DOI:
10.1039/D4NA00217B
(Paper)
Nanoscale Adv., 2024,
6, 4470-4478
Chiral porphyrin-SiO2 nano helices-based sensors for vapor enantiomers recognition†
Received
16th March 2024
, Accepted 15th July 2024
First published on 19th July 2024
Abstract
The ability of olfaction to distinguish odors is based on many different properties deriving from the molecular structure, including chirality. Even if the electronic nose (e-nose) concept has been widely used in strict analogy with biological systems to implement sensor arrays that recognize and distinguish complex odor matrices, the fabrication of an enantioselective e-nose remains a challenge. This paper introduces an array of quartz microbalances (QMB) functionalized with sensitive materials made of a combination of achiral receptors and silica nanohelices grafted by chiral and achiral porphyrins. In this combination, nanohelices provide a chiral template for the spatial arrangement of porphyrins, while porphyrins act as receptors that can interact differently with analytes. Remarkably, even if single sensors show scarce enantioselectivity, the signals of the overall array achieve recognition of the chiral identity of the five diverse enantiomeric pairs tested when the data are processed with proper multivariate algorithms. Such an innovative and generalizable approach is expected to enable the formation of an extensive library of readily integrable chiral receptors in enantioselective sensor arrays, potentially revolutionizing diverse fields such as agrochemicals, medicine, and environmental sciences.
1. Introduction
The intrinsically asymmetric nature of living systems is demonstrated dramatically by the effect of chirality on life processes. For example, two enantiomers of the same compound can interact differently with biomacromolecules, such as enzymes, proteins, and peptides, leading to different pharmacological and biological effects with severe repercussions for efficacy and potential impacts on human health and the preservation of the environment.1,2 Therefore, the massive production and utilization of chiral drugs and agrochemicals imperatively require the development of analytical systems capable of recognizing, quantifying, and monitoring the life of chiral substances at different stages. Chiral receptors or materials with increasingly high-performance recognition properties3–5 and analytical techniques are equally developed and constantly optimized to meet this practical challenge.6,7 In addition to the more conventional methods based on chromatography and mass spectroscopy,8,9 the utilization of chemical sensors continuously increases due to rapid, in situ, and low-cost analysis.10–12 However, significant synthetic effort is generally required to achieve a specific sensor toward a target enantiomer since, in practice, most receptors are only partially or broadly selective. In this latter case, recognizing a chiral compound is not feasible because the sensor response may depend on the concentration of each enantiomer and the interaction with other analytes. An alternative solution relies on sensor arrays,13 where the signals of several sensors, some of them not necessarily chiral, might exhibit chiral recognition once processed adequately with machine learning algorithms. In these platforms, the selectivity does not depend on single sensors but rather arises from the different response patterns produced by all of them. This approach is the basis for the design of artificial sensory systems operating in gas or liquid environments, usually called electronic noses (e-noses) and tongues (e-tongues), respectively.14,15
In the literature, several enantioselective electronic noses have been reported.16–21 Among them, the most promising results have been obtained utilizing Metal Organic Frameworks (MOFs) as sensing materials, thanks to the possibility of creating a rigid and well-defined chiral environment for gas adsorption. For example, Okur et al. utilized an array of chiral and achiral MOF deposited onto quartz microbalances to recognize five pairs of chiral volatile compounds.18
On the contrary, here, we investigate the enantioselective properties of hybrid materials based on a microscopical chiral template based on silica nanohelices, which induce chiroptical properties to molecular receptors. While the possibility of conferring chirality to optical receptors in this way is well-known, the enantioselective properties of these materials are still not reported. This approach should allow straightforwardly tuning the sensor sensitivity by changing the covalently deposited molecules, opening the way to fabricating a large set of different chiral sensors with a low synthetic effort, corroborating the possibility of using these systems as a valid alternative to current analytical methods22–24 and chemical sensors25–30 for vapors enantiorecognition.
For this purpose, we utilized porphyrins as molecular receptors since they are among the most versatile and tunable materials used in multisensory platforms since distinct sensitivity patterns can be easily obtained from varying molecular components, such as coordinated metal and peripheral substituents.31 Over the years, some of us have reported many examples of porphyrin-based nanogravimetric sensors in food analysis and medical applications,32–34 with the more recent result in the enantiodiscrimination of some classes of chiral vapors combining several metalloporphyrins with chiral effectors such as cyclohexanohemicucurbit[8]urils.35 Remarkably, the sensing performances of these macrocycles are improved by using hybrid systems, where porphyrin sensing units are layered on a nanostructured inorganic material since it is possible to deposit thin and porous films due to the high surface/volume ratio of the inorganic component.36–39
Specifically, porous films allow the analyte to diffuse within the sensing material bulk, determining slower and potentially more specific adsorption and desorption processes. Consequently, the dynamic analysis of sensor response may enclose essential information, as recently shown by us in the case of a conductometric sensor array based on silica nanoparticles functionalized with different porphyrinoids to detect COVID-19 from blood serum volatilome analysis.40
Herein, we develop a sensor array comprising chiral and achiral sensors based on quartz microbalance (QMBs) transducers to explore the possibility of discriminating chiral vapors (Fig. 1). The achiral sensor is obtained by spray casting a zinc complex of 5,10,15,20-tetrakis-(4-butyloxyphenyl)porphyrin (Znbut-TPP). Concerning chiral sensors, we have recently reported the amplification of chiroptical properties of different porphyrin derivatives when these macrocycles organize themselves into the chiral nanospace of the silica helices.41 In this work, similar hybrid systems are used consisting of either Zn complex of chiral porphyrin (D) and (L)Zn 5-[4-{carboxyphenyl(sodium-N-l-prolinatoyl)}]-10,15,20-triphenylporphyrin [(D) and (L)ZnP] or achiral porphyrin Zn 5-(4-carboxyphenyl)-10,15,20-triphenylporphyrin (ZnpCTPP) derivatives,42 covalently grafted onto inorganic chiral silica nanohelices having two opposite helicities (Right-Handed and Left-Handed Helices, RHH or LHH, respectively)43–45 (Fig. 1). The synthetic steps leading to hybrid chiral nanohelices are described in detail in the Experimental section and are schematically depicted in Fig. 1. These materials explore the possibility of combining the morphological chirality of nanohelix structures with the molecular chirality of receptors, if present. Indeed, the intermolecular interaction between the chiral analytes and the proline residue may be strongly affected by a larger, macroscopic chiral environment established by chiral nanohelices.
