC) under the Canadian Environmental Assessment Act, (2012)) represents an important shift in the way surface water status is assessed and has led to substantial improvements in understanding how ecosystems respond to natural as well as anthropogenic stress. However, the past decades have also revealed significant limitations of traditional biomonitoring approaches (e.g., lack of sufficiently informative indicators, low resolution, time and cost-intensive). Against the backdrop of increasing pressure on our freshwater ecosystems, it is therefore of particular value to address these limitations and further improve the biomonitoring process. In the subsequent sections, major challenges and uncertainties, but also opportunities for future biomonitoring approaches are discussed.
2.1 Low resolution and emerging threats in freshwater ecosystems
Methods currently used for ecosystem biomonitoring follow a traditional approach based on sampling at the local scale that is often limited to specific groups of organisms, such as macroinvertebrates, algae, and fish. The assessment of these organisms is based on their direct observation, which is costly in time and resources and depends on taxonomic expertise, which has become increasingly rare in recent years. The premise underlying this approach is that the presence or absence of certain indicator species at a given site reflects its environmental quality. In the past, particular emphasis was placed on nutrient pollution and the associated eutrophication of water bodies as a dominant factor of stress.25 This is reflected by the classical biomonitoring methods that are still in use today. Many biomonitoring metrics are based on known or assumed oxygen demands of individual species, which in turn are often derived from the former saprobic system, including algae, invertebrates, mollusks, and to some extent fish.20 The saprobic system is a biological classification system that is used to describe the oxygen content of watercourses, which is significantly influenced by the degree of pollution with organic constituents (i.e. nutrients) that are biodegradable under oxygen depletion. Different pollution intensities lead to unique biotic communities. Pollution levels are evaluated by the saprobic system through analyzing the respective biotic communities both in terms of their composition and abundance, allowing for categorization based on saprobic index values at different locations.26 However, the saprobic system has limited applicability to assessing the increasing contamination with emerging pollutants, including pharmaceutical and personal care products (PPCPs), pesticides, endocrine-disrupting compounds (EDCs), nanomaterials, and microplastics, have been identified recently. These pollutants pose a challenge for traditional assessment methods because they represent a more complex array of stressors beyond the traditional concerns associated with nutrient pollution. Consequently, many monitoring studies now lack sufficiently informative indicators,27–29 and the number of species considered is rarely large enough to identify and isolate specific stressors,30 as the information content of classical biomonitoring studies is insufficient to map comparable variability. This issue is exacerbated by financial and time constraints, which lead to a further reduction in taxonomic depth by limiting the determination to a specific taxonomic level (e.g., family-level identification).30 As a result, the spatial and temporal coverage of biomonitoring programs is often limited.25 Furthermore, focusing exclusively on specific taxonomic groups as indicators does not provide coverage of the full range of species and their interactions within an ecosystem. Therefore, the consequences of certain stressors can be overseen and rarely be predicted, as they can have unintended consequences on disregarded taxa that propagate across the network of species interactions at different spatial and temporal scales.31
Biomonitoring studies must adequately reflect the state of the ecosystem, requiring holistic approaches. Understanding biodiversity patterns as well as their dynamics is crucial to protect rare species or to mitigate the consequences of shifts in species distributions to ensure the conservation and sustainable management of these ecosystems in the long term. Given the research findings of recent years, the current dominance of taxonomy-based community metrics will undoubtedly be increasingly challenged. Powerful molecular techniques based on new sequencing technologies have not only begun to provide alternative species recognition tools but are also giving scientists unprecedented new insights into the hidden biological diversity within ecosystems, that is not visible to the naked eye.
2.2 The structure–function discrepancy
Besides the lack of depth of information discussed in the previous section, common monitoring approaches mostly focus on structural changes but do not include functional changes. The term “structure” in the context of ecosystem monitoring refers to the physical and chemical conditions as well as the biological structure, such as biodiversity and/or community composition in a given ecosystem. Linked to this is ecosystem functioning, which has become a prominent term in ecology. Yet, the term “function” has been used with a variety of meanings in the past. Function may refer to multiple processes occurring within the ecosystem, the roles of individual species, the functioning of the ecosystem itself as a complex system of interactions, or the rates, patterns, and relative importance of ecosystem services provided by them.32,33 Ecosystem services thereby are defined as “the benefits people obtain from ecosystems”,5 including provisioning services (e.g., food, fibre, biochemicals, fresh water), regulating services (e.g., air quality regulation, climate regulation, water regulation, pollination, pest regulation), cultural services (e.g., recreation and ecotourism, educational values, aesthetic values), and supporting services (e.g., soil formation, photosynthesis, primary production, nutrient cycling, water cycling).
As described within the Millennium Ecosystem Assessment (2005), biodiversity depends on structural and functional variability, which is why information on both is required to adequately characterize ecosystem health. The importance of this becomes particularly clear given that some stressors can cause changes in structure but not in function, and vice versa.32,34 Although it seems intuitively plausible that a diverse community supports the maintenance of a variety of ecosystem services, research has shown that focusing on structural parameters is not sufficient to predict the consequences of biodiversity loss on ecosystem services.35,36 Even though structure and functioning are influencing each other, their relationship is not straightforward. Commonly, a positive biodiversity and ecosystem functioning relationship is suggested and three primary mechanisms are thought to result in beneficial effects of diversity on ecosystem processes: (1) niche complementarity, (2) positive selection effects, and (3) facilitation. The positive selection effect (also referred to as the sampling effect) describes the increased probability of species occurrence with very specific properties within species-rich communities.37 In contrast, niche complementarity occurs when species show niche differences and/or facilitative interactions. They may differ in their resource requirements, their ratios of amounts of resource types needed, and their temporal and spatial exploitation of resources leading to a more complete use of environmental resources.38,39 An example would be the coexistence of plant species as a result of niche partitioning resulting in a higher production of biomass. Facilitation describes the effect of higher productivity of an individual when there are interspecific neighbours that modify the environment in a favourable way, usually through resource enhancement or mitigation of stress perturbations.38 An example would be the occurrence of mycorrhizal fungi leading to a higher net primary production in terrestrial ecosystems as these species increase nutrient availability. In the past, niche complementarity and facilitation have been collectively referred to as complementarity, as it is often difficult to determine which mechanism prevails.40 Previous studies, however, also showed negative selection effects due to higher biodiversity.41 In this case, an increasing number of competing organisms, i.e., an increase in biodiversity, can lead to the loss of so-called keystone species. Despite their relatively low abundances within the ecosystem, these species are thought to be essential for specific ecosystem functions and can therefore exert a disproportionally high impact on ecosystem properties.41,42 It is despite this that clear functional metrics are still scarce in biomonitoring, especially in aquatic ecosystems, although their importance for ecosystem health has already been recognized by policymakers.20,43
The only functional metrics that are used routinely as criteria for ecosystem quality today are the biochemical oxygen demand (BOD) and the sediment oxygen demand (SOD) giving a measure of the potential to consume oxygen at a standard temperature.32,44 One of the most important ecosystem services provided by freshwater ecosystems is organic matter degradation, which is an essential component of trophic dynamics. Hence, corresponding measures have been proposed in the past as important additional sources of information in biomonitoring.45 Measurement of oxygen is one of the most commonly used functional metrics in this regard as it integrates metabolic activity in aquatic ecosystems.46,47 Community respiration (CR) and gross primary productivity (GPP) measurements allow inferences to be made about net oxygen turnover, which is an indicator of the relative balance between carbon productivity and mineralization, and therefore, ecosystem metabolism. As autotrophs, aquatic plants and phytoplankton—including algae, cyanobacteria, and a few other bacteria—produce organic carbon, which serves as a food source for other organisms. The process of respiration is driven by all heterotrophic organisms within an ecosystem, including fish, invertebrates, algae, aquatic plants, and microbes. Accordingly, a higher GPP represents net autotrophy, as more oxygen is produced than consumed, while a higher ER value reflects net heterotrophy.47–49 In addition, their ratio allows conclusions to be drawn about the ratio of the two main energy sources in river ecosystems: (1) primary production by photosynthesis and (2) terrestrial organic matter entry. A heterotrophic stream, with higher respiration rates than primary production, must be supported by inputs of organic matter from upstream regions or the surrounding catchment. These processes are known to be sensitive to environmental stressors and at the same time easy and inexpensive to measure, making them interesting for assessing functional changes in ecosystem metabolism.32 In addition to the measurement of GPP and CR, most other measures of ecosystem processes in freshwater ecosystems have focussed on leaf-litter decomposition (e.g., Gulis et al., 2006
50). Leaf-litter decomposition is governed by the activity of bacteria, fungi, and macroinvertebrates that may respond to anthropogenic stress in different ways. Both approaches have in common that they are rather integrative measures of ecosystem health. Nevertheless, these processes do not necessarily respond to pressures in the same way since they are underlying a complex network of different actors and direct as well as indirect interactions. By focusing on fluxes of biomass, nutrients, or energy between often coarse aggregations of functional units that often contain unrelated groups of taxa (e.g., cyanobacteria, diatoms, and plants grouped as primary producers), it is not possible to draw conclusions about the link between structure and function.51 Clear causal relationships between specific stressors and observed changes in structure and function can therefore rarely be derived. Focusing on these simple rates (e.g., litter decomposition) also risks underestimating the consequences of biodiversity loss required to maintain multifunctional ecosystems when the species that drive them are functionally unique, or some degree of functional redundancy occurs for specific processes.20,52–54
To adequately capture ecological complexity and assess the ecological status of highly variable systems new monitoring strategies are needed which incorporate both community structure and system functioning while being cost-effective.29 Recent breakthroughs in omics technologies have paved the way for promising methodologies in this domain. Consequently, the following section will introduce next-generation sequencing (NGS) techniques and explore their potential to profoundly alter the landscape of biodiversity assessment in biomonitoring.
3. The NGS revolution: integrating molecular insights into biomonitoring
Beginning with the release of the first truly high-throughput sequencing platform, the Roche 454 GS 20 sequencer in 2005,55 DNA and RNA sequencing technologies have undergone a rapid evolution. Also known as next-generation sequencing (NGS) platforms, these new systems can deliver billions of nucleotide reads in a single experiment, by allowing massively parallel sequencing – an improvement of at least five orders of magnitude over traditional Sanger sequencing.56 Because of this dramatic leap in capacity, a new field of nucleic acid-based research has emerged, analyzing DNA or RNA found in environmental samples. The examination of this genetic material may provide information about the organisms present in the ecosystem under study, which is the objective of both eDNA metabarcoding and eDNA barcoding. The origin of this genetic material varies depending on the taxonomic group under investigation, including sources such as feces, mucus, skin cells, organelles, gametes, extracellular DNA, or even living cells like bacteria.56 Both approaches have in common that they are investigating certain barcodes, i.e., short characteristic sequences within the genome of the targeted taxa. These sequences can be identified using specific primer pairs which can bind to the regions preceding and succeeding the respective barcode.
