Fate of influent microbial populations during medium chain carboxylic acid recovery from brewery and pre-fermented food waste streams†
Received
12th September 2021
, Accepted 10th December 2021
First published on 24th December 2021
Abstract
Chain elongation is an emerging biotechnology for medium chain carboxylic acid (MCCA) production from diverse waste streams. Food and brewery waste were upgraded to MCCAs using mixed-culture microbial communities in an anaerobic sequencing batch reactor. A maximum MCCA volumetric production rate of 9.1 mmole L−1 d−1 (1.1 g L−1 d−1) was achieved with caproate as the major MCCA. MCCA toxicity induced at acidic pH limited greater MCCA production. Microbial community dynamics were investigated using time-series 16S rRNA gene (referred to as rDNA) and 16S rRNA sequencing data. The relative activities (as determined by 16S rRNA sequencing) of microbial populations belonging to the Clostridiales order and Pseudoramibacter genus positively correlated to MCCA production. The ratio of relative activity and relative abundance (rRNA/rDNA) and a mass balance-based approach to calculate specific growth rates were used to identify influent populations that were active in the bioreactor. Some of the most abundant and active microbial populations in the influent (e.g., Prevotella) were less active in the bioreactor. On the other hand, chain elongating microbial groups (Clostridiales and Pseudoramibacter) were enriched in the bioreactor even though they were present at low relative abundances and activities in the influent, suggesting that selection dominated bioreactor community assembly. The approaches developed in this study allow identification of active and inactive microbial populations, which will improve the linkage of process performance with microbial community structure during process modeling.
Water impact
Food and brewery waste were fed to an anaerobic bioreactor to produce high value platform chemicals. These waste streams contain microbial populations that are introduced to the bioreactor, but their contribution to bioreactor process performance has so far been overlooked. Distinguishing active and inactive influent microbial populations in the bioreactor will contribute to the management of microbial communities for desired outputs.
|
1. Introduction
Global municipal solid waste generation is expected to increase from 2.01 to 2.59 billion tons per year from 2016 to 2050, with a concurrent increase in waste management costs and human and environmental health impacts.1 To address this concern, efforts to divert waste from landfills and reduce anthropogenic methane emissions have been implemented in different parts of the world,2,3 but low-cost technologies that can convert organic waste streams into high-value products remain elusive. Chain elongation of short chain carboxylic acids (SCCAs) using mixed microbial communities for production of medium chain carboxylic acids (MCCAs) from waste streams may provide such an opportunity. MCCAs are saturated fatty acids with chain lengths from six to twelve carbons including one carboxyl group and MCCAs with up to eight carbons have been produced by microbially mediated chain elongation processes. They are used as platform chemicals for the manufacturing of pharmaceuticals, fragrances, lubricants, rubbers, dyes, and liquid biofuels or can be used directly as livestock feed additives and antimicrobials.4,5 They are currently produced from plant based oils with considerable environmental impacts.6–8
Chain elongation involves the oxidation of an electron donor, such as ethanol, which also serves as a source of carbon, reducing equivalents (NADH), and energy (ATP). Ethanol chain elongation includes ethanol oxidation to acetyl-CoA and chain elongation of SCCAs via reverse β oxidation.5 SCCAs and electron donors required for MCCA production can be added from an exogenous source, may be present in the substrate, or can be produced in situ as an intermediate during fermentation. Several chain elongation studies have used crude ethanol to mediate chain elongation,9–15 while others have utilized waste ethanol such as yeast fermentation beer,4,16–18 wine lees,19 and syngas effluent.20 Some of these previous studies used pre-fermented food waste as a source of SCCAs and crude ethanol as the electron donor, but the use of pre-fermented food waste combined with waste ethanol has not been studied yet. Upgrading ethanol-containing brewery waste into MCCAs may be a promising option, given that the rise in breweries21,22 has brought new challenges related to infrastructure and waste management.23,24 Considerable opportunities exist to diversify brewery waste treatment practices and recover resources from the various organic-rich waste streams generated in breweries. Deriving ethanol from brewery waste would not only eliminate the cost and environmental impact of using crude ethanol in chain elongation,25 but would also be beneficial for brewery waste treatment. A potentially under-appreciated brewery waste stream is waste beer, which is produced at a rate of 2 to 19% of the brewery volumetric beer production (Doug Knox, Sustainability Manager, Jolly Pumpkin Brewery, MI, personal communication). Waste beer is a waste stream produced as a result of faulty bottling, development of off-flavors, improper fermentation, incorrect storage, or beer returned from the market because it is close to or beyond its expiration date.26
To develop microbial-based management of bioengineered systems, such as chain elongation bioreactors, we need to understand how microbial communities respond to different operational and environmental conditions. Previous chain elongation studies that have used heterogeneous waste streams have focused on studying the bioreactor microbial community only.19,27,28 However, when waste streams are used as the influent substrate, microorganisms continuously enter the chain elongation bioreactor as waste streams contain their own microbial communities. Influent microbial communities and their roles have been studied for anaerobic digesters and activated sludge systems.29–33 Mei et al.30 reported that a large fraction of the microbial populations originating from the influent were inactive in the downstream system and removing the inactive immigrant populations from their data analysis improved predictions of operating parameters that influenced process performance. Thus, to accurately link microbial community data with bioreactor conditions and performance data, it is important to identify the microbial immigrants that remain active and affect process performance and stability. While some chain elongation studies have analyzed the microbial community in one or more influent sample,34–36 a detailed characterization of influent microbial community dynamics and how such dynamics impact the chain elongation bioreactor have not yet been explored.
Most chain elongation microbiome studies have used 16S ribosomal (rRNA) gene sequencing to gain insights into the microbial community governing chain elongation systems.13,16,19,20,27,28 However, this DNA-based approach also targets 16S rRNA genes associated with inactive cells as well as extracellular DNA37 resulting in a biased understanding of which populations are active. The half-life of RNA is much shorter than that of DNA and monitoring the 16S rRNA abundance of specific microbial populations can be used to infer their overall activity,38 as long as the well-described limitations of this approach are addressed.39–41
The objective of this study was to evaluate the fate of influent microbial populations in a chain elongation bioreactor fed with ethanol-rich waste beer and pre-fermented food waste for MCCA production without exogenous ethanol addition. The chain elongating microbial community dynamics in the influent and the bioreactor were investigated using a combination of 16S rRNA and 16S rRNA gene sequencing data. We calculated the ratio of relative activity and relative abundance (rRNA/rDNA) and specific growth rate of individual influent populations to distinguish the inactive influent populations from the active ones in the chain elongating bioreactor.
2. Materials and methods
2.1. Experimental setup
A 7 L semi-continuous anaerobic sequencing batch reactor (ASBR) with a working volume of 5 L was operated for 229 days on a 24 h cycle consisting of four steps: i) feeding (8–10 min), ii) continuous mixing and pH adjustment (22 h 40 min), iii) settling (1 h), and iv) decanting for withdrawal of effluent equal to the volume of the influent (8–10 min) (Fig. S1†). The ASBR was controlled remotely by LabVIEW (National Instruments, Austin, TX, USA) data acquisition software. The bioreactor headspace was connected to a 5 L Tedlar gas bag. The bioreactor was equipped with a water jacket connected to a recirculating bath (Polyscience, Niles, IL, USA) for temperature control and was operated at 40 °C until day 73 and at 37 °C for the remainder of the time. The temperature was decreased to 37 °C to be closer to the optimal growth temperature of several chain elongating species, such as Clostridium kluyveri, Clostridium sp. BS-1, Eubacterium pyruvativorans, Eubacterium limosum, and Megasphaera elsdenii.5,42,43 The bioreactor pH was maintained at slightly acidic conditions (pH 5.5 ± 0.1) to minimize methanogenesis through automatic addition of 3 M NaOH using LabVIEW during the well-mixed react phase. The bioreactor was operated at a hydraulic retention time (HRT) of 2–4 days (Fig. S2†) and an organic loading rate (OLR) of 10.5 ± 7.0 g soluble chemical oxygen demand (sCOD) L−1 d−1 (Fig. S2†).