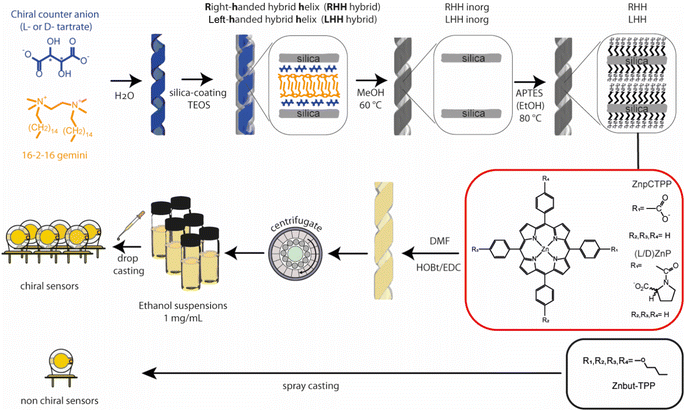 |
| Fig. 1 Schematic representation of synthetic steps of chiral nano helices, porphyrin receptors utilized, (D) and (L)ZnP and ZnpCTPP, and deposition steps on the QMBs surface. | |
2. Experimental part
2.1 Instruments
TEM micrographs were recorded using CM120 (Philips) at 120 kV to visualize the hybrid helices nanostructures. CD and UV-Vis spectra were measured on a J-815 (JASCO) Circular dichroism Spectrometer using a 10 mm quartz cell at 20 °C. IR spectra were recorded with a Thermo-Nicolet Nexus 670 FTIR spectrometer at a resolution of 4 cm−1.
2.2 Materials and methods
Reagents and solvents were purchased from Sigma Aldrich and used as received. Porphyrins are prepared according to the reported literature procedure,46 while silica helices are synthesized following the procedures in the ESI (Paragraph S1.1).† (R)-(−)-Butan-2-ol (99% purity, optical purity: N/A), (S)-(+)-butan-2-ol (99% purity, optical purity: N/A), (R)-(−)-carvone (98% purity, optical purity: N/A), (S)-(−)-carvone (98% purity, optical purity: N/A), methyl (R)-(+)-2-hydroxypropionate (98% purity, optical purity: ee 96%), methyl (S)-(−)-2-hydroxypropionate (98% purity, optical purity: ee 97%), (R)-(+)-limonene (97% purity, optical purity: ee 98%), (S)-(−)-limonene (96% purity, optical purity: N/A), methyl (+)-(R)-2-chloropropionate (98% purity, optical purity: ee 95%), methyl (−)-(S)-2-chloropropionate (98% purity, optical purity: ee 97%) were commercially available from Sigma Aldrich.
2.3 Circular dichroism studies
The quartz was cut into 2 × 2 cm plates. Then, 200 μL of each prepared system suspension (1 mg mL−1) was drop cast on the quartz surface and dried at room temperature for 24 h. After drying, Transmittance Circular Dichroism (CD) was measured with optimal parameters. CD, Linear Dichroism (LD), and UV-visible absorption spectral measurements in transmission mode were carried out using J-815(JASCO). All samples were measured with two angles (0° and 90°) rotated around the light beam axis. The final CD spectra were obtained by the mean values of data measured at 0° and 90°. The LD is also measured with two angles (0° and 90°) to know whether the LD affects the CD.
2.4 Quartz microbalances (QMBs)
Quartz microbalances are gravimetric sensors whose resonance frequency changes upon mass loading on the surface. The QMBs utilized are AT-cut quartz, and their oscillating frequency is approximately 20 MHz, measured with 0.1 Hz of resolution, with an estimated sensitivity of 7.20 Hz ng−1 according to the Sauerbrey equation and experimental outcomes.47 In total, seven sensors were used in the array comprising six chiral sensors that combine (D)ZnP/(L)ZnP/ZnpCTPP with RHH/LHH and an achiral sensor based on Znbut-TPP. Chiral solid-state films are produced by drop-casting a starting solution containing 1 mg mL−1 porphyrin-based nanohelices in ethanol until reaching a frequency decrease of about 20 kHz for each side of QMB. The achiral sensor was obtained by spray casting the porphyrin solution in chloroform at mM concentration. Table S1† reports the details about the mass loading onto each QMB. SEM images confirm the preservation of fibrous structures preserving the helical conformation (data not shown).
