DOI:
10.1039/D2EA00012A
(Critical Review)
Environ. Sci.: Atmos., 2022,
2, 829-851
Review of methods for assessing deposition of reactive nitrogen pollutants across complex terrain with focus on the UK
Received
21st February 2022
, Accepted 2nd July 2022
First published on 6th July 2022
Abstract
This review is a summary of the most up-to-date knowledge regarding assessment of atmospheric deposition of reactive nitrogen (Nr) pollutants across complex terrain in the UK. Progress in the understanding of the mechanisms and quantification of Nr deposition in areas of complex topography is slow, as no concerted attempts to measure the components of Nr in complex terrain have been made in the last decade. This is likely due to the inherent complexity of the atmospheric processes and chemical interactions which contribute to deposition in these areas. More than 300 studies have been reviewed, and we have consulted with a panel of international experts which we assembled for that purpose. We report here on key findings and knowledge gaps identified regarding measurement and modelling techniques used to quantify deposition of Nr across complex terrain in the UK, which depending on definition, may represent up to 60% of land coverage across Great Britain. The large body of peer reviewed papers, reports and other items reviewed in this study has highlighted both the strengths and weaknesses in the tools available to scientists, regulators and policy makers. This review highlights that there is no coherent global research effort to constrain the uncertainties in Nr deposition over complex terrain, despite the clearly identified risk of N deposition to ecosystems and water quality. All evidence identified that enhanced Nr deposition across complex terrain occurs, and magnitude of the enhancement is not known; however, there are major uncertainties particularly in the differences between modelled and measured wet deposition in complex terrain and representing accurate surface interactions in models. Using simplified estimates for Nr deposition, based on current understanding of current measurement and model approaches, an enhancement across UK complex terrain in the range of a factor of 1.4–2.5 (i.e. 40–150% larger than current estimates) is likely over complex upland terrain. If at the upper limits of this, then significantly more ecosystems in the UK would be at a direct risk of degradation, and the potential for long-term non-remediable water quality issues increased.
Environmental significance
No concerted attempts to measure the components of reactive nitrogen (Nr) in complex terrain have been made in the last decade due to the inherent complexity of the atmospheric processes and chemical interactions which contribute to deposition. This review highlights that there is no coherent effort to constrain the uncertainties in Nr deposition over complex terrain, despite the clearly identified risk to ecosystems and water quality. Based on current understanding, an enhancement of Nr deposition across UK complex terrain (up to 60% land coverage) is in the range of a factor of 1.4–2.5 (i.e. 40–150% larger than current estimates), meaning many ecosystems are at increased risk of degradation, and long-term non-remediable water quality issues.
|
1 Introduction
Complex terrain is an often-used term with different definitions depending on context, referring to areas of land which have irregular topographic features, such as mountains or coastlines, and variations in land use and surface roughness, such as mixed urban, rural, irrigated, and unirrigated. Complex terrain can modify synoptic weather features and generate unique local airflow changes to create local atmospheric effects such as thermally-driven winds, orographic cloud formation, accelerated flows, increased turbulence and sea breezes. In upland areas, air pollutant deposition to complex terrain determines the inputs onto sensitive habitats and their transport into water catchments. In the UK, water quality is strongly affected by inputs across catchments, which includes atmospheric deposition.1–4 The contribution of atmospheric deposition to total nutrient loading is highly variable, but in remote semi-natural ecosystems (i.e. an ecosystem with most of its processes and biodiversity intact, though altered by human activity), it is the primary route of input.5 Quantifying atmospheric pollutant deposition in these locations is a persistent modelling and measurement challenge. Research to date suggests that wet and cloud deposition dominate the upland deposition of eutrophying and acidifying compounds in the UK, especially in the west.6 However, there is a generally unaccounted for but potentially significant enhancement, as well as spatial and temporal uncertainty, in dry deposition in these terrains.7–10
Atmospheric deposition of eutrophying and acidifying compounds, including reactive nitrogen (Nr) compounds such as ammonia (NH3), is of particular concern because of their adverse impacts on habitat condition and on water quality.11 In the 1980s the primary concern around upland deposition was on acid deposition, dominated by SO2.12 Emissions of SO2 have declined dramatically across Europe and North America, and in these countries the focus has now shifted towards Nr compounds.13 When Nr is deposited in upland areas, it can be transported through the environment via stream water or groundwater runoff, which in some cases can account for 20–50% of the total atmospheric N deposited to the surface.7,14 A fraction of the deposited Nr is transformed into a gaseous chemical species (NO, N2O or N2) via denitrification.15 The remainder can accumulate in ecosystems on which they are deposited, in biomass and soil. The balance between emission and accumulation is influenced by the drainage conditions of the site (i.e. soil moisture controlled).16
When Nr is added to semi-natural ecosystems, it can lead to decreases in biodiversity and long-term water quality issues, including elevated nitrate levels and discolouration.17 Many areas are already at risk even from levels of Nr deposition already accounted for. The critical level (CLe, concentration below which harmful effects cannot be detected) for NH3 to mosses and lichens is 1 μg m−3, and 3 μg m−3 for other plants.18 Much of the UK is already exposed to NH3 concentrations which exceed this. Similarly, the critical load (prolonged exposure to pollutant above which harm may occur) for nitrogen deposition is also exceeded in many environments within the UK.19 Establishing long-term sustainable levels of Nr in vulnerable ecosystems and quantifying the pathways of Nr in complex terrain remains both a modelling and a measurement challenge. Even on flat terrain, measurement and modelling of Nr deposition is not a trivial exercise. It normally involves model-measurement fusion methods to quantify deposition, with significant uncertainties. Complex or rough terrain, with hills, mountains, cliffs, gullies etc., is even more challenging and many modelling and measurement methods which would be applicable for flat terrain cannot be used reliably as assumptions in calculations are not always met. There are several processes at work in complex terrain that have the potential to increase deposition compared with flat homogeneous surfaces, including dry deposition enhancement through edge effects and topography induced turbulence, wet deposition enhancement through the formation of topographic cloud, the seeder–feeder effect (precipitation from an upper-level precipitating cloud falling through a lower-level orographic stratus cloud that caps elevated terrain) and directional rain, as well as enhanced cloud deposition. Some of these effects are spatially highly variable and result in enhanced deposition loading not only at the catchment scale, but also in localised deposition hotspots, which may coincide with sensitive ecosystem types.
Evidence that large uncertainties exist in estimates of Nr deposition to upland catchments comes from measurements of deposition,20 uncertainty of complex terrain flows,21 and from model inter-comparisons.22 For example, in the UK, the upland deposition estimated by the concentration-driven CBED (Concentration Based Estimated Deposition) model23 exceeds that predicted by the EMEP4UK model24,25 and the UK Integrated Assessment Model (UKIAM26), both of which are based on an Atmospheric Chemistry and Transport Modelling (ACTM) approach driven by emissions (Fig. 1). Further assessment suggests that this difference is dominated by a difference in the estimation of wet deposition and this is also reflected by the comparison of the dry and wet deposition Nr budgets between CBED and the EMEP4UK ACTM for the entire UK (Fig. 2). CBED uses national measurements from bulk deposition samplers combined with a national precipitation map and a correction mechanism for the seeder–feeder effect to estimate wet deposition. In this approach, an overestimation could arise from this correction or the contribution of a dry deposition artefact to the bulk deposition sampling.27 By contrast, in the ACTM models concentration estimates are derived from emission estimates also taking into account chemical transformations and atmospheric transport and removal based on measurement-constrained modelled meteorology, rather than in situ concentration measurements.
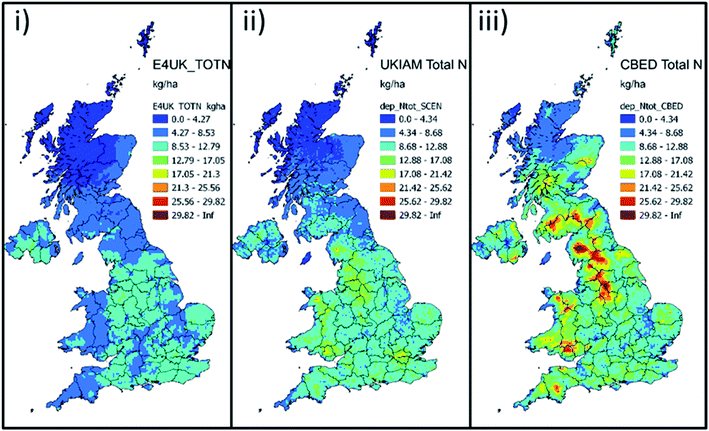 |
| Fig. 1 Total nitrogen (Nr) deposition for the year 2016, as estimated by (i) EMEP4UK; (ii) UKIAM and (iii) CBED models.28 | |
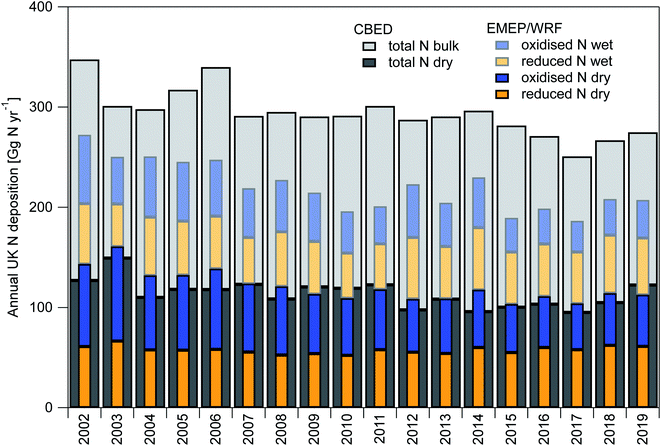 |
| Fig. 2 Time-line of annual total UK deposition budgets of total Nr as modelled based on measured concentrations by CBED and emissions by EMEP4UK rv4.36 driven with meteorology generated with WRF 4.2.2. Note that EMEP4UK results for 2020 and 2021 are based on the 2019 emission estimates, but 2020 and 2021 meteorology. | |
A comparison of seven ACTMs or ACTM implementations22 similarly highlighted particularly large relative differences in Nr wet deposition in upland regions – for NOy the range covered approximately a factor 3 and for NHx a factor of 4 (Fig. 3). It is possible that the uncertainty in both the dry deposition estimate and the contribution of dry deposition to total deposition may be underestimated by this comparison because both approaches use similar dry deposition parameterisations based on the assumption that deposition occurs to flat, homogeneous landscapes and they are therefore not fully independent.
