DOI:
10.1039/C4AN02001D
(Communication)
Analyst, 2015,
140, 2086-2092
Rapid identification of goblet cells in unstained colon thin sections by means of quantum cascade laser-based infrared microspectroscopy†
Received
31st October 2014
, Accepted 26th January 2015
First published on 26th January 2015
Abstract
Changes in the volume covered by mucin-secreting goblet cell regions within colon thin sections may serve as a means to differentiate between ulcerative colitis and infectious colitis. Here we show that rapid, quantum cascade laser-based mid-infrared microspectroscopy might be able to contribute to the differential diagnosis of colitis ulcerosa, an inflammatory bowel disease. Infrared hyperspectral images of mouse colon thin sections were obtained within 7.5 minutes per section with a pixel size of 3.65 × 3.65 μm2 and a field of view of 2.8 × 3.1 mm2. The spectra were processed by training a random decision forest classifier on the basis of k-means clustering on one thin section. The trained algorithm was then applied to 5 further thin sections for a blinded validation and it was able to identify goblet cells in all sections. The rapid identification of goblet cells within these unstained, paraffinized thin sections of colon tissue was enabled by the high content of glycopeptides within the goblet cells as revealed by the pronounced spectral signatures in the 7.6 μm–8.6 μm and the 9.2 μm–9.7 μm wavelength ranges of the electromagnetic spectrum. More so, the simple calculation of the ratio between the absorbance values at 9.29 μm and 8.47 μm provides the potential to further shorten the time for measurement and analysis of a thin section down to well below 1 minute.
Introduction
Among the autoimmune disorders of the digestive system is the inflammatory bowel disease (IBD). Ulcerative colitis and Crohn's disease constitute the most prevalent forms of idiopathic IBD. These chronic inflammatory disorders of the gastrointestinal tract are characterized by distinct modifications of clinical, pathological, endoscopic and radiological features.1,2
In practice, a common problem is the differentiation of an infectious colitis from an inflammatory bowel disease such as ulcerative colitis. While some severe cases of infectious colitis need to be treated with antibiotics, ulcerative colitis is usually treated in a different manner. Diagnosis of ulcerative colitis and the differentiation from other conditions is done by the interpretation of biopsies in combination with clinical, laboratory, radiographic and endoscopic observations. One of the histological characteristics to distinguish between these two vastly different forms of colitis might be achieved by comparing the amount of epithelial goblet cells in colonic mucosa; while ulcerative colitis will cause depletion (or emptying) of goblet cells,3 non-chronic intestinal infections usually do not affect the size of the histological area containing goblet cells. Further examples for changes in goblet cell population caused by intestinal infections include hyperplasia caused by helminth infections and the depletion in population caused by chronic infections.4–6
In those cases, in which gastrointestinal biopsies are performed, standard histopathology is performed on stained thin sections that originate from the biopsies. For general purposes, including the highlighting of goblet cells, haematoxylin and eosin (H&E) staining is used. As goblet cells produce mucins, Alcian blue and PAS staining can be used to highlight the goblet cell population.
Due to recent technical advances, the reagent-free assessment of thin sections of tissue raises increased interest. The use of light sources of high spectral power density in the mid-infrared spectral region, namely tunable quantum cascade lasers (QCLs),7 in combination with, potentially, low cost microbolometer arrays enables a rapid, reagent free analysis on the basis of mid-infrared microspectroscopy.8–11 Beyond the initial reagent-free, QCL-based investigations of biomedical samples at a single wavelength in vitro9,12 and in vivo,13 Kröger et al. were recently able to show in a one-to-one comparison, that a reagent-free chemical analysis of unstained tissue samples can be performed within 5 minutes (as compared to hours and days when using standard broadband FT-IR microspectroscopy) using a tunable QCL.11 While the traditional FT-IR techniques offer a good signal-to-noise ratio and a broad spectral range, extensive acquisition times are needed for FT-IR microspectroscopy, which can only be circumvented by costly equipment such as large, liquid nitrogen cooled MCT array detectors or synchrotron radiation.
In this work we demonstrate the rapid visualization of colonic mucosa via hyperspectral imaging using a QCL-based setup with particular emphasis on the identification of goblet cell rich regions in the colonic epithelium.