2.5 Gas sensor measurements
Fig. S1† shows the experimental setup. An airtight chamber with a gas inlet and outlet contains the sensor array. The system was tested toward six saturated vapor concentrations of five different enantiomeric pairs (butan-2-ol, carvone, methyl 2-hydroxypropionate, limonene, methyl 2-chloropropionate). Each concentration is measured seven times, and each sequence replica has a random order, giving 420 measurements. Saturated analyte vapors were obtained by fluxing molecular nitrogen through bubblers, thermostated at 20 °C, containing the analyte liquid samples, and calculated considering the Antoine law: for carvone, butan-2-ol, and methyl 2-hydroxypropionate the parameters are reported on the Sigma-Aldrich website (https://www.sigmaaldrich.com/, last accessed January 2024), for limonene reported on ref. 48 and for methyl 2-hydroxypropionate on ref. 49. A mass-flow controller system then diluted saturated vapors of analytes with a pure nitrogen flow to have the desired concentration. In all experiments, constant flux was maintained at 200 sccm. Each measurement consists of a 2 minute exposure to analyte vapor and a 15 minute exposure to the carrier gas (N2). In all sequences, enantiomers at the same concentration were delivered alternately to reduce the influence of time on measurements. Fig. S1† reports the chiral analytes and all tested concentrations.
2.6 Multivariate data analysis
Data have been analyzed using homemade algorithms developed in Matlab to extract features and perform Principal Component Analysis (PCA) and Linear Discriminant Analysis (LDA). In the case of multivariate models, the data have been autoscaled.
3. Results and discussion
3.1 Morphological characterization
Transmission Electron Microscopy (TEM) images reveal the conservation of the helical morphology of nanostructures during the grafting of dye molecules. Furthermore, the increase in contrast before and after the helical functionalization (Fig. S2A–C†) confirms the linkage of porphyrin macrocycles that has also been evaluated by spectroscopic methods.
3.2 Spectroscopic characterization
Fourier Transform Infrared Spectroscopy (FTIR) analysis was used to control the samples by monitoring the key changes during the various stages (Fig. S3†). All FTIR spectra of silica nanohelices show the presence of absorption bands at 797 cm−1 and 1058 cm−1, which correspond to the internal and external asymmetric O–Si–O stretching modes.50 The FTIR spectra of RHH hybrid and LHH hybrid (Fig. S3A,† blue lines) additionally reveal other representative absorption bands: asymmetric (νa,carboxy) and symmetric (νs,carboxy) stretching vibrations of tartrate carboxylate (respectively 1601 cm−1 and 1351 cm−1); bending of methyl group and methylene bridge (respectively 1500 cm−1 and 1469 cm−1) and stretching of the same groups at 2957, 2923, and 2853 cm−1 respectively.51 All these bands disappear after surfactants and tartrates removal (inorganic helices, Fig. S3A,† red lines). The chiral nano helices are consequently functionalized using APTES to anchor on the siloxane network CH2–CH2–CH2–NH2 moieties to react later with the carboxyl group of the porphyrin derivatives. The FTIR spectra of these materials (Fig. S3B,† green lines) show specific absorption bands due to the insertion of this group: symmetric –NH2 stretching, C–N band, the asymmetric (νa,CH2) and symmetric (νs,CH2) stretching vibrations of the alkyl chain of APTES (respectively 1561, 1379, 2853, and 2923 cm−1).50,52 Following the insertion of porphyrins by covalent chemical modification, the FTIR spectra of new chiral nanostructures (e.g., LHH-(L)ZnP and RHH-(L)ZnP) exhibit some noticeable changes, including a remarkable reduction in the intensity of symmetric –NH2 stretching groups together with the emergence of a new absorption band centered at ca. 1649 cm−1 corresponding to amide groups, confirming the grafting through covalent bonding mediated by HOBt-EDC coupling reagents (Fig. S3C,† orange lines). It must be said that although porphyrin anchoring on the silica surface mainly occurs covalently, the participation of other phenomena, such as electrostatic interactions and hydrogen bonding, cannot be excluded. Additional details on band assignment are reported in Table S2.†
UV-Vis and CD spectroscopic techniques were used to investigate the optical properties and chirality of pristine organic and inorganic materials. UV-Vis spectra of porphyrin ethanol solutions (Fig. S4A†) show a very sharp Soret band (λmax = 424 nm) without displaying any CD signal (Fig. S4B†). On the other hand, the suspensions of silica helices where porphyrins are grafted have a pale yellow color, typically indicative of porphyrin aggregation phenomena. The UV-Vis spectra (Fig. S5A†) support this hypothesis, showing broadened bands compared to the porphyrin monomers. Specifically, the Soret band appears as double coupled and unresolved bands (λmax = 404 and 430 nm). CD spectra of hybrid nanohelix suspensions show multi-coupled bands with crossover points in correspondence with absorption UV-Vis maxima (Fig. S5B†).
As shown in Fig. S5B,† the CD spectra of the left-handed helix (LHH) are mirror images of those of the right-handed helix (RHH).41 This confirms that when porphyrins are covalently bound to nano helices, the helix acts as a template determining the spatial arrangement of the grafted porphyrins in the chiral curvature of silica. Interestingly, the handedness of the silica helices dominates the enantiomeric configuration at the porphyrin stereogenic center. The dissymmetric g-factors are observed from 3.0 to 3.4 × 10−4 (at λ = 440 nm) for all systems (see Table S3†). This value is similar to our previous findings with covalent grafting of pyrene derivatives.53
Subsequently, materials were deposited on quartz slides, and solid films were characterized by UV-Vis and CD techniques (Fig. 2). Broader Soret band peaks are observed, producing higher g-factor values than those observed in suspensions. The stronger porphyrin-to-porphyrin interaction is probably responsible for the increased g-factor values in solid films.
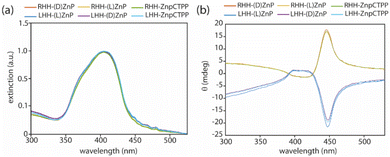 |
| Fig. 2 (a) UV-Vis and (b) CD spectra of porphyrins functionalized chiral nanohelices drop-casted on quartz slides. | |
As previously observed, the handedness of the helix is the determining factor for the sign of CD signals and dominates the molecular chirality of macrocycles.