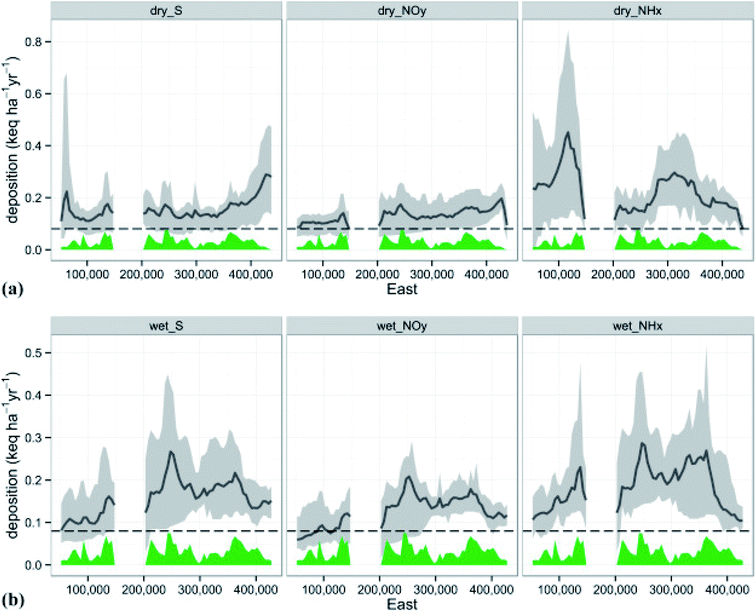 |
| Fig. 3 From Dore et al.22 (their Fig. 4). (a) Transect of dry deposition along a west–east trajectory (m) across the UK. (b) Transect of wet deposition along a west–east trajectory across the UK. The black line illustrates deposition averaged for all models and the grey shaded area shows the range of minimum and maximum modelled deposition. Terrain height is illustrated in green. | |
Uncertainty in upland nitrogen deposition enhancement is not purely an academic issue in regions with complex topography and sensitive upland ecosystems such as the UK – it is a potential long-term threat to the natural capital of ecosystems and water resources. This review summarises current knowledge on the topic, and hence provides a starting point to inform the steps needed to enable policymakers to quantify the risk of enhanced N-deposition over complex terrain, and develop a pathway for improving the state-of-knowledge and action-routes to understand, account for and possibly mitigate the risk from excess Nr inputs to sensitive ecosystems. This review summarises the most up to date knowledge on relevant methods used to model and measure deposition of Nr pollution in complex terrain, with a focus on the UK.
2 Measurements of pollutant deposition in complex terrain
Deposition to the Earth's surface of a chemical through dry deposition in the gas or particle phase, wet deposition through rainfall (rainout and washout), and occult deposition (i.e. deposition of cloud and fog droplets), is a function of chemical concentrations, meteorology, land cover, surface topography and chemical reactivity. Vet et al.29 pointed out that large-scale air pollutant concentration monitoring networks for Nr are used to inform national atmospheric pollutant assessments despite not capturing some important forms such as organic nitrogen, nor, importantly, the fine scale variations across specific landscapes. A review of the Nr deposition budget for the continental United States (US), and measurements of pollutant deposition to semi-natural ecosystems concluded that across the continental US, dry deposition contributes slightly more (55%) to total deposition than wet deposition, and it is the dominant process (>90%) over broad areas of the Southwest and Western US.30,31 Walker et al.30 noted that the lack of dry deposition measurements imposes a reliance on models (as discussed below), resulting in a high degree of uncertainty relative to wet deposition. However, there are also large uncertainties associated with wet deposition measurement methods that are routinely used. Cape et al.27 undertook a study of wet, bulk and flushed rain gauge deposition and found significant artefacts with both bulk and wet-only collector measurements, with a highly variable dry deposition artefact which is likely to be very dependent on local conditions. With respect to Nr flux measurements in natural ecosystems, low elevation forests and grasslands have been studied most extensively. There is an extensive literature on Nr emission fluxes of NO and N2O, but relatively few geographical locations have been characterized for total annual Nr deposition, particularly at background sites. Few studies32,33 have been undertaken at high elevation, mostly in North America (∼3000 m elevation). Precipitation (and fog) sampling at exposed and windy high elevation locations remains a significant challenge in terms of collecting precipitation that is representative of the amount reaching the ground.34–36 This applies to measurements of rainfall amount and chemical composition and thus rain gauge networks tend to be biased towards lower elevation sites with models used to extrapolate to upland areas.37
There are numerous sources of uncertainty in estimates of Nr deposition. Organic forms of Nr are not usually included in the wet or bulk deposition estimates but are known to often contribute 10–40% of the total Nr in precipitation.38–40 Emission and re-emission pathways of NH3 – from emission source, via the semi-volatile pollutant “hopping” as it volatilises, redeposits and is converted to other forms of Nr, to final loss to ecosystems, including freshwater systems, is rather poorly represented in most models and has not been monitored in an intensive, long-term way. Temporal variability of wet, dry and occult deposition and the emissions cycle (for example, spreading of nitrogenous material to land is undertaken seasonally) is an area in which both measurements and modelling are required to understand the dominant form of the risk across the UK. Most emission models use highly parameterised annual emission profiles, which have minimal field validation, and are particularly uncertain for agricultural emissions such as NH3. In reality, NH3 emissions vary with meteorology41 and there is a significant amount of inter-annual variability in the farming calendar, driven by legislation, meteorology and local practice. This, combined with surface interactions of NH3, means that spatio-temporal changes in atmospheric NH3 concentration, and therefore the deposition profile, are largely unquantified. New information on seasonal and inter-year variability comes from inversions using ground and Earth Observation data.42,43
2.1 Dry deposition flux measurements using micrometeorological and remote sensing approaches
Direct flux measurements would in theory be the ideal approach to quantify dry deposition to a range of surfaces and to assess the impact of topography and surface properties on deposition. However, for micrometeorological flux measurements to represent the surface flux they need to be conducted over extensive homogeneous and reasonably flat surfaces under conditions of stationary and well-developed turbulence.44 Whilst it has been shown that they are still reasonably applicable in some non-ideal situations,45 they are unsuited to measurements in truly complex terrain with steep slopes, cliff edges and heterogeneous vegetation cover. The principles of flux measurements have been reviewed, e.g., by Hicks and Baldocchi,46 who cover many of the major techniques and limitations. Tower-based micrometeorological methods provide a means to quantify fluxes of gases and aerosols averaged over large areas (typically 1000s to 100
000s of m2, depending on tower height and atmospheric stability), and characterise the turbulence and interactions of the pollutants with the surface. The methods do not disturb ambient conditions unlike, for example, chamber measurements. Methods include eddy-covariance (EC)47,48 and associated methods such as Relaxed Eddy Accumulation (REA)49,50 as well as flux-gradient approaches.51
Particular challenges for micrometeorological measurement of Nr fluxes include: (a) a comprehensive assessment of Nr requires many different chemical forms to be measured (e.g. NH3, HNO3, NO2, NH4+, NO3−), (b) instrumentation that is sufficiently fast for eddy-covariance (typically 10 Hz resolution) or sufficiently precise to resolve vertical gradients (+/− a few percent), where available, is expensive and difficult to operate, (c) this instrumentation is more suitable to measuring high concentrations (i.e. near source) and challenged by the low concentrations typically encountered in complex upland areas and small fluxes.
In particular, fast-response instruments for eddy-covariance measurement of NH3 concentrations are only just emerging and are still challenged by low concentration environments with few studies using closed-path52,53 and also open-path analysers.54
A recently developed eddy-covariance flux system for total Nr fluxes, based on a Total Reactive Atmospheric Nitrogen Converter (TRANC) coupled to a fast-response NO analyser arguably provides the most cost-effective eddy covariance approach for the direct measurement of Nr fluxes by avoiding the need for multiple instruments.55–57 However, it is still costly, and does not provide data that would help develop specific knowledge within the process of exchange of the individual Nr forms.
Requirements for fast instrumentation can be overcome by using gradient sampling approaches. Dry deposition flux of Nr can be estimated as the sum of dry deposition fluxes of NH3, nitric acid (HNO3), nitrogen dioxide (NO2) as well as aerosol components (NH4+ and NO3−) (recognising that there can be other significant Nr chemical species in the atmosphere) with the concentrations of pollutants and micrometeorological measurements at multiple heights.58 Although this approach has been used in challenging upland sites,32 concentration gradients are particularly sensitive to measurement location in complex terrain, because each height effectively sees a different footprint which get small for the lowest height where the influence of local heterogeneity effects are largest. Hence when these approaches are used, spatial replication is ideally needed but is rarely done so that care needs to be taken in interpreting and generalising any results. The Conditional Time-Averaged Gradient (COTAG) approach has been developed to provide long-term (weekly to monthly) average flux gradient measurements for a range of trace gases, between land and atmosphere, allowing annual deposition loads to be quantified without the need for real-time analysers.59,60 Single-height flux measurement approaches like EC and REA quantify the local flux at the measurement height and the challenge becomes to relate this flux to the actual surface exchange. By contrast, gradient approaches under non-ideal conditions are more difficult to interpret. However, despite these caveats, given the paucity of flux data each additional flux measurement is potentially of value. Although these latter approaches do not require eddy-covariance resolution (10 Hz) instrumentation, they are still micrometeorological approaches and so are limited to flat, homogenous surfaces.
Long-term observations of deposition rates are valuable: inferential modelling techniques use dry deposition velocities which in the ideal case are estimated in relation to meteorology, land use as well as canopy and site characteristics, but parameterisations tend to be crude and insensitive to the nutritional stage of the plants61 and chemical interactions on the surfaces.62,63 It is noted that as the chemical composition of the air and the surface characteristics change, it is likely that deposition parameterisations historically measured may therefore not be valid in the current and future chemical climates.
