DOI:
10.1039/D4FD00069B
(Paper)
Faraday Discuss., 2024,
252, 262-278
Computation-guided engineering of distal mutations in an artificial enzyme†
Received
28th March 2024
, Accepted 22nd April 2024
First published on 22nd April 2024
Abstract
Artificial enzymes are valuable biocatalysts able to perform new-to-nature transformations with the precision and (enantio-)selectivity of natural enzymes. Although they are highly engineered biocatalysts, they often cannot reach catalytic rates akin those of their natural counterparts, slowing down their application in real-world industrial processes. Typically, their designs only optimise the chemistry inside the active site, while overlooking the role of protein dynamics on catalysis. In this work, we show how the catalytic performance of an already engineered artificial enzyme can be further improved by distal mutations that affect the conformational equilibrium of the protein. To this end, we subjected a specialised artificial enzyme based on the lactococcal multidrug resistance regulator (LmrR) to an innovative algorithm that quickly inspects the whole protein sequence space for hotpots which affect the protein dynamics. From an initial predicted selection of 73 variants, two variants with mutations distant by more than 11 Å from the catalytic pAF residue showed increased catalytic activity towards the new-to-nature hydrazone formation reaction. Their recombination displayed a 66% higher turnover number and 14 °C higher thermostability. Microsecond time scale molecular dynamics simulations evidenced a shift in the distribution of productive enzyme conformations, which are the result of a cascade of interactions initiated by the introduced mutations.
Introduction
Biocatalysis is playing an invaluable role in the transition towards a more sustainable chemistry,1–4 as it combines mild reaction conditions (e.g. no need for polluting solvents, neutral pH, mesophilic temperatures) with exceptional stereo- and chemo-selectivity and unrivalled rate acceleration. However, the catalytic scope of enzymes is limited by the narrow set of amino acids naturally available, which allow only a finite number of catalytic reactivities. Often, the manufacture of fine-chemicals requires specific types of transformations that are not naturally occurring.5 In order to leverage the vast benefits of enzymes in these processes, artificial enzymes have been designed to generate new-to-nature catalytic reactivities.6–8 Artificial enzymes can be generated by introducing an abiotic catalytic component in natural proteins, giving rise to rudimentary enzymes that can then be subjected to the power of directed evolution to achieve the high activities and selectivities typically associated with enzyme catalysis.9,10 However, despite significant progress, the catalytic efficiency of most artificial enzymes is not yet comparable to that of natural enzymes.11 To date, most directed evolution campaigns using artificial enzymes have only targeted the residues in the immediate proximity of the catalytic centre to directly affect its chemical environment. It has become increasingly clear that, just as is the case with natural enzymes,12 structural cooperation of the whole protein is also required for efficient catalysis with artificial enzymes. For instance, Lewis and co-workers observed increased enantioselectivity in a model cyclopropanation reaction catalysed by an artificial rhodium enzyme upon introduction of mutations distant from the active site.13 Often, the introduction of distal mutations generates subtle structural rearrangements determined by the protein’s innate structural dynamics, which has been fine-tuned over aeons of evolution in natural enzymes.14,15 Those residues that can indirectly influence the catalytic activity by modulating structural dynamics are called distal sites or hotspots of dynamics.16,17 Targeting these hotspots in the directed evolution algorithm can shift the conformation dynamics towards catalytically productive conformations, resulting in a highly efficient designer enzyme.18,19 A topical example is the case of the Kemp eliminase HG3.17 designed by Hilvert et al. which was able to reach a 108-fold improvement in rate acceleration by developing catalytically competent conformation ensembles at the expense of inactive ones.20 Currently, their identification often relies on extensive molecular dynamics (MD) simulations, which poses significant limitations on the throughput of the workflow.21 Although new machine-learning based strategies have recently been described and hold the promise of greatly easing the computational expense,22 the need for large training datasets hinders their application in lesser-known systems.
In order to identify the role of distal mutations and long-range networks in an artificial enzyme, we took the lactococcal multidrug resistance regulator (LmrR)23,24 as example of a promiscuous protein scaffold tailored to a wide range of new-to-nature reactivities. This protein belongs to the PadR family of transcription factors and regulates the expression of the lmr operon in Lactococcus lactis. LmrR is characterised by a unique conformational flexibility and structural plasticity25,26 that gives rise to a broad ligand promiscuity within its large hydrophobic pore. Introducing various artificial catalytic moieties, either metal complexes,27 non-canonical amino acids (ncAA),28 or even both,29 endowed LmrR with several new-to-nature catalytic reactivities. These rudimentary enzymes were then subjected to directed evolution, resulting in specialist enzymes that showed significantly increased activities and (enantio-)selectivities. To date, however, directed evolution was focused exclusively on the residues within the pore to optimise the structure of the newly created active site. Here, we show how distal mutations can further increase the activity of one of these designer enzymes by leveraging LmrR's conformation dynamics.
For this purpose, we focused on an LmrR-based designer enzyme that uses the genetically encoded ncAA p-aminophenylalanine (pAF), introduced via stop-codon suppression,30 as catalytic residue. The variant with pAF at position V15, LmrR_V15pAF, was shown to catalyse the hydrazone formation reaction.31 In this reaction, the pAF residue activates an aldehyde by forming an iminium ion intermediate that is, then, subjected to nucleophilic attack by a hydrazine. Directed evolution focused on the pore32 generated the triple mutant A92R_N19M_F93H (LmrR_RMH or parent hereafter), which showed a 90-fold improvement in turnover number (kcat). This was a remarkable result, but it still falls short compared to the activities typical for natural enzymes.
Therefore, we decided to explore mutations at distal sites to further improve activity, by identifying and mutating important residues that modulate structural dynamics and, consequently, affect catalysis. Typically, this would entail error prone PCR methods for the random introduction of mutations in the protein,33 followed by screening for hydrazone formation activity. But even though this is conveniently done by monitoring colour formation in a 96-well format, the sequence space that can be explored is limited by the moderate throughput of this assay. Hence, we decided on computationally exploring and identifying distal positions that affect conformational dynamics and predicting suitable mutations and, in this way, generating a focused library of LmrR_RMH mutants. For this, we employed a novel algorithm that does not employ molecular dynamics simulations in its pipeline, making it a suitable high-throughput distal mutation predictive tool.
Results and discussion
Identification of improved variants
In silico prediction of distal mutations.
