DOI:
10.1039/D2CC06517G
(Highlight)
Chem. Commun., 2023,
59, 4838-4851
Carbon nanopores for DNA sequencing: a review on nanopore materials
Received
30th November 2022
, Accepted 14th March 2023
First published on 15th March 2023
Abstract
In the past few decades, nanometer-scale pores have been employed as a powerful tool for sensing biological molecules. In pursuit of this technology, a variety of nanotechnology-based approaches have been explored and established, especially nanopore sequencing. In comparison to the existing pores in other materials such as Si3N4, carbon nanopores have the ability to rapidly sense various biological molecules at single-molecule resolution and with reduced cost. Different from most reviews about nanopore sequencing, herein, we focus on the nanopore materials employed for sequencing applications. Initially, we provide an overview on the general issues associated with nanopore sequencing, concentrating on the recent progress and achievements in nanopore sequencing, especially using various carbon nanomaterials such as graphene and carbon nanotubes. Finally, the future research directions using carbon nanomaterials for nanopore sequencing are discussed and outlined.
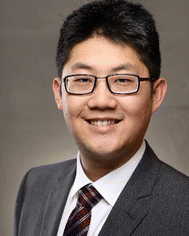
Jing Xu
| Dr Jing Xu obtained his PhD degree in 2019 under the supervision of Prof. Xin Jiang at the University of Siegen (Siegen, Germany). Currently, he works as a Distinguished Professor and Academic Leader at the College of Materials and Metallurgy, Guizhou University, China. He works on the growth and electrochemical applications of advanced carbon materials and is committed to cross-field research work on energy, sensors and water treatment. |
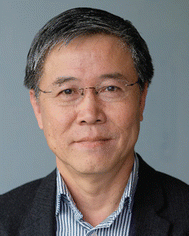
Xin Jiang
| Dr Xin Jiang has been a Professor and the Chair of Surface and Materials Technology at the University of Siegen (Siegen, Germany) since 2003. He received the award ‘‘State Specially Recruited Expert’’ of China in 2013. He was a Changjiang-Visiting Chair Professor at Dalian University and Science and Technology, China. His current research fields cover the growth and applications of diamond thin films, nanomaterials and materials characterization. |
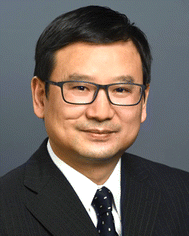
Nianjun Yang
| Dr Nianjun Yang has been a Professor of Electrochemistry and Catalysis at the Department of Chemistry and Institute of Materials Research (IMO-IMOEC) of Hasselt University (Belgium) since March 2023. His Habilitation in the fields of Materials Science and Surface Engineering finished in 2020 at the University of Siegen. He works on the growth and electrochemical applications of various functional materials. |
Introduction
Biological molecule sequencing is one of the most important approaches to explore the blueprint of life on Earth.1 In 1953, Francis Crick and James D. Watson firstly found the double helix structure of DNA molecules, which consists of a deoxyribose sugar and a phosphate backbone with sequences of four nucleic acid bases, namely, adenine (A), guanine (G), cytosine (C), and thymine (T).2–4 The number and specific order of these four nucleobases in DNA strands determine biological information and functionalities. To get genomic information, sequencing of these nucleobases are required, which provides an opportunity to prevent and diagnose various human diseases and further develop specific and personal medicines.5,6
The process of biological molecule sequencing involves precisely determining the amount and distribution of the four nucleobases in DNA molecules.7–9 It should be noted that biological genomes have large variations and complexity due to different biological functions. Taking the human genome as an example, it consists of approximately three billion nucleobases.10,11 Thus, the development of inexpensive, fast and simple DNA sequencing methods is essential to enable the detection of entire genomes and can increase the pace of genome technology development and revolutionize medicine and technology.12,13 Consequently, the National Human Genome Research Institute of the National Institutes of Health has launched a program, widely known as the Advanced Sequencing Technology Program, for the development of new DNA sequencing methods. The goal of this program is to reduce the cost of sequencing to $1000, and simultaneously increase the accuracy (<1 error/10
000 bases), long read length (>10 kb or longer), and high throughput (within hours or even minutes).14
Triggered by this program, various techniques have been proposed and developed to visualize DNA sequences. In general, they can be classified into four generations including chain-termination-based Sanger sequencing as the first generation, amplification-based cyclic-array sequencing as the second generation, single-molecule sequencing as the third generation, and nanopore sequencing as the fourth generation.15–21
In the mid-70s, Sanger and Coulson used fluorescently labeled di-deoxynucleotides as chain terminators.22 The variation in Sanger sequencing, such as Maxam and Gilbert sequencing, can shorten the sequencing time by simplifying the template preparation.23 This method was later known as the first generation of sequencing techniques. Its main limitation is low throughput (80–100 kb per hour). Furthermore, its capillary nature limits its scalable use. Besides, large projects such as the Human Genome Project, which emerged in 1990, requires tremendous workload and extremely high cost.24,25
The second generation of DNA sequencing technologies relies on the sequencing of a dense array of DNA molecules. It features iterative cycles of enzymatic manipulation and imaging-based data collection.26 This type of array-based DNA sequencing enables a much higher degree of parallelism sequencing. Specifically, millions of sequencing reads can be obtained in parallel by rastered imaging of an effective size. Given that it broke through the bottleneck of the electrophoresis process, which limited the efficiencies of the first-generation sequencing technologies,27 this second-generation sequencing technology provided the chance to sequence an entire genome at an unprecedented speed with a reasonable cost. In 2005, the pyrosequencing method, developed by 454 Life Sciences (acquired by Roche now), was released on market. It uses the cyclic flowing of nucleotide reagents (repeatedly flowing T, A, C, G) over a platform.28,29 This was the first commercial setup of second-generation sequencing technology. The platform contains approximately one million wells, which are loaded with sequencing enzymes and primer. Then, the platform is exposed to a flow of one unlabeled nucleotide, allowing the synthesis of the complementary DNA strand. When a nucleotide is incorporated, pyrophosphate is released. The resultant light emission is monitored in real time. The 454 Sequencer generates about 200
000 reads (20 Mb) of 110 base-pairs (bp).9,30 However, second-generation sequencing technologies suffer from low read-length and accuracy compare to the first-generation Sanger sequencing.26,31
Third-generation sequencing technology is based on single-molecule sequencing.1,18,32 Currently, several platforms of this generation are available in the market from companies such as HeliScope Biosciences TIRM and Pacific Biosciences SMART. Compared to the previous generations, single-molecule sequencing does not require cloning, amplification and fluorescent labelling, further reducing costs and increasing the sequencing speeds.33–35 An exonuclease enzyme is used to cleave individual nucleotide molecules from the DNA strands. These nucleotides can be identified in the correct order, when they are coupled to an appropriate detection system.36 This real-time DNA sequencing technology provides read lengths that typically exceed 5 kb, facilitating high confidence mapping across a greater percentage of the genome. Unfortunately, the individual read accuracy of the single-molecular reading length is relatively low (∼85%)37 due to the low signal intensity and high background noise. Therefore, single-molecule sequencing technology requires multiple repetitions to calibrate the DNA sequencing results.38,39
Fourth-generation sequencing technology is widely known as nanopore sequencing.21,40 In this technology, nanopores, also called nanochannels, nanoribbons or nanopipettes in many cases as well as their arrays are essential. They provide the fundamentals and theoretical concepts of nano-fluidics for future technologies such as single-molecule analytics and lab-on-a-chip applications.41,42 It should be noted that these non-nanopore sequencing technologies require complex sample preparation and further complicated algorithms for data processing.43,44 Therefore, the costs of these technologies are high, but their throughput is low and the related read lengths are short. Differently, nanopore sequencing is derived from Coulter counter and ion channels, namely, based on the molecular translocation events passing through a tiny nanopore. Nanopore analysis is an emerging technique, which involves monitoring the change in ionic current as biological molecules move through a nanopore.45,46 In this case, the ionic current signal is reduced or even blocked when a DNA molecule is driven through a nanopore. Determined by the amplitudes of reduced ionic currents, both long-length polymers (e.g., single-stranded genomic DNA or RNA) and small-sized molecules (e.g., nucleosides) can be identified and characterized even without amplification or labeling.47,48 This unique technology enables inexpensive and rapid DNA sequencing. In the past few years, significant progress and achievements in DNA nanopore sequencing have been achieved. In 2014, several companies, including Oxford Nanopore Technologies (ONT) have commercially marketed nanopore sequencing devices (e.g., MiniION), which achieves a read length of up to 2 Mb.49
In this review article, we summarize the recent advances in DNA sequencing using carbon nanopores (Fig. 1). Firstly, we introduce to the progress in technology for nanopore sequencing, covering the characteristics of nanopores, employed materials, and existing challenges. In the next section, the recent progress and achievements in the use of carbon nanomaterials such as graphene and carbon nanotubes (CNTs) for nanopore sequencing are highlighted. As future perspectives of nanopore sequencing, the fabrication of novel members and their nanopores (e.g., ultrathin diamond membranes and nanopores) as well as their applications for nanopore sequencing are discussed and outlined. It is worth mentioning that this review focuses on the materials with respect to the selection of carbon nanomaterials and technologies for nanopore formation. This is different from most of the published review articles, which focused on the performance of nanopore sequencing events (e.g., sensitivity and devices).
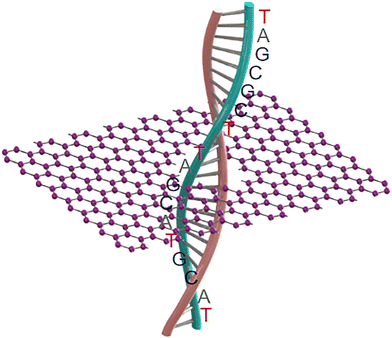 |
| Fig. 1 Schematic DNA sequencing through a carbon nanopore. | |
1. Nanopore sequencing
1.1. DNA sequencing
DNA is a biological heteropolymer, consisting of four nucleotide monomers, i.e., adenine (A), cytosine (C), guanine (G), thymine (T). The DNA sequencing is the process of determining the exact order of these nucleotides in a DNA molecule.
The significance of DNA sequencing is its ability to unlock the secrets of the genetic code. This information can be used to understand the genetic basis of various traits, diseases, and conditions. It also helps in the identification of genetic mutations and variations, which can have significant implications in medical diagnosis, treatment, and drug development.