Much of the fundamental modelling work on flows over hills has been motivated by a desire to understand the impact of slopes and hills on eddy-covariance flux measurements and to quantify advection errors so that tower measurements can be related to the true surface exchange, and to develop strategies for the siting of flux measurement towers. The impact on flux measurements has been studied numerically for idealised hills,64 but the results remain challenging to generalise to more complex real-life situations. Eddy-covariance measurements can in principle be used to quantify the various advection terms during flux measurements in complex terrain which would allow meaningful surface exchange fluxes to be derived. For example, the ADVEX advection experiment65 deployed four towers with four measurement heights (i.e. 16 eddy-covariance systems) to study the advection problem for CO2 at a number of forest sites, and even with this suite of instruments it was impossible to quantify advective fluxes along the mean flow as they were associated with very small horizontal gradients that were difficult to resolve which appears to be a practical constraint on this approach. In any case, such a study represents a major step-change in complexity compared to traditional use of the method, in which studies typically use one point to measure 3-D wind directions and for Nr deposition would be extremely challenging: instrumentation is much more expensive and inter-instrumentation differences larger than for relatively high concentration gases such as CO2.
Surface and tower meteorological measurement installations can be equipped with remote sensing as well as in situ instrumentation. Doppler lidar systems have been used to supplement, and in some cases replace, meteorological towers and are being increasingly used to measure high frequency 3-D movement of wind (both mean flow and turbulent fluctuations). A range of experiments, both onshore and offshore, were performed within the New European Wind Atlas (NEWA) project to evaluate meso- and microscale models,66 primarily driven by the wind-energy sector requirements rather than meteorological forecasting or air pollution modelling. From the literature reviewed this dataset and the NEWA landscape instrumentation and datasets have not been applied to air pollution physics. It used sites across a significant range of area and slope scales in Europe, with varying topography. Remote sensing of flows around complex topography and vegetation at the sub-planetary-boundary-layer scale is very problematic. Scanning Doppler lidars are currently the only instruments with the required resolution, but the evidence and experience is that they are difficult to deploy in steep topography.
Doppler lidars have also started to be combined with collocated Raman lidars or sun photometers to derive spatially resolved eddy-covariance fluxes of aerosols,67–69 but little is still understood about the quality of the results. Remote sensing methods are clearly applicable for complex terrain for monitoring precipitation, aerosol dynamics and non-reactive gases. In particular, precipitation flux receives significant attention globally and there are satellite, surface remote sensing and combined products available.70–72 There is potential for combining methods which have been used for spatially resolved eddy-covariance fluxes with surface–exchange methods for reactive gases, however the concept and design of those experiments still remains to be developed.
2.2 Gas and aerosol chemical concentration measurements
Pollutant gas and particle chemical concentration measurements, in particular of e.g. NH3, HNO3, NO2, NH4+, NO3− (but also potentially other Nr species including HONO, peroxyacetlynitrates (PANs), organic nitrates, reduced organic nitrogen compounds) can be combined with theoretical or empirically measured deposition parameterisations for the specific surface, to calculate pollutant dry deposition. In general, the parameterisations have an assumption of a flat surface. With this approach, the requirement for fast response measurements or gradients is removed, however the generalisations introduce additional uncertainty particularly for complex terrain and variable surface roughness apply. Deposition schemes are either uni-directional (deposition) or bi-directional (deposition and emission).
Though it is possible to use high temporal resolution data (e.g. hourly) to calculate dry deposition from concentration, most pollutant deposition approaches use lower resolution concentration data. The time averaged, low cost approaches have been applied, across large spatial scales, and in national networks have long time series of data. The time-averaged samplers (e.g. passive or low volume active sampling methods) are used to make measurements of Nr species such as gaseous NH3, HNO3, NO2 and aerosol NH4+ and NO3− in many countries,73–75 and a few continent-wide experiments have been done also.76 Gas and aerosol chemical concentration measurements are then available to combine with meteorology and deposition schemes to calculate the amount deposition per unit time (usually annual). Passive samplers are particularly suited to measurement in remote environments as they do not require electricity. However, they are limited to gaseous pollutants and for Nr components most commonly used for NO2 and NH3. Passive samplers were derived from the original work of Palmes in the 1970s,77 and further work in the 1990s.78,79 Ammonia passive sampling was reviewed for the CEN Working Group80 with a standard for measurement published in 2020 (EN 17346:2020). The low-volume active sampling method (UKCEH DELTA®), with fairly low power requirements, was most recently described for Europe and the UK by.76,81 There are long-term spatial measurements across national scales.73 Passive samplers for aerosol measurements are also becoming more commonplace,82,83 however, they capture particles via deposition and require an estimate of the deposition velocity to infer air concentrations. The deposition rate to such artificial surfaces is not the same as to real vegetation (cf. Section 2.5 below), but is nevertheless likely to be impacted by the heterogeneous turbulence associated with complex terrain. This introduces additional uncertainty in the relationship between the captured amount and the concentration in air at complex sites.
There is a large amount of literature associated with aerosol lidar measurements, which can provide three-dimensional pictures of the distribution of air constituents (e.g. PM) under different conditions.84–86 Chazette et al.87 in the French Alps attributed the vertical dispersion of aerosol layers to increased or decreased convection, depending on snow cover.
The combination of airborne or surface scanning lidar techniques and in situ measurements can be a solution to track pollution dispersion over complex topography. There is a gap in the literature, and given technological developments both in remote sensing and in measurement techniques in the past two decades there appears to be a very significant possibility of combining methods to deliver step changes in knowledge, with the caveat that the extrapolation to generic understanding is not assured.
Wet deposition of pollutants is routinely measured using bulk precipitation sampling with off-line chemical analysis. With passive or active measurements the uncertainty can be calculated, both from measurement technique approaches as well as chemical specificity – NH3 and HNO3 in particular are challenging to measure accurately. There are also significant uncertainties in elevated altitudes where measurement using passive or low flow methods will be impacted by high humidity, changes in temperature range, non-vertical rain, snow and being in-cloud/fog, which add considerable uncertainties in the data obtained.
2.3 Measurements of wet and bulk deposition
Chemical wet deposition measurements are usually performed with bulk or wet-only samplers with off-line chemical composition analysis, combined with a measurement of rainfall amount. The EMEP protocol is wet-only sampling where the sample funnel and bottle are covered by a lid, which opens when rain starts. These instruments can be set up to collect either hourly or daily – the latter being the EMEP standard method. For other networks including the UK Precip-Net (https://uk-air.defra.gov.uk) a bulk sampler, which is open continuously, is used, providing lower temporal resolution and measurements with higher uncertainties.
A study by Cape et al.88 assesses changes in orographic enhancement at the one site in the UK which conducted long-term measurements of both cloud and rain sampling and found no trends in the cloud
:
rain scavenging ratios over the 1994–2008 period. However, this broad scale (5 km × 5 km) resolution mapping and the measurements at one point cannot address the potential risk at specific sites and fine scale complexity. Using the same methodology, Tang et al.79 explored the variability of NH3 in a Scottish upland landscape. Substantial variability in NH3 air concentrations and dry deposition was demonstrated within a 5 km × 5 km study area. Ammonia concentrations were typically a factor of 4 larger in the valley compared with the hill areas which correlated with farming activities.
2.4 Occult deposition
Fog water levels vary significantly across a landscape depending on wind exposure, orography and meteorology. It is therefore to be expected that deposition of Nr pollutants via this route will also be highly spatially variable. In high-elevation environments cloud and fog water samples are typically 5 to 20 times more concentrated in pollutants than rain water.89 Several papers have studied aspects of sulphur pollution in Polish mountains and typically, within individual mountain ranges in Poland, precipitation totals show limited differences between 80 and 120% of the aerial average but fog water deposition in the same area may differ, respectively, from 10% to 1000%.90 In the Sudetes Mountains total pollutant deposition was composed of around 30–40% by precipitation, 20–25% by dry deposition and 35–50% by direct fog/cloud deposition, while the associated proportion at the nearby lowland sites were around 75%, 20% and 5%, respectively.
Cloud water deposition to the surface can be measured directly using micrometeorological methods and deposition rates are straightforward to estimate as, unlike the trace Nr gases and small particles, there is no surface resistance.91,92 Long term monitoring of cloud water and the Nr deposited through this pathway is generally achieved by combining surface sampling of the cloud water composition using passive collectors with modelled deposition velocities, derived from the micrometeorological studies.
Fog water is difficult to collect quantitatively, in a way that reflects the amount that is actually deposited, and the collected volume varies greatly between collector types, more than fogwater chemistry.93 Isil et al.94 reported one of the most comprehensive studies of high elevation cloud water and PM and gaseous pollutant concentrations and modelled deposition fluxes. In the high elevation sites, cloud deposition was dominant; however, when (as in this study) NH3 is added to the total dry Nr deposition budget, the contribution from dry is similar to, or even greater than the contribution from the wet component. The challenge of measuring quantitative fog and cloud deposition fluxes is large, however, and requires a programme of monitoring which is undertaken in the US, but not currently across Europe or in the UK.
2.5 Indirect and proxy deposition measurements
Newer, indirect methods which may be of use in understanding deposition, and potentially suitable/adaptable to complex terrain, include mapping of pollutant isotope distributions through an environmental system (“isoscapes”).95 Bourgeois et al.96 studied NO3− export from montane (slopes of mountain) streams using three isotopes in addition to standard chemical analyses. They concluded that atmospheric fluxes were underestimated in previous studies. However, the measurements reported required intensive effort to collect and showed high variability. Similarly, Tsunogai et al.97 indicated that atmospheric nitrate in streams could be accurately measured. The isoscape method (coupled with fine scale modelling and source apportionment of wet and dry deposition) would be an indirect approach to assess catchment scale deposition and contribute to model validation and have the potential to identify local enhancements due to the rough terrain – though it would not mechanistically explain the cause of atmospheric enhancement. This type of study requires both intensive field work and laboratory capability for large volumes of samples for isotopic analysis.