2.2. Inoculum and influent substrate
5 L of rumen content (17.1 ± 1.0 g volatile solids (VS) L−1) collected from the rumen of a fistulated cow from a dairy farm at Michigan State University (East Lansing, MI, USA) was added to the ASBR and allowed to degasify for 48 h before starting to feed the bioreactor. 2.5 L of fresh rumen content was again added to the bioreactor on day 178 after an accidental loss of biomass. The bioreactor was fed once a day with a mixture of waste beer collected from Jolly Pumpkin Brewery (Dexter, MI, USA) and permeate produced by an acidogenic dynamic membrane bioreactor treating food waste44 containing high levels (62.1 ± 11.0 mM) of SCCAs. The chemical characteristics of the inoculum, waste beer, and permeate are summarized in Table S1.† The acidogenic food waste bioreactor was inoculated with rumen content, which had been degasified for 24 h before startup, and operated at a pH of 6.3 ± 0.1, a temperature of 39 °C, an HRT of 5–20 h, a solids retention time (SRT) of 40–90 h, and an OLR of 18 g VS L−1 d−1. Permeate was collected once per week from the acidogenic bioreactor, stored at 4 °C in a tightly closed container until use, and mixed with waste beer for influent substrate preparation. The SCCAs and ethanol present in permeate and waste beer were considered to determine the ratio of both substrates. The amount of ethanol required for chain elongation of SCCAs was calculated according to the chain elongation stoichiometric equations5 (4
:
1 for ethanol
:
acetate, 2.4
:
1 for ethanol
:
propionate, 1.2
:
1 ethanol
:
butyrate, and 1.2
:
1 ethanol
:
valerate) given in Table S2† except during the first three weeks when the ethanol
:
acetate ratio in the influent was 9
:
1.
2.3. Chemical analyses
We collected samples for various chemical analyses to assess the ASBR performance. Inoculum samples were collected at the time of inoculation (day 0 and day 178) and influent samples were collected once a week. Bioreactor content samples were collected once a week during the ASBR mixing phase while effluent samples were collected two to three times a week during the ASBR settling phase. Total solids (TS) and VS, total suspended solids (TSS), and volatile suspended solids (VSS) analyses were determined according to standard methods.45 The sCOD analysis was performed using Lovibond™ medium-range (0–1500 mg L−1) COD digestion vials (Tintometer, Germany). Gas volume was measured every day with a 100 mL gas-tight glass syringe, while gas composition (H2, CO2, and CH4) was determined two to three times a week using a Gow-Mac Series gas chromatograph (Bethlehem, PA, USA) equipped with a thermal conductivity detector (TCD). The temperature of the column, injector, and detector were set to 104 °C, 80 °C, and 115 °C, respectively, and the current was set at 120 mA. Hydrogen was used as the carrier gas for carbon dioxide and methane measurements, while nitrogen was used as the carrier gas to measure hydrogen. Standard gas samples (ShopCross, Greensboro NC, USA) with varying mixtures of methane, carbon dioxide, and hydrogen were used for GC-TCD calibration.
Concentrations of carboxylates from C2 to C8, including iso-forms of C4 and C5, and ethanol were determined using an Agilent Technologies 7890B gas chromatograph (Santa Clara, CA) equipped with a Stabilwax-DA column (Restex) and a flame ionization detector. The oven temperature was held at 55 °C for 1 min, then increased to 205 °C at 10 °C min−1, and held at 205 °C for 8 min. Injector and detector temperatures were set to 250 °C and 300 °C, respectively, and nitrogen was used as the carrier gas. The liquid samples were acidified with phosphoric acid for GC-FID analysis and with sulfuric acid for sCOD analysis, centrifuged, and filtered through 0.45 μm nylon membrane filters (TISCH Scientific, North Bend, OH, USA) before analyses.
2.4. Calculations
In this study, the undissociated carboxylic acid and the corresponding dissociated carboxylate are together referred to as carboxylate. Furthermore, the terms MCCAs and SCCAs, respectively, refer to the sum of caproate (C6), enanthate (C7), and caprylate (C8) and the sum of acetate (C2), propionate (C3), n-butyrate (C4), and n-valerate (C5); the concentrations of iso-butyrate and iso-valerate values were negligible and hence excluded from the results unless stated explicitly. The SCCA and MCCA results are expressed on a molar basis and are net values calculated after subtracting the concentration in the influent from the corresponding concentration measured in the effluent. The volumetric production rate (mmole L−1 d−1) was determined by dividing the bioreactor effluent concentrations (mmole L−1) by the corresponding HRT (d; working volume (L) divided by effluent flow rate (L d−1)). The product yield was calculated by dividing soluble oxygen demand (sCOD) of MCCAs produced in the bioreactor by fermentable influent sCOD. The fraction of sCOD contributed by MCCAs already present in the influent was subtracted from the fermentable influent sCOD used for the MCCA yield calculation.
2.5. Microbial analyses
Biomass samples were collected from the inoculum and waste beer upon starting the bioreactor and from the influent substrate mixture and bioreactor content periodically (see Table S3† for specific days of biomass sampling). The samples were immediately pelletized by centrifuging at 10
000 × g for 10 min, flash-frozen on dry ice, and stored at −80 °C until DNA and RNA extractions were performed. DNA was extracted using a cetyl trimethylammonium bromide (CTAB) method described by Porebski et al.46 with an additional bead-beating step (Mini-Beadbeater-96, BioSpec Products, Bartlesville, OK, USA) for 1.5 min using 0.1 mm diameter zirconium beads. Total RNA was extracted using TRIzol (Invitrogen, CA, USA) following the manufacturer's instructions with some modifications. In the lysis step, 1.5 min bead-beating with 0.1 mm diameter zirconium beads was included for mechanical cell lysis after adding TRIzol reagent to the samples. The RNA precipitation step was slightly modified to include 2.6 M sodium acetate in addition to ice-cold absolute ethanol. Glycoblue was added to visualize the RNA pellets and the RNA samples were stored at −20 °C for 24–48 h followed by ethanol washing and resuspension in water. ezDNase (Thermo Scientific, MA, USA) was used to remove residual DNA from RNA extracts following the manufacturer's guidelines. The effectiveness of DNA removal was evaluated by quantifying the abundance of bacterial 16S rRNA genes using bacterial primers47 by qPCR. A SuperScript® IV VILO cDNA synthesis kit (Invitrogen, Carlsbad, CA) was used to convert RNA into single-stranded complementary DNA (cDNA) according to the manufacturer's instructions. DNA and RNA quantities were determined using a Qubit 2.0 Fluorometer (Invitrogen, Life Technologies, CA, USA).
cDNA and DNA samples were submitted to the Microbial Systems Molecular Biology Laboratory (University of Michigan, Ann Arbor, MI, USA) for 16S rRNA and 16S rRNA gene sequencing, respectively, on the Illumina MiSeq platform (San Diego, CA, USA). Primers F515 and R80648 targeting the V4 region of the 16S rRNA gene were modified for dual-index sequencing as described by Kozich et al.49 A total of 1
636
675 high-quality reads were generated.
2.6. Sequencing data analysis
The sequences were processed using the mothur platform (version 1.42.0) following the MiSeq SOP.50 The reference SILVA database (version 132) implemented in mothur was customized to align with the filtered sequences. The UCHIME algorithm was used for chimera removal. The Ribosomal Database Project (version 16) was used for taxonomic classification of sequences to the genus level and sequences were grouped into operational taxonomic units (OTUs) based on the average neighbor algorithm at 3% sequence divergence cutoff. The raw sequences are available under NCBI BioProject ID PRJNA738485. Good's coverage was estimated in mothur for each sample as a measure of sampling coverage of OTUs represented by more than one read (Table S4†). OTUs that could not be classified at the genus level were denoted as “unclassified” followed by the name of the lowest taxonomic group in which the OTU could be classified.
16S rRNA gene and 16S rRNA sequencing data were used to study the total and active microbial community, respectively. Singletons were removed for relative abundance and relative activity calculations. The dominant OTUs or genera were defined as OTUs or genera present at a relative abundance or relative activity ≥1% in at least 50% of the samples. For simplicity, 16S rRNA gene and 16S rRNA are referred to as rDNA and rRNA, respectively, in the following ratio calculations. As an indirect measure of activity, the ratios of relative activity (rRNA) and relative abundance (rDNA) for each genus observed in the bioreactor (rRNA/rDNAreactor) and the influent samples (rRNA/rDNAinfluent) were calculated. Genera with increasing rRNA/rDNA ratios were assumed to be active as their ribosome abundance increases more than their genome copy number.51 The ratios of [rRNA/rDNA]reactor
:
[rRNA/rDNA]influent were used to compare the activities of the different genera in the bioreactor with those in the influent. Lastly, a mass balance approach similar to the one used by Mei et al.31 with some modifications was used to calculate the specific growth rate (μ) for populations observed in both influent and bioreactor samples (a detailed presentation of the calculation is given in the ESI†). The specific growth rates were calculated two different ways: one method used the amount of DNA/RNA recovered from the cell (method I) and the other method used VSS as an indirect measure of cell concentration (method II).31 Representative sequences obtained from mothur for dominant bacterial OTUs observed in the bioreactor were used for phylogenetic analysis. The closest relatives of the dominant OTUs were determined using a BLAST analysis and chosen as reference sequences. The 16S rRNA gene sequences of the reference sequences were downloaded from NCBI GenBank Database. MEGA752 was used to align and trim the sequences and compute the evolutionary distances using maximum likelihood analysis.