With eDNA barcoding, the presence of a barcode specific to a taxonomic group is detected. This method has primarily been employed to detect invasive or rare species within the environment under study.57–59 Unlike metabarcoding, eDNA barcoding does not assess the exact sequence of the barcode; instead, it focuses solely on its occurrence. Consequently, barcodes utilized in eDNA barcoding studies must exhibit a high level of specificity to the taxon under investigation.
In contrast, eDNA metabarcoding enables the identification of multiple taxa without prior knowledge about the species likely present within the studied ecosystem.60–62 Metabarcoding employs marker genes to unveil the diversity and composition of specific taxonomic groups in an environment (see Fig. 1). Key marker genes used in microbial ecology include the 16S ribosomal RNA (rRNA) gene (for analyzing Archaea and bacteria),63 the internal transcribed spacer (ITS) region (for characterizing fungal communities),64 and the 18S rRNA gene (for reporting eukaryotes' occurrence).65 These markers exhibit high inter- and low intraspecific variability, crucial for accurately capturing genetic diversity within environmental samples. By comparing these sequences from specimens of unknown identity within eDNA samples to reference sequences, taxonomic inferences can be drawn. Consequently, metabarcoding presents a novel avenue for investigating community composition and biodiversity within biomonitoring studies. Moreover, it serves as a valuable tool for overcoming key limitations of classical morphological identification, including the identification of species exhibiting high phenotypic plasticity and the recognition of morphologically cryptic species,66 or the identification of life stages that are difficult to determine (e.g., larvae). This has led to the idea of “Biomonitoring 2.0”,30 where metabarcoding can be used in different ways to further improve ecosystem status assessment. One approach here is to use NGS methods to replace or support existing morpho taxonomy-based determination, e.g., for diatoms67–70 or macroinvertebrates.71–73 Greater sensitivity of DNA-based compared to traditional morpho taxonomy-based approaches has been illustrated previously.61,74,75
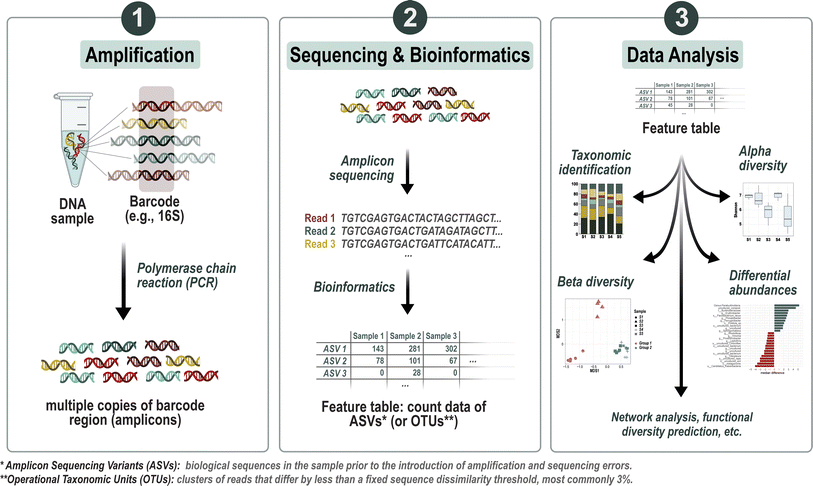 |
| Fig. 1 Overview of the metabarcoding analysis workflow. The process starts with the amplification of target marker genes (e.g., 16S rRNA for bacteria), followed by DNA sequencing to generate a pool of sequence reads. These reads undergo clustering or denoising steps to generate Operational Taxonomic Units (OTUs) or Amplicon Sequence Variants (ASVs), respectively, which represent unique microbial entities. Subsequent to clustering or denoising, bioinformatics tools are employed to process the raw data into a feature table, summarizing the abundances of ASVs (or OTUs) across samples. Taxonomic identification is then accomplished through comparison with reference databases, allowing for the classification of OTUs or ASVs. The resulting dataset can then be used for statistical analyses, including assessments of alpha and beta diversity, as well as other potential statistical tests tailored to uncover specific patterns or relationships within the microbial community data. | |
However, some limitations have so far prevented the implementation of metabarcoding in biomonitoring. A fundamental problem arises from the fact that NGS methods and classical morphotaxonomic methods sample different units of presence (molecules vs. individuals) resulting in different biases that affect estimates of richness, abundance, and taxonomic composition.76 Reasons for this are cryptic diversity,77 intragenomic or intraspecific marker variations,78,79 as well as the presence of DNA from dead and inactive organisms,80 or even extracellular DNA. No direct relationship has yet been found between the number of sequences obtained per taxon and the density and/or biomass of the species studied.81–83 Calibration and establishment of eDNA techniques is a rapidly growing area of research, and some promising methods to address these issues include applying correction factors to read counts based on knowledge of biovolume84 or spiking samples with known internal standards for quantitative determinations.85
One further possibility to use NGS methods in biomonitoring is the exploration of novel bioindicator taxa, such as bacteria86–90, Archaea87 and microbial eukaryotes.87,91 The inclusion of further taxa in the biomonitoring process may thus lead to a more holistic view and therefore address the issue of low resolution as discussed in Section 2.1.
3.1 Using metabarcoding to discover new microbial bioindicators
The use of microbial bioindicators in biomonitoring is still scarce although the assessment of microbial parameters has its provenance in biomonitoring.92 This is primarily due to methodological constraints which, however, could change profoundly in the near future with the rapid evolution of NGS techniques. In the past, it has been impossible to capture the entirety of the microbial community as more than 99% of the microorganisms observed in nature cannot generally be cultivated or phenotypically identified using conventional methods.93,94 Consequently, most microbial groups (e.g., bacteria, protozoa, viruses, or fungi), apart from algae and cyanobacteria, are largely understudied in the field of aquatic biodiversity. However, microorganisms are the most abundant living organisms on Earth. In addition, they possess key roles in a variety of biogeochemical cycles and ecosystem services.95–98 Individual microbes within a microbial community differ strongly in their metabolism and their sensitivity to environmental stressors. Moreover, rare microorganisms are important when it comes to disturbances as they may provide resistance and resilience.99–101 Microorganisms are therefore considered the “hidden backbone” of an ecosystem. Due to their sessile habits in sediment and biofilms, they are continuously exposed to the water column integrating the effects of stressors over extended periods of time.102 As a community, these populations of different species overlap in time and space interacting with each other and are referred to as an ecological unit. Accordingly, whole community assessment has some critical advantages over other bioindicators, as this approach allows the estimation of direct and indirect effects at a higher-tier level, as it simultaneously assesses the changes in ecological status in both structure and function.103,104 Evidence for this has likewise been provided in some studies, in which a greater sensitivity of microbial communities to stressors was observed than for individual species.105,106 Microbial communities may therefore be a better proxy for evaluating environmental impacts and increasing efforts have been made in the past to include metabarcoding data of microbial organisms in routine biomonitoring.99,107–110 In this respect, a bioindicator of pollution could be based on changes in microbial community composition (richness, or diversity) resulting from selection pressure exerted by a pollutant, or the occurrence of specific microbial taxa indicating a specific stressor. However, one of the most challenging aspects to translate into regulatory requirements is the inference of biotic indices/metrics as environmental quality assessment requires at least a basic knowledge of the ecological function of the identified taxa, which is generally unavailable for most microorganisms.111 To overcome this issue, alternative “taxonomy-free” or “de novo” sequence-centered approaches have been proposed recently.68,104,112,113 These approaches aim to establish new bioindicators using metabarcoding techniques and independently generated ecological status or known disturbance gradients, such as the effects of pesticides on freshwater invertebrates,114 benthic eukaryotes,115 diatoms,67 and bacteria.86,116,117 As shown by Apothéloz-Perret-Gentil et al. (2017)68 as well as by Feio et al. (2020),118 a de novo sequence-centric approach can significantly improve the accuracy of the assessment by using a larger number of taxa or OTUs (operational taxonomic units as a proxy for species in the absence of traditional systems of biological classification) for the analysis in comparison to an approach that relies solely on taxonomic assignments.
3.2 Linking microbial community composition & ecosystem functioning
Functional biodiversity, which encompasses the suite of functions performed by organisms in the ecosystem, has been identified in recent years as the missing link between biodiversity patterns and ecosystem functioning, especially for microorganisms.119–121 Various studies have shown a strong and well-supported linkage between microbial community structure and ecosystem functions;92,122,123 however, microbial functional ecology still lags behind its macrobial counterpart.119 In recent years, the field has seen the emergence of several tools designed to predict the functional gene content of microbial communities based solely on taxonomic composition derived from metabarcoding data. These tools include PICRUSt2 (Phylogenetic Investigation of Communities by Reconstruction of Unobserved States),124 which utilizes an ancestral state reconstruction approach to infer functional gene content from marker gene (e.g., 16S rRNA) sequencing data; Tax4Fun2,125 which employs computational methods to estimate functional capabilities based on taxonomic information obtained from metabarcoding data; or FAPROTAX126 assigning putative ecological functions to bacterial taxa based on known traits and functions compiled in a comprehensive database.
