DOI:
10.1039/D0RA06445A
(Review Article)
RSC Adv., 2020,
10, 35257-35264
Diagnostic technologies for COVID-19: a review
Received
24th July 2020
, Accepted 12th September 2020
First published on 23rd September 2020
Abstract
Since the outbreak of COVID-19 in December 2019, the highly contagious SARS-CoV-2 virus has spread rapidly worldwide. Although the governments across the world have adopted different preventative measures, the spread of the virus still cannot be effectively controlled, and the number of infections and deaths continues to grow. Early diagnosis of COVID-19 is one of the key measures to control the spread of the pandemic and timely treatment of infected people. This review summarizes current COVID-19 diagnostic techniques based on virology, serology, and imaging diagnostics and discusses their advantages and limitations with the aim of providing a reference for rapid and accurate diagnosis of COVID-19.
1. Introduction
Multiple cases of unexplained viral pneumonia were reported in December, 2019 in Wuhan, China. Patients presented fever, cough, fatigue, shortness of breath, and other symptoms.1 On January 11, 2020, researchers sequenced the genome of the virus causing these unexplained cases of viral pneumonia and confirmed it was a novel coronavirus.2 Further research found that this coronavirus shared 79.5%, 50% and 96% of its genome with severe acute respiratory syndrome virus (SARS-CoV), middle east respiratory syndrome virus (MERS-CoV) and TG13 (a bat coronavirus), respectively.3 On February 11, 2020, the International Virus Taxonomy Committee (IVTC) formally named the new coronavirus severe acute respiratory syndrome coronavirus 2 (SARS-CoV-2) and the World Health Organization (WHO) named the disease 2019 coronavirus disease (COVID-19). The SARS-CoV-2 invades human cells by its spike protein binding to the cell membrane protein receptor (angiotensin-converting enzyme 2, ACE2).3 The receptor-binding domain (RBD) of SARS-CoV-2 spike protein shows 10–20 times higher affinity to the ACE2 receptor compared with that of SARS-CoV,4 which demonstrated the stronger infectivity of SARS-CoV-2. In a short time frame, what started as a localized outbreak of COVID-19 in December, quickly evolved into a global pandemic, as declared by the WHO on March 11, 2020. Although governments across the globe have implemented a range of prevention and containment measures, the spread of COVID-19 continues to increase. As of July 15, 2020, there have been more than 13.5 million confirmed cases of COVID-19 worldwide and more than 580
000 deaths (https://coronavirus.jhu.edu/map.html).
Since hydroxychloroquine and remdesivir have been found to be ineffective in the treatment of COVID-19,5,6 there remains no miracle drug treatment for COVID-19. Furthermore, vaccine development is a complicated process characterized by significant uncertainty and will require testing on safety and effectiveness, before large scale production. Given this, early diagnosis and timely treatment of COVID-19 is key to control the further spread of the pandemic. This review aims to provide references to develop appropriate diagnostic strategies for COVID-19 by summarizing the most recent literature on laboratory diagnostic technologies for COVID-19.
2. Isolation, culture and identification of SARS-CoV-2
Isolation of viral pathogens in cell culture is the gold standard for the diagnosis of viral infections. Although it requires a long cultivation period, specialized equipment, and good experimental skills, it is still an effective method for identifying emerging viruses. Adriana et al., reported that a 7 week-old baby was diagnosed with SARS-CoV-2 infection before the COVID-19 outbreak in Italy, through cell-culture isolation, transmission electron microscopy, and real-time reverse-transcription polymerase chain reaction (RT-PCR) identification.7 In addition, live virus propagation allows for large amounts of virus to be developed and used for further research, such as antiviral drug testing, vaccine development, and comparative genomic analyses of viruses isolated from different regions. Finally, rapid and coordinated sharing of research findings across scientific communities and public health agencies is critical for the effective study of viruses.
3. Virology-based detection methods
SARS-CoV-2 is a novel, enveloped, positive-sense RNA virus, with a single-stranded RNA genome of ∼30 kilobases and four structural proteins (Fig. 1), belonging to genus β, of the coronaviridae family.2,3 Researcher have confirmed that the SARS-CoV-2 enters into human cells by spike protein binding to ACE2 receptors on the surface of human cells. Human ACE2 receptors are present in arterial and venous endothelial cells and arterial smooth muscle cells in almost all organs, and human ACE2 mRNA is highly expressed in renal, cardiovascular, and gastrointestinal tissues.8 The SARS-CoV-2 virus has been isolated from blood samples, sputum, cerebrospinal fluid, urine, nasal swab, tracheal aspirates, throat swabs, anal swabs, and bronchoalveolar lavage fluid.9,10 Pan et al., found that sputum samples and nasal swabs contain the highest viral load, with a median of 105 copies per mL, providing a target for the detection of SARS-CoV-2.11
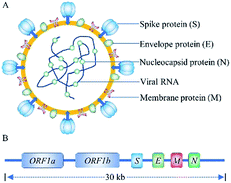 |
| Fig. 1 The structure (A) and genome (B) schematic of SARS-CoV-2. | |
3.1 Nucleic acid-based detection methods
As of July, 15, 2020, of total of 66
000 viral genomic sequences of SARS-CoV-2 had been shared to Global Influenza Data Initiative (GISAID). This genetic information laid the foundation for the development of primers and probes for specific detection of SARS-CoV-2. Rangan et al., analyzed the genome sequences of SARS-CoV-2 and SARS-associated viruses and found 59 conserved regions, which were primarily located in the RNA-dependent RNA polymerase gene, envelope protein gene, and nucleocapsid protein gene.12,13 These studies provide a reference to establish nucleic acid-based diagnostic methods. A number of SARS-CoV-2 nucleic acid-based detection methods from different research groups are listed in Table 1.