2.7. Statistical analyses
All statistical analyses of microbial community data were performed using R (version 3.6.1) with packages vegan (version 2.5–6),53 phyloseq (version 1.30.0),54 dplyr (version 0.8.5),55 and ggplot2 (version 3.3.0).56 Statistical significance was set at α ≤ 0.05. Kruskal–Wallis rank sum test with Benjamini–Hochberg correction for multiple testing (non-parametric one-way ANOVA) was used to test the statistical significance of the difference between groups and conditions for bioreactor performance data. The Pearson correlation coefficient was calculated using the cor.test function in R. Alpha-diversity indices such as observed OTUs for richness, Shannon index, and Pielou's evenness were calculated using the vegan package to compare DNA and RNA community profiles. Beta-diversity analyses included nonmetric multidimensional scaling (NMDS) using the Jaccard (using the binary = TRUE option, community membership-based) and Bray–Curtis (community structure-based) dissimilarity matrices. The statistical difference in microbial community structure and membership among and between the influent and bioreactor samples were tested with analysis of similarities (ANOSIM). The higher the ANOSIM R value, the more dissimilar the groups are. A linear regression model was fitted with MCCA volumetric production rate (log transformed) as the response variable and HRT, SRT, temperature, and operational days as the explanatory variables. A sinusoidal term was fitted to the “operational days” term in the model to investigate whether the MCCAs production followed a cyclical behavior.
3. Results and discussion
3.1. MCCA recovery from brewery and pre-fermented food waste streams
SCCA-rich permeate derived from a food waste fermentation process and ethanol containing waste beer were used to produce MCCAs in a chain-elongation ASBR system. Production of MCCAs, including caproate, enanthate, and octanoate, began within a few days of bioreactor startup as shown in Fig. 1.
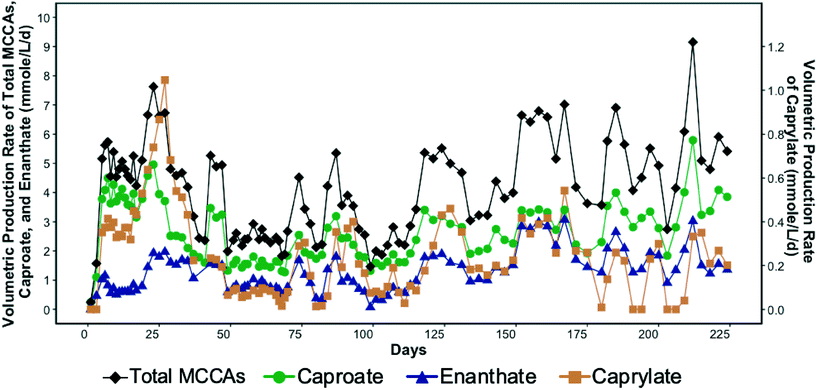 |
| Fig. 1 Volumetric production rate of total medium chain carboxylic acids (MCCAs), caproate, enanthate, and caprylate (secondary y-axis) in the bioreactor over time. | |
The MCCA volumetric production rate was high in the first four weeks of operation with caprylate reaching a maximum rate of 1.05 mmole L−1 d−1 (0.3 g L−1 d−1) on day 27 (Fig. 1). The corresponding caprylate to caproate product ratio was 0.4 (on a carbon basis). The MCCA volumetric production rate then decreased likely due to inhibition by undissociated MCCAs and high ethanol concentration. The ethanol concentration in the influent was kept high initially to promote MCCA production.5,13 While ethanol has been shown to be toxic to C. kluyveri when in excess of 200–400 mM,5 the ethanol toxicity threshold for an adapted chain elongating microbial community has not been determined. The ethanol concentration in the bioreactor was maintained in the range of 194.4–602.2 mM until day 27. Since 30.5 ± 7.2% of the ethanol fed was not consumed, the ethanol concentration in the influent was decreased (Fig. S2†).
The average MCCA volumetric production rate was 4.1 ± 1.6 mmole L−1 d−1 (0.5 ± 0.2 g L−1 d−1) with a maximum of 9.1 mmole L−1 d−1 (1.1 g L−1 d−1) on day 212 (Fig. 1) when 27% of sCOD in the influent was converted to MCCAs. Caproate was the major MCCA produced comprising on average 62.3 ± 9.8% of the total MCCAs, while enanthate and caprylate constituted, respectively, 31.2 ± 9.4% and 6.5 ± 4.1% of the total MCCAs produced on a carbon basis. The maximum MCCA yield and volumetric production rate achieved were lower than in other chain elongation studies that used complex organic waste streams containing ethanol (14.5–29.2 mmole L−1 d−1), but those studies used in-line extraction systems to continuously remove produced MCCAs.4,17,19 Roghair et al.14 achieved a maximum caproate volumetric production rate of 47.3 mmole L−1 d−1 in a two-stage system treating food waste and crude ethanol and avoided MCCA toxicity without an in-line extraction process by maintaining a neutral pH. Furthermore, in our study, most of the sCOD in the influent, including ethanol, was converted into SCCAs, particularly acetate, which was not further elongated into MCCAs despite the availability of sufficient ethanol. Excessive ethanol oxidation to acetate, a competing reaction that took place in our system, is discussed in more detail by Shrestha.57
As indicated above, chain elongation can be a self-limiting process due to the inhibitory effect of MCCAs.5,17 A pH of 5.5 was maintained in the bioreactor to minimize methanogenesis, but since this pH value was only slightly above the pKa values of MCCAs (4.8–4.9), a considerable fraction of the MCCAs were present in their undissociated forms. The undissociated forms are more hydrophobic than their corresponding conjugate bases and diffuse through the membranes of microbial cells decreasing the intracellular pH. Consistent with this, we observed that the MCCA volumetric production rate varied cyclically in the bioreactor (Fig. 1), which was supported by a linear regression model (R2 = 0.6 with significant sine term, p = 8.9 × 10−5). This observation suggests that MCCAs accumulated to an inhibitory level, after which their production decreased, allowing the microbial community to recover. We calculated that a maximum concentration of undissociated caprylic acid of 0.4 mM (0.06 g L−1) occurred on day 27 after which the MCCA production decreased drastically suggesting inhibition. Furthermore, the maximum undissociated caproic acid concentration was observed on day 212 when its concentration reached 3.2 mM (0.4 g L−1). Past work has shown undissociated caprylic acid to be inhibitory above 0.6 mM at pH 5.2,13 whereas undissociated caproic acid has been reported to be inhibitory above 7.5 mM at pH 5.5.17 The undissociated MCCA concentrations in these studies were kept below their inhibitory levels by continuous removal of MCCAs using an in-line extraction unit. The inhibitory MCCA concentrations observed in our study are lower than those reported by other studies, but concentrations at which product toxicity occurs depend on the type of bioreactor system (i.e., with or without in-line extraction unit), the undissociated concentration of other carboxylic acids, and the microbial community.
3.2. Microbial populations involved in MCCA production
Based on the 16S rRNA gene sequencing data, the dominant OTUs in the bioreactor belonged to Acidaminococcus, unclassified bacteria, Bifidobacterium, unclassified Clostridiales, unclassified Erysipelotrichaceae, unclassified Lachnospiraceae, Megasphaera, Methanobrevibacter, Olsenella, Prevotella, and Succiniclasticum (Fig. 2 and S3†). The dominant active OTUs, determined by 16S rRNA sequencing, belonged to Acidaminococcus, Bifidobacterium, unclassified Clostridiales, unclassified Lachnospiraceae, Megasphaera, Methanobrevibacter, Olsenella, Prevotella, and Pseudoramibacter (Fig. 2 and S3†).