The naturally occurring lead isotope 210Pb and the artificial radionuclide 137Cs attach themselves to ambient atmospheric aerosol and the study of their deposition can provide insights into the deposition of other fine aerosol compounds such as NH4+ and NO3−. Because of their long half-life of 22.3 and 30.2 years, respectively, it is possible to use 210Pb and 137Cs inventories of undisturbed soils to investigate the spatial pattern of the accumulated deposited amount deriving from wet and dry deposition. Likuku98 studied wet deposition of 210Pb over two areas of contrasting topographies. The inventories of deposited 210Pb measured in moorland soils of mid-Wales sites increased by a factor of 2.4 at or near the summit (741 m asl) of hills rising from the West coast of Wales relative to values at the coast (∼15 m asl), whereas rainfall increased by a factor of 1.8 over the same height range. They did not partition the enhancement of deposition between wet and dry deposition. On average, the summit to valley ratio of 210Pb concentration in rainfall was a factor of 1.3 due to scavenging of the feeder clouds by the seeder rain. The long-term 210Pb wet deposition field data could be an important input parameter for modelling wet deposition throughout the uplands of the UK and elsewhere where the seeder–feeder process is a common occurrence, though there are complexities and assumptions made. The 210Pb studies show the long-term average scale of enhancement of wet deposition by seeder–feeder processes,92,99,100 which is reasonably consistent with the CBED model (see Fig. 1). Further studies include Mourne,101 Moghaddam,102 Choubedar103 and Likuku104 which also report similar enhancements.
Pitcairn et al.105 studied the relationship between foliar N content and Nr deposition across Scandinavia including regions of complex terrain. The enhancement in Nr deposition by topography and canopy effects was estimated using 210Pb inventorying and ranged from 16–90% 210Pb enhancement. Where foliar N-enhancement was measured between open and forested at the elevated locations (2 sites) N enhancement in the forested area over the open location was 8% and 23%. The study highlights foliar N in a standardised plant as a further proxy for Nr deposition, although co-varying factors that impact plant growth (temperature, exposure to wind and sun) confound the interpretation of field data. The same applies to other bio-indicators for Nr deposition such as the N content of mosses and the presence of lichens,106 which have been used to augment direct concentration measurements.107 Plants are often more reliable accumulators of metals, which similar to the 210Pb budgets of soils, can provide information at least on the relative spatial pattern of deposition of air pollutants across variation of surface properties.108–111
Throughfall measurements derive total deposition from the amount of pollutant that drips off vegetation and is collected by throughfall and stemflow (accounting for the quantity of water and solutes that run down the stems of the trees) collectors. For many pollutants, including Nr, this requires a correction for the amount that is likely to have been taken up into the plants via the leaves and the amount that might have leached out of the leaves.112,113 This correction is subject to large uncertainties and depends on the state of the vegetation, but also the chemical form of Nr. Ammonia, for example, can enter the leaves directly in gaseous form via the stomata114 whereas aerosols or wet-deposited material relies on transport via water films.115 Nevertheless, throughfall measurements have been used to assess small-scale variability in deposition, e.g. across forest edges116 and in relation to mountain slope orientation.20 This approach is only available for tall vegetation canopies, typically forests, and therefore does not lend itself to much of the UK's uplands.
Washings of real leaf surfaces and artificial surface collectors have been used to estimate dry and fog deposition during periods without precipitation.117–119 Here real leaves are subject to similar interferences with uptake, leaching and chemical conversions as throughfall measurements, whilst artificial surfaces are often a poor representation of the physico-chemical properties and morphologies (e.g. microscopic hairs) that govern the atmosphere–surface exchange of real plants.
Wind tunnel measurements have been used to examine deposition over vegetation in general,120–123 but also to simplified complex terrain in order to separate processes and understand the drivers of deposition in the landscape.124,125
3 Deposition: processes and models
3.1 Overview of research modelling pollutant deposition to complex upland terrain
Literature specifically related to modelling of Nr deposition in complex terrain is very limited, but aspects of the problem studied in other contexts can help inform a strategy to improve Nr deposition modelling. For example, studies have looked at the transport and deposition of radioactive material, seed and pollen dispersal, as well as the spatial variability of snow accumulation. Nr deposition occurs through different routes (dry, wet and occult) as well as via different chemical forms. Fog droplets behave very much like coarse particles,91 ammonium and nitrate aerosols like fine particles and NH3, HNO3, NO2 and HONO are gases. When transferring the results of these alternative studies to the problem of Nr deposition, it needs to be considered that if the proxy is a particle (pollen, seed) the inertia of particles means that, unlike gases, they do not always move with the velocity of the local mean or the turbulent components of the fluid flow. This tends to result in enhanced deposition on the windward side and reduced deposition on the leeward side of hills.126
3.1.1 Dry deposition parameterisations.
Any model that predicts dry deposition needs to describe the interaction of the various compounds with the surface elements. Conventionally, dry deposition is modelled using either prescribed deposition velocities (Vd = − flux/concentration at a specified height above the surface) or the resistance analogue,127 in which the deposition velocity is calculated as a series of, at a minimum, three resistances: the aerodynamic resistance (Ra) which describes the transport from the height of the lowest model layer (zref) to the ground through turbulent processes, a resistance that describes the transport through the quasi-laminar boundary-layer (Rb) and a canopy resistance (Rc) which describes the interaction with the surface (leaves, ground). The latter may again be prescribed for different compounds and ecosystem types or parameterised, potentially as a network of various resistances that describe different exchange pathways. The height-dependent Ra is a function of the friction velocity (u*), a measure of the efficiency with which momentum is transferred to the ground, and the surface roughness length (z0), as well as atmospheric stability.
Gases overcome the quasi-laminar boundary-layers by Brownian diffusion and this governs the parameterisation of Rb. Particles have additional mechanisms to overcome the boundary-layers (i.e. impaction, interception and gravitation settling), and for particles, the concepts of Rb and Rc are typically replaced by alternative parameterisations that simulate these processes. The concept of Ra still applies, except for the additional need to describe the gravitation settling flux.
These standard concepts assume a large homogeneous surface of a homogenous roughness height and constant fluxes between the surface and zref, and therefore need to be modified to model dry deposition in complex terrain. The standard “flat-earth” relationships implicitly reflect a certain evolution of the above-canopy wind profile into the plant canopy and this profile is modified through topographic effects and changes in vegetation. In addition, they only describe the vertical turbulent transport to the ground, driven by large-scale average turbulence. Complex terrain however additionally gives rise to horizontal advection, locally increased turbulence generation and infiltration into canopies, and these processes can substantially add to the scale of flux to the surface.128,129 Thus, the underlying Monin-Obukhov Similarity Theory (MOST) is not strictly applicable to atmospheric boundary layer flows in complex terrain.130
In addition to the single-layer (or “big-leaf”) deposition models described above, a range of multi-layer exchange models have been developed to describe the interactions with different levels within the canopy131,132 and these often relate the collection efficiency of a given layer within the canopy to the modelled wind profile. As long as these wind profiles can be predicted correctly these concepts can be extended to parameterise transport of scalars in complex environments. Whilst significant work has gone into the development of multi-layer canopy exchange models for particles,133 work on multi-layer modelling of gaseous Nr compounds such as ammonia is particularly limited, and models would be poorly constrained by measurements currently available.134
Realistically, in a spatially extensive model, the near-ground and in-canopy wind velocity and turbulence profiles are unlikely to be resolvable explicitly. Hicks129 proposed an as-yet untested approach based on McMillen,135 to increasingly reduce Ra at locations with slopes exceeding 1
:
7. This approach assumes that Rb remains unaffected by complex terrain.128 However, the concept of a canopy value of Rb implicitly combines effects such as the thickness of the quasi-laminar boundary layers that surround surface elements (i.e. leaves) at different heights in the canopy and in-canopy transport and relate them to the friction velocity above the canopy. The concept of a friction velocity itself requires a height-invariant momentum flux it therefore does not apply to complex terrain and the relationship between the turbulence above and inside plant canopies is modified by additional infiltration of turbulence into the canopy. Thus, it would be expected that Rb is also lowered compared with its flat-earth formulation.
3.1.2 Inferential deposition models.
Inferential deposition models are used to derive dry deposition in situations where concentration is measured but the dry deposition flux is not. This approach uses parameterisations of the factors controlling dry deposition as described in the previous section. The inferential approach requires meteorological parameters as inputs. These can either be taken from direct measurements, predicted using Numerical Weather Prediction (NWP) models, or parameterised from measurements. For example, wind speed, surface roughness and atmospheric stability may be combined to estimate the friction velocity needed to estimate Ra, Rb and Rc.
In the UK, a dry deposition map is routinely generated by applying an inferential estimate of deposition flux to a spatially interpolated concentration field derived using the CBED modelling system,23 the latter making use of measurements from the UK National Ammonia Measurement and the Acid Gases and Aerosols Monitoring networks. In the US, the National Atmospheric Deposition Program (NADP) similarly infers deposition fields, combining concentration measurements of HNO3, NO3−, NH4+, SO2 and SO42− from the Clean Air Status and Trends Network (CASTNET) with deposition velocities derived from the Community Multiscale Air Quality Model (CMAQ).31,136 Deposition velocities are not measured routinely and most available data are used to develop the model parameterisations, so the assessment of deposition velocity estimates with independent measured values is not easily available.
A strength of the inferential approach is that it does not rely on estimates of emissions, which are subject to large uncertainties. A downside is that it is even more sensitive to the dry and wet deposition parameterisations than deposition estimates derived with ACTMs, which are by their nature mass-conserved. For example, whilst in the inferential approach a doubling of the deposition velocity infers twice the deposition, this is not the case in ACTMs as deposition lowers the concentration, and hence flux, downwind.
Differences in the parameterisations of the relevant properties of various surface types can lead to considerable differences in the deposition rates predicted by different inferential models even when assuming idealised flat-earth conditions.81 This is reflected in the parameterisation of Rc for gases and the surface value of Vd for particles. One study found uncertainties of >200% for particle deposition velocity.8 This is partly due to small-scale variability affecting the underlying flux database from which the parameterisations were developed. For example, Brook et al.137 compared estimates of deposition velocities derived from four measurement locations within 500 m of each other and derived variability of ±40% for O3 and SO2 and ±90% for aerosol SO42−. The variability was primarily attributed to complex terrain and abrupt changes in vegetation (i.e. differences between flux footprints or horizontal heterogeneity).
3.1.3 Pushing traditional atmospheric chemistry and transport models to increasingly higher spatial resolution.
Numerical models are the standard tool to quantify deposition over large spatial scales. Normally, the dry (and wet) deposition of pollutants in general, and Nr in particular, is predicted using atmospheric chemistry and transport models (ACTMs) that simulate the pollutant emission, transport, chemical transformation and deposition. Such models require the outputs from a numerical weather prediction (NWP) model as an input, which can be coupled to the ACTM online or offline.