Table 1 Examples of SARS-CoV-2 nucleic acid-based detection methods
Method |
Sample source |
Target gene |
Sample tested |
Sensitivity |
Test time |
Reference |
RT-PCR |
BLF, nasal and pharyngeal swabs |
ORF1ab and N |
4880 |
— |
— |
14 |
RT-PCR |
Throat and nose swabs |
E and RdRP |
297 |
5.2 and 3.8 copies per test |
— |
13 |
Digital PCR |
Sputum, blood, urine nasal and throat swabs |
ORF1ab and N |
323 |
11.1 copies per test |
>2 h |
15 |
Digital PCR |
Pharyngeal swabs, stool and blood |
ORF1ab and N |
109 |
28.3 and 35.2 copies per μL |
— |
16 |
RT-LAMP |
Swabs and BLF |
ORF1ab and S |
130 |
20 and 200 copies per test |
26.28 ± 4.48 min |
17 |
RT-LAMP |
Throat swabs |
ORF1ab, N and S |
16 |
80 copies per mL |
30 min |
18 |
DETECTR |
Nasal and pharyngeal swabs |
E gene and N |
78 |
10 copies per μL |
45 min |
19 |
SHERLOCK |
Nasal swabs |
N |
17 |
100 copies per test |
40 or 70 min |
20 |
Gene sequencing |
Throat swabs |
ORF1ab and N |
61 |
10 copies per mL |
6–10 h |
21 |
3.1.1 Real-time reverse-transcription polymerase chain reaction. Currently, RT-PCR is the most common method for diagnosing COVID-19 due to its high sensitivity, strong specificity, and high penetration rate. The principle of RT-PCR is shown in Fig. 2. Briefly, SARS-CoV-2 genomic RNA was converted into complementary DNA (cDNA) by reverse transcriptase. The DNA was amplified by DNA polymerase using the cDNA template and the PCR reaction was monitored in real time by fluorescent dye or TaqMan DNA probe. RT-PCR detection of SARS-CoV-2 includes the steps of specimen collection, RNA extraction, PCR, and result analysis. Yang et al., used commercial RT-PCR kits recommended by China Food and Drug Administration (CFDA) to analyze 866 samples collected from the respiratory tracts of patients including nasal swabs, throat swabs, sputum and BALF. Sputum samples showed the highest positive rate in both severe (88.9%) and mild (82.2%) cases, followed by nasal swabs (73.3%, 72.1%) and throat swabs (60.0%, 61.3%). These results provide a reference for the selection of SARS-CoV-2 sample collection sites.22
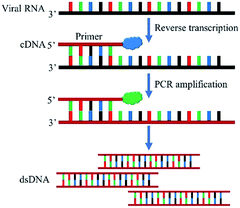 |
| Fig. 2 Schematic diagram of RT-PCR detection of SARS-CoV-2. | |
False negative results caused by improper sampling or patient viral load is a major limitation of RT-PCR detection of SARS-CoV-2. Feng reported a case where a 34 year-old man finally positive result after five RT-PCR tests.23 In order to improve the accuracy of the RT-PCR method, Chan et al., developed and evaluated three novel RT-PCR assays that target different gene regions of the SARS-CoV-2 genome. They found that the novel COVID-19-RdRp/Hel assay was highly sensitive and specific for the detection of SARS-CoV-2 RNA in vitro and in clinical specimens, with the lowest limit of detection being 11.2 RNA copies per reaction in vitro.24
Low detection throughput is another major limitation of RT-PCR detection of SARS-CoV-2.25 Given this, there is a focus on developing high-throughput RT-PCR detection platforms by diagnostic companies. Liu reported a high-throughput nucleic acid detection mode (Huo-Yan Laboratory platform), which uses an automated process and rigorous quality control strategies to ensure the stability and reliability of detection in large-scale specimens. The automated nucleic acid extraction based on the MGISP-960 sample preparation system, is comparable to manual extraction, but requires less time and fewer resources. As of July 15, 2020, total expected daily reached a maximum testing capacity of 325
600 per day (https://huoyan.bgi.com).26
3.1.2 Digital PCR. At present, RT-PCR is widely used as the gold standard for the diagnosis of COVID-19. However, the large number of reports of false negatives, is a significant limitation. In addition to inadequate sampling or improper handling of samples, low viral load in infected patients can also lead to false negative results.27 For more accurate COVID-19 diagnosis, a more sensitive RNA detection method is needed to address the limitations of RT-PCR. Digital PCR employs sample dilution, end-point PCR, and Poisson statistics to achieve an absolute quantitation of nucleic acids. Suo et al. explored the feasibility of droplet digital PCR (ddPCR) to detect SARS-CoV-2 from 57 clinical pharyngeal swab samples and compared the sensitivity and accuracy with RT-PCR. Under optimal conditions, the limit of detection (LOD) of ddPCR is 0.109 copies per μL and 0.021 copies per μL for ORF1ab and N primers/probe sets, respectively. This is approximately 500 times lower than that of the RT-PCR. Further, 64% (9/14) of patients that tested negative in two consecutive RT-PCR tests, were found to be positive when using digital PCR, demonstrating the superiority of ddPCR for clinical detection of SARS-CoV-2 and reduce the false negatives.28
3.1.3 Reverse transcription loop-mediated isothermal amplification. Reverse transcription loop-mediated isothermal amplification (RT-LAMP) is another promising alternative technology for the rapid detection of SARS-CoV-2. The RT-LAMP utilizes four to six specially designed primers and a Bst DNA polymerase with chain displacement activity under a constant temperature (60–65 °C) to synthesize the strand displacement DNA. The amplified product is continuously extended, circularized, and re-extended and the DNA with different stem-loop structures are finally produced. The amplification reaction is monitored by measuring turbidity or fluorescence. Compared with RT-PCR, the RT-LAMP has the advantages of simple operation, short reaction time, no need for special instruments, and high sensitivity, which is particularly suitable for rapid on-site detection.29,30 Yan et al., developed a RT-LAMP assay for the detection of SARS-CoV-2 in clinical samples and designed five sets of primers that target the orf1ab gene and the spike gene for optimization of this assay. The detection of 130 clinical samples by RT-LAMP showed that the sensitivity and specificity of this method were 100%, and the detection time was 26.28 ± 4.48 min, demonstrating the RT-LAMP assay is a powerful tool for the detection of SARS-CoV-2.17