Our strategy to predict distal mutations on the LmrR_RMH scaffold involved the use of Zymevolver, an enzyme engineering software developed at Zymvol.34 In particular, we used its utility to depict distal hotspots, known as Zymspot. This algorithm uses bioinformatics and structure-based approaches to depict distal mutations with the potential to perturb the enzyme's conformational dynamics, ultimately impacting the population of catalytically productive conformations. In the past, Zymevolver and its utility Zymspot were successfully applied to improve the activity, stability and/or operational stability of various enzymes.35–38 In this study, Zymspot was used to identify hotspots of dynamics in the artificial enzyme LmrR_RMH that could in turn be used as targets to improve the new-to-nature catalytic condensation of 4-hydroxybenzaldehyde (4-HBA) and 4-hydrazino-7-nitro-2,1,3-benzoxadiazole hydrazine (NBD-H) into a chromogenic hydrazone (Fig. 1C).
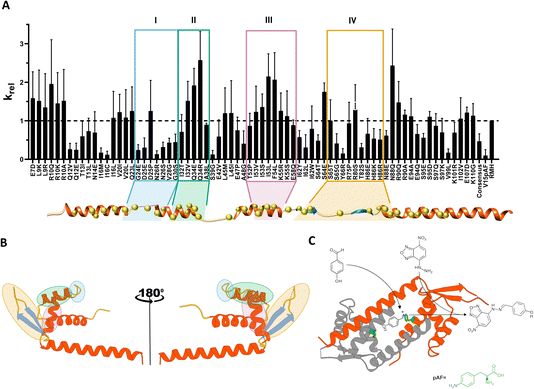 |
| Fig. 1 (A) Relative activity profile observed for the predicted mutants in cell lysate screening. The screening was performed with 50 μM NBD-H, 5 mM 4-HBA in buffer R, pH 7.5, 5% DMF at 25 °C. The error bars represent the standard deviation of three independent measurements. Four groups of residues (clusters I, II, III and IV) with similar behaviour are marked in different colours (cyan, green, magenta, yellow) and illustrated over the LmrR secondary structure. Yellow spheres represent the positions selected for mutagenesis. (B) The four clusters identified are represented over the LmrR tertiary structure. (C) Hydrazone formation reaction scheme between 4-HBA and NBD-H. The iminium ion intermediate is represented bound to the catalytic ncAA pAF (green). | |
As a result, 49 distal hotspots (42% of the protein) were identified as positions able to modulate the conformational dynamics in LmrR. Notably, this comprises almost half of the protein, which is significantly different from observations on other protein families (10–20% of the protein; data not published). This fact indicates that LmrR's conformational dynamics is very susceptible to changes at many points of its structure. In order to reduce the possibility of incorporating harmful mutations, leading to unexpressed or insoluble protein, one to three mutations were selected based on the most represented residues at those specific positions found in a multisequence alignment (MSA) of the wild-type (Fig. S1†) LmrR. This resulted in a library of 96 variants (Table S1†). Additionally, 6 consensus mutations, i.e. variants with significantly higher representation in the MSA at a specific position in LmrR, were added to this list, resulting in a final list of 102 suggested mutants. Some of these were already explored in previous directed evolution studies32 and therefore excluded. Thus, 92 variants were then targeted experimentally.
Experimental verification of the distal mutations.
Out of the 92 variants suggested from the computational experiment, 73 were expressed successfully and the activity profile of these was assessed in a cell lysate screening (see ESI†). The results showed a diverse landscape based on the impact that the distal mutations showed in the activity of the enzyme (Fig. 1A and B). At least four clusters of residues that differently impact the catalytic activity could be identified: the clusters I and IV, comprising the residues 24–30, and 65–86, respectively, showed a negative effect on catalysis, whereas the clusters II and III, comprising the residues 32–38 and 52–58, respectively, showed a beneficial effect.
Based on the screening performed on cell lysates, six beneficial mutations, i.e. R10Q, Q34R, I53L, F54L, S64E, N88Q, were selected for further study. Additionally, three neutral (N14E, E47P, I62W) and one detrimental (Q12V) variants were selected.
The screening of catalytic activity of the purified enzymes (Fig. S2†) identified the mutations F54L, I62W and N88Q as the most beneficial, giving rise to an improvement in catalytic efficiency (kcat/KM) of up to 1.6-fold over the parent LmrR_RMH. While F54L was identified as a consensus mutation, I62W and N88Q resulted from Zymspot. Interestingly, these mutations are located 12.3 Å, 12.6 Å and 11.3 Å (from Cα to Cα), respectively, away from the catalytic pAF residue. The mutations R10Q, Q34R, E47P, I53L and S64E did not show any significant improvement compared to LmrR_RMH, however, their protein expression yield was significantly higher than that of the parent, thereby explaining the increased activity observed in the cell lysate screening. Lastly, the mutations Q12V and N14E had a detrimental effect on the hydrazone formation reaction, showing about 25% and 50%, respectively, of the reaction rate of the parent.
Aiming to further explore the positions F54 and N88, these were subjected to partial site saturation mutagenesis (Fig. S3†). The results of cell lysate screening showed that the conversion of F54 into a charged (Asp, Lys) or polar (Asn, Gln, Thr, Ser) amino acid leads to loss of solubility, presumably due to misfolding of the protein. In contrast, all the explored hydrophobic residues (Ala, Val, Ile, Leu, Met) at the same position showed a detectable catalytic activity, indicating the presence of a correctly folded and soluble protein. However, none of these outperformed the mutant F54L. On the other hand, the saturation of the position N88 showed a more diverse catalytic profile. Hydrophobic residues appeared to have an overall negative impact on the catalytic performance, except for the mutation N88A, which gave a mild improvement compared to LmrR_RMH. Positively charged amino acids (Arg, Lys) were shown to be detrimental for catalysing the hydrazone formation reaction, whereas negatively charged amino acids (Asp, Glu) showed mostly neutral effects. Again, the initial N88Q mutation proved to be the best-performing.
Additionally, since both N88 and I62 belong to a “hinge” region of the enzyme,39 connecting the DNA-binding site (DBS) with the hydrophobic pore, mutations in this area may affect the geometry of the active site and consequently, the catalytic activity. Therefore, we also screened a combinatorial library of mutants of positions I62 and N88. Unfortunately, no combination of mutations gave rise to a significant improvement in the catalytic activity (Table S2 and Fig. S2†).
Altogether, F54L, I62W and N88Q were the most beneficial hits found and were selected for kinetic characterization.
Characterization of the improved mutants
Kinetic characterization of F54L, I62W and N88Q mutations.