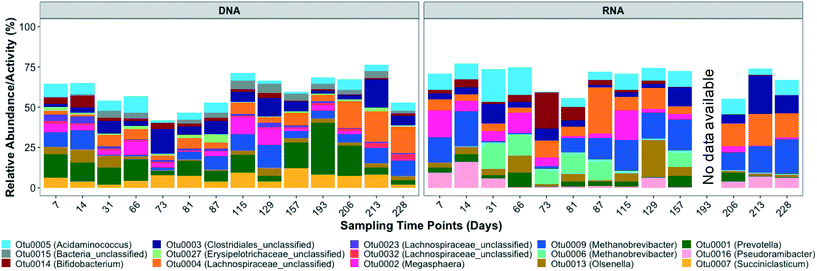 |
| Fig. 2 Relative abundance and relative activity of dominant microbial populations at OTU level in bioreactor samples over time. The dominant OTUs present at relative abundance and relative activity greater than 1% in at least 50% of the samples (n = 14 in DNA and n = 13 in RNA group) are shown here. | |
The order Clostridiales averaged 24.3 ± 8.2% and 26.8 ± 11.9% of the total and active microbial community, respectively, and was comprised of 31 genera, among which Pseudoramibacter, unclassified Lachnospiraceae, and some unclassified Clostridiales were enriched in the bioreactor. Populations within the order Clostridiales, especially C. kluyveri, have frequently been identified in ethanol chain elongation studies.16,19,36,58 Unclassified Clostridiales exhibited high relative abundance and relative activity of 8.3 ± 5.1% and 8.0 ± 6.1%, respectively. The dominant Clostridiales OTU, i.e. OTU 3, clustered with different Eubacterium species, including Eubacterium pyruvativorans (Fig. S4†), which has been shown previously to produce valerate and caproate.59 The relative abundance (correlation coefficient = 0.54, p = 0.05) and activity (correlation coefficient = 0.71, p = 0.01) of Clostridiales OTU 3 significantly correlated with the MCCA volumetric production rate. Similarly, the volumetric production rate of even chained MCCAs (caproate and caprylate) was positively correlated with the relative activity (correlation coefficient = 0.55, p = 0.05) of Pseudoramibacter OTU 16 but it did not correlate significantly with its relative abundance (correlation coefficient = 0.35, p = 0.22). OTU 16, the most abundant Pseudoramibacter OTU (Fig. 2) among the four Pseudoramibacter OTUs observed in the bioreactor, was phylogenetically closest to Pseudoramibacter alactolyticus (Fig. S4†), which has been shown to produce both SCCAs and MCCAs as end products of glucose and sucrose fermentations.60 It has been associated with MCCA production from lactate in other mixed-culture studies,27,61 but its ability to use ethanol for MCCA production has not been described previously. Pseudoramibacter OTU 16 was most active in the first month of operation after which its relative activity decreased from 5.9% on day 31 to 0.22% on day 66 before increasing again starting from day 206 (Fig. 2). This decrease in relative activity aligns with the highest caprylate production on day 27 (Fig. 1). The high caprylate concentration was likely inhibitory and the later increase in relative activity suggests slow adaptation and reduced product toxicity. Furthermore, the increase in relative abundance and activity of unclassified Clostridiales aligns with the maximum volumetric production rate of 9.1 mmole L−1 d−1 observed on day 212. Specifically, the relative activity of unclassified Clostridiales increased from 5.5% to 24.1% from day 206 to 213. Besides MCCA producers, OTUs belonging to Acidaminococcus,62Lachnospiraceae,63Megasphaera,64Succiniclasticum,65 and Prevotella,66 which are generally functionally associated with acidogenesis, were also enriched in the bioreactor (Fig. 2 and S3†).
3.3. Microbial community in the inoculum, influent, and bioreactor
In addition to investigating the influent and bioreactor microbial communities, we also studied the influence of the inoculum microbial community. We inoculated our bioreactor with rumen content because rumen bacteria (e.g., E. limosum,67E. pyruvativorans,59 and M. elsdenii64) can produce MCCAs via chain elongation and are well adapted to high concentrations of SCCAs given typical rumen conditions.68 By day 7 (first biomass sample point after startup), the bioreactor microbial community composition had diverged from that of the inoculum (Fig. 3 and S5 and S6†). The rumen inoculum was more diverse than the bioreactor biomass (Fig. S3 and S7†). The dominant bacterial populations in the rumen inoculum were Fibrobacter, Prevotella, Ruminobacter, unclassified Bacteroidetes, unclassified Gammaproteobacteria, unclassified Lachnospiraceae, and Treponema. Except for Lachnospiraceae and Prevotella, these populations were present at a low relative abundance and activity or were undetected in the bioreactor. Some inoculum microbial populations might not have been maintained in the bioreactor due to the relatively short SRT of 9.7 ± 5.8 days from days 20–81. The effect of SRT on the microbial community, particularly the methanogens, is discussed in detail in the ESI.† The microbial community structure changed gradually during early bioreactor operation (day 7 to day 73), but had changed substantially by day 81 (Fig. 3a). After day 81, the microbial community formed a separate cluster (Fig. 3a), while the active fraction of the microbial community did not show this change except on day 129 (possibly due to an accidental pH increase in the food waste bioreactor, the permeate of which was used to prepare the influent) (Fig. 3b). The day 81 influent sample clustered with the rumen inocula samples likely because the food waste bioreactor had been re-inoculated with rumen content a few days before the day 81 influent was collected. The chain elongation bioreactor was re-inoculated with rumen content on day 178, but the bioreactor microbial community had already diverged from the rumen inoculum on day 193, the first sampling date after re-inoculation (Fig. 3 and S5 and S6†). The relative importance of the inoculum microbial community on the bioreactor microbial community depends on the source of the inoculum, the bioreactor operating conditions, and the bioreactor configuration (for example, a membrane bioreactor with a long SRT better retains inoculum populations).
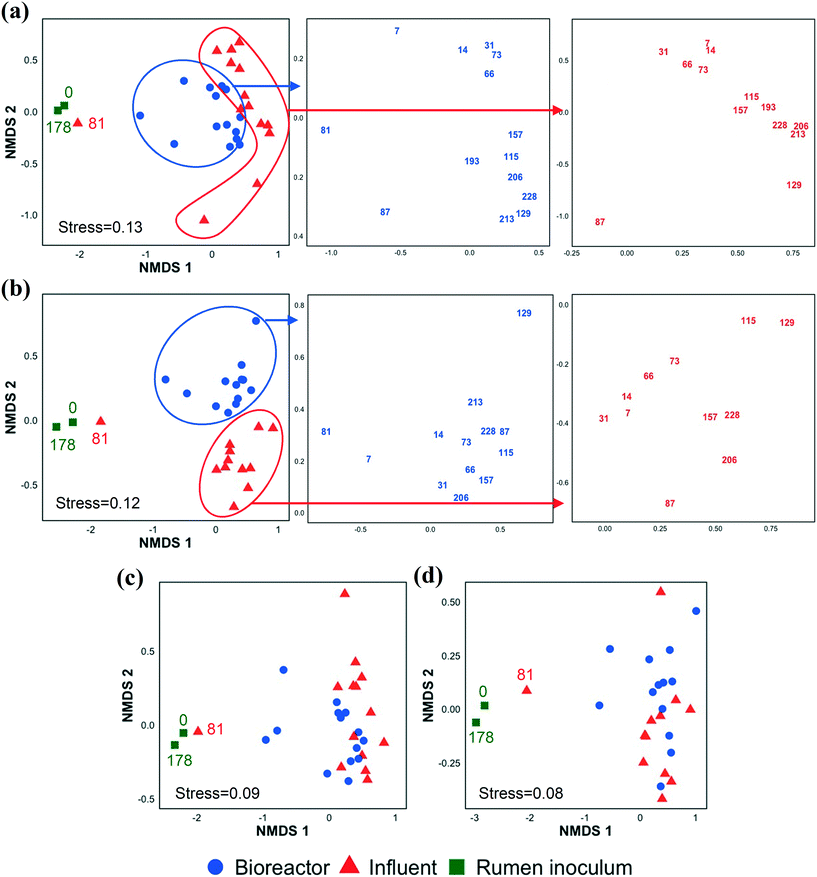 |
| Fig. 3 Non-metric multidimensional scaling (NMDS) ordination plot of the microbial community based on the Bray–Curtis dissimilarity index using 16S rRNA gene sequencing (a) and 16S rRNA sequencing data (b) and Jaccard index using 16S rRNA gene sequencing (c) and 16S rRNA sequencing data (d) at OTU level in the rumen inocula, bioreactor, and influent samples. The numbers correspond to sampling days. | |
Alpha and beta diversity indices were determined to compare the bacterial and archaeal community structure in the inoculum, influent, and bioreactor samples (Fig. S7†). The alpha diversity indices were statistically similar between the bioreactor and influent samples. NMDS ordination analysis based on Bray–Curtis dissimilarity (Fig. 3a, S5, and S6†) showed that the microbial community structures (OTU memberships and abundance) in the influent samples collected at different time points were similar to each other. Similarly, the bioreactor samples obtained at various time points were more similar to each other than the community structures in the corresponding influent and bioreactor samples collected at the same time points (ANOSIM R value = 0.55, p = 0.001, Table S5†). A similar observation was made when the 16S rRNA sequencing data were used for the Bray–Curtis dissimilarity analysis (Fig. 3b, Table S5,† ANOSIM R value = 0.67, p = 0.001). However, the NMDS analyses based on the Jaccard index indicated that the influent and bioreactor microbial community compositions (OTU memberships) were similar (Fig. 3c and d). This was supported by the ANOSIM analysis based on the Jaccard index, which showed that the R values obtained by comparing influent and bioreactor samples were small (Table S5†). These observations suggest that the influent and bioreactor samples collected at the same time were similar based on shared OTUs (Jaccard index), but that they differed when considering OTU abundance in addition to membership (Bray–Curtis index). Similar observations were made when bacterial and archaeal communities were compared separately using Bray–Curtis and Jaccard indices (Fig. S5 and S6, Table S5†).