Such models can be run from global to local scale. All transport models include processes that are explicitly resolved by the numerical solution of a set of differential equations over a gridded model domain and represented by approximated parameterisations at the sub-grid scale. There is an interplay between the spatial and temporal resolution (time-step) of the numerical solver in terms of computational effort and the likelihood and speed of convergence of the numerical model.
As a result, the spatial resolution that can be achieved with an ACTM is not just limited by computational resources and the resolution of the input fields (e.g. emissions, land cover, topography and meteorology) but also by model structure and the scales over which the numerically resolved and parameterised processes can be applied. At a time when computational resources were more limited, ACTMs were originally designed for fairly low-resolution applications, and resolutions in the range of 25 to 5 km are common for annual regional or country-wide simulations. At such resolution inadequacies in resolving complex flows have been reported: Thompson et al.138 identified the inability of the underlying NWP model to resolve upslope flows as a key uncertainty when modelling Nr deposition to Rocky Mountain National Park at 4 km × 4 km grid resolution using the CAMx model. Using the same model Zhang et al.139 assessed Nr deposition in the Greater Yellowstone area at 12 km × 12 km grid resolution and noted meteorological model overestimation of precipitation as a source of disagreement between measured and modelled wet deposition. Difficulty of modelling precipitation in complex terrain was specifically noted.140
The resolution to which model resolution can be pushed is often not well documented or may not have been fully established. This applies to both the ACTM and the underlying NWP model. For example, Viatte et al.141 applied the WRF-CHEM model at a resolution down to 333 m × 333 m and then switched to a different modelling approach (LES, see below) for a nested domain at 111 m × 111 m resolution. Similarly, the CMAQ modelling system has been available to be applied at a resolution down to 1 km × 1 km,142,143 and Garcia-Menendez et al.144 developed an adaptive grid version of CMAQ with localised resolutions of 100 m × 100 m. Land use-specific dry deposition estimates from the Surface Tiled Aerosol and Gaseous Exchange (STAGE) option in CMAQ v5.3 (ref. 145) can be applied to the underlying CMAQ land use data, e.g. 500 m MODIS, 30 m National Land Cover Database (NLCD), to estimate deposition at a finer spatial scale. In the UK, the highest resolution that has been achieved for full annual runs covering the entire country appears to be 1 km × 1 km,146 using a high-resolution implementation of the EMEP model, driven with WRF meteorology.
Although horizontal grid resolutions down to about 300 m × 300 m are therefore achievable with a range of models, this does not mean these models reflect changes of all parameters at this resolution. For example, even in the 1 km × 1 km application the lowest vertical model layer of the EMEP model is still limited to at least 45 m because in the tiling approach used: in the EMEP and similar models, the grid-cell average concentration and meteorology needs to be representative for all land-cover types in the grid cell and is then extrapolated to the ground for each tile with land-cover specific parameterisations. In addition, the dry deposition is parameterised with the standard “big-leaf” resistance analogue, which reflects the deposition to large, flat and homogeneous surfaces using the same parameterisations as in the inferential approach outlined in the previous section. Thus, current ACTMs do not account for turbulence created by local terrain, the impacts of small-scale heterogeneity on dry deposition or the horizontal transport into plant canopies. Similarly, orographic impacts on wet deposition are limited to those scales that are resolved by the underlying NWP models, where the effective model resolution typically exceeds the grid resolution by a factor of 5. The main difference between ACTM and CBED estimates of Nr dep. in the UK is due to wet deposition. The orographic enhancement processes demonstrated in experimental and monitoring studies seems to be the main contributor to this difference and is explicitly included in CBED and not in the ACTMs.
Several approaches have been developed for Eulerian ACTMs to provide higher resolution concentration (not deposition) maps than their grid resolution can provide. For example, in several studies local scale Gaussian plume dispersion models such as ADMS or OPS-ST have been coupled to the coarser scale model to simulate concentration fields along roads and near point sources, including for Nr compounds such as NH3.147,148 These approaches use additional information on the source location rather than the receptor sites and they are therefore normally more suited to modelling the concentration and deposition of pollutants that originate from complex line and point sources rather than modelling the deposition to complex terrain. In an alternative approach the grid average concentrations provided by the ACTMs are remapped within a grid cell using land use regression approaches. Neither approach utilises higher-resolution turbulence data.
Several countries operate dispersion modelling systems, such as NAME (Numerical Atmospheric-Dispersion Modelling Environment) in the UK,149 WSPEEDI-II in Japan,150 based on either Eulerian, Lagrangian, hybrid Eulerian/Lagrangian, or Gaussian puff models. A widely used example of an Eulerian/Gaussian puff modelling system is the CALMET/CALPUFF modelling system,151 which was used, for example, to model radionuclide deposition from the Chernobyl accident152 and from a former uranium mill to complex terrain in Colorado,153 and to tested e.g. against the variability in 210Pb/137Cs deposition across a complex landscape in Italy.154 Another puff modelling system for nuclear accident assessment is the COMPLEX/TAIPUFF modelling system.155 By contrast, offline Lagrangian models such as NAME use the flow field from a NWP and then virtually release into it a large number of ‘stochastic fluid particles’ with a random walk element to slowly build up a mean picture of transport and dispersion. These models can be operated at fairly high resolution (e.g. 1 km × 1 km), and whilst their focus was initially on dispersion and transport of chemically inert tracers and the deposition in particular of particles, NAME has been developed further for a range of air quality applications, including the modelling of sulphur and nitrogen compounds. However, many Lagrangian models are used for a wide range of applications including air quality and have chemistry schemes as well as inert tracers, and do represent deposition of gases as well as particles. The ‘stochastic fluid particles’ are a computational concept and they represent a certain mass of the specie(s), which could be gases or particles. Such models have been used in complex terrain. One such example is a modelling study of pollen dispersion in a complex island environment.156,157 Viner and Arritt156 derived a spatial pattern in pollen deposition due to terrain-induced up and down-drafts, predicting ‘gaps’ in deposition to the up-slopes, the opposite of what was found in other studies.158,159 However, in their model, pollen was deemed deposited if it came within 1 m of the ground, so their model lacked a mechanistic description of the turbulent deposition process itself. Even where model resolution can be improved to improve the transport in complex terrain, high resolution models still rely on dry deposition process descriptions developed for flat terrain.
The higher the spatial resolution of the underlying NWP model, the better, in principle, is its ability to predict orographic clouds and precipitation. High horizontal resolution also requires high vertical resolution and short model time-steps, which increases computational cost. In principle, the Unified Model of the UK Met Office, for example, can be operated at high spatial horizontal resolution (e.g. 50 m) and the same is true for WRF in LES mode.
However, although NWP models may be operational down to high resolution, their Sub-grid Scale (SGS) components are not necessarily capable of reproducing the physics that control the flow at this scale130,160 reviewed current knowledge and remaining challenges regarding the modelling (and measurement) of dispersion in complex terrain, and pointed out that advancements in the parameterizations for turbulence processes suitable for complex terrain are urgently needed to move NWPs to sub-kilometre resolutions (some wind farm forecasts use such parameterizations). They also noted the uncertainty of input data at this scale, such as vegetation and soil characteristics and, in high terrain, snow cover. Without enhanced capacity of Nr deposition measurements over multiple complex terrains, parameterizations in models are still assumed or averaged based on known land-use types.
Sensitivity studies suggest that a grid resolution of 1.5 km predicts area-integrated rainfall over the Lake District to within 2%, whilst coarser resolutions of 12 and 40 km result in a rainfall under-prediction of 11–24% and 33–48%, respectively.161 Nevertheless, even at the 1.5 km scale local errors still occur due to unresolved peaks and valleys. The UK Met Office has invested substantial modelling effort to improve understanding of significant rain events and flooding across complex terrain and Roberts et al.162 demonstrated the benefit of coupling the high-resolution rainfall forecasts to the Probability-Distributed Moisture (PDM) and the improvement in timeliness of flood warning that might have been possible.
Whilst the prediction of transport and orographic rainfall might be expected to improve with increased spatial resolution, measurements are still lacking to ascertain that this is really the case.
3.1.4 Modelling flows and pollutant transport over hills.
There is a significant body of work that has investigated the effect of hills on boundary layer flow, turbulence and fluxes, and the additional effect of vegetation as recently reviewed by Finnigan et al.160 This work has not usually been motivated by an interest in deposition modelling, but rather for informing activities such as siting wind turbines and predicting wind-driven erosion and sediment transport, as well as for interpreting micrometeorological (carbon) flux measurements made under non-ideal conditions. Nevertheless, much of the underlying physics and methods are clearly applicable to deposition problems also. Finnigan et al.160 distinguish in their review between (a) investigations into the fundamental physical processes leading to the development of (mostly analytical) models for idealised hills, (b) development of Reynolds-Averaged Navier–Stokes (RANS) models that solve the Navier–Stokes equation based on (higher order) turbulence closure schemes and (c) eddy-resolving large-eddy simulations (LES). The review is comprehensive and not repeated here. Instead, we are here focussing on the few studies that have explicitly considered the deposition problem.
(i) Exploration of physics and analytical approaches.
Hill et al.163 modelled sulphate fog droplet deposition to Great Dun Fell, a hill in the northern Pennines which was the subject of intensive hill cap cloud experiments in the 1980s and 90s. The focus of that study was on the cloud chemistry, but it had to treat the deposition process as well. In their approach the flow field was generated with the 2-dimensional (linear) airflow model of Carruthers and Choularton164 and the droplet deposition rate was considered to be limited only by turbulent diffusion (i.e. Ra). The model was also coupled with a seeder–feeder algorithm to model the wet deposition to an extended area of complex topography.165 The model was later applied to other study areas, such as Lower Silesia, Poland,166 and Snowdonia, Wales.167
Stout et al.168 modelled particle deposition to a simplified sinusoidal terrain, coupling a linear wave solution for the wind flow with equations of particle motion to show that under strong stratification regions of enhanced deposition occur on the leeward side of the hills.