Apparent Michaelis–Menten parameters were determined for the three improved mutants and compared to the parent LmrR_RMH (Table 1 and Fig. S4†). LmrR_RMH_F54L displayed a modest increase in the kcat which resulted in about 20% higher enzyme efficiency ((kcat/KM)app) compared to the parent. N88Q showed a similarly modest increase in apparent kcat, together with a larger reduction in the apparant Michaelis constant (KM) for NBD-H (30% lower) which yielded an overall catalytic efficiency about 1.6-times higher than LmrR_RMH and 119-times higher than the original artificial enzyme LmrR_V15pAF. LmrR_RMH_I62W displayed the highest improvement in kcat but also a 1.5-times higher KM for NBD-H, which led to a modest 20% improvement in catalytic efficiency.
Table 1 Apparent kinetic parameters of the three best performing single mutants and the recombination of F54L and N88Q (LmrR_RMH_L-Q). Each data point was measured in duplicate with biological replicates, for a total of four measurements. The conditions used were 50 μM NBD-H, 5 mM 4-HBA, 2.5 μM of buffer R, pH 7.5, 5% DMF, 25 °C. The standard deviation of KM and kcat is reported in parentheses
Variant |
K
M,app [μM] |
k
cat,app × 10−3 [s−1] |
(kcat/KM)app [M−1 s−1] |
(kcat/KM)appvs. LmrR_RMHa |
(kcat/KM)appvs. LmrR_V15pAFa,b |
The comparisons with LmrR_RMH and LmrR_V15pAF were made based on newly measured values.
The newly measured activity for LmrR_V15pAF was 10-fold higher from what was originally reported.32 This variation is mostly due to the relatively low-rate acceleration achieved by the non-engineered LmrR_V15pAF, which introduces a higher error rate during measurement.
|
LmrR_RMH_F54L |
48.7 (±9.5) |
6.35 (±0.56) |
129 |
1.2 |
87 |
LmrR_RMH_I62W |
71.6 (±9.4) |
9.68 (±0.68) |
135 |
1.2 |
91 |
LmrR_RMH_N88Q |
35.3 (±6.5) |
6.20 (±0.46) |
176 |
1.6 |
119 |
LmrR_RMH_L-Q |
48.6 (±8.0) |
8.52 (±0.63) |
174 |
1.6 |
117 |
To investigate the possibility of a synergistic effect, rational combinations of the three beneficial mutations were generated, yielding the double mutants LmrR_RMH_LW (LmrR_RMH_F54L_I62W), LmrR_RMH_WQ (LmrR_RMH_I62W_N88Q), and LmrR_RMH_L-Q (LmrR_RMH_F54L_N88Q) and the triple mutant LmrR_RMH_LWQ (LmrR_RMH_F54L_I62W_N88Q). Any combination that entailed the presence of the I62W mutation resulted in the loss of the improvement of activity compared to the single mutations (Fig. S2†), which, for LmrR_RMH_LWQ, was the result of a reduced affinity for the hydrazine substrate (Table S3†). However, the double mutant L-Q showed a significant increase in rate acceleration predominated by an increase in the apparent turnover number (kcat,app), 1.6-times higher than LmrR_RMH and 1.4-times higher than the single mutant LmrR_RMH_N88Q. This shows a synergistic effect between positions F54 and N88.
Thermostability of F54L and N88Q.
LmrR_RMH_F54L, LmrR_RMH_N88Q and LmrR_RMH_L-Q were further investigated for their operational thermostability via comparison of their T1050, which indicates the temperature at which 50% of the residual catalytic activity can be found after a 10 minutes incubation (Fig. 2A). Both F54L and N88Q mutations provided a highly stabilising effect for LmrR_RMH, leading to an increase of approximately 8 and 5 °C respectively. LmrR_RMH_L-Q showed a significant additive effect, increasing the T1050 with 13 °C (Fig. S5 and Table S4†).
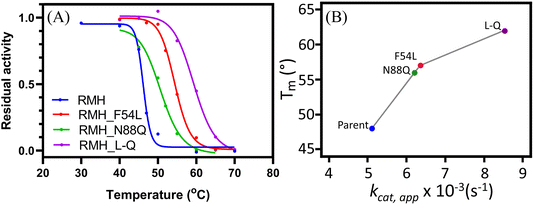 |
| Fig. 2 (A) Determination of the operational thermostability T1050 for the parent LmrR_RMH enzyme (blue), single mutants F54L (red) and N88Q (green) and double mutant LmrR_RMH_L-Q (purple). T1050 was calculated as the temperature at which 50% of residual activity is found. (B) Structural thermostability (Tm) of the three mutants (F54L, N88Q and L-Q) compared to the apparent turnover number (kcat). Reaction conditions: 2.5 μM of enzyme, 50 μM NBD-H and 5 mM of 4-HBA in buffer R pH 7.5 and 5% DMF, 25 °C. | |
To confirm that the increase in operational stability resulted from a more robust structure, circular dichroism spectroscopy was employed to measure the protein melting temperature (Tm). These measurements confirmed the thermostability improvement previously observed, with an observed increase in Tm of 9, 7 and 14 °C for LmrR_RMH_F54L, LmrR_RMH_N88Q and LmrR_RMH_L-Q, respectively (Fig. 2B and S6†).
The improved thermostability was verified by the results of the catalysis of the hydrazone formation reaction at higher temperature (i.e. 50 °C). The increased thermostability of LmrR_RMH_L-Q allowed the enzyme to have a more efficient retention of the activity, catalysing the reaction with 3.4-fold higher rate than the parent (Fig. S7†). This increase in retention of activity at high temperature could suggest a thermoadaptation similar to what was observed for a designer Kemp eliminase.19,40,41 Understanding the possible relationship between activity and temperature for this catalyst will require further study.
Rationalisation of the role of F54L and N88Q
In silico rationalisation of the F54L and N88Q mutations.
In order to understand the role of F54L and N88Q mutations and their effect on catalysis, the parent LmrR_RMH and the mutants LmrR_RMH_F54L and LmrR_RMH_N88Q were submitted to 700 ns MD simulations calculated in triplicate, resulting in a time scale of 2.1 μs for each system. The RMSD calculations showed that the three systems quickly reached a plateau (Fig. S8†), so the entire MD time scale was considered for the analysis.