3.4. Influent microbial populations in the chain elongation bioreactor
The microbial community composition and structure in influent and bioreactor samples were compared to study the fate of influent populations during chain elongation. The most abundant and active bacterial phyla in the influent were Bacteroidetes, Firmicutes, and Proteobacteria (Fig. S8†). On the other hand, the bioreactor samples were dominated by Firmicutes, Bacteroidetes, Euryarchaeota, and Actinobacteria (Fig. S8a†); the relative activity data were similar, except for Bacteroidetes (Fig. S8b†). A substantial fraction of the OTUs observed in the bioreactor originated from the influent. Specifically, 44 ± 6% and 33 ± 6% of total and active influent OTUs were present in the bioreactor and these shared OTUs accounted for 39 ± 9% and 41 ± 8% of the total and active OTUs in the bioreactor (Table S6†). We note that the detection of OTUs does not necessarily indicate they are metabolically active as some of these immigrant OTUs might represent dead or inactive cells.
Two approaches were used to distinguish active and inactive immigrant populations. The first approach used rRNA/rDNA ratios in the bioreactor and influent (Fig. 4); the second approach calculated the specific growth rate of immigrant populations (Table S7†). It should be noted that both approaches suffer from the well-described biases associated with using relative abundance/activity data because changes in the absolute abundance of a single taxon affect the relative abundance/activity of all other taxa. Most bacterial OTUs with a high relative abundance and activity in the influent (depicted by large values on the Y axes in Fig. 4a and b) were found to be less active in the bioreactor than in the influent, i.e., the [rRNA/rDNA]reactor
:
[rRNA/rDNA]influent < 1. This included OTUs belonging to the genus Prevotella, Succiniclasticum, unclassified Bacteroidales, and Megasphaera. Prevotella, the most dominant genus in the influent (Fig. S9†), was still abundant in the bioreactor (Fig. 2). However, Prevotella was less active in the bioreactor than in the influent (Fig. 2 and 4). The genus Prevotella has been mostly associated with fermentation and is commonly found in the rumen.69Megasphaera OTU 2 present in the bioreactor (Fig. 2), was also found in the influent suggesting that it originated from the influent, but it was less active in the bioreactor than in the influent (Fig. 4). As Megasphaera can produce both SCCAs and MCCAs,27,64 its broad fermentative capacity might have supported its growth in both the acidogenic food waste bioreactor and the chain elongation bioreactor. On the other hand, some populations, such as Olsenella, unclassified Clostridiales, and unclassified Lachnospiraceae, which exhibited comparatively low relative abundance and activity levels in the influent, became dominant in the bioreactor (shown by larger Y-axis values in Fig. 4c and d) with [rRNA/rDNA]reactor
:
[rRNA/rDNA]influent > 1. For example, Clostridiales OTU 3 and Pseudoramibacter OTU 16 levels correlated with MCCA production (as discussed above), but Clostridiales OTU 3 was present at low relative abundance (0.2 ± 0.3%) and relative activity (0.2 ± 0.2%) in the influent and Pseudoramibacter OTU 16 was only detected in some influent samples. Feeding ethanol-rich substrate and controlling bioreactor conditions selected for OTUs such as Clostridiales OTU 3 and Pseudoramibacter OTU 16 capable of MCCA production, even though they were present at low relative abundance and activity in the influent.
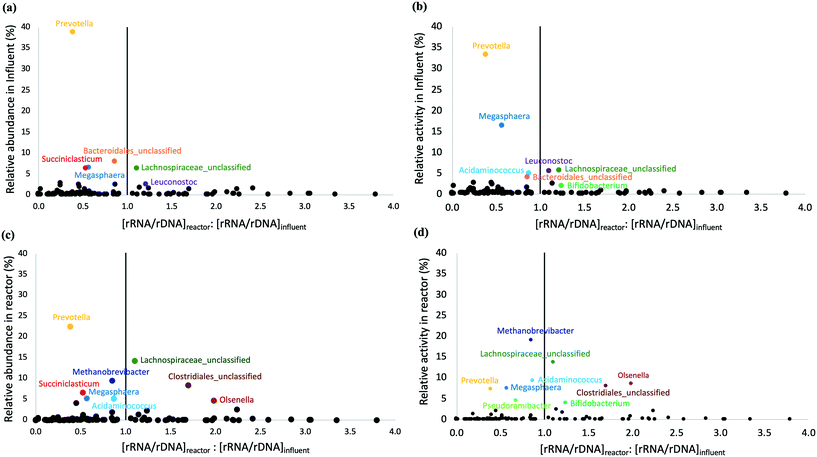 |
| Fig. 4 Comparison of rRNA/rDNA ratios of microbial populations in the bioreactor and influent versus relative abundance (a) and relative activity (b) in the influent and relative abundance (c) and relative activity (d) in the bioreactor. The vertical line is drawn at [rRNA/rDNA]reactor : [rRNA/rDNA]influent = 1. The microbial populations on the left of the vertical line are more active in the influent, whereas those on the right are more active in the bioreactor. Populations with a high relative abundance and activity (large Y-axis values) are labeled. A few populations with [rRNA/rDNA]reactor : [rRNA/rDNA]influent > 4 were excluded from the figure to improve clarity. | |
The relative abundance and activity data were highly correlated to each other in the influent samples (correlation coefficient = 0.94), but less so in the bioreactor samples (correlation coefficient = 0.78) (Fig. S10†). Such differential correlations introduce bias when calculating the [rRNA/rDNA]reactor
:
[rRNA/rDNA]influent ratio. Some studies have pointed out that sequencing depth and the presence of populations with different physiologies and growth strategies can distort the interpretation of the rRNA/rDNA ratio.31,70 Both variable ribosomal RNA (rrn) gene copy number and sequence variability introduce biases in the relative abundance and relative activity calculations and diversity estimates. The rrn gene copy number varies considerably among different microorganisms and methanogens generally have a much lower rrn gene copy number (1–4) compared to bacteria (1–15).71,72 Furthermore, the variation in rrn sequences within the same genome tends to be greater for microorganisms with more rrn gene copy numbers.39 These observations suggest that comparing rRNA/rDNA ratios in the bioreactor and influent is not adequate to identify all the active immigrant populations.
A mass balance approach was used as an additional tool to identify active influent populations with a positive specific growth rate that contributed to the bioreactor microbial community. MCCA producers such as Pseudoramibacter and unclassified Clostridiales had positive specific growth rates (Table S7†) suggesting that these populations were active in the bioreactor. We also note that the specific growth rate values calculated using method I were lower than those estimated by method II (Table S7†). As VSS measures all suspended organic matter in the bioreactor including live and dead microbial cells and other microbial components such as extracellular polymeric substances, method II might have overestimated cell concentration and skewed the specific growth rate calculation.
