DOI:
10.1039/D4TB00936C
(Review Article)
J. Mater. Chem. B, 2024, Advance Article
Advancements in DNA computing: exploring DNA logic systems and their biomedical applications
Received
30th April 2024
, Accepted 3rd September 2024
First published on 6th September 2024
Abstract
DNA computing is regarded as one of the most promising candidates for the next generation of molecular computers, utilizing DNA to execute Boolean logic operations. In recent decades, DNA computing has garnered widespread attention due to its powerful programmable and parallel computing capabilities, demonstrating significant potential in intelligent biological analysis. This review summarizes the latest advancements in DNA logic systems and their biomedical applications. Firstly, it introduces recent DNA logic systems based on various materials such as functional DNA sequences, nanomaterials, and three-dimensional DNA nanostructures. The material innovations driving DNA computing have been summarized, highlighting novel molecular reactions and analytical performance metrics like efficiency, sensitivity, and selectivity. Subsequently, it outlines the biomedical applications of DNA computing-based multi-biomarker analysis in cellular imaging, clinical diagnosis, and disease treatment. Additionally, it discusses the existing challenges and future research directions for the development of DNA computing.
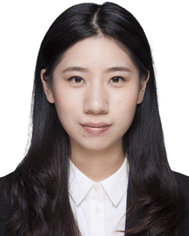 Yuewei Zhao | Yuewei Zhao is a laboratory technician at the Shanghai Chest Hospital, Shanghai Jiao Tong University School of Medicine. She is currently pursuing her master's degree under the supervision of Prof. Lin Huang at the Department of Clinical Laboratory Diagnostics, Shanghai Jiao Tong University School of Medicine. Her research interests include diagnostics based on functional nucleic acids and nanomaterials. |
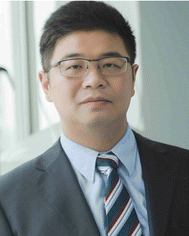 Jiayi Wang | Prof. Jiayi Wang received his PhD in Clinical Laboratory Diagnostics from Shanghai Jiao Tong University School of Medicine. Currently, he serves as a principal investigator (PI) at the Shanghai Chest Hospital. The research direction of his group is the development and enhancement of cancer diagnostic systems. |
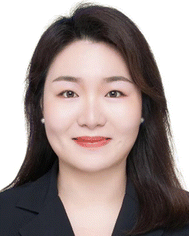 Lin Huang | Prof. Lin Huang received her PhD degree in Biomedical Engineering from Shanghai Jiao Tong University, China. Currently, she is a senior research fellow at the Shanghai Chest Hospital, Shanghai Jiao Tong University School of Medicine. Her research interests focus on the development of diagnostic assays based on spectrometric methods. |
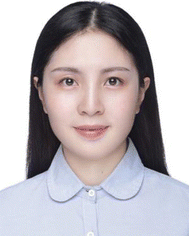 Fanyu Meng | Dr Fanyu Meng received her PhD in Biomedical Engineering from the University of Science and Technology of China in 2020. She is now an assistant researcher at the Shanghai Chest Hospital, Shanghai Jiao Tong University School of Medicine, China. Her research interests primarily focus on nanoengineered materials for diverse applications, including biosensors, chemical sensors, and in vitro diagnostics. |
1. Introduction
DNA computing represents a novel paradigm in molecular computation, integrating principles from information science and biology. The fundamental concept involves leveraging the information processing capabilities of organic biological molecules to replace traditional digital physical switch components.1–3 The duplex helical configuration and base complementarity of DNA molecules enable the encoding of problems into specific DNA sequences. When paired with a specified DNA sequence acting as a switch, an output DNA sequence with a concentration proportional to the input DNA concentration is generated, resembling a simple analog circuit.4,5 DNA circuits utilize the concentration of specific DNA strands as signals, achieving addition, subtraction, and multiplication operations through the synthesis or cleavage of chemical bonds.6–8 In contrast, these DNA molecule reaction strategies are relatively simple, with few reports focusing on their application capabilities in analytical science. With the swift progress of synthetic biology technologies, DNA computing has garnered widespread research interest due to its powerful programmable and parallel computing capabilities.9–11
Currently, although bioelectronics based on DNA computing is still in its developmental stage, the concept of assembling microscale biological component circuits to construct parallel biological computing systems has been preliminarily established.12 In 2004, Okamoto and colleagues first merged digital circuits with DNA computing, cascading DNA logic gates to form complex circuits, ultimately achieving universal DNA computing.13–15 By integrating the structural and functional characteristics of DNA, various DNA logic systems can be designed and integrated into autonomous computing devices.16,17 These systems employ binary-coded DNA or other triggers as inputs (with “0” representing absence and “1” representing presence), with DNA strands or optical/electrochemical signals serving as outputs (with “0” indicating low and “1” indicating high).18–20 Leveraging the inherent advantages of DNA, along with its strict Boolean logic and biological compatibility, DNA computing finds extensive applications in fields such as biological analysis, diagnostics, imaging, and drug delivery.21–23 However, DNA computing remains limited in its clinical applications, owing to insufficient clarification of the relationship between biological computing and analytical performance.
Previous reviews mainly focused on the basic concepts and functional principles of DNA in data storage,2,24,25 logic programming,20,26 and molecular computing.13,14 For comparison, the novelty and significance of this work lie in the development of novel DNA logic gates designed to enhance sensing performance (such as sensitivity and specificity), and to expand the scope of computational applications especially for biomedical use. In the past three years, significant progress has been made in DNA logic gates, particularly through the use of DNA motifs, nanomaterials, and DNA nanostructures. Therefore, we concentrated on exploring improvements in the efficiency, sensitivity, and selectivity of cascading molecular reactions, providing insights that may guide the future development of analytical science. Additionally, we comprehensively reviewed and analyzed the latest advancements in DNA computation for biomedical applications, including but not limited to cellular imaging, clinical diagnosis, and disease treatment. Finally, we discussed the challenges faced by DNA computing and proposed future prospects for this field. Scheme 1 illustrates DNA logic gates constructed using various materials, along with their associated biomedical applications, including cellular imaging, clinical diagnosis, and disease treatment. We anticipate that the review will contribute to advancing the field of DNA computing and provide insights for intelligent biomedical diagnostic applications.
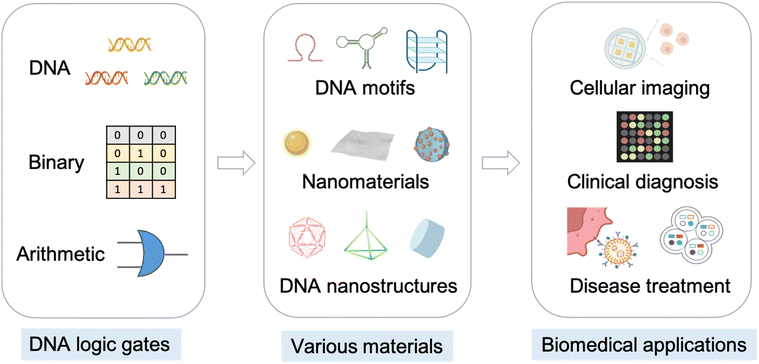 |
| Scheme 1 DNA logic gates, various materials for constructing DNA logic gates, and related biomedical applications. | |
2. DNA logic systems
To construct a DNA-based computer, it is indispensable to initially build DNA logic gates, which function as devices that transform sets of inputs into observable outputs through logical processes. From fundamental logic gates (AND, OR, YES, NOT, XOR, and INHIBIT) to complex logical operations (addition, subtraction, and multiplication), these are essential computing components for constructing DNA logic systems.27,28 DNA molecules are designed to perform logical operations on binary inputs. In a DNA logic system, “inputs” are typically DNA strands with specific sequences, and “outputs” are other DNA strands produced as a result of biochemical reactions.29,30 The presence or absence of specific DNA strands in the system can represent binary values (0 or 1). Logic gates can be represented in terms of equivalent logic circuits and truth tables (Table 1). For example, the “AND” system has two input DNA strands, A and B, each representing a binary “1”. If both strands are present, they bind to a complementary “template” strand, triggering the production of a new output strand, C, which represents the binary “1”. If either A or B is missing, the reaction doesn't proceed, and no output is produced (binary “0”). Additionally, fundamental arithmetic logic operations (the half-adder/subtractor) include addition, which combines two numbers to produce a sum, and subtraction, which determines the difference between two numbers (Table 2).14,31 In summary, a DNA logic system is a molecular computing approach where DNA molecules and biochemical reactions are engineered to perform logical operations.