4. Sensor measurements
After the characterization of the materials, the hybrid nanohelices were deposited from ethanol suspensions by drop casting onto QMB surfaces. Using gravimetric sensors allows us to control the amount of sensing material on the QMB surface to obtain highly reproducible sensors. The sensor array was tested toward several vapor concentrations of different commercially available chiral analytes in both enantiomeric forms. Specifically, we used two terpenes (i.e., carvone and limonene), one alcohol (butan-2-ol), and two esters (i.e., methyl 2-hydroxypropionate and methyl 2-chloropropionate). Because the chosen analytes belong to different classes of chemical compounds, they may bind to sensing materials by distinct interaction mechanisms. Finally, the array includes a non-chiral sensor employing the Zn complex of an alkyloxy-substituted meso-tetraphenylporphyrin (Znbut-TPP) as the sensing material. This sensor also rules out that enantiomers are not differentiated because of systemic error, sample impurities, or unbalances in the microfluidic system. Considering the Sauerbrey equation and estimated mass sensitivity, the amount of sensing material deposit is 5–6 μg for each sensor (estimated values are reported in Table S1†).
4.1 Sensor responses
Fig. 3 reports some examples of sensor responses to the different classes of volatile compounds utilized. For simplicity, we report as an example the responses of non-chiral porphyrin layer (Znbut-TPP) and nanohelices grafted with achiral (RHH-ZnpCTPP) or chiral (RHH-(D)ZnP) porphyrins as representative for the sensing behavior of materials utilized. For the sake of clarity, all the characteristic curves of sensors are reported in the ESI in Fig. S6–S12.†
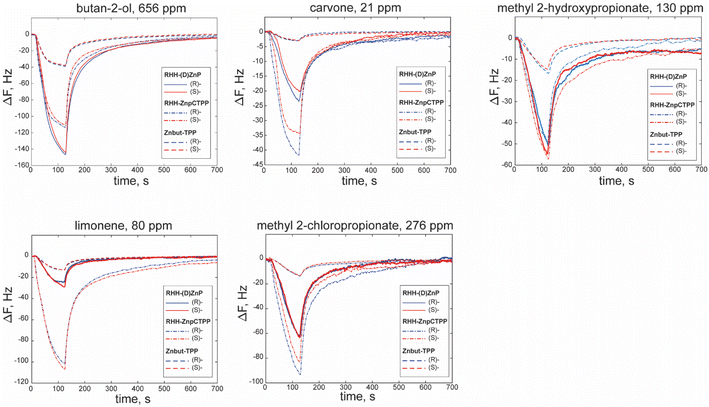 |
| Fig. 3 The frequency shift of non-chiral sensor (Znbut-TPP), achiral porphyrins onto RHH (RHH-ZnpCTPP), and chiral porphyrin on chiral nanohelices (RHH-(D)ZnP) exposed to a concentration of each of the five compounds considered. | |
First, let us analyze the response pattern of sensors to the volatile compounds without considering the chirality of samples. The Znbut-TPP sensor shows the lowest response intensities in all the cases, owing to the lower surface-to-volume ratio of soft films made by only porphyrins, considering that the amount of deposited material is comparable in all sensors and that chiral (ZnP) and achiral (ZnpCTPP) porphyrins grafted on silica nanohelices bear the same metal and fundamental structure as Znbut-TPP. In the case of hybrid materials, the responses are up to eight times higher than in the previous case. This outcome is another critical benefit of grafted nano helices because of their highest signal-to-noise ratios and potentially lower detection limits of these sensors. Furthermore, it is possible to observe the different patterns of responses produced by the five compounds, e.g., the highest responding sensor is not always the same. Indeed, the possibility of having different patterns of response is at the basis of pattern recognition.
Concerning the chiral recognition task, as expected, the achiral film does not show any difference in response within the enantiomer pairs (Fig. S12†). Then, we can consider the response of sensors where the handedness of helices is introduced as a single element of chirality (RHH- and LHH-ZnpCTPP) (Fig. S10 and S11†). In this case, characteristic curves have small differences between enantiomers that are more evident in the case of methyl 2-chloropropionate and methyl 2-hydroxypropionate. Furthermore, an opposite trend in the responses slightly emerges despite the statistical variation of samples.
A more complex case concerns the last four sensors, which combine the molecular chirality of porphyrins ((L)- and (D)-ZnP) and the handedness of helices (LHH and RHH), and in these cases, it is arduous to rationalize the trend in the characteristic curves (Fig. S6–S9†) due to the presence of multiple chiral environments at different scales, all of which may participate in the interaction with VCs.
Eventually, the Pearson correlation matrix of sensor responses may help to figure out the similarities between selectivity patterns of the hybrid chiral materials (Fig. S13†). As expected, the two sensors based on the achiral porphyrin, LHH- and RHH-ZnpCTPP, correlate more with each other than with other sensors. In the more complex case of chiral porphyrin, a higher correlation occurs in the sensor based on helices with the same handedness (RHH or LHH). This outcome is in line with the CD investigation, evidencing helices' prominent role in the chirality induction and suggesting a predominant role also in the interactions with VCs.
Finally, it is interesting to note that the dynamic behavior of the response depends on the pairing between sensing material and compounds. Response and recovery are sometimes faster and others slower. As mentioned above, the time required for adsorption and desorption of volatile molecules is intimately correlated with the porosity of films, diffusion, and the number and strength of interactions. Thus, a dynamic analysis of sensor response may usually provide more information about the chemical nature of analytes. In this case, it can also improve the identification of the enantiomers from each other. The average time constants (T90) during the adsorption phase are reported in Table S3.† For this reason, we decided to utilize a simple set of features to describe and represent both the sensors' response patterns and dynamic behavior.