The problem with microbial functional diversity is that a wide range of traits observed in microbes cannot be summarized by a single marker gene, such as 16S, 18S, or ITS, which are generally used in microbial metabarcoding studies.119 This arises due to ecological incoherence, where inconsistency between microbial taxonomic classification and functional traits is common, owing to significant genomic variation even among closely related organisms.127–129 As a result, bacterial strains sharing identical 16S rRNA gene sequences may demonstrate limited niche overlap, manifesting in distinct growth rates and substrate utilization profiles.127 Furthermore, the depth of phylogenetic conservation for microbial traits varies.130 While functional traits involving only a few functional genes tend to occur at a shallow level in phylogenetic trees and are often not shared by all members of the given taxon, more complex traits involving multiple genes tend to be conserved at a higher level in phylogeny.130 The weaker phylogenetic signal for functional traits at shallower levels could be also due to the horizontal gene transfer occurring between prokaryotes,130 as simple traits are more likely to be carried on phages, plasmids, or transposons.119
Horizontal gene transfer, occurring through mechanisms like conjugation, transformation, or transduction, can uncouple functional traits from taxonomy. This is because taxonomy is primarily determined by the evolutionary history of microorganisms. Consequently, microorganisms can rapidly evolve through ecological and evolutionary adaptation to new niches. This diminishes the influence of evolutionary history and complicates our ability to explain ecosystem functioning solely through taxonomy. As an example, the analysis of soil samples derived from North American pine forests by Talbot et al. (2014) showed that, despite taxonomic differences among soil fungi, their cellulase activity exhibited remarkable consistency across varying scales, spanning from local to continental levels.131 This discovery underscores the prevalence of significant functional redundancy within these ecosystems. In contrast, there is evidence of functional plasticity, wherein taxonomically similar communities exhibit distinct functions, particularly evident in the rapid responses of microbial communities to environmental disturbances.132,133 These observations underscore the growing realization that microbial taxonomic and functional traits are somewhat decoupled, as they are influenced by markedly distinct processes.129
Furthermore, the availability of physiological, physical, and metabolic information remains limited, largely because many microbes have yet to be cultured to assess their functional properties. As a result, the unequivocal assignment of distinct functional traits to individual taxa has been achievable only for specialized and well-conserved functions, such as methanogenesis.119,130 This difficulty in linking specific taxa with functional traits in the case of microorganisms has led to the idea of community-centered, rather than taxa-centered approaches. By focusing on a higher level of phylogenetic organization, the confounding effects of horizontal gene transfer at lower levels can be minimized. However, research indicates that there is no clear taxonomic resolution level that directly correlates with functional differentiation, particularly given the redundancy of microbial traits.134
The issue of functional redundancy within the diverse microbial community has prompted the consideration of a departure from a taxonomic perspective to a more thorough examination of functional properties. The search for bioindicators is essentially a classification challenge: ecosystems are classified based on their inherent community of organisms to identify environmental impacts that may compromise ecosystem services. While historically this classification has relied on phylogenetic data (species composition), the fundamental determinants are the functional characteristics of the community members, which dictate their ability to compete within the ecosystem. This raises the question: Instead of focusing on who provides these functions, would it not be more beneficial to classify environmental samples directly based on the functional properties of the community, particularly concerning the preservation of ecosystem services? Regarding microbial communities, the focus on functional characteristics could thus provide a higher discriminatory power as there is likely less noise interpreting functional profiles since a given anthropogenic disturbance might trigger a similar response across multiple taxa.135 Therefore, functional profiles may be less sensitive to biogeographical effects, demographic shifts, and species dispersal limitations.104 The focus here lies on the presence and abundance of functional genes and pathways within the metagenome or the metatranscriptome of a community.
3.2.1 Assessing functional diversity and activity of microbial communities.
To assess the metagenome of a community through shotgun sequencing (metagenomics), the DNA of all cells in this community is extracted, just like for metabarcoding. But instead of targeting specific marker genes (e.g., 16S), all DNA is sheared into small fragments which are sequenced separately followed by the reconstruction of them into consensus sequences (see Fig. 2). This way, coding sequences that provide information about biological functions are assessed, in addition to taxonomically informative regions, such as 16S.136
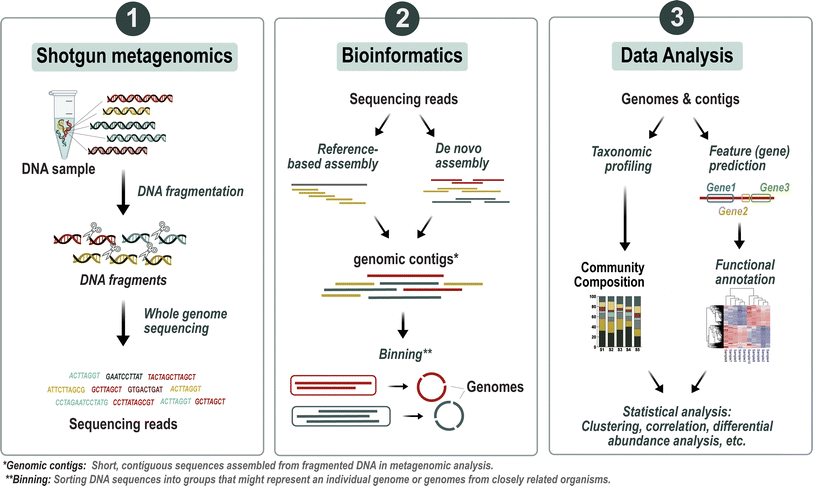 |
| Fig. 2 Overview of the metagenomic analysis workflow. The process begins with breaking down the DNA into smaller fragments through fragmentation, followed by employing high-throughput sequencing to produce short DNA sequences. These sequences are then utilized in either reference-based or de novo assembly techniques to reconstruct genomic contigs, which represent segments of microbial genomes. After assembly, the contigs are analyzed for taxonomic profiling, categorizing them into specific taxonomic groups. Furthermore, functional profiling is carried out to annotate the genes and pathways within the microbial communities. Subsequently, statistical analyses are applied to the data to unveil significant associations with environmental or clinical parameters. | |
The assessment of the whole metagenome therefore provides insight into community biodiversity and function answering the question: Who is there and what are they capable of doing? In the past, this approach has led to a tremendous increase in information about energy and nutrient cycling, genome structure, gene function, population genetics, and lateral gene transfer between members of an uncultured community.137–139 In addition, metagenomics has enabled the discovery of new genes and gene products. As an example, Béjà et al. (2000) discovered photoorganotrophy as a new metabolic pathway in marine bacteria that allows them to obtain energy from the sun when carbon from organic material is limited.140 Another great example of the potential of metagenomics is the work of Venter et al. (2004), who analyzed seawater samples from the Sargasso Sea and discovered 148 previously unknown bacterial phylotypes and about 70
000 new genes spanning a wide range of biogeochemical pathways.141 However, the analysis of metagenomics data is complicated due to the complex structure of the data and the high computational power required (Table 1). In addition, depending on the environment, some communities are so diverse that most genomes are not fully represented by reads, making it impossible to reconstruct all genes by sequence alignment.142
Table 1 Comparison of metabarcoding, metagenomics, and metatranscriptomics
|
Metabarcoding |
Metagenomics |
Metatranscriptomics |
Target biomolecules |
Specific DNA regions (barcodes) |
Entire genomic DNA (metagenome) |
Transcribed RNA (metatranscriptome) |
Taxonomic resolution |
High (species to genus level) |
Broad (species and functional) |
Moderate to high (active species) |
Functional information |
Limited |
Comprehensive |
Comprehensive |
Environmental status assessment |
Assessing biodiversity changes in ecosystems |
Provides insights into community structure and functional potential of microbial populations |
Offers information on active microbial processes and responses to environmental changes |
Data analysis complexity |
Moderate |
High |
Moderate to high |
Targeting specific functional genes is another way to assess the functional potential of microbial communities. A large number of functional genes have been extensively studied and their corresponding primers have been validated, allowing their evaluation by quantitative polymerase chain reaction (qPCR).99 Examples of such genes are, e.g., the cbbl gene and the cbbM gene encoding the large ribulose-1,5-bisphosphate carboxylase/oxygenase (RuBisCO) subunit form I (cbbl) and II (cbbM), a key enzyme of the Calvin–Benson cycle of autotrophic CO2 fixation;98,143 the pufM gene, encoding the M subunit of the reaction center of aerobic anoxygenic photosynthesis in γ-Proteobacteria;144 the mcrA (methyl coenzyme-M reductase) gene to assess methanogenesis in Euryarchaeota;145 or narG, encoding the membrane-bound nitrate reductase, to assess denitrification,146 to name a few examples. A potential approach for conducting high-throughput functional community profiling based on known functional genes involves the utilization of microarrays. These arrays can be customized to evaluate the functional capabilities of communities, which are crucial for specific biogeochemistry, ecological, and environmental investigations. Several types of microarrays have been created for studying microbial communities, encompassing both phylogenetic and functional methodologies. One widely used microarray for investigating biogeochemical, ecological, and environmental processes is the GeoChip which has since been updated to the 5.0 version.147,148 Compared to sequencing techniques, microarrays offer targeted analysis and high-throughput capabilities, making them cost-effective options for functional gene profiling. However, they may suffer from limited coverage and potential biases in probe design.
Although eDNA metabarcoding and metagenomics have revealed a great number of novel insights into microbial community composition and their functional potential, they only describe the presence of organisms or genes but not whether they are active members within these communities.149 It has thus been suggested that metatranscriptomics can derive a more accurate picture of the functional response of microbial communities to changing environmental conditions.150 Metatranscriptomics is defined as the study of gene expression of microbes within natural environments, i.e., the metatranscriptome, using RNA sequencing (RNAseq). So other than metagenomics, which focuses on the genomic content and therefore functional potential of a community, metatranscriptomics can be used to survey active genes and their expression levels and therefore helps answer the question “What genes are collectively expressed under different conditions?”.150 It is obtained by capturing the total mRNA of the microbiome, its reverse transcription into cDNA (complementary DNA), and subsequent whole metatranscriptomics shotgun sequencing (see Fig. 3). Usually, the resulting reads or assembled contigs (a set of overlapping DNA segments that together represent a consensus region of DNA) are used to deduce the relative expression of certain genes.151 Furthermore, after annotation, enzymatic functions can be mapped to known metabolic pathways using tools such as MINPATH152 or IPATH.153 This way, functional changes in response to changing environmental conditions can be assessed (Table 1).