In bioengineered systems, microbial communities can be shaped by both deterministic factors (operational and environmental conditions) as well as stochastic processes (microbial immigration in an open mixed-culture bioreactor system). The influence of the influent microbial community on shaping the bioreactor community varies depending on how different the upstream and downstream systems are. In the current study, some of the dominant influent OTUs were washed out and some were inactive or decreased in relative abundance and activity in the bioreactor, whereas some low-abundant/low-activity influent OTUs (Clostridiales and Pseudoramibacter) were found to be involved in MCCA production. Our study shows that despite continuous influx of immigrant populations from the influent, there was a selection of microbial populations to achieve optimal function (i.e., MCCA production) and the microbial community tolerated a high level of stochasticity (immigration). Therefore, we can infer that selection dominated bioreactor community assembly. Community assembly theory predicts that immigration is important in shaping bioreactor communities when competitive selection is weak, and when population sizes are small, alpha diversity is low, and environmental conditions are dynamic.73 Overall, our quantitative approaches to differentiate active and inactive microbial populations provided insights into the fate of influent microbial populations in the chain elongation bioreactor fed with complex waste streams, but also highlighted the importance of identifying environmental conditions that allow desirable selection of the bioreactor community.
Conclusions and future directions
In this study, MCCAs were produced using waste ethanol and pre-fermented food waste using mixed-culture microbial communities. Additionally, we studied both the influent and bioreactor microbial communities and specifically assessed the presence and activity of influent populations in the chain elongation bioreactor. The following conclusions can be drawn from this study:
• A cyclic trend in MCCA production was observed indicating that MCCA accumulated to a level inhibitory to the microbial community, after which their production decreased allowing the microbial community to recover, resulting in increased production once again. This observation corroborated previous findings about MCCA toxicity.
• Even though influent microbial populations were introduced to the bioreactor on a daily basis, the microbial community structure in the bioreactor differed from that in the influent.
• A significant fraction of the bioreactor chain elongation microbial community originated from the influent, but not all influent microbial populations remained active.
• The bioreactor conditions selected for influent microbial populations capable of chain elongation, including populations that were not abundant in the influent such as Clostridiales and Pseudoramibacter.
Coupling specific growth rate estimates of individual microbial populations with an rRNA/rDNA approach enabled us to differentiate potentially active and inactive immigrant populations. Such approaches, when included into future studies, will avoid potential bias introduced by including inactive yet dominant populations in process modeling, thus improving our ability to identify engineering conditions that affect the active fraction of the microbial community and thus process performance. However, the varying rrn gene copy number in different populations can introduce bias. The mass balance model relied on relative activity and relative abundance data calculated from 16S rRNA and 16S rRNA gene sequencing, respectively, to estimate the total number of cells entering and exiting the system. Furthermore, future studies should look into using absolute abundance by quantifying cell count using flow cytometry or quantitative PCR methods. Lastly, besides beneficial microbial populations, the influent can provide non-desirable microbial populations which may invade the bioreactor microbial community depending on its resilience. Therefore, studying microbial immigration can help us to identify if the microbial immigrants are beneficial (active), neutral, inactive, or invasive. This aspect of microbial immigration can be particularly useful in chain elongation studies that use more than one substrate (i.e., co-digestion) to determine the contribution of each co-substrate in shaping the downstream microbial community. As this characteristic of co-digestion is still unexplored, the methods presented here to study influent microbial populations can be applied to select suitable co-substrates that can contribute microbial populations important to the downstream process.
Conflicts of interest
The authors declare no competing financial interests.
Acknowledgements
The authors would like to thank Steve Donajkowski and Ethan Kennedy for their assistance with the bioreactor system and Doug Knox for providing waste beer. This work was supported by the U.S. National Science Foundation (Sustainability Research Networks 1444745). SS was supported by an Integrated Training in Microbial Systems Fellowship from the University of Michigan (UM). The ITiMS program “Instructional Program Unifying Population and Laboratory Based Sciences” at UM was funded by the Burroughs Wellcome Fund. SS also received support through a UM Rackham Postdoctoral Fellowship and a Water Environment Federation Canham Graduate Studies Scholarship.
References
-
S. Kaza, L. Yao, P. Bhada-Tata and F. Van Woerden, What a waste 2.0: a global snapshot of solid waste management to 2050, World Bank Publications, 2018 Search PubMed.
-
C. A. Jones, C. Coker, K. Kirk and L. Reynolds, Food Waste Co-Digestion at Water Resource Recovery Facilities: Business Case Analysis, 2019 Search PubMed.
- J. Malinauskaite, H. Jouhara, D. Czajczyńska, P. Stanchev, E. Katsou, P. Rostkowski, R. J. Thorne, J. Colón, S. Ponsá, F. Al-Mansour, L. Anguilano, R. Krzyżyńska, I. C. López, A. Vlasopoulos and N. Spencer, Municipal solid waste management and waste-to-energy in the context of a circular economy and energy recycling in Europe, Energy, 2017, 141, 2013–2044 CrossRef.
- C. Urban, J. Xu, H. Strauber, T. R. dos S. Dantas, J. Muhlenberg, C. Hartig, L. T. Angenent and F. Harnisch, Production of drop-in fuels from biomass at high selectivity by combined microbial and electrochemical conversion, Energy Environ. Sci., 2017, 10(10), 2231–2244 RSC.
- L. T. Angenent, H. Richter, W. Buckel, C. M. Spirito, K. J. Steinbusch, C. M. Plugge, D. P. Strik, T. I. M. Grootscholten, C. J. Buisman and H. V. M. Hamelers, Chain Elongation with Reactor Microbiomes: Open-Culture Biotechnology to Produce Biochemicals, Environ. Sci. Technol., 2016, 50(6), 2796–2810 CrossRef CAS PubMed.
-
D. J. Anneken, S. Both, R. Christoph, G. Fieg, U. Steinberner and A. Westfechtel, Fatty acids:Ullmann's Encyclopedia of Industrial Chemistry, 2012 Search PubMed.
-
C. Petrenko, J. Paltseva and S. Searle, Ecological Impacts of Palm Oil Expansion in Indonesia, Washingt. Int. Counc. Clean Transp., 2016 Search PubMed.
- K. M. Carlson, L. M. Curran, G. P. Asner, A. M. Pittman, S. N. Trigg and J. M. Adeney, Carbon emissions from forest conversion by Kalimantan oil palm plantations, Nat. Clim. Change, 2013, 3(3), 283–287 CrossRef CAS.
- T. I. M. Grootscholten, K. J. J. Steinbusch, H. V. M. Hamelers and C. J. N. Buisman, Chain elongation of acetate and ethanol in an upflow anaerobic filter for high rate MCFA production, Bioresour. Technol., 2013, 135, 440–445 CrossRef CAS PubMed.
- T. I. M. Grootscholten, K. J. J. Steinbusch, H. V. M. Hamelers and C. J. N. Buisman, Improving medium chain fatty acid productivity using chain elongation by reducing the hydraulic retention time in an upflow anaerobic filter, Bioresour. Technol., 2013, 136, 735–738 CrossRef CAS PubMed.
- T. I. M. Grootscholten, K. J. J. Steinbusch, H. V. M. Hamelers and C. J. N. Buisman, High rate heptanoate production from propionate and ethanol using chain elongation, Bioresour. Technol., 2013, 136, 715–718 CrossRef CAS PubMed.
- T. I. M. Grootscholten, D. P. Strik, K. J. Steinbusch, C. J. Buisman and H. V. M. Hamelers, Two-stage medium chain fatty acid (MCFA) production from municipal solid waste and ethanol, Appl. Energy, 2014, 116, 223–229 CrossRef CAS.
- L. Kucek, C. M. Spirito and L. T. Angenent, High n-caprylate productivities and specificities from dilute ethanol and acetate: Chain elongation with microbiomes to upgrade products from syngas fermentation, Energy Environ. Sci., 2016, 9(11), 3482–3494 RSC.
- M. Roghair, Y. Liu, D. P. Strik, R. A. Weusthuis, M. E. Bruins and C. J. Buisman, Development of an Effective Chain Elongation Process From Acidified Food Waste and Ethanol Into n-Caproate, Front. Bioeng. Biotechnol., 2018, 6, 50 CrossRef PubMed.
- M. V. Venkateswar, S. Hayashi, D. Choi, H. Cho and Y. C. Chang, Short chain and medium chain fatty acids production using food waste under non-augmented and bio-augmented conditions, J. Cleaner Prod., 2018, 176, 645–653 CrossRef.