4.2 Features selection
QMBs are, in principle, non-selective transducers, given that any analyte interacting with a sensing film produces a frequency decrement. Generally, sensor responses are calculated as the difference in oscillating frequency measured before and at the end of vapor exposure. Despite this, extracting meaningful information from data analysis is possible by analyzing the temporal behavior of sensor responses since the kinetic behavior of the adsorption and desorption phases can also depend on the strength and number of interactions between sensing film and airborne molecules. A second consideration should concern an intrinsic difference between the adsorption and desorption phases. A molecule is detected after the first binding event during adsorption, making the sensor response usually fast.
In the same way, the desorption phase consists of molecular desorption from the film and bonds breaking with porphyrins. In this latter phase, we can suppose that a molecule is no longer detected after the last binding event, making the sensor response typically slower and depending on the diffusion process and sensing film porosity. As a result, the analysis of both absorption and desorption phases can contain complementary information. Consequently, we extracted a set of dynamic features (fully described in Table S4† and schematically represented in Fig. S5†) separately from sensor responses during the adsorption and desorption phases. In detail, we selected the frequency shifts between the end of the adsorption phase and baseline on non-normalized data. Then, we normalize each temporal sensor response between 0 and 1, separating the adsorption (2 minutes) and desorption (15 minutes) phases. To characterize the dynamic trend of responses, we considered the normalized frequency shifts at five different times: T05, T10, T25, T50, and T75. As a consequence, 11 features from each sensor have been extracted (see Fig. S14†).
4.3 Multivariate analysis
A simple method to analyze array properties is Principal Component Analysis (PCA), which is a way to compress the information of multidimensional data in fewer dimensions expressed by Principal Components (PCs). Each Principal Component (PC1, PC2, PC3, etc.) is a linear composition of all the features we selected done in a way that maximizes the information content. Principal components are ordered according to the amount of the variance explained, reported in Fig. 4 as a percentage (%). As a consequence, PC1 has a higher percentage of explained variance than PC2, and so on. A more detailed description of this technique is provided in the SI. Fig. 4 reports separately the PCA plots of our samples along the first six PCs (containing approximately 83% of the data variance). We expected three main sources of data variance here: the concentration of samples, the chemical nature of compounds, and the configuration of enantiomers. Concentration is responsible for the intraclass variance, producing a dispersion of data from the same class. For example, butan-2-ol data along PC1 are aligned along six rows, corresponding to the concentrations tested. The second source of variation concerns the five probe compounds utilized. For example, butan-2-ol samples are well separated from carvone ones along PC1. These two sources are typical of most electronic nose applications. Enantioselective electronic noses should also show data displacement based on the enantiomeric configuration of the analytes.
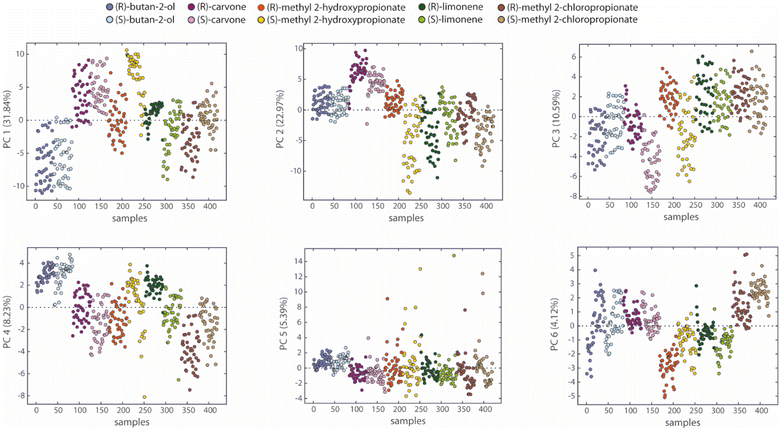 |
| Fig. 4 Results of PCA applied to sensors data: scores plot of samples vs. principal components from PC1 to PC6 for all tested analytes. | |
Interestingly, the separation between some enantiomers occurs along the first principal components, pointing out the relevance of data variance responsible for a potential enantiodiscrimination. Indeed, the first three principal components disclose a separation between the distributions of the enantiomers belonging to methyl 2-hydroxypropionate, limonene, and carvone. Since PCA is an unsupervised technique, the outcomes suggest an intrinsic capability of the array of discriminating enantiomers.
4.4 Linear discriminant analysis
The PCA outcomes encouraged us to use a classification model to investigate the performance of arrays under different cases.
Thus, we further verified the sensor array performance by classifying the samples using a linear model such as the Linear Discriminant Analysis (LDA). Specifically, the data set (420 samples) was randomly divided into two groups: one was used to build the classification model (representing the training), and the other validated the model during the testing phase. These two groups were generated without balancing concentrations or classes. In a balanced case, the training and test subsets have an equal number of samples of the different classes evenly distributed among the concentrations tested. In a non-balanced case, the measurements are divided into the training and test subsets without an equal distribution of samples among classes and concentrations. Usually, this latter case is more challenging than the balanced one since some classes or concentrations may be less represented during the model training, improving the possibility of generalizing the results obtained. Furthermore, this protocol was repeated 100 times to avoid potential bias in the results due to the peculiar training and test dataset choice.