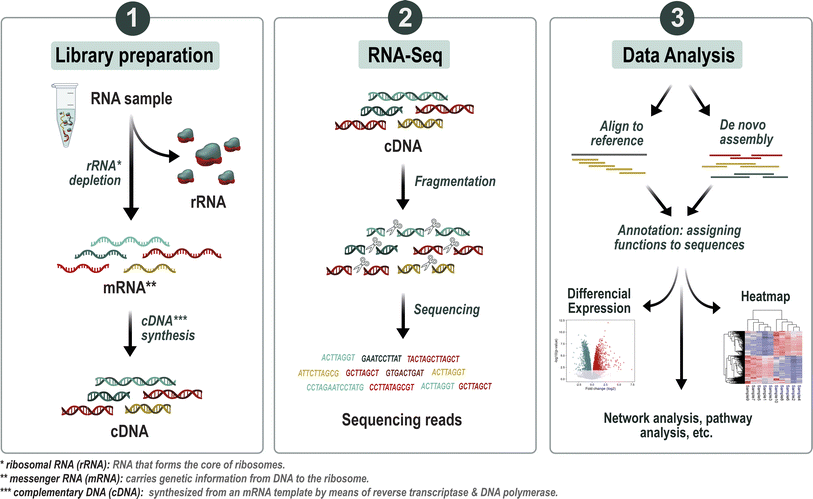 |
| Fig. 3 Overview of the metatranscriptomics analysis workflow. First step after RNA extraction is ribosomal RNA (rRNA) depletion leading to an enrichment of messenger RNA (mRNA), thereby improving the detection and analysis of gene expression. The purified mRNA is then reverse transcribed into complementary DNA (cDNA), a process that converts RNA into a stable DNA form. Subsequently, the cDNA undergoes high-throughput sequencing to produce short sequence reads. These reads are assembled into transcripts, which represent the expressed genes in the microbial community. Annotation tools are applied to these transcripts, assigning functional labels to elucidate their biological roles. Following annotation, statistical analyses such as differential expression analysis are employed to discern gene expression patterns. Visualization techniques, including heatmaps or volcano plots, are then utilized to explore and depict differential expression patterns across various experimental conditions or environmental gradients. | |
A good example of the advantages of metatranscriptomics is the study of Yergeau et al. (2012). In this study, functional gene expression was investigated to characterize the response of freshwater biofilms to four pharmaceuticals (erythromycin, gemfibrozil, sulfamethazine, and sulfamethoxazole) at low concentrations.154 Bacterial composition was found to change very slightly, but the expression of gene categories related to the N, P, and C cycles was strongly affected, highlighting the importance of capturing functional changes to better understand the mechanistic link between ecosystem stressors and the impairment of ecosystem processes.
In summary, both metagenomics and metatranscriptomics provide direct avenues for evaluating the functional diversity and activity of microbial communities, enabling the application of functional environmental genomics (Table 1). These approaches address the challenge of taxon-function decoupling discussed earlier, and previous studies have highlighted the effectiveness of certain metagenomic contigs and functional transcripts as bioindicators of anthropogenic pollution,155–158 underscoring the potential of these methods. This understanding could be integrated into predictive models to anticipate shifts in biodiversity and their potential impacts on ecosystem services across various disturbance scenarios. However, the practical development of an ecosystem monitoring framework based on this theoretical ecological research is ongoing, with only partial experimental validation accomplished thus far.159 While discussing the implementation of these strategies is premature, it is crucial to recognize and anticipate their ecological benefits. Additionally, as more datasets accumulate, significant progress towards realizing these opportunities can be expected.
3.3 Navigating challenges in integrating NGS methods for aquatic biomonitoring
As discussed in the previous sections, NGS methods hold great potential to address key limitations of traditional monitoring. Nonetheless, the implementation of NGS methods into aquatic biomonitoring faces several key challenges that must be overcome for successful data integration and interpretation. The resulting data from NGS methods are fundamentally different than from those generated during traditional biomonitoring and therefore require tailored workflows for data analysis. Data produced by the NGS methods described present various analytical challenges, such as their (1) compositional nature (the relative abundances of different species or genes are interdependent due to the fixed total amount of sequencing data obtained from a sample, leading to spurious correlations and challenges in interpreting absolute abundance levels), (2) sparsity (many species or genes are represented by only a few sequences which can make it challenging to accurately assess the abundance or presence of rare species or transcripts in the sample), (3) over-dispersion (the variance of the data is higher than would be expected based on a particular statistical model, that can be caused by factors such as uneven distribution of species or genes across samples, or by technical variability introduced during sequencing and data processing), (4) high dimensionality (high number of dimensions, such as different species or genes), (5) multi-collinearity (two or more variables, e.g., species or genes, are highly correlated with each other which complicates statistical analyses such as regression models, as it can lead to unstable parameter estimates and difficulties in determining the true relationships between variables), (6) multivariance, and (7) high variability.
These analytical challenges highlight the need for robust data processing pipelines, advanced statistical methods, and bioinformatics tools specifically designed to address these issues. Researchers working in this field must carefully consider these challenges when designing experiments, collecting samples, performing sequencing, and analyzing the resulting data to obtain accurate and meaningful insights into environmental biodiversity, ecosystem health, and responses to environmental changes. The challenge of data sparsity is particularly relevant in environmental studies focused on the conservation of rare species. One significant limitation of NGS in this context is the requirement for deep sequencing to detect and quantify rare taxa accurately, which can be cost-prohibitive. Additionally, biases introduced during DNA/RNA extraction, PCR amplification, and library preparation can disproportionately affect rare species, further complicating their detection. Current bioinformatics approaches also struggle with distinguishing true rare species from sequencing artifacts.160,161 Prospective advancements, such as improved sample preparation techniques, the development of more sensitive and specific primers, and enhanced bioinformatics algorithms, are necessary to mitigate these limitations.
Furthermore, standardization of protocols, data formats, and analytical workflows is essential for ensuring data reproducibility and comparability across diverse studies. Collaborative initiatives among researchers, institutions, and regulatory bodies are pivotal in developing standardized guidelines and best practices for sample collection, DNA/RNA extraction, library preparation, and data analysis in NGS-based biomonitoring. These collaborative efforts will strengthen the reliability and utility of NGS methods in advancing our understanding of aquatic ecosystems and supporting informed conservation and management decisions.
Finally, to effectively integrate NGS data into risk management and surpass simple descriptive models of ecosystem structure and change, it becomes imperative to construct explanatory and predictive models concerning ecosystem function and services. Exploring, testing, and comprehending these models, potentially harnessing advancements in artificial intelligence, will signify a paradigm shift in biomonitoring. This evolution involves transitioning from conventional concepts of ecosystem indicators and indices, which lack explanatory power regarding ecosystem changes, toward holistic models that offer a comprehensive understanding of ecological dynamics. These advanced models not only elucidate the underlying mechanisms driving ecosystem functions but also acknowledge the intricate and dynamic nature of ecosystems, encompassing all trophic levels and their interdependencies. Consequently, biomonitoring must evolve from being purely descriptive to serving as a proactive risk assessment tool, grounded in novel hypotheses and robust predictive models.
4. Conclusion
NGS techniques have revolutionized the study of communities, allowing for the precise identification of both taxonomic and functional traits at exceptionally detailed levels. This advancement has opened new avenues for integrating microbial data into traditional freshwater biomonitoring practices, offering profound insights into ecosystem health and functionality. However, realizing the full potential of these techniques necessitates overcoming various challenges, including ensuring data quality and standardization, navigating complex bioinformatic analyses, addressing spatial and temporal variations, mitigating sampling biases, and validating interpretations. Such endeavors require interdisciplinary collaboration and concerted efforts from researchers across different fields. Furthermore, transitioning towards evaluating functional diversity, rather than solely relying on compositional datasets, holds great promise for enhancing biomonitoring efforts. By focusing on the functional capabilities of microbial communities, researchers can develop a more comprehensive understanding of ecosystem dynamics and responses to environmental changes. Combining microarray technology with NGS data provides an exciting opportunity to enhance biomonitoring efforts. NGS datasets offer in-depth insights into microbial gene diversity, facilitating the identification of specific target sequences ideal for developing microarray probe sets. This integration not only validates results obtained from microarray analysis but also broadens the range of targets, thereby enhancing the reliability of biomonitoring methodologies.
In conclusion, the utilization of NGS technologies and the integration of a functional perspective hold immense promise for advancing freshwater biomonitoring and ecosystem management. The capacity to observe the entire ecosystem as a unified entity rather than isolated components, with enhanced detail, precision, and accuracy, enables a shift towards a holistic approach in ecosystem science. Embracing these advancements offers the potential to inform decisions and implement precise conservation strategies, thereby safeguarding freshwater ecosystems for the well-being of both current and future generations.
Conflicts of interest
The authors declare no competing financial interest.
Acknowledgements
This work was funded by the Global Water Futures program; Canada First Research Excellence Fund (CFREF; award number 419205). Additional information is available at https://www.globalwaterfutures.ca. Additional funding was provided to M. B. and M. H. through the Discovery Grants program of the Natural Sciences and Engineering Research Council of Canada (NSERC). M. H. was supported by the Canada Research Chairs Program.
References
- B. Lehner and P. Döll, Development and validation of a global database of lakes, reservoirs and wetlands, J. Hydrol., 2004, 296(1–4), 1–22 CrossRef.
-
E. V. Balian, H. Segers, K. Martens, and C. Lévéque, The Freshwater Animal Diversity Assessment: an overview of the results, in Freshwater Animal Diversity Assessment, Springer Netherlands, Dordrecht, 2007, pp. 627–37 Search PubMed.
- P. A. Harrison, P. M. Berry, G. Simpson, J. R. Haslett, M. Blicharska and M. Bucur,
et al., Linkages between biodiversity attributes and ecosystem services: A systematic review, Ecosyst. Serv., 2014, 9, 191–203 CrossRef.
- J. S. Albert, G. Destouni, S. M. Duke-Sylvester, A. E. Magurran, T. Oberdorff and R. E. Reis,
et al., Scientists' warning to humanity on the freshwater biodiversity crisis, Ambio, 2021, 50(1), 85–94 CrossRef PubMed.
-
Millennium Ecosystem Assessment, Ecosystems and Human Well-Being: Synthesis, Island Press, Washington, DC, 2005 Search PubMed.
- S. L. Pimm, C. N. Jenkins, R. Abell, T. M. Brooks, J. L. Gittleman and L. N. Joppa, The biodiversity of species and their rates of extinction, distribution, and protection, Science, 2014, 344(6187), 987 CrossRef CAS PubMed.
- O. E. Sala, F. Stuart Chapin III, J. J. Armesto, E. Berlow and J. Bloomfield,
et al., Global Biodiversity Scenarios for the Year 2100, Science, 2000, 287(5459), 1770–1774 CrossRef CAS PubMed.
- A. Ricciardi and J. B. Rasmussen, Extinction Rates of North American Freshwater Fauna, Conserv. Biol., 1999, 13(5), 1220–1222 CrossRef.