The ultimate goal of DNA sequencing is to achieve cheap, fast and accurate sequencing. One of the most exciting areas of research is the field of personalized medicine, which aims to tailor treatments based on individual genetic information. Nanopore sequencing technologies enable greater insight into the basis of genetic diseases. For example, DNA sequencing has been used in clinical applications to identify mutations that cause inherited diseases and tumor development pathways.50 It has also been used to track and diagnose the spread of infectious diseases such as novel coronavirus disease 2019 (COVID-19).51
1.2. Nanopore characteristics
Nanometer-scale pores have been widely used for various applications such as energy conversion,52 energy storage,53 drug delivery,54 enzymology,55 polymer data storage,56 biosensors,57 biomarker detection,58 nanoparticle fabrication,59 and nanoscale chemical reactors.60 It has been confirmed that sequencing DNA with nanopores offers exciting potential advantages over other sequencing technologies.61,62 A nanopore sequencing device consists of a nanometer-sized hole in an impermeable membrane, which separates two chambers containing electrolyte solution (e.g., KCl).63 When a voltage is applied across the membrane, ions flow through the pore, resulting in a steady-state ionic current.64,65 The presence of a single molecule in the nanopore leads to a transient change in the ionic current, and this change can be detected with an electronic equipment. A distinguishing feature of nanopore sequencing is that it can be used to analyze not only small molecules but also long biopolymers (e.g., DNA, RNA and proteins),66–68 where good understanding of the interactions between the molecules and the nanopore is required. Thus, the geometry of a nanopore is critical, i.e., its depth and diameter. The former depends on the thickness of the membrane, which is determined during the fabrication steps. This characteristic length scale determines the current and selectivity of the ions in the confined area outside the nanopore.69 The latter determines the largest molecule that can move through the nanopore or the type of molecules that can be analyzed. The local electric field of a nanopore, influenced by the nanopore surface chemistry, is another factor influencing the performance of nanopore sequencing.70 For example, the introduction of surface charges (positive, negative or neutral) and/or a variation in the wettability (hydrophobic or hydrophilic) of a nanopore by the addition of functional groups (e.g., carboxylic, hydroxide silane, S–H and S–S groups) on the surface of a membrane change the performance of nanopore sequencing.71
Based on the applied materials, the used nanopores for DNA sequencing can be classified into biological and solid-state nanopores. A biological nanopore is usually composed of a pore containing proteins, which are self-assembled or inserted into a transmembrane. This type of biological nanopore has been widely used in single-molecule detection, disease diagnosis, and DNA sequencing.72,73 In the case of synthetic solid-state nanopores, dielectric materials (e.g., silicon nitride and aluminum oxide) and nanocarbons (e.g., graphene and carbon nanotube) have been frequently employed.
1.3. Biological nanopores
A cylindrical nanopore or channel can be naturally formed in a protein membrane.74 The functions of biological pores are diverse in nature, for example, toxins (e.g., α-hemolysin75), viral pores (e.g., phi2976), mycobacterial porins (e.g., MspA77) and nuclear pore complexes (e.g., nucleoporins78). Once a typical biological nanopore is embedded in a soft substrate (e.g., liposome or lipid membrane), cis and trans events can be separated in a reservoir filled with an electrolyte solution. Consequently, various biological nanopores have been utilized for nanopore sequencing.
There are many advantages in using biological nanopores for DNA sequencing. For example, biological nanopores show well-defined and highly reproducible sizes and structures. Taking the α-hemolysin nanopore as an example, it consists of a 3.6 nm cap and a 2.6 nm transmembrane β-barrel in diameter. Consequently, it can be facilely inserted into membrane bilayers or other artificial supporters. Its narrow and short channel is close to the diameter (∼1.3 nm) of a single-stranded DNA (ssDNA) molecule, allowing the analysis of single nucleotides by use of reduced/blocked ionic currents inside the nanopore.
Although bacterial toxins are inherently stable, the main weakness of biological nanopores comes from their supporting membranes, i.e., lipid bilayers. This is because the bilayer is very sensitive to temperature, voltage, induced stress and pH. Specifically, it has a short lifetime. Another challenge of biological nanopores is their limited pore size. For example, the MspA nanopore has a size of ∼1.2 nm, while the Phi 29 nanopore has a pore size in the range of ∼3.6–6 nm. Therefore, a reliable technique needs to control the size of biological nanopores. Given that most biological nanopores are formed by repeated arrangement of the monomers, various nanopore sizes/shapes can be obtained by engineering the protein oligomeric composition. For example, it was observed that self-assembled nanopores on Fragaceatoxin C (FraC) can possess varying shapes and size distributions, simply through engineering the protein oligomeric compositions and the modification of the related lipid interfaces (Fig. 2a).79 The size of the nanopores was controlled by mixing three types of FraC nanopores with different proportions and sizes. Type I FraC exhibits the widest nanopore with a diameter of 1.6 nm. The nanopore in Type II and Type III of FraC has a diameter of 1.1 and 0.84 nm, respectively (Fig. 2b). The types of FraC nanopores were adjusted by using different preparation conditions. During the oligomerization, lower concentrations of monomers increased the content of lower molecular mass oligomers, leading to smaller nanopores (Type II and Type III). The oligomerization of monomers under alkaline conditions (e.g., pH 7.5) tended to enlarge the pores sizes compared to that obtained under acid conditions (e.g., pH 4.5). More importantly, these three nanopores could be separated by chromatography using an imidazole gradient. The obtained FraC nanopores allowed direct analysis of a wide range of peptide lengths with high sequencing speeds.
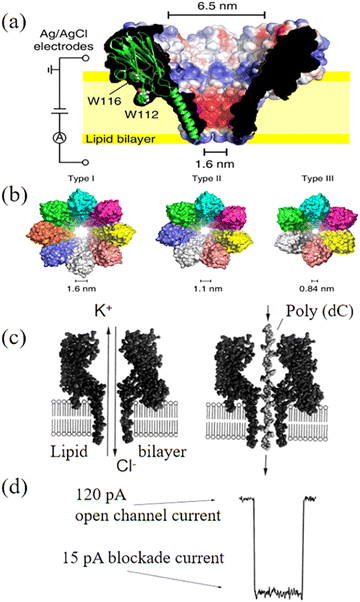 |
| Fig. 2 (a) Cut through of a surface representation of wild-type FraC. (b) Molecular models of the three types of FraC nanopores constructed from the FraC crystal structure.79 (c) DNA strand driven through the pore under ionic current of KCl solution and (d) appearance of blockade of ionic current due to the translocation.80 Figures adapted with permission from National Academy of Sciences, U.S.A., Copyright (1996) and the American Chemical Society, Copyright (2022). | |
The use of nanoscopic pores to investigate macromolecules in solution has been widely researched. The ionic solution (e.g., KCl)-filled chambers are separated by a voltage-biased membrane. The negative ions and positive ions are contained on either side of membrane, which refer to cis and trans chambers, respectively. An applied electric field drives the K+ ions from the trans chamber to the cis chamber and Cl− ions from the cis to trans through the nanopores. Generally, the applied voltage is positive on the trans side. During the analysis, the DNA is electrophoretically driven through biological nanopores from the cis and trans chamber to produce an electrical signal containing sequence information (Fig. 2c). Translocation of the polynucleotide through the nanopore is controlled by a motor enzyme, resulting in transient blockade of the ionic current (Fig. 2d).80
1.4. Solid-state nanopores
Solid-state nanopores have attracted more attention than biological nanopores for fourth-generation DNA sequencing due to their high stability in a wide range of analyte solutions and environments81,82 and their advantages of robustness and processability over biological nanopores.83 More importantly, the size and shape of solid-state nanopores can be flexibly controlled. Solid-state nanopores are usually fabricated in very thin (<50 nm) synthetic membranes. Several dielectric membranes (e.g., Al2O3, HfO2, TiO2, and SiNx) have been utilized as supporting membranes for as-fabricated solid-state nanopores.83,84 Later, SiO2, polymers, MoS2, hBN, WS2 and MXenes have also been applied for nanopore applications.85–89
Several methods have been utilized to fabricate nanopores on these relatively hard materials.90,91 Coupled with advanced semiconductor fabrication techniques such as laser etching,92 focus ion beam (FIB) milling,93 and transmission electron microcopy (TEM) drilling,94 the nanopore dimensions can be tuned to meet environmental and analyte conditions in a wide range. Nanopores with dimensions of a few nanometers were firstly fabricated on an Si3N4 membrane via reactive ion etching. This nanopore was bowl-shaped, and thus required further milling through Ar+ ions. Currently, it is more common to drill nanopores in a solid-state membrane using a TEM (typically with an accelerated voltage of about 200–300 kV). The shape, dimensions, and location of the nanopores can be monitored and controlled in real time. In this regard, electron beam drill technology conceptually provides the opportunity for the scalable production of nanopores and their nanopore arrays with high accuracy (in the order of sub-nanometers) and desired shapes.95 However, electron/ion beam techniques require expensive precision devices. Due to the physical characteristics of dielectric materials, the fabrication of ultrathin, defect-free and stress-free membranes is practically difficult.82 In addition, drilling nanopores with diameters of less than 10 nm is still challenging. To date, DNA sequencing with single-base resolution with these materials is still unsuccessful.96 The thickness of these nanopores is usually much thicker than the length of nucleotide bases, which makes them hard to read single nucleotide information from a long chain of DNA strands. The sensitivity of nanopore sequencing technology needs to be further improved. Therefore, the formation of solid-state nanopores from other new membrane materials such as carbon nanopores is still significant.
2. Carbon nanopores
Carbon, the sixth element in the periodic table, forms a variety of bulk materials (e.g., graphite and diamond) and nanomaterials (e.g., fullerene, carbon nanotubes, graphene, and graphyne). Among them, carbon nanomaterials are extremely appealing due to their low mass densities, excellent thermal conductivities, and high biocompatibility.97–99 Carbon-based materials provide abundant resources for the design of various micro- and nanostructures such as nanopores and nanochannels. For example, graphene nanopores can be initially generated through TEM milling of single-layered graphene layers. When the size of the graphene nanopore is small enough or comparable with the size of DNA molecules, the passage of a DNA molecule leads to the blockage of the related ionic currents. To record the blocked ionic current, the graphene sheet with the nanopore needs to be inserted in an electrolyte and a voltage needs to be further applied on the two sides of this graphene sheet. Due to their different properties (e.g., size and density of electrons), the four DNA bases block the ionic current differently. According to the amplitudes and frequencies of the blocked ionic currents, the type and order of the four DNA bases inside DNA molecules can be identified. This nanopore sequencing technique has been shown to have many potential applications in biomolecular sensing, DNA nanopore sequencing, and early disease diagnosis. The structures and properties of different carbon materials are dependent on the arrangement of carbon atoms, namely, their hybrid states.100–102 These unique properties of carbon nanomaterials have led to their high potential for sensing and sequencing applications (Fig. 3).
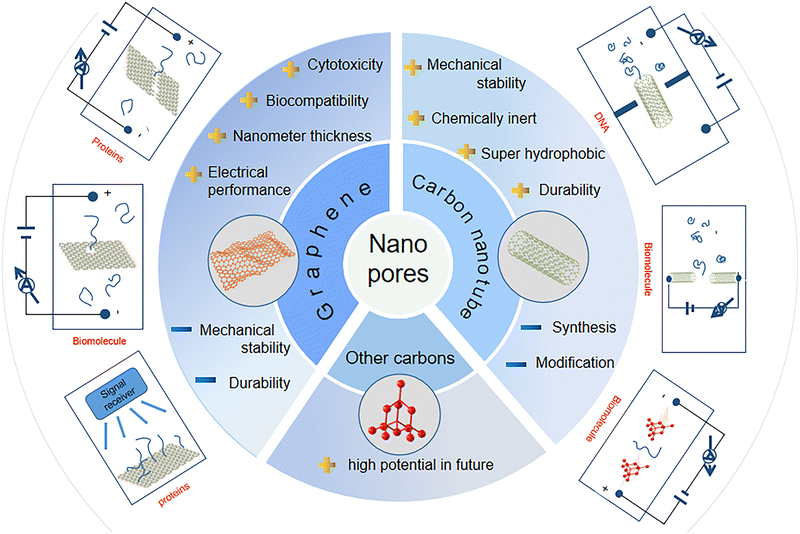 |
| Fig. 3 Overview of carbon nanopores for DNA sequencing technologies. | |
2.1. Graphene
Graphene is a member of the carbon nanomaterial family. It contains sp2-hybridized carbon atoms, which are positioned in a honeycomb lattice in two dimensions.103 In 2004, British scientists Andre Geim and Konstantin Novoselov successfully separated graphene from graphite using a micro-computer peeling method.104 The structure of graphene is composed of a layer of independent sp2 hybrid carbon atoms, which are arranged in a hexagonal honeycomb crystal structure.105 Every carbon atom in graphene is bonded to three adjacent carbon atoms through a σ bond. The bonding direction is in a lateral plane. Due to the short C–C bond length (∼0.142 nm), the structure of graphene is stable.106 The thickness of monolayer graphene is 0.34 nm, which is equivalent to the spatial interval between two adjacent nucleotides.20 In this context, a graphene nanopore offers the possibility of DNA sequencing at a single-base resolution.
