DOI:
10.1039/D1LC01161H
(Paper)
Lab Chip, 2022,
22, 1187-1205
Exploration and application of a liver-on-a-chip device in combination with modelling and simulation for quantitative drug metabolism studies†
Received
22nd December 2021
, Accepted 19th January 2022
First published on 25th January 2022
Abstract
Microphysiological systems (MPS) are complex and more physiologically realistic cellular in vitro tools that aim to provide more relevant human in vitro data for quantitative prediction of clinical pharmacokinetics while also reducing the need for animal testing. The PhysioMimix liver-on-a-chip integrates medium flow with hepatocyte culture and has the potential to be adopted for in vitro studies investigating the hepatic disposition characteristics of drug candidates. The current study focusses on liver-on-a-chip system exploration for multiple drug metabolism applications. Characterization of cytochrome P450 (CYP), UDP-glucuronosyl transferase (UGT) and aldehyde oxidase (AO) activities was performed using 15 drugs and in vitro to in vivo extrapolation (IVIVE) was assessed for 12 of them. Next, the utility of the liver-on-a-chip for estimation of the fraction metabolized (fm) via specific biotransformation pathways of quinidine and diclofenac was established. Finally, the metabolite identification opportunities were also explored using efavirenz as an example drug with complex primary and secondary metabolism involving a combination of CYP, UGT and sulfotransferase enzymes. A key aspect of these investigations was the application of mathematical modelling for improved parameter calculation. Such approaches will be required for quantitative assessment of metabolism and/or transporter processes in systems where medium flow and system compartments result in non-homogeneous drug concentrations. In particular, modelling was used to explore the effect of evaporation from the medium and it was found that the intrinsic clearance (CLint) might be underestimated by up to 40% for low clearance compounds if evaporation is not accounted for. Modelling of liver-on-a-chip in vitro data also enhanced the approach to fm estimation allowing objective assessment of metabolism models of different complexity. The resultant diclofenac fm,UGT of 0.64 was highly comparable with values reported previously in the literature. The current study demonstrates the integration of mathematical modelling with experimental liver-on-a-chip studies and illustrates how this approach supports generation of high quality of data from complex in vitro cellular systems.
1. Introduction
High quality estimates of pharmacokinetic (PK) parameters are essential during drug discovery for candidate selection and human dose predictions. Drug absorption, distribution, metabolism, and excretion (ADME) are the core PK processes which need to be optimized to obtain the desired clinical exposure. Multiple in vitro systems in conjunction with physiologically based pharmacokinetic (PBPK) modelling are routinely used for the experimental assessment and extrapolation of ADME properties. Primary hepatocytes cultured in either plated or suspension formats are often employed for the determination of the metabolic and transporter-mediated clearance, enzyme phenotyping and metabolite identification. However, established in vitro assays still leave gaps in the quantitative translation which can potentially be addressed by the introduction of novel cell culture techniques. One limitation of hepatocytes cultured in a 2D format is the rapid loss of enzyme activity during the experiment which hinders accurate prediction of metabolic clearance, especially for metabolically stable drugs.1,2 Additional limitations include the assessment of other ADME-related drug properties such as biliary and sinusoidal efflux, accurate in vitro to in vivo scaling of active uptake clearance for substrates of drug transporters, prediction of in vivo relevant metabolites for metabolically stable drugs, and the reliable prediction of time-dependent inhibition and induction.2–5 Microphysiological systems (MPS) hold promise to address more complex in vitro ADME, toxicology and pharmacology questions in a more physiologically relevant manner.6,7 The PhysioMimix liver-on-a-chip is a microfluidic device that enables continuous perfusion of the hepatocytes to provide nutrients, oxygen, and mechanical stimuli through the shear stress of the media flow (Fig. 1).8 The system used is closely related to the system previously described in a proof of principle study by Tsamandouras et al. who examined inter-individual variation in diclofenac metabolism in vitro and modelled this to the in vivo situation.9 Vivares and colleagues10 demonstrated prolonged maintenance of cell phenotype and higher metabolic activity in the liver-on-a-chip compared to 2D-cultured plated hepatocytes by using the same hepatocyte lot in both in vitro formats. Sarkar and colleagues11 simultaneously assessed the metabolic profile as well as complex metabolite toxicity of diclofenac by combining primary human hepatocytes and Kupffer cells. Rubiano and colleagues12 tested the ability of the liver-on-a-chip device to reproduce drug-induced hepatotoxic effects, metabolism, and intracellular accumulation and, importantly, demonstrated reproducibility of the experiments. The system is attractive for ADME applications as it offers the capability to seed a high number of hepatocytes per incubation chamber (referred to hereafter as “well”), has substantial medium volume enabling multiple drug concentration measurements and a growing body of prior evaluation data.9,13,14 Despite these promising initial efforts, integration of MPS into pharmaceutical development workflows still requires verification that the platforms fulfil minimum requirements for ADME studies. The cells cultured in the liver-on-a-chip format need to demonstrate a physiologically relevant and stable phenotype2,15 and, in addition, the results generated in MPS need to be robust and reproducible. Only after demonstration of these attributes and a broader validation including diverse molecules, can the systems be considered for more complex studies and for adoption by pharmaceutical companies.
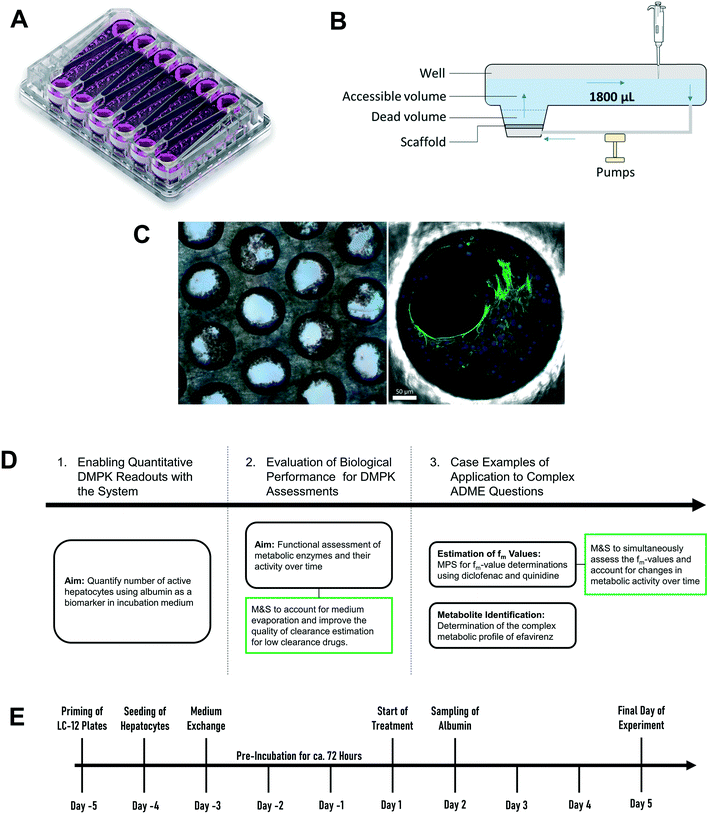 |
| Fig. 1 Schematic representation of A) picture of the LC12 plate (©CN Bio Ltd., reproduced with permission); B) representation of the well in the LC12 plate which highlights the micropump system and the positon of sampling; C) scaffold and seeded hepatocytes: bright field light microscopy (left), and confocal microscopy (right) (BF, nuclei-DAPI and actin-phalloidin Alexa 488). D) The study outline and E) default procedure of plate preparation and experimental time course for the evaluation of CP, UGT and AO activity in the liver-on-a-chip. DMPK: Drug metabolism and pharmacokinetics; M&S: modelling and simulation; MPS: microphysiological system; fm: fraction metabolized by individual enzyme isoforms. | |
The current study aimed to expand the evaluation of liver-on-a-chip system for applications in the drug metabolism and pharmacokinetic (DMPK) area, as illustrated in the framework in Fig. 1D. Firstly, the seeding efficiency of the system was determined by measuring the number of active hepatocytes residing in the scaffold. This evaluation is essential for quantitative translation of data from in vitro to in vivo, since the assumption that all cells seeded into a system are retained and active can be highly inaccurate. To allow this analysis to be performed in a non-invasive manner, albumin production rate was established as a surrogate marker for cell number estimation. Secondly, this study assessed the functional activity using multiple drug compounds known to be substrates of important drug metabolizing enzymes such as cytochromes P450 (CYPs), UDP-glucuronosyltransferases (UGTs), and aldehyde oxidase (AO). Thirdly, more complex endpoints were investigated, namely metabolite identification and fraction of drug metabolized via a single enzyme pathway (fm), to demonstrate the potential of the liver-on-a-chip system. Modelling and simulation has been applied throughout as an essential part of experiment planning and data evaluation.
2. Experimental
2.1 Materials
Ketoprofen, zidovudine, dextromethorphan, dextrorphan, diclofenac, repaglinide, carbazeran, irinotecan, telmisartan, and quinidine were purchased from Sigma-Aldrich (St. Louis, MO, USA). Lorazepam, naloxone, 4′-hydroxydiclofenac, diclofenac-glucuronide, 3-hydroxyquinidine, 4-hydroxycarbazeran, naloxone-glucuronide, efavirenz, 7-hydroxyefavirenz, telmisartan-glucuronide, and posaconazole were purchased from Toronto Research Chemicals (Toronto, Canada). Cryopreserved hepatocyte recovery medium, William's E medium, primary hepatocyte plating supplements, primary hepatocyte maintenance supplements, and human hepatocytes (lot: Hu8264) were purchased from Thermo Fisher Scientific (Grand Island, NY, USA). Oxazepam, midazolam, and 1′-hydroxymidazolam were synthesized at F. Hoffmann-La Roche Ltd. (Basel, Switzerland). All CN Bio components (controller, docking station, and drivers) and consumables (LC-12 plates) were acquired from CN Bio Innovations (Cambridge, UK) (Fig. S1†).