3.1.4 CRISPR-based assays. Clustered Regularly Interspaced Short Palindromic Repeats (CRISPR) and CRISPR-associated (Cas) proteins (CRISPR/Cas), provide adaptive immunity against virus invasion in bacteria and archaea. In 2012, Doudna et al., demonstrated that the CRISPR-associated protein Cas9 and crRNA ribonucleoprotein complex can cleave specific sites of target DNA sequences.31 Since this discovery, the CRISPR/Cas system has become the most popular gene editing tool. In recent years, research has found that some class II Cas proteins have collateral cleavage activity and subsequently used them in the rapid detection of nucleic acids.32,33Recently, CRISPR-based nucleic acid detection methods have shown significant potential in the development of next-generation molecular diagnostic technology given its high specificity, sensitivity, simplicity, and reliability, and has been applied to the diagnosis of COVID-19. Broughton et al., developed a rapid (<40 min), easy-to-implement, CRISPR–Cas12-based lateral flow assay for the detection of SARS-CoV-2 from respiratory swab RNA extracts (Fig. 3). The proposed CRISPR-based DETECTR assay showed 95% positive predictive agreement and 100% negative predictive agreement in 36 patients with COVID-19 infection and 42 patients with other viral respiratory infections.19
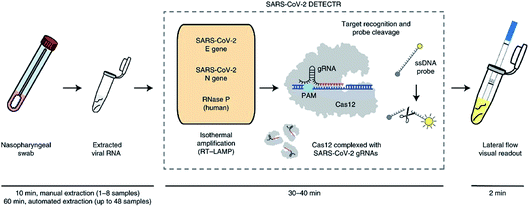 |
| Fig. 3 Schematic of CRISPR–Cas12-based assay workflow for detection of SARS-CoV-2.19 | |
3.1.5 Gene sequencing. For unexplained infectious diseases, gene sequencing is the best way to identify pathogenic microorganisms. The first genomic sequence of SARS-CoV-2 was completed by metagenomic RNA sequencing and shared with GenBank (MN908947) and GISAID on January 2020.2 For the detection of known viruses, targeted sequencing is a popular method due to its accuracy, comprehensiveness, and sensitivity. Wang et al., developed a nanopore targeted sequencing (NTS) method, combining the advantages of target amplification and long-read, real-time nanopore sequencing, to be used for diagnosing COVID-19 and simultaneously detecting other common respiratory viruses and mutated nucleic acid sequences, within 6–10 h. Parallel testing of 61 nucleic acid samples from suspected COVID-19 cases with commercial RT-PCR kits and the proposed NTS method, showed the NTS method was more sensitive for SARS-CoV-2 detection and identified more positive cases.21 Although the accuracy of gene sequencing to detect SARS-CoV-2 is high, there are limitations in its clinical application due to testing duration, high cost, and complex equipment. Finally, with the ongoing spread of COVID-19, genome sequencing has shifted from initial virus identification to investigation of the origin and evolution of the SARS-CoV-2 virus.34–36
3.2 Protein-based detection methods
In addition to nucleic acid, the viral structural proteins (spike protein, nucleocapsid protein, envelope protein, and membrane protein) and virus particles can also be used as the target molecule to diagnose COVID-19. These diagnostic methods include instrument-based methods, such as mass spectrometry and rapid detection methods based on antigen–antibody recognition, such as chemiluminescence immunoassay, lateral flow immunoassay, and biosensors.
3.2.1 Mass spectrometry. Mass spectrometry-based detection of proteins can be performed with either a targeted strategy or without any a priori, making it useful for identifying novel viruses.37 Similar to targeted sequencing, for known viruses, the targeted proteomics methods using mass spectrometry also showed potential in the diagnosis of COVID-19. Bezstarosti evaluated the detection limit by parallel reaction monitoring on an Orbitrap Eclipse mass spectrometer for structural proteins of SARS-CoV-2 in samples of virus-infected Vero cells. Nucleocapsid protein was found to be the most abundant viral protein with a limit of detection of 0.9 × 10−12 g, corresponding to about 10
000 SARS-CoV-2 virus particles.38 Further, the targeted proteomics and metabolomics assay of patients through mass spectrometry can be used for illness evaluation. Shen et al., performed a systematic proteomic and metabolomic investigation of serum samples from 46 COVID-19 patient groups and 53 control groups based on mass spectrometry and machine learning. In total, 22 proteins and 7 metabolites characteristic of critical patients were screened out, demonstrating the potential of this method in identifying potentially severe COVID-19 cases. This work may provide useful information in the allocation of medical resources.39
3.2.2 Biosensor. Biosensor diagnosis COVID-19 relies on converting the antigen–antibody binding reaction into a measurable signal through optical, enzymatic, gravimetric, electrical, or other methods to detect SARS-CoV-2. Seo et al., developed a field-effect transistor-based biosensor to detect SARS-CoV-2 in clinical samples (Fig. 4). In this device, graphene is functionalized with the SARS-CoV-2 spike antibody for use as a SARS-CoV-2 virus detection platform. The device has a limit of detection of 1.6 × 101 pfu mL−1 in culture medium and 2.42 × 102 copies per mL in clinical samples, respectively, demonstrating its potential for clinical application.40 Unfortunately, the development of traditional antibodies requires complicated processes including animal immunization and cell screening, which make it difficult and time-consuming to obtain suitable antibodies. As an alternative, some novel recognition elements for SARS-CoV-2 virus, such as aptamer41,42 and nanobody43 have been developed and applied in the diagnosis of COVID-19.