Whilst several studies have focussed on the flow and transport of the pollutants, most have ignored the fact that the standard description of the deposition process does not hold in complex terrain (see Section 3.2.1). For aerosol deposition,169,170 investigated the interaction between the hill flow and the canopy with a 2-dimensional model that combined the multi-layer dry deposition modelling approaches for particles of Petroff et al.133 with modelling concepts for quantifying the flows inside plant canopies over gentle hilly terrain.64,171,172 Their model results suggest that after the hill summit the rate of deposition (deposition velocity, Vd) fell to 30% of its flat terrain value for super-micrometre particles, but that the hill summit effect was smaller on sub-micrometre particles and may therefore the hill summit effect would be smaller for gases also. A combination of wind tunnel experiments, analytical models and LES comparisons for 2D and 3D hills covered with vegetation has shown significant differences between flow patterns and diffusion of particles (both heavy and light) over 2D and axisymmetric hills with the same profile.173–176
(ii) Reynolds-averaged Navier–Stokes (RANS) models.
These types of closure models have developed continuously over the past decades, for example through the introduction of higher-order closure schemes and introduction of non-linear equations, which has gradually extended their range of applicability to steeper slopes and across different atmospheric stability regimes. However, RANS models continue to have problems representing the flow created by abrupt surface features and/or where flow separation occurs.
There are a large number of RANS models used for modelling dispersion in complex terrain, but few studies have focussed on deposition and fewer still on Nr compounds specifically. Michioka et al.177 simulated dry and wet deposition across idealised ridges and cone-shaped hills in an attempt to reproduce the wind tunnel results of Parker and Kinnersley.125 They achieved reasonable agreement, but their use of a constant deposition velocity again does not capture the impact of the flow and turbulence field on the deposition process itself. The authors also note that because there are no reliable experimental data for the wet deposition rate for a cone or a two-dimensional ridge, the accuracy of their proposed wet deposition procedure has not yet been confirmed. They point out that, to construct a reliable model, a comparison of the wet deposition obtained by the proposed wet deposition model with observations is required. However ambitious field experiments to generate understanding of the processes and data for the modellers to compare are needed to make significant progress.
A number of higher resolution models have been developed to model the flow and turbulence over complex terrain. One example is the commercial semi-analytical FLOWSTAR model,178 based on the theoretical work of Jackson and Hunt179 and Hunt et al.180 Coe et al.181 used FLOWSTAR to model occult deposition to complex terrain. Whilst the model aimed to reproduce the transport pattern, the deposition rate was taken from micrometeorological flux measurements which were necessarily taken over relatively flat terrain. Therefore it does not mechanistically account for the impact of topography on the deposition velocity itself.
A number of studies have used models that use the Eulerian flow fields from RANS models to drive a stochastic Lagrangian particle model, similar to the larger scale models discussed below. Using this approach, Ahmadi and Li182 simulated the impact of buildings on size-segregated aerosol deposition. Arritt183 simulated sulphur dioxide (SO2) deposition from a point source to complex terrain. As with the other studies, though, the study used a flat-earth representation for the deposition rate.
Blocken et al.184 provided a review of the RANS studies that existed at the time and noted that most dealt with isolated hills rather than more complex situations, a situation that does not appear to have changed. They themselves applied a model with modified k-ε closure to simulate the wind flow in an area of irregular hilly terrain in Galicia, Spain.
(iii) Large eddy simulation.
Large-eddy simulation is a computational fluid dynamics (CFD) approach that, like NWP models and RANS schemes, solves the Navier–Stokes equation to derive the flow and turbulence field, but unlike NWP and RANS models attempts to resolve the motion of individual turbulent eddy motions down to a specifiable size below which a sub-grid parameterisation comes into effect. WRF models can be used as LES model as well as NWP models, which have planetary boundary layer (PBL) schemes to address gaps in turbulence spectra.
These models can explicitly resolve the impact of terrain, obstacles, and changes in surface roughness down to a specifiable scale and therefore offer advantages over RANS configuration. They can also be embedded into other larger scale models. However, they are computationally very demanding, and studies have therefore focussed on small study volumes and short-term simulations. Most applications of LES models have been targeted at the simulation of cloud simulations over complex terrain, turbulence and, in the area of air pollution, concentration fields. They can be used, for example, to model the turbulence within street canyons and its impact on concentrations, which can vary greatly across the street canyon, in response to wind direction.
LES has been used to model the wind flows and turbulence fields over complex terrain. Wood185 summarised the approaches available at the time and expansion of computational capability in the past 20 years have led to an increase in the number of studies since. For example, Bhuiyan and Alam,186 Liu et al.187 and Yang et al.188 all modelled the flow over idealised complex terrain, such as isolated hills and ridges.
LES studies aimed at simulating small-scale variations in dry deposition are comparably rare, however: a number of studies have applied LES to quantify the uptake of pollutants to urban trees planted, e.g., within tree canopies.189
3.1.5 Modelling of wet deposition.
The Parameter-elevation Regression on Independent Slopes Model (PRISM190) adds influences of topographical and geographical features to the mapping of wet deposition based on the measurements of the US American National Atmospheric Deposition Program/National Trends Network (NADP/NTN).191
In an alternative approach, the inferential UK CBED model accounts for topographic enhancement of wet deposition by estimating the fraction of annual precipitation that is due to orographic enhancement by the seeder feeder process using mapped precipitation (Met office) data. The additional precipitation in the uplands is assumed to be entirely due to seeder–feeder scavenging of orographic cloud water whose concentration exceeds that of the low-level precipitation field (by a factor of two) and derived from measurements described by Dore et al.192,193
3.1.6 Parameterising the impact of complex topography in large-scale models.
The more sophisticated RANS models and especially the LES models are currently too computationally demanding to provide annual deposition maps at a national scale. They are, however, suited to assess the importance of orographic effects on deposition in small study areas or to derive sub-grid parameterisations or meta-models which could be used to develop simplified representations of the effects suitable for use in larger scale ACTMs.
An example modelling system was described by Parker,159 who used a GIS system to combine empirical deposition modification factors based on the wind tunnel work of Parker and Kinnersley125 with high resolution DEM (Digital Elevation Model) data to identify areas (slopes) in which particle dry deposition would be expected to be enhanced or suppressed for a given wind direction. Such a model could theoretically be linked to a dispersion model such as NAME. Model predictions compared favourably with a data set for deposition of radioactive material to Cumbrian hills during the Windscale nuclear accident derived by Argyraki et al.194 from 134Cs and 137Cs data recorded by an aerial survey and corrected for subsequent deposition from the Chernobyl accident.
In another scoping study, for the deposition parameterisation itself Hicks195 proposed a pragmatic approach to reduce Ra in regions with slopes exceeding 1 in 7 as mentioned above, but conceded that a validation measurement programme still had to be devised.
Weathers et al.196 presented an empirical approach to modelling Nr and reactive sulphur (Sr) deposition to a mountainous forested landscape, using a GIS implementation of a statistical model in which a base deposition was modified by correction factors that accounted for elevation, slope and aspect, topographic exposure, forest type and tree characteristics. For this the scaling factors were derived by applying a multi-linear statistical model to the ratio between local deposition derived from throughfall measurements and a ‘baseline’ deposition from a nearby NAPD/CASTNET measurement site.
Following a similar approach, Kirchner et al.20 surveyed Nr deposition along differently exposed slopes in the Bavarian Alps via forest throughfall measurements and modelled the dry deposition contributions at the sites with an inferential model that used literature deposition rates, modified by a number of semi-empirical correction factors that were meant to compensate for inclination, wind exposure, frequency of inversions and the frequency of upslope winds. Total correction factors ranged from 0.5 to 2.1. The origin of the values used for these factors is somewhat unclear. They refer to a publication of Benedict et al.197 which reported observations of Nr species in Rocky Mountain National Park. However, that study reports on the impact of winds on concentrations (related to transport patterns and air mass origin for that particular site) rather than on deposition velocities. Nevertheless, Kircher et al.20 provide another example of how, in principle, local site characteristics may be used to derive correction factors for deposition rates.
Draaijers et al.116,198 developed an upscaling approach for the related problem of deposition enhancements to forest edges for Sr, Nr and base cations. They also used throughfall measurements to derive aspect-specific enhancements factors across forest edges, and then applied these to Dutch national forest stand data to conclude that edge effects increase the national total deposition of acidifying compounds to forest by 5–10% and of sea salt particles by 20–25%.
Being derived from throughfall measurements these approaches are subject to large uncertainties and they lend themselves only to forest canopy and cannot easily be extended to short vegetation where estimates of terrain-influenced variation in deposition velocity are more difficult to obtain. In addition, absolute deposition values derived in this way are subject to uncertainties related to uptake, leaching and transformations within the canopy (see Section 2.1).
3.2 Summary of status on model availability and development to estimate dry deposition to complex terrain
The modelling of pollutant dry deposition to complex terrain consists of three elements: (i) prediction of the flow and turbulence field, (ii) modelling of pollutant transport and dispersion and (iii) modelling of the interaction with the surface and plant canopies. In addition, for wet deposition the models need to predict the presence of (iv) cloud and the location and amount of precipitation and accurately simulate (v) the washout/rainout processes.
Whilst both RANS and LES models have been developed to achieve steps (i) and (ii) for limited areas in great detail, the impact of complex terrain on the dry deposition process has hardly been studied at all. In addition, most effort has gone into modelling particles as a function of size, to simulate, for example, nuclear accidents, industrial releases, pollen and seed dispersal, erosion and dune drift. No mechanistic work could be identified that has looked at the interaction between hills, plant and the dry deposition of gaseous pollutants.
Although the problem that current ACTM approaches perform inadequately in complex upland terrain has been recognised widely in the literature, very few operational solutions have been suggested that may be introduced into ACTMs to represent these processes. In the short term a pragmatic approach could be adopted in which “flat earth” estimates of deposition are modified for terrain influences using heuristics derived from field measurements or from more computationally intensive models. A limiting factor for this is the paucity of measurements made in areas of non-flat topography which could be used to test models and derive heuristics and this is again related to the difficulty of making reliable measurements of all three types of deposition (dry, wet, cloud) at complex and exposed sites.