Intriguingly, cluster analysis (Fig. S9 and S10†) evidenced significant changes in the overall structure for the three systems analysed, especially in the helices α4 and α4′, which comprise the W96 and W96′ residues. These residues are known to maintain the shape of the entrance pore by π-stacking interactions, and many states where these were displaced with respect to each other were found for all three systems, significantly affecting the shape of the catalytic pore. A more detailed analysis revealed that the main residues responsible for these global changes were R92/R92′, which showed different patterns of interactions along the MD time scale. Intriguingly, this residue was introduced in the first round of our initial directed evolution engineering campaign, which led to the LmrR_RMH variant.32
We grouped the structures into three main conformational states based on the R92/R92′ orientation (Fig. 3): (1) “cis-back”, in which both R92/R92′ were pointing towards the back of the pore of LmrR, where the DNA binding site (DBS) is located, without causing noticeable distortions of the overall structure; (2) “trans”, in which one arginine was changing its conformation towards the entrance pore where catalysis occurs while the other one remains oriented towards the back. In this state, which creates a significant distortion in the active site shape, the arginine that points forward has interaction partners in close proximity to one of the catalytic pAF residues; and (3) “cis-front”, where the two R92/R92′ are pointing towards the entrance of the cavity, establishing a salt bridge with D100′/D100. This conformation gives rise to an ordered organisation of the pore, where the side chain of R92 is oriented in-between W96 and pAF15, driven by π-cation interactions. This disposition brings the permanent positive charge of the arginine guanidinium moiety near the reactive aryl amino group of pAF, which potentially could have a detrimental effect on the formation of the iminium ion intermediate required for the hydrazone formation reaction to proceed. Therefore, we hypothesise that the R92/R92′ in the cis-front orientation might represent a catalytically inactive conformation of the enzyme.
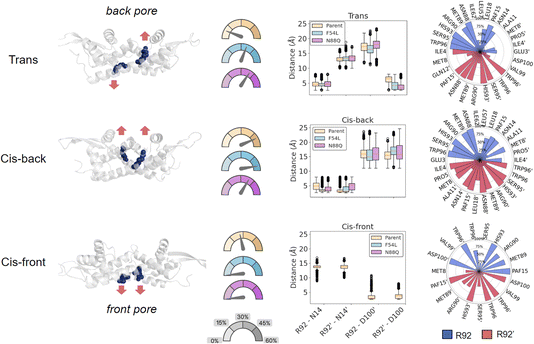 |
| Fig. 3 Left, representative structures of the three states observed during the molecular dynamics simulation, (trans, cis-back and cis-front). Residues R92 and R92′ are depicted in blue with ball and stick representation. Centre, gauge plot representing the percentage of trajectory spent by each protein variant in each of the conformations and box plot of the distances between R92 and either N14 or D100 in both monomers used to identify the three states along the MD trajectory. Notably, the cis-front orientation was not observed in either of the mutants. LmrR_RMH: bisque, LmrR_RMH-F54L: light blue, LmrR_RMH_N88Q: plum. Right, list of residues establishing contacts with either R92 (blue) or R92′ (red) in LmrR_RMH along with their frequency of interactions observed in each conformation state. A cut-off of 10% contact frequency was used. | |
In order to monitor the population of these conformations in each variant, each simulation was split into the three observed states based on the geometric distances of R92 to both N14 and D100 (Fig. 3 and S11†). Interestingly, the cis-front state was only found for the template LmrR_RMH, and not for the mutants. In contrast, the cis-back state appeared well represented in all the three systems, while the trans state appeared mainly represented in both mutants (Table S5†). This suggests that a major effect of the mutations F54L and N88Q is that they modulate the orientation of R92/R92′ in the pore. This arginine residue is important for the activity: it was the mutation that was found in the first round of directed evolution of the pore. Its precise role in catalysis is not yet known, but the current results suggest that it might very well be structural, to achieve a more optimal arrangement of the pore harbouring the catalytic pAF residue. However, our results also show that the evolved enzyme exists in multiple conformations, with the arginine in different orientations. The F54L and N88Q mutants have a significantly larger fraction of conformations with the arginine in the back orientation compared to the LmrR_RMH template, especially the trans conformation. This suggests this, or both conformations with the arginine in a back orientation, might be the catalytically productive conformation. Hence, the F54L and N88Q mutations result in a favouring of catalytically productive conformations at the expense of the cis-front conformation, which is hypothesized to be a less active conformation.
To gain further insight into how the conformational distributions identified were affecting the properties of LmrR, the global contacts of the protein as well as the residue interaction paths between the mutations and the catalytic residue were analysed. Overall, both mutants showed an increased frequency of contacts localised in the back and centre of the hydrophobic pocket compared to the parent (Fig. S12†). More specifically, increased contacts were observed near the N-terminus end of both monomers, which suggests the formation of tighter interactions that might prevent unfolding events driven by the disordered region of the termini. Additionally, the introduction of mutations F54L and N88Q resulted in more compact LmrR structures, as evidenced by an overall lower radius of gyration measured considering the alpha helices of the structure (Fig. S13†). Altogether, these observations could be translated into a higher structural robustness, which could also explain the increased thermostability observed experimentally.
The rationalisation of the exact role of the F54L and N88Q mutations is far from trivial as they seem to affect the structural properties of the enzyme, causing a cascade of rearrangements that ultimately modulate the active site behaviour. To gain a deeper understanding of how these changes occur, the close surroundings of both mutations were assessed based on representative structures from the cluster analysis. In the parent LmrR_RMH, the residue N88 showed a direct interaction with R92 by means of hydrogen bonding between their side chains. Although frequent, it seems that the short length of the N88 side chain prevents R92 from being sufficiently stabilized at such position. In contrast, the extra methylene group introduced with the N88Q mutation enables R92 to establish hydrogen bonding with both N88Q and N14, thus increasing the occurrence of back or catalytically productive states.
Regarding the F54L mutation, no direct connection between the close surroundings of this position and the R92 conformational distribution could be found, as occurred for N88Q. In this case, dynamical network analysis42 was key to shedding light on the effect of this mutation by identifying the nodes that separate F54L from the catalytic pAF. In this method, using the graph theory, the residues within a protein can be described as nodes of a network, while the connections (i.e. the edges) may identify allosteric communication. The ‘betweenness’ of an edge refers to the number of short paths passing through it, giving a hint of the relevance of such edge across the network. The dynamical network analysis showed significantly different scenarios between the parent and the F54L mutation. The communication between the residues F54L and pAF was found to be either shorter or stronger than in the parent (Fig. 4). These new paths of communication might be related to the shorter distance between the helices α3′ and α1′, which show an increased number of interactions, and to a tighter hydrogen bonding network arising between E7 from helix α1 and R56′ from helix α3′ (Fig. S14†). In turn, E7 was shown to establish more hydrogen bonds with N14′ than the parent, therefore potentially affecting the conformation distribution of R92 in an indirect fashion.