2.2 Preparation of LC-12 plates and seeding of cryopreserved human hepatocytes
The diagram in Fig. 1E depicts the time scale of the process from plate preparation to the experiment. Detailed descriptions of the priming of the plates, the seeding process of the hepatocytes, and the media exchange program are provided in Supplementary Information. Briefly, five days before the start of the experiment (day – 5), LC-12 plates were primed by flooding the microchannels with plating medium (William's E medium containing 5% fetal bovine serum, 1 μM dexamethasone, 100 U mL−1 penicillin, 100 μg mL−1 streptomycin, 4 μg mL−1 human recombinant insulin, 2 mM GlutaMAX™, 15 mM HEPES). The day after (day – 4), hepatocytes were seeded on the scaffolds of the LC-12 plates. Primary cryopreserved human hepatocytes (one vial per LC-12 plate) were thawed and suspended in cryopreserved hepatocyte recovery medium. Supernatant was aspirated after centrifugation (100 G for 10 minutes) and hepatocytes were re-suspended in 3 mL plating medium. Cells were counted with a hemocytometer (Bioswisstec Ltd., Schaffhausen, Switzerland) and seeded at between 400
000 and 650
000 hepatocytes per well, depending upon the individual experiment (consensus: 600
000 cells per well). Plates were incubated at 37 °C in a 5% CO2 atmosphere overnight to allow for the attachment of hepatocytes. The media was changed after attachment of the cells to the scaffold (at day – 3) to maintenance medium (William's E medium containing 0.1 μM dexamethasone, 50 U mL−1 penicillin, 50 μg mL−1 streptomycin, 6.25 μg mL−1 human recombinant insulin, 6.25 μg mL−1 human transferrin, 6.25 ng mL−1 selenous acid, 1.25 mg mL−1 bovine serum albumin, 5.35 μg mL−1 linoleic acid, 2 mM GlutaMAX™, 15 mM HEPES) and cells were pre-incubated without media exchange for three days at 37 °C in a 5% CO2 atmosphere until the first treatment day (day 1).
2.3 Quantification of cell number
On day – 4 of each experiment the remaining hepatocyte suspension not needed for seeding was used to investigate the total protein content per million hepatocytes. The hepatocyte suspension was diluted with 20 mL PBS and centrifuged at 100G for 10 minutes. The supernatant was aspirated and the hepatocyte pellet was lysed with 5 mL PBS containing 1% Triton-X for twenty minutes. Total protein content was measured using the Pierce™ BCA Protein Assay Kit (Thermo Fisher Scientific).
To relate the total cellular protein to the albumin production on day 2, three control wells in each experiment were used to determine the number of hepatocytes via lysis of the cells, assuming that all attached cells remained viable. By using the measured albumin concentration in the medium of the respective wells on day 2 (24 hours after media exchange at day 1) the normalized albumin production rate was calculated according to eqn (1):
|  | (1) |
where
Calb is the concentration of albumin (in μg μL
−1) in the medium after 24 hours (
t),
Vmed is the volume of medium (1800 μL) and
NH is the number of hepatocytes (expressed in million cells) determined in the lysates from the control wells by protein concentration. The average albumin production rate calculated from the control wells was then used to derive the number of hepatocytes in the remaining wells based on the measured albumin concentrations in the medium. This was performed separately for each experiment, compensating for any changes in albumin generation rate between experiments performed on different occasions or for different culture durations.
The albumin concentration was measured using the human albumin ELISA kit (Immunology Consultants Laboratory, Inc., Portland, USA) and total protein was measured using the Pierce™ BCA protein assay kit to assess the number of cells in the control wells (Thermo Fisher Scientific, Grand Island, NY, USA). A detailed protocol about the lysis of the hepatocytes in the scaffolds of the control wells is provided in the Supplementary Information.
2.4 Assessment of drug metabolism capability
The major enzymes involved in the metabolism of the selected drug molecules are summarized in Table 1. Drug concentrations, time points of medium sampling, and sampling volumes are summarized in ESI† Table S1. On the first study day (day 1), the maintenance medium was replaced with a fresh maintenance medium containing the test drugs with a total volume of 1800 μL (200 μL dead volume plus 1600 μL added volume). Afterwards, no additional media exchanges were performed during the time course of the experiment. The media was first allowed to distribute into the whole well for at least half an hour before the first sample was taken. At different time points, media samples were removed and quenched in acetonitrile containing internal standard (128 ng mL−1 D6-midazolam for all test compounds), centrifuged at 6200 G for 10 minutes at 4 °C and stored at −20 °C until preparation for measurement in liquid chromatography tandem mass spectrometry (LC-MS/MS). Drug concentration was measured in triplicate for each compound. To determine the depletion of the probe substrates, the natural logarithm of the drug concentration in the incubation samples was plotted against time and linear regression analysis was applied using GraphPad Prism version 7.04 for Windows (GraphPad Software, La Jolla, CA). The in vitro intrinsic clearance (CLint) was calculated from the depletion rate constant (k) of the linear regression (min−1) for each well individually and assuming a rapid passive diffusion into the cells: |  | (2) |
where Vmed was 1800 μL, and NH is the number of cells estimated from the measured albumin production rate in the respective wells.
Table 1 Comparison of unbound hepatic intrinsic clearance values calculated from in vivo studies with extrapolated unbound in vivo intrinsic clearance from liver-on-a-chip data for 12 compounds. The RBP, fuplasma and observed in vivo CLint were taken from the references provided. The mean in vitro unbound CLint and standard deviation are obtained using the fuinc which was either measured or calculated as indicated. In vitro data for carbazeran and irinotecan which were not included in the IVIVE were also reported in the table
Compound |
Major metabolic enzymes |
fuplasma |
R
BP
|
fuinc |
In vitro CLint,u |
In vivo CLint,u |
Fold errorg |
Predicted |
Observed |
μL per min per million cells |
mL/min/kg |
Measured data.
Calculated data from fuplasma, Ka in human serum albumin or bovine serum albumin (ESI† Table S5).
In vitro CLint,u were estimated by evaporation model of triplicate measurements with the respective ± SD.
In vitro CLint,u were estimated by modelling of triplicate measurements with the respective ± SD. For quinidine and diclofenac CLint from model 4 and 6 were respectively used.
In vitro CLint,u were estimated by mono-exponential decay of the substrate in triplicate measurements with the respective ± SD (eqn (3)).
The observed CLint,u was evaluated from oral administration assuming the intestinal availability and fraction absorbed equal to 1.25 The unbound plasma and bold plasma ratio were taken from Lutz et al.63 and Silva et al.,64 respectively.
Fold error was calculated as a ratio between predicted and observed CLint.
|
Carbazeran |
AO (ref. 52) |
|
|
0.74b |
12 ± 1e |
|
|
|
Dextromethorphan |
CYP2D6/3A4 (ref. 53) |
0.72 |
1.5 |
0.77b |
20 ± 2e |
51 |
576 (ref. 25)f |
0.089 |
Diclofenac |
CYP2C9, UGT2B7 (ref. 17, 54) |
0.010 |
0.55 |
0.045a |
95 ± 9d |
4 |
561 (ref. 25) |
0.43 |
Irinotecan |
CES36 |
|
|
0.76a |
0.92 ± 0.04c |
2.4 |
|
|
Lorazepam |
UGT2B7/15 (ref. 55) |
0.11 |
1.0 |
0.80b |
2.1 ± 0.3e |
6.5 |
14 (ref. 25) |
0.46 |
Midazolam |
CYP3A4/5 (ref. 56) |
0.031 |
0.55 |
0.10a |
219 ± 23e |
579 |
440 (ref. 21) |
1.3 |
Naloxone |
UGT2B7 (ref. 37) |
0.56 |
1.22 |
0.87a |
65 ± 2e |
167 |
200 (ref. 21) |
0.84 |
Oxazepam |
UGT1A9/2B15 (ref. 57) |
0.045 |
1.1 |
0.60b |
14 ± 1e |
37 |
34 (ref. 25) |
1.1 |
Posaconazole |
UGT1A4 (ref. 58) |
0.017 |
1.0 |
0.37b |
8.0 ± 2.0e |
25 |
148 (ref. 20) |
0.17 |
Quinidine |
CYP3A4/5 (ref. 59) |
0.13 |
0.87 |
0.63a |
12 ± 2d |
31 |
61 (ref. 25) |
0.50 |
Repaglinide |
CYP2C8/3A4 (ref. 39, 60) |
0.014 |
0.60 |
0.36b |
5.5 ± 1.7e |
14 |
1380 (ref. 21) |
0.010 |
Telmisartan |
UGT1A3 (ref. 61) |
0.005 |
1.0 |
0.15a |
64 ± 8e |
197 |
4519 (ref. 20) |
0.044 |
Tolbutamide |
CYP2C9 (ref. 35) |
0.024 |
0.75 |
0.48a |
1.2 ± 0.1c |
3.1 |
3.6 (ref. 25) |
0.88 |
Zidovudine |
UGT2B7 (ref. 62) |
0.74 |
0.99 |
0.98b |
1.7 ± 0.5e |
5.2 |
66 (ref. 20) |
0.079 |
In order to determine the tolerance of the system to repeated wash-out and re-use, measurement of compound depletion was performed repeatedly over four days (i.e. day 1, day 2, day 3, and day 4) with a washing step after every 24 hours before each incubation. This investigation of the repeated use activity retention was conducted for midazolam, diclofenac, repaglinide, carbazeran, quinidine, dextromethorphan, and naloxone. The retention of metabolic activity was assessed by comparing the depletion rates for the drug molecules at the different days (Fig. S2†).
2.5 Determination of in vitro intrinsic clearance for drugs with high metabolic stability
Three compounds with a high metabolic stability (tolbutamide, irinotecan, and ketoprofen) were selected to assess the impact of prolonged incubation times on the determination of the in vitro intrinsic clearance. Concentrations, sampling time points, and sampling volumes are summarized in ESI† Table S1. Incubations were run for 96 hours without performing media exchange during the time course of the experiment.
For such prolonged incubations, evaporation from the medium may have an impact on the calculated intrinsic clearance, especially for metabolically stable drugs (Fig. 4A). The metabolism-driven reduction in drug amount may be partially masked by media evaporation which concentrates the remaining drug substance. In contrast, the amount of compound transformed may be greater in conditions of higher evaporation of the medium due to the increased drug concentration and more frequent passage through the cells (which may be considered as an increase in the number of cells per unit incubation medium volume, Fig. 4A). Fig. 4B illustrates the relationship between the CLint the volume of medium, amount, concentration of the substrate, and cells/volume ratio when kev is maintained (0.07 μL min−1). In addition, Fig. 4 shows the impact of the sampling volume on the total media volume, amount, and cells/volume ratio as a sudden reduction of volume compared to the constant process of evaporation. The sampling volume does not immediately impact the concentration vs. time profile but has an effect over time and affects the number of data points which may be collected. In order to reduce the impact of the sampling volume in the actual experiments, it has been minimised to 18 μL considering the sample preparation and analysis processes. Therefore, a more complex model (referred to here as the evaporation model) is proposed to account for medium evaporation and sampling while estimating the CLint. For comparison, the linear model (eqn (3) without evaporation) and the evaporation model (eqn (3) and (4)) were both used to fit the observed concentration-time profiles and to estimate the in vitro intrinsic clearances.
The evaporation model assumed that the combination of media circulation and passive diffusion into cells is rapid and a one-compartment model was sufficient to describe the concentration of drug in the medium (full model is detailed in ESI,†In silico modelling section). Concentrations of the drug at different time points were measured and the amount of drug in the system calculated by multiplying the drug concentration by the system media volume. For modelling, the change in amount of drug in the system with time is described by eqn (3):
| 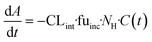 | (3) |
where fu
inc is the unbound fraction of drug in the incubation medium;
C and
A are the concentration and amount of drug, respectively, at given time points
t. Media volume decreased over time due to evaporation and sampling as described by
eqn (4):
| V(t) = Vi − kev·t − ns(t)·Vs | (4) |
where
Vi represents the initial volume (1818 μL) and
t represents the time. The evaporation constant
kev (in μL min
−1) was experimentally determined by measurement of the volume of the incubation medium in all wells at the final day of the experiment for the calculation of medium volume from medium weight. In addition, the sudden removal of medium and drug amount due to the sampling (18 μL per time point) was considered with the
Vs term at the specific time-dependent sampling points ns (
Fig. 4).