- M. T. Agler, C. M. Spirito, J. G. Usack, J. J. Werner and L. T. Angenent, Chain elongation with reactor microbiomes: upgrading dilute ethanol to medium-chain carboxylates, Energy Environ. Sci., 2012, 5(8), 8189–8192 RSC.
- S. Ge, J. G. Usack, C. M. Spirito and L. T. Angenent, Long-Term n -Caproic Acid Production from Yeast-Fermentation Beer in an Anaerobic Bioreactor with Continuous Product Extraction, Environ. Sci. Technol., 2015, 49(13), 8012–8021 CrossRef CAS PubMed.
- J. Xu, J. J. L. Guzman, S. J. Andersen, K. Rabaey and L. T. Angenent, In-line and selective phase separation of medium-chain carboxylic acids using membrane electrolysis, Chem. Commun., 2015, 51(31), 6847–6850 RSC.
- L. Kucek, J. Xu, M. Nguyen and L. T. Angenent, Waste conversion into n-caprylate and n-caproate: resource recovery from wine lees using anaerobic reactor microbiomes and in-line extraction, Front. Microbiol., 2016, 7, 1892 Search PubMed.
- S. Gildemyn, B. Molitor, J. G. Usack, M. Nguyen, K. Rabaey and L. T. Angenent, Upgrading syngas fermentation effluent using Clostridium kluyveri in a continuous fermentation, Biotechnol. Biofuels, 2017, 10(1), 1–15 CrossRef PubMed.
-
Brewers Association, Statistics: Number of Breweries, https://www.brewersassociation.org/statistics-and-data/national-beer-stats/, (accessed 29 May 2020).
-
The Brewers of Europe, Brewers statistics 2017 edition, 2017 Search PubMed.
-
Brewers Association, Wastewater Management Guidance Manual, 2017 Search PubMed.
-
Brewers Association, Water and Wastewater: Treatment/Volume Reduction Manual, 2017 Search PubMed.
- W. S. Chen, D. P. Strik, C. J. Buisman and C. Kroeze, Production of Caproic Acid from Mixed Organic Waste : An Environmental Life Cycle Perspective, Environ. Sci. Technol., 2017, 51(12), 7159–7168 CrossRef CAS PubMed.
- L. G. Seluy and M. A. Isla, A Process To Treat High-Strength Brewery Wastewater via Ethanol Recovery and Vinasse Fermentation, Ind. Eng. Chem. Res., 2014, 53(44), 17043–17050 CrossRef CAS.
- M. J. Scarborough, G. Lynch, M. Dickson, M. McGee, T. J. Donohue and D. R. Noguera, Increasing the economic value of lignocellulosic stillage through medium-chain fatty acid production, Biotechnol. Biofuels, 2018, 11(1), 1–17 CrossRef PubMed.
- J. Xu, J. Hao, J. L. Juan, C. M. Spirito, L. A. Harroff and L. T. Angenent, Temperature-Phased Conversion of Acid Whey Waste Into Medium-Chain Carboxylic Acids via Lactic Acid : No External e-Donor, Joule, 2018, 2(2), 280–295 CrossRef CAS.
- J. Shin, S. K. Cho, J. Lee, K. Hwang, J. W. Chung, H. N. Jang and S. G. Shin, Performance and microbial community dynamics in anaerobic digestion of waste activated sludge: Impact of immigration, Energies, 2019, 12(3), 573 CrossRef CAS.
- R. Mei, J. Kim, F. P. Wilson, B. T. W. Bocher and W. T. Liu, Coupling growth kinetics modeling with machine learning reveals microbial immigration impacts and identifies key environmental parameters in a biological wastewater treatment process, Microbiome, 2019, 7(1), 1–9 CrossRef PubMed.
- R. Mei, T. Narihiro, M. K. Nobu, K. Kuroda and W. T. Liu, Evaluating digestion efficiency in full-scale anaerobic digesters by identifying active microbial populations through the lens of microbial activity, Sci. Rep., 2016, 6(1), 1–10 CrossRef PubMed.
- R. Mei, M. K. Nobu, T. Narihiro, K. Kuroda, J. Muñoz Sierra, Z. Wu, L. Ye, P. K. H. Lee, P. H. Lee, J. B. Van Lier, M. J. McInerney, Y. Kamagata and W. T. Liu, Operation-driven heterogeneity and overlooked feed-associated populations in global anaerobic digester microbiome, Water Res., 2017, 124, 77–84 CrossRef CAS PubMed.
- R. H. Kirkegaard, S. J. Mcilroy, J. M. Kristensen, M. Nierychlo, M. Karst, M. S. Dueholm, M. Albertsen and P. H. Nielsen, The impact of immigration on microbial community composition in full-scale anaerobic digesters, Sci. Rep., 2017, 7(1), 1–11 CrossRef CAS PubMed.
- S. J. Andersen, V. De Groof, W. C. Khor, H. Roume, R. Props, M. Coma and K. Rabaey, A Clostridium Group IV Species Dominates and Suppresses a Mixed Culture Fermentation by Tolerance to Medium Chain Fatty Acids Products, Front. Bioeng. Biotechnol., 2017, 5, 8 Search PubMed.
- A. Duber, L. Jaroszynski, R. Zagrodnik, J. Chwialkowska, W. Juzwa, S. Ciesielski and P. Oleskowicz-Popiel, Exploiting the real wastewater potential for resource recovery-: N -caproate production from acid whey, Green Chem., 2018, 20(16), 3790–3803 RSC.
- S. Shrestha, S. Xue, D. Kitt, H. Song, C. Truyers, M. Muermans, I. Smets and L. Raskin, Anaerobic Dynamic Membrane Bioreactor Development to Facilitate Organic Waste Conversion to Medium Chain Carboxylic Acids and their Downstream Recovery, ACS ES&T Eng., 2021 DOI:10.1021/acsestengg.1c00273 , in press.
- R. Li, H. M. Tun, M. Jahan, Z. Zhang, A. Kumar, W. D. Fernando, A. Farenhorst and E. Khafipour, Comparison of DNA-, PMA-, and RNA-based 16S rRNA Illumina sequencing for detection of live bacteria in water, Sci. Rep., 2017, 7(1), 1–11 RSC.
- J. A. Klappenbach, J. M. Dunbar and T. M. Schmidt, rRNA operon copy number reflects ecological strategies of bacteria, Appl. Environ. Microbiol., 2000, 66(4), 1328–1333 CrossRef CAS PubMed.
- T. Větrovský and P. Baldrian, The Variability of the 16S rRNA Gene in Bacterial Genomes and Its Consequences for Bacterial Community Analyses, PLoS One, 2013, 8(2), e57923 CrossRef PubMed.
- R. Poretsky, L. M. Rodriguez-r, C. Luo, D. Tsementzi and K. T. Konstantinidis, Strengths and Limitations of 16S rRNA Gene Amplicon Sequencing in Revealing Temporal Microbial Community Dynamics, PLoS One, 2014, 9(4), e93827 CrossRef PubMed.
- S. J. Blazewicz, R. L. Barnard, R. A. Daly and M. K. Firestone, Evaluating rRNA as an indicator of microbial activity in environmental communities: limitations and uses, ISME J., 2013, 7(11), 2061–2068 CrossRef CAS PubMed.
- P. J. Weimer and D. M. Stevenson, Isolation, characterization, and quantification of Clostridium kluyveri from the bovine rumen, Appl. Microbiol. Biotechnol., 2012, 94(2), 461–466 CrossRef CAS PubMed.
- B. S. Jeon, B. C. Kim, Y. Um and B. I. Sang, Production of hexanoic acid from d-galactitol by a newly isolated Clostridium sp. BS-1, Appl. Microbiol. Biotechnol., 2010, 88(5), 1161–1167 CrossRef CAS PubMed.
-
X. Fonoll, T. Meuwissen, L. Aley, S. Shrestha and L. Raskin, in IWA 16th World Congress on Anaerobic Digestion, The Netherlands, 2019 Search PubMed.
-
W. R. Eugene, B. B. Rodger, D. E. Andrew and S. C. Lenore, Standard Methods for the Examination of Water and Wastewater, 2012 Search PubMed.
- S. Porebski, L. G. Bailey and B. R. Baum, Modification of a CTAB DNA Extraction Protocol for Plants Containing High Polysaccharide and Polyphenol Components, Plant Mol. Biol. Rep., 1997, 15(1), 8–15 CrossRef CAS.