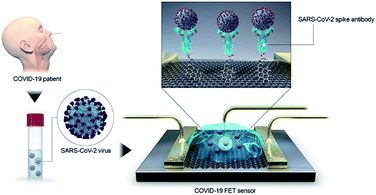 |
| Fig. 4 Schematic diagram of COVID-19 FET sensor operation procedure.40 | |
The recent emerging microfluidic biosensors have the advantages of portability, simplicity and low reagent/sample consumption, which have attracted the attention of many researchers. Lin et al., developed a portable microfluidic immunoassay system for highly sensitive detection of IgG/IgM/antigen of SARS-CoV-2 simultaneously within 15 min. This method was successfully applied for detecting SARS-CoV-2 antigen in pharyngeal swabs from 26 patients with COVID-19 infection and 28 uninfected people and demonstrated high sensitivity and specificity, showing great promise for applications in COVID-19 pandemic monitoring and control.44
4. Serology-based detection methods
Although nucleic acid-based detection methods have been widely used in the diagnosis of COVID-19, the serology-based diagnosis methods are also widely widespread attention due to it not only can be used with molecular diagnostic technology to improve the detection accuracy and exclude false negative result.45–47 It can also collect information on SARS-CoV-2 infection in the general population, contributing to epidemiological investigations of COVID-19.48−50 Some current reported COVID-19 serology-based diagnosis methods are listed in Table 2.
Table 2 Examples of COVID-19 serology-based diagnosis methods
Method |
Sample source |
Detected target |
Sample tested |
Test time |
Reference |
ELISA |
Serum |
IgA and IgG |
200 |
— |
51 |
ELISA |
Serum |
IgM |
86 |
— |
52 |
ELISA |
Serum |
IgG and IgM |
238 |
— |
53 |
ELISA |
Serum |
IgG and IgM |
216 |
— |
54 |
Lateral flow immunoassay |
Blood, serum, plasma |
IgG and IgM |
134 |
15 min |
55 |
Lateral flow immunoassay |
Serum |
IgG and IgM |
814 |
10 min |
56 |
Lateral flow immunoassay |
Serum |
IgG |
19 |
10 min |
57 |
Lateral flow immunoassay |
Blood, serum and plasma |
IgG and IgM |
525 |
15 min |
58 |
Chemiluminescence immunoassay |
Serum |
IgG and IgM |
125 |
— |
46 |
Chemiluminescence immunoassay |
Serum |
IgA, IgG and IgM |
699 |
— |
59 |
Chemiluminescence immunoassay |
Serum |
IgG and IgM |
367 |
— |
60 |
Chemiluminescence immunoassay |
Blood |
IgA, IgG and IgM |
95 |
— |
61 |
Chemiluminescence immunoassay |
Serum |
IgG and IgM |
29 |
— |
62 |
4.1 Enzyme-linked immunosorbent assay
The enzyme-linked immunosorbent assay (ELISA) method is simple to perform and highly popular. It has been widely used for the rapid quantitative detection of IgM/IgG antibodies in serum. In this method, the antigen protein immobilized on the surface of microplate wells, binds to the target antibody (IgM/IgG) in serum and an enzyme-labeled anti-IgM/IgG secondary antibody is used for quantitative detection. Liu et al., evaluated the performance of nucleocapsid and spike protein-based ELISA assays in the detection of SARS-CoV-2 IgM and IgG antibodies in human serum, respectively. They found that the sensitivity of the spike protein-based ELISA (85.7%) for IgM detection was significantly higher than that of the nucleocapsid protein-based ELISA (57.1%) for detecting antibodies against SARS-CoV-2. These results indicate that the spike protein may be more suitable for early diagnosis of COVID-19 through the detection of IgM antibody in serum samples.63
4.2 Lateral flow assay
Colloidal gold-immunochromatographic assay (GICA) is the primary method for on-site diagnosis in primary medical institutions and disease control centers, given its ease of use, rapid results, and low cost. Zhang et al., prepared six recombinant antigens of nucleocapsid protein and spike protein fragments. The recombinant antigens (rS1 and rS-RBD-mFc) with the highest IgM/IgG antibody titers were chosen for the GICA development. The performance of the GICA was evaluated with 814 serum samples from SARS-CoV-2 infected patients, and demonstrated high detection sensitivity (86.89%) and specificity (99.39%). Furthermore, 65.63% (21/32) of the clinically confirmed, but RT-PCR negative samples, were found to be positive when using GICA, demonstrating that it is a powerful auxiliary tool to improve the accuracy of COVID-19 diagnosis.56
4.3 Chemiluminescence assay
Chemiluminescence immunoassay (CLIA) is a technological innovation of the traditional immunoassay that combines chemiluminescence techniques with immunochemical reactions. Its working principle is similar to the other labeled immunoassays, in that it uses chemical probes or substrate to generate light emission through chemical or enzymatic reactions to detect a target. In recent years, CLIA has been very attractive in different fields, such as clinical diagnosis, environmental monitoring, and food safety due to its high sensitivity, automated operation, less time, and wide linear range. Cai et al., developed a peptide-based magnetic chemiluminescence enzyme immunoassay for serological diagnosis of COVID-2019. To evaluate the performance of the immunoassay, 276 serum samples from confirmed patients were tested and the positive rate of IgG and IgM was 71.4% (197/276) and 57.2% (158/276), respectively. By combining with RT-PCR detection, the immunoassay may help to improve the accuracy of the diagnosis of COVID-19.60
5. Imaging diagnostics
A recent study investigating a large sample of SARS-CoV-2 infected patients found that approximately 57% of patients had abnormal lung imaging.64 These finding suggest that imaging diagnostics can be another complementary tool to support the diagnosis of COVID-19. Imaging diagnostics primarily includes chest computed tomography (CT) and lung ultrasound, both of which are rapid diagnostic tools.