2.1.1. Graphene synthesis.
Presently, there are numerous methods for the synthesis of graphene, including mechanical stripping,107 liquid-phase exfoliation,108 chemical vapor deposition (CVD),109 and epitaxial growth methods.110 Among them, the CVD growth of graphene111 on transition metal substrates such as copper (Cu), nickel (Ni) and cobalt (Co)112–114 has become the most promising approach for the synthesis of graphene. During the CVD process, gas precursors (e.g., a mixture of H2 and CH4) are fed into a heated CVD reactor, where the hydrocarbon precursors decompose into carbon radicals. Once they are diffused and adsorbed on the metal substrate surface, the growth of single-layer and few-layers graphene occurs.115,116 During the CVD process, the kinetics of the CVD growth of graphene is dependent on the metal substrate employed (e.g., material type, roughness, lattice, and purity) and growth parameters (e.g., precursors, gas pressure, gas flow rate, growth time, and temperature).109,117–119 Given that different transition metals possess varying catalytic activity and solubility, they determine the deposition mechanisms of graphene on them. In turn, they define the morphology (e.g., domain size and boundaries) and thickness of the as-grown graphene layers. For example, the graphene films grown on Ni foils do not possess uniform monolayers. This is because Ni can dissolve carbon atoms, even at their high concentrations. Thus, the growth of graphene is mainly attributed to the precipitation during the cooling process. Consequently, a mixture of graphene monolayers and few-layered graphene is obtained in most cases.120 Alternatively, a Cu plate is an excellent candidate to produce ultrathin graphene films with large areas and uniform thicknesses. This is due to the low solubility (0.001–0.008 wt% at 1084 °C) of carbon atoms in a Cu plate. Given only soft bonds between Cu and carbon can be formed, graphitic carbon formation is facilitated, ultimately contributing to the improved uniformity of the graphene layer thickness.120,121 To obtain monolayer graphene, it is crucial to precisely control the number of graphene layers during CVD. In most cases, post-growth layer transfer and etching processes (for nanopores opening is very desired) are required.122,123 It should be highlighted here that the CVD method is inexpensive, and thus can be considered a reliable and controllable technological process to fabricate large-area and high-quality graphene on transition metals. To date, the size of graphene has already reached as large as 30 inches via the CVD method.
2.1.2. Graphene nanopores.
Graphene nanopores inherit most of the unique properties of graphene. Due to the excellent electrical sensitivity and single-atom thickness of graphene itself, the transport rates of molecules through graphene nanopores are expected to be high.124 To achieve a high-quality sequencing performance, the technique for the formation of graphene nanopores needs the following characteristics. Firstly, the size of the as-fabricated graphene nanopores should be comparable to the diameters of DNA molecules. Only in this situation the change in ionic current can be enhanced when a DNA molecule passes through the nanopore. Secondly, the method must be effective, controllable, and cost-effective.125 To date, the reported methods to produce graphene nanopores can be categorized into direct drilling techniques (also called top-down approach), chemical etching techniques, and on-surface synthetic techniques.
The direct drilling technique is mainly based on the irradiation of graphene with highly energetic electrons or ion beams, such as focused ion beam (FIB), focused electron beam (FEB), block copolymer lithography (BCL), nano-particle lithography (NPL), nano-imprint lithography (NIL) and oxygen plasma etching. These focused beam irradiation methods produce nanopores directly on single- or multi-layer graphene in only one step. In 2008, graphene nanopores were firstly fabricated in suspended multilayer graphene using FEB irradiation in a transmission electron microscope (TEM).126 Utilizing this technique, graphene nanopores with various shapes (such as Hall rods,127 nanobelts,128 quantum dots129 and nanogaps130) and sizes have been obtained. The size of graphene nanopores is usually determined by the energy of ion/electron irradiation and the diameter of the beam spot. Therefore, directly “drilling” nanopores to the desired sizes on graphene layers is theoretically the most straightforward method to fabricate nanopores. Practically, the realization of controlled nanometer-scale drilling is still challenging, especially using FIB. Experimentally, the size of graphene nanopores fabricated by traditional FIB is usually above 10 nm. To obtain smaller graphene nanopores such as those with the sizes of sub-5 nm, shrinkage of the graphene nanopores has been realized in the temperature range of 400–1200 °C by setting the irradiation energies.131 To further increase the crystallization of the graphene layers, various pore-forming temperatures have been applied in the apparatus. The utilization of a helium ion beam (HIM) led to the generation of ultrasmall (∼3.7 nm) graphene nanopores given that the diameter of the ion source beam can reach as small as ∼0.5 nm with an accelerating voltage of 30–35 kV (Fig. 4a).132 The size of the nanopores was easily controlled by various exposure times of the HIM. It should be noted that for all these direct drilling techniques, expensive equipment is required together with experienced personnel. Therefore, they cannot be applied for the industrial production of graphene nanopores in most cases.
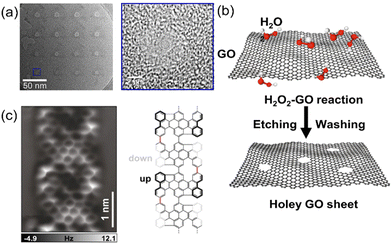 |
| Fig. 4 (a) TEM image of a representative graphene nanopore array and magnified TEM image of a nanopore with an exposure time of 0.1 s. A helium ion beam microscope was used to produce the single-layer graphene.132 (b) Carbon atoms in the actively defective zones of GO can be oxidized by H2O2, thereby generating nanopores gradually.144 (c) AFM image acquired on the graphene nanoribbon segment and a scheme of its chemical structure.145 Figures adapted with permission from Elsevier, Copyright (2021), Nature Publishing Group, Copyright (2022), and John Wiley and Sons, Copyright (2022). | |
The chemical etching technique is the second approach to prepare graphene nanopores, which allows the large-scale production of graphene nanopores with low costs and less time.133 For example, graphene nanopores with a diameter as small as 2 nm were fabricated in both exfoliated and CVD-grown graphene layers.134–138 As a derivative of graphene, graphene oxide (GO) has been utilized to produce nanopores. It is comprised of carbon and oxygen atoms in plate-like structure.139–141 GO is often prepared using the Hummers’ method, where a strong oxidant mixture (e.g., a combination of potassium permanganate and sulfuric acid) is used to oxidize graphite.142,143 These atomically thin sheets or flakes are stacked into a laminate structure with atomic-scale point defects and pathways, allowing molecular transport (Fig. 4b).144 In contrast, an exfoliated graphene layer contains defects, enabling the selection of graphene sheets with a range of thicknesses. During the chemical etching process, the shape and size of the graphene nanopores are determined by the concentration of the etching solution and the etching time or temperature. Clearly, chemical etching is very hard to precisely control the size, shape, and density of graphene nanopores.
Recently, on-surface synthesis under ultrahigh vacuum condition or at the solid–liquid or solid–vapor interface has been extensively used as a new approach to fabricate low-dimensional carbon nanostructures.145 The most representative on-surface reaction is Ullmann coupling (Fig. 4c), which has been applied for the fabrication of a variety of graphene-related nanostructures.146–148 This technology requires the careful design of the monomer precursors (e.g., diphenyl-10,10′-dibromo-9,9′-bianthracene146 and 2,7,11,16-tetrabromotetrabenzo149). These monomers are further employed for related polymer chain reactions on selected substrates, usually on a gold surface. Subsequently, the polymerized graphene nanoribbons are activated through thermal treatment/reactions. In the last step, ordered graphene nanopore arrays can be obtained via the interconnection of the graphene nanoribbons, which have sizes of around 1 nm. Depending on the inner edge structure, these graphene nanopores can have either a planar or a nonplanar geometry.146 In this context, the size, density, and structure of these graphene nanopores are defined with atomic precision via the careful design/selection of the monomer precursors.
2.1.3. Challenges associated with graphene nanopores.
Previous studies have clearly shown that graphene nanopores are extremely promising for DNA sequencing. Table 1 summarizes the graphene nanopores that have been either experimentally fabricated or simulated by calculation for various DNA sequencings. Unfortunately, the signal-to noise ratio (SNR) of this approach is typically lower than 10.150–154 This is because the graphene nanopore sits at high ionic current noise levels, which are several orders of magnitude larger than that of dielectric materials (e.g., silicon nitride).155 In general, the noise spectrum is composed of both a high frequency regime (f > 1 kHz) and a low frequency one (f < 1 kHz).156 The former is associated with the membrane capacitance, whereas the latter with current fluctuation due to the 1/f characteristics.155 For graphene nanopores, the noises may come from both regimes. Moreover, graphene contains various surface defects.157 During the irradiation process, graphene nanopores are shown to heal spontaneously by filling up with non-hexagon, graphene-like structures. The resultant graphene nanopores have irregular geometries and are not stable.158 Specifically, graphene nanopores have poor stability and their sizes may change during the sequencing processes.