Table 1 Corresponding truth tables of logic gates
Input |
Output |
(A) |
(B) |
YES(A) |
NOT(A) |
AND |
OR |
INHIBIT |
XOR |
0 |
0 |
0 |
1 |
0 |
0 |
0 |
0 |
0 |
1 |
0 |
1 |
0 |
1 |
1 |
1 |
1 |
0 |
1 |
0 |
0 |
1 |
0 |
1 |
1 |
1 |
1 |
0 |
1 |
1 |
0 |
0 |
|
|
 |
 |
 |
 |
 |
 |
Table 2 Equivalent logic circuits of fundamental arithmetic operations
Half-adder |
Half-subtractor |
Full-adder |
Full-subtractor |
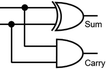 |
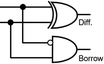 |
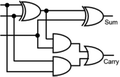 |
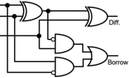 |
DNA computing combines the analytical and computational capabilities of Boolean logic with the functionality of DNA as a material.32,33 DNA logic systems possess programmability, parallel processing capability, and biocompatibility, allowing for the design and adaptation of logic circuits while facilitating data processing and real-time monitoring. In biological analysis, integrating various materials into DNA logic systems can significantly enhance detection efficiency, sensitivity, and specificity. Here, we classify DNA logic systems based on various materials, including functional DNAs (motifs, DNAzymes, G-quadruplexes, etc.), nanomaterials (metal nanoparticles, metal nanoclusters, graphene oxides, magnetic beads, etc.), and DNA nanostructures (DNA tetrahedrons, DNA origamis, DNA tile assembly, etc.).34
2.1. Functional DNA-based DNA logic systems
2.1.1. DNA motifs. DNA motifs refer to specific nucleotide sequences (such as base pair patterns) or structural features (such as hairpins, loops, or bulges) that serve distinct biological or chemical functions.35 In DNA logic systems, they play several crucial roles, such as recognition elements, structural formation, catalytic sites, signal transducers, and contributors to overall system functionality. What's more, DNA hybridizations like toehold-mediated strand displacement (TMSD), catalytic hairpin assembly (CHA), and hybridization chain reaction (HCR) are extensively utilized for logic computation and enhanced biosensing.36,37 Miao et al. developed an electrochemical platform for multiplex miRNA (miR-21, miR-20a, and miR-106a) detection by combining cascade strand displacement and bipedal DNA walking.38 A series of logic gates including NOT, AND, and OR, are effectively constructed after structure transformation by TMSD, achieving a low detection limit (10 aM) for miRNA diagnostics (threshold current: 2 μA). Similarly, Chai et al. developed these typical logic operations based on HCR and CHA.39 The cyclic DNA machine facilitated the analysis of apurinic/apyrimidinic endonuclease 1 (APE1) with a limit of detection (LOD) of 7.8 × 10−5 U mL−1. Due to the complexity of sequence design resulting from exact strand displacement (ESD), an alternative approach called fuzzy strand displacement (FSD) was proposed. Wang et al. utilized Klenow polymerase for the FSD reactions, which enabled the displacement of multiple substrate strands by one input strand or the displacement of one substrate strand by multiple input strands (Fig. 1A).40 They constructed fuzzy modules and proved that the recognition network required less sequence design complexity (ESD
:
FSD = 20
:
8). What's more, Lapteva et al. have advanced DNA strand-displacement circuits by combining temporal memory and logic computation (AND gate), allowing these circuits to make decisions based on specific input combinations and timing (Fig. 1B).41 The approach preserved temporal information within memory strands, with each one encoding the relative timing. The incorporation of temporal information into Boolean logic computation, holds promise for diverse circuit structures, including DNA-based neural networks.
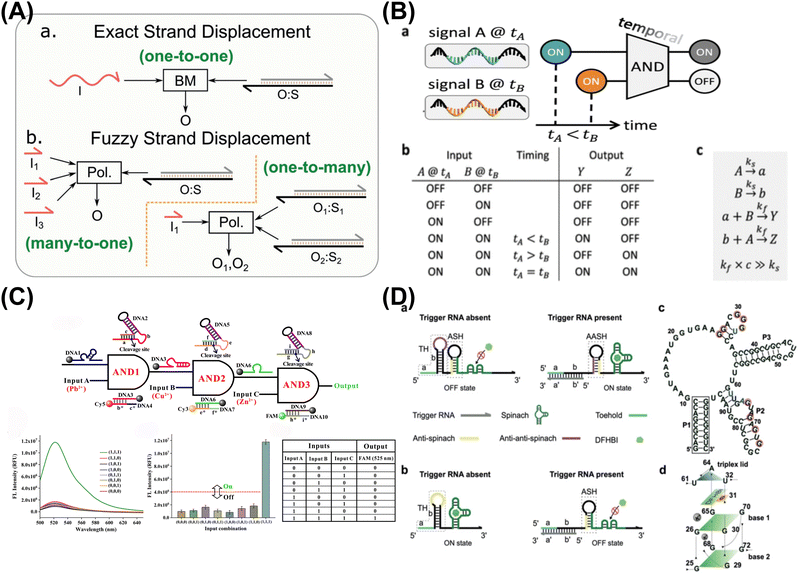 |
| Fig. 1 Functional DNA-based DNA logic systems. (A) Fuzzy DNA strand displacement to decrease the complexity of the DNA network. Reproduced with permission.40 Copyright 2020, Wiley-VCH. (B) DNA strand-displacement temporal logic circuits. Reproduced with permission.41 Copyright 2022, American Chemical Society. (C) DNAzyme-based scalable logic circuits with multiple outputs and automatic reset functions. Reproduced with permission.42 Copyright 2021, American Chemical Society. (D) G-quadruplex-based riboswitch architectures for the switchable fluorescent logic gate platform. Reproduced with permission.43 Copyright 2023, Wiley-VCH. | |
2.1.2. DNAzymes. DNAzymes are synthetic nucleic acid enzymes that catalyze specific biochemical reactions, such as the cleavage or ligation of nucleic acids, by adopting unique three-dimensional structures. Metal ions are crucial for their activity, as they stabilize the DNAzyme structure and enhance catalytic efficiency. Metal ion-dependent DNAzymes are excellent biocatalysts for signal amplification and have been effectively utilized in DNA logic systems for detecting various targets. Hu et al. presented DNAzyme-based TMSD and introduced circular and dissipative characteristics.44 With the assistance of active DNAzyme and Mg2+, two distinct temporal AND gates were designed for miRNA and APE 1 detection. This method used less than 10 strands, thereby obviating the necessity for intricate network designs. To improve sensitivity and specificity, Zeng et al. developed AND operation-controlled DNAzyme nanoflower and reconfigured it with APE1 and O6-methylguanine methyltransferase (MGMT) to generate readable fluorescence.45 Due to the enrichment facilitated by the DNAzyme nanoflower, the fluorescence response increased by 60% compared to the unbound DNAzyme duplex. In the presence of APE1 (10 U mL−1) and MGMT (580 nM), the LODs were calculated to be 2.40 nM and 0.07 U mL−1, respectively. Since the non-resettable nature of logic systems limits the effectiveness of logic operations, Shi et al. developed concatenated AND logic circuits using heavy metal ion-dependent DNAzymes and a series of TMSD reactions (Fig. 1C).42 Three inputs (Pb2+, Cu2+, and Zn2+) and corresponding fluorescence outputs were integrated, with LODs of 2, 30, and 50 nM, respectively. In the DNAzyme circuits, the logic network acted as an automatic reset keypad lock owing to the spontaneous cleavage process that occurred. This multi-output, auto-reset and easily scalable logic platform holds great promise for information processing.
2.1.3. G-quadruplex. The G-quadruplex is recognized as one of the most popular materials, and its properties have been extensively leveraged in the function of multifunctional DNA-based logic devices. Wang et al. utilized riboswitch-based switching mechanisms to develop fluorescent light-up RNA aptamers (FLAPs) that could be activated by RNA triggers and metabolites (adenine and guanine) (Fig. 1D).43 These FLAPs contained G-quadruplexes in their fluorophore binding pocket, the critical nucleotides of which could be bound through anti-FLAP chains, rendering them inactive. Logic gates (AND, NOR, and NIMPLY) were constructed by using two-input switches with the toehold- and ligand-controlled FLAPs, showing high ON/OFF fluorescence ratios with minimal signal leakage. Based on the photosensitizer loading function of the G-quadruplex, the Sgc4f and Sgc8c aptamers were designed for AND logic computation, resulting in a 7.7-fold amplification of fluorescence signals.46 G-quadruplex structures have additionally been discovered to possess catalytic properties similar to peroxidase enzymes, enabling them to catalyze oxidation reactions of substrates in the presence of a hydrogen peroxide (H2O2) cofactor. Li et al. used the G-quadruplex structure as chromogenic catalysts and constructed the complementary NOR and AND logic gates.47 The linear detection range and limit for CA125 are 35–500 U mL−1 and 0.91 U mL−1, respectively, while for CEA, they are 5–500 ng mL−1 and 0.88 ng mL−1. Similarly, Wang et al. reported on the G-quadruplex/Cu(II) (G4/Cu) metal-nanozyme's behavior toward dual luminescent substrates (Amplex Ultrared and Scopoletin) with contrasting reactions when H2O2 is present.48 They achieved multilevel contrary logic computing and detected cysteine/bleomycin, resulting in detection limits calculated to be 6.7 nM and 10 nM, respectively. In summary, these DNA logic platforms, which depend on the interaction of the G-quadruplex with labeled probes or other organic dyes, continue to drive the advancement of innovative DNA computing systems.
2.2. Nanomaterial-based DNA logic systems
2.2.1. Metal nanomaterials. In addition to biological materials, the diverse development of metal nanomaterials undoubtedly enhances the functionality and performance of DNA logic systems.49,50 Gold nanoparticles (AuNPs) are ideal for biomedical applications due to their biocompatibility, tunable size, and ease of functionalization. Gao et al. utilized DNA-based nanostructures to apply the Hamming code, which enables error detection and correction within communication systems (Fig. 2A).51 Utilizing AuNPs as nanocarriers, DNA nanostructures were assembled to perform XOR and Erasure Code logic tasks such as calculating check codes through strand displacement reaction (SDR) and correcting erroneous data through fluorescence signals. This system could protect the confidentiality and integrity of data during transmission, which depends on the marking and structural support function of the metal nanomaterials. Yu et al. established an AND logic platform with dual output modes of fluorescence and photothermal detection. CeO2@Au nanoparticles synergize the catalytic and redox properties of cerium oxide with the high reactivity of gold, thereby enhancing their performance in biosensing applications. CeO2@Au nanoparticles functioned as nanoenzymes, with CeO2 exhibiting oxidase-like activity catalyzing the oxidation of TMB to TMBox, and AuNPs possessing efficient DNA binding ability.52 The catalytic assembly of the hairpin (CHA), triggered by cancer associated miRNAs, propelled the DNA walker through SDR that led to the amplification of fluorescence signals. By the AND logic gates, both miR-21 and miR-155 were measured ranging from 10 pM to 100 nM and 10 pM to 200 nM, respectively. It can be seen that metal nanomaterials can precisely control and manipulate the interaction between nanomaterials and DNA, promoting the development and execution of complex DNA logic circuits.