- C. J. Vörösmarty, P. B. McIntyre, M. O. Gessner, D. Dudgeon, A. Prusevich and P. Green,
et al., Global threats to human water security and river biodiversity, Nature, 2010, 467(7315), 555–561 CrossRef PubMed.
-
J. Sendzimir, and S. Schmutz, Challenges in Riverine Ecosystem Management, in Riverine Ecosystem Management, Springer International Publishing, Cham, 2018, pp. 1–16 Search PubMed.
- A. J. Reid, A. K. Carlson, I. F. Creed, E. J. Eliason, P. A. Gell and P. T. J. Johnson,
et al., Emerging threats and persistent conservation challenges for freshwater biodiversity, Biol. Rev., 2019, 94(3), 849–873 CrossRef PubMed.
- B. Malmqvist and S. Rundle, Threats to the running water ecosystems of the world, Environ. Conserv., 2002, 29(2), 134–153 CrossRef.
- M. Meybeck, Global analysis of river systems: from Earth system controls to Anthropocene syndromes, Philos. Trans. R. Soc. Lond. B Biol. Sci., 2003, 358(1440), 1935–1955 CrossRef CAS PubMed.
- M. J. Benotti, R. A. Trenholm, B. J. Vanderford, J. C. Holady, B. D. Stanford and S. A. Snyder, Pharmaceuticals and Endocrine Disrupting Compounds in U.S. Drinking Water, Environ. Sci. Technol., 2009, 43(3), 597–603 CrossRef CAS PubMed.
- D. Dudgeon, A. H. Arthington, M. O. Gessner, Z. Kawabata, D. J. Knowler and C. Lévêque,
et al., Freshwater biodiversity: importance, threats, status and conservation challenges, Biol. Rev., 2006, 81(2), 163–182 CrossRef PubMed.
- N. Helmer, C. Hörweg, H. Sattmann, S. Reier, N. U. Szucsich and J. Bulantová, DNA Barcoding of Trichobilharzia (Trematoda: Schistosomatidae) Species and Their Detection in eDNA Water Samples, Diversity, 2023, 15(1), 104 CrossRef CAS.
- N. G. Sales, M. B. McKenzie, J. Drake, L. R. Harper, S. S. Browett and I. Coscia,
et al., Fishing for mammals: Landscape-level monitoring of terrestrial and semi-aquatic communities using eDNA from riverine systems, J. Appl. Ecol., 2020, 57(4), 707–716 CrossRef CAS.
- Z. U. Rehman, L. Fortunato, T. Cheng and T. Leiknes, Metagenomic analysis of sludge and early-stage biofilm communities of a submerged membrane bioreactor, Sci. Total Environ., 2020, 701, 134682 CrossRef CAS PubMed.
- J. Bergsveinson, J. Roy, C. Maynard, S. Sanschagrin, C. N. Freeman and G. D. W. Swerhone,
et al., Metatranscriptomic Insights Into the Response of River Biofilm Communities to Ionic and Nano-Zinc Oxide Exposures, Front. Microbiol., 2020, 11, 104 CrossRef PubMed.
-
N. Friberg, N. Bonada, D. C. Bradley, M. J. Dunbar, F. K. Edwards, and J. Grey, et al., Biomonitoring of Human Impacts in Freshwater Ecosystems: The Good, the Bad, and the Ugly, in Advances in Ecological Research, 2011, pp. 1–68 Search PubMed.
- J. L. Metcalfe, Biological water quality assessment of running waters based on macroinvertebrate communities: History and present status in Europe, Environ. Pollut., 1989, 60(1–2), 101–139 CrossRef CAS PubMed.
- L. Li, B. Zheng and L. Liu, Biomonitoring and Bioindicators Used for River Ecosystems: Definitions, Approaches and Trends, Procedia Environ. Sci., 2010, 2, 1510–1524 CrossRef.
- G. A. Burton, The focus on chemicals alone in human-dominated ecosystems is inappropriate, Integr. Environ. Assess. Manage., 2017, 13(4), 568–572 CrossRef PubMed.
- S. Birk, W. Bonne, A. Borja, S. Brucet, A. Courrat and S. Poikane,
et al., Three hundred ways to assess Europe's surface waters: An almost complete overview of biological methods to implement the Water Framework Directive, Ecol. Indic., 2012, 18, 31–41 CrossRef.
- J. Pawlowski, M. Kelly-Quinn, F. Altermatt, L. Apothéloz-Perret-Gentil, P. Beja and A. Boggero,
et al., The future of biotic indices in the ecogenomic era: Integrating (e)DNA metabarcoding in biological assessment of aquatic ecosystems, Sci. Total Environ., 2018, 637–638, 1295–1310 CrossRef CAS PubMed.
-
S. Zahradkova, and T. Soldan, Saprobic System, in Reference Module in Earth Systems and Environmental Sciences, Elsevier, 2013 Search PubMed.
- S. A. Cushman, K. S. McKelvey, B. R. Noon and K. McGarigal, Use of Abundance of One Species as a Surrogate for Abundance of Others, Conserv. Biol., 2010, 24(3), 830–840 CrossRef PubMed.
- S. J. Andelman and W. F. Fagan, Umbrellas and flagships: Efficient conservation surrogates or expensive mistakes?, Proc. Natl. Acad. Sci. U. S. A., 2000, 97(11), 5954–5959 CrossRef CAS PubMed.
- V. N. de Jonge, M. Elliott and V. S. Brauer, Marine monitoring: Its shortcomings and mismatch with the EU Water Framework Directive's objectives, Mar. Pollut. Bull., 2006, 53(1–4), 5–19 CrossRef CAS PubMed.
- D. J. Baird and M. Hajibabaei, Biomonitoring 2.0: a new paradigm in ecosystem assessment made possible by next-generation DNA sequencing, Mol. Ecol., 2012, 21(8), 2039–2044 CrossRef PubMed.
- S. A. P. Derocles, D. A. Bohan, A. J. Dumbrell, J. J. N. Kitson, F. Massol and C. Pauvert, Biomonitoring for the 21st Century: Integrating Next-Generation Sequencing Into Ecological Network Analysis, Adv. Ecol. Res., 2018, 1–62 Search PubMed.
- R. G. Young, C. D. Matthaei and C. R. Townsend, Organic matter breakdown and ecosystem metabolism: functional indicators for assessing river ecosystem health, J. North Am. Benthol. Soc., 2008, 27(3), 605–625 CrossRef.
- K. Jax, Function and “functioning” in ecology: what does it mean?, Oikos, 2005, 111(3), 641–648 CrossRef.
- R. G. Death, Z. S. Dewson and A. B. W. James, Is structure or function a better measure of the effects of water abstraction on ecosystem integrity?, Freshwater Biol., 2009, 54(10), 2037–2050 CrossRef CAS.
- R. Thompson and B. M. Starzomski, What does biodiversity actually do? A review for managers and policy makers, Biodivers. Conserv., 2007, 16(5), 1359–1378 CrossRef.
- L. Sandin and A. G. Solimini, Freshwater ecosystem structure–function relationships: from theory to application, Freshwater Biol., 2009, 54(10), 2017–2024 CrossRef.
- M. A. Huston, Hidden treatments in ecological experiments: re-evaluating the ecosystem function of biodiversity, Oecologia, 1997, 110(4), 449–460 CrossRef PubMed.
- J. D. Fridley, The influence of species diversity on ecosystem productivity: how, where, and why?, Oikos, 2001, 93(3), 514–526 CrossRef.
- D. Tilman, C. L. Lehman and K. T. Thomson, Plant diversity and ecosystem productivity: Theoretical considerations, Proc. Natl. Acad. Sci. U. S. A., 1997, 94(5), 1857–1861 CrossRef CAS PubMed.
- B. Vanelslander, A. De Wever, N. Van Oostende, P. Kaewnuratchadasorn, P. Vanormelingen and F. Hendrickx,
et al., Complementarity effects drive positive diversity effects on biomass production in experimental benthic diatom biofilms, J. Ecol., 2009, 97(5), 1075–1082 CrossRef.
- L. Jiang, Z. Pu and D. R. Nemergut, On the importance of the negative selection effect for the relationship between biodiversity and ecosystem functioning, Oikos, 2008, 117(4), 488–493 CrossRef.
- K. G. Lyons, C. A. Brigham, B. H. Traut and M. W. Schwartz, Rare Species and Ecosystem Functioning, Conserv. Biol., 2005, 19(4), 1019–1024 CrossRef.
- A. Burns and D. S. Ryder, Potential for biofilms as biological indicators in Australian riverine systems, Ecol. Manag. Restor., 2001, 2(1), 53–64 CrossRef.
- S. Jouanneau, L. Recoules, M. J. Durand, A. Boukabache, V. Picot and Y. Primault,
et al., Methods for assessing biochemical oxygen demand (BOD): A review, Water Res., 2014, 49, 62–82 CrossRef CAS PubMed.
- J. J. Piggott, D. K. Niyogi, C. R. Townsend and C. D. Matthaei, Multiple stressors and stream ecosystem functioning: climate warming and agricultural stressors interact to affect processing of organic matter, J. Appl. Ecol., 2015, 52(5), 1126–1134 CrossRef.
- U. Uehlinger, Annual cycle and inter-annual variability of gross primary production and ecosystem respiration in a floodprone river during a 15-year period, Freshwater Biol., 2006, 51(5), 938–950 CrossRef CAS.
- B. O. L. Demars, J. Russel Manson, J. S. Ólafsson, G. M. Gíslason, R. Gudmunddóttir and G. Woodward,
et al., Temperature and the metabolic balance of streams, Freshwater Biol., 2011, 56(6), 1106–1121 CrossRef.
- H. T. Odum, Primary Production in Flowing Waters1, Limnol. Oceanogr., 1956, 1(2), 102–117 CrossRef.
- E. K. Richmond, E. J. Rosi-Marshall, S. S. Lee, R. M. Thompson and M. R. Grace, Antidepressants in stream ecosystems: influence of selective serotonin reuptake inhibitors (SSRIs) on algal production and insect emergence, Freshw. Sci., 2016, 35(3), 845–855 CrossRef.
- V. Gulis, V. Ferreira and M. A. S. Graça, Stimulation of leaf litter decomposition and associated fungi and invertebrates by moderate eutrophication: implications for stream assessment, Freshwater Biol., 2006, 51(9), 1655–1669 CrossRef CAS.