The kev was then calculated using eqn (5) by assuming a volume decrease following zero-order kinetics:
|  | (5) |
where
Vi,
Vf, and
tf, represent the initial, final volumes per well, and the incubation time, respectively. The loss of medium due to sampling (
Vs) and the dead volume (200 μL) remaining in the well after aspirating the medium were taken into account when deriving
Vf.
2.6 Evaporation model exploration
In addition to their application for CLint estimation from the observed in vitro data, the linear model and the evaporation model were also used to perform a preliminary in silico simulation assessment to test the adequacy of the two analyses. For this assessment, the evaporation model was applied to generate in vitro concentration-time profiles for a range of theoretical in vitro CLint values from 0.3 to 1.25 μL per min per million cells and different rates of evaporation from 0.01 to 0.12 μL min−1. The model used for all simulations included a constant number of hepatocytes (NH = 275
000 cells per well) and random effects (ω) for NH (CV = 20%) and kev (CV = 20%) which were based on our experience of experimental variability. In addition, the sampling volume of 18 μL was considered in the simulation in order to take into account the amount of substrate removed from the medium at the time of sampling. Moreover, a residual unexplained variability in the observed data was modelled with an exponential error of 10% represented by eqn (6).where CiObs represents the observed concentration affected by εi which is the deviation from the population value for each time point.
In order to assess variability, 1000 concentration-time profiles were simulated with the evaporation model using the conditions described above and three replicates were randomly sampled from the full set of simulated data at time points up to 96 hours. The random sampling was repeated 100 times. CLint values were then estimated by fitting either with the linear model (eqn (3) only) assuming V(t) is constant and equal to Vi or with the evaporation model (details about the fitting procedure are available in Software and libraries for modelling section). The sampling times used for the fitting of both models were: 0, 48, 56, 80, and 96 h.
Finally, the accuracy of the estimated mean CLint values was computed with the root-mean-square error (RMSE, eqn (7)) while the absolute average fold error (AAFE, eqn (8)) was used to evaluate the bias. In addition, the median of the uncertainty of 100 experiments were also evaluated. The percentage relative standard error (RSE) was evaluated and % RSE above 30% was considered as threshold of CLint uncertainty; details are summarized in the ESI† (Fig. S4).
| 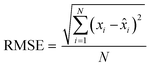 | (7) |
| 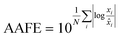 | (8) |
where
N is the number of observations, and
xi and
i are the predicted and observed parameters respectively. In addition for this set of 100 random triplicates, the median of the distribution of the 100 estimated CL
int values was also evaluated. Finally, we evaluated in which experimental conditions of
kev and CL
int the approximated linear model may be used to estimate CL
int with low bias.
2.7 Determination of fractions metabolized and metabolite formation for quinidine and diclofenac
Quinidine and diclofenac were incubated as described above and samples were taken at specific time points to measure drug disappearance and metabolite formation. The data for drugs and metabolites were subsequently analysed with different in silico models to estimate the fractions metabolised and rates of metabolite formation. The structure of the models is displayed in Fig. 7.
Four different in silico models with different levels of mechanistic detail were used to describe the observed concentration-time profiles of quinidine and 3-hydroxyquinidine. Model 1 assumed a single metabolic pathway and that quinidine depletion was solely due to 3-hydroxyquinidine formation. Model 2 considered also subsequent 3-hydroxyquinidine metabolism. Model 3 assumed that depletion of quinidine is due to the formation of 3-hydroxyquinidine and one or more additional primary metabolites16 (e.g. quinidine N-oxide). The latter was also assumed by model 4 which was a combination of model 2 and 3.
For diclofenac, in silico modelling was applied to estimate simultaneously CLint and contributions from oxidation (by CYP enzymes such as CYP2C9, CYP2C8 and CYP3A4) and glucuronidation (e.g. by UGT2B7) to overall metabolism (fm,CYP and fm,UGT).11,17 Two models were applied to describe the concentration-time profiles for diclofenac and its metabolites at different levels of mechanistic details (Fig. 7). Model 4 assumed the formation of 4′-hydroxydiclofenac and diclofenac-glucuronide, whereas model 5 considered also an additional but not measured primary metabolism pathway (e.g. 5′-hydroxydiclofenac formation). In order to define the best model for describing the experimental data, the Bayesian information criterion (BIC) was used for diclofenac and quinidine and their respective models 1–6. The model with the lowest BIC rank and strongest support from current literature information was selected for parameter estimation.
2.8 Protein binding
Fraction unbound in the incubations (fuinc values) was determined in the maintenance medium using equilibrium dialysis against 133 mM phosphate buffer at pH 7.4, over 5 hours at 37 °C in a 5% CO2 atmosphere, using a Teflon equilibrium dialysis plate (96-well, 150 μL, half-cell capacity) and cellulose membranes (12–14 kDa molecular weight cut-off) from HT-dialysis (Gales Ferry, Connecticut). Aliquots from the donor and receiver compartments were combined with buffer or blank media to make 10% media (v/v) matrix-matched samples, which were further prepared by the addition of acetonitrile-containing internal standard.
2.9 Sample preparation and liquid chromatography-mass spectrometry analysis
The drug concentration was measured with LC-MS/MS using the HTS CTC PAL autosampler together with Shimadzu pumps for the UHPLC system (Nexera X2) and a QTRAP5500 (AB Sciex) equipped with a TurboIonSpray source for the mass spectrometry. Detections were made in positive or negative ion MRM mode and data processing was conducted with Analyst software (version 1.6.2, SCIEX). ESI† Tables S2 and S3 provides more detailed information about the parameter settings in the MRM mode, analytical columns, mobile phases, sampling volumes, flow rates, and retention times.
For the protein binding assessment the samples were quantified by liquid chromatography coupled with triple quad mass spectrometry. After correcting for dilution during sample work-up, peak area ratios of analyte vs. internal standard were used in the calculation of unbound fraction. All recovery values were in the range 80–120%. No observable volume shift was seen over the duration of incubation and potential volume shifts were therefore not factored into the free fraction calculations.
2.10 Metabolite identification for efavirenz metabolism
Metabolite identification was performed using the same experimental conditions reported for low clearance compounds. The incubation time was 96 h with sampling times were 0, 48, 72, 80, 96 h and number of cells around 300
000 for the three replicates. Each sample was diluted and the 10 μL of supernatant was used for LC-MS/MS analysis. For the instrumental analysis were used: Dionex Ultimate 3000 RS System UHPLC (ThermoFisher), with injection system a PAL HTC xt Autosampler (CTC), and the column Waters Acquity UPLC BEH C18 (1.7 μm, 2.1 × 100 mm). The column temperature was maintained at 40 °C. As chromatography solvent A: 0.1% formic acid in water and solvent B: 0.1% formic acid in acetonitrile with a gradient and flow rate of 0.4 mL min−1. The mass analyser was a ThermoFisher Q Exactive hybrid quadropole-orbitrap mass spectrometer equipped with Xcalibur version 4.2 and the Tune 2.9 software package. The scan event cycle consisted of acquisition of one full mass spectrum (m/z 135 to 1200) at a resolving power of 70
000 (@m/z 200), followed by data-dependent MS/MS scans. The ESI conditions were: spray voltage: 3500 V, probe heater temperature 300 °C, capillary temperature 320 °C, sheath gas 35, and auxiliary gas 10. The measure of the drug compound and its metabolites were based on the peak area intensity. The % peak area of metabolites was estimated by comparison of peak areas of MS ion intensities. This semi-quantitative approach is based on several simplified assumptions (equimolar response of different analytes, no matrix effect, etc.) and consequently, it cannot be excluded that in some cases the semi-quantitative data will be different to data generated by validated bioanalytical methods using individual reference compounds or by structure independent radioactivity measurements. 7-Hydroxyefavirenz (M6) was used as standard to separate it from the regioisomer metabolite 8-hydroxyefavirenz (M1).
2.11 Prediction of in vivo intrinsic clearance
In vitro to in vivo extrapolation (IVIVE) of the metabolic clearance was performed for all compounds that were investigated in this study except irinotecan, ketoprofen and carbazeran. Ketoprofen was excluded due to poor in vitro PK profile. Irinotecan was excluded due to the complex pharmacokinetics involving the lactone and inactive carboxylate forms, which are dependent on the pH and albumin concentration.18 Carbazeran was excluded because of high extrahepatic extraction.19 For compounds that were measured in the repeated incubation experiment, only the CLint derived on the first day was used.
The measured in vitro CLint values were transformed to the predicted unbound in vivo CLint using eqn (9):
|  | (9) |
where the hepatocellularity (HC) was 120 million hepatocytes per gram of liver,
20 LW was the average weight of the liver per kg of body weight which was taken as 21.4 g liver per kg,
21 and fu
inc was the unbound fraction of drugs investigated in the medium.
When no measured unbound fractions in the incubation medium (fuinc) were available (zidovudine, lorazepam, posaconazole, dextromethorphan, repaglinide, and oxazepam), the values were estimated from reported plasma protein binding values, albumin unbound fraction or association constant with BSA (ESI† Table S5). When plasma or human albumin data were used, the compound was assumed to have the same affinity between human albumin and BSA. The fuinc,pred estimated by the plasma unbound fraction eqn (10) was used:
| 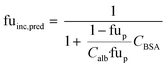 | (10) |
where fu
p is the reported unbound fraction of the drug in the plasma and
Calb the concentration of human albumin in plasma (42.5 g L
−1) and
CBSA is the concentration of BSA in the medium.
The compounds were assigned to the ECCS (extended clearance classification system) using the available literature. Midazolam, dextropmetorphan, repagnalide, quinidine, posaconazole, tolbutamide, and diclofenac classification were collected from Umehara et al.22 Naloxone, zidovudine, lorazepam, and oxazepam from Docci et al.23 and telmisartan from Tess et al.24
2.12 Calculation of observed in vivo intrinsic clearance
In vivo hepatic clearance values were collected from previously published literature reports. Whenever possible, clearance estimates derived after an intravenous administration were used. One exception was dextromethorphan, where oral clearance was used and the intestinal availability and fraction absorbed were set equal to 1.25 Metabolic plasma clearance was calculated from total plasma clearance using eqn (11): | CLH,p (mL min−1 kg−1) = CLp·fmet | (11) |
where CLp is the total metabolic plasma clearance and fmet is the fraction metabolized by hepatic metabolism which considered any extrahepatic or biliary elimination (and assumes no extrahepatic metabolic clearance) as reported in eqn (12): | fmet = 1 − frenal − fbiliary | (12) |
The in vivo blood clearance was estimated by scaling the metabolic plasma clearance with the blood-to-plasma concentration ratio (RBP): | 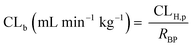 | (13) |
The observed hepatic intrinsic clearance was estimated by assuming the well-stirred model using eqn (14):21 | 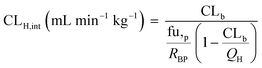 | (14) |
where QH is the average hepatic blood flow of 20.7 mL min−1 kg−1.21
2.13 Software and libraries for modelling
The in silico modelling was performed in R using RxODE for simulations and nlmixr for model fitting. These libraries are open source and available in the R repository CRAN.