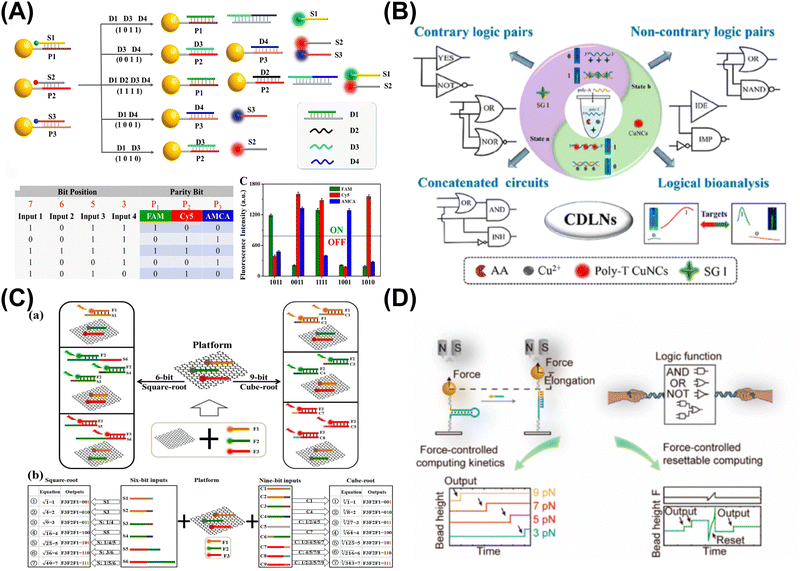 |
| Fig. 2 Nanomaterial-based DNA logic systems. (A) Metal nanomaterials and leveraging DNA-based nanostructures for data communication. Reproduced with permission.51 Copyright 2023, American Chemical Society. (B) Metal nanoclusters-based DNA logic nanodevices for minute-level DNA computing. Reproduced with permission.53 Copyright 2023, American Chemical Society. (C) Graphene oxide-based computing system for multiplexing logic operations. Reproduced with permission.54 Copyright 2020, Elsevier. (D) Magnetic tweezers-based single-molecule resettable DNA computing. Reproduced with permission.55 Copyright 2022, American Chemical Society. | |
2.2.2. Metal nanoclusters. DNA-templated metal nanoclusters have been acknowledged as exceptional tools for biosensing owing to their straightforward synthesis and adjustable luminescence properties. Han et al. constructed concurrent DNA logic nanodevices (CDLNs) by integrating Cu nanoclusters (poly-T CuNCs) with SYBR Green I for dual outputs (Fig. 2B).53 All CDLNs, including contrary logic pairs (YES∧NOT, OR∧NOR, and Even∧Odd number classifier) and noncontrary ones (IDE∧IMP and OR∧NAND), were achieved within just 10 minutes via a “one-stone-two-birds” approach that spent 1/12 of the time and 1/4 of the cost compared to previous methods. By AND∧NAND pair, the polyadenine/polymerase could be detected in vitro with an LOD of 2 × 10−4 U μL−1. They took advantages of concurrent contrary logic, meeting the requirements of potential early diagnosis. Similarly, Lv et al. developed a series of parallel reverse logic gates (CCLGs) with opposing functionalities (OR∧NOR, YES∧NOT, XOR∧XNOR, INHIBIT∧IMPLICATION, and MAJORITY∧MINORITY) and expanded cascade logic circuits using a hairpin DNA template Ag nanocluster (H-AgNCs) as a versatile dual-output producer.56 As the hairpin structure unfolded, H-AgNCs, acting as noncovalent signaling reporter molecules, also changed their two emissions inversely with the hairpin structure. This logical system simplified the complexity and instability of the system, eliminating the need for cumbersome and costly labeling procedures. When using these systems, issues such as photobleaching and background interference need to be considered.
2.2.3. Graphene oxides. Graphene oxide (GO) is extensively utilized as an optimal biocomputational nanomaterial due to its varying affinities with single/double DNA and its property of bursting nearby fluorescence through fluorescence resonance energy transfer (FRET). Geng et al. programmed the hybridization process between input DNA and the DNA/GO response substrate to implement two large-scale logic operation circuits, the 6-bit square root and the 9-bit cube root (Fig. 2C).54 This work offered possibilities for additional integration and modularity in biocomputing. He et al. proposed an integrated design strategy, constructing DNA nanotripods and combining them with GO to fabricate the versatile integration platform for various logic operations.57 By adjusting the spatial position between fluorophore ROX and GO through the DNA nanotripod, they controlled the FRET efficiency, resulting in changes in ROX fluorescence. The platform enabled the implementation of three-input majority gates, multiple basic logic gates (AND, OR, XOR, INHIBIT), and integrated gates (INHIBIT–AND). DNA nanotripod-GO platform-based logic operations can be easily customized to create intelligent sensors and analyzers for various biomedical applications. However, the use of GO in DNA logic systems faces challenges such as biocompatibility, integration with DNA, signal transduction, etc.
2.2.4. Other 2D materials. In addition to graphene, other two-dimensional (2D) materials like MoS2 and antimonene can also significantly enhance the performance of DNA logic gates. Su et al. developed a MoS2-based electrochemical aptasensor for the simultaneous detection of thrombin and ATP (acting as an AND logic gate).58 The sensor used MoS2 to stabilize AuNPs to form hierarchical nanocomposites that exhibit excellent electrochemical properties. The sensor demonstrated high sensitivity, detecting as low as 0.74 nM ATP and 0.0012 nM thrombin. Due to the stronger interaction between antimonene and single stranded nucleic acids or peptides, Bu et al. demonstrated the use of antimonene as a platform for peptide-based DNA logic gates.59 Peptides were combined with antimonene to detect Pb2+ and perform logic operations. Fluorescently labelled peptides acted as probes, with fluorescence recovery signalling the logic output, showcasing antimonene's potential in molecular sensing and information processing. These 2D materials demonstrate potential in semiconductor nanocomposite applications and emerging electronic devices; however, both face challenges related to material fabrication and environmental stability.
2.2.5. Magnetic beads. The unique characteristics of magnetic separation have enabled its incorporation into DNA logic systems, offering notable efficiency and specificity.60 Pei et al. implemented single-molecule DNA logic gates and circuits using magnetic tweezers, which could be manipulated and reset by supporting forces (Fig. 2D).55 By reading the hairpin DNA reaction signal, the rate of the stretch-controlled TMSD reaction was directly measured. A kinetics-controllable TMSD reaction was achieved, exhibiting a range of approximately 19-fold change in reaction rate under various stretching forces. Furthermore, OR, AND, and NOT gates were also implemented. This study demonstrated the potential in accurately regulating the computing process. To address the scalability limitations faced by multi bit adders in circuits, Xie et al. introduced cooperative SDR to build DNA full adders.61 By leveraging a parity-check algorithm, two logic gates XOR–AND were formed using a group of double-helix DNAs. A one-bit full adder was realized through just three gates comprising 13 chains, resulting in a 90% reduction in chain requirement compared to previous circuit configurations. Employing this structure alongside a starter on magnetic beads, they demonstrated the DNA 6-bit adder, which is comparable in scale to a traditional electronic full adder chip. This approach is anticipated to achieve specific applications through the most effective DNA operation and be implemented in extended digital computing.
2.2.6. Polymers. Versatile polymers can provide structural support and signal transmission for complex DNA logic devices. Fan et al. utilized the polydopamine nanospheres (PDs) to adsorb and quench fluorescent-labeled single-stranded DNA, and developed a DNA-based molecular parity generator and checker (pG/pC) for error detection during data transmission.62 The total operation (error checking/output correction) of this non-G4 pG/pC system was completed in just 1 hour (about 1/3 of other versions). Additionally, PD-based logic system could perform complex three-input concatenated logic calculations (XOR-Inhibit). To overcome slow DNA computation (often requiring several hours), Engelen et al. employed supramolecular polymers to accelerate SDR reactions by 100 times through non-covalent interactions between DNA molecules and the supramolecular polymer benzene-1,3,5-tricarboxamide (BTA).63 By recruiting DNA circuit assemblies with a 10-nucleotide stalk chain, they demonstrated improved efficiency and product stability in multi-input AND gates, catalytic hairpin assembly, and hybridization chain reactions. Although the above work significantly improves computing speed, the polymers used typically require complex chemical synthesis and purification steps, and their effectiveness depends on specific experimental conditions, which limits their feasibility for large-scale applications.