- N. Fierer, J. A. Jackson, R. Vilgalys and R. B. Jackson, Assessment of soil microbial community structure by use of taxon-specific quantitative PCR assays, Appl. Environ. Microbiol., 2005, 71(1), 4117–4120 CrossRef CAS PubMed.
- J. G. Caporaso, C. L. Lauber, W. A. Walters, D. Berg-Lyons, C. A. Lozupone, P. J. Turnbaugh, N. Fierer and R. Knight, Global patterns of 16S rRNA diversity at a depth of millions of sequences per sample, Proc. Natl. Acad. Sci. U. S. A., 2011, 108(1), 4516–4522 CrossRef CAS PubMed.
- J. J. Kozich, S. L. Westcott, N. T. Baxter, S. K. Highlander and P. D. Schloss, Development of a dual-index sequencing strategy and curation pipeline for analyzing amplicon sequence data on the miseq illumina sequencing platform, Appl. Environ. Microbiol., 2013, 79(17), 5112–5120 CrossRef CAS PubMed.
- P. D. Schloss, S. L. Westcott, T. Ryabin, J. R. Hall, M. Hartmann, E. B. Hollister, R. A. Lesniewski, B. B. Oakley, D. H. Parks, C. J. Robinson, J. W. Sahl, B. Stres, G. G. Thallinger, D. J. Van Horn and C. F. Weber, Introducing mothur: Open-source, platform-independent, community-supported software for describing and comparing microbial communities, Appl. Environ. Microbiol., 2009, 75(23), 7537–7541 CrossRef CAS PubMed.
- R. C. Mueller, L. Gallegos-Graves, D. R. Zak and C. R. Kuske, Assembly of Active Bacterial and Fungal Communities Along a Natural Environmental Gradient, Microb. Ecol., 2016, 71(1), 57–67 CrossRef PubMed.
- S. Kumar, G. Stecher and K. Tamura, MEGA7: Molecular Evolutionary Genetics Analysis Version 7.0 for Bigger Datasets, Mol. Biol. Evol., 2016, 33(7), 1870–1874 CrossRef CAS PubMed.
-
J. Oksanen, F. G. Blanchet, M. Friendly, R. Kindt, P. Legendre, D. McGlinn, P. R. Minchin, R. B. O'Hara, G. L. Simpson, P. Solymos, M. H. H. Stevens, E. Szoecs and H. Wagner, Package ‘vegan’: Community Ecology Package, R Package. version 2.5–6 Search PubMed.
- P. J. McMurdie and S. Holmes, Phyloseq: An R Package for Reproducible Interactive Analysis and Graphics of Microbiome Census Data, PLoS One, 2013, 8(4), e61217 CrossRef CAS PubMed.
-
H. Wickham, R. Francois, L. Henry and K. Müller, dplyr: A Grammar of Data Manipulation, R Package. version 1.0.5, 2015, p. 156 Search PubMed.
-
H. Wickham, W. Chang, L. Henry, T. L. Pedersen, K. Takahashi, C. Wilke, K. Woo, H. Yutani and D. Dunnington, ggplot2: Create elegant data visualisations using the grammar of graphics, R Package. version 3.3.0, 2020 Search PubMed.
-
S. Shrestha, Ph.D. Thesis, University of Michigan, 2020 Search PubMed.
- S. L. Wu, J. Sun, X. Chen, W. Wei, L. Song, X. Dai and B. J. Ni, Unveiling the mechanisms of medium-chain fatty acid production from waste activated sludge alkaline fermentation liquor through physiological, thermodynamic and metagenomic investigations, Water Res., 2020, 169, 115218 CrossRef CAS PubMed.
- R. J. Wallace, N. McKain, N. R. McEwan, E. Miyagawa, L. C. Chaudhary, T. P. King, N. D. Walker, J. H. A. Apajalahti and C. J. Newbold, Eubacterium pyruvativorans sp. nov., a novel non-saccharolytic anaerobe from the rumen that ferments pyruvate and amino acids, forms caproate and utilizes acetate and propionate, J. Med. Microbiol., 2003, 53(4), 965–970 CAS.
- A. Willems and M. D. Collins, Phylogenetic relationships of the genera Acetobacterium and Eubacterium sensu stricto and reclassification of Eubacterium alactolyticum as Pseudoramibacter alactolyticus gen. nov., comb. nov., Int. J. Syst. Bacteriol., 1996, 46(4), 1083–1087 CrossRef CAS PubMed.
- P. Yang, L. Leng, G. Y. A. Tan, C. Dong, S. Y. Leu, W. H. Chen and P. H. Lee, Upgrading lignocellulosic ethanol for caproate production via chain elongation fermentation, Int. Biodeterior. Biodegrad., 2018, 135, 103–109 CrossRef CAS.
- M. Rogosa, Acidaminococcus gen. n., Acidaminococcus fermentans sp. n., Anaerobic Gram-negative Diplococci Using Amino Acids as the Sole Energy Source for Growth, J. Bacteriol., 1969, 98(2), 756–766 CrossRef CAS PubMed.
-
M. Cotta and R. Forster, The Family Lachnospiraceae, Including the Genera Butyrivibrio, Lachnospira and Roseburia, Prokaryotes, 2006, vol. 4, pp. 1002–1021 Search PubMed.
- P. J. Weimer and G. N. Moen, Quantitative analysis of growth and volatile fatty acid production by the anaerobic ruminal bacterium Megasphaera elsdenii T81, Appl. Microbiol. Biotechnol., 2013, 97(9), 4075–4081 CrossRef CAS PubMed.
- N. O. Van Gylswyk, Succiniclasticum ruminis gen. nov., sp. nov., a Ruminal Bacterium Converting Succinate to Propionate as the Sole Energy-Yielding Mechanism, Int. J. Syst. Evol. Microbiol., 1995, 45(2), 297–300 CAS.
- E. Emerson and P. Weimer, Fermentation of model hemicelluloses by Prevotella strains and Butyrivibrio fibrisolvens in pure culture and in ruminal enrichment cultures, Appl. Microbiol. Biotechnol., 2017, 101(10), 4269–4278 CrossRef CAS PubMed.
- B. R. Genthner, C. L. Davis and M. P. Bryant, Features of rumen and sewage sludge strains of Eubacterium limosum, a methanol-utilizing and H2-CO2-utilizing species, Appl. Environ. Microbiol., 1981, 42(1), 12–19 CrossRef CAS PubMed.
- S. Shrestha, X. Fonoll, S. Kumar and L. Raskin, Biological strategies for enhanced hydrolysis of lignocellulosic biomass during anaerobic digestion : Current status and future perspectives, Bioresour. Technol., 2017, 245, 1245–1257 CrossRef CAS PubMed.
-
A. K. Puniya, R. Singh and D. N. Kamra, Rumen Microbiology: From Evolution to Revolution, Springer, 2015 Search PubMed.
- B. Steven, C. Hesse, J. Soghigian, L. V. Gallegos-Graves and J. Dunbar, Simulated rRNA/DNA ratios show potential to misclassify active populations as dormant, Appl. Environ. Microbiol., 2017, 83(11), e00696-17 CrossRef PubMed.
- D. L. Sun, X. Jiang, Q. L. Wu and N. Y. Zhou, Intragenomic heterogeneity of 16S rRNA genes causes overestimation of prokaryotic diversity, Appl. Environ. Microbiol., 2013, 79(19), 5962–5969 CrossRef CAS PubMed.
- S. F. Stoddard, B. J. Smith, R. Hein, B. R. K. Roller and T. M. Schmidt, rrnDB: Improved tools for interpreting rRNA gene abundance in bacteria and archaea and a new foundation for future development, Nucleic Acids Res., 2015, 43(D1), D593–D598 CrossRef CAS PubMed.
- D. Frigon and G. Wells, Microbial immigration in wastewater treatment systems: analytical considerations and process implications, Curr. Opin. Biotechnol., 2019, 57, 151–159 CrossRef CAS PubMed.
Footnotes |
† Electronic supplementary information (ESI) available. See DOI: 10.1039/d1ew00656h |
‡ Current affiliations: Joint Bioenergy Institute, Emeryville, CA 94608, USA; Biological Systems and Engineering Division, Lawrence Berkeley National Laboratory, Berkeley, CA 94720, USA. |
§ Current affiliations: Chevron Corporation, San Ramon, CA 94583, USA. |
¶ Current affiliations: Great Lakes Water Authority, Detroit, MI 48226, USA. |
|
This journal is © The Royal Society of Chemistry 2022 |
Click here to see how this site uses Cookies. View our privacy policy here.