The fitting of diclofenac and quinidine data was performed in Phoenix 64 version 8.2.0.4383 (Certara, New Jersey, US) using naïve pooled as the fitting algorithm.
For models 4, 5, and 6 (Fig. 7) an a priori identifiability was performed in the REDUCE language using the open source library DAISY (differential algebra for identifiability of systems).26,27 The technical application and the codes are reported in the ESI† (Modelling session).
Analytical graphs were generated with GraphPad Prism version 7.04 for Windows (GraphPad Software, La Jolla, CA) and in R using the ggplot and gridExtra libraries.
3. Results and discussion
3.1 Seeding efficacy and hepatocyte number
In vitro drug metabolism studies mainly explore the biotransformation and transport of drugs by the cells (in contrast to in vitro pharmacology and toxicology studies that examine the impact of drug exposure on the cells). In this context, quantification of the hepatocyte seeding efficiency and cell number are important assessments to enable quantitative readouts that are normalized by cell number, such as drug clearance and metabolite formation rates. Accurate knowledge of effective cell number is essential for comparing results between in vitro systems, for selection of the most suitable system for a given experiment and for the robustness of the subsequent study design (e.g. selection of time points, length of experiment, and analysis of cell lysates). In parallel to seeding the plates (at day – 4), unused cells were counted and the amount of total hepatocyte protein was determined on seven separate occasions. Protein content was consistently between 1.015 and 1.078 mg per million cells in six occasions with one outlier (1.302 mgtotal protein per million cells; Z-score = 2.4). Linear regression showed a strong correlation (R2 = 0.995) between the amount of total protein and the number of cells, with a mean value of 1.051 ± 0.019 mgtotal protein per million cells (after excluding one outlier Fig. 2A), consistent with other publications.28 The number of hepatocytes at day 2 (i.e. five days after seeding) in the control wells (n = 15) from each experiment (n = 5) was on average 260
000 ± 25
000 (CV: 9.3%) and ranged from 230
000 to 310
000 hepatocytes. Comparison of the number of hepatocytes measured in the control wells at day 2 with the number initially seeded at day – 4 showed an average seeding efficiency of 52.0 ± 7.1% (CV: 14%) with values ranging from 39.0 to 66.5% (Fig. 2B). Seeding efficiency was more consistent within experiments than between experiments. Cell number was therefore determined for each experiment using satellite wells reserved for this purpose. Reasons for the incomplete seeding efficiency are unknown, but are thought to be a combination of partial attachment of hepatocytes to the surface of the scaffold and stress to the hepatocytes during medium exchange. Correction for actual cell number also has important implications for quantitative translation to in vivo considering the commonly reported trend of clearance under-prediction.29
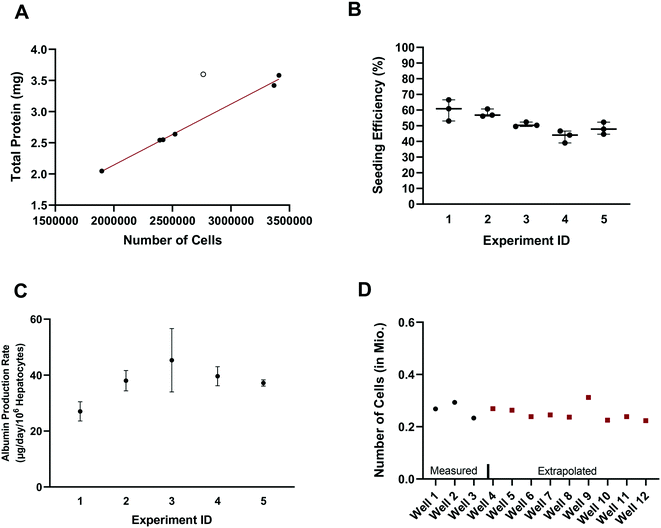 |
| Fig. 2 A) Correlation between total protein and number of cells. Data shown as filled circles were used for the correlation. B) Seeding efficiency of the experiments comparing the initial amount of hepatocytes seeded and the measured total protein amount at day 1 of the experiment in control wells. Number on the x-axis indicates the experiment number. C) Albumin production rate measured in three dedicated control wells in five different experiments as mean ± SD. D) Measured (black dots) and interpolated (red squares) number of cells in different wells in an example experiment. | |
Production of albumin is frequently used as a marker for hepatocyte-like activity. It was explored here as a non-invasive proxy for cell number determination via cell lysis and protein concentration measurement. The mean albumin amount in all experiments (n = 5) and wells (n = 120) was 10.3 ± 3.7 μg (CV: 36%) at day 2 of the experiment (24 h after media exchange). Normalizing the albumin concentration by the measured number of hepatocytes in the control wells (total n = 15), allowed the calculation of the average albumin production rate and was 39 ± 8 μg per day per million cells (CV: 22% with values ranging from 23 to 58 μg per day per million cells). The mean (n = 3) albumin production rate in individual experiments measured in satellite wells was 27 ± 3 (CV: 10%), 38 ± 3 (CV: 8%), 45 ± 9 (CV: 20%), 40 ± 3 (CV: 7%), and 37 ± 1 (CV: 2.5%) μg per day per million cells in experiments 1, 2, 3, 4, and 5, respectively (Fig. 2C). This is at the lower boundary of the estimated albumin output of human liver in vivo of 37 to 105 μg per day per million cells30 but superior to albumin production rates reported for hepatocyte spheroids recently.31 The measurement of hepatocyte number (via hepatocyte protein amount determination in the satellite wells of each experiment) and the albumin production in all wells of an experiment allowed the hepatocyte number in each test well to be calculated and used in data processing, to account for any well to well differences in cell number (Fig. 2D). This approach also compensated for potential differences in albumin production over time for the different experiments as albumin amount per million hepatocytes was calculated in each experiment.
3.2 Assessment of drug metabolizing enzyme activities
At very low drug concentrations the rate of drug metabolism is typically proportional to drug concentration, which therefore decreases exponentially with incubation time. Intrinsic clearance (CLint) is an absolute measurement parameter calculated from fitting an exponential decay function to the drug concentration vs. incubation time data and the parameter of choice for comparison and extrapolation of data between different in vitro systems or between in vitro and in vivo. The intrinsic clearances of four cytochrome P450 (CYP) substrates, seven UDP-glucuronosyl transferase (UGT) substrates, and one aldehyde oxidase (AO) substrate were measured in order to assess the metabolic capabilities of the hepatocyte cultures in the liver-on-a-chip system. Fig. 3 shows the media concentration-time profiles of the drugs. Inter-well variability of the calculated cell number was low and a mean CV of 12% (range from 2 to 21%) was attained. Comparison of measured CLint from the LoC, and leading alternative systems (suspended hepatocytes, and HepatoPac micropatterned cocultured hepatocytes) was preformed and reported in ESI† Table S6. The CLint values were within 2-fold between liver-on-a-chip and HepatoPac for 8 compounds out of 13. When compared with suspended hepatocytes, CLint values for 11 compounds out of 14 were within 2-fold. For a new system using single donor hepatocytes to compare as well as this to highly optimized pooled hepatocyte test systems indicates the promise of the LoC. Non-specific binding can also cause loss of drug from the media onto device surfaces. It was not assessed in detail in this study since little or no adsorption to the plastic was reported in the available literature for the system9,32 and as essentially complete recovery of quinidine and diclofenac was recorded in the quantitative experiments. In addition, the presence of BSA in the media also reduces the potential for compound adsorption.33,34
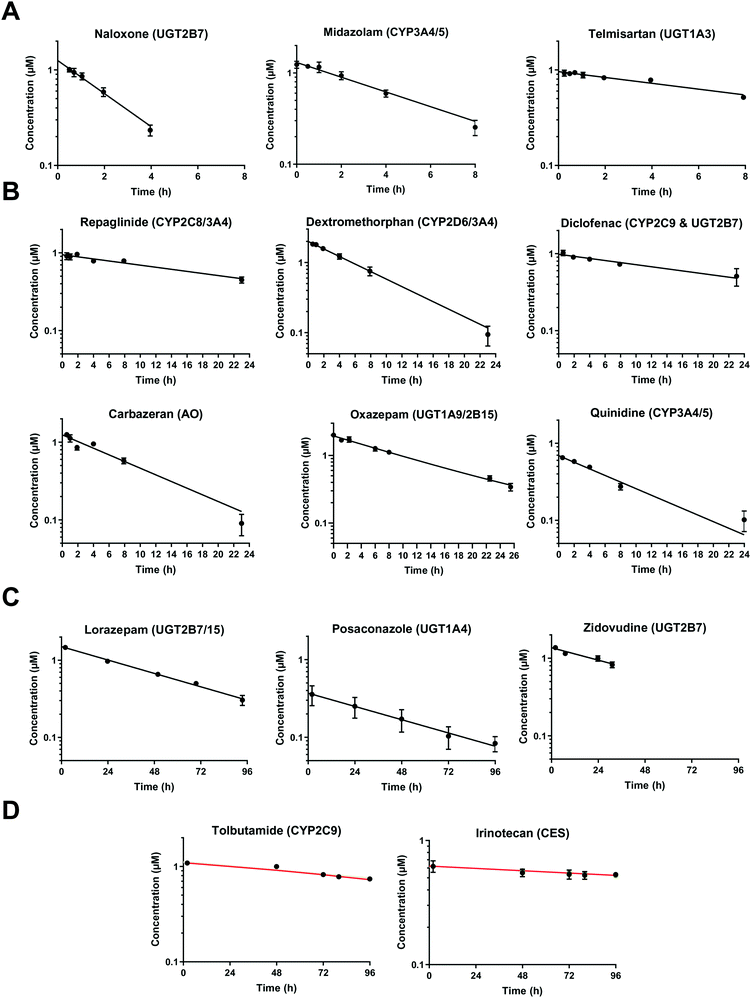 |
| Fig. 3 Drug concentration in media plotted versus incubation time. A) Midazolam, naloxone, and telmisartan were incubated up to 8 h. The sample of naloxone after 4 h were not reported since it was below the limit of quantification. B) Diclofenac, quinidine, repaglinide, dextromethorphan, oxazepam, and carbazeran were incubated for up to 24 h. C) Lorazepam, zidovudine, and posaconazole were investigated for more than 24 h and linear regression was applied for the CLint estimation. D) Low turnover compounds (tolbutamide and irinotecan) were incubated for 96 h and the evaporation model was used to estimate CLint (model fit reported with red line). All data are shown on a log scale as a mean of triplicates with the respective standard deviation. Incubation at day 1 was reported for the compounds tested successively over 4 days (diclofenac, midazolam, quinidine, dextromethorphan, and carbazeran). The specific sampling points for each compound are reported in the ESI.† | |