2.3. DNA nanostructure-based DNA logic systems
2.3.1. DNA tetrahedrons. The flourishing development of DNA nanotechnology has sparked increasing interest in three-dimensional (3D) DNA nanostructures, such as DNA tetrahedra/polyhedra.64,65 These molecular self-assemblies can serve as innovative elements in DNA computing systems, enhancing the programmability and diversity of logic systems.66,67 Lin et al. introduced a molecular computing device based on a DNA tetrahedron for logic sensing of two miRNAs.68 The device utilized the DNA tetrahedron as a framework to combine all elements of the AND logic gate. Dual miRNA inputs (miR-21 and miR-122) were recognized via computation cascades activated by strand hybridization, removing a quencher and fluorophore duplex and producing fluorescent outputs. This design strategy avoided diffusive components in the solution and could be easily reconfigured to detect other endogenous nucleic acid inputs with potential therapeutic applications. To develop a multifunctional sensor, Pan et al. developed the coronavirus analysis system by constructing a dynamic network based on a tetrahedral framework (Fig. 3A).69 Some concatenated logic gates were fabricated, including AND–OR, INHIBIT–AND, AND–AND–AND, and AND–INHIBIT. The coronavirus biosensor showed different accuracy for COVID-19, Bat-SL-CoVZC45, and SARS-CoV analysis, with LODs of 2.5, 3.1, and 2.9 fM, respectively. The biosensing platform offers a flexible sensing approach for smart diagnosis of various coronaviruses with minimal false-negative rates. The modular assembly enables building multifunctional logic systems, but addressing challenges like limited and synthetic complexity is necessary to fully unlock biological computing's potential.
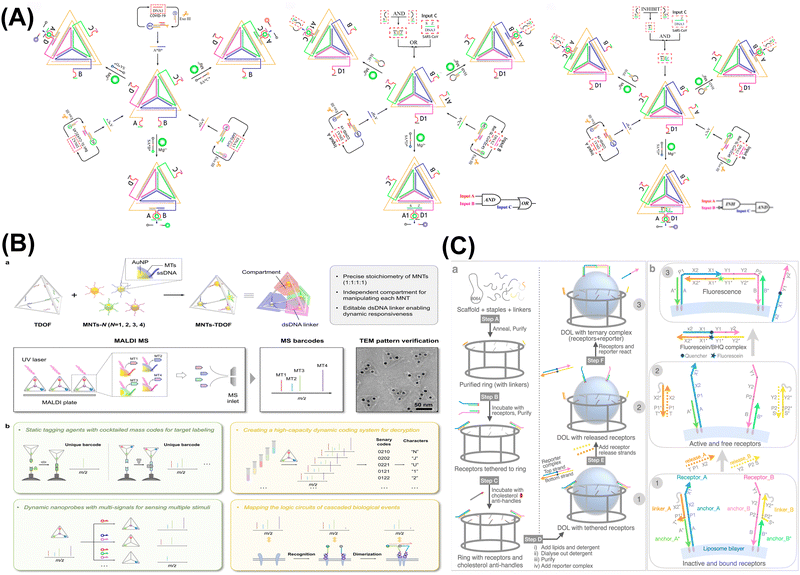 |
| Fig. 3 DNA nanostructure-based DNA logic systems. (A) Tetrahedron-based constitutional dynamic network. Reproduced with permission.69 Copyright 2022, American Chemical Society. (B) Compartmented DNA origami frames for precision information coding and logic mapping. Reproduced with permission.70 Copyright 2024, Wiley-VCH. (C) Three-dimensional digital nanoreactors to control absolute stoichiometry and spatiotemporal behavior of DNA receptors. Reproduced with permission.71 Copyright 2023, Springer Nature. | |
2.3.2. DNA origamis. In addition to DNA tetrahedra, DNA origamis are another type of artificially designed 3D self-assembled DNA nanostructures, enabling precise control over nanoscale structures for various applications in DNA computing.72,73 Li et al. developed a DNA origami triangular prism scaffold with a hairpin-swing arm core for the modular nanodevice, enabling the establishment of an orthogonal communication network through coding and decoding signal domains on the output strands.74 They demonstrated the implementation of AND, OR, and NOT logic response of input strands. The system connected multiple nanodevices into a functional platform capable of various functions like signal amplification, input registration, logical operations, and infectious disease modeling. By utilizing the repeatability of mass spectrometry (MS) and the spatial addressability of DNA origami, Zhang et al. proposed a quality management technique for establishing mass-coding nanodevices.70 This methodology involved embedding mass nanotags (MNTs) within separated tetrahedral DNA origami frames (TDOFs), enabling precise regulation of the distribution and measurement of four MNTs to produce characteristic MS signals (Fig. 3B). The programmability and orthogonality of composite systems have facilitated the development of MNTs-TDOFs into both static makers and dynamic nanoprobes for labeling and sensing multiple targets. Meanwhile, the high synthesis and preparation costs of DNA origami in large-scale applications or customized designs limit its widespread application in DNA logic systems.
2.3.3. Other nanodevices. Maingi et al. constructed digital nanoreactors capable of regulating coactions between lipid-bound molecular receptors over stoichiometric, spatial, and temporal (Fig. 3C).71 The reaction system utilized a DNA origami ring and served as a template for liposome synthesis while providing docking sites for DNA-conjugated receptors mimicking membrane proteins. The assembly of receptors into specific stoichiometries, such as 1
:
1 and 2
:
2 homodimers and heterodimers (which refer to the ratios of DNA strands involved in interactions and are crucial for determining the binding affinities and functional properties of DNA constructs), was facilitated through SDR and monitored using a DNA logic gate and fluorescence measurements. Understanding these stoichiometries advances the design of more efficient DNA logic systems with predictable behaviors and enhanced performance in various applications. This tethering allowed the determination of the native stoichiometry and kinetics of membrane protein complexes, advancing the understanding of signaling pathways and drug discovery. The approach provided a versatile platform for studying membrane protein behavior and interaction dynamics in a variety of ratios and contexts. Lv et al. developed a programmable DNA integrated circuit system by integration of multilayer DNA-based programmable gate arrays (DPGAs) for multifunctional logic computing.75 They utilized DNA origami to address issues like transient binding and signal attenuation, thereby overcoming limitations on circuit size and depth by controlling molecular interactions in solution. The integration of DNA origami registers was critical to achieving higher-order integration of DPGAs, providing directionality for asynchronous computational processing in cascaded DPGAs. This work introduced a highly organized and reconfigurable DNA computing platform, representing a significant advancement in the progress of general-purpose DNA computing.
3. Applications in biomedical diagnostics
Through the development and implementation of DNA logic systems, DNA computing-based technologies and platforms find applications in biomedical diagnosis.76,77 These include cell imaging, disease monitoring, biomarker analysis, drug therapy, and personalized healthcare.78–80 DNA computing in biomedical diagnosis offers advantages such as high sensitivity, specificity, and multiplexing ability, making it a promising approach for advancing diagnostic methodologies and improving patient care.81,82
3.1. DNA computing for cellular imaging
3.1.1. Protein biomarkers. To gain a deeper understanding of organisms, DNA computing has also been introduced into cells and tissues, enabling applications of logic cancer cellular imaging.26,83 DNA computing is employed for cellular analysis through its functionality on the cell membrane. Feng et al. constructed a ternary complex-based nanodevice that could perform AND logic operations on cell membranes, enabling in situ imaging analysis of dual-target proteins (Fig. 4A).84 The DNA logic gate triggered rolling circle amplification (RCA), performed an AND operation, and generated amplified “ON” fluorescence signals upon recognition of two coexisting membrane proteins (MUC1 and EpCAM). This nanomachine has achieved imaging analysis of two protein biomarkers in situ and subtype identification of target cells in intricate samples, demonstrating the preferable specificity and robustness of the system. Our group developed a ratiometric biosensor for the targeted detection of biomarkers (EpCAM and CEA) based on OR logic gate and HCR signal amplification.85 By analyzing 60 lung cancer patients and 15 healthy controls, we obtained a diagnostic sensitivity of 98.3% and specificity of 86.7% with an AUC of 0.973, effectively distinguishing between cancer and healthy groups. Additionally, the biosensor exhibited a diagnostic sensitivity of 93.3% in the early stage (stage I), which was superior to the conventional electrochemical detection method.