4 Summarising discussion
The considerable body of peer-reviewed papers, reports and other items reviewed in this study has highlighted both the strengths and weaknesses in the tools available to scientists, regulators and policy makers. This review highlights that there has not been a coherent global research effort to constrain the uncertainties in Nr deposition over complex terrain, despite the clearly identified risk of N deposition to ecosystems and water quality. All evidence identified enhanced Nr deposition occurs across complex terrain occurs, both locally (e.g. on windward slopes and forest edges) and at the catchment scale, and the magnitude of the enhancement is not known. The process is especially important in uplands, where precipitation is enhanced, and especially in the areas where annual precipitation exceeds 1000 mm. In general, most biases in methods used currently appear to lead to an under-prediction of Nr deposition across complex terrain, with the exception of the emissions of gaseous Nr compounds from surfaces (i.e. negative deposition terms), which is also under-predicted in models and not generally measured in complex terrain. The spatial variability due to dry deposition and turbulence is important though there is discussion in the community as to the relative magnitude of this compared to the importance of the importance of the seeder–feeder effect – in particular for deposition in the UK. However further evidence is needed to prove this.
In order to understand the scale of the potential issue, a highly speculative estimate of the magnitude of enhancement compared to current understanding of the process for simple terrain, for each type of Nr deposition, is summarised in Table 1. Combining our current assessment of the likely scale of the biases of the individual processes, and assuming equal contributions of each process to deposition (though the authors note that this will be variable dependent on location), this would lead to a net increase of Nr deposition in complex terrain by a factor of 1.4 to 3.6. This calculation is not tested and highly uncertain; however, it is very likely that the Nr deposition across complex terrain is underestimated and may be almost four times higher than currently predicted in some circumstances. This calculation is done for the furthering of discussion of this topic and should be refined in future work.
Table 1 Estimation of general Nr deposition process biases for models and measurements
N
r dep. type |
Component |
Model/measure |
Bias due to complex terrain misrepresentation |
Effect on amount of N dep. when bias added in |
Comment |
Confidence on BIAS estimate |
E
std factor |
Occult |
Fog/mist/cloud |
Modelled |
Under-predicted |
Up |
Fog interactions at surface increases surface uptake. Will be very specific site |
Confident |
2–10 |
Occult |
Fog |
Measure |
Large uncertainty |
Do not know |
Few studies |
Low |
Wet dep. |
Rainfall |
Modelled |
Under-predicted |
Up |
Assume gas and PM precursor in model not limited |
Confident |
1.5–2 |
Wet dep. |
Bulk deposition |
Measure |
Dry deposition = positive bias (locally driven); evaporation/bioprocessing = negative bias |
Do not know |
Likely counteracting processes |
Medium |
Dry dep. |
PM |
Modelled |
Under-predicted |
Up |
More surface interactions |
Medium |
1.5–3 |
Dry dep. |
PM |
Measure |
Under-predicted |
Up |
|
Medium |
Dry dep. |
N
r gases |
Modelled |
Under-predicted |
Up |
Surface area and RH/T/wetness not well represented. Terrain – atmosphere not well characterised |
Confident |
1.5–3 |
Dry dep. |
N
r gases |
Measure |
Under-predicted |
UP |
Measurements not in complex terrain |
Confident |
Dry emission |
N
r gases |
Modelled |
Under-predicted |
Down |
Biochemical and bidirectional physical chemistry processes |
Confident |
2–10 |
Dry emission |
N
r gases |
Measure |
Under-predicted |
Down |
Measurements not done |
Confident |
The damage costs of Nr pollution across both ecosystems and human health and economies are well established.11 From the literature assessed in this study there is clear evidence that there is a significant risk of enhanced Nr deposition, and hence enhanced damage, in complex terrain via wet, dry and occult deposition compared to the “flat earth” case. Additional complications also exist, such as NH3 gas deposition being significantly more damaging to plant life than deposition of an equivalent amount of Nrvia wet deposition or as aerosol.199 The short and long-term effects of this enhanced deposition have not been quantified in the UK; however, there are many studies in the literature which identify ecosystem effects and water quality. It is therefore reasonable to expect that there is an additional risk due to complex terrain in the UK, particularly in areas in proximity to high Nr emissions (such as NH3 point sources).
Improvements in computational capacity and associated developments in model development over the past 30 years now permit terrain-resolving models to be operated at high resolution, at least over limited domains and time-spans. Most of the model applications have focussed on concentrations, however, or the interpretation of fluxes of compounds that are limited by production/consumption (such as CO2). We only identified a single effort169,170 that explicitly addressed the interaction between hill flow and dry deposition where the flux is limited by turbulence, in this case focussing on the deposition of particles to forested terrain. Over the same time, there has been even less improvement in ambient measurement approaches, e.g. for measuring cloud and wet deposition under windy conditions, or in methods to derive dry deposition fluxes to complex terrain. As a result, robust measurement data are lacking to validate any emerging modelling approaches. Wind tunnel studies addressing the effect of complex terrain on dry deposition remain rare.
Expanded monitoring and additional process-level research, including new wind tunnel studies, are both needed to better understand deposition of reduced nitrogen compounds (NHx), its contribution to total Nr deposition budgets, and the processes by which it deposits to ecosystems. There is a case for repeating the large-scale hill cloud experiments of the 1990s,200,201 with state-of-the-art instrumentation and moving from single hills to larger areas of complex terrain, e.g. to study the impact of hill chains. This would require a large community effort. Experiments need to be designed to deliver quantitative information, fluxes and the factors driving them, for model validation and to inform parameterisation of operational models. In particular there is a high priority for establishment of long-term sites for process level measurements of reactive chemical fluxes to serve the atmospheric chemistry and ecological communities. However it is also noted that significant method innovation and adaptation may be required to ensure the uncertainties in measurements are lower than the magnitude of the fluxes to be measured.
5 Author contributions
This manuscript was compiled by multiple authors, each with expertise on one or more aspects of the review. Each of the authors have contributed both to the manuscript and to discussions during the writing of the report. Nicholas Cowan: carried out literature review, contributed to writing and managed the author contributions to the manuscript. Eiko Nemitz: primary contributor to the modelling aspects of the review. John T. Walker: expert advisor on nitrogen deposition and national-scale deposition modelling. David Fowler: expert advisor on measurements and historical data and modelling efforts. John J. Finnigan: expert advisor on deposition and dispersion modelling. Helen N. Webster: expert on atmospheric dispersion modelling. Peter Levy: expert in micrometeorological monitoring and flux measurements. Marsailidh Twigg: expert on Nr measurement methods and ammonia deposition. Sim Y. Tang: expert on Nr measurement methods and ammonia deposition. Nuria Bachiller-Jareno: UK scale mapping and terrain expert. Philip Trembath: UK scale mapping and terrain expert. Robert P. Kinnersley: expert advisor on dry deposition and particle physics. Christine F. Braban: primary contributor to the measurement aspects of the review.89
Conflicts of interest
The authors declare no conflict of interest.
Acknowledgements
We would like to thank the UK Department for Environment, Food and Rural Affairs (DEFRA) and the Environment Agency (EA) for the funding to carry out this review as part of the Natural Capital and Ecosystem Assessment programme pilot (Contract Reference: 32150). We also acknowledge contribution from UKSCAPE programme, funded by the Natural Environment Research Council as National Capability (Award number NE/R016429/1). We thank expert contributors who joined discussions, which helped inform us during the review. These include Matt Hort, Samantha Smith, Humphry Lean and Gerd Folbeth from the UK Met Office, and Benjamin Loubet, Christophe Flechard and Sylvain Dupont from the Institut national de la recherche agronomique (INRA, France).We acknowledge Dr Massimo Vieno as well as Beth Raine and Cristina Martin Hernandez, all of UKCEH, for providing the EMEP and CBED model data, respectively. The views expressed in this paper are those of the authors and do not necessarily reflect the views of the U.S. Environmental Protection Agency.
References
- T. E. H. Allott, C. J. Curtis, J. Hall, R. Harriman and R. W. Battarbee, The impact of nitrogen deposition on upland surface waters in Great Britain: a regional assessment of nitrate leaching, Water, Air, Soil Pollut., 1995, 85, 297–302, DOI:10.1007/bf00476845.
-
C. Curtis, G. L. Simpson, J. Shilland, S. Turner, M. Kernan, D. T. Monteith, N. Rose, C. Evans, D. Norris, E. Rowe, B. Emmett, A. Sowerby, T. Heaton, S. Maberly, N. Ostle and H. Grant, Freshwater umbrella-the effects of nitrogen deposition & climate change on freshwaters in the UK, Final report, 2007, available from: https://uk-air.defra.gov.uk/library/reports?report_id=502 Search PubMed.
-
G. Farr, J. Hall, L. Jones, M. Whiteman, A. Haslam, N. Phillips, S. Tang, H. Williams, P. Davison and D. Lapworth, Atmospheric deposition at groundwater dependent wetlands phase 2: nutrient source apportionment case studies from England and Wales, British Geological Survey Internal Report OR/17/021, 2019 Search PubMed.
- V. A. Bell, P. S. Naden, E. Tipping, H. N. Davies, E. Carnell and J. A. C. Davies,
et al., Long term simulations of macronutrients (C, N and P) in UK freshwaters, Sci. Total Environ., 2021, 776, 145813, DOI:10.1016/j.scitotenv.2021.145813.
- E. Tipping, J. A. C. Davies, P. A. Henrys, G. J. D. Kirk, A. Lilly and U. Dragosits,
et al., Long-term increases in soil carbon due to ecosystem fertilization by atmospheric nitrogen deposition demonstrated by regional-scale modelling and observations, Sci. Rep., 2017, 7, 1890, DOI:10.1038/s41598-017-02002-w.
-
RoTAP, Review of Transboundary Air Pollution: Acidification, Eutrophication, Ground Level Ozone and Heavy Metals in the UK. Contract Report to the Department for Environment, Food and Rural Affairs. Centre for Ecology & Hydrology, 2012, available from: https://www.rotap.ceh.ac.uk/ Search PubMed.
-
G. Farr and J. Hall, Atmospheric deposition at groundwater dependent wetlands: implications for effective catchment management and Water Framework Directive groundwater classification in England and Wales, British Geological Survey, 2014, available from: https://rgdoi.net/10.13140/RG.2.2.13249.15209 Search PubMed.
- R. D. Saylor, B. D. Baker, P. Lee, D. Tong, L. Pan and B. B. Hicks, The particle dry deposition component of total deposition from air quality models: right, wrong or uncertain?, Tellus B, 2019, 71, 1550324, DOI:10.1080/16000889.2018.1550324.