Materials and methods
Colon samples
A colon sample was obtained from a healthy male C57BL/6 mouse (age: 6 months). The sample was immersion fixed in 4% buffered formaldehyde for 24 h and then embedded in paraffin using a Leica TP1020. For this purpose, the sample was post-fixed, dehydrated in a series of graded alcohols and infiltrated with paraffin as follows: 4% buffered formaldehyde (2 × 1.5 h), 70% ethanol (1.5 h), 80% ethanol (1.5 h), 96% ethanol (1.5 h), 99% ethanol (2 × 1.5 h), xylol (2 × 1.5 h), and paraffin (3 × 1.5 h). The tissue sample was collected according to the regulations of the German Animal Welfare Act (I13/10, Animal Welfare Office, Medical Faculty Mannheim of the University of Heidelberg). Two 8 μm thin sections were cut using a Leica RM 2165 microtome. The thin sections were placed on a barium fluoride substrate (Korth Kristalle GmbH, Germany). Each slice features three colon tissue sections. For comparison, multiple adjacent slices of 3 μm thickness were prepared from the vicinity of the 8 μm thick sections and positioned on glass substrates for imaging with a standard microscope (Zeiss Axio Scope.A1, Carl Zeiss Microscopy GmbH, Germany). Haematoxylin and eosin (H&E) staining was performed on the third adjacent slice per layer while Alcian blue and PAS staining was performed on the first adjacent slice per layer to visualize goblet cells.
Infrared microscopy
QCL-based infrared chemical imaging was performed using the home built setup described in ref. 10. Due to the particular interest in goblet cells, the available spectral region needed to include glycosylation bands which are typical markers for mucins produced by goblet cells. For human colonic tissue, glycosylation bands have been located at 1044, 1076 and 1125 cm−1.14 According to the literature, close similarities between the spectral features of mouse and human colonic tissue can be expected.15 Two quantum cascade lasers (Daylight Solutions Inc., USA) were successively tuned to the wavenumber regions between 1027–1087 cm−1 and 1167–1319 cm−1, corresponding to wavelengths of 9.74 μm–9.20 μm and 8.57 μm–7.58 μm. For a sample measurement, each laser was tuned over the entire tuning range within 11.3 seconds 20 times. A 640 × 480 pixel microbolometer array (Infratec GmbH, Germany) was used to record the transmission through the sample at any given wavelength. The frame rate was 50 Hz. All measurements were performed with a projected pixel pitch in the sample plane of 3.65 μm. Two infrared lenses were used for a 4
:
1 magnification. The field of view amounted to 2.8 × 3.1 mm2. The spatial resolution was determined to be 9.4 ± 1.8 μm (at 1065 cm−1).
Synchronization of the laser tuning time and the bolometer array's frame rate resulted in an effective wavenumber resolution of 4 cm−1 which suffices for analyzing mid-infrared spectra of the paraffin-embedded tissue thin sections.16,17
The total acquisition time for infrared microscope images over the full tuning ranges of both lasers was determined to be 7.5 minutes for each of the six samples. A single background measurement was performed within an additional 7.5 minutes once, in order to serve as a reference for all of the 6 hyperspectral tissue images. Transmittance was calculated by normalizing the single channel spectrum of the sample with that of the blank substrate. Absorbance spectra were calculated as the negative natural logarithm of the transmission spectra for each pixel individually. No further pre-processing was performed apart from the background correction, spectral binning (average bin size of 2.4 cm−1), water vapour correction, and a Gaussian smoothing filter with a size of 3 pixels. Spectral binning and background correction can be performed at the measurement's runtime. Water vapour correction was performed after clustering and binning to adjust the imperfect purging of the microscope setup with dried air. As humidity did not match perfectly between reference and sample measurements but was constant during the sample measurements, water vapour correction did not affect the clustering or classification results.
Because the lateral resolution is not limited by the pixel size for the measurements described here, a small Gaussian filter appears to be beneficial for the visual inspection of the images since it reduces pixelation effects. While further data pre-processing – especially scattering correction – would greatly enhance the quality of the data, it is also very time consuming. Depending on the application, the excess amount of computation time needed might not be justified.
Data analysis
When analyzing the mid-infrared spectral image information, there is a risk of the so-called overfitting (see e.g.ref. 16). In order to avoid overfitting we use a four-step approach:
(1) Random selection of one out of the six thin sections, henceforth referred to as the “training sample”
(2) Unsupervised classification of the sample data by using the information from the training sample only. k-means clustering was performed on the training dataset. A total of 10 clusters were chosen, 3 of which were attributed to paraffin spectra (dotted lines in Fig. 2(d)) or the blank substrate and 7 were attributed to tissue (see, for example, ref. 18). A total of 323
300 single spectra were analysed. The resulting cluster memberships of these spectra were used as the ground truth for training of the random decision forest classifier in step 3.