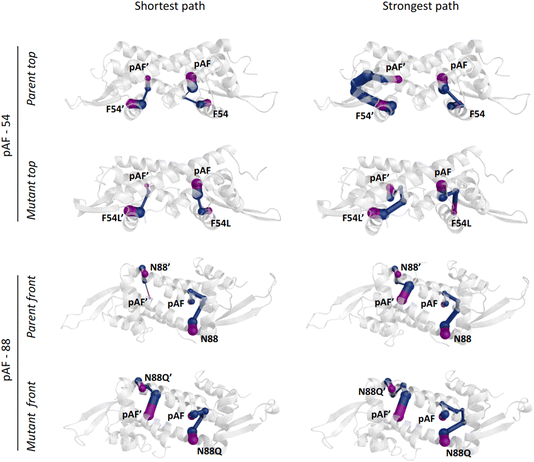 |
| Fig. 4 Allosteric pathways connecting the position mutated (either 54/54′ or 88/88′), considered as the “source” of the path, to the catalytic pAF, considered as the “sink” of the path, based on dynamical network analysis. The edges are weighted based on their betweenness. The source and sink are depicted in purple, while the rest of the pathway is depicted in blue. On the left, the shortest path, considered as the path involving the least number of nodes, is shown. On the right, the strongest path, considered as the path with edges having the highest betweenness based on visual inspection, is shown. | |
Materials and methods
Prediction of long-range hotspots
Zymspot43 uses bioinformatics and structure-based approaches to depict distal mutations with the potential to perturb the enzyme's conformational dynamics, ultimately impacting the population of catalytically productive conformations. Zymspot does not rely on time-consuming strategies such as molecular dynamics simulations to capture the impact of the mutations on the conformational dynamics of the protein; but quickly extracts bioinformatics and structural metrics that were benchmarked against experimental data (data not shown). This allows the fast screening (a few hours per enzyme) of protein scaffolds regardless of their size or catalytic activity with minimum human intervention and without the need for empirical data related to the target protein. 49 positions, comprising a library of 96 variants resulted from this study. The amino acids selected at each position were based on the most representative candidates found in a Multi Sequence Alignment (MSA) performed with the ClustalO software.44 Additionally, since consensus mutations are known to often lead to changes in the enzymatic properties, such as activity or thermostability,45–47 six consensus mutations were added to the list, resulting in a total of 102 variants.
Preparation of distal mutations library
Plasmid pET17b_LmrR_V15X_A92R_N19M_F93H (pET17b_LmrR_RMH), which was prepared in a previous study,32 was used as the template for site-directed mutagenesis (QuikChange, Agilent Technologies). PCR was performed in 25 μL total volume containing Cloned Pfu reaction buffer (1× final concentration), dNTPs mixture (0.2 mM each dNTP), DNA template (10–50 ng), primers (0.3 μM), DMSO (3% v/v) and PfuTurbo DNA polymerase (2.5 U). The mutations were introduced using the appropriate primer pairs (see Tables S6–S8†) with a Touch-Down PCR program (see ESI†). The resulting PCR product was treated with 20 U of DpnI and 5 μL was transformed into either E. coli NEB10β cells or E. coli BL21(DE3) containing the plasmid pEVOL-pAFRS2.t1 (gift from Farren Isaacs (Addgene plasmid #73546)) for protein expression. The transformation mixture was spread onto Luria–Bertani (LB)-agar plates containing the necessary antibiotics. Single colonies were transferred into 5 mL of LB and incubated overnight at 37 °C. This densely grown culture was used for Sanger sequencing of the mutated plasmid and as inoculum for protein expression.
Cell lysate screening
E. coli BL21(DE3) cells containing the mutated plasmids were inoculated in a 96-deep well plate in 1 mL of LB supplemented with ampicillin (100 μg mL−1) and chloramphenicol (34 μg mL−1) and incubated overnight at 37 °C, 900 rpm. Afterwards, 50 μL of the overnight culture was used to inoculate at least 3 plates containing 1100 μL of fresh LB and incubated for 4 h at 37 °C, 900 rpm. Subsequently, protein production was induced by the addition of 50 μL LB media, containing 0.8 mM IPTG, 0.017% arabinose and 1 mM p-azidophenylalanine (pAzF). Plates were then incubated at 30 °C for 16 hours and harvested by centrifugation (3000×g at 4 °C for 20 minutes). The pellet was washed in 500 μL of Buffer R (50 mM Na2HPO4, 150 mM NaCl, pH = 7.5), centrifuged again (3000×g at 4 °C for 20 minutes) and then frozen overnight at −20 °C. Lysis of the cells was performed by resuspension in 300 μL of lysis buffer containing Buffer R, protease inhibitor cocktail (Roche cOmplete), lysozyme (1 mg mL−1), DNase I (0.1 mg mL−1) and MgCl2 (10 mM). Cells were incubated for 2 h at 30 °C at 900 rpm, then pAzF was reduced to p-aminophenylalanine (pAF) via Staudinger reduction by supplying 10 mM TCEP to the lysate. The reduction was performed at room temperature for at least 1 h. Cell debris was removed with centrifugation for 45 min at 4 °C, 3000×g. The cleared lysate was immediately assayed for activity.
The activity assay procedure followed the previously reported protocol32 with minor adjustments. Briefly, 80 μL of cleared lysate was mixed with 100 μL of Buffer R in a clean 96-well plate. A stock solution (20 μL) containing 100 mM 4-HBA and 1 mM NBD-H in Buffer R (50% DMF) was added and measurement started immediately using a 96-well plate reader (Powerwave X, Biotek Instruments Inc.). Product formation was followed at 472 nm for about 2 h at 25 °C and the slope between 10–60 min was used to assess enzyme efficiency for every mutant relative to the parent in the same 96-well plate.
Representative procedure for protein expression and purification
One mL of a densely grown small culture (5 mL) of E. coli BL21(DE3) cells harbouring the desired pET17b_LmrR_V15pAF_RMH mutant was used to inoculate fresh LB medium (100 mL) and incubated at 37 °C, 135 rpm. Protein expression was induced when OD600 ∼ 0.8–1 (typically after 3 h) with isopropyl β-D-1-thiogalactopyranoside (IPTG), L-arabinose and pAzF (1 mM, 0.2% w/v and 1 mM final concentration respectively). The culture was incubated overnight (30 °C, 135 rpm) and the cells were harvested by centrifugation (4 °C, 6000×g, 30 min) and resuspended with 15 mL of lysis buffer (50 mM Na2HPO4, 150 mM NaCl, 10 mM PMSF, pH = 8). The cells were disrupted by sonication, and the cell debris removed by centrifugation (4 °C, 12
000×g, 50 minutes). The supernatant was passed through a syringe-filter (0.45 μm, Whatman) and applied to Streptag II resin (IBA Lifesciences) (4 mL). The resin was then washed twice with 10 mL of Buffer A (50 mM Na2HPO4, 150 mM NaCl, pH = 8) and the protein was eluted with Buffer A containing desthiobiotin (IBA Lifesciences, 5 mM, 10 mL). The protein was concentrated to ∼1 mL with a centrifugal filter (4 °C, 4000×g, 30 minutes). The reduction of pAzF into pAF was performed by addition of 10 mM TCEP and incubated for at least 1 h. The excess of TCEP was then removed with a desalting column (GE Healthcare) and the buffer was changed to Buffer R (same as Buffer A, pH = 7.5) for catalysis. The protein concentration was determined by absorbance at 280 nm with extinction coefficients corrected for the presence of the pAF residue (ε280nm = 1333 M−1 cm−1).