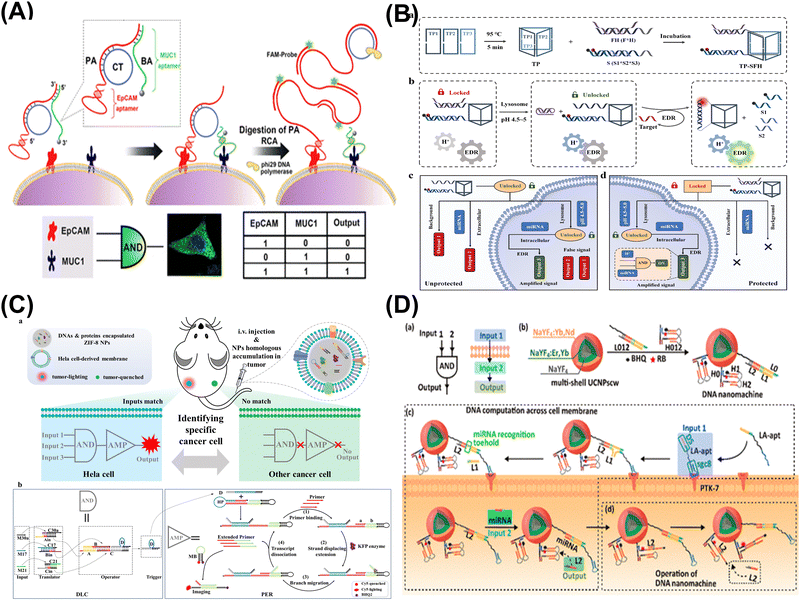 |
| Fig. 4 DNA computing for cellular imaging. (A) DNA logic nanomachine for imaging analysis of proteins on cell membranes. Reproduced with permission.84 Copyright 2020, American Chemical Society. (B) Intracellular activated logic nanomachines for detection of miRNAs in living cells. Reproduced with permission.86 Copyright 2023, Royal Society of Chemistry. (C) DNA logic circuit equipped with biomimetic ZIF-8 nanoparticles for the identification of specific cancers in vivo. Reproduced with permission.87 Copyright 2023, Wiley-VCH. (D) DNA nanomachine activated via computation across cancer cell membranes for therapy of solid tumors. Reproduced with permission.88 Copyright 2021, American Chemical Society. | |
3.1.2. miRNA biomarkers. After cellular uptake, the DNA logic gate further expands to detect intracellular biomarkers. Yang et al. integrated three recognition and reporting modules into a 3D logic gate nano-hexahedral framework, resulting in three “AND” logic gates for discriminating cancer cell subtypes using bispecific miRNA recognition (miR-21, miR-373, and miR-155).89 When each Boolean operator “AND” returned an “ON” signal, the system was not only able to distinguish cancer cells A549 (red fluorescence intensity: 14.18-fold higher) and MCF-7 (green fluorescence intensity: 21.82-fold higher; yellow fluorescence intensity: 101.69-fold higher; red fluorescence intensity: 25.53-fold higher) from normal cells, but also different types of cancer cells. Further, Li et al. developed intracellular triggered logic nanodevices based on DNA scaffolds for low background analysis of miRNAs within living cells (Fig. 4B).86 By combining transfer-sensor-computation-output functions, pH nanoswitches activated the nanomachines upon cell entry, and the nanoscale DNA triangular prism confined complex molecular biochemical reactions to a certain space, leading to increased sensitivity (762-fold) and reduced response time (6-fold) compared to diffuse-dominated logic circuits. In order to effectively transport DNA logic circuit components to the intended site in vivo, Wei et al. introduced “Primer Exchange Reaction” as the cascaded biological amplifier module (Fig. 4C).87 They used three upregulated HeLa cell miRNAs (miR-30a, miR-17, and miR-21) as the “AND” gate inputs and constructed biomimetic ZIF-8 nanoparticles as the carrier to cells. The logic circuits had a lower detection threshold of 0.68 pM for miRNAs compared to 0.2 nM of individual circuits, a loading capacity of greater than 90%, and a signal gain 3.1-fold higher than individual circuits. The system successfully distinguished the HeLa tumor subtypes from other tumors and identified MCF cancer cells at different stages in vivo.
3.1.3. Multi-biomarkers. DNA logic circuits hold promise for facilitating the precise identification of cancer cells, yet they encounter obstacles associated with suboptimal signal strength and a lack of effective therapeutic platforms.90 To address these issues, Zhang et al. developed a transmembrane DNA logic operations to prompt DNA nanomachines within cancer cells from the intricate solid tumor microenvironment (Fig. 4D).88 The DNA nanomachine, comprising multishell upconversion nanoparticles loaded with aptamers targeting protein tyrosine kinase-7 on the cancer cell membrane and intracellular miR-21, acted as two inputs for AND gate operation. The output DNA strands activated the photosensitizer Rose Bengal for active oxygen production. The system holds promise as a precise therapy strategy for cancer cells within complex solid tumor microenvironments. However, implementing DNA-based logic circuits can be complex and may require signal amplification methods, while ensuring stability and efficient delivery to the tumor microenvironment remains a challenge.In summary, DNA computing shows promise in cellular imaging, enabling intelligent analysis of cell membranes and intracellular biomarkers. Through logic computation, it not only facilitates precise analysis of different cell subtypes in clinical samples but can also be utilized for targeted therapy of tumors in vivo, providing more efficient and applicable strategies for future intelligent diagnosis and treatment.
3.2. DNA computing for clinical diagnosis
3.2.1. miRNA biomarkers. DNA arithmetic computing can accurately implement mathematical models at the molecular level and enable complex detection of diagnostic markers. The analysis of miRNAs based on DNA logic computing is beneficial for in vitro disease diagnosis, particularly for early cancer screening.91 Zhang et al. proposed a DNA molecular computing platform to analyze miRNA profiles in clinical serum samples for non-small cell lung cancer (NSCLC) diagnosis.92 They trained a computer aided classifier using miRNA-seq profiles, followed by DNA molecular computing implementation, as well as miRNA in situ amplification and transformation of circular DNA. With weight multiplication, summation and subtraction, a winner-take-all computational scheme was validated using clinical serum samples (8 healthy individuals and 14 NSCLC patients) with a sensitivity of 92.9%, specificity of 75.0% and overall accuracy of 86.4%. This DNA computing system provides the possibility for disease diagnosis, but there is still a need for optimizing clinical applications.
3.2.2. mRNA biomarkers. Paving the way for intelligent diagnostic devices, Ma et al. designed an automated system based on DNA computing, which includes sample processing, nucleic acid amplification, reaction implementation, and result analysis, for rapid etiological diagnostics (Fig. 5A).93 They trained an in silico classifier model using support-vector machines and designed a DNA-based molecular classifier. They reported the results on transcriptional signals in solution by performing mathematical calculations, such as weighting, summing, and subtraction. They automated the system's diagnostic process by integrating a mechanical sample loader, enabling the fully automated diagnosis of acute respiratory infection (ARI). With this approach, their diagnostic accuracy for classifying bacterial and viral ARI in 80 human blood samples within 4 hours was 87%, while the accuracy of commonly used clinical tests such as C-reactive protein testing and complete blood count ranged from 54% to 74%.
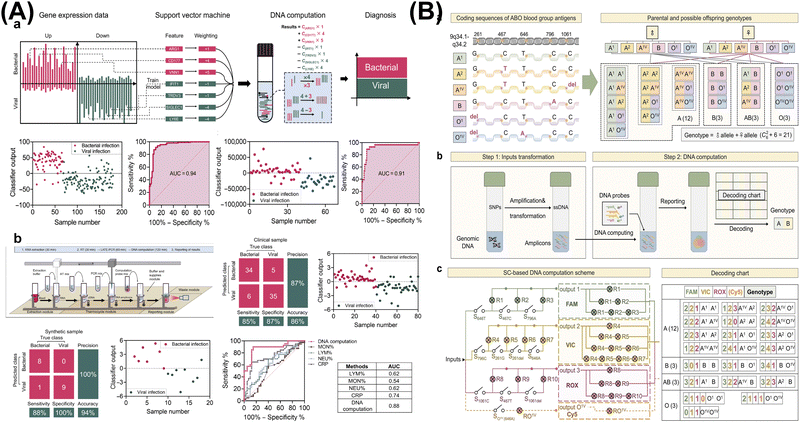 |
| Fig. 5 DNA computing for clinical diagnosis. (A) An automated DNA computing platform for rapid etiological diagnostics. Reproduced with permission.93 Copyright 2022, American Association for the Advancement of Science. (B) Programmable DNA molecular computation based logical analysis for clinical diagnostics. Reproduced with permission.94 Copyright 2022, Wiley-VCH. | |
3.2.3. Multi-biomarkers. The implementation of DNA molecular computing marks significant progress in molecular classification, but processing multiple types of molecular data remains a challenge. Addressing this hurdle, Yin et al. developed a DNA-encoded molecular classifier that could screen and analyze six biomarkers across 3D databases for prostate cancer (PCa) classification.95 They designed valence-encoded signal reporters based on a DNA tetrahedral framework (about 12 nm with 37 base pairs) to create programmable atom-like nanoparticles. These reporters, combined with a weighting system for each molecular input, facilitated substantial signal amplification of the target molecules for bioanalysis. The detection limits were estimated as 100
fM for miRNAs, 1
pM for mRNA, 0.05
ng mL−1 for prostate-specific antigen, and 10
nM for sarcosine. By analyzing 32 patients with PCa and 50 healthy controls, they accurately distinguished between the cancer groups and the healthy groups (P value
<
0.01), with an AUC of 100%, a specificity of 100%, and a sensitivity of 100%. The utilization of multidimensional molecular classifiers for the analysis of diverse molecular biomarkers illuminates the path towards precision diagnosis and therapy.
3.2.4. Multi-SNPs. In recent research, the integration of DNA computing with bioinformatics methods has been employed for biomarker screening and disease diagnosis. The molecular computing of DNA is effective in concurrently perceiving and analyzing intricate molecular data, and is therefore also used to analyze complex single nucleotide polymorphisms (SNPs) in the genome. Zhang et al. devised a switching circuit-based DNA computation strategy that combined multiplexed sensing and logic analysis to correlate detected single-nucleotide mutations (including insertions, deletions and SNPs) with corresponding phenotypic results for clinical diagnosis (Fig. 5B).94 Accurate identification of blood group genotypes is crucial for transfusion medicine and transplantation medicine. It can significantly reduce the risk of transfusion reactions and improve transplant success rates; otherwise, it may lead to serious health risks or even death. They accurately identified 21 distinct blood group genotypes from 83 clinical samples within 3 hours, achieving an accuracy rate of 100%. This study not only improves the speed and accuracy of detection, but also advances the application of single nucleotide mutation detection in clinical diagnostics. The potential of DNA computing can inspire numerous clinical applications, achieve economical, noninvasive, and precise disease diagnosis, and guide personalized medication.In total, DNA arithmetic computation enables precise execution of mathematical models at the molecular level, facilitating complex assay of diagnostic biomarkers. By intelligently designing systems to cascade different logic gates and generate corresponding computational outputs, more precise disease diagnosis and analysis can be achieved. This will inspire further clinical applications, thereby advancing intelligent biomedical diagnosis and treatment.