3.3 Intrinsic clearance determinations for metabolically stable drugs and application of modelling to control for media sampling and evaporation
In vitro drug metabolism studies monitor drug and metabolite concentrations in quenched incubates or media samples. Multiple samples taken from the media compartment of the liver-on-a-chip system were used to create an in vitro PK profile and calculate intrinsic parameters such as drug clearance. Human hepatocyte CLint values are an important selection criterion for drug candidates and are used directly in prediction of human PK. Drugs with low intrinsic clearance (e.g. <3 μL per min per million hepatocytes) often exhibit moderate-high in vivo stability. In contrast, drugs with high intrinsic clearances may have short in vivo half-lives and low bioavailability. Traditional drug metabolism studies using hepatocytes make use of relatively short incubation times and one experimental incubation well per time point which is quenched in its entirety using organic solvent containing internal standard. This treatment largely compensates for evaporation effects because the total drug material is recovered from each well at the end of the experiment. In contrast, organ-on-a-chip systems are likely to have fewer but larger incubation chambers from which multiple samples are removed. Greater consideration of drug amount and concentration within the experiment then becomes important for drug metabolism scientists. The metabolic assessment in the liver-on-a-chip performed here integrates modelling and simulation as a vital part of the study in order to understand in detail what is happening within the experiment and to obtain correct intrinsic clearance estimates. Mathematical modelling was especially needed for the CLint estimations for highly metabolically stable compounds and our study shows the importance of this approach as well as describing under what conditions it is necessary. For the CLint estimation of low turnover compounds an evaporation model was used, which took into account media evaporation (drug concentration and effective cell concentrations increase) and media removal due to sampling (effective cell concentration increases but drug concentration unchanged). The extent of evaporation was measured for each well separately. The individual well rate of evaporation (kev) value was used in determination of individual well drug intrinsic clearance before mean values were calculated (eqn (5), for individual well evaporation rates see ESI† Table S4). Experimental data showed significant evaporation (>20%) over 4 days of incubation. Having established the evaporation rate and effect in the liver-on-a-chip system, in silico modelling was applied to assess the impact of evaporation on the accuracy of the estimated in vitro intrinsic clearance using simulated data (Fig. 4). For the analysis, theoretical concentration-time profiles were simulated using the evaporation model over a range of CLint and kev values which corresponded to those experimentally observed. Subsequently, both the linear model and the evaporation model were used to assess the simulated concentration-time profiles. Fig. 5 shows the possible bias tackled by the use of the evaporation model and more detailed results are reported in the ESI† (Fig. S4). Not accounting appropriately for evaporation from the media results in significant underestimation of the CLint, in particular for low turnover drugs with CLint below 1 μL per min per million cells and the kev above 0.05 μL min−1 (Fig. S4†). With the simulated experiments, we established in which circumstances of CLint and kev the linear model needed to be replaced with the more realistic, but also more complex evaporation model (Fig. 5).
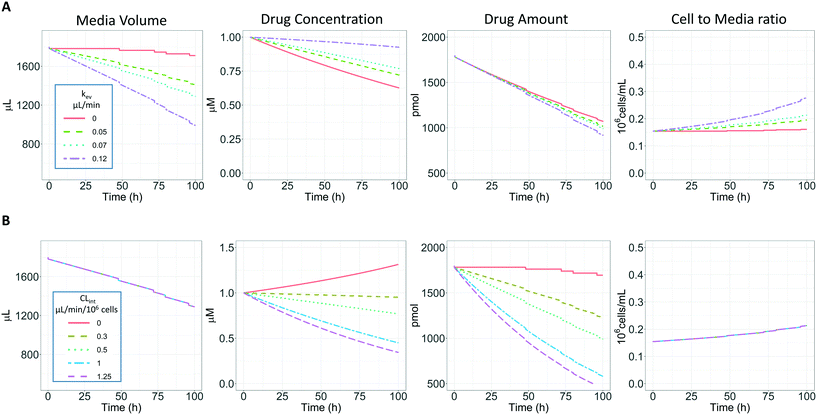 |
| Fig. 4 A) Representation of the effect of evaporation and sampling on the media volume, drug substrate concentration, drug amount and cell to media ratio over 96 h of incubation with PhysioMimix liver-on-a-chip. Four different kev were evaluated (0, 0.05, 0.07, and 0.1 μL min−1) with a CLint of 0.5 μL per min per million cells and a sampling volume of 18 μL. B) Representation of the effect of CLint (0, 0.3, 0.5, 1.0 and 1.25 μL min−1 10−6) on the same variables reported in panel a with a constant kev of 0.07 μL min−1. | |
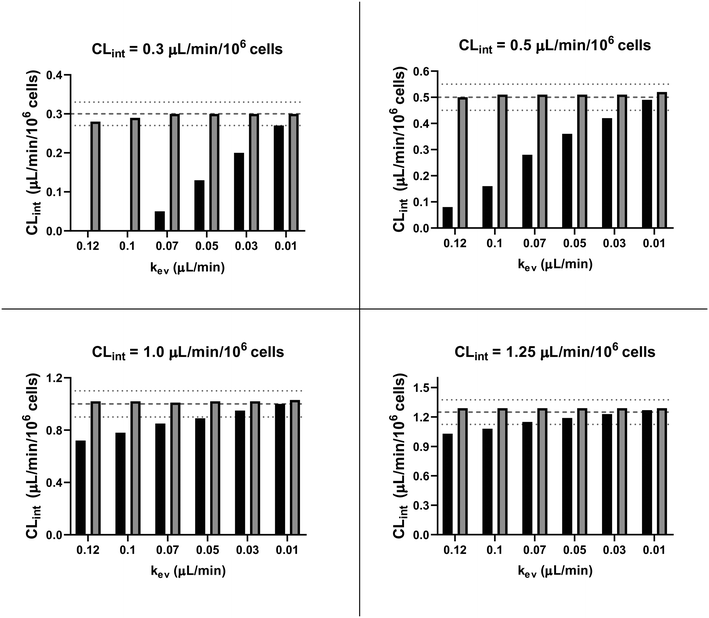 |
| Fig. 5
In silico comparison of the estimated CLint using the linear (black bars) and the evaporation (grey bars) models. The dashed lines represent the expected value for CLint and the dotted lines its variance of ±10%. For CLint value of 0.3 μL per min per million cells with kev of 0.12 and 0.10 μL min−1 the median of the estimated clearance with the linear model was negative and so the respective bars are not reported. | |
In addition, the evaporation model used in the current study also takes into account the media removal due to sampling. Although lorazepam and posaconazole were tested up to 96 h of incubation, the effect of media evaporation was small compared with the rate of drug depletion and the evaporation model did not need to be applied to obtain an accurate CLint estimation. Metabolically stable compounds tested over 96 hours included tolbutamide, irinotecan, and ketoprofen which are CYP2C9,35 carboxylesterase,36 and UGT2B7/UGT1A3 (ref. 37) substrates, respectively. From the experimental data reported in Table 2 and the concentration-time profiles shown in Fig. 3D for tolbutamide and irinotecan the inclusion of the measured constant of evaporation in each well and the application of the evaporation model reduced CLint underestimation which would have occurred using a simple linear model up to ∼40% (additional information available in Table S4†). (Unfortunately ketoprofen concentration-time data showed high bias dependent upon inclusion of day 1 or day 2 values and was therefore not considered for detailed CLint evaluation and IVIVE). It is important to consider that media evaporation is highly dependent on several factors including the surface area of the well, the humidity of the plate/incubator, air flow around the device and the incubation temperature. The extent of evaporation therefore needs to be determined for each new microphysiological system, its impact on the planned experiments assessed and the relevant data interpretation model implemented.
Table 2 Estimated CLint values of metabolically stable drugs using the evaporation model and the linear model. CLint values are expressed in μL per min per million cells and are reported as mean ± standard deviation from three replicates
Compound |
Linear model |
Evaporation model |
CLint (μL per min per million cells) |
Drug depletion may have occurred also by non-enzymatic activity involving the carbamate moiety at the physiological condition of 37 °C and pH of 7.4. Constant of evaporation (kev) for each well were reported in Table S4.†
|
Tolbutamide |
0.41 ± 0.06 |
0.59 ± 0.07 |
Irinotecana |
0.41 ± 0.03 |
0.70 ± 0.02 |
3.4 Extrapolation of in vitro intrinsic clearance to predict in vivo intrinsic clearance
The estimated CLint data for 12 of the compounds measured using the liver-on-a-chip were extrapolated to in vivo hepatic clearance values using physiologically based scaling. Parameters used for the extrapolation of the in vitro clearance data obtained in the liver-on-a-chip for the 12 compounds investigated are reported in Table 1, together with the respective references for the in vivo studies. Understanding the translatability of the in vitro CLint data to in vivo clearance is essential, in order to understand the applicability of the current system and goals for future system enhancements.29,38 Comparison of extrapolated and observed hepatic in vivo CLint values is displayed in Fig. 6. Predicted hepatic intrinsic clearance for 7 out of the 12 substrates used in the analysis (midazolam, quinidine, diclofenac, naloxone, oxazepam, tolbutamide, and lorazepam) were within 3-fold of the observed data. In particular, the prediction was satisfactory for the more permeable compounds cleared from the body via metabolism processes (extended clearance classification system (ECCS) classes 1a and 2). The analysis did not suggest a difference in the prediction success between CYP and UGT substrates.