Table 1 Different graphene nanostructures and pore-forming processes used for DNA sequencing
Geometries of nanopores |
Pore-forming process |
Analyte |
Analytic method |
Ref. |
1.4–2.2 nm nanopore |
Helium ion beam |
sDNA of poly-dN20, poly-dN5, poly-dN3, and dNTP |
Ionic current-based sensing, current in bias of 200 mV |
132
|
4.5–48 nm nanopore |
FIB drilling and shrinking in SEM |
Homopolymer DNA |
Ionic current-based sensing, current in bias of 1000 mV |
172
|
5 nm nanopore, 30 nm nanoribbons |
E-beam lithography and oxygen plasma etching |
DNA |
Ionic current-based sensing, current at resistance and capacitance in bias of 300 mV |
154
|
10–25 nm nanopore |
Electrochemical etching |
λ-DNA |
Ionic current-based sensing, translocation time in 200 mV |
173
|
1.6–2.1 nm nanopore |
MD simulation |
Poly ssDNAs |
Ionic current-based sensing, current in bias of 2 V |
174
|
5 nm nanopore |
Helium ion beam |
Poly(dA), poly(dG), poly(dC), and poly(dT) |
Ionic current-based sensing, current in bias of 500 mV |
175
|
5 nm nanopore |
MD simulation |
DNA methylation |
Ionic current-based sensing and field-effect based sensing, in energy window from −0.2 to 0.1 eV |
176
|
1 nm hybrid nanopore |
MD simulation |
ssDNA |
Field-effect based sensing, the corresponding binding energy for each target molecule |
177
|
1.5, 2.1, 3.1, 4.1, and 5.1 nm nanopore |
Simulation |
dsDNA |
Ionic current-based sensing, current in bias of 2 V |
178
|
1–2 nm nanopore in nanoribbons |
Simulation |
DNA |
Field-effect based sensing, the corresponding binding energy for each target molecule |
179
|
1.4 nm nanopore |
Simulation |
ssDNA |
Field-effect based sensing, current sensitivity in bias of 1.1 V |
180
|
To overcome the noise of graphene membranes, one effect way is to increase the sensitivity of the graphene nanopores (e.g., by surface modification).159–162 For example, carboxyl group-terminated graphene nanochannels were obtained by immersing graphene nanochannels in a mixture of 1% polyethylenimine (PEI) and zirconium acetate solution.163 The functionalized graphene nanochannel was positively charged due to the presence of PEI and Zr4+ ions on its surface (Fig. 5). Under an external electric field, the long-chain molecules are easily accumulated on the nanochannel surface via the electrostatic interaction. The adsorption of the negatively charged dsDNA molecules altered the charges of the nanochannel surface with only a small amount of target miRNA. Consequently, the detection signal was enhanced and the detection concentration was in the range of 100 aM to 1 pM.172
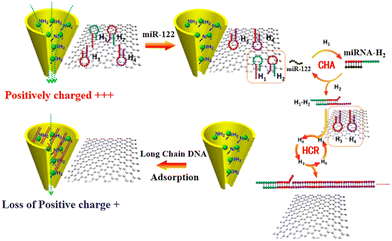 |
| Fig. 5 Schematic of the sensing strategy based on Zr4+–PEI-coated nanochannel biosensor for miR-122.163 Figure adapted with permission from the American Chemical Society, Copyright (2020). | |
It must be noted that it is difficult to directly observe the migration of molecules through nanopores in solution using traditional experimental analysis tools such as atomic force microscopy (AFM), TEM, and X-ray diffraction (XRD). Thus, understanding the dynamic behavior of molecules inside nanopores and related signal variation during the process of atomic-scale transport is extremely important given that it can provide important guidance to optimize nanopore sequencing technology.150,153,160,164 For example, molecular dynamics (MD) can directly track the trajectory of each molecule, ion, or water molecule inside a nanopore.165,166 The dynamic transport of DNA molecules through the nanopore and the corresponding ionic current can be simulated. In addition, the computational methods of quantum mechanics, such as density functional theory (DFT), can accurately predict the interaction of molecules with nanopores.167 This technique is based on the nuclear electron interaction mechanism and the principles of quantum mechanics.168 Combined with the non-equilibrium Green function, the transverse conductance or current in the nanopore can be calculated. The interatomic interactions between the analyte and nanopores can be calculated and predicted even without real tests. By using MD, information such as the interaction between the DNA and nanopore during the translocation process has been revealed.159,169–171 For example, the simulation of a graphene nanoribbon-based microfluid distinguished different peptide bonds.150 The nanopores located at different positions in the graphene nanoribbon array were used to detect different parts of the peptide chain. The nanopore in middle of the array was specifically used to collect signals triggered from other nanopores during translocation.165 The non-equilibrium Green's function method based on DFT was used to simulate the collected signals. Thus, the sequence information of peptide chain and the sequencing principle of the graphene nanoribbon array was obtained through MD simulations.
2.2. Carbon nanotubes
Carbon nanotubes (CNTs) consist of cylindrical nanostructures, made up of carbon atoms arranged in a unique pattern. Due to their high strength, thermal and electrical conductivity, and unique electronic properties, CNTs have gained attention in a wide range of applications such as DNA sequencing. CNTs offer potential advantages over other nanopore materials, such as improved signal-to-noise ratios and enhanced translocation speeds. For example, when a DNA molecule passes through a CNT, a large increase in the net ion current can be observed. This is because the large electro-osmotic flow from the CNT can be turned into a large net current, rather than a current blockage. Meanwhile, the construction of nanopores is relatively simple once CNTs are employed. Since their discovery in the late 20 century, carbon nanotubes (CNTs) have become the most studied one-dimensional (1D) nanostructures.181–184 They are comprised of sp2 carbon atoms, in the form of either single-wall nanotubes (SWNTs) or multi-walled nanotubes (MWNTs).185 SWNTs consist of a single graphene sheet, involving only hexagonal rings with double and single carbon–carbon bonding.186 CNTs are primarily produced by arc discharge,187 laser ablation,188 and catalyzed CVD method.189 However, the former two methods only produce low yields of CNTs. Similar to graphene, the CVD method is a more reliable technique for the large-scale production of CNTs.190 The CVD growth of CNTs involves the following basic steps: the dissociation of hydrocarbon gas molecules, atomic carbon saturation on the surface of catalytic nanoparticles, and carbon atom diffusion. Thus, the morphologies, structures, and properties of CNTs are determined by both the catalyst preparation and subsequent growth conditions. For example, the catalyst is critical for the CVD growth of CNTs. Different compositions and sizes of catalysts can lead to as-grown CNTs with different morphologies. In detail, the size of the catalyst often determines the diameter of the grown CNTs. A number of transition metals (e.g., Fe, Mo, Co, and Ni) have been applied for the catalytic growth of SWNTs, owing to the high solubility of carbon atoms and high diffusion rates of carbon atoms in these metallic catalysts.191 With respect to carbon sources, the most commonly fed gases are methane (CH4), ethylene (C2H4) and acetylene (C2H2). Their flow rates and related growth conditions (e.g., temperature and growth time) affect the length and morphology of the CNTs. In the CVD growth of CNTs, there are three growth modes, i.e., tip growth, base growth, and symmetrical growth. According to the different growth modes, the encapsulated catalytic nanoparticle is located at the top, bottom and middle of the CNT.192
It has been reported that long-length CNTs, especially those with large inner diameters (>50 nm) are not suitable for the translocation of biological molecules.193,194 Given that the fabrication of ultrashort CNTs is still technically challenging, it is important to develop a precise and effective “cutting” method to produce ultrashort CNTs. Meanwhile, the “cutting” method must avoid the formation of defects on the CNT walls. In this regard, various cutting processes such as sonication-assisted, chemical and plasma etching have been used to shorten ultralong CNTs.193,195 Using a mechanical shear force, long CNTs were cut into short ones.194 The obtained CNTs were further used to fabricate nanofluidic chips, revealing high potential for sensing single molecules, cations and ssDNA strands.
Another way to read the sequence of DNA molecules using CNTs is to let a DNA molecule pass through the nanogap between two aligned and functionalized CNTs (Fig. 6a).196,197 The current recorded on the CNT electrodes is from the tunneling current conducted via molecules passing through the membrane. Here, the CNTs act as transverse tunneling tips (Fig. 6b).197 By selecting the potential between the CNT electrodes, the speed of the molecule translocation can easily be controlled. Through this transverse tunneling, the current from CNTs was measured in the range of nano-amperes, which can probably solve the problem of the fast translocation speed of a DNA molecule given that the generated ionic current is only in the pico-ampere range, especially in a high frequency area.198 In this case, the movement of molecules in the electrolyte is only dependent on the gravity and drag force. The four DNA bases can be distinguished by their different electrical resistances.196–198
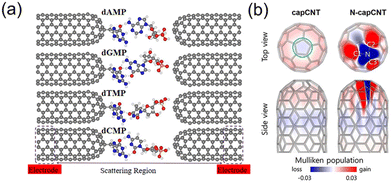 |
| Fig. 6 (a) Atomic structure of the functionalized closed-end cap CNT-based nanogap setup for the detection of four different nucleotides (dAMP, dGMP, dTMP, and dCMP). The CNT electrodes (left and right) are semi-infinite and periodic along the transport direction (z-axis).196 (b) Charge distributions in the pristine and N-doped capped CNTs.197 Figures adapted with permission from the American Chemical Society, Copyright (2018) and The Royal Society of Chemistry, Copyright (2020). | |
However, many challenges exist and hinder the development and practical applications of CNT nanopore sequencers. For example, the large-scale fabrication of CNTs with a particular structure still remains a major challenge. This is because CNTs are often prepared using flow-through heated reactive gases. Specifically, the size and geometrical uniformity of the CNTs, which determine the performance of CNT nanopore sequencers, are difficult to be precisely controlled. Also, the separation of different CNTs, especially in a particular structure is still difficult. Furthermore, the interactions between DNA and CNTs vary for each case, dramatically affecting the sensitivity of the CNT nanopore sequencer. It should be noted that the properties of CNTs are strongly dependent on the physical and chemical properties of the applied electrolytes. Once the temperature, content, and concentration of the electrolyte are changed during the sequencing analysis, it is possible to alter the accuracy of the sequencing results using a CNT nanopore sequencer.
2.3. Alternative carbon materials
The development of nanopore sequencing technologies is strongly dependent on the materials employed for the formation of the nanopore and the supporting membranes. In comparison to the existing and reported materials, diamond membranes are extremely attractive. They are expected to possess many advantages for nanopores sequencing, such as excellent chemical stability, biocompatibility, and long-term stability under extremely harsh conditions.199–202 Moreover, diamond films or free-standing diamond membranes feature flexibility with a reduction in the film thickness.21 Modification of the surface of diamond (e.g., hydrophilic or hydrophobic surface) can easily be achieved by varying its terminations or adding functional groups on its surface.8 The doping during the CVD growth of diamond can make diamond films possess various electronic conductivity and electrochemical potential windows.203,204 For example, boron-doped diamond exhibits high stability for physisorption and chemisorption.205 It should be noted that one of the reoccurring problems of current nanopores is the reproducibility of the measurements. During the translocation under an electric field, a lot of molecules stack and block the nanopores, leading to insufficient spatial/temporal resolution and “biofouling” of the sequencing devices. Meanwhile, the reuse and regeneration of conventional microfluid devices require complex processing, which may even damage the core component of fragile bilayers. Furthermore, the reactivation of diamond membranes can be easily realized by applying high currents/potentials under ambient conditions, which generates strong oxidant (OH radicals) in electrolyte solutions and mineralizes (or “cold burn”) organic substances on the diamond surface.156,203 All these advantages make diamond films/membranes extremely attractive for the formation of nanopores and for DNA sequencing technologies. To realize diamond nanopore sequencing, the growth of ultrathin diamond films/membranes and the subsequent formation of well-shaped diamond nanopores are key. Unfortunately, both issues have not been well solved to date.