3.3. DNA computing for disease treatment
3.3.1. Precise therapy. Numerous studies have demonstrated that DNA logic gates or circuits can precisely recognize cancer cells and activate therapeutic responses, while remaining inactive in normal cells, thus ensuring highly selective treatment. Wang et al. developed a DNAzyme logic gate strategy to detect and process signals from high levels of miR-21 and H2O2, activating the system in cancer cells while remaining silent in normal cells (Fig. 6A).96 The metal–organic framework (MOF) enabled selective chemodynamic therapy (CDT), effectively killing cancer cells with minimal harm to normal cells. Mao et al. developed a DNA nanoreactor (iDNR) with an “AND” Boolean logic algorithm for simultaneous tumor imaging and precise photothermal therapy (Fig. 6B).97 Both the biomarker nucleolin and H2O2 in the tumor microenvironment (TME) enriched iDNR, which mediated PDA deposition. PDA converted near infrared signals into heat, enabling imaging and imaging-guided tumor ablation. In conclusion, the specific recognition and manipulation of cancer cells by DNA circuits enable high-precision treatment, targeting only cancer cells within the complex tumor microenvironment.
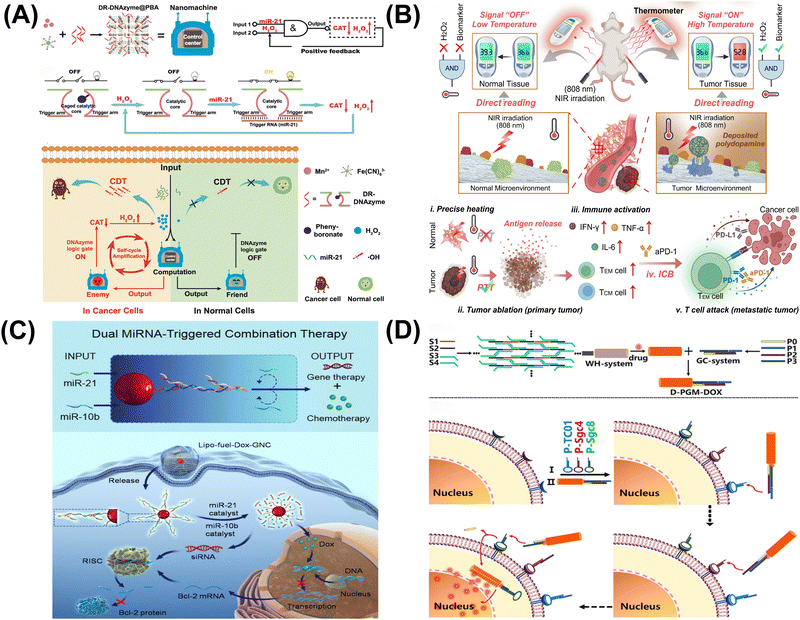 |
| Fig. 6 DNA computing for disease treatment. (A) DNAzyme logic-guided nanomachine for precise therapy.96 Copyright 2022, Wiley-VCH. (B) Intelligent DNA nanoreactor for tumor imaging and therapy.97 Copyright 2024, Wiley-VCH. (C) Dual microRNA logic-triggered drug release system.98 Copyright 2020, American Chemical Society. (D) Logic-guided missile-like DNA structure for targeted drug delivery.99 Copyright 2020, American Chemical Society. | |
3.3.2. Drug delivery. DNA computing is also widely used in drug delivery, which can effectively improve treatment efficacy and reduce side effects by using DNA logic gates to control the release of drugs in target tissues.100 Yue et al. developed a drug delivery system using AND logic gates, which allows for the catalytic release of doxorubicin (Dox) and small interfering RNA (siRNA) in cancerous cells specifically (Fig. 6C).98 Intracellular miR-21 and miR-10b acted as molecular triggers, catalyzing the nanocarrier's disassembly, which facilitated the release of Dox and the in situ generation of siRNA (siBcl-2). Ouyang et al. developed a DNA nanoscale precision-guided missile (D-PGM), for targeted anti-cancer drug delivery (Fig. 6D).99 It consists of a guidance/control (GC) and a warhead (WH). The GC, an aptamer-based logic circuit, recognizes target cells through sequential disassembly mediated by aptamers Sgc8, Sgc4f, and TC01. The WH carries a high payload of DOX drugs, which are precisely delivered to diseased cells, reducing systemic toxicity and overcoming multidrug resistance.In conclusion, DNA logic gates offer targeted cancer treatment by precisely recognizing and acting within cancer cells, reducing systemic toxicity and improving outcomes. However, their complexity and reliance on specific conditions present challenges to widespread application, though they show promise in overcoming multidrug resistance.
4. Conclusions
This review aims to summarize the latest developments in the field of DNA computing, including recent advances in constructing logic systems based on various materials and the progress made in intelligent biomedical diagnostic applications. However, DNA computing is still in the exploratory stage and faces several challenges: (1) DNA biochemical reactions can be readily constrained by implementation conditions, and as computational complexity increases, enhancing reaction efficiency becomes a crucial factor in determining computational accuracy. Factors such as temperature, pH, ionic strength, and reactant concentration can affect the efficiency and accuracy of DNA computing. (2) Most DNA logic platforms can only perform limited logic operations and address specific types of problems, hindering the widespread adoption of DNA computing due to the lack of universal computing systems. Current DNA logic platforms can only perform simple operations (like AND, OR, NOT) and cannot handle complex data processing or multi-step operations, limiting their potential to become general-purpose computing systems. (3) The majority of DNA computing systems are still in the concept validation or lab-scale stages, presenting significant challenges for normal operation in complex biological samples. Applying DNA computing in complex biological environments requires overcoming challenges related to scalability, stability, and integration with biological processes.
Therefore, key directions to address these challenges include finding novel DNA hybridization strategies, integrated nanomaterials, and designing multifunctional DNA nanostructures. Firstly, developing more robust DNA hybridization strategies and optimizing implementation conditions (such as temperature, ionic strength, and reactant concentration) to operate effectively in diverse and less controlled environments, while enhancing reaction efficiency and maintaining accuracy, is crucial for transitioning DNA computing from the laboratory to clinical applications. Secondly, it is necessary to design general-purpose computing systems capable of handling more complex and diverse tasks. In addition to new CRISPR–Cas editing tools, DNAzymes, and DNA barcoding technologies, integrating advanced nanomaterials (such as hydrogel, metal–organic frameworks, MXenes, two-dimensional transition metal dichalcogenides, and novel quantum dots) can increase the versatility of DNA logic gates.101,102 Finally, designing multifunctional DNA nanostructures, such as DNA nanorobots, DNA origami, and dynamic nanostructures, that can operate reliably, in combination with new DNA computers and molecular logic circuits, can pave the way for more powerful and versatile DNA computing systems in clinical settings.103,104 As these systems evolve, their enhanced analytical performance and diagnostic capabilities are expected to revolutionize precision medicine, providing more accurate and personalized diagnostic tools.
It is worth noting that the integration of artificial intelligence (AI) and machine learning (ML) with DNA computing can significantly optimize the design of DNA logic operations, improve data processing efficiency, and support personalized precision medical decisions.105 AI and ML can enhance the design of DNA logic operations by using predictive and optimization algorithms to achieve higher computational accuracy and efficiency. They also enable the rapid analysis and processing of complex biological data, extracting key biological information to accelerate data processing. Based on DNA computing results, AI and ML can assist in developing personalized treatment plans for more precise medical interventions.106,107 The integration of DNA computing with other emerging technologies, such as AI and ML, could further amplify its impact, enabling faster, more reliable, and more targeted precision medicine solutions, thereby significantly improving patient outcomes in the future.
Author contributions
Conceptualization: Jiayi Wang, Lin Huang, and Fanyu Meng; writing – original draft: Yuewei Zhao, Xvelian Li, and Yan Zhou; writing – review and editing: Jiayi Wang, Lin Huang, and Fanyu Meng; visualization: Xiaoting Tian and Yayou Miao.
Data availability
No primary research results, software or code have been included and no new data were generated or analysed as part of this review.
Conflicts of interest
There are no competing financial interests among the authors.
Acknowledgements
This work was supported by the National Natural Science Funds (Grant No. 82372148) and the National Key R&D Program of China (No. 2021YFF0703500 and 2022YFE0103500).
Notes and references
- L. M. Adleman, Science, 1994, 266, 1021–1024 CrossRef CAS.
- S. Yang, B. W. A. Bögels, F. Wang, C. Xu, H. Dou, S. Mann, C. Fan and T. F. A. de Greef, Nat. Rev. Chem., 2024, 8, 179–194 CrossRef.
- P. G. Moerman and R. Schulman, Nat. Nanotechnol., 2020, 15, 626–627 CrossRef CAS PubMed.
- A. Okamoto, K. Tanaka and I. Saito, J. Am. Chem. Soc., 2004, 126, 9458–9463 CrossRef CAS PubMed.
- F. Meng, W. Yu, C. Chen, S. Guo, X. Tian, Y. Miao, L. Ma, X. Zhang, Y. Yu, L. Huang, K. Qian and J. Wang, Small, 2022, 18, 2200784 CrossRef CAS PubMed.
- J. A. N. Brophy and C. A. Voigt, Nat. Methods, 2014, 11, 508–520 CrossRef CAS PubMed.
- L. Qian, E. Winfree and J. Bruck, Nature, 2011, 475, 368–372 CrossRef CAS PubMed.
- M. Manikandan, P. Nicolini and P. Hapala, ACS Nano, 2024, 18, 9969–9979 CrossRef CAS PubMed.
- J. Li, A. A. Green, H. Yan and C. Fan, Nat. Chem., 2017, 9, 1056–1067 CrossRef CAS.
- L. Huang, L. Wang, X. Hu, S. Chen, Y. Tao, H. Su, J. Yang, W. Xu, V. Vedarethinam, S. Wu, B. Liu, X. Wan, J. Lou, Q. Wang and K. Qian, Nat. Commun., 2020, 11, 3556 CrossRef CAS PubMed.