- K. J. Purdy, P. J. Hurd, J. Moya-Laraño, M. Trimmer, B. B. Oakley and G. Woodward, Systems Biology for Ecology: From Molecules to Ecosystems, Adv. Ecol. Res., 2010, 43, 87–149 Search PubMed.
- J. Reiss, J. R. Bridle, J. M. Montoya and G. Woodward, Emerging horizons in biodiversity and ecosystem functioning research, Trends Ecol. Evol., 2009, 24(9), 505–514 CrossRef PubMed.
- J. Reiss, R. A. Bailey, F. Cássio, G. Woodward and C. Pascoal, Assessing the Contribution of Micro-Organisms and Macrofauna to Biodiversity–Ecosystem Functioning Relationships in Freshwater Microcosms, Adv. Ecol. Res., 2010, 151–176 Search PubMed.
- A. Hector and R. Bagchi, Biodiversity and ecosystem multifunctionality, Nature, 2007, 448(7150), 188–190 CrossRef CAS PubMed.
- M. Margulies, M. Egholm, W. E. Altman, S. Attiya, J. S. Bader and L. A. Bemben,
et al., Genome sequencing in microfabricated high-density picolitre reactors, Nature, 2005, 437(7057), 376–380 CrossRef CAS PubMed.
-
P. Taberlet, A. Bonin, L. Zinger, and E. Coissac, Environmental DNA, Oxford University PressOxford, 2018 Search PubMed.
- T. Dejean, A. Valentini, C. Miquel, P. Taberlet, E. Bellemain and C. Miaud, Improved detection of an alien invasive species through environmental DNA barcoding: the example of the American bullfrog Lithobates catesbeianus, J. Appl. Ecol., 2012, 49(4), 953–959 CrossRef.
- L. R. Harper, L. Lawson Handley, C. Hahn, N. Boonham, H. C. Rees and K. C. Gough,
et al., Needle in a haystack? A comparison of eDNA metabarcoding and targeted qPCR for detection of the great crested newt (Triturus cristatus), Ecol. Evol., 2018, 8(12), 6330–6341 CrossRef PubMed.
- M. Scriver, A. Marinich, C. Wilson and J. Freeland, Development of species-specific environmental DNA (eDNA) markers for invasive aquatic plants, Aquat. Bot., 2015, 122, 27–31 CrossRef CAS.
- E. A. Andruszkiewicz, H. A. Starks, F. P. Chavez, L. M. Sassoubre, B. A. Block and A. B. Boehm, Biomonitoring of marine vertebrates in Monterey Bay using eDNA metabarcoding, PLoS One, 2017, 12(4), e0176343 CrossRef PubMed.
- A. Valentini, P. Taberlet, C. Miaud, R. Civade, J. Herder and P. F. Thomsen,
et al., Next-generation monitoring of aquatic biodiversity using environmental DNA metabarcoding, Mol. Ecol., 2016, 25(4), 929–942 CrossRef CAS PubMed.
- M. Stat, J. John, J. D. DiBattista, S. J. Newman, M. Bunce and E. S. Harvey, Combined use of eDNA metabarcoding and video surveillance for the assessment of fish biodiversity, Conserv. Biol., 2019, 33(1), 196–205 CrossRef PubMed.
- R. J. Case, Y. Boucher, I. Dahllöf, C. Holmström, W. F. Doolittle and S. Kjelleberg, Use of 16S rRNA and rpoB Genes as Molecular Markers for Microbial Ecology Studies, Appl. Environ. Microbiol., 2007, 73(1), 278–288 CrossRef CAS PubMed.
- C. L. Schoch, K. A. Seifert, S. Huhndorf, V. Robert, J. L. Spouge and C. A. Levesque,
et al., Nuclear ribosomal internal transcribed spacer (ITS) region as a universal DNA barcode marker for Fungi, Proc. Natl. Acad. Sci. U. S. A., 2012, 109(16), 6241–6246 CrossRef CAS PubMed.
- K. Hadziavdic, K. Lekang, A. Lanzen, I. Jonassen, E. M. Thompson and C. Troedsson, Characterization of the 18S rRNA Gene for Designing Universal Eukaryote Specific Primers, PLoS One, 2014, 9(2), e87624 CrossRef PubMed.
- D. Bickford, D. J. Lohman, N. S. Sodhi, P. K. L. Ng, R. Meier and K. Winker,
et al., Cryptic species as a window on diversity and conservation, Trends Ecol. Evol., 2007, 22(3), 148–155 CrossRef PubMed.
- V. Vasselon, F. Rimet, K. Tapolczai and A. Bouchez, Assessing ecological status with diatoms DNA metabarcoding: Scaling-up on a WFD monitoring network (Mayotte island, France), Ecol. Indic., 2017, 82, 1–12 CrossRef CAS.
- L. Apothéloz-Perret-Gentil, A. Cordonier, F. Straub, J. Iseli, P. Esling and J. Pawlowski, Taxonomy-free molecular diatom index for high-throughput eDNA biomonitoring, Mol. Ecol. Resour., 2017, 17(6), 1231–1242 CrossRef PubMed.
- L. Kermarrec, A. Franc, F. Rimet, P. Chaumeil, J. M. Frigerio and J. F. Humbert,
et al., A next-generation sequencing approach to river biomonitoring using benthic diatoms, Freshw. Sci., 2014, 33(1), 349–363 CrossRef.
- L. Kermarrec, A. Franc, F. Rimet, P. Chaumeil, J. F. Humbert and A. Bouchez, Next-generation sequencing to inventory taxonomic diversity in eukaryotic communities: a test for freshwater diatoms, Mol. Ecol. Resour., 2013, 13(4), 607–619 CrossRef CAS PubMed.
- E. Aylagas, Á. Borja and N. Rodríguez-Ezpeleta, Environmental Status Assessment Using DNA Metabarcoding: Towards a Genetics Based Marine Biotic Index (gAMBI), PLoS One, 2014, 9(3), e90529 CrossRef PubMed.
- V. Elbrecht, E. E. Vamos, K. Meissner, J. Aroviita and F. Leese, Assessing strengths and weaknesses of DNA metabarcoding-based macroinvertebrate identification for routine stream monitoring, Methods Ecol. Evol., 2017, 8(10), 1265–1275 CrossRef.
- M. Hajibabaei, J. L. Spall, S. Shokralla and S. van Konynenburg, Assessing biodiversity of a freshwater benthic macroinvertebrate community through non-destructive environmental barcoding of DNA from preservative ethanol, BMC Ecol., 2012, 12(1), 28 CrossRef CAS PubMed.
- T. M. Wilcox, K. S. McKelvey, M. K. Young, A. J. Sepulveda, B. B. Shepard and S. F. Jane,
et al., Understanding environmental DNA detection probabilities: A case study using a stream-dwelling char Salvelinus fontinalis, Biol. Conserv., 2016, 194, 209–216 CrossRef.
- C. L. Jerde, A. R. Mahon, W. L. Chadderton and D. M. Lodge, “Sight-unseen” detection of rare aquatic species using environmental DNA, Conserv. Lett., 2011, 4(2), 150–157 CrossRef.
- T. Cordier, L. Alonso-Sáez, L. Apothéloz-Perret-Gentil, E. Aylagas, D. A. Bohan and A. Bouchez,
et al., Ecosystems monitoring powered by environmental genomics: A review of current strategies with an implementation roadmap, Mol. Ecol., 2021, 30(13), 2937–2958 CrossRef PubMed.
- N. E. Stork, How Many Species of Insects and Other Terrestrial Arthropods Are There on Earth?, Annu. Rev. Entomol., 2018, 63(1), 31–45 CrossRef CAS PubMed.
- H. M. Bik, D. Fournier, W. Sung, R. D. Bergeron and W. K. Thomas, Intra-Genomic Variation in the Ribosomal Repeats of Nematodes, PLoS One, 2013, 8(10), e78230 CrossRef CAS PubMed.
- D. L. Sun, X. Jiang, Q. L. Wu and N. Y. Zhou, Intragenomic Heterogeneity of 16S rRNA Genes Causes Overestimation of Prokaryotic Diversity, Appl. Environ. Microbiol., 2013, 79(19), 5962–5969 CrossRef CAS PubMed.
- R. A. Collins, O. S. Wangensteen, E. J. O'Gorman, S. Mariani, D. W. Sims and M. J. Genner, Persistence of environmental DNA in marine systems, Commun. Biol., 2018, 1(1), 185 CrossRef PubMed.
- R. P. Kelly, J. A. Port, K. M. Yamahara and L. B. Crowder, Using Environmental DNA to Census Marine Fishes in a Large Mesocosm, PLoS One, 2014, 9(1), e86175 CrossRef PubMed.
- K. C. Beng and R. T. Corlett, Applications of environmental DNA (eDNA) in ecology and conservation: opportunities, challenges and prospects, Biodivers. Conserv., 2020, 29(7), 2089–2121 CrossRef.
- S. Wang, Z. Yan, B. Hänfling, X. Zheng, P. Wang and J. Fan,
et al., Methodology of fish eDNA and its applications in ecology and environment, Sci. Total Environ., 2021, 755, 142622 CrossRef CAS PubMed.
- V. Vasselon, A. Bouchez, F. Rimet, S. Jacquet, R. Trobajo and M. Corniquel,
et al., Avoiding quantification bias in metabarcoding: Application of a cell biovolume correction factor in diatom molecular biomonitoring, Methods Ecol. Evol., 2018, 9(4), 1060–1069 CrossRef.
- Y. Ji, T. Huotari, T. Roslin, N. M. Schmidt, J. Wang and D. W. Yu,
et al., SPIKEPIPE: A metagenomic pipeline for the accurate quantification of eukaryotic species occurrences and intraspecific abundance change using DNA barcodes or mitogenomes, Mol. Ecol. Resour., 2020, 20(1), 256–267 CrossRef CAS PubMed.
- R. K. Salis, A. Bruder, J. J. Piggott, T. C. Summerfield and C. D. Matthaei, High-throughput amplicon sequencing and stream benthic bacteria: identifying the best taxonomic level for multiple-stressor research, Sci. Rep., 2017, 7(1), 44657 CrossRef CAS PubMed.
- O. Laroche, S. A. Wood, L. A. Tremblay, G. Lear, J. I. Ellis and X. Pochon, Metabarcoding monitoring analysis: the pros and cons of using co-extracted environmental DNA and RNA data to assess offshore oil production impacts on benthic communities, PeerJ, 2017, 5, e3347 CrossRef PubMed.