Alternatively, tremendous efforts have been devoted to the development of synthesis processes for (ultra-)thin diamond membranes with controlled film thickness.206 Different from the thermal CVD growth of graphene, microwave chemical vapor deposition (MWCVD) and hot filament chemical vapor deposition (HFCVD) are widely used for the synthesis of ultrathin diamond on non-diamond substrates. During these CVD processes, gaseous reactants (e.g., methane and hydrogen in most cases) are fed into the CVD reactor. The diffusion and adsorption of activated or initiated species by a hot filament or plasma lead to the growth of diamond. This growth involves two major processing steps including the nucleation and growth of diamond. For example, diamond nanoparticles (e.g., few nanometers in diameter) act as nucleuses. Diamond deposition is controlled and optimized independently by adjusting the process parameters, such as gas composition and concentration (or flow rate), chamber pressure, growth temperature and time. For the fabrication of ultrathin diamond, a slow growth rate is favorable. Namely, ultrathin diamond films can be grown at low temperature (down to 300 °C) and a long growth time (to hours) during the CVD process. Ultra-thin diamond films feature either insulating or semiconductive properties. The thicknesses of diamond films must be comparable with other 2D materials. For DNA sequencing with high resolutions, diamond films must be as thin as a few angstroms, the same scale as the spacing between DNA bases. To obtain pure diamond membranes, the substrates need to be removed or separated by wet-chemical etching in boiled solutions (e.g., 30 wt% NaOH solution at 80 °C to remove the Si substrate) or precise laser cutting technique.207
To generate diamond nanopores, different nanotechnologies can be employed such as top-down etching method and bottom-up overgrowth approach.208 In the case of top-down etching methods, diamond films are etched by plasma (oxygen) or thermocatalytic (graphitization or burning) reaction through a porous mask, resulting in the generation of porous diamond films.209 The bottom-up growth is either guided by diamond nucleation/deposition at selective areas or achieved by direct diamond growth on a porous template (e.g., silica spheres,210,211 SiO2 nanofibers,212 carbon foam,213 and Titan foam214). The quality of the obtained diamond pores from the top-down approaches is mainly determined by the etching mask (e.g., nature, size and shape) and etching conditions (e.g., time, temperature, and pressure). Given that the pore sizes of these porous templates can range from a few nanometers to micrometers, the diamond pores generated from the bottom-up overgrowth approach are expected to have suitable pore sizes for DNA sequencing. It should be noted that the nanopore size is extremely important to achieve the translocation of molecules with the required selectivity and sensitivity. Unfortunately, the creation of diamond nanopores remains a technological challenge due to high hardness of diamond and its chemical inertness. To date, there is no setup or example available with respect to diamond nanopore sequencing.
3. Conclusions
As the fourth-generation sequencing technique, the concept of nanopore sequencing has witnessed unprecedented advances in measuring the structure of nucleotides in DNA molecules. As a label-free DNA sequencing technology, nanopore sequencing is expected to achieve long read lengths and high sequencing speeds. For this potential sequencing technology, the employed nanopore plays the key role. Compared to biological nanopores, artificially fabricated solid-state nanopores seem to be more promising. These nanopores fabricated on carbon nanoparticles shed light on the direction and bright future of DNA nanopore sequencing. The three commonly used carbon materials, namely, graphene, CNTs and diamond were summarized and discussed for their potential nanopore sequencing applications. It is known that they possess varying physical, chemical, electrical, and mechanical properties, stemming from the different hybrid states of their carbon atoms and geometric features. Among them, graphene is regarded as the best pore and membrane material. This is because graphene layers can act as both the membrane and the electrode for DNA sequencing. The interactions of DNA molecules with graphene sheets and nanopores are very complicated, depending heavily on the existing surface charges, defects, and functional groups. CNTs can provide nanopores with similar dimensions to that of DNA molecules. However, they suffer from poor uniformity, leading to uncertain sequencing performances. A free-standing diamond film exhibits excellent chemical stability, biocompatibility, and long-term stability under extremely harsh conditions. It is hard, and therefore diamond nanopores can be fabricated as required. For example, with advanced nanotechnology, the production of diamond nanopores in the range of sub-nanometers to few nanometers is expected to be possible. However, the formation of ultra-thin and large-sized diamond membranes, especially those with similar dimensions to the height of DNA bases is still impossible using currently available chemical vapor deposition methods. We expect that this review article will present readers with more ideas with respect to the selection of carbon materials for nanopore sequencing in future studies. Many new exciting discoveries of molecular biology are expected at the single-molecule scale when suitable carbon nanopores are designed, produced and employed.
Author contributions
Jing Xu: writing – original draft; Xin Jiang & Nianjun Yang: writing – review & editing.
Conflicts of interest
There are no conflicts to declare.
Acknowledgements
J. X. thanks the financial support from the National Natural Science Foundation of China (22269004), Guizhou Provincial Science and Technology Projects ZK[2022] General 122 and Guizhou University (GZUQLXK21004).
Notes and references
- E. R. Mardis, Nat. Protoc., 2017, 12, 213–218 CrossRef CAS PubMed.
- S. B. Smith, Y. J. Cui and C. Bustamante, Science, 1996, 271, 795–799 CrossRef CAS PubMed.
- M. R. Tucker, S. Piana, D. Tan, M. V. LeVine and D. E. Shaw, J. Phys. Chem. B, 2022, 126, 4442–4457 CrossRef CAS.
- A. Vologodskii and M. D. Frank-Kamenetskii, Phys. Life Rev., 2018, 25, 1–21 CrossRef.
- G. S. Baldwin, N. J. Brooks, R. E. Robson, A. Wynveen, A. Goldar, S. Leikin, J. M. Seddon and A. A. Kornyshev, J. Phys. Chem. B, 2008, 112, 1060–1064 CrossRef CAS PubMed.
- C.-L. Lai, C. Chen, S.-C. Ou, M. Prentiss and B. M. Pettitt, Phys. Rev. E, 2020, 101, 032414 CrossRef CAS PubMed.
- C. J. Houldcroft, M. A. Beale and J. Breuer, Nat. Rev. Microbiol., 2017, 15, 183–192 CrossRef CAS PubMed.
- T. Hu, N. Chitnis, D. Monos and A. Dinh, Hum. Immunol., 2021, 82, 801–811 CrossRef CAS PubMed.
- J. M. Heather and B. Chain, Genomics, 2016, 107, 1–8 CrossRef CAS PubMed.
- S. Agah, M. Zheng, M. Pasquali and A. B. Kolomeisky, J. Phys. D: Appl. Phys., 2016, 49, 413001 CrossRef.
- A. L. V. Coradini, C. B. Hull and I. M. Ehrenreich, Nat. Commun., 2020, 11, 6177 CrossRef CAS PubMed.
- J. Shendure, S. Balasubramanian, G. M. Church, W. Gilbert, J. Rogers, J. A. Schloss and R. H. Waterston, Nature, 2017, 550, 345–353 CrossRef CAS PubMed.
- J. Antonio Garrido-Cardenas, F. Garcia-Maroto, J. Antonio Alvarez-Bermejo and F. Manzano-Agugliaro, Sensors, 2017, 17, 588 CrossRef PubMed.
- J. Shendure, R. D. Mitra, C. Varma and G. M. Church, Nat. Rev. Genet., 2004, 5, 335–344 CrossRef CAS PubMed.
- L. M. Baudhuin, S. A. Lagerstedt, E. W. Klee, N. Fadra, D. Oglesbee and M. J. Ferber, J. Mol. Diagn., 2015, 17, 456–461 CrossRef CAS PubMed.
- M. G. Kluesner, D. A. Nedveck, W. S. Lahr, J. R. Garbe, J. E. Abrahante, B. R. Webbor and B. S. Moriarity, CRISPR J., 2018, 1, 239–250 CrossRef CAS PubMed.
- P. Kumaresan, C. J. Yang, S. A. Cronier, R. G. Blazej and R. A. Mathies, Anal. Chem., 2008, 80, 3522–3529 CrossRef CAS PubMed.
- D. Pushkarev, N. F. Neff and S. R. Quake, Nat. Biotechnol., 2009, 27, 847 CrossRef CAS PubMed.
- T. D. Harris, P. R. Buzby, H. Babcock, E. Beer, J. Bowers, I. Braslavsky, M. Causey, J. Colonell, J. DiMeo, J. W. Efcavitch, E. Giladi, J. Gill, J. Healy, M. Jarosz, D. Lapen, K. Moulton, S. R. Quake, K. Steinmann, E. Thayer, A. Tyurina, R. Ward, H. Weiss and Z. Xie, Science, 2008, 320, 106–109 CrossRef CAS PubMed.
- D. Branton, D. W. Deamer, A. Marziali, H. Bayley, S. A. Benner, T. Butler, M. Di Ventra, S. Garaj, A. Hibbs, X. Huang, S. B. Jovanovich, P. S. Krstic, S. Lindsay, X. S. Ling, C. H. Mastrangelo, A. Meller, J. S. Oliver, Y. V. Pershin, J. M. Ramsey, R. Riehn, G. V. Soni, V. Tabard-Cossa, M. Wanunu, M. Wiggin and J. A. Schloss, Nat. Biotechnol., 2008, 26, 1146–1153 CrossRef CAS PubMed.
- N. Yang and X. Jiang, Carbon, 2017, 115, 293–311 CrossRef CAS.
- F. Sanger, S. Nicklen and A. R. Coulson, Proc. Natl. Acad. Sci. U. S. A., 1977, 74, 5463–5467 CrossRef CAS PubMed.
- A. M. Maxam and W. Gilbert, Proc. Natl. Acad. Sci. U. S. A., 1977, 74, 560–564 CrossRef CAS PubMed.
-
B. R. Pittendrigh, J. M. Clark, S. H. Lee, W. Sun and E. Kirkness, in Advances in Human Vector Control, ed. J. M. Clark, J. R. Bloomquist and H. Kawada, 2009, vol. 1014, pp. 191–202 Search PubMed.
- J. D. Watson, Science, 1990, 248, 44–49 CrossRef CAS PubMed.
- A. Grada and K. Weinbrecht, J. Invest. Dermatol., 2013, 133, E1–E4 CrossRef CAS PubMed.
- M. C. Schatz, A. L. Delcher and S. L. Salzberg, Genome Res., 2010, 20, 1165–1173 CrossRef CAS PubMed.
- J. M. Rothberg and J. H. Leamon, Nat. Biotechnol., 2008, 26, 1117–1124 CrossRef CAS PubMed.
- M. Oepik, M. Metsis, T. J. Daniell, M. Zobel and M. Moora, New Phytol., 2009, 184, 424–437 CrossRef PubMed.
- M. K. Midha, M. Wu and K.-P. Chiu, Hum. Genet., 2019, 138, 1201–1215 CrossRef CAS PubMed.
- I. M. Berry, M. C. Melendrez, K. A. Bishop-Lilly, W. Rutvisuttinunt, S. Pollett, E. Talundzic, L. Morton and R. G. Jarman, J. Infect. Dis., 2020, 221, S292–S307 CAS.
- M. Pendleton, R. Sebra, A. W. C. Pang, A. Ummat, O. Franzen, T. Rausch, A. M. Stuetz, W. Stedman, T. Anantharaman, A. Hastie, H. Dai, M. H.-Y. Fritz, H. Cao, A. Cohainl, G. Deikusl, R. E. Durrett, S. C. Blanchard, R. Altman, C.-S. Chin, Y. Guo, E. E. Paxinos, J. O. Korbe, R. B. Darnell, W. R. McCombiemii, P.-Y. Kwok, C. E. Mason, E. E. Schadt and A. Bashirl, Nat. Methods, 2015, 12, 780–786 CrossRef CAS PubMed.
- A. Ameur, W. P. Kloosterman and M. S. Hestand, Trends Biotechnol., 2019, 37, 72–85 CrossRef CAS PubMed.
- S. Ganesh, K. Venkatakrishnan and B. Tan, Nat. Commun., 2020, 11, 1135 CrossRef CAS PubMed.
- J. Lei and H. Ju, Chem. Soc. Rev., 2012, 41, 2122–2134 RSC.
- J. Clarke, H.-C. Wu, L. Jayasinghe, A. Patel, S. Reid and H. Bayley, Nat. Nanotechnol., 2009, 4, 265–270 CrossRef CAS PubMed.
- M. J. P. Chaisson, J. Huddleston, M. Y. Dennis, P. H. Sudmant, M. Malig, F. Hormozdiari, F. Antonacci, U. Surti, R. Sandstrom, M. Boitano, J. M. Landolin, J. A. Stamatoyannopoulos, M. W. Hunkapiller, J. Korlach and E. E. Eichler, Nature, 2015, 517, 608–611 CrossRef CAS PubMed.