- Y. Wu, L. Zhang, S. Berretti and S. Wan, IEEE Trans. Ind. Inform., 2023, 19, 2089–2098 Search PubMed.
- L. Huang, Y. Zhou, Y. Zhu, H. Su, S. Yang, L. Feng, L. Zhao, S. Liu and K. Qian, Biosens. Bioelectron., 2022, 210, 114254 CrossRef CAS PubMed.
- D. Fan, J. Wang, E. Wang and S. Dong, Adv. Sci., 2020, 7, 2001766 CrossRef CAS.
- Q. Ma, C. Zhang, M. Zhang, D. Han and W. Tan, Small Struct., 2021, 2, 2100051 CrossRef CAS.
- W. Xu, J. Lin, M. Gao, Y. Chen, J. Cao, J. Pu, L. Huang, J. Zhao and K. Qian, Adv. Sci., 2020, 7, 2002021 CrossRef CAS.
- Y. Zhu, X. Xiong, M. Cao, L. Li, C. Fan and H. Pei, Sci. Adv., 2023, 9, eaax7983 CrossRef CAS PubMed.
- R. Wang, Z. Gu, Y. Wang, X. Yin, W. Liu, W. Chen, Y. Huang, J. Wu, S. Yang, L. Feng, L. Zhou, L. Li, W. Di, X. Pu, L. Huang and K. Qian, Adv. Funct. Mater., 2022, 32, 2206670 CrossRef CAS.
- F. Wang, H. Lv, Q. Li, J. Li, X. Zhang, J. Shi, L. Wang and C. Fan, Nat. Commun., 2020, 11, 121 CrossRef CAS PubMed.
- S. Piranej, A. Bazrafshan and K. Salaita, Nat. Nanotechnol., 2022, 17, 514–523 CrossRef CAS PubMed.
- Y. Zhang, N. Hu, J. Xu and Z. Wang, View, 2024, 5, 20230062 CrossRef CAS.
- M. Cao, X. Xiong, Y. Zhu, M. Xiao, L. Li and H. Pei, TrAC, Trends Anal. Chem., 2023, 159, 116911 CrossRef CAS.
- A. A. Green, J. Kim, D. Ma, P. A. Silver, J. J. Collins and P. Yin, Nature, 2017, 548, 117–121 CrossRef CAS.
- T. Kitada, B. DiAndreth, B. Teague and R. Weiss, Science, 2018, 359, eaad1067 CrossRef.
- Q. Liu, K. Yang, J. Xie and Y. Sun, IEEE Internet Things J., 2022, 9, 897–915 Search PubMed.
- L. Ceze, J. Nivala and K. Strauss, Nat. Rev. Genet., 2019, 20, 456–466 CrossRef CAS.
- F. Yin, F. Wang, C. Fan, X. Zuo and Q. Li, View, 2021, 2, 20200038 CrossRef CAS.
- X. Yin, D. Yao, M. H. W. Lam and H. Liang, J. Mater. Chem. B, 2022, 10, 3055–3063 RSC.
- H. Yan, G. Cao, J. Wang, X. Zhu, S. Dong, Y. Huang, M. Chao, Y. Li, F. Gao and L. Hua, Biosens. Bioelectron., 2024, 256, 116278 CrossRef CAS.
- X. Chang, C. Zhang, C. Lv, Y. Sun, M. Zhang, Y. Zhao, L. Yang, D. Han and W. Tan, J. Am. Chem. Soc., 2019, 141, 12738–12743 CrossRef CAS.
- M. Zhang, C. Yancey, C. Zhang, J. Wang, Q. Ma, L. Yang, R. Schulman, D. Han and W. Tan, Sci. Adv., 2024, 10, eadn3329 CrossRef CAS.
- Y. Liu, Y. Zhai, H. Hu, Y. Liao, H. Liu, X. Liu, J. He, L. Wang, H. Wang, L. Li, X. Zhou and X. Xiao, Adv. Sci., 2024, 11, 2400011 CrossRef CAS PubMed.
- K. N. Lin, K. Volkel, C. Cao, P. W. Hook, R. E. Polak, A. S. Clark, A. San Miguel, W. Timp, J. M. Tuck, O. D. Velev and A. J. Keung, Nat. Nanotechnol., 2024, 1–11 Search PubMed.
- N. Diao, J. Hou, X. Peng, Y. Wang, A. He, H. Gao, L. Yang, P. Guo, J. Wang and D. Han, Angew. Chem. Int. Ed., 2024, e202406330 Search PubMed.
- B. Wang, S. S. Wang, C. Chalk, A. D. Ellington and D. Soloveichik, Proc. Natl. Acad. Sci. U. S. A., 2023, 120, e2217330120 CrossRef CAS PubMed.
- N. Chen, J. Yu, Z. Liu, L. Meng, X. Li and K. C. Wong, Nucleic Acids Res., 2024, 52, 4137–4150 CrossRef PubMed.
- D. Y. Zhang and G. Seelig, Nat. Chem., 2011, 3, 103–113 CrossRef CAS PubMed.
- Y. Zhang, Y. Chen, X. Liu, Q. Ling, R. Wu, J. Yang and C. Zhang, ACS Nano, 2024, 18, 5089–5100 CrossRef CAS.
- P. Miao and Y. Tang, ACS Cent. Sci., 2021, 7, 1036–1044 CrossRef CAS.
- Q. Chai, J. Chen, S. Zeng, T. Zhu, J. Chen, C. Qi, G. Mao and Y. Liu, Small, 2023, 19, 2207736 CrossRef CAS.
- Z. Wang, Y. Hu and L. Pan, Angew. Chem. Int. Ed., 2020, 59, 14979–14985 CrossRef CAS PubMed.
- A. P. Lapteva, N. Sarraf and L. Qian, J. Am. Chem. Soc., 2022, 144, 12443–12449 CrossRef CAS.
- G. Shi, C. Yan and J. Chen, Anal. Chem., 2021, 93, 3273–3279 CrossRef CAS.
- T. Wang and F. C. Simmel, Angew. Chem. Int. Ed., 2023, 62, e202302858 CrossRef CAS PubMed.
- M. Hu, X. Li, J. Wu, M. Yang and T. Wu, ACS Nano, 2024, 18, 2184–2194 CrossRef CAS.
- W. J. Zeng, X. R. Li, W. Liu, R. Yuan, W. B. Liang and Y. Zhuo, Anal. Chem., 2024, 96, 2117–2123 CrossRef CAS.
- Z. Li, M. Yang, Y. Shu and J. Wang, Sens. Actuators, B, 2023, 391, 134063 CrossRef CAS.
- M. Li, B. Yao, C. Jing, H. Chen, Y. Zhang and N. Zhou, Anal. Chim. Acta, 2022, 1198, 339559 CrossRef CAS.
- J. Wang, J. Han, J. Wang, X. Lv, D. Fan and S. Dong, Biosens. Bioelectron., 2024, 244, 115801 CrossRef CAS PubMed.
- W. Y. Lv, C. H. Li, F. F. Yang, Y. F. Li, S. J. Zhen and C. Z. Huang, Angew. Chem. Int. Ed., 2022, 61, e202115561 CrossRef CAS PubMed.
- E. Bethur, R. Guha, Z. Zhao, B. B. Katz, P. D. Ashby, H. Zeng and S. M. Copp, ACS Nano, 2024, 18, 3002–3010 CrossRef CAS PubMed.
- R. Gao, X.-S. Wei, Z. Chen, A. Xie and W. Dong, ACS Nano, 2023, 17, 18055–18061 CrossRef CAS PubMed.
- J. Yu, Q. Liu, L. Qi, Q. Fang, X. Shang, X. Zhang and Y. Du, Biosens. Bioelectron., 2024, 252, 116137 CrossRef CAS PubMed.
- J. Han, X. Lv, Y. Zhang, J. Wang, D. Fan and S. Dong, Anal. Chem., 2023, 95, 16725–16732 CrossRef CAS.
- H. Geng, Z. Yin, C. Zhou and C. Guo, Acta Biomater., 2020, 118, 44–53 CrossRef CAS PubMed.
- Y. Pei, T. Bian, Y. Liu, Y. Liu, Y. Xie and J. Song, Nano Lett., 2022, 22, 3003–3010 CrossRef CAS PubMed.
- M. Lv, W. Zhou, D. Fan, Y. Guo, X. Zhu, J. Ren and E. Wang, Adv. Mater., 2020, 32, 1908480 CrossRef CAS.
- K. He, H. Yang, L. Wang, J. Guan, M. Wu, H. He, S. Gunasekaran, X. Wang, Q. Wang and X. Xu, Chem. Eng. J., 2019, 368, 877–887 CrossRef CAS.
- S. Su, H. Sun, W. Cao, J. Chao, H. Peng, X. Zuo, L. Yuwen, C. Fan and L. Wang, ACS Appl. Mater. Interfaces, 2016, 8, 6826–6833 CrossRef CAS.
- Z. Q. Bu, Q. F. Yao, Q. Y. Liu, M. X. Quan, J. Y. Lu and W. T. Huang, ACS Appl. Mater. Interfaces, 2022, 14, 8311–8321 CrossRef CAS PubMed.
- N. M. Figueiredo, I. V. Voroshylova, E. S. C. Ferreira, J. M. C. Marques and M. N. D. S. Cordeiro, Chem. Rev., 2024, 124, 3392–3415 CrossRef CAS.
- N. Xie, M. Li, Y. Wang, H. Lv, J. Shi, J. Li, Q. Li, F. Wang and C. Fan, J. Am. Chem. Soc., 2022, 144, 9479–9488 CrossRef CAS PubMed.