Kinetic characterization
Kinetic assays for the hydrazone formation between 4-hydroxybenzaldehyde (4-HBA) and 4-hydrazino-7-nitro-2,1,3-benzoxadiazole hydrazine (NBD-H) were performed according to the previously reported procedure32 with minor changes. In brief, product formation was followed at 472 nm (ε472 = 25
985 M−1 cm−1) and all reactions were measured at 25 °C in a total volume of 1 mL containing Buffer R, 5% (v/v) DMF, 2.5 μM of enzyme catalyst, 5 mM of 4-HBA and varying concentrations of NBD-H (12.5, 25, 40, 50, 75, 100 μM). The background reaction was measured by addition of NBD-H in the solution containing all components but the enzyme. Background rates were recorded for each measurement (10–20 minutes) before addition of the enzyme, after which absorption was recorded for an additional 10–20 minutes. The observed initial rates were corrected for the background reaction and apparent kinetic parameters were obtained by fitting (Rstudio) a standard Michaelis–Menten kinetic equation for a pseudo-first order reaction rate. Each value represents the average of two independent experiments each measured at least in duplicate (for a total of at least four data points). The kinetics of LmrR_V15pAF was measured only in duplicate. For a preliminary assessment of performance between mutants, a single point measurement was obtained in triplicate in the same conditions reported for kinetics and with the concentration of NBD-H fixed at 50 μM.
Operational thermostability characterization
Each mutant was diluted to about 144 μM in 20 μL with Buffer R, each in triplicate. Each aliquot was thermally challenged for 10 minutes at the desired constant temperature in a PCR thermocycler (Eppendorf Mastercycler) and then cooled down on ice for at least 30 minutes prior to kinetic analysis. The activity assay was performed at 25 °C in a total volume of 1 mL containing Buffer R, 5% (v/v) DMF, enzyme catalyst (2.5 μM), 4-HBA (5 mM) and NBD-H (50 μM) as previously described. At least 7 temperatures were selected for each mutant to achieve a reliable representation of the activity decay profile ranging from 30 °C up to 70 °C. Measured points were fitted (Graphpad Prism 9) with a standard sigmoidal four parameter logistic (4PL) curve.
CD spectroscopy
Purified proteins were diluted to 4 μM in 200 μL of 50 mM sodium phosphate buffer pH 7.5. A CD spectrum was recorded before and after the heat treatment when the temperature was stable at 20 °C, in the interval 200–260 nm with 50 nm min−1 scan speed, bandwidth of 2 nm and a digital integration time (D.I.T.) of 1 s. A baseline was recorded containing only the buffer. Thermal denaturation was followed by monitoring the ellipticity at 222 nm at 2 °C intervals from 15 °C to 90 °C with ramping of 0.5 °C min−1, bandwidth of 2 nm and D.I.T. of 2 s. Additionally, the ellipticity of all samples was measured with the same settings from 90 °C to 15 °C to monitor for possible refolding events. The measured ellipticity was normalised with reference values measured for each fully denatured mutant. The reference value was obtained by diluting each protein to 4 μM in 300 μL of 50 mM sodium phosphate buffer pH 7.5 and heating at 95 °C for more than 5 h. A CD spectrum of the fully denatured samples was collected. Tm was calculated by fitting a standard sigmoidal five parameter logistic (5PL) curve using non-linear regression (Graphpad Prism 9) and constraining the top and bottom value to 1 and 0 respectively.
Molecular dynamics simulations
The parent and the single mutants F54L and N88Q were parameterized with the AmberTools' tleap program. The standard residues were parameterized using the ff14SB force field,48 while the non-standard p-aminophenylalanine (pAF) residue was parameterized using the GAFF force field49 and its RESP charges were calculated at the B3LYP/6-31G(d) level of theory using Psi4,50 as the QM engine and the RESP charges were calculated using a Python implementation of the RESP charge model.51 A cubic box of TIP3P water molecules was built around the protein with its closest edge to the protein at a distance of 10 Å. As a result, a system of around 118
000 atoms, with 3800 of them from the protein, was obtained. The obtained topology and coordinates files were then converted into the Gromacs formats using the AmberTools' amb2gro_top_gro.py script.
Using the Gromacs version 2022,52 three replicas were calculated for each of the three systems, so a total of 9 MD simulations were performed. For each of them, an initial minimization was carried out followed by a heating phase from 0 to 310 K during 200 ps. Then, 200 ps of NPT simulation was carried out at 310 K and 1 atm for the equilibration of the system. Once equilibrated, 700 ns of NVT simulations were calculated at 310 K. A time step of 2 fs was used for the whole simulation. Bonds involving H atoms were restrained in all the stages. A cut-off of 10 Å for the non-bonding and electrostatic interactions was used. The PME method53 was used to treat the long-range effects. PBC conditions were also used. Each trajectory was post-processed to correct the protein's non-continuities in the unit cell caused by PBC imaging. To do so, AmberTools' cpptraj program was used.
Conclusions
The capability of grafting novel reactivities borrowed from the organic chemist's toolbox into existing protein scaffolds opens exciting new avenues for biocatalysis. However, the resulting artificial enzymes are not yet as efficient as natural enzymes, due to the lack of a comprehensive understanding of all the interactions underpinning enzymatic catalysis.
This work focused on the role of distal mutations in the catalysis of a new-to-nature reaction by an LmrR-based artificial enzyme featuring a non-canonical pAF catalytic residue. A computational prediction pipeline targeting hotspots affecting protein dynamics named Zymspot was employed here to build a smart library of variants and was shown to be able to provide key functional hotspots in just a few hours of simulations per protein scaffold. Hence this approach is a viable alternative to comprehensive directed evolution studies and may provide complementary information.54 With the screening of as little as 73 mutants, two single remote mutations were identified which resulted in both an increased catalytic activity as well as a remarkable increase in thermostability. 2.1 μs molecular dynamics simulations highlighted how such distal mutations can manipulate the distribution of the protein conformations, favouring catalytically productive ones at the expensive of potentially less efficient conformations, by means of a cascade of small rearrangements that could have hardly been predicted rationally. The current study focused exclusively on the resting state of the enzyme. Our observations are in line with studies on canonical enzymes, where mutations favour the appearance of productive conformations, enhancing the enzymatic efficiency12,17,40 For an in-depth understanding of the effect of distal mutations on catalysis, further work involving the substrate and reaction intermediates will be required to understand how these conformational ensembles relate to the appearance of transition-state-like conformations in LmrR. Furthermore, combining the advantages of different algorithms for the identification of long-range pathways will provide a more holistic understanding of LmrR structure.55 Yet, the current study already illustrates the power of computational predictive tools for the engineering of artificial enzymes beyond the active site.