(3) Training of a random decision forest on the basis of step 2, whereby only the training sample is used. A total of 100 decision trees were grown (see, for example, ref. 19).
(4) After steps 1–3, no retraining or changes of any kind are acceptable. We then applied the trained random decision forest to the remaining 5 thin sections (“blinded validation”) and provided an analysis of the outcome. Each pixel was assigned to one of the 10 cluster groups (which are resulting from k-means clustering) by applying the random decision forest classifier.
All analyses were performed by means of MATLAB (R2014a, The MathWorks Inc., USA) on a standard computer with an Intel® Core™ i7-3930k-CPU at 3.20 GHz and with 32 GB RAM.
Results
A first impression of the hyperspectral dataset (resulting from infrared microspectroscopy) may be obtained by visualizing a transmission image at a single wavelength already. In Fig. 1(a), infrared transmission at 1218 cm−1 (±1.2 cm−1) through the entire colon cross section is shown: the central lumen is surrounded by colonic epithelium, connective tissue (lamina propria mucosae) and smooth muscle tissue (lamina muscularis mucosae). The paraffin matrix surrounding the smooth muscle tissue is visible. A digital zoom (Fig. 1(b)) indicates a substructure in the epithelium.
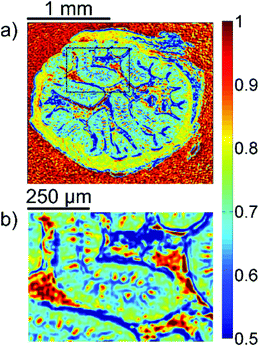 |
| Fig. 1 Infrared transmission of the colon sample. The data shown represent the infrared transmission at 1218 cm−1 (±1.2 cm−1). (a) The complete tissue section, including the surrounding smooth muscle tissue. (b) A digital zoom, highlighting the structures in the epithelium. | |
The single wavelength infrared image reveals surprisingly rich information about the tissue structure. For further information, however, the full spectral ranges were analyzed based on step #2 of the Data analysis section, namely k-means clustering (k = 10). Fig. 2 shows the results of the k-means clustering of the slice used as a training set. In the right column of Fig. 2a–c the label image is shown. The 3 clusters with lowest average absorbance can be attributed to paraffin of the blank substrate and are coloured white for clarity. Average spectra of each of the remaining 7 clusters are shown in Fig. 2(d). In order to link the tissue clusters to tissue types, the unstained slice (Fig. 2a–c, central column) may be compared to the cluster image and the H&E stained adjacent slices (Fig. 2a–c left column). Additionally, the distribution of the green pixel class is compared to the microscopic image of the Alcian blue and PAS stained colon tissue slice in Fig. 3.
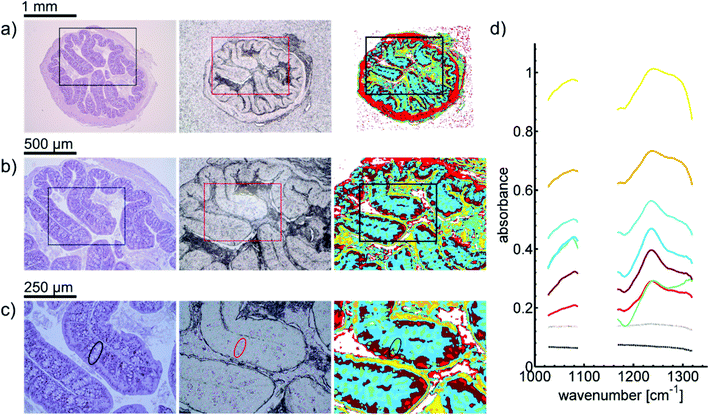 |
| Fig. 2 (a)–(c) Microscopic image of a H&E stained colon slice (left column), an unstained colon tissue slice (central column), and label images obtained via k-means clustering of infrared data of the training set (right column). For the images of H&E stained and unstained slices, (a)–(c) represent optical zoom steps, with the magnified area highlighted by a box. The corresponding series of label images results from a digital zoom. Goblet cell rich regions in crypts are labelled green (marked by circle). (d) The mean cluster spectra obtained by clustering. Dotted lines represent the average spectra for clusters which were assigned to the paraffin background. | |