- D. Fan, E. Wang and S. Dong, ACS Appl. Mater. Interfaces, 2017, 9, 1322–1330 CrossRef CAS PubMed.
- W. Engelen, S. P. W. Wijnands and M. Merkx, J. Am. Chem. Soc., 2018, 140, 9758–9767 CrossRef CAS PubMed.
- A. Phillips, Nature, 2024, 625, 454–455 CrossRef CAS PubMed.
- B. Yang, D. Guneri, H. Yu, E. P. Wright, W. Chen, Z. A. E. Waller and Y. Ding, Nucleic Acids Res., 2024, 52, 2188–2197 CrossRef.
- L. Zhang, X. Ma, G. Wang, X. Liang, H. Mitomo, A. Pike, A. Houlton and K. Ijiro, Nano Today, 2021, 39, 101154 CrossRef CAS.
- X. Xiong, T. Zhu, Y. Zhu, M. Cao, J. Xiao, L. Li, F. Wang, C. Fan and H. Pei, Nat. Mach. Intell., 2022, 4, 625–635 CrossRef.
- Q. Lin, A. Wang, S. Liu, J. Li, J. Wang, K. Quan, X. Yang, J. Huang and K. Wang, Chem. Commun., 2020, 56, 5303–5306 RSC.
- J. Pan, Y. He, Z. Liu and J. Chen, Anal. Chem., 2022, 94, 714–722 CrossRef CAS PubMed.
- X. Zhang, Y. Dong, Y. Wang, Z. Zhang, X. Zhang, J. J. Zhu, Y. Tian and Q. Min, Angew. Chem. Int. Ed., 2024, 63, e202313446 CrossRef CAS PubMed.
- V. Maingi, Z. Zhang, C. Thachuk, N. Sarraf, E. R. Chapman and P. W. K. Rothemund, Nat. Commun., 2023, 14, 1532 CrossRef CAS.
- S. Dey, C. Fan, K. V. Gothelf, J. Li, C. Lin, L. Liu, N. Liu, M. A. D. Nijenhuis, B. Saccà, F. C. Simmel, H. Yan and P. Zhan, Nat. Rev. Methods Primers, 2021, 1, 1–24 CrossRef.
- M. DeLuca, D. Duke, T. Ye, M. Poirier, Y. Ke, C. Castro and G. Arya, Nat. Commun., 2024, 15, 3015 CrossRef CAS.
- H. D. Li, P. Q. Ma, J. Y. Wang, B. C. Yin and B. C. Ye, Small, 2023, 19, 2302301 CrossRef CAS.
- H. Lv, N. Xie, M. Li, M. Dong, C. Sun, Q. Zhang, L. Zhao, J. Li, X. Zuo, H. Chen, F. Wang and C. Fan, Nature, 2023, 622, 292–300 CrossRef CAS PubMed.
- J. J. Tabor and A. D. Ellington, Nat. Biotechnol., 2003, 21, 1013–1015 CrossRef CAS.
- T. Yuan, L. Wu, S. Li, J. Zheng, N. Li, X. Xiao, H. Zhang, T. Fei, L. Xie, Z. Zuo, D. Li, P. Huang, H. Feng, Y. Cao, N. Yan, X. Wei, L. Shi, Y. Sun, W. Wei, Y. Sun and E. Zuo, Cell Discovery, 2024, 10, 1–19 Search PubMed.
- F. Meng, H. Chai, X. Ma, Y. Tang and P. Miao, J. Mater. Chem. B, 2019, 7, 1926–1932 RSC.
- W. Chen, H. Yu, Y. Hao, W. Liu, R. Wang, Y. Huang, J. Wu, L. Feng, Y. Guan, L. Huang and K. Qian, ACS Nano, 2023, 17, 19779–19792 CrossRef CAS PubMed.
- X. Li, W. Chen, M. Wu, W. Yu, M. Wang, M. Niu, F. Meng, Y. Zhao, A. Osman, N. O. Mousa, H. Shi, K. Qian, J. Wang and L. Huang, Chem. Eng. J., 2024, 486, 150224 CrossRef CAS.
- J. Yu, T. Han, X. Lin, X. Song, H. Xie, J. He and W. Chen, J. Clin. Oncol., 2019, 37, e13051 Search PubMed.
- C. Company, M. J. Schmitt, Y. Dramaretska, M. Serresi, S. Kertalli, B. Jiang, J. A. Yin, A. Aguzzi, I. Barozzi and G. Gargiulo, Nat. Commun., 2024, 15, 897 CrossRef CAS PubMed.
- Y. Wang, S. Wang, L. Li, Y. Zou, B. Liu and X. Fang, View, 2023, 4, 20220048 CrossRef.
- C. Feng, T. Chen, D. Mao, F. Zhang, B. Tian and X. Zhu, ACS Sens., 2020, 5, 3116–3123 CrossRef CAS.
- F. Meng, W. Yu, M. Niu, X. Tian, Y. Miao, X. Li, Y. Zhou, L. Ma, X. Zhang, K. Qian, Y. Yu, J. Wang and L. Huang, J. Nanobiotechnol., 2023, 21, 104 CrossRef CAS PubMed.
- X. Q. Li, Y. L. Jia, Y. W. Zhang, H. Y. Chen and J. J. Xu, Chem. Sci., 2023, 14, 7699–7708 RSC.
- K. Wei, M. He, J. Zhang, C. Zhao, C. Nie, T. Zhang, Y. Liu, T. Chen, J. Jiang and X. Chu, Angew. Chem. Int. Ed., 2023, 62, e202307025 CrossRef CAS PubMed.
- Y. Zhang, W. Chen, Y. Fang, X. Zhang, Y. Liu and H. Ju, J. Am. Chem. Soc., 2021, 143, 15233–15242 CrossRef CAS.
- Q. Yang, F. Yang, W. Dai, X. Meng, W. Wei, Y. Cheng, J. Dong, H. Lu and H. Dong, Adv. Healthcare Mater., 2021, 10, 2101130 CrossRef CAS.
- J. Luo, B. Wang, X. Tang, P. Huang, S. Yang, S. Zhao, S. Xie, Q. Li, K. Chang and M. Chen, View, 2024, 5, 20230086 CrossRef CAS.
- J. Liu, C. Zhang, J. Song, Q. Zhang, R. Zhang, M. Zhang, D. Han and W. Tan, Adv. Sci., 2023, 10, 2206343 CrossRef CAS.
- C. Zhang, Y. Zhao, X. Xu, R. Xu, H. Li, X. Teng, Y. Du, Y. Miao, H. Lin and D. Han, Nat. Nanotechnol., 2020, 15, 709–715 CrossRef CAS PubMed.
- Q. Ma, M. Zhang, C. Zhang, X. Teng, L. Yang, Y. Tian, J. Wang, D. Han and W. Tan, Sci. Adv., 2022, 8, eade0453 CrossRef CAS PubMed.
- C. Zhang, T. Zheng, Q. Ma, L. Yang, M. Zhang, J. Wang, X. Teng, Y. Miao, H. Lin, Y. Yang and D. Han, Angew. Chem. Int. Ed., 2022, 61, e202117658 CrossRef CAS.
- F. Yin, H. Zhao, S. Lu, J. Shen, M. Li, X. Mao, F. Li, J. Shi, J. Li, B. Dong, W. Xue, X. Zuo, X. Yang and C. Fan, Nat. Nanotechnol., 2023, 18, 677–686 CrossRef CAS.
- Z. Wang, J. Yang, G. Qin, C. Zhao, J. Ren and X. Qu, Angew. Chem. Int. Ed., 2022, 61, e202204291 CrossRef CAS PubMed.
- D. Mao, Z. Dong, X. Liu, W. Li, H. Li, C. Gu, G. Chen, X. Zhu and Y. Yang, Angew. Chem. Int. Ed., 2024, 63, e202311309 CrossRef CAS PubMed.
- R. Yue, M. Chen and N. Ma, ACS Appl. Mater. Interfaces, 2020, 12, 32493–32502 CrossRef CAS PubMed.
- C. Ouyang, S. Zhang, C. Xue, X. Yu, H. Xu, Z. Wang, Y. Lu and Z. S. Wu, J. Am. Chem. Soc., 2020, 142, 1265–1277 CrossRef CAS PubMed.
- C. Li, M. Wang, P.-F. Li, J. Sheng and Q. Fu, Small, 2024, 20, e2306257 CrossRef PubMed.
- R. Fu, J. Hou, Z. Wang and Y. Xianyu, ACS Nano, 2024, 18, 14754–14763 CrossRef CAS PubMed.
- J. Chen, J. Hu and R. Kapral, Adv. Sci., 2024, 11, 2305695 CrossRef CAS.
- L. Yang, Q. Tang, M. Zhang, Y. Tian, X. Chen, R. Xu, Q. Ma, P. Guo, C. Zhang and D. Han, Nat. Commun., 2024, 15, 4583 CrossRef CAS PubMed.
- L. Yang, Y. Zhao, X. Xu, K. Xu, M. Zhang, K. Huang, H. Kang, H.-C. Lin, Y. Yang and D. Han, Angew. Chem., Int. Ed., 2020, 59, 17697–17704 CrossRef CAS PubMed.
- A. Pucchio, E. A. Eisenhauer and F. Y. Moraes, Nat. Biotechnol., 2021, 39, 388–389 CrossRef CAS.
- D. A. Winkler, Small, 2020, 16, e2001883 CrossRef PubMed.
- B. Babic, S. Gerke, T. Evgeniou and I. G. Cohen, Nat. Mach. Intell., 2021, 3, 283–287 CrossRef.
|
This journal is © The Royal Society of Chemistry 2024 |