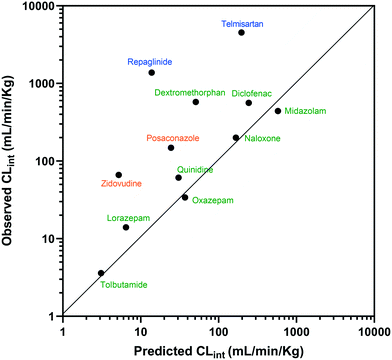 |
| Fig. 6 IVIVE of CLint for 12 compounds tested with the liver-on-a-chip and comparison to observed hepatic clearance. Compounds were coloured according to the ECCS classification (class 1a and 2 in green, class 3 in blue, and class 4 in orange). The solid and dotted lines show the line of unity and the interval of ±3-fold, respectively. | |
Zidovudine, dextromethorphan, repaglinide and telmisartan clearance were under-predicted with in vivo predicted CLint values <10% of the observed value. Telmisartan4 and repaglinide39,40 are substrates of OATP1B3 and OATP1B1, respectively, for which the in vivo clearance is likely to be rate-determined by active hepatic uptake prior to biliary elimination. The under-prediction seen for these drugs is in agreement with trends observed for transporter substrates in other ‘closed’ in vitro cellular systems41 (in which transported drug cannot be eliminated from the test system) and highlights a need for further development of systems capable of mimicking bile flow. Another relevant factor is the use of single donor hepatocytes for the experiments, which may have less than average activities for some metabolic enzymes due to differences in enzyme expression levels or enzyme polymorphisms. The current study demonstrated the application of liver-on-a-chip data for IVIVE of hepatic clearance for a range of CYP and UGT substrates. IVIVE attempts using liver-on-a-chip data have been reported previously, but with a smaller set of drugs.9 More studies are required to investigate the IVIVE of metabolic clearance based on the PhysioMimix liver-on-a-chip by using a hepatocyte pool containing multiple donors (ideally at least 10 donors) if the system is to represent a population average in a similar manner to recently adopted cellular systems such as micropatterned hepatocyte co-cultures.20
3.5 Application of the PhysioMimix liver-on-a-chip to fraction metabolized estimation
The fraction metabolized (fm) by a given enzyme is an important parameter when trying to estimate inter-individual variability in drug exposure or the magnitude of drug–drug interactions (DDI). For example, if a single metabolic enzyme is responsible for 90% of drug clearance (i.e. fm = 0.9) then drug metabolism can be expected to be reduced 10-fold on inhibition of that enzyme pathway, resulting in elevated exposure and potential toxicity. In contrast, if two or more enzymes contribute equally, full inhibition of one enzyme will maximally reduce the rate of drug metabolism only 2-fold. Several reports have shown that in vitro estimated fm can be combined with physiologically based pharmacokinetic (PBPK) modelling for prediction of clinically reported DDIs.42,43 The fm represents a key input parameter in the PBPK modelling and uncertainties in fm estimates are likely to result in poor predictions of the in vivo DDI risk. Determination of fraction metabolized is therefore an important consideration in drug metabolism studies and hepatocyte systems containing a full complement of metabolic enzymes in as physiological environment as possible are the systems of choice for investigating this.
3.5.1 Metabolism pathway analysis for quinidine.
Quantitative measurements of the drug (quinidine) and metabolite (3-hydroxyquinidine) concentration-time profiles on day 1 were used to estimate the CLint values of quinidine and the fraction of quinidine metabolized to form 3-hydroxyquinidine (Fig. 8A). Four different models incorporating different metabolic schemes were investigated (Fig. 7A). The simplest model (1), which considered the formation of 3-hydroxyquinidine as the only metabolic pathway did not describe the observed data accurately (Table 3). While models 2 and 3 which considered the further metabolism of 3-hydroxyquinidine and additional metabolism pathways, respectively, both produced good fits to the observed data and showed significantly better BIC values compared to model 1. The more complex model 4 which represents a combination of models 2 and 3 showed similar BIC compared to that of model 3. Whereas model 4 was structurally globally identifiable from a priori identifiability study, the estimation of CLint of quinidine and the metabolism of 3-hydroxyquinidine had a quite large uncertainty (∼50% for the CLint of 3-hydroxyquinidine) (Table 3). However, the fraction of metabolism proceeding via the 3-hydroxyquinidine formation pathway estimated from models 3 and 4 provided relatively similar values of 0.64, and 0.71, respectively. The analysis of quinidine data suggested the existence of an additional metabolism pathway besides the measured transformation to the 3-hydroxy metabolite, which might be the N-oxide formation44 (model 3). Resolution of which model to use therefore requires further data, for instance measurement of quinidine N-oxide formation or of the further metabolism of 3-hydroxyquinidine in the in vitro system. However, model 3 might be a likely representation of the actual metabolic profile of quinidine, since available literature reported that the 3-hydroxyquinidine is eliminated without further metabolic transformation in the urine.16 In order to better distinguish between the various modelling fitting options, a longer incubation without medium change (>24 h) is recommended for a future study. It is anticipated that with extended incubation time and the quantification of quinidine N-oxide in addition a better resolution of the optimal model to use may be reached.
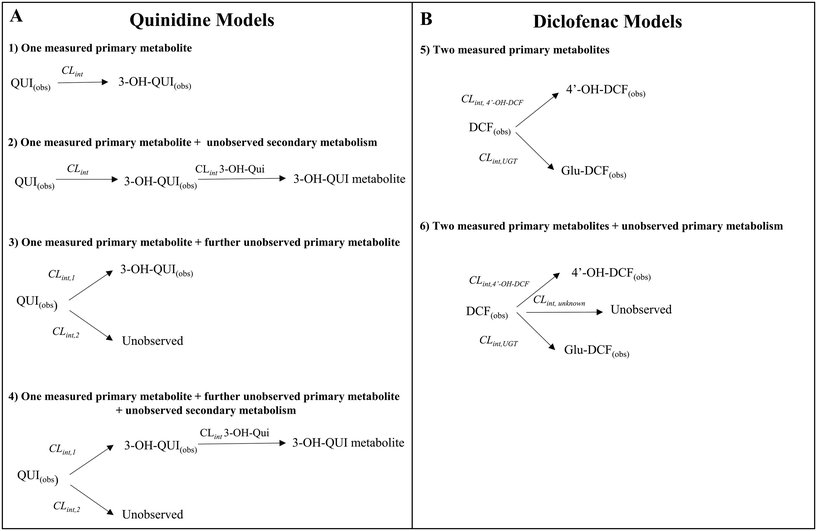 |
| Fig. 7 A) Schematic representation of the metabolic pathways investigated with the in silico model for the experimental data of quinidine. B) In silico models for the fm,UGT and estimation of diclofenac. The subscript Obs refers to the measured medium concentration of the respective compound. | |
Table 3 Four different models were applied to estimate CLint of quinidine to form 3-hydroxyquinidine, an unobserved clearance pathway, and 3-hydroxyquinidine clearance. The respective BIC associated with all four models are displayed. Each value is reported with the respective ± standard error
Model |
BIC |
CLint |
f
m(3-OH-Qui Pathway)
|
Qui. → 3-OH-Qui. |
Qui. → unobserved |
3-OH-Qui → 3-OH-Qui met |
μL per min per million cells |
μL per min per million cells |
μL per min per million cells |
1 |
−65.9 |
8.2 ± 0.5 |
|
|
1.0 |
2 |
−75.2 |
10 ± 2 |
|
4.9 ± 1.0 |
1.0 |
3 |
−82.4 |
7.9 ± 0.4 |
4.4 ± 0.8 |
|
0.64 ± 0.10 |
4 |
−81.5 |
8.6 ± 0.7 |
3.5 ± 1.0 |
2.1 ± 1.2 |
0.71 ± 0.14 |
3.5.2 Fraction metabolized estimation for diclofenac.
A similar investigation was performed with diclofenac which is metabolized to diclofenac-glucuronide mostly by UGT2B7,45 whereas 4′-hydroxydiclofenac and 5′-hydroxydiclofenac46 are formed by CYP2C9 and CYP3A4, respectively, with additional contribution from CYP2C8.46 To our knowledge, we applied for the first time the PhysioMimix liver-on-a-chip in combination with modelling to determine the metabolic contribution of UGTs for diclofenac. The application of the mathematical models enabled simultaneous assessment of different metabolic pathways of diclofenac. Two models (Fig. 7B) were applied to the analysis of diclofenac in vitro data to estimate CLint and fm,UGT. Model 6 (3 primary metabolic pathways, no subsequent metabolism) was the best model to describe the experimental data (Fig. 8B) based on the BIC (Table 4). The total unbound CLint was 95 ± 9 μL per min per million cells and the fm,UGT value was 0.64. The fm,UGT reported by Kilford et al.17 in the HLM was 0.62, in close agreement with the value estimated in this study. One or more unassessed metabolic pathway(s) in addition to 4′-hydroxydiclofenac and diclofenac glucuronide formation were suggested by modelling to potentially also contribute ∼20% of the drug clearance. Possible explanations include formation of 5′-hydroxydiclofenac but it must also be considered that potential inaccuracies in drug and metabolite quantitation could result in a similar effect. This underlines the need for highly accurate quantification of metabolite formation and drug depletion if fm estimates are to be made with confidence.
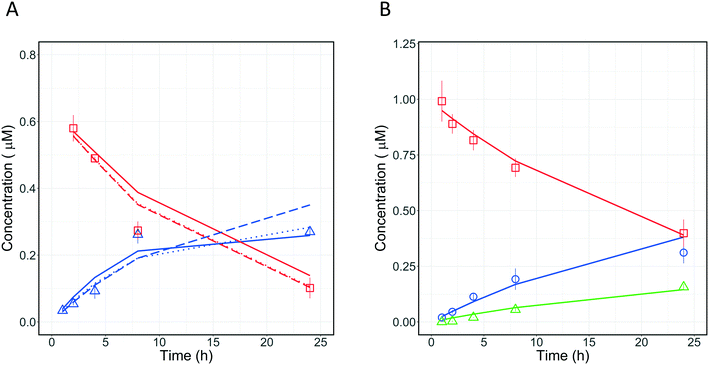 |
| Fig. 8 A) Observed (symbols) and predicted (lines) concentration-time profiles of quinidine and its metabolite 3-hydroxyquinidine on incubation day 1. The experimental data are reported with red squares and blue triangle for quinidine, and 3-hydroxyquinidine, respectively. The fitting profiles from models 2, 3, and 4 are reported with solid, dashed, and dotted line, respectively. B) Comparison of observed (symbols) and predicted (lines) concentration-time profiles of diclofenac and its metabolites 4-hydroxydiclofenac and diclofenac glucuronide on incubation day 1. The experimental data are reported with red squares, blue circles, and green triangles for diclofenac, 4-hydroxydiclofenac, and diclofenac glucuronide, respectively. All experimental data were measured as triplicates and each point represents the mean with the respective standard deviation. | |
Table 4 Two different models were applied to estimate CLint and fm,UGT of diclofenac. CLint values from the observed metabolites and the unobserved metabolic pathway is displayed together with the estimated fm,UGT for both models. The respective BIC associated with models are displayed to indicate the goodness of the fitting. Each value is reported with the respective ± standard error
Model |
BIC |
CLint |
f
m,UGT
|
Observed metabolites |
Unobserved |
μL per min per million cells |
μL per min per million cells |
5 |
−178 |
86 ± 3 |
|
0.80 ± 0.03 |
6 |
−192 |
73 ± 4 |
22 ± 5 |
0.64 ± 0.02 |
3.6 Application to the efavirenz metabolite identification
Establishing what metabolites are generated from a new drug candidate forms an essential part of drug metabolism studies. As with fm estimation, hepatocyte systems which contain a full complement of metabolism enzymes are often used. In vitro determinations of metabolic clearance and metabolite formation for compounds with a high metabolic stability may require extended incubation of the test drug. Standard in vitro systems (e.g. suspended hepatocytes, liver microsomes) have a rapid decrease in metabolic activity, which may prevent the detection of primary, secondary or tertiary metabolites of low clearance drugs. We selected efavirenz as an example drug with low metabolic turnover and quite complex metabolic pathways with various secondary and tertiary metabolites formed both in vitro and in vivo47 (Fig. 9). In addition, efavirenz metabolism represents a good example for metabolite identification investigations because multiple classes of enzymes including CYP isoforms (CYP2B6 with minor contributions from CYPs 1A2, 1A6, 3A4 and 3A5), UGTs (UGT2B7 in the direct N-glucuronidation of efavirenz) and sulfotransferases are involved in the primary and further metabolism of the drug.48 All metabolites reported from in vivo analysis: 8-hydroxyefavirenz (M1), efavirenz-N-glucuronide (M5), 8-hydroxyefavirenz-glucuronide (M4) and 7-hydroxyefavirenz glucuronide (M8) were identified in the liver-on-a-chip system incubates. The metabolite 7-hydroxyefavirenz (M6) was not detected, most likely because of further metabolism, which produced its observed glucuronidated (M8) and sulphated (M7) conjugate metabolites (see ESI† Fig. S5 for time course profiles of efavirenz and all detected metabolites). In addition, secondary metabolism of 8-hydroxyefavirenz to 8-hydroxyefavirenz glucuronide (M4) was detected as well as metabolites formed via the hydroxylation of the cyclopropane moiety of 8-hydroxyefavirenz (M2) and further glucuronidation (M3). The pair of regioisomer metabolites M4–M8 were separated by chromatography, although minimal differences in fragmentation and the absence of standards prevented a definitive identification. As almost all metabolites of efavirenz were detected including metabolites from tertiary metabolism, the liver-on-a-chip system might allow for complex metabolite identification studies for the characterization of the preclinical drug candidates. The large media volume allows larger volumes to be taken for multiple analyses and the cell longevity permits to detect metabolites with low formation rates after extended incubation times.