5.1 Computed tomography
Computed tomography is a common auxiliary detection technology that is widely used in the diagnosis of different diseases. Changes to the lungs imaging caused by SARS-CoV-2 infection can be used as the basis for the diagnosis of COVID-19. To evaluate the feasibility of CT in the early detection of COVID-19 pneumonia. Caruso et al., compared the diagnostic performance of chest CT with RT-PCR. In 158 study participants, the sensitivity, accuracy and specificity of chest CT was 97%, 72% and 56%, respectively,65 which is similar to the results reported by Ai et al.66 Although the CT scan showed higher sensitivity in the diagnosis of COVID-19, there are some limitations. For example, CT scans are more likely to generate false positives and false negatives due to the difficulty of distinguishing COVID-19 from other viral pneumonias by radiologists and the hysteresis of abnormal CT imaging.
5.2 Lung ultrasound
Lung ultrasound is another promising auxiliary tool in the diagnosis of COVID-19. Compared with CT, lung ultrasound can be performed directly at bedside by a doctor in isolation ward, reducing the risk of the spread of SARS-CoV-2in the hospital. Importantly, lung ultrasound is a radiation-free test, making it suitable for special populations, particularly desirable for pregnant women.67,68 Kalafat et al., reported on a case involving a pregnant woman who initially tested negative using RT-PCR, but was later found to be positive through lung ultrasound. This demonstrates the potential for lung ultrasound to play an important role in the triage of pregnant women with suspected COVID-19.67
6. Summary
At present, the COVID-19 epidemic is still spreading, meanwhile, the effective vaccines or drugs have been not approved by regulatory agencies. Given this, early diagnosis and social distancing still are the most effective ways to protect the public. This review aimed to summarize available COVID-19 diagnostic technologies and discuss their benefits and limitations. Although RT-PCR is the gold standard for the detection of SARS-CoV-2, other nucleic acid assays include digital PCR, RT-LAMP, DETECTR, SHERLOCK, and gene sequencing are under development or have been approved. Antibody-based serological diagnosis is also available widely and can be used with molecular diagnostic technology to improve the detection accuracy. In addition, this method can collect epidemiological information on COVID-19 and provide data to support public health policy decisions. In short, within the diagnostic technologies available, future research should focus on improving the sensitivity, accuracy, and screening throughput, as well as automatization.
Conflicts of interest
There are no conflicts to declare.
Acknowledgements
This research was funded by the Natural Science Foundation of Hainan Province (grant number 219QN149 and 2019RC119) and the National Natural Science Foundation of China (grant number 31760493 and 31901800). The APC was funded by the Scientific Research Foundation of Hainan University [grant number KYQD1631 and KYQD(ZR)1957].
References
- Y. Du, L. Tu, P. Zhu, M. Mu, R. Wang, P. Yang, X. Wang, C. Hu, R. Ping and P. Hu, Am. J. Respir. Crit. Care Med., 2020, 201, 1372–1379 CrossRef CAS.
- F. Wu, S. Zhao, B. Yu, Y.-M. Chen, W. Wang, Z.-G. Song, Y. Hu, Z.-W. Tao, J.-H. Tian and Y.-Y. Pei, Nature, 2020, 579, 265–269 CrossRef CAS.
- P. Zhou, X.-L. Yang, X.-G. Wang, B. Hu, L. Zhang, W. Zhang, H.-R. Si, Y. Zhu, B. Li and C.-L. Huang, Nature, 2020, 579, 270–273 CrossRef CAS.
- D. Wrapp, N. Wang, K. S. Corbett, J. A. Goldsmith, C.-L. Hsieh, O. Abiona, B. S. Graham and J. S. McLellan, Science, 2020, 367, 1260–1263 CrossRef CAS.
- D. R. Boulware, M. F. Pullen, A. S. Bangdiwala, K. A. Pastick, S. M. Lofgren, E. C. Okafor, C. P. Skipper, A. A. Nascene, M. R. Nicol and M. Abassi, N. Engl. J. Med., 2020, 383, 517–525 CrossRef CAS.
- Y. Wang, D. Zhang, G. Du, R. Du, J. Zhao, Y. Jin, S. Fu, L. Gao, Z. Cheng and Q. Lu, Lancet, 2020, 395, 1569–1578 CrossRef CAS.
- A. Calderaro, M. C. Arcangeletti, F. De Conto, M. Buttrini, P. Montagna, S. Montecchini, F. Ferraglia, F. Pinardi and C. Chezzi, Int. J. Infect. Dis., 2020, 96, 387–389 CrossRef CAS.
- I. Hamming, W. Timens, M. Bulthuis, A. Lely, G. v. Navis and H. van Goor, J. Pathol., 2004, 203, 631–637 CrossRef CAS.