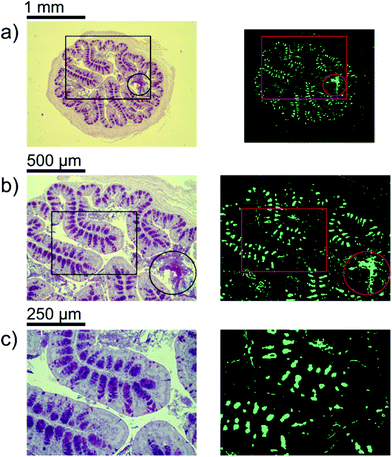 |
| Fig. 3 (a)–(c) Microscopic image of Alcian blue and PAS stained colon slice (left column) and distribution of green pixels in label images obtained via k-means clustering of infrared data of the training set (right column). For the images of the Alcian blue and PAS stained slice. (a)–(c) represent optical zoom steps, with the magnified area highlighted by a box. The corresponding series of label images results from a digital zoom. An area having secreted mucus is highlighted by circles. | |
In order to illustrate the predictive potential of the classification scheme, a blinded validation was performed (step#4 of the Data analysis section). The data from all the tissue sections of the validation set were visualized using the trained random decision forest classifier. Results of the classification can be seen in Fig. 4. Analogous analysis of the remaining four tissue sections can be found in the ESI (Fig. S1–S4†).
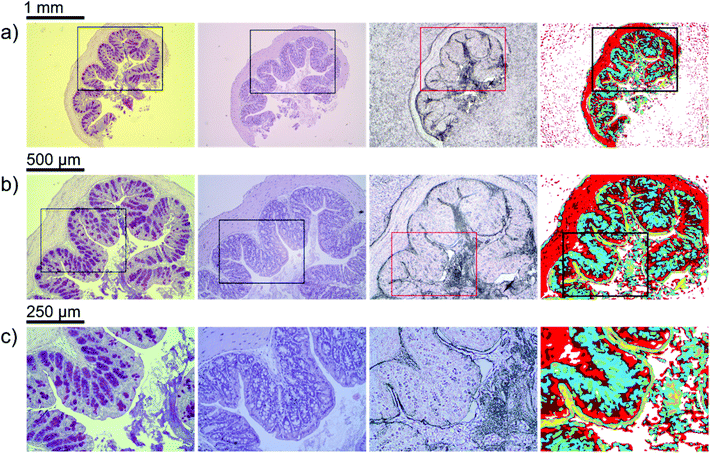 |
| Fig. 4 (a)–(c) Microscopic image of an Alcian blue and PAS stained colon tissue slice, a H&E stained colon tissue slice, an unstained colon tissue slice, and label images obtained via random decision forest classification of infrared data (from left to right). For the images of stained and unstained slices, the images (a)–(c) represent optical zoom steps, with the magnified area highlighted by a box, because Alcian blue and PAS stained slices need to be considered as well. The corresponding series of label images results from a digital zoom. | |
Discussion
At first sight, the infrared images clearly resemble the morphology of the H&E stained as well as the unstained thin sections. However, given the spectral character of the acquired data, further assignment of the features and their biochemical interpretation is desirable.
Goblet cell rich regions
Inside the colon, the mucin-containing mucus forms an essential barrier that protects the body from pathogens and irritants. Mucin is produced in the goblet cells that are present in the colonic mucosa. Since mucin consists of highly glycosylated peptides, strong infrared absorption in the carbohydrate region (1000–1100 cm−1) is expected. By comparing the result of the k-means clustering of the training set or the results of the random-decision forest classification of the validation set with Alcian blue and PAS stained colon tissue slices, the green pixel class appears to be strongly correlated to the location of patches with high goblet cell numbers. Note that an area containing the secreted mucus in the central lumen (marked by circles in Fig. 3) is also assigned to the green cluster, leading to the conclusion that areas of high mucin concentration are assigned to the green class. This observation is supported by the increased ratio of absorbance in the carbohydrate spectral region, with a maximum absorbance at the glycosylation band at 1076 cm−1, to the absorbance in the 1200–1300 cm−1 region. Analysis of the training data illustrates the potential for discrimination between different tissue types. The ratio of absorbance at 1076 cm−1 over 1181 cm−1, for example, illustrates the potential for spectral differentiation between the mucin-producing goblet cells and the rest of the tissue (Table 1 and Fig. 5(a)).