- P. Shrestha, D. Yang, T. E. Tomov, J. I. MacDonald, A. Ward, H. T. Bergal, E. Krieg, S. Cabi, Y. Luo, B. Nathwani, A. Johnson-Buck, W. M. Shih and W. P. Wong, Nat. Nanotechnol., 2021, 16, 1362–1367 CrossRef CAS PubMed.
- Y. H. Sun, A. Wang, C. Song, G. Shankar, R. K. Srivastava, K. F. Au and X. Z. Li, Nat. Commun., 2021, 12, 1361 CrossRef CAS PubMed.
- R. Ke, M. Mignardi, T. Hauling and M. Nilsson, Hum. Mutat., 2016, 37, 1363–1367 CrossRef CAS PubMed.
- G. Pérez-Mitta, M. E. Toimil-Molares, C. Trautmann, W. A. Marmisollé and O. Azzaroni, Adv. Mater., 2019, 31, 1901483 CrossRef PubMed.
- B. M. Floyd and E. M. Marcotte, Annu. Rev. Biophys., 2022, 51, 181–200 CrossRef PubMed.
- Y.-L. Ying, R. Gao, Y.-X. Hu and Y.-T. Long, Small Methods, 2018, 2, 1700390 CrossRef.
- D. Deamer, M. Akeson and D. Branton, Nat. Biotechnol., 2016, 34, 518–524 CrossRef CAS PubMed.
- C. Dekker, Nat. Nanotechnol., 2007, 2, 209–215 CrossRef CAS PubMed.
- B. M. Venkatesan and R. Bashir, Nat. Nanotechnol., 2011, 6, 615–624 CrossRef CAS PubMed.
- R. M. Leggett and M. D. Clark, J. Exp. Bot., 2017, 68, 5419–5429 CrossRef CAS PubMed.
- A. Pomerantz, N. Penafiel, A. Arteaga, L. Bustamante, F. Pichardo, L. A. Coloma, C. L. Barrio-Amoros, D. Salazar-Valenzuela and S. Prost, GigaScience, 2018, 7, 033 CrossRef PubMed.
- M. Jain, H. E. Olsen, B. Paten and M. Akeson, Genome Biol., 2016, 17, 1–11 CrossRef PubMed.
- J. Chmielecki and M. Meyerson, Annu. Rev. Med., 2014, 65, 63–79 CrossRef CAS PubMed.
- J. C. Gomes, A. I. Masood, L. H. Silva, J. R. da Cruz Ferreira, A. A. Freire Júnior, A. L. Rocha, L. C. de Oliveira, N. R. da Silva, B. J. Fernandes and W. P. dos Santos, Sci. Rep., 2021, 11, 11545 CrossRef CAS PubMed.
- J. Lee, H. Kim, A. Kim and H. Jung, Microporous Mesoporous Mater., 2020, 293, 109794 CrossRef CAS.
- X.-Z. Chen, Q. Li, X. Chen, X. Guo, H.-X. Ge, Y. Liu and Q.-D. Shen, Adv. Funct. Mater., 2013, 23, 3124–3129 CrossRef CAS.
- P. Kapruwan, J. Ferre-Borrull and L. F. Marsal, Adv. Mater. Interfaces, 2020, 7, 2001133 CrossRef CAS.
- T. Ma, E. Balanzat, J.-M. Janot and S. Balme, Biosens. Bioelectron., 2019, 137, 207–212 CrossRef CAS PubMed.
- N. Kostoglou, C. Koczwara, S. Stock, C. Tampaxis, G. Charalambopoulou, T. Steriotis, O. Paris, C. Rebholz and C. Mitterer, Chem. Eng. J., 2022, 427, 131730 CrossRef CAS.
- N. Yang, H. Zhuang, R. Hoffmann, W. Smirnov, J. Hees, X. Jiang and C. E. Nebel, Anal. Chem., 2011, 83, 5827–5830 CrossRef CAS PubMed.
- G. Rajeev, B. Prieto Simon, L. F. Marsal and N. H. Voelcker, Adv. Healthcare Mater., 2018, 7, 1700904 CrossRef PubMed.
- R. Gao, Y.-L. Ying, Y.-J. Li, Y.-X. Hu, R.-J. Yu, Y. Lin and Y.-T. Long, Angew. Chem., Int. Ed., 2018, 57, 1011–1015 CrossRef CAS PubMed.
- H. Koga, N. Namba, T. Takahashi, M. Nogi and Y. Nishina, ChemSusChem, 2017, 10, 2560–2565 CrossRef CAS PubMed.
- N. K. Thomas, V. C. Poodari, M. Jain, H. E. Olsen, M. Akeson and R. L. Abu-Shumays, ACS Nano, 2021, 15, 16642–16653 CrossRef CAS PubMed.
- A. D. Ewing, N. Smits, F. J. Sanchez-Luque, J. Faivre, P. M. Brennan, S. R. Richardson, S. W. Cheetham and G. J. Faulkner, Mol. Cell, 2020, 80, 915–928 CrossRef CAS PubMed.
- R. R. Wick, L. M. Judd and K. E. Holt, Genome Biol., 2019, 20, 1–10 CrossRef CAS PubMed.
- A. Payne, N. Holmes, T. Clarke, R. Munro, B. J. Debebe and M. Loose, Nat. Biotechnol., 2021, 39, 442–450 CrossRef CAS PubMed.
- E. L. Moss, D. G. Maghini and A. S. Bhatt, Nat. Biotechnol., 2020, 38, 701–707 CrossRef CAS PubMed.
- O. Begik, M. C. Lucas, L. P. Pryszcz, J. M. Ramirez, R. Medina, I. Milenkovic, S. Cruciani, H. Liu, H. G. S. Vieira, A. Sas-Chen, J. S. Mattick, S. Schwartz and E. M. Novoa, Nat. Biotechnol., 2021, 39, 1278–1291 CrossRef CAS PubMed.
- R. A. Bull, T. N. Adikari, J. M. Ferguson, J. M. Hammond, I. Stevanovski, A. G. Beukers, Z. Naing, M. Yeang, A. Verich, H. Gamaarachchi, K. W. Kim, F. Luciani, S. Stelzer-Braid, J.-S. Eden, W. D. Rawlinson, S. J. van Hal and I. W. Deveson, Nat. Commun., 2020, 11, 6272 CrossRef CAS PubMed.
- K. Shafin, T. Pesout, R. Lorig-Roach, M. Haukness, H. E. Olsen, C. Bosworth, J. Armstrong, K. Tigyi, N. Maurer, S. Koren, F. J. Sedlazeck, T. Marschall, S. Mayes, V. Costa, J. M. Zook, K. J. Liu, D. Kilburn, M. Sorensen, K. M. Munson, M. R. Vollger, J. Monlong, E. Garrison, E. E. Eichler, S. Salama, D. Haussler, R. E. Green, M. Akeson, A. Phillippy, K. H. Miga, P. Carnevali, M. Jain and B. Paten, Nat. Biotechnol., 2020, 38, 1044–1053 CrossRef CAS PubMed.
- I. Vlassiouk, S. Smirnov and Z. Siwy, Nano Lett., 2008, 8, 1978–1985 CrossRef CAS PubMed.
- J. Zhang, L. Hou, Z. Zuo, P. Ji, X. Zhang, Y. Xue and F. Zhao, Nat. Biotechnol., 2021, 39, 836–845 CrossRef CAS PubMed.
- J. Kudr, S. Skalickova, L. Nejdl, A. Moulick, B. Ruttkay–Nedecky, V. Adam and R. Kizek, Electrophoresis, 2015, 36, 2367–2379 CrossRef CAS PubMed.
- L. Restrepo-Perez, G. Huang, P. R. Bohlander, N. Worp, R. Eelkema, G. Maglia, C. Joo and C. Dekker, ACS Nano, 2019, 13, 13668–13676 CrossRef CAS PubMed.
- Y. Wang, Y. Zhang, X. Chen, X. Guan and L. Wang, Talanta, 2021, 223, 121684 CrossRef CAS PubMed.
- G. F. Schneider and C. Dekker, Nat. Biotechnol., 2012, 30, 326–328 CrossRef CAS PubMed.
- A. Crnković, M. Srnko and G. Anderluh, Life, 2021, 11, 27 CrossRef PubMed.
- D. Wendell, P. Jing, J. Geng, V. Subramaniam, T. J. Lee, C. Montemagno and P. Guo, Nat. Nanotechnol., 2009, 4, 765–772 CrossRef CAS PubMed.
- A. H. Laszlo, I. M. Derrington and J. H. Gundlach, Methods, 2016, 105, 75–89 CrossRef CAS PubMed.
- A. N. Ananth, A. Mishra, S. Frey, A. Dwarkasing, R. Versloot, E. van der Giessen, D. Görlich, P. Onck and C. Dekker, eLife, 2018, 7, 31510 CrossRef PubMed.
- G. Huang, A. Voet and G. Maglia, Nat. Commun., 2019, 10, 835 CrossRef PubMed.
- D. W. Deamer and D. Branton, Acc. Chem. Res., 2002, 35, 817–825 CrossRef CAS PubMed.
- M. Drndic, Nat. Rev. Phys., 2021, 3, 606 CrossRef.
- C. Plesa, D. Verschueren, S. Pud, J. van der Torre, J. W. Ruitenberg, M. J. Witteveen, M. P. Jonsson, A. Y. Grosberg, Y. Rabin and C. Dekker, Nat. Nanotechnol., 2016, 11, 1093–1097 CrossRef CAS PubMed.
- C. C. Chau, S. E. Radford, E. W. Hewitt and P. Actis, Nano Lett., 2020, 20, 5553–5561 CrossRef CAS PubMed.
- T. Hayashida, M. Tsutsui, S. Murayama, T. Nakada and M. Taniguchi, ACS Appl. Mater. Interfaces, 2021, 13, 10632–10638 CrossRef CAS PubMed.
- D. J. Niedzwiecki, B. DiPaolo, C.-Y. Lin, A. Castan, R. Keneipp and M. Drndic, ACS Sens., 2021, 6, 2534–2545 CrossRef CAS PubMed.
- S. Wen, T. Zeng, L. Liu, K. Zhao, Y. Zhao, X. Liu and H.-C. Wu, J. Am. Chem. Soc., 2011, 133, 18312–18317 CrossRef CAS PubMed.
- B. Luan and M. A. Kuroda, ACS Nano, 2020, 14, 13137–13145 CrossRef CAS PubMed.
- G. Danda, P. M. Das, Y.-C. Chou, J. T. Mlack, W. M. Parkin, C. H. Naylor, K. Fujisawa, T. Zhang, L. B. Fulton, M. Terrones, A. T. C. Johnson and M. Drndic, ACS Nano, 2017, 11, 1937–1945 CrossRef CAS PubMed.
- P. Yadav, Z. Cao and A. B. Farimani, ACS Nano, 2021, 15, 4861–4869 CrossRef CAS PubMed.
- A. B. Farimani, K. Min and N. R. Aluru, ACS Nano, 2014, 8, 7914–7922 CrossRef CAS PubMed.
- K. Liu, J. Feng, A. Kis and A. Radenovic, ACS Nano, 2014, 8, 2504–2511 CrossRef CAS PubMed.
- J. P. Fried, J. L. Swett, B. P. Nadappuram, J. A. Mol, J. B. Edel, A. P. Ivanov and J. R. Yates, Chem. Soc. Rev., 2021, 50, 4974–4992 RSC.