We have trained two different classifiers to prove the possibility of employing these materials in electronic noses and their chiral version. The initial model aims to recognize only the five compounds as in standard e-noses, while the second one has also been trained to discriminate the enantiomeric pairs (10 classes). The results obtained are summarized using the confusion matrices (Fig. 5). In particular, the confusion matrix represents a simple method to evaluate the classifier performances: the columns contain predicted classes, and the rows refer to the actual classes. For the sake of simplicity, we reported the average classification percentages for each single actual class (i.e., the sum over each row is 100%). The correctly classified samples are reported on the diagonal, whereas the other cells report classification errors since the predicted classes differ from the actual ones.
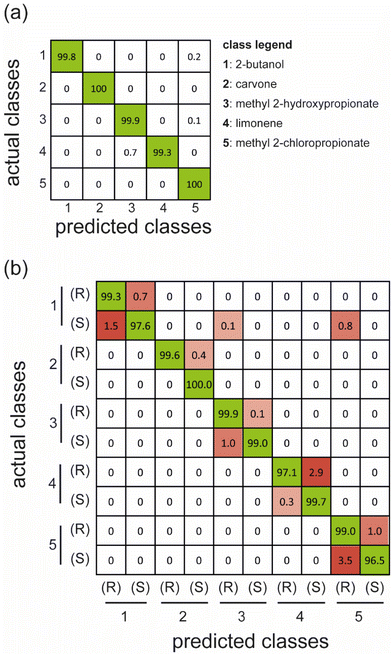 |
| Fig. 5 Confusion matrices report the test performance of over 100 runs of LDA classifiers built on separated training subsets, considering five non-chiral classes (a) and ten chiral compounds (b). | |
The results show that the classifier trained in five classes almost perfectly distinguishes the compounds tested. As mentioned above, we can consider this a non-chiral recognition task since enantiomers are grouped in the same class. This first outcome proves that the array can recognize single odors at different concentrations, as in traditional e-noses.
Subsequently, we analyzed the case where 10 classes are considered to include the chirality of analytes in the task ((R)/(S) labels). The results show that the system can discriminate not only the different compounds but also their enantiomeric pairs, with an average accuracy greater than 98.5% and a single class accuracy of 96.5% in the worst case of (S)-methyl 2-chloropropionate. In particular, the array displays excellent capability to recognize 2-butanol, carvone, and methyl-2-hydroxypropionate enantiomers, while the other pairs of enantiomers, especially with methyl 2-chloropropionate enantiomers, are classified with slightly lower accuracy. These outcomes reflect the separation of enantiomers on the PCs (Fig. 4), where the better classified enantiomers are the ones better separated on the first principal components. In contrast, the performances are lower for the compounds whose enantiomers are separated on lower-order PCs.
Furthermore, we decided to train the 10-class classifier using a more challenging scenario in which the models are trained on samples belonging only to three random concentrations, and the predictions are made considering samples that have the other concentrations. This approach avoids any possible benefits due to sample clustering because of the use of discrete concentrations and generalizes the classification performance over the concentration range considered. The training and test protocol was repeated 100 times in this case. The confusion matrices obtained are shown in Fig. S15.† As expected, the accuracy of the classifiers is lower than previously obtained, although the array offers good capabilities in recognition of both the chemical identity and chirality of the compounds, with an average accuracy of 95.9%.
Compared with previous works and the literature, the hybrid system illustrated in this work shows remarkable performance in separating all the pure chiral compounds tested, considering a wide range of concentrations. Regarding supramolecular chiral induction, we noted that porphyrins showed enantioselectivity only toward a limited number of chiral probes among those tested. Here, we found that inducing chirality by a silica helix scaffold is simple, reliable, and highly reproducible.
Furthermore, the sensor responses are amplified due to the high surface-to-volume ratio. At the same time, the porosity of the material allows the extraction of dynamic features at different times, which improves the discrimination of chiral compounds in a complex classification scenario. Finally, the synthetic flexibility of porphyrins may allow for the easy change of the metal coordinated and peripheral groups to improve sensing performance further.
Conclusions
This work explored the protocol for synthesizing chiral sensing materials based on chiral silica nanohelix scaffolds and chiral and achiral receptors, such as metalloporphyrins. Circular dichroism shows that the chiroptical features of materials depend on helix handedness rather than on the asymmetry of porphyrin stereogenic centers. Dynamic features were extracted from the sensor responses, considering the adsorption and desorption phases separately, to exploit silica nanostructures' intrinsic porosity. As expected, the sensing materials showed to be sensitive to the compounds, with scarce capability to discriminate between two enantiomers when considering single sensors. The findings further indicate that implementing all chiral sensors in an array, as in the electronic nose approach, and using standard multivariate analysis can identify the chemical identity and chirality of various types of compounds. Interestingly, desorption and adsorption phases collaborate with complementary features to enantiomer discrimination by multivariate data analysis techniques. Thus, developing enantioselective e-noses with these materials is made possible by the simplicity of the fabrication protocol and the ability to change the structure of porphyrin receptors, making tuning the sensor's chemical sensitivity much easier. These results are promising in extending the e-nose concept to chiral recognition, promoting the diffusion of chemical sensors for enantiodiscrimination to play a key role in medical, environmental, and agrochemical fields.
Data availability
The data that support the findings of this study are available from the corresponding author, GM, upon reasonable request.
Author contributions
Ilaria Di Filippo: investigation, writing – original draft, methodology, visualization, formal analysis; Zakaria Anfar: investigation, writing – original draft, methodology; Gabriele Magna: conceptualization, writing – review & editing, methodology, visualization, formal analysis; Piyanan Pranee: investigation; Donato Monti: conceptualization, writing – review & editing; Manuela Stefanelli: supervision, conceptualization, writing – review & editing; Reiko Oda: supervision, conceptualization, writing – review & editing, resources; Corrado Di Natale: validation, supervision, conceptualization, writing – review & editing, resources; Roberto Paolesse: supervision, conceptualization, writing – review & editing, resources.