- C. T. T. Binh, T. Tong, J. F. Gaillard, K. A. Gray and J. J. Kelly, Acute Effects of TiO2 Nanomaterials on the Viability and Taxonomic Composition of Aquatic Bacterial Communities Assessed via High-Throughput Screening and Next Generation Sequencing, PLoS One, 2014, 9(8), e106280 CrossRef PubMed.
- V. Kisand, A. Valente, A. Lahm, G. Tanet and T. Lettieri, Phylogenetic and Functional Metagenomic Profiling for Assessing Microbial Biodiversity in Environmental Monitoring, PLoS One, 2012, 7(8), e43630 CrossRef CAS PubMed.
- A. Borja, Testing the efficiency of a bacterial community-based index (microgAMBI) to assess distinct impact sources in six locations around the world, Ecol. Indic., 2018, 85, 594–602 CrossRef CAS.
- H. M. Bik, K. M. Halanych, J. Sharma and W. K. Thomas, Dramatic Shifts in Benthic Microbial Eukaryote Communities following the Deepwater Horizon Oil Spill, PLoS One, 2012, 7(6), e38550 CrossRef CAS PubMed.
-
H. Guasch, B. Bonet, C. Bonnineau, and L. Barral, Microbial Biomarkers, in Microbial Ecotoxicology, Springer International Publishing, Cham, 2017, pp. 251–81 Search PubMed.
- N. R. Pace, A Molecular View of Microbial Diversity and the Biosphere, Science, 1997, 276(5313), 734–740, DOI:10.1126/science.276.5313.734.
- A. H. Lee, J. Lee, S. Hong, B. O. Kwon, Y. Xie and J. P. Giesy,
et al., Integrated assessment of west coast of South Korea by use of benthic bacterial community structure as determined by eDNA, concentrations of contaminants, and in vitro bioassays, Environ. Int., 2020, 137, 105569 CrossRef CAS PubMed.
- S. Dequiedt, N. P. A. Saby, M. Lelievre, C. Jolivet, J. Thioulouse and B. Toutain,
et al., Biogeographical patterns of soil molecular microbial biomass as influenced by soil characteristics and management, Global Ecol. Biogeogr., 2011, 20(4), 641–652 CrossRef.
- P. G. Falkowski, T. Fenchel and E. F. Delong, The Microbial Engines That Drive Earth's Biogeochemical Cycles, Science, 2008, 320(5879), 1034–1039 CrossRef CAS PubMed.
- L. Jiang, Negative selection effects suppress relationships between bacterial diversity and ecosystem functioning, Ecology, 2007, 88(5), 1075–1085 CrossRef PubMed.
- J. M. Shively, R. S. English, S. H. Baker and G. C. Cannon, Carbon cycling: the prokaryotic contribution, Curr. Opin. Microbiol., 2001, 4(3), 301–306 CrossRef CAS PubMed.
- M. Sagova-Mareckova, J. Boenigk, A. Bouchez, K. Cermakova, T. Chonova and T. Cordier,
et al., Expanding ecological assessment by integrating microorganisms into routine freshwater biomonitoring, Water Res., 2021, 191, 116767 CrossRef CAS PubMed.
- A. Jousset, C. Bienhold, A. Chatzinotas, L. Gallien, A. Gobet and V. Kurm,
et al., Where less may be more: how the rare biosphere pulls ecosystems strings, ISME J., 2017, 11(4), 853–862 CrossRef PubMed.
- S. L. Garcia, S. L. R. Stevens, B. Crary, M. Martinez-Garcia, R. Stepanauskas and T. Woyke,
et al., Contrasting patterns of genome-level diversity across distinct co-occurring bacterial populations, ISME J., 2018, 12(3), 742–755 CrossRef CAS PubMed.
- S. A. Wakelin, M. J. Colloff and R. S. Kookana, Effect of wastewater treatment plant effluent on microbial function and community structure in the sediment of a freshwater stream with variable seasonal flow, Appl. Environ. Microbiol., 2008, 74(9), 2659–2668, DOI:10.1128/AEM.02348-07.
- F. Li, Y. Zhang, F. Altermatt and X. Zhang, Consideration of Multitrophic Biodiversity and Ecosystem Functions Improves Indices on River Ecological Status, Environ. Sci. Technol., 2021, 55(24), 16434–16444 CrossRef CAS PubMed.
- T. Cordier, Bacterial communities' taxonomic and functional turnovers
both accurately predict marine benthic ecological quality status, Environ. DNA, 2020, 2(2), 175–183 CrossRef.
- L. Proia, S. Morin, M. Peipoch, A. M. Romaní and S. Sabater, Resistance and recovery of river biofilms receiving short pulses of Triclosan and Diuron, Sci. Total Environ., 2011, 409(17), 3129–3137 CrossRef CAS PubMed.
- M. Ricart, D. Barceló, A. Geiszinger, H. Guasch, M. L. de Alda and A. M. Romaní,
et al., Effects of low concentrations of the phenylurea herbicide diuron on biofilm algae and bacteria, Chemosphere, 2009, 76(10), 1392–1401 CrossRef CAS PubMed.
- E. Capo, D. Debroas, F. Arnaud, M. Perga, C. Chardon and I. Domaizon, Tracking a century of changes in microbial eukaryotic diversity in lakes driven by nutrient enrichment and climate warming, Environ. Microbiol., 2017, 19(7), 2873–2892 CrossRef CAS PubMed.
- T. Cordier, P. Esling, F. Lejzerowicz, J. Visco, A. Ouadahi and C. Martins,
et al., Predicting the Ecological Quality Status of Marine Environments from eDNA Metabarcoding Data Using Supervised Machine Learning, Environ. Sci. Technol., 2017, 51(16), 9118–9126 CrossRef CAS PubMed.
- J. Pawlowski, F. Lejzerowicz, L. Apotheloz-Perret-Gentil, J. Visco and P. Esling, Protist metabarcoding and environmental biomonitoring: Time for change, Eur. J. Protistol., 2016, 55, 12–25 CrossRef CAS PubMed.
- X. Pochon, S. A. Wood, N. B. Keeley, F. Lejzerowicz, P. Esling and J. Drew,
et al., Accurate assessment of the impact of salmon farming on benthic sediment enrichment using foraminiferal metabarcoding, Mar. Pollut. Bull., 2015, 100(1), 370–382 CrossRef CAS PubMed.
- T. Cordier, A. Lanzén, L. Apothéloz-Perret-Gentil, T. Stoeck and J. Pawlowski, Embracing Environmental Genomics and Machine Learning for Routine Biomonitoring, Trends Microbiol., 2019, 27(5), 387–397 CrossRef CAS PubMed.
- E. Aylagas, J. Atalah, P. Sánchez-Jerez, J. K. Pearman, N. Casado and J. Asensi,
et al., A step towards the validation of bacteria biotic indices using DNA metabarcoding for benthic monitoring, Mol. Ecol. Resour., 2021, 21(6), 1889–1903 CrossRef CAS PubMed.
- L. Frühe, T. Cordier, V. Dully, H. Breiner, G. Lentendu and J. Pawlowski,
et al., Supervised machine learning is superior to indicator value inference in monitoring the environmental impacts of salmon aquaculture using eDNA metabarcodes, Mol. Ecol., 2021, 30(13), 2988–3006 CrossRef PubMed.
- C. Andújar, P. Arribas, C. Gray, C. Bruce, G. Woodward and D. W. Yu,
et al., Metabarcoding of freshwater invertebrates to detect the effects of a pesticide spill, Mol. Ecol., 2018, 27(1), 146–166 CrossRef PubMed.
- A. A. Chariton, S. Stephenson, M. J. Morgan, A. D. L. Steven, M. J. Colloff and L. N. Court,
et al., Metabarcoding of benthic eukaryote communities predicts the ecological condition of estuaries, Environ. Pollut., 2015, 203, 165–174 CrossRef CAS PubMed.
- M. Martínez-Santos, A. Lanzén, J. Unda-Calvo, I. Martín, C. Garbisu and E. Ruiz-Romera, Treated and untreated wastewater effluents alter river sediment bacterial communities involved in nitrogen and sulphur cycling, Sci. Total Environ., 2018, 633, 1051–1061 CrossRef PubMed.
- M. Esser, C. Hoggarth, H. Baulch, J. K. Challis, Y. Xie and J. P. Giesy,
et al., Wastewater discharges alter microbial community composition in surface waters of the canadian prairies, Chemosphere, 2023, 334, 138991 CrossRef CAS PubMed.
- M. J. Feio, S. R. Q. Serra, A. Mortágua, A. Bouchez, F. Rimet and V. Vasselon,
et al., A taxonomy-free approach based on machine learning to assess the quality of rivers with diatoms, Sci. Total Environ., 2020, 722, 137900 CrossRef CAS PubMed.
- A. Escalas, L. Hale, J. W. Voordeckers, Y. Yang, M. K. Firestone and L. Alvarez-Cohen,
et al., Microbial functional diversity: From concepts to applications, Ecol. Evol., 2019, 9(20), 12000–12016 CrossRef PubMed.
- P. Lamarque, S. Lavorel, M. Mouchet and F. Quétier, Plant trait-based models identify direct and indirect effects of climate change on bundles of grassland ecosystem services, Proc. Natl. Acad. Sci. U. S. A., 2014, 111(38), 13751–13756 CrossRef CAS PubMed.
- R. D. Bardgett and W. H. van der Putten, Belowground biodiversity and ecosystem functioning, Nature, 2014, 515(7528), 505–511 CrossRef CAS PubMed.
-
K. J. Purdy, P. J. Hurd, J. Moya-Laraño, M. Trimmer, B. B. Oakley, and G. Woodward. Systems Biology for Ecology: From Molecules to Ecosystems. 2010. pp. 87–149. available from: https://linkinghub.elsevier.com/retrieve/pii/B9780123850058000034.
- Z. He, M. Xu, Y. Deng, S. Kang, L. Kellogg and L. Wu,
et al., Metagenomic analysis reveals a marked divergence in the structure of belowground microbial communities at elevated CO 2, Ecol. Lett., 2010, 13(5), 564–575 CrossRef PubMed.
- G. M. Douglas, V. J. Maffei, J. R. Zaneveld, S. N. Yurgel, J. R. Brown and C. M. Taylor,
et al., PICRUSt2 for prediction of metagenome functions, Nat. Biotechnol., 2020, 38(6), 685–688 CrossRef CAS PubMed.