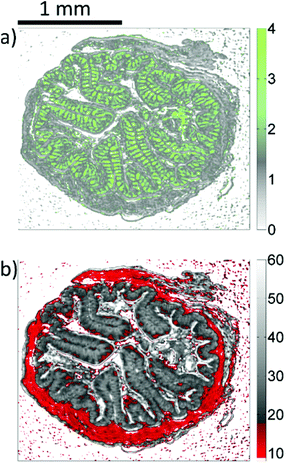 |
| Fig. 5 Microscopic images resulting from the analysis of infrared data of the training set. (a) Represents the ratio of absorbances at 1076 cm−1 and 1181 cm−1. (b) Represents the integral absorbance over the 1027 cm−1–1087 cm−1 spectral range (see also in Table 1). | |
Table 1 Overview of the color assignments. The ratio of absorbance at 1076 cm−1 over 1181 cm−1 and the absorbance integral from 1027 cm−1 to 1087 cm−1 was calculated for each pixel in the training set for the random decision forest classifier. For each pixel class defined by the initial k-means clustering, the median value of the results was calculated. The difference of median to the 5% and 95% quantile is given by −Δq0.05, +Δq0.95
Color |
Biomedical association |
Absorbance ratio: 1076 cm−1/1181 cm−1 [arb. units] |
Absorbance integral: 1027 cm−1 to 1087 cm−1 [arb. units] |
Median −Δq0.05, +Δq0.95 |
|
Green |
Mucin/goblet cells |
2.97−1.45, +7.24 |
26.07−6.10, +5.95 |
|
Light red |
Lamina muscularis, Lamina propria, colon enterocytes |
1.08−0.45, +0.82 |
12.48−3.22, +4.39 |
|
Dark red |
Lamina propria |
1.29−0.58, +0.70 |
19.25−5.06, +4.60 |
|
Light blue |
Colon epithelial cells |
1.43−0.58, +0.82 |
26.03−5.15, +7.05 |
|
Teal |
Colon epithelial cells, epithelium in the vicinity of goblet cells |
1.10−0.41, +0.56 |
31.24−8.11, +9.46 |
|
Yellow |
Mucus layer in the lumen, interfaces |
1.08−0.30, +0.41 |
61.16−14.23,+32.53 |
|
Orange |
Mucus layer in the lumen, interfaces |
1.04−0.33, +0.47 |
42.41−10.87, +13.44 |
Muscularis (lamina propria/enterocytes)
Surrounding each colon section, a ring representing the lamina muscularis is clearly visible. It is associated with the light red pixel class. This class features the lowest average absorbance compared with all other classes that were not considered as background spectra. Also, the lamina propria as well as some of the colon enterocytes is labelled light red. The absorbance integral over the carbohydrate spectral region (see Table 1 and Fig. 5(b)) may be sufficient for a simplified differentiation between the lamina muscularis and the rest of the tissue.
Lamina propria
There appears to be an increase of pixels of the dark red class below the enterocytes. A small amount of pixels in the lamina muscularis was also assigned to the dark red cluster, which indicates the connective tissue surrounding vessels. Dark red regions can also be found within the mucosal folds. These observations indicate that the dark red class is connected to the lamina propria.
Epithelium
The most prominent class in the epithelium is light blue. However, a considerable amount of pixels are assigned to the teal class. The location of teal pixels appears to correlate with the location of the goblet cells. This could indicate the influence of scattering interferences on the spectra in the teal labelled regions. Also, the colour distribution might resemble the location of crypt lumina and connective tissue. The surface epithelium is largely composed of light red and dark red clusters, possibly caused by higher concentration of enterocytes.
Further findings
The yellow and orange pixel classes appear to be located at the intersections of the epithelial layer to the lumen. Also, some regions of the lamina propria and mucus inside the lumen are labelled yellow and orange, respectively. Contributions from scattering are enhanced by refractive index mismatch, which occurs at these locations. Refractive index mismatching is also likely to occur in the central lumen of crypts, possibly affecting the spectra of the surrounding pixels, which are mostly assigned to green and teal.