Author Contributions
G. R., F. C. and L. A.-C conceived the project. F. C. performed all the wet lab experiments and wrote the original draft, M. C.-N. performed the molecular dynamics simulations, L. A.-C. and F. C. analysed the molecular dynamics data, L. A.-C. prepared the input protein structures for molecular dynamics and supervised the dry lab analyses. G. R. supervised the wet lab analyses. F. C., G. R. and L. A.-C. reviewed and edited the manuscript.
Conflicts of interest
L. A.-C. and M. C.-N. are employed by Zymvol Biomodeling S. L. which owns proprietary rights on the algorithm Zymspot used in this study.
Acknowledgements
The authors thank J. Hekelaar for analytical support with the mass spec and Dr L. Longwitz for fruitful discussion in the development of this study. This work was supported by the European Research Council (ERC advanced grant 885396).
References
- R. Wohlgemuth, Curr. Opin. Biotechnol., 2010, 21, 713–724 CrossRef CAS PubMed.
- R. A. Sheldon and J. M. Woodley, Chem. Rev., 2018, 118, 801–838 CrossRef CAS PubMed.
- S. Wu, R. Snajdrova, J. C. Moore, K. Baldenius and U. T. Bornscheuer, Angew. Chem., Int. Ed., 2021, 60, 88–119 CrossRef CAS PubMed.
- R. Buller, S. Lutz, R. J. Kazlauskas, R. Snajdrova, J. C. Moore and U. T. Bornscheuer, Science, 2023, 382, eadh8615 CrossRef CAS PubMed.
-
R. A. Sheldon, I. W. C. E. Arends and U. Hanefeld, in Green Chemistry and Catalysis, John Wiley & Sons, Ltd, 2007, pp. 223–264 Search PubMed.
- I. Drienovská and G. Roelfes, Nat. Catal., 2020, 3, 193–202 CrossRef.
- S. L. Lovelock, R. Crawshaw, S. Basler, C. Levy, D. Baker, D. Hilvert and A. P. Green, Nature, 2022, 606, 49–58 CrossRef CAS PubMed.
- F. Schwizer, Y. Okamoto, T. Heinisch, Y. Gu, M. M. Pellizzoni, V. Lebrun, R. Reuter, V. Köhler, J. C. Lewis and T. R. Ward, Chem. Rev., 2018, 118, 142–231 CrossRef CAS.
- E. A. Althoff, L. Wang, L. Jiang, L. Giger, J. K. Lassila, Z. Wang, M. Smith, S. Hari, P. Kast, D. Herschlag, D. Hilvert and D. Baker, Protein Sci., 2012, 21, 717–726 CrossRef CAS PubMed.
- F. Christoffel, N. V. Igareta, M. M. Pellizzoni, L. Tiessler-Sala, B. Lozhkin, D. C. Spiess, A. Lledós, J.-D. Maréchal, R. L. Peterson and T. R. Ward, Nat. Catal., 2021, 4, 643–653 CrossRef CAS.
- D. Baker, Protein Sci., 2010, 19, 1817–1819 CrossRef CAS PubMed.
- D. Petrović, V. A. Risso, S. C. L. Kamerlin and J. M. Sanchez-Ruiz, J. R. Soc., Interface, 2018, 15, 20180330 CrossRef PubMed.
- H. Yang, A. M. Swartz, H. J. Park, P. Srivastava, K. Ellis-Guardiola, D. M. Upp, G. Lee, K. Belsare, Y. Gu, C. Zhang, R. E. Moellering and J. C. Lewis, Nat. Chem., 2018, 10, 318–324 CrossRef CAS PubMed.
- B. Spiller, A. Gershenson, F. H. Arnold and R. C. Stevens, Proc. Natl. Acad. Sci. U. S. A., 1999, 96, 12305–12310 CrossRef CAS PubMed.
- A. Shimotohno, S. Oue, T. Yano, S. Kuramitsu and H. Kagamiyama, J. Biochem., 2001, 129, 943–948 CrossRef CAS PubMed.
- J. Lee and N. M. Goodey, Chem. Rev., 2011, 111, 7595–7624 CrossRef CAS PubMed.
- M. A. Maria-Solano, E. Serrano-Hervás, A. Romero-Rivera, J. Iglesias-Fernández and S. Osuna, Chem. Commun., 2018, 54, 6622–6634 RSC.
- A. Romero-Rivera, M. Garcia-Borràs and S. Osuna, ACS Catal., 2017, 7, 8524–8532 CrossRef CAS PubMed.
- H. A. Bunzel, J. L. R. Anderson, D. Hilvert, V. L. Arcus, M. W. van der Kamp and A. J. Mulholland, Nat. Chem., 2021, 13, 1017–1022 CrossRef CAS PubMed.
- R. Otten, R. A. P. Pádua, H. A. Bunzel, V. Nguyen, W. Pitsawong, M. Patterson, S. Sui, S. L. Perry, A. E. Cohen, D. Hilvert and D. Kern, Science, 2020, 370, 1442–1446 CrossRef CAS PubMed.
- S. Osuna, Wiley Interdiscip. Rev. Comput. Mol. Sci., 2021, 11, e1502 CrossRef CAS.
- M. Cagiada, S. Bottaro, S. Lindemose, S. M. Schenstrøm, A. Stein, R. Hartmann-Petersen and K. Lindorff-Larsen, Nat. Commun., 2023, 14, 4175 CrossRef CAS PubMed.
- P. K. Madoori, H. Agustiandari, A. J. M. Driessen and A.-M. W. H. Thunnissen, EMBO J., 2009, 28, 156–166 CrossRef CAS PubMed.
- G. Roelfes, Acc. Chem. Res., 2019, 52, 545–556 CrossRef CAS PubMed.
- L. Alonso-Cotchico, J. Rodríguez-Guerra Pedregal, A. Lledós and J.-D. Maréchal, Front. Chem., 2019, 7, 211 CrossRef CAS PubMed.