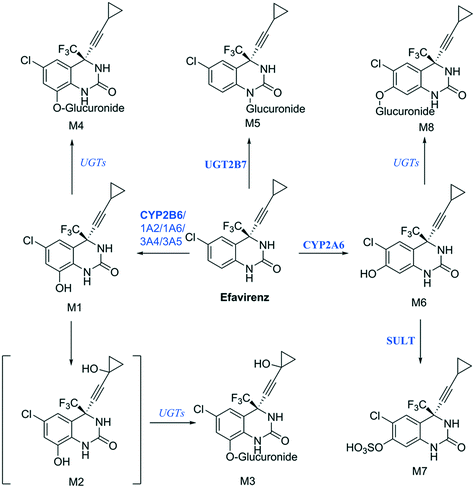 |
| Fig. 9 Proposed efavirenz metabolic pathway from human urine metabolites detection.47 Above each arrow the metabolic enzymes involved in the reaction are reported. The main enzyme isoforms in the metabolism are reported in bold from Cong Xu et al.,48 and in italic when the specific isoform involved was not available or unknown. | |
3.7 Opportunities for further liver-on-a-chip system development
A significant loss of 40–50% of the initial metabolic activity has been reported for 2D-monocultured hepatocytes within less than 24 hours after seeding.1,15 Such a loss of enzyme activity is easily observed in the concentration-time profiles of drugs in the incubations. In each of the intrinsic clearance experiments performed in this study there was no indication of substantive enzyme activity loss during undisturbed incubation, with the potential exception of ketoprofen where the drug depletion profile was less well defined. In order to explore the use of the system further and potentially generate larger amounts of data from the same cells, the retention of drug metabolism capacity on repeated use of the system was assessed for seven drugs using the same cell culture wells but with drug and media replacement each day (see ESI† Fig. S2 and S3 for substrate depletion and metabolite generation profiles, respectively). This experiment may represent a worst case scenario showing the retention of drug metabolizing enzyme activities under harsher cell treatment conditions than were actually applied in the work presented. Nevertheless, it shows the limits of the system usability. It was informative to see that >80% activity was retained on day 2 for all substrates tested, whereas with extended culture time and repeated media exchange the activity was decreased on subsequent days. For substrates mainly metabolized by CYP enzymes (midazolam, dextromethorphan, repaglinide, and quinidine) or AO (carbazeran), the activity at day 4 was between 47–51% of that on day 1, whereas the activity for UGT substrates was better retained with 62% retention for diclofenac and 70% for naloxone (Fig. S2†). These findings were not consistent with the linear in vitro PK profiles observed for lorazepam, posaconazole or tolbutamide, incubated over 4 days in undisturbed culture and suggest that daily media removal and replacement may affect the enzyme activities of the hepatocytes. In the case of ketoprofen it is possible that activity loss during extended culture affected the concentration-time profile and therefore intrinsic clearance determination and so it was not used for IVIVE assessments. Decreasing activity in oxidative metabolism was observed in a previous study conducted by Rubiano and colleagues, who reported an approximately 50% decrease in CYP3A4 activity over the first 10 days of culture.12 Culturing of hepatocytes as spheroids was also subject to activity loss over time,49 and the choice of medium was highlighted as relevant to retain a stable metabolic activity. Hepatocytes benefit from co-culture with other cells, as exemplified by the HepatoPac or HμREL cultures.50,51 Both of these systems involve co-culture with fibroblasts, which enable the hepatocytes to survive for multiple weeks with regular media exchange or for up to one week in the absence of media replenishment.51 Building upon the demonstrated culture benefits of media flow shown in the liver on a chip system, co-culture of hepatocytes in the PhysioMimix system may therefore represent a potential next step in further system development for DMPK applications.
4. Conclusion
Testing of microphysiological systems to establish their cellular performance, suitability for use with today's drug molecules and validation for the proposed application are needed if the systems are to contribute to drug candidate optimization and selection in the pharmaceutical industry. The current investigations demonstrated that measurement of seeding efficiency and quantification of the active cell number using biomarkers such as albumin were essential for quantitative understanding of the system. Having passed basic system suitability assessments, more advanced studies were conducted to explore the merit of the system for DMPK studies. Mathematical modelling of the data enabled more correct results to be obtained by accounting for relevant evaporation from the media over time, particularly for metabolically stable drugs, and enabled simultaneous determinations of clearance and fraction metabolized values. Extrapolation of the in vitro clearance to in vivo clearance demonstrated that the system already offers reasonable human translatability, although not exceeding that of leading hepatocyte co-culture systems. This work represents a step along the path of liver-on-a-chip optimization and highlights some of the validation aims and approaches relevant to a drug metabolism setting in the pharmaceutical industry. In order to elicit its full potential, future adaptations of the liver-on-a-chip can be envisaged which would further extend the lifetime of drug metabolizing enzyme activities, assess ability to capture transporter-mediated clearance and make use of pooled hepatocyte cultures as representative of population average activities. This should then enable application of liver-on-a-chip to 1) more precise CLint determination for low clearance drugs, 2) prediction of pharmacokinetic variability and drug–drug interactions (in particular in case of metabolism-transporter interplay) and 3) more confidence in detection of active or toxic metabolites prior to clinical development.
Author contributions
Participated in research design: Docci, Milani, Gertz, Galetin, Parrott, Fowler. Conducted experiments: Docci, Milani, Ramp. Performed data analysis: Docci, Milani, Ramp, Romeo, Gertz, Parrott, Fowler. Wrote or contributed to the writing of the manuscript: Docci, Milani, Romeo, Godoy, Ortiz Franyuti, Krähenbühl, Gertz, Galetin, Parrott, Fowler.
Conflicts of interest
NM, TR, AAR, PG, DOF, MG, NP and SF are employees of F. Hoffmann-La Roche or were employees of F. Hoffmann-La Roche at the time this work was performed. SK is an employee of the University of Basel. AG is an employee of the University of Manchester. The authors do not have any conflicts of interest to declare.
Acknowledgements
Technical support and guidance from Isabelle Walter, Evelyne Durr, Nathalie Schaub, NaHong Qiu and Aynur Ekiciler is gratefully acknowledged.
References
- C. M. Smith, C. K. Nolan, M. A. Edwards, J. B. Hatfield, T. W. Stewart, S. S. Ferguson, E. L. Lecluyse and J. Sahi, J. Pharm. Sci., 2012, 101, 3989–4002 CrossRef CAS PubMed.
- S. Fowler, W. L. K. Chen, D. B. Duignan, A. Gupta, N. Hariparsad, J. R. Kenny, W. G. Lai, J. Liras, J. A. Phillips and J. Gan, Lab Chip, 2020, 20, 446–467 RSC.
- E. Kimoto, R. Li, R. J. Scialis, Y. Lai and M. V. Varma, Mol. Pharmaceutics, 2015, 12, 3943–3952 CrossRef CAS PubMed.
- T. De Bruyn, A. Ufuk, C. Cantrill, R. E. Kosa, Y.-A. Bi, M. Niosi, S. Modi, A. D. Rodrigues, L. M. Tremaine and M. V. Varma, Drug Metab. Dispos., 2018, 46, 989–1000 CrossRef CAS PubMed.
- W. R. Proctor, A. J. Foster, J. Vogt, C. Summers, B. Middleton, M. A. Pilling, D. Shienson, M. Kijanska, S. Strobel, J. M. Kelm, P. Morgan, S. Messner and D. Williams, Arch. Toxicol., 2017, 91, 2849–2863 CrossRef CAS PubMed.
- M. L. Sutherland, K. M. Fabre and D. A. Tagle, Stem Cell Res. Ther., 2013, 4(Suppl 1), I1 Search PubMed.
- E. W. Esch, A. Bahinski and D. Huh, Nat. Rev. Drug Discovery, 2015, 14, 248–260 CrossRef CAS PubMed.
-
https://cn-bio.com/, (access August 2021).
- N. Tsamandouras, T. Kostrzewski, C. L. Stokes, L. G. Griffith, D. J. Hughes and M. Cirit, J. Pharmacol. Exp. Ther., 2017, 360, 95–105 CrossRef CAS PubMed.
- A. Vivares, S. Salle-Lefort, C. Arabeyre-Fabre, R. Ngo, G. Penarier, M. Bremond, P. Moliner, J.-F. Gallas, G. Fabre and S. Klieber, Xenobiotica, 2015, 45, 29–44 CrossRef CAS PubMed.
- U. Sarkar, K. C. Ravindra, E. Large, C. L. Young, D. Rivera-Burgos, J. Yu, M. Cirit, D. J. Hughes, J. S. Wishnok, D. A. Lauffenburger, L. G. Griffith and S. R. Tannenbaum, Drug Metab. Dispos., 2017, 45, 855–866 CrossRef PubMed.
- A. Rubiano, A. Indapurkar, R. Yokosawa, A. Miedzik, B. Rosenzweig, A. Arefin, C. M. Moulin, K. Dame, N. Hartman and D. A. Volpe, Clin. Transl. Sci., 2020, 14, 1049–1061 CrossRef PubMed.