In order to avoid strong over-segmentation before training of the classifier, the number of clusters was chosen to be ten. By doing so, a link between most pixel classes and tissue features was easily established. However, a large number of classes may be beneficial to limit the effect of scattering interferences on the analysis. The appearance of the light red (lamina muscularis) class on a paraffin background could be reduced, as well as the amount of yellow and orange pixels (interferences) in the central lumen. A multi-staged cluster analysis could be used to gain more insight, without introducing too many colors for the final representation.
Apart from the good qualitative agreement between the stained images and the infrared label image, further analysis reveals deviations in the detected distribution of goblet cell rich regions (Fig. 3) between the Alcian blue and PAS stained colon slices and the label image of their adjacent slice. We believe that these deviations are mainly due to comparison with non-identical thin sections, and artefacts caused by microtome cutting as well as the significant difference in the slice thickness (3 μm compared to 8 μm). In turn, the correlation between the label images and the visible images of the identical, unstained slices is found to be much better. It is noteworthy that the complexity of the clustering and classification approach may compromise sensitivity. A simplified and potentially faster strategy is provided by a dual-wavelength measurement and the distribution of mucin concentration may be visualized in gray scale images as shown in Fig. 5(a).
The acquisition time for a hyperspectral image of a sample was 7.5 minutes and covered the full spectral range of 1027–1087 cm−1 and 1167–1319 cm−1 at a pixel size of 3.65 × 3.65 μm2 and a field of view of 2.8 × 3.1 mm2. On the one hand, this is more time consuming than the standard H&E staining. On the other hand, H&E staining requires the tissue sample to be deparaffinized, whereas the IR spectroscopy is best in paraffinized tissue. Deparaffinizing a thin section usually takes 35 minutes.
The acquisition time for QCL-based hyperspectral imaging may be further reduced by limiting the tuning ranges. Measurements at merely two wavenumbers (1076 cm−1 and 1181 cm−1, see Table 1) could, for example, already be sufficient for high contrast imaging of goblet cell rich regions in the epithelium, as shown in Fig. 5(a).
Conclusions
We have successfully demonstrated the automated detection of mucin-rich regions in mouse colon mucosa using QCL-based hyperspectral imaging and a random decision forest classifier. The total field of view of a single measurement was 3.1 × 2.8 mm2. The medically relevant identification and enumeration of the mucin-containing goblet cell population may be performed within 7.5 minutes (including measurement and data analysis). Given the results presented in this manuscript, the process could be further shortened down to an overall time requirement for measurement and analysis of well below 1 minute. Further experiments are needed to clarify to what extent the procedure could be used to augment differential diagnosis of gastrointestinal diseases and in particular the differentiation between ulcerative colitis and intestinal infections.
Acknowledgements
The authors would like to thank S. Spiegel for his technical support and E. Seelinger for sample preparation. A. Schönhals acknowledges support by the Heidelberg Graduate School of Fundamental Physics. This work was financed by Baden-Württemberg Stiftung.
Notes and references
- R. J. Xavier and D. K. Podolsky, Unravelling the pathogenesis of inflammatory bowel disease, Nature, 2007, 448, 427–434 CrossRef CAS PubMed.
- D. K. Podolsky, Inflammatory bowel disease, N. Engl. J. Med., 2002, 347, 417–429 CrossRef CAS PubMed.
- M. Gersemann, S. Becker, I. Kübler, M. Koslowski, G. Wang, K.R. Herrlinger, J. Griger, P. Fritz, K. Fellermann, M. Schwab, J. Wehkamp and E.F. Stange, Differences in goblet cell differentiation between Crohn's disease and ulcerative colitis, J. Diff., 2009, 77, 84–94 CrossRef CAS PubMed.
- D. Jenkins, M. Balsitis, S. Gallivan, M. F. Dixon, H. M. Gilmour, N. A. Shepherd, A. Theodossi and G. T. Williams, Guidelines for the initial biopsy diagnosis of suspected chronic idiopathic inflammatory bowel disease, J. Clin. Pathol., 1997, 50, 93–105 CrossRef CAS.
- Y. S. Kim and S. B. Ho, Intestinal Goblet Cells and Mucins in Health and Disease: Recent Insights and Progress, Curr. Gastroenterol, 2010, 319–330 CrossRef CAS PubMed , Rep 12.
-
M. Classen, G. N. J. Tytgat and C. J. Lightdale, Gastroenterological Endoscopy, Thieme, Stuttgart, Germany, 2010, pp. 607–608, ISBN (Americas): 9783131258526 Search PubMed.