- J. D. Spitzberg, A. Zrehen, X. F. van Kooten and A. Meller, Adv. Mater., 2019, 31, 1900422 CrossRef PubMed.
- T. Gilboa, E. Zvuloni, A. Zrehen, A. H. Squires and A. Meller, Adv. Funct. Mater., 2020, 30, 1900642 CrossRef CAS.
- S. S. Sarkar, A. K. Katiyar, A. Sarkar, A. Dhar, A. Rudra, R. K. Khatri and S. K. Ray, Appl. Surf. Sci., 2018, 437, 144–151 CrossRef CAS.
- J. Kong, N. A. W. Bell and U. F. Keyser, Nano Lett., 2016, 16, 3557–3562 CrossRef CAS PubMed.
- V. S. Bhat, P. Kanagavalli, G. Sriram, R. B. Prabhu, N. S. John, M. Veerapandian, M. Kurkuri and G. Hegde, J. Energy Storage, 2020, 32, 101829 CrossRef.
- L.-H. Zhang, Y. Shi, Y. Wang and N. R. Shiju, Adv. Sci., 2020, 7, 1902126 CrossRef CAS PubMed.
- N. Yang, G. M. Swain and X. Jiang, Electroanalysis, 2016, 28, 27–34 CrossRef CAS.
- J. Gao, Y. Wang, H. Wu, X. Liu, L. Wang, Q. Yu, A. Li, H. Wang, C. Song, Z. Gao, M. Peng, M. Zhang, N. Ma, J. Wang, W. Zhou, G. Wang, Z. Yin and D. Ma, Angew. Chem., Int. Ed., 2019, 58, 15089–15097 CrossRef CAS PubMed.
- T. A. Schaub, E. A. Prantl, J. Kohn, M. Bursch, C. R. Marshall, E. J. Leonhardt, T. C. Lovell, L. N. Zakharov, C. K. Brozek, S. R. Waldvogel, S. Grimme and R. Jasti, J. Am. Chem. Soc., 2020, 142, 8763–8775 CrossRef PubMed.
- L. Liu, Z. Niu and J. Chen, Chem. Soc. Rev., 2016, 45, 4340–4363 RSC.
- S. K. Tiwari, S. Sahoo, N. Wang and A. Huczko, J. Sci.: Adv. Mater. Devices, 2020, 5, 10–29 Search PubMed.
- R. Ye and J. M. Tour, ACS Nano, 2019, 13, 10872–10878 CrossRef CAS PubMed.
- D. G. Papageorgiou, I. A. Kinloch and R. J. Young, Prog. Mater. Sci., 2017, 90, 75–127 CrossRef CAS.
- X. Yu, H. Cheng, M. Zhang, Y. Zhao, L. Qu and G. Shi, Nat. Rev. Mater., 2017, 2, 17046 CrossRef CAS.
- S. Ren, P. Rong and Q. Yu, Ceram. Int., 2018, 44, 11940–11955 CrossRef CAS.
- Y. Xu, H. Cao, Y. Xue, B. Li and W. Cai, Nanomaterials, 2018, 8, 942 CrossRef PubMed.
- M. R. Habib, T. Liang, X. Yu, X. Pi, Y. Liu and M. Xu, Rep. Prog. Phys., 2018, 81, 036501 CrossRef PubMed.
- X. Huang, J. Guan, Z. Lin, B. Liu, S. Xing, W. Wang and J. Guo, Nano Lett., 2017, 17, 4619–4623 CrossRef CAS PubMed.
- A. Cabrero-Vilatela, R. S. Weatherup, P. Braeuninger-Weimer, S. Caneva and S. Hofmann, Nanoscale, 2016, 8, 2149–2158 RSC.
- J. Plutnar, M. Pumera and Z. Sofer, J. Mater. Chem. C, 2018, 6, 6082–6101 RSC.
- B. Deng, Z. Liu and H. Peng, Adv. Mater., 2019, 31, 1800996 CrossRef PubMed.
- X. Wang, Q. Yuan, J. Li and F. Ding, Nanoscale, 2017, 9, 11584–11589 RSC.
- Y. Zhang, H. Zhang, F. Li, H. Shu, Z. Chen, Y. Sui, Y. Zhang, X. Ge, G. Yu, Z. Jin and X. Liu, Carbon, 2016, 96, 237–242 CrossRef CAS.
- B. Huet, J.-P. Raskin, D. W. Snyder and J. M. Redwing, Carbon, 2020, 163, 95–104 CrossRef CAS.
- J. Dong, L. Zhang and F. Ding, Adv. Mater., 2019, 31, 1801583 CrossRef PubMed.
- M. Huang, B. Deng, F. Dong, L. Zhang, Z. Zhang and P. Chen, Small Methods, 2021, 5, 2001213 CrossRef CAS PubMed.
- S. Chaitoglou and E. Bertran, J. Mater. Sci., 2017, 52, 8348–8356 CrossRef CAS.
- M. Huang and R. S. Ruoff, Acc. Chem. Res., 2020, 53, 800–811 CrossRef CAS PubMed.
- B. Huet and J.-P. Raskin, Carbon, 2018, 129, 270–280 CrossRef CAS.
- J. Kraus, M. Boebel and S. Guenther, Carbon, 2016, 96, 153–165 CrossRef CAS.
- P. Braeuninger-Weimer, B. Brennan, A. J. Pollard and S. Hofmann, Chem. Mater., 2016, 28, 8905–8915 CrossRef CAS PubMed.
- X. Wu, M. Fengwen and H. Zhao, Proc. Nat. Res. Soc., 2018, 2, 02003 CrossRef.
- C. A. Merchant, K. Healy, M. Wanunu, V. Ray, N. Peterman, J. Bartel, M. D. Fischbein, K. Venta, Z. Luo, A. T. C. Johnson and M. Drndic, Nano Lett., 2010, 10, 2915–2921 CrossRef CAS PubMed.
- M. D. Fischbein and M. Drndić, Appl. Phys. Lett., 2008, 93, 113107 CrossRef.
- B. T. Schaefer, L. Wang, A. Jarjour, K. Watanabe, T. Taniguchi, P. L. McEuen and K. C. Nowack, Nat. Commun., 2020, 11, 4163 CrossRef CAS PubMed.
- P. K. Kannan, S. A. Moshkalev and C. S. Rout, RSC Adv., 2016, 6, 11329–11334 RSC.
- X. T. Zheng, A. Ananthanarayanan, K. Q. Luo and P. Chen, Small, 2015, 11, 1620–1636 CrossRef CAS PubMed.
- T. Terse-Thakoor, P. Ramnani, C. Villarreal, D. Yan, T. Thien-Toan, P. Tung and A. Mulchandani, Biosens. Bioelectron., 2019, 126, 838–844 CrossRef CAS PubMed.
- T. Xu, X. Xie and L. Sun, IEEE Int. Conf. Nano/Micro Eng. Mol. Syst., 8th, 2013, 637–640 CAS.
- Z.-Y. Zhang, H.-L. Cui, D.-P. Huang and D.-Q. Wang, Sens. Actuators, B, 2021, 349, 130792 CrossRef CAS.
- D. Wang, R. Dai, X. Zhang, L. Liu, H. Zhuang, Y. Lu, Y. Wang, Y. Liao and Q. Nian, Carbon, 2020, 161, 880–891 CrossRef CAS.
- K.-P. Schlichting and D. Poulikakos, ACS Appl. Mater. Interfaces, 2020, 12, 36468–36477 CrossRef CAS PubMed.
- L. Madauss, J. Schumacher, M. Ghosh, O. Ochedowski, J. Meyer, H. Lebius, B. Ban-d’Etat, M. E. Toimil-Molares, C. Trautmann, R. G. H. Lammertink, M. Ulbricht and M. Schleberger, Nanoscale, 2017, 9, 10487–10493 RSC.
- H. Yao, J. Zeng, P. Zhai, Z. Loa, Y. Cheng, J. Liu, D. Mo, J. Duan, L. Wang, Y. Sun and J. Liu, ACS Appl. Mater. Interfaces, 2017, 9, 11000–11008 CrossRef CAS PubMed.
- A. Guirguis, J. W. Maina, L. Kong, L. C. Henderson, A. Rana, L. H. Li, M. Majumder and L. F. Dumee, Carbon, 2019, 155, 660–673 CrossRef CAS.
- H. Qi, Z. Zhang, Z. Li, H. Nan, K. Bi and Y. Chen, J. Phys. Chem. C, 2021, 125, 507–514 CrossRef CAS.
- R. Tarcan, O. Todor-Boer, I. Petrovai, C. Leordean, S. Astilean and I. Botiz, J. Mater. Chem. C, 2020, 8, 1198–1224 RSC.
- A. T. Dideikin and A. Y. Vul, Front. Phys., 2019, 6, 149 CrossRef.
- S. Priyadarsini, S. Mohanty, S. Mukherjee, S. Basu and M. Mishra, J. Nanostruct. Chem., 2018, 8, 123–137 CrossRef CAS.
- Z. P. Smith and B. D. Freeman, Angew. Chem., Int. Ed., 2014, 53, 10286–10288 CrossRef CAS PubMed.
- Q. Yang, Y. Su, C. Chi, C. T. Cherian, K. Huang, V. G. Kravets, F. C. Wang, J. C. Zhang, A. Pratt, A. N. Grigorenko, F. Guinea, A. K. Geim and R. R. Nair, Nat. Mater., 2017, 16, 1198–1202 CrossRef CAS PubMed.
- Y. Hou, Z. Sheng, C. Fu, J. Kong and X. Zhang, Nat. Commun., 2022, 13, 1227 CrossRef CAS PubMed.
- M. R. Ajayakumar, M. Di Giovannantonio, C. A. Pignedoli, L. Yang, P. Ruffieux, J. Ma, R. Fasel and X. Feng, J. Polym. Sci., 2022, 60, 1912–1917 CrossRef CAS.
- C. Moreno, M. Vilas-Varela, B. Kretz, A. Garcia-Lekue, M. V. Costache, M. Paradinas, M. Panighel, G. Ceballos, S. O. Valenzuela, D. Pena and A. Mugarza, Science, 2018, 360, 199–203 CrossRef CAS PubMed.
- P. H. Jacobse, R. D. McCurdy, J. Jiang, D. J. Rizzo, G. Veber, P. Butler, R. Zuzak, S. G. Louie, F. R. Fischer and M. F. Crommie, J. Am. Chem. Soc., 2020, 142, 13507–13514 CrossRef CAS PubMed.
- M. Shekhirev, P. Zahl and A. Sinitskii, ACS Nano, 2018, 12, 8662–8669 CrossRef CAS PubMed.
- R. Pawlak, X. Liu, S. Ninova, P. D'Astolfo, C. Drechsel, S. Sangtarash, R. Haner, S. Decurtins, H. Sadeghi, C.
J. Lambert, U. Aschauer, S.-X. Liu and E. Meyer, J. Am. Chem. Soc., 2020, 142, 12568–12573 CrossRef CAS PubMed.
- S. J. Heerema and C. Dekker, Nat. Nanotechnol., 2016, 11, 127–136 CrossRef CAS PubMed.
- M. Shankla and A. Aksimentiev, Nat. Nanotechnol., 2019, 14, 858 CrossRef CAS PubMed.
- W. Si, Y. Zhang, G. Wu, Y. Kan, Y. Zhang, J. Sha and Y. Chen, Small, 2019, 15, 1900036 CrossRef PubMed.