- W. Wang, Y. Xu, R. Gao, R. Lu, K. Han, G. Wu and W. Tan, J. Am. Med. Assoc., 2020, 323, 1843–1844 CAS.
- K. K.-W. To, O. T.-Y. Tsang, W.-S. Leung, A. R. Tam, T.-C. Wu, D. C. Lung, C. C.-Y. Yip, J.-P. Cai, J. M.-C. Chan and T. S.-H. Chik, Lancet Infect. Dis., 2020, 20, 565–574 CrossRef CAS.
- Y. Pan, D. Zhang, P. Yang, L. L. M. Poon and Q. Wang, Lancet Infect. Dis., 2020, 20, 411–412 CrossRef CAS.
- R. Rangan, I. N. Zheludev and R. Das, RNA, 2020, 076141 Search PubMed.
- V. M. Corman, O. Landt, M. Kaiser, R. Molenkamp, A. Meijer, D. K. Chu, T. Bleicker, S. Brunink, J. Schneider, M. L. Schmidt, D. G. Mulders, B. L. Haagmans, B. van der Veer, S. van den Brink, L. Wijsman, G. Goderski, J. L. Romette, J. Ellis, M. Zambon, M. Peiris, H. Goossens, C. Reusken, M. P. Koopmans and C. Drosten, Euro Surveill., 2020, 25, 2000045 Search PubMed.
- R. Liu, H. Han, F. Liu, Z. Lv, K. Wu, Y. Liu, Y. Feng and C. Zhu, Clin. Chim. Acta, 2020, 505, 172–175 CrossRef CAS.
- F. Yu, L. Yan, N. Wang, S. Yang, L. Wang, Y. Tang, G. Gao, S. Wang, C. Ma and R. Xie, Clin. Infect. Dis., 2020, 71, 793–798 CrossRef CAS.
- R. Lu, J. Wang, M. Li, Y. Wang, J. Dong and W. Cai, medRxiv, 2020 DOI:10.1101/2020.03.24.20042689.
- C. Yan, J. Cui, L. Huang, B. Du, L. Chen, G. Xue, S. Li, W. Zhang, L. Zhao, Y. Sun, H. Yao, N. Li, H. Zhao, Y. Feng, S. Liu, Q. Zhang, D. Liu and J. Yuan, Clin. Microbiol. Infect., 2020, 26, 773–779 CrossRef CAS.
- W. E. Huang, B. Lim, C. C. Hsu, D. Xiong, W. Wu, Y. Yu, H. Jia, Y. Wang, Y. Zeng and M. Ji, Microb. Biotechnol., 2020, 13, 950–961 CrossRef CAS.
- J. P. Broughton, X. Deng, G. Yu, C. L. Fasching, V. Servellita, J. Singh, X. Miao, J. A. Streithorst, A. Granados, A. Sotomayor-Gonzalez, K. Zorn, A. Gopez, E. Hsu, W. Gu, S. Miller, C. Y. Pan, H. Guevara, D. A. Wadford, J. S. Chen and C. Y. Chiu, Nat. Biotechnol., 2020, 1–5 Search PubMed.
- J. Joung, A. Ladha, M. Saito, M. Segel, R. Bruneau, M.-l. W. Huang, N.-G. Kim, X. Yu, J. Li and B. D. Walker, medRxiv, 2020 DOI:10.1101/2020.05.04.20091231.
- M. Wang, A. Fu, B. Hu, Y. Tong, R. Liu, J. Gu, J. Liu, W. Jiang, G. Shen, W. Zhao, D. Men, L. Yu, Z. Deng, Y. Li and T. Liu, Small, 2020, 16, 2002169 CrossRef CAS.
- Y. Yang, M. Yang, C. Shen, F. Wang, J. Yuan, J. Li, M. Zhang, Z. Wang, L. Xing, J. Wei, L. Peng, G. Wong, H. Zheng, M. Liao, K. Feng, J. Li, Q. Yang, J. Zhao, Z. Zhang, L. Liu and Y. Liu, medRxiv, 2020 DOI:10.1101/2020.02.11.20021493.
- H. Feng, Y. Liu, M. Lv and J. Zhong, Jpn. J. Radiol., 2020, 38, 409–410 CrossRef CAS.
- J. F.-W. Chan, C. C.-Y. Yip, K. K.-W. To, T. H.-C. Tang, S. C.-Y. Wong, K.-H. Leung, A. Y.-F. Fung, A. C.-K. Ng, Z. Zou and H.-W. Tsoi, J. Clin. Microbiol., 2020, 58, e00310-20 CrossRef.
- S. Pfefferle, S. Reucher, D. Nörz and M. Lütgehetmann, Eurosurveillance, 2020, 25, 2000152 CrossRef.
- C. Liu, B. Huang, R. Zhou, H. Wu, M. Qiao, C. Chen, W. Zhang, J. Dong, S. Zhu and J. Huang, OSF Preprints, 2020 DOI:10.31219/osf.io/khtp4.
- Y. Li, L. Yao, J. Li, L. Chen, Y. Song, Z. Cai and C. Yang, J. Med. Virol., 2020, 92, 903–908 CrossRef CAS.
- T. Suo, X. Liu, J. Feng, M. Guo, W. Hu, D. Guo, H. Ullah, Y. Yang, Q. Zhang, X. Wang, M. Sajid, Z. Huang, L. Deng, T. Chen, F. Liu, K. Xu, Y. Liu, Q. Zhang, Y. Liu, Y. Xiong, G. Chen, K. Lan and Y. Chen, medRxiv, 2020 DOI:10.1080/22221751.2020.1772678.