- J. Faist, F. Capasso, D. L. Sivco, C. Sirtori, A. L. Hutchinson and A. Y. Cho, Quantum Cascade Laser, Science, 1994, 264, 553–556 CAS.
- M. C. Phillips and B. E. Bernacki, Hyperspectral microscopy of explosives particles using an external cavity quantum cascade laser, Opt. Eng., 2013, 52, 061302 CrossRef PubMed.
- M. R. Kole, R. K. Reddy, M. V. Schulmerich, M. K. Gelber and R. Bhargava, Discrete frequency infrared microspectroscopy and imaging with a tunable quantum cascade laser, Anal. Chem., 2012, 84, 10366–10372 CrossRef CAS PubMed.
- N. Kröger, A. Egl, M. Engel, N. Gretz, K. Haase, I. Herpich, S. Neudecker, A. Pucci, A. Schönhals and W. Petrich, Rapid hyperspectral imaging in the mid-infrared, Proc. SPIE – Int. Soc. Opt. Eng., 2014, 8939, 89390Z Search PubMed.
- N. Kröger, A. Egl, M. Engel, N. Gretz, K. Haase, I. Herpich, B. Kränzlin, S. Neudecker, A. Pucci, A. Schönhals, J. Vogt and W. Petrich, Quantum cascade laser-based hyperspectral imaging of biological tissue, J. Biomed. Opt., 2014, 19, 111607 CrossRef PubMed.
- P. Bassan, M. J. Weida, J. Rowlette and P. Gardner, Large scale infrared imaging of tissue micro arrays (TMAs) using a tunable Quantum Cascade Laser (QCL) based microscope, Analyst, 2014, 139, 3856 RSC.
- C. Vrančić, N. Kröger, N. Gretz, S. Neudecker, A. Pucci and W. Petrich, A Quantitative Look Inside the Body: Minimally Invasive Infrared Analysis in vivo, Anal. Chem., 2014, 86, 10511–10514 CrossRef PubMed.
- A. Travo, O. Piot, R. Wolthuis, C. Gobinet, M. Manfait, J. Bara, M.-E. Forgue-Lafitte and P. Jeannesson, IR spectral imaging of secreted mucus: a promising new tool for histopathological recognition of human colonic adenocarcinomas, Histopathology, 2010, 56, 921–931 CrossRef PubMed.
- M. A. Cohenford, S. J. Lim, C. Brown, M. A. Chaudhry, S. Sigdel, E. Beckelhimer and B. Rigas, FT-IR Microspectroscopy of Mouse Colon Tissues: Insight into the Chemistry of Carcinogenesis and Diagnostic Potential, Am. J. Pathol., 2012, 181, 1961–1968 CrossRef CAS PubMed.
-
W. Petrich, From study design to data analysis, in Biomedical Vibrational Spectroscopy, ed. P. Lasch, J. Kneipp, Wiley & Sons, Hoboken, New Jersey, USA, 2008, 315–332, ISBN 978-0-470-22945-3 Search PubMed.
-
P. Lasch and W. Petrich, Data Acquisition and Analysis in Biomedical Vibrational Spectroscopy, in Biomedical Applications of Synchrotron Infrared Microspectroscopy: A Practical Approach, RSC Analytical Spectroscopy Series v. 11, ed. D. Moss, Royal Society of Chemistry, Cambridge, UK, 2010, 192–225 Search PubMed.
- P. Lasch, W. Haensch, D. Naumann and M. Diem, Imaging of colorectal adenocarcinoma using FT-IR microspectroscopy and cluster analysis, Biochim. Biophys. Acta, 2004, 1688, 176–186 CrossRef CAS PubMed.
- D. M. Mayerich, M. Walsh, A. Kadjacsy-Balla, S. Mittal and R. Bhargava, Breast histopathology using random decision forests-based classification of infrared spectroscopic imaging data, Proc. SPIE – Int. Soc. Opt. Eng., 2014, 9041, 904107 CrossRef PubMed.
Footnotes |
† Electronic supplementary information (ESI) available. See DOI: 10.1039/c4an02001d |
‡ Next to his affiliation with the Kirchhoff-Institute for Physics, W. Petrich is an employee of Roche Diagnostics GmbH, Germany. |
|
This journal is © The Royal Society of Chemistry 2015 |