Conflicts of interest
There are no conflicts to declare.
Acknowledgements
Financial support from EU (Horizon2020 FET-OPEN project 828779 INITIO) and from Project ECS 0000024 Rome Technopole, – CUP B83C22002820006, NRP Mission 4 Component 2 Investment 1.5, Funded by the European Union – NextGenerationEU.
Notes and references
- H. Alkadi and R. Jbeily, Infect. Disord.: Drug Targets, 2018, 18, 88 CAS.
- Y. Liu, Z. Wu, D. W. Armstrong, H. Wolosker and Y. Zheng, Nat. Rev. Chem, 2023, 7, 355 CrossRef CAS.
- Q. Cheng, H. Pei, Q. Ma, R. Guo, N. Liu and Z. Mo, Chem. Eng. J., 2023, 452, 139499 CrossRef CAS.
- X. Han, C. Yuan, B. Hou, L. Liu, H. Li, Y. Liu and Y. Cui, Chem. Soc. Rev., 2020, 49, 6248 RSC.
- Y. Lu, H. Zhang, Y. Zhu, P. J. Marriott and H. Wang, Adv. Funct. Mater., 2021, 31, 2101335 CrossRef CAS.
- H. L. Qian, S. T. Xu and X. P. Yan, Anal. Chem., 2023, 95, 388 CrossRef.
- E. L. Izake, J. Pharm. Sci., 2007, 96, 1659 CrossRef CAS PubMed.
- A. Tarafder and L. Miller, J. Chromatogr. A, 2021, 1638, 461878 CrossRef CAS PubMed.
- K. A. Schug and W. Lindner, J. Sep. Sci., 2005, 28, 1932 CrossRef CAS.
- E. Zor, H. Bingol and M. Ersoz, TrAC, Trends Anal. Chem., 2019, 121, 115662 CrossRef CAS.
- R. Bhushan, Chem.–Asian J., 2023, 18, e202300825 CrossRef CAS.
- J. Zou, G. Q. Zhao, G. L. Zhao and J. G. Yu, Coord. Chem. Rev., 2022, 471, 214732 CrossRef CAS.
- G. Magna, M. Stefanelli, G. Pomarico, M. L. Naitana, D. Monti, C. Di Natale and R. Paolesse, Chemosensors, 2022, 10, 308 CrossRef CAS.
- F. Röck, N. Barsan and U. Weimar, Chem. Rev., 2008, 108, 705 CrossRef.
- Y. Tahara and K. Toko, IEEE Sens. J., 2013, 13, 3001 Search PubMed.
- K. Bodenhöfer, A. Hierlemann, J. Seemann, G. Gauglitz, B. Koppenhoefer and W. Göpel, Nature, 1997, 387, 577–580 CrossRef.
- K. Brudzewski, J. Ulaczyk, S. Osowski and T. Markiewicz, Sens. Actuators, B, 2007, 122, 493–502 CrossRef CAS.
- S. Okur, P. Qin, A. Chandresh, C. Li, Z. Zhang, U. Lemmer and L. Heinke, Angew Chem. Int. Ed. Engl., 2021, 60, 3566–3571 CrossRef CAS.
- P. Maho, C. Herrier, T. Livache, G. Rolland, P. Comon and S. Barthelmé, Biosens. Bioelectron., 2020, 159, 112183 CrossRef CAS.
- K. Zhan, Y. Jiang and L. Heinke, Chem. Commun., 2023, 59(56), 8704–8707 RSC.
- S. Hassanpour, N. Niaei and J. Petr, Chemosensors, 2022, 11, 29 CrossRef.
- C. Cagliero, B. Sgorbini, C. Cordero, E. Liberto, P. Rubiolo and C. Bicchi, Isr. J. Chem., 2016, 56, 925 CrossRef CAS.
- J. Pico, E. M. Gerbrandt and S. D. Castellarin, Food Chem., 2022, 368, 130812 CrossRef CAS PubMed.
- C. Freissinet, A. Buch, C. Szopa and R. Sternberg, J. Chromatogr. A, 2013, 1306, 59 CrossRef CAS.
- X. Wu, X. Han, Q. Xu, Y. Liu, C. Yuan, S. Yang, Y. Liu, J. Jiang and Y. Cui, J. Am. Chem. Soc., 2019, 141, 7081 CrossRef CAS.
- Y. Yamazaki, T. Hitomi, C. Homma, T. Rungreungthanapol, M. Tanaka, K. Yamada, H. Hamasaki, Y. Sugizaki, A. Isobayashi, H. Tomizawa, M. Okochi and Y. Hayamizu, ACS Appl. Mater. Interfaces, 2024, 16, 18564 CrossRef CAS PubMed.
- C. Li, X.-X. Yang, M.-Y. Zheng, Z.-G. Gu and J. Zhan, Adv. Funct. Mater., 2024, 2401102 CrossRef.
- S. Okur, P. Qin, A. Chandresh, C. Li, Z. Zhang, U. Lemmer and L. Heinke, Angew Chem. Int. Ed. Engl., 2021, 60, 3566 CrossRef CAS.
- P. Maho, C. Herrier, T. Livache, G. Rolland, P. Comon and S. Barthelmé, Biosens. Bioelectron., 2020, 159, 112183 CrossRef CAS PubMed.
- L. Torsi, G. M. Farinola, F. Marinelli, M. C. Tanese, O. Hassan Omar, L. Valli, F. Babudri, F. Palmisano, P. G. Zambonin and F. Naso, Nat. Mater., 2008, 7, 412 CrossRef CAS PubMed.