- N. Tsamandouras, W. L. K. Chen, C. D. Edington, C. L. Stokes, L. G. Griffith and M. Cirit, AAPS J., 2017, 19, 1499–1512 CrossRef CAS PubMed.
- M. Tachibana, M. Tanaka, Y. Masubuchi and T. Horie, Drug Metab. Dispos., 2005, 33, 803 CrossRef CAS PubMed.
- L. Docci, N. Parrott, S. Krahenbuhl and S. Fowler, SLAS Discovery, 2019, 24, 523–536 CrossRef CAS PubMed.
- F. Nielsen, K. K. Nielsen and K. Brøsen, J. Chromatogr. B: Biomed. Sci. Appl., 1994, 660, 103–110 CrossRef CAS PubMed.
- P. J. Kilford, R. Stringer, B. Sohal, J. B. Houston and A. Galetin, Drug Metab.
Dispos., 2009, 37, 82–89 CrossRef CAS PubMed.
- M. I. Blonk, C. C. Langemeijer, A. P. Colbers, K. E. Hoogtanders, R. H. van Schaik, B. J. Schouwenberg and D. M. Burger, Antiviral Ther., 2016, 21, 143–152 CrossRef CAS PubMed.
- B. Kaye, J. Offerman, J. Reid, H. Elliott and W. Hillis, Xenobiotica, 1984, 14, 935–945 CrossRef CAS PubMed.
- L. Docci, F. Klammers, A. Ekiciler, B. Molitor, K. Umehara, I. Walter, S. Krähenbühl, N. Parrott and S. Fowler, AAPS J., 2020, 22, 1–12 CrossRef PubMed.
- M. Gertz, A. Harrison, J. B. Houston and A. Galetin, Drug Metab. Dispos., 2010, 38, 1147–1158 CrossRef CAS PubMed.
- K. Umehara, C. Cantrill, M. B. Wittwer, E. Di Lenarda, F. Klammers, A. Ekiciler, N. Parrott, S. Fowler and M. Ullah, Drug Metab. Dispos., 2020, 48, 849–860 CrossRef CAS PubMed.
- L. Docci, F. Klammers, A. Ekiciler, B. Molitor, K. Umehara, I. Walter, S. Krähenbühl, N. Parrott and S. Fowler, AAPS J., 2020, 22, 131 CrossRef CAS PubMed.
- D. A. Tess, H. Eng, A. S. Kalgutkar, J. Litchfield, D. J. Edmonds, D. A. Griffith and M. V. Varma, J. Med. Chem., 2020, 63, 11831–11844 CrossRef CAS PubMed.
- H. S. Brown, M. Griffin and J. B. Houston, Drug Metab. Dispos., 2007, 35, 293–301 CrossRef CAS PubMed.
-
M. P. Saccomani and L. D'angiò, IFAC Proceedings Volumes, 2009, vol. 42, pp. 48–53 Search PubMed.
- G. Bellu, M. P. Saccomani, S. Audoly and L. D'Angiò, Comput. Methods Programs Biomed., 2007, 88, 52–61 CrossRef PubMed.
- N. A. Kratochwil, C. Meille, S. Fowler, F. Klammers, A. Ekiciler, B. Molitor, S. Simon, I. Walter, C. McGinnis, J. Walther, B. Leonard, M. Triyatni, H. Javanbakht, C. Funk, F. Schuler, T. Lavé and N. J. Parrott, AAPS J., 2017, 19, 534–550 CrossRef CAS PubMed.
- F. L. Wood, J. B. Houston and D. Hallifax, Drug Metab. Dispos., 2017, 45, 1178–1188 CrossRef CAS PubMed.
- A. R. Baudy, M. A. Otieno, P. Hewitt, J. Gan, A. Roth, D. Keller, R. Sura, T. R. Van Vleet and W. R. Proctor, Lab Chip, 2020, 20, 215–225 RSC.
- J. Riede, B. M. Wollmann, E. Molden and M. Ingelman-Sundberg, Drug Metab. Dispos., 2021, 49, 501–508 CrossRef CAS PubMed.
- P. M. van Midwoud, A. Janse, M. T. Merema, G. M. Groothuis and E. Verpoorte, Anal. Chem., 2012, 84, 3938–3944 CrossRef CAS PubMed.
- J. I. Wakayama, H. Sekiguchi, S. Akanuma, T. Ohtani and S. Sugiyama, Anal. Biochem., 2008, 380, 51–58 CrossRef CAS PubMed.
- S. I. Kovalchuk, N. A. Anikanov, O. M. Ivanova, R. H. Ziganshin and V. M. Govorun, Anal. Chim. Acta, 2015, 893, 57–64 CrossRef CAS PubMed.
- C. R. Lee, J. A. Pieper, A. L. Hinderliter, R. F. Frye, J. A. Blaisdell and J. A. Goldstein, J. Clin. Pharmacol., 2003, 43, 84–91 CrossRef CAS PubMed.
- M. Femke, A. K. Goey, R. H. van Schaik, R. H. Mathijssen and S. Bins, Clin. Pharmacokinet., 2018, 57, 1229–1254 CrossRef PubMed.
- A. Di Marco, M. D'Antoni, S. Attaccalite, P. Carotenuto and R. Laufer, Drug Metab. Dispos., 2005, 33, 812–819 CrossRef CAS PubMed.
-
A. Galetin, in Enzyme Kinetics in Drug Metabolism, Springer, 2014, pp. 255–288 Search PubMed.
- C. Säll, J. B. Houston and A. Galetin, Drug Metab. Dispos., 2012, 40, 1279–1289 CrossRef PubMed.
- M. Gertz, N. Tsamandouras, C. Säll, J. B. Houston and A. Galetin, Pharm. Res., 2014, 31, 2367–2382 CrossRef CAS PubMed.
- K. Ménochet, K. E. Kenworthy, J. B. Houston and A. Galetin, J. Pharmacol. Exp. Ther., 2012, 341, 2 CrossRef PubMed.
- M. Rowland, C. Peck and G. Tucker, Annu. Rev. Pharmacol. Toxicol., 2011, 51, 45–73 CrossRef CAS PubMed.
- E. Luzon, K. Blake, S. Cole, A. Nordmark, C. Versantvoort and E. G. Berglund, Clin. Pharmacol. Ther., 2017, 102, 98–105 CrossRef CAS PubMed.
- T. L. Nielsen, B. B. Rasmussen, J.-P. Flinois, P. Beaune and K. Brøsen, J. Pharmacol. Exp. Ther., 1999, 289, 31–37 CAS.
- K. E. Lazarska, S. J. Dekker, N. P. Vermeulen and J. N. Commandeur, Toxicol. Lett., 2018, 284, 70–78 CrossRef CAS PubMed.
- T. Wei, Curr. Drug Metab., 2003, 4, 319–329 CrossRef PubMed.
- A. Mutlib, H. Chen, G. Nemeth, J. Markwalder, S. Seitz, L. Gan and D. Christ, Drug Metab. Dispos., 1999, 27, 1319–1333 CAS.
- C. Xu, S. K. Quinney, Y. Guo, S. D. Hall, L. Li and Z. Desta, Drug Metab. Dispos., 2013, 41, 2004–2011 CrossRef CAS PubMed.
- K. P. Kanebratt, A. Janefeldt, L. Vilén, A. Vildhede, K. Samuelsson, L. Milton, A. Björkbom, M. Persson, C. Leandersson and T. B. Andersson, J. Pharm. Sci., 2020, 110, 422–431 CrossRef PubMed.
- P. Chao, T. Maguire, E. Novik, K.-C. Cheng and M. Yarmush, Biochem. Pharmacol., 2009, 78, 625–632 CrossRef CAS PubMed.
- U. Zanelli, N. P. Caradonna, D. Hallifax, E. Turlizzi and J. B. Houston, Drug Metab. Dispos., 2012, 40, 104–110 CrossRef CAS PubMed.
- M. Zientek, Y. Jiang, K. Youdim and R. S. Obach, Drug Metab. Dispos., 2010, 38, 1322–1327 CrossRef CAS PubMed.
- A. Yu and R. L. Haining, Drug Metab. Dispos., 2001, 29, 1514–1520 CAS.
- W. Tang, Curr. Drug Metab., 2003, 4, 319–329 CrossRef CAS PubMed.
- V. Uchaipichat, C. Suthisisang and J. O. Miners, Drug Metab. Dispos., 2013, 41, 1273 CrossRef CAS PubMed.
- Y. S. Lin, A. L. Dowling, S. D. Quigley, F. M. Farin, J. Zhang, J. Lamba, E. G. Schuetz and K. E. Thummel, Mol. Pharmacol., 2002, 62, 162–172 CrossRef CAS PubMed.
- M. H. Court, S. X. Duan, C. Guillemette, K. Journault, S. Krishnaswamy, L. L. Von Moltke and D. J. Greenblatt, Drug Metab. Dispos., 2002, 30, 1257–1265 CrossRef CAS PubMed.
- A. Ghosal, N. Hapangama, Y. Yuan, J. Achanfuo-Yeboah, R. Iannucci, S. Chowdhury, K. Alton, J. E. Patrick and S. Zbaida, Drug Metab. Dispos., 2004, 32, 267 CrossRef CAS PubMed.
- T. L. Nielsen, B. B. Rasmussen, J.-P. Flinois, P. Beaune and K. Brøsen, J. Pharmacol. Exp. Ther., 1999, 289, 31 CAS.
- T. B. Bidstrup, I. Bjørnsdottir, U. G. Sidelmann, M. S. Thomsen and K. T. Hansen, Br. J. Clin. Pharmacol., 2003, 56, 305–314 CrossRef CAS PubMed.
- A. Yamada, K. Maeda, N. Ishiguro, Y. Tsuda, T. Igarashi, T. Ebner, W. Roth, S. Ikushiro and Y. Sugiyama, Pharmacogenet. Genomics, 2011, 21, 523–530 CrossRef CAS PubMed.
- O. Barbier, D. Turgeon, C. Girard, M. D. Green, T. R. Tephly, D. W. Hum and A. Bélanger, Drug Metab. Dispos., 2000, 28, 497–502 CAS.
- J. D. Lutz and N. Isoherranen, Drug Metab. Dispos., 2012, 40, 159–168 CrossRef CAS PubMed.
- A. R. Silva and R. J. Dinis-Oliveira, Drug Metab. Rev., 2020, 52, 258–282 CrossRef CAS PubMed.
Footnotes |
† Electronic supplementary information (ESI) available. See DOI: 10.1039/d1lc01161h |
‡ Current Affiliation: Idorsia Pharmaceuticals Ltd, Hegenheimermattweg 91, 4123, Allschwil, Switzerland. |
§ These authors contributed equally to this work. |
|
This journal is © The Royal Society of Chemistry 2022 |
Click here to see how this site uses Cookies. View our privacy policy here.