- X. Zhang, S. B. Lowe and J. J. Gooding, Biosens. Bioelectron., 2014, 61, 491–499 CrossRef CAS.
- L. Becherer, N. Borst, M. Bakheit, S. Frischmann, R. Zengerle and F. von Stetten, Anal. Methods, 2020, 12, 717–746 RSC.
- M. Jinek, K. Chylinski, I. Fonfara, M. Hauer, J. A. Doudna and E. Charpentier, Science, 2012, 337, 816–821 CrossRef CAS.
- J. S. Gootenberg, O. O. Abudayyeh, M. J. Kellner, J. Joung, J. J. Collins and F. Zhang, Science, 2018, 360, 439–444 CrossRef CAS.
- J. S. Gootenberg, O. O. Abudayyeh, J. W. Lee, P. Essletzbichler, A. J. Dy, J. Joung, V. Verdine, N. Donghia, N. M. Daringer and C. A. Freije, Science, 2017, 356, 438–442 CrossRef CAS.
- X. Tang, C. Wu, X. Li, Y. Song, X. Yao, X. Wu, Y. Duan, H. Zhang, Y. Wang and Z. Qian, Natl. Sci. Rev., 2020, 7, 1012–1023 CrossRef CAS.
- P. Forster, L. Forster, C. Renfrew and M. Forster, Proc. Natl. Acad. Sci. U.S.A., 2020, 117, 9241–9243 CrossRef CAS.
- Z. Shen, Y. Xiao, L. Kang, W. Ma, L. Shi, L. Zhang, Z. Zhou, J. Yang, J. Zhong and D. Yang, Clin. Infect. Dis., 2020, 71, 713–720 CrossRef CAS.
- Z.-P. Yao, P. A. Demirev and C. Fenselau, Anal. Chem., 2002, 74, 2529–2534 CrossRef CAS.
- K. Bezstarosti, M. M. Lamers, B. L. Haagmans and J. A. Demmers, bioRxiv, 2020 DOI:10.1101/2020.04.23.057810.
- B. Shen, X. Yi, Y. Sun, X. Bi, J. Du, C. Zhang, S. Quan, F. Zhang, R. Sun, L. Qian, W. Ge, W. Liu, S. Liang, H. Chen, Y. Zhang, J. Li, J. Xu, Z. He, B. Chen, J. Wang, H. Yan, Y. Zheng, D. Wang, J. Zhu, Z. Kong, Z. Kang, X. Liang, X. Ding, G. Ruan, N. Xiang, X. Cai, H. Gao, L. Li, S. Li, Q. Xiao, T. Lu, Y. Zhu, H. Liu, H. Chen and T. Guo, Cell, 2020, 182, 59–72 CrossRef CAS.
- G. Seo, G. Lee, M. J. Kim, S. H. Baek, M. Choi, K. B. Ku, C. S. Lee, S. Jun, D. Park, H. G. Kim, S. J. Kim, J. O. Lee, B. T. Kim, E. C. Park and S. I. Kim, ACS Nano, 2020, 14, 5135–5142 CrossRef CAS.
- S. Yanling, S. Jia, W. Xinyu, H. Mengjiao, S. Miao, Z. Lin, L. Bingqian, S. Haicong, Z. Zhi and Y. Chaoyong, Anal. Chem., 2020, 92, 9895–9900 CrossRef.
- Z. Chen, Q. Wu, J. Chen, X. Ni and J. Dai, Virol. Sin., 2020, 35, 351–354 CrossRef CAS.
- J. Huo, A. Le Bas, R. R. Ruza, H. M. Duyvesteyn, H. Mikolajek, T. Malinauskas, T. K. Tan, P. Rijal, M. Dumoux and P. N. Ward, Nat. Struct. Mol. Biol., 2020, 1–9 Search PubMed.
- Q. Lin, D. Wen, J. Wu, L. Liu, W. Wu, X. Fang and J. Kong, Anal. Chem., 2020, 92, 9454–9458 CrossRef CAS.
- M. Lipsitch, R. Kahn and M. J. Mina, Nat. Med., 2020, 1–2 Search PubMed.
- M. Infantino, V. Grossi, B. Lari, R. Bambi, A. Perri, M. Manneschi, G. Terenzi, I. Liotti, G. Ciotta, C. Taddei, M. Benucci, P. Casprini, F. Veneziani, S. Fabbri, A. Pompetti and M. Manfredi, J. Med. Virol., 2020, 1–5 Search PubMed.
- R. Liu, X. Liu, L. Yuan, H. Han, M. A. Shereen, J. Zhen, Z. Niu, D. Li, F. Liu and K. Wu, Int. Immunopharmacol., 2020, 106746 CrossRef CAS.
- S. E. F. Yong, D. E. Anderson, W. E. Wei, J. Pang, W. N. Chia, C. W. Tan, Y. L. Teoh, P. Rajendram, M. P. H. S. Toh and C. Poh, Lancet Infect. Dis., 2020, 20, 809–815 CrossRef CAS.
- P. R. Randad, N. Pisanic, K. Kruczynski, Y. C. Manabe, D. Thomas, A. Pekosz, S. Klein, M. J. Betenbaugh, W. A. Clarke and O. Laeyendecker, medRxiv, 2020 DOI:10.1101/2020.05.24.20112300.
- W. H. Organization, Population-based age-stratified seroepidemiological investigation protocol for coronavirus 2019 (COVID-19) infection, 26 May 2020, World Health Organization, 2020 Search PubMed.
- M. Isabel, G. Damien, K. Benoit, D. Hafid, R. Soleimani, C. Vincenzo, V. Olivier, G. Beatrice, W. Fleur and R.-V. Hector, J. Clin. Virol., 2020, 104413 Search PubMed.