- A. B. Farimani, P. Dibaeinia and N. R. Aluru, ACS Appl. Mater. Interfaces, 2017, 9, 92–100 CrossRef PubMed.
- S. J. Heerema, L. Vicarelli, S. Pud, R. N. Schouten, H. W. Zandbergen and C. Dekker, ACS Nano, 2018, 12, 2623–2633 CrossRef CAS PubMed.
- M. Kulkarni and A. Mukherjee, RSC Adv., 2016, 6, 46019–46029 RSC.
- S. Yu, J. Xu, H. Kato, N. Yang, A. Schulte, H. Schoenherr and X. Jiang, ChemElectroChem, 2019, 6, 1088–1093 CrossRef CAS.
- J. Wilson, L. Sloman, Z. He and A. Aksimentiev, Adv. Funct. Mater., 2016, 26, 4830–4838 CrossRef CAS PubMed.
- R. Zan, Q. M. Ramasse, U. Bangert and K. S. Novoselov, Nano Lett., 2012, 12, 3936–3940 CrossRef CAS PubMed.
- Y. Zhou and H. Wang, ACS Omega, 2022, 7, 16422–16429 CrossRef CAS PubMed.
- E. Paulechka, T. A. Wassenaar, K. Kroenlein, A. Kazakov and A. Smolyanitsky, Nanoscale, 2016, 8, 1861–1867 RSC.
- C. Sun and B. Bai, Sci. Bull., 2017, 62, 554–562 CrossRef CAS PubMed.
- Z. Wang, T.-Y. Lv, Z.-B. Shi, S.-S. Yang and Z.-Y. Gu, Dalton Trans., 2021, 50, 13608–13619 RSC.
- S. Zhang, J. Cheng, W. Shi, K.-B. Li, D.-M. Han and J.-J. Xu, Anal. Chem., 2020, 92, 5952–5959 CrossRef CAS PubMed.
- L. Zhang and X. Wang, Nanomaterials, 2016, 6, 111 CrossRef PubMed.
- T. Civitarese and G. Zollo, ACS Appl. Nano Mater., 2021, 4, 363–371 CrossRef CAS.
- A. E. Rossini, F. Gala, M. Chinappi and G. Zollo, Nanoscale, 2018, 10, 5928–5937 RSC.
- J. Sponer and P. Hobza, Int. J. Quantum Chem., 1996, 57, 959–970 CrossRef CAS.
- C. Huang, X. Zhu, N. Li, X. Ma, Z. Li and J. Fan, J. Phys. Chem. Lett., 2021, 12, 793–799 CrossRef CAS PubMed.
- M. Belkin and A. Aksimentiev, ACS Appl. Mater. Interfaces, 2016, 8, 12599–12608 CrossRef CAS PubMed.
- A. Nicolai, A. Rath, P. Delarue and P. Senet, Nanoscale, 2020, 12, 22743–22753 RSC.
- Y. Liu, Y. Deng, Y. Yang, Y. Qu, C. Zhang, Y.-Q. Li, M. Zhao and W. Li, Nanoscale Adv., 2021, 3, 5941–5947 RSC.
- L. Zhou, K. Li, Z. Li, P. He, K. Lin, J. Mo and J. Ma, J. Vac. Sci. Technol., B: Nanotechnol. Microelectron.: Mater., Process., Meas., Phenom., 2019, 37, 061809 Search PubMed.
- X. Zhang, P. M. G. van Deursen, W. Fu and G. F. Schneider, ACS Sens., 2020, 5, 2317–2325 CrossRef CAS PubMed.
- A. Barati Farimani, P. Dibaeinia and N. R. Aluru, ACS Appl. Mater. Interfaces, 2017, 9, 92–100 CrossRef CAS PubMed.
- Y. Deng, Q. Huang, Y. Zhao, D. Zhou, C. Ying and D. Wang, Nanotechnology, 2017, 28, 045302 CrossRef PubMed.
- A. Sarathy, H. Qiu and J.-P. Leburton, J. Phys. Chem. B, 2017, 121, 3757–3763 CrossRef CAS PubMed.
- F. A. L. de Souza, G. Sivaraman, M. Fyta, R. H. Scheicher, W. L. Scopel and R. G. Amorim, Nanoscale, 2020, 12, 18289–18295 RSC.
- R. Balasubramanian, S. Pal, A. Rao, A. Naik, B. Chakraborty, P. K. Maiti and M. M. Varma, ACS Appl. Bio Mater., 2021, 4, 451–461 CrossRef CAS PubMed.
- M. B. Henry, M. Tumbapo and B. O. Tayo, AIP Adv., 2021, 11, 035324 CrossRef CAS.
- R. L. Kumawat and B. Pathak, ACS Appl. Electron. Mater., 2021, 3, 3835–3845 CrossRef CAS.
- M. Sianipar, S. H. Kim, F. Iskandar and I. G. Wenten, RSC Adv., 2017, 7, 51175–51198 RSC.
- V. D. Punetha, S. Rana, H. J. Yoo, A. Chaurasia, J. T. McLeskey, Jr., M. S. Ramasamy, N. G. Sahoo and J. W. Cho, Prog. Polym. Sci., 2017, 67, 1–47 CrossRef CAS.
- K. Gnanasekaran, T. Heijmans, S. van Bennekom, H. Woldhuis, S. Wijnia, G. de With and H. Friedrich, Appl. Mater. Today, 2017, 9, 21–28 CrossRef.
- B. K. Choi, G. H. Yoon and S. Lee, Composites, Part B, 2016, 91, 119–125 CrossRef CAS.
- V. Negri, J. Pacheco-Torres, D. Calle and P. Lopez-Larrubia, Top. Curr. Chem., 2020, 378, 15 CrossRef CAS PubMed.
- I. A. Kinloch, J. Suhr, J. Lou, R. J. Young and P. M. Ajayan, Science, 2018, 362, 547–553 CrossRef CAS PubMed.
- A. Ben Belgacem, I. Hinkov, S. Ben Yahia, O. Brinza and S. Farhat, Mater. Today Commun., 2016, 8, 183–195 CrossRef CAS.
- M. Kim, S. Osone, T. Kim, H. Higashi and T. Seto, KONA Powder Part. J., 2017, 34, 80–90 CrossRef CAS.
- L. M. Esteves, H. A. Oliveira and F. B. Passos, J. Ind. Eng. Chem., 2018, 65, 1–12 CrossRef CAS.
- Y. M. Manawi, S. Ihsanullah, A. Samara, T. Al-Ansari and M. A. Atieh, Materials, 2018, 11, 822 CrossRef PubMed.
- Y. Yan, J. Miao, Z. Yang, F.-X. Xiao, H. B. Yang, B. Liu and Y. Yang, Chem. Soc. Rev., 2015, 44, 3295–3346 RSC.
-
Y. Ma, N. Yang and X. Jiang, in Carbon Nanoparticles and Nanostructures, ed. N. Yang, X. Jiang and D.-W. Pang, Springer International Publishing, Cham, 2016, pp. 239–256 Search PubMed.
- M. D. Ellison, S. Menges, L. Nebel, G. D'Arcangelo, A. Kramer, L. Drahushuk, J. Benck, S. Shimizu and M. S. Strano, J. Phys. Chem. C, 2017, 121, 2005–2013 CrossRef CAS.
- R. Peng, X. S. Tang and D. Li, Small, 2018, 14, 1800013 CrossRef PubMed.
- S. Agah, M. Zheng, M. Pasquali and A. B. Kolomeisky, J. Phys. D: Appl. Phys., 2016, 49, 413001 CrossRef.
- R. L. Kumawat and B. Pathak, Nanoscale Adv., 2020, 2, 4041–4050 RSC.
- S. W. Jung, H. S. Kim, A. E. Cho and Y.-H. Kim, ACS Appl. Mater. Interfaces, 2018, 10, 18227–18236 CrossRef CAS PubMed.
- L. Gasparyan, I. Mazo, V. Simonyan and F. Gasparyan, Open J. Biophys., 2019, 9, 29 Search PubMed.
- A. H. Piracha, K. Ganesan, D. W. M. Lau, A. Stacey, L. P. McGuinness, S. Tomljenovic-Hanic and S. Prawer, Nanoscale, 2016, 8, 6860–6865 RSC.
- J. Xu, X. Zhang and F. Zhu, Int. J. Mech. Sci., 2022, 234, 107686 CrossRef.
- J.-F. Wang, F.-F. Yan, Q. Li, Z.-H. Liu, H. Liu, G.-P. Guo, L.-P. Guo, X. Zhou, J.-M. Cui, J. Wang, Z.-Q. Zhou, X.-Y. Xu, J.-S. Xu, C.-F. Li and G.-C. Guo, Phys. Rev. Lett., 2020, 124, 223601 CrossRef CAS PubMed.
- J. Xu, N. Yang, S. Heuser, S. Yu, A. Schulte, H. Schoenherr and X. Jiang, Adv. Energy Mater., 2019, 9, 1803623 CrossRef.
-
S. J. Cobb, Z. J. Ayres and J. V. Macpherson, in Annual Review of Analytical Chemistry, ed. P. W. Bohn and J. E. Pemberton, 2018, vol. 11, pp. 463–484 Search PubMed.
- N. Yang, S. Yu, J. V. Macpherson, Y. Einaga, H. Zhao, G. Zhao, G. M. Swain and X. Jiang, Chem. Soc. Rev., 2019, 48, 157–204 RSC.
- V. Mortet, Z. V. Zivcova, A. Taylor, O. Frank, P. Hubik, D. Tremouilles, F. Jomard, J. Barjon and L. Kavan, Carbon, 2017, 115, 279–284 CrossRef CAS.
- S. Handschuh-Wang, T. Wang and Y. Tang, Small, 2021, 17, 2007529 CrossRef CAS PubMed.
- Z. Jian, J. Xu, N. Yang, S. Han and X. Jiang, Curr. Opin. Electrochem., 2021, 30, 100835 CrossRef CAS.
- M. Varga, Š. Potocký, M. Domonkos, T. Ižák, O. Babčenko and A. Kromka, ACS Omega, 2019, 4, 8441–8450 CrossRef CAS PubMed.
- S. Yu, N. Yang, H. Zhuang, S. Mandal, O. A. Williams, B. Yang, N. Huang and X. Jiang, J. Mater. Chem. A, 2017, 5, 1778–1785 RSC.
- F. Gao, M. T. Wolfer and C. E. Nebel, Carbon, 2014, 80, 833–840 CrossRef CAS.
- H. Kato, J. Hees, R. Hoffmann, M. Wolfer, N. Yang, S. Yamasaki and C. E. Nebel, Electrochem. Commun., 2013, 33, 88–91 CrossRef CAS.
- S. Baluchová, A. Taylor, V. Mortet, S. Sedláková, L. Klimša, J. Kopeček, O. Hák and K. Schwarzová-Pecková, Electrochim. Acta, 2019, 327, 135025 CrossRef.
- M. Marton, M. Vojs, M. Kotlár, P. Michniak, Ľ. Vančo, M. Veselý and R. Redhammer, Appl. Surf. Sci., 2014, 312, 139–144 CrossRef CAS.
- W. Yang, Z. Deng, Y. Wang, L. Ma, K. Zhou, L. Liu and Q. Wei, Sep. Purif. Technol., 2022, 293, 121100 CrossRef CAS.
|
This journal is © The Royal Society of Chemistry 2023 |