- F. Wemheuer, J. A. Taylor, R. Daniel, E. Johnston, P. Meinicke and T. Thomas,
et al., Tax4Fun2: prediction of habitat-specific functional profiles and functional redundancy based on 16S rRNA gene sequences, Environ. Microbiome, 2020, 15(1), 11 CrossRef CAS PubMed.
- Z. Yang, C. Peng, H. Cao, J. Song, B. Gong and L. Li,
et al., Microbial functional assemblages predicted by the FAPROTAX analysis are impacted by physicochemical properties, but C, N and S cycling genes are not in mangrove soil in the Beibu Gulf, China, Ecol. Indic., 2022, 139, 108887 CrossRef CAS.
- E. Jaspers and J. Overmann, Ecological Significance of Microdiversity: Identical 16S rRNA Gene Sequences Can Be Found in Bacteria with Highly Divergent Genomes and Ecophysiologies, Appl. Environ. Microbiol., 2004, 70(8), 4831–4839 CrossRef CAS PubMed.
- L. Philippot, S. G. E. Andersson, T. J. Battin, J. I. Prosser, J. P. Schimel and W. B. Whitman,
et al., The ecological coherence of high bacterial taxonomic ranks, Nat. Rev. Microbiol., 2010, 8(7), 523–529 CrossRef CAS PubMed.
- Y. Yang, Emerging Patterns of Microbial Functional Traits, Trends Microbiol., 2021, 29(10), 874–882 CrossRef CAS PubMed.
- M. Goberna and M. Verdú, Predicting microbial traits with phylogenies, ISME J., 2016, 10(4), 959–967 CrossRef PubMed.
- J. M. Talbot, T. D. Bruns, J. W. Taylor, D. P. Smith, S. Branco and S. I. Glassman,
et al., Endemism and functional convergence across the North American soil mycobiome, Proc. Natl. Acad. Sci. U. S. A., 2014, 111(17), 6341–6346 CrossRef CAS PubMed.
- K. G. Peay, P. G. Kennedy and J. M. Talbot, Dimensions of biodiversity in the Earth mycobiome, Nat. Rev. Microbiol., 2016, 14(7), 434–447 CrossRef CAS PubMed.
- J. Comte, L. Fauteux and P. A. del Giorgio, Links between metabolic plasticity and functional redundancy in freshwater bacterioplankton communities, Front. Microbiol., 2013, 4, 112 Search PubMed.
- S. Louca, M. F. Polz, F. Mazel, M. B. N. Albright, J. A. Huber and M. I. O'Connor,
et al., Function and functional redundancy in microbial systems, Nat. Ecol. Evol., 2018, 2(6), 936–943 CrossRef PubMed.
- Z. Xu, D. Malmer, M. G. I. Langille, S. F. Way and R. Knight, Which is more important for classifying microbial communities: who's
there or what they can do?, ISME J., 2014, 8(12), 2357–2359 CrossRef CAS PubMed.
- A. Barberan, A. Fernández-guerra, B. J. M. Bohannan and E. O. Casamayor, Exploration of community traits as ecological markers in microbial metagenomes, Mol. Ecol., 2012, 21(8), 1909–1917 CrossRef CAS PubMed.
- H. Grossart, R. Massana, K. D. McMahon and D. A. Walsh, Linking metagenomics to aquatic microbial ecology and biogeochemical cycles, Limnol. Oceanogr., 2020, 65(S1), S2–S20 CrossRef CAS.
- J. Handelsman, Metagenomics: Application of Genomics to Uncultured Microorganisms, Microbiol. Mol. Biol. Rev., 2004, 68(4), 669–685 CrossRef CAS PubMed.
- L. Chistoserdova, Functional Metagenomics: Recent Advances and Future Challenges, Biotechnol. Genet. Eng. Rev., 2009, 26(1), 335–352 CrossRef.
- O. Béjà, L. Aravind, E. V. Koonin, M. T. Suzuki, A. Hadd and L. P. Nguyen,
et al., Bacterial Rhodopsin: Evidence for a New Type of Phototrophy in the Sea, Science, 2000, 289(5486), 1902–1906 CrossRef PubMed.
- J. C. Venter, K. Remington, J. F. Heidelberg, A. L. Halpern, D. Rusch and J. A. Eisen,
et al., Environmental Genome Shotgun Sequencing of the Sargasso Sea, Science, 2004, 304(5667), 66–74 CrossRef CAS PubMed.
- T. J. Sharpton, S. J. Riesenfeld, S. W. Kembel, J. Ladau, J. P. O'Dwyer and J. L. Green,
et al., PhylOTU: A High-Throughput Procedure Quantifies Microbial Community Diversity and Resolves Novel Taxa from Metagenomic Data, PLoS Comput. Biol., 2011, 7(1), e1001061 CrossRef CAS PubMed.
- A. Alfreider, A. Baumer, T. Bogensperger, T. Posch, M. M. Salcher and M. Summerer, CO 2 assimilation strategies in stratified lakes: Diversity and distribution patterns of chemolithoautotrophs, Environ. Microbiol., 2017, 19(7), 2754–2768 CrossRef CAS PubMed.
- L. A. Waidner and D. L. Kirchman, Diversity and Distribution of Ecotypes of the Aerobic Anoxygenic Phototrophy Gene pufM in the Delaware Estuary, Appl. Environ. Microbiol., 2008, 74(13), 4012–4021 CrossRef CAS PubMed.
- Y. Yang, J. Chen, T. Tong, S. Xie and Y. Liu, Influences of eutrophication on methanogenesis pathways and methanogenic microbial community structures in freshwater lakes, Environ. Pollut., 2020, 260, 114106 CrossRef CAS PubMed.
- W. Liu, L. Yao, X. Jiang, L. Guo, X. Cheng and G. Liu, Sediment denitrification in Yangtze lakes is mainly influenced by environmental conditions but not biological communities, Sci. Total Environ., 2018, 616–617, 978–987 CrossRef CAS PubMed.
- Z. He, T. J. Gentry, C. W. Schadt, L. Wu, J. Liebich and S. C. Chong,
et al., GeoChip: a comprehensive microarray for investigating biogeochemical, ecological and environmental processes, ISME J., 2007, 1(1), 67–77 CrossRef CAS PubMed.
- Z. He, Y. Deng, J. D. Van Nostrand, Q. Tu, M. Xu and C. L. Hemme,
et al., GeoChip 3.0 as a high-throughput tool for analyzing microbial community composition, structure and functional activity, ISME J., 2010, 4(9), 1167–1179 CrossRef CAS PubMed.
- M. Shakya, C. C. Lo and P. S. G. Chain, Advances and Challenges in Metatranscriptomic Analysis, Front. Genet., 2019, 10, 904 CrossRef CAS PubMed.
- V. Aguiar-Pulido, W. Huang, V. Suarez-Ulloa, T. Cickovski, K. Mathee and G. Narasimhan, Metagenomics, Metatranscriptomics, and Metabolomics Approaches for Microbiome Analysis, Evol. Bioinform. Online, 2016, 12s1(EBO.S36436), 5–16 Search PubMed.
- N. Segata, D. Boernigen, T. L. Tickle, X. C. Morgan, W. S. Garrett and C. Huttenhower, Computational meta’omics for microbial community studies, Mol. Syst. Biol., 2013, 9(1), 666 CrossRef PubMed.
- Y. Ye and T. G. Doak, A Parsimony Approach to Biological Pathway Reconstruction/Inference for Genomes and Metagenomes, PLoS Comput. Biol., 2009, 5(8), e1000465 CrossRef PubMed.
- T. Yamada, I. Letunic, S. Okuda, M. Kanehisa and P. Bork, iPath2.0: interactive pathway explorer, Nucleic Acids Res., 2011, 39(suppl_2), W412–W415 CrossRef CAS PubMed.
- E. Yergeau, S. Sanschagrin, M. J. Waiser, J. R. Lawrence and C. W. Greer, Sub-inhibitory concentrations of different pharmaceutical products affect the meta-transcriptome of river biofilm communities cultivated in rotating annular reactors, Environ. Microbiol. Rep., 2012, 4(3), 350–359 CrossRef CAS PubMed.
- P. E. Galand, S. Lucas, S. K. Fagervold, E. Peru, A. M. Pruski and G. Vétion,
et al., Disturbance Increases Microbial Community Diversity and Production in Marine Sediments, Front. Microbiol., 2016, 7, 1950 Search PubMed.
- S. C. Birrer, K. A. Dafforn, M. Y. Sun, R. B. H. Williams, J. Potts and P. Scanes,
et al., Using meta-omics of contaminated sediments to monitor changes in pathways relevant to climate regulation, Environ. Microbiol., 2019, 21(1), 389–401 CrossRef CAS PubMed.
- N. Falk, T. Reid, A. Skoyles, A. Grgicak-Mannion, K. Drouillard and C. G. Weisener, Microbial metatranscriptomic investigations across contaminant gradients of the Detroit River, Sci. Total Environ., 2019, 690, 121–131 CrossRef CAS PubMed.
- B. Cheaib, M. Le Boulch, P. L. Mercier and N. Derome, Taxon-Function Decoupling as an Adaptive Signature of Lake Microbial Metacommunities Under a Chronic Polymetallic Pollution Gradient, Front. Microbiol., 2018, 9, 869 CrossRef PubMed.
- T. Cordier, L. Alonso-Sáez, L. Apothéloz-Perret-Gentil, E. Aylagas, D. A. Bohan and A. Bouchez,
et al., Ecosystems monitoring powered by environmental genomics: A review of current strategies with an implementation roadmap, Mol. Ecol., 2021, 30(13), 2937–2958 CrossRef PubMed.
- A. Zhan, W. Xiong, S. He and H. J. MacIsaac, Influence of Artifact Removal on Rare Species Recovery in Natural Complex Communities Using High-Throughput Sequencing, PLoS One, 2014, 9(5), e96928 CrossRef PubMed.
- S. P. Brown, A. M. Veach, A. R. Rigdon-Huss, K. Grond, S. K. Lickteig and K. Lothamer,
et al., Scraping the bottom of the barrel: are rare high throughput sequences artifacts?, Fungal Ecol., 2015, 13, 221–225 CrossRef.
|
This journal is © The Royal Society of Chemistry 2024 |