- Q. Wang, Q. Du, B. Guo, D. Mu, X. Lu, Q. Ma, Y. Guo, L. Fang, B. Zhang and G. Zhang, J. Clin.
Microbiol., 2020, 58, e00375 CrossRef.
- L. Liu, W. Liu, Y. Zheng, X. Jiang, G. Kou, J. Ding, Q. Wang, Q. Huang, Y. Ding and W. Ni, Microb. Infect., 2020, 22, 206–211 CrossRef CAS.
- F. Xiang, X. Wang, X. He, Z. Peng, B. Yang, J. Zhang, Q. Zhou, H. Ye, Y. Ma and H. Li, Clin. Infect. Dis., 2020, ciaa461 CrossRef.
- Y. Pan, X. Li, G. Yang, J. Fan, Y. Tang, J. Zhao, X. Long, S. Guo, Z. Zhao, Y. Liu, H. Hu, H. Xue and Y. Li, J. Infect., 2020, 81, 28–32 CrossRef.
- P. Zhang, Q. Gao, T. Wang, Y. Ke, F. Mo, R. Jia, W. Liu, L. Liu, S. Zheng, Y. Liu, L. Li, Y. Wang, L. Xu, K. Hao, R. Yang, S. Li, C. Lin and Y. Zhao, medRxiv, 2020 DOI:10.1101/2020.03.17.20036954.
- Z. Chen, Z. Zhang, X. Zhai, Y. Li, L. Lin, H. Zhao, L. Bian, P. Li, L. Yu and Y. Wu, Anal. Chem., 2020, 92, 7226–7231 CrossRef CAS.
- Z. Li, Y. Yi, X. Luo, N. Xiong, Y. Liu, S. Li, R. Sun, Y. Wang, B. Hu and W. Chen, J. Med. Virol., 2020, 92, 1518–1524 CrossRef CAS.
- H. Ma, W. Zeng, H. He, D. Zhao, Y. Yang, D. Jiang, P. Zhou, Y. Qi, W. He, C. Zhao, R. Yi, X. Wang, B. Wang, Y. Xu, Y. Yang, A. J. Kombe Kombe, C. Ding, J. Xie, Y. Gao, L. Cheng, Y. Li, X. Ma and T. Jin, medRxiv, 2020 DOI:10.1101/2020.04.17.20064907.
- X. Cai, J. Chen, J. Hu, Q. Long, H. Deng, K. Fan, P. Liao, B. Liu, G. Wu, Y. Chen, Z. Li, K. Wang, X. Zhang, W. Tian, J. Xiang, H. Du, J. Wang, Y. Hu, N. Tang, Y. Lin, J. Ren, L. Huang, J. Wei, C. Gan, Y. Chen, Q. Gao, A. Chen, C. He, D. Wang, P. Hu, F. Zhou, A. Huang, P. Liu and D. Wang, J. Infect. Dis., 2020, 222, 189–193 CrossRef CAS.
- G. Lippi, G. L. Salvagno, M. Pegoraro, V. Militello, C. Caloi, A. Peretti, S. Gaino, A. Bassi, C. Bovo and G. Lo Cascio, Clin. Chem. Lab. Med., 2020, 58, 1156–1159 CAS.
- A. Padoan, C. Cosma, L. Sciacovelli, D. Faggian and M. Plebani, Clin. Chem. Lab. Med., 2020, 58, 1081–1088 CAS.
- W. Liu, L. Liu, G. Kou, Y. Zheng, Y. Ding, W. Ni, Q. Wang, L. Tan, W. Wu and S. Tang, J. Clin. Microbiol., 2020, 58, e00461 Search PubMed.
- W. J. Guan, Z. Y. Ni, Y. Hu, W. H. Liang, C. Q. Ou, J. X. He, L. Liu, H. Shan, C. L. Lei, D. S. C. Hui, B. Du, L. J. Li, G. Zeng, K. Y. Yuen, R. C. Chen, C. L. Tang, T. Wang, P. Y. Chen, J. Xiang, S. Y. Li, J. L. Wang, Z. J. Liang, Y. X. Peng, L. Wei, Y. Liu, Y. H. Hu, P. Peng, J. M. Wang, J. Y. Liu, Z. Chen, G. Li, Z. J. Zheng, S. Q. Qiu, J. Luo, C. J. Ye, S. Y. Zhu, N. S. Zhong and C. China Medical Treatment Expert Group, N. Engl. J. Med., 2020, 382, 1708–1720 CrossRef CAS.
- D. Caruso, M. Zerunian, M. Polici, F. Pucciarelli, T. Polidori, C. Rucci, G. Guido, B. Bracci, C. de Dominicis and A. Laghi, Radiology, 2020, 201237 Search PubMed.
- T. Ai, Z. Yang, H. Hou, C. Zhan, C. Chen, W. Lv, Q. Tao, Z. Sun and L. Xia, Radiology, 2020, 200642 Search PubMed.
- D. Buonsenso, F. Raffaelli, E. Tamburrini, D. Biasucci, S. Salvi, A. Smargiassi, R. Inchingolo, G. Scambia, A. Lanzone and A. Testa, Ultrasound Obstet. Gynecol., 2020, 56, 106–109 CrossRef CAS.
- G. Secco, M. Delorenzo, C. Zattera, B. G. Moore, L. Demitry, G. Vezzoni, F. Resta, B. Barcella, G. Cappa and T. Perrone, Emerg. Care J., 2020, 16, 35–38 Search PubMed.
|
This journal is © The Royal Society of Chemistry 2020 |
Click here to see how this site uses Cookies. View our privacy policy here.