- C. Di Natale, R. Paolesse, A. MacAgnano, A. Mantini, P. Mari and A. D'Amico, Sens. Actuators, B, 2000, 68, 319 CrossRef CAS.
- C. Di Natale, E. Martinelli, G. Magna, F. Mandoj, D. Monti, S. Nardis, M. Stefanelli and R. Paolesse, J. Porphyrins Phthalocyanines, 2017, 21, 769 CrossRef CAS.
- C. Di Natale, A. Macagnano, E. Martinelli, R. Paolesse, G. D'Arcangelo, C. Roscioni, A. Finazzi-Agrò and A. D'Amico, Biosens. Bioelectron., 2003, 18, 1209 CrossRef CAS.
- C. Di Natale, G. Olafsdottir, S. Einarsson, E. Martinelli, R. Paolesse and A. D'Amico, Sens. Actuators, B, 2000, 68, 572 CrossRef.
- G. Magna, M. Šakarašvili, M. Stefanelli, G. Giancane, S. Bettini, L. Valli, L. Ustrnul, V. Borovkov, R. Aav, D. Monti, C. Di Natale and R. Paolesse, ACS Appl. Mater. Interfaces, 2023, 15, 30674 CrossRef CAS.
- M. Stefanelli, G. Magna, F. Zurlo, F. M. Caso, E. Di Bartolomeo, S. Antonaroli, M. Venanzi, R. Paolesse, C. Di Natale and D. Monti, ACS Appl. Mater. Interfaces, 2019, 11, 12077 CrossRef CAS.
- G. Magna, M. Muduganti, M. Stefanelli, Y. Sivalingam, F. Zurlo, E. Di Bartolomeo, A. Catini, E. Martinelli, R. Paolesse and C. Di Natale, ACS Appl. Nano Mater., 2021, 4, 414 CrossRef CAS.
- M. Mudiganti, G. Magna, L. di Zazzo, M. Stefanelli, R. Capuano, A. Catini, L. Duranti, E. Di Bartolomeo, Y. Sivalingam, S. Bernardini, R. Paolesse and C. Di Natale, Sens. Actuators, B, 2022, 369, 132329 CrossRef CAS.
- G. Magna, Y. Sivalingam, E. Martinelli, G. Pomarico, F. Basoli, R. Paolesse and C. Di Natale, Anal. Chim. Acta, 2014, 210, 86 CrossRef.
- G. Magna, S. Dinc Zor, A. Catini, R. Capuano, F. Basoli, E. Martinelli, R. Paolesse and C. Di Natale, Sens. Actuators, B, 2017, 524, 524–532 CrossRef.
- Z. Anfar, B. Kuppan, A. Scalabre, R. Nag, E. Pouget, S. Nlate, G. Magna, I. Di Filippo, D. Monti, M. L. Naitana, M. Stefanelli, T. Nikonovich, V. Borovkov, R. Aav, R. Paolesse and R. Oda, J. Phys. Chem. B, 2024, 128(6), 1550 CrossRef CAS.
- M. Stefanelli, G. Magna, C. Di Natale, R. Paolesse and D. Monti, Int. J. Mol. Sci., 2022, 23, 15587 CrossRef CAS PubMed.
- A. Brizard, C. Aimé, T. Labrot, I. Huc, D. Berthier, F. Artzner, B. Desbat and R. Oda, J. Am. Chem. Soc., 2007, 129, 3754 CrossRef CAS.
- T. Delclos, C. Aimé, E. Pouget, A. Brizard, I. Huc, M. H. Delville and R. Oda, Nano Lett., 2008, 8, 1929 CrossRef CAS.
- Z. Cao, A. Scalabre, S. Nlate, S. Buffière, R. Oda, E. Pouget and B. Bibal, Chem.–Eur. J., 2020, 27, 427 CrossRef PubMed.
- M. Stefanelli, M. Savioli, F. Zurlo, G. Magna, S. Belviso, G. Marsico, S. Superchi, M. Venanzi, C. Di Natale, R. Paolesse and D. Monti, Front. Chem., 2020, 8, 587842 CrossRef CAS PubMed.
- G. Magna, R. Belugina, F. Mandoj, A. Catini, A. V. Legin, R. Paolesse and C. Di Natale, Sens. Actuators, B, 2020, 320, 128373 CrossRef CAS.
- M. A. Espinosa Díaz, T. Guetachew, P. Landy, J. Jose and A. Voilley, Fluid Phase Equilib., 1999, 157, 257 CrossRef.
- M. T. Sanz, S. Beltrán, B. Calvo, J. L. Cabezas and J. Coca, J. Chem. Eng. Data, 2003, 48, 1446 CrossRef CAS.
- W. Liu, J. Zheng, S. Li, R. Wang, Z. Lin and H. Yang, J. Mater. Chem. B, 2015, 31, 6528 RSC.
- R. Nag, Y. Okazaki, A. Scalabre, Z. Anfar, S. Nlate, T. Buffeteau, R. Oda and E. Pouget, Chem. Commun., 2022, 97, 13515 RSC.
- X. Shen, Q. Wang, Y. Liu, W. Xue, L. Ma, S. Feng, M. Wan, F. Wang and C. Mao, Sci. Rep., 2016, 6, 28989 CrossRef CAS PubMed.
- A. Scalabre, A. M. Gutiérrez-Vílchez, Á. Sastre-Santos, F. Fernández-Lázaro, D. M. Bassani and R. Oda, J. Phys. Chem. C, 2020, 124, 23839 CrossRef CAS.
Footnote |
† Electronic supplementary information (ESI) available: Materials, QMBs preparation, spectroscopic characterization and sensor measurements. See DOI: https://doi.org/10.1039/d4na00217b |
|
This journal is © The Royal Society of Chemistry 2024 |