- L. Villarino, S. Chordia, L. Alonso-Cotchico, E. Reddem, Z. Zhou, A.-M. W. H. Thunnissen, J.-D. Maréchal and G. Roelfes, ACS Catal., 2020, 10, 11783–11790 CrossRef CAS PubMed.
- S. Chordia, S. Narasimhan, A. Lucini Paioni, M. Baldus and G. Roelfes, Angew. Chem., Int. Ed., 2021, 60, 5913–5920 CrossRef CAS PubMed.
- I. Drienovská, A. Rioz-Martínez, A. Draksharapu and G. Roelfes, Chem. Sci., 2015, 6, 770–776 RSC.
- Z. Zhou and G. Roelfes, Nat. Catal., 2020, 3, 289–294 CrossRef CAS.
- L. Wang, A. Brock, B. Herberich and P. G. Schultz, Science, 2001, 292, 498–500 CrossRef CAS PubMed.
- I. Drienovská, C. Mayer, C. Dulson and G. Roelfes, Nat. Chem., 2018, 10, 946–952 CrossRef PubMed.
- C. Mayer, C. Dulson, E. Reddem, A.-M. W. H. Thunnissen and G. Roelfes, Angew. Chem., Int. Ed., 2019, 58, 2083–2087 CrossRef CAS PubMed.
- R. C. Cadwell and G. F. Joyce, Genome Res., 1992, 2, 28–33 CrossRef CAS PubMed.
-
Zymevolver: A ZYMVOL computational tool for enzyme engineering, https://zymvol.com/enzyme-technology/.
- E. Z. L. Zhong-Johnson, Z. Dong, C. T. Canova, F. Destro, M. Cañellas, M. C. Hoffman, J. Maréchal, T. M. Johnson, M. Zheng, G. S. Schlau-Cohen, M. F. Lucas, R. D. Braatz, K. G. Sprenger, C. A. Voigt and A. J. Sinskey, J. Biol. Chem., 2024, 300, 105783 CrossRef CAS PubMed.
-
A. Deplace, E. Monza, D. Panigada and A. Steinmetz, US Pat.20230130811A1, 2023 Search PubMed.
-
A. Deplace, E. Monza, D. Panigada and A. Steinmetz, US Pat.20230140642A1, 2023 Search PubMed.
-
N. Loncar and L. Alonso-Cotchico, WO Pat.2022066007A1, 2022 Search PubMed.
- K. Takeuchi, M. Imai and I. Shimada, Sci. Rep., 2017, 7, 267 CrossRef PubMed.
- E. J. Walker, C. J. Hamill, R. Crean, M. S. Connolly, A. K. Warrender, K. L. Kraakman, E. J. Prentice, A. Steyn-Ross, M. Steyn-Ross, C. R. Pudney, M. W. van der Kamp, L. A. Schipper, A. J. Mulholland and V. L. Arcus, ACS Catal., 2024, 14, 4379–4394 CrossRef CAS PubMed.
- J. K. Hobbs, W. Jiao, A. D. Easter, E. J. Parker, L. A. Schipper and V. L. Arcus, ACS Chem. Biol., 2013, 8, 2388–2393 CrossRef CAS PubMed.
- M. C. R. Melo, R. C. Bernardi, C. de la Fuente-Nunez and Z. Luthey-Schulten, J. Chem. Phys., 2020, 153, 134104 CrossRef CAS PubMed.
-
Zymspot: A ZYMVOL computational tool for identifying distal mutations, https://zymvol.com/enzyme-technology/.
- F. Madeira, Y. mi Park, J. Lee, N. Buso, T. Gur, N. Madhusoodanan, P. Basutkar, A. R. N. Tivey, S. C. Potter, R. D. Finn and R. Lopez, Nucleic Acids Res., 2019, 47, W636–W641 CrossRef CAS PubMed.
- M. Sternke, K. W. Tripp and D. Barrick, Proc. Natl. Acad. Sci. U. S. A., 2019, 116, 11275–11284 CrossRef CAS PubMed.
-
M. Sternke, K. W. Tripp and D. Barrick, in Methods in Enzymology, ed. D. S. Tawfik, Academic Press, 2020, vol. 643, pp. 149–179 Search PubMed.
- Y. Zhao, K. Chen, H. Yang, Y. Wang and X. Liao, J. Agric. Food Chem., 2024, 72, 6454–6462 CrossRef CAS.
- J. A. Maier, C. Martinez, K. Kasavajhala, L. Wickstrom, K. E. Hauser and C. Simmerling, J. Chem. Theory Comput., 2015, 11, 3696–3713 CrossRef CAS PubMed.
- J. Wang, R. M. Wolf, J. W. Caldwell, P. A. Kollman and D. A. Case, J. Comput. Chem., 2004, 25, 1157–1174 CrossRef CAS PubMed.
- D. G. A. Smith, L. A. Burns, A. C. Simmonett, R. M. Parrish, M. C. Schieber, R. Galvelis, P. Kraus, H. Kruse, R. Di Remigio, A. Alenaizan, A. M. James, S. Lehtola, J. P. Misiewicz, M. Scheurer, R. A. Shaw, J. B. Schriber, Y. Xie, Z. L. Glick, D. A. Sirianni, J. S. O'Brien, J. M. Waldrop, A. Kumar, E. G. Hohenstein, B. P. Pritchard, B. R. Brooks, H. F. Schaefer III, A. Yu. Sokolov, K. Patkowski, A. E. DePrince III, U. Bozkaya, R. A. King, F. A. Evangelista, J. M. Turney, T. D. Crawford and C. D. Sherrill, J. Chem. Phys., 2020, 152, 184108 CrossRef CAS PubMed.
- A. Alenaizan, L. A. Burns and C. D. Sherrill, Int. J. Quantum Chem., 2020, 120, e26035 CrossRef CAS.
- M. J. Abraham, T. Murtola, R. Schulz, S. Páll, J. C. Smith, B. Hess and E. Lindahl, SoftwareX, 2015, 1–2, 19–25 CrossRef.
- P. P. Ewald, Annal. Phys., 1921, 369, 253–287 CrossRef.
- H. A. Bunzel, J. L. R. Anderson and A. J. Mulholland, Curr. Opin. Struct. Biol., 2021, 67, 212–218 CrossRef CAS PubMed.
- M. Castelli, F. Marchetti, S. Osuna, A. S. F. Oliveira, A. J. Mulholland, S. A. Serapian and G. Colombo, J. Am. Chem. Soc., 2024, 146, 901–919 CrossRef CAS PubMed.
|
This journal is © The Royal Society of Chemistry 2024 |
Click here to see how this site uses Cookies. View our privacy policy here.