- J. T. Walker, M. D. Bell, D. Schwede, A. Cole, G. Beachley and G. Lear,
et al., Aspects of uncertainty in total reactive nitrogen deposition estimates for North American critical load applications, Sci. Total Environ., 2019, 690, 1005–1018, DOI:10.1016/j.scitotenv.2019.06.337.
- D. K. Farmer, E. K. Boedicker and H. M. DeBolt, Dry Deposition of Atmospheric Aerosols: Approaches, Observations, and Mechanisms, Annu. Rev. Phys. Chem., 2021, 72, 375–397, DOI:10.1146/annurev-physchem-090519-034936.
-
M. A. Sutton, C. M. Howard, J. W. Erisman, G. Billen, A. Bleeker, P. Grennfelt, H. Van Grinsven and B. Grizzetti, The European nitrogen assessment: sources, effects and policy perspectives, Cambridge University Press, 2011, available from: https://www.nine-esf.org/node/204/ENA.html Search PubMed.
-
Acid Deposition at High Elevation Sites, in NATO ASI Series. Series C: Mathematical and Physical Sciences, ed. Unsworth M. H. and Fowler D., Springer Netherlands, Dordrecht, 1988, vol. 252, p. 670, ISBN 90-277-2835-6 Search PubMed.
- W. Aas, A. Mortier, V. Bowersox, R. Cherian, G. Faluvegi and H. Fagerli,
et al., Global and regional trends of atmospheric sulfur, Sci. Rep., 2019, 9, 953, DOI:10.1038/s41598-018-37304-0.
- Ø. Kaste, K. Austnes and H. A. de Wit, Streamwater responses to reduced nitrogen deposition at four small upland catchments in Norway, Ambio, 2020, 49, 1759–1770, DOI:10.1007/s13280-020-01347-3.
- E. A. Davidson, M. Keller, H. E. Erickson, L. V. Verchot and E. Veldkamp, Testing a Conceptual Model of Soil Emissions of Nitrous and Nitric Oxides, BioScience, 2000, 50, 667, DOI:10.1641/0006-3568(2000)050[0667:TACMOS]2.0.CO;2.
- W. Ouyang, X. Xu, Z. Hao and X. Gao, Effects of soil moisture content on upland nitrogen loss, J. Hydrol., 2017, 546, 71–80, DOI:10.1016/j.jhydrol.2016.12.053.
- J. N. Galloway, F. J. Dentener, D. G. Capone, E. W. Boyer, R. W. Howarth and S. P. Seitzinger,
et al., Nitrogen Cycles: Past, Present, and Future, Biogeochemistry, 2004, 70, 153–226, DOI:10.1007/s10533-004-0370-0.
- J. N. Cape, L. J. van der Eerden, L. J. Sheppard, I. D. Leith and M. A. Sutton, Evidence for changing the critical level for ammonia, Environ. Pollut., 2009, 157, 1033–1037, DOI:10.1016/j.envpol.2008.09.049.
-
E. Rowe, K. Sawicka, S. Tomlinson, P. Levy, L. F. Banin, C. Martín Hernandez, A. Fitch and L. Jones, Trends Report 2021: Trends in critical load and critical level exceedances in the UK, 2021, available from; https://www.cldm.ceh.ac.uk/sites/cldm.ceh.ac.uk/files/2106241035_Trends_Report_2021.pdf Search PubMed.
- M. Kirchner, W. Fegg, H. Römmelt, M. Leuchner, L. Ries and R. Zimmermann,
et al., Nitrogen deposition along differently exposed slopes in the Bavarian Alps, Sci. Total Environ., 2014, 470–471, 895–906, DOI:10.1016/j.scitotenv.2013.10.036.
- C. L. Meunier, M. J. Gundale, I. S. Sánchez and A. Liess, Impact of nitrogen deposition on forest and lake food webs in nitrogen-limited environments, GCB Bioenergy, 2015, 22, 164–179, DOI:10.1111/gcb.12967.
- A. J. Dore, D. C. Carslaw, C. Braban, M. Cain, C. Chemel and C. Conolly,
et al., Evaluation of the performance of different atmospheric chemical transport models and inter-comparison of nitrogen and sulphur deposition estimates for the UK, Atmos. Environ., 2015, 119, 131–143, DOI:10.1016/j.atmosenv.2015.08.008.
- R. I. Smith, D. Fowler, M. A. Sutton, C. Flechard and M. Coyle, Regional estimation of pollutant gas dry deposition in the UK: model description, sensitivity analyses and outputs, Atmos. Environ., 2000, 34, 3757–3777, DOI:10.1016/S1352-2310(99)00517-8.
- M. Vieno, M. R. Heal, M. L. Williams, E. J. Carnell, E. Nemitz and J. R. Stedman,
et al., The sensitivities of emissions reductions for the mitigation of UK PM2.5, Atmos. Chem. Phys., 2016, 16, 265–276, DOI:10.5194/acp-16-265-2016.
- Y. Ge, M. R. Heal, D. S. Stevenson, P. Wind and M. Vieno, Evaluation of global EMEP MSC-W (rv4.34) WRF (v3.9.1.1) model surface concentrations and wet deposition of reactive N and S with measurements, Geosci. Model Dev., 2021, 14, 7021–7046, DOI:10.5194/gmd-14-7021-2021.
- H. ApSimon, T. Oxley, H. Woodward, D. Mehlig, A. Dore and M. Holland, The UK Integrated Assessment Model for source apportionment and air pollution policy applications to PM2.5, Environ. Int., 2021, 153, 106515, DOI:10.1016/j.envint.2021.106515.
- J. N. Cape, N. van Dijk and Y. S. Tang, Measurement of dry deposition to bulk precipitation collectors using a novel flushing sampler, J. Environ. Monit., 2009, 11, 353–358, 10.1039/B813812E.
- H. Woodward, T. Oxley, E. C. Rowe, A. J. Dore and H. ApSimon, An exceedance score for the assessment of the impact of nitrogen deposition on habitats in the UK, Environ. Model. Softw., 2022, 150, 105355, DOI:10.1016/j.envsoft.2022.105355.
- R. Vet, R. S. Artz, S. Carou, M. Shaw, C. U. Ro and W. Aas,
et al., A global assessment of precipitation
chemistry and deposition of sulfur, nitrogen, sea salt, base cations, organic acids, acidity and pH, and phosphorus, Atmos. Environ., 2014, 93, 3–100, DOI:10.1016/j.atmosenv.2013.10.060.
- J. T. Walker, G. Beachley, H. M. Amos, J. S. Baron, J. Bash and R. Baumgardner,
et al., Toward the improvement of total nitrogen deposition budgets in the United States, Sci. Total Environ., 2019, 691, 1328–1352, DOI:10.1016/j.scitotenv.2019.07.058.
- J. T. Walker, G. Beachley, L. Zhang, K. B. Benedict, B. C. Sive and D. B. Schwede, A review of measurements of air-surface exchange of reactive nitrogen in natural ecosystems across North America, Sci. Total Environ., 2020, 698, 133975, DOI:10.1016/j.scitotenv.2019.133975.
- G. Rattray and H. Sievering, Dry deposition of ammonia, nitric acid, ammonium, and nitrate to alpine tundra at Niwot Ridge, Colorado, Atmos. Environ., 2001, 35, 1105–1109, DOI:10.1016/S1352-2310(00)00276-4.
- D. Pan, K. B. Benedict, L. M. Golston, R. Wang, J. L. Collett Jr and L. Tao,
et al., Ammonia Dry Deposition in an Alpine Ecosystem Traced to Agricultural Emission Hotpots, Environ. Sci. Technol., 2021, 55, 7776–7785, DOI:10.1021/acs.est.0c05749.
- L. C. Sieck, S. J. Burges and M. Steiner, Challenges in obtaining reliable measurements of point rainfall, Water Resour. Res., 2007, 43, W01420, DOI:10.1029/2005WR004519.
- K. F. A. Frumau, R. Burkard, S. Schmid, L. A. S. Bruijnzeel, C. Tobón and J. C. Calvo-Alvarado, A comparison of the performance of three types of passive fog gauges under conditions of wind-driven fog and precipitation, Hydrol. Processes, 2010, 25, 374–383, DOI:10.1002/hyp.7884.
- R. Rasmussen, B. Baker, J. Kochendorfer, T. Meyers, S. Landolt and A. P. Fischer,
et al., How Well Are We Measuring Snow: The NOAA/FAA/NCAR Winter Precipitation Test Bed, Bull. Am. Meteorol. Soc., 2012, 93, 811–829, DOI:10.1175/BAMS-D-11-00052.1.
- J. N. Cape, R. I. Smith, D. Fowler, K. Beswick and T. Choularton, Long-term trends in rain and cloud chemistry in a region of complex topography, Atmos. Res., 2015, 153, 335–347, DOI:10.1016/j.atmosres.2014.09.003.
- J. C. Neff, A. R. Townsend, G. Gleixner, S. J. Lehman, J. Turnbull and W. D. Bowman, Variable effects of nitrogen additions on the stability and turnover of soil carbon, Nature, 2002, 419, 915–917, DOI:10.1038/nature01136.
- J. N. Cape, M. Anderson, A. P. Rowland and D. Wilson, Organic nitrogen in precipitation across the United Kingdom, Water, Air, Soil Pollut.: Focus, 2005, 4, 25–35, DOI:10.1007/s11267-005-3010-3.
- J. N. Cape, Y. S. Tang, J. M. González-Beniacute, M. Mitošinková, U. Makkonen and M. Jocher,
et al., Organic nitrogen in precipitation across Europe, Biogeosciences, 2012, 9, 4401–4409, DOI:10.5194/bg-9-4401-2012.
- M. A. Sutton, S. Reis, S. N. Riddick, U. Dragosits, E. Nemitz and M. R. Theobald,
et al., Towards a climate-dependent paradigm of ammonia emission and deposition, Philos. Trans. R. Soc., B, 2013, 368, 20130166, DOI:10.1098/rstb.2013.0166.
- F. Paulot, D. J. Jacob, R. W. Pinder, J. O. Bash, K. Travis and D. K. Henze, Ammonia emissions in the United States, European Union, and China derived by high-resolution inversion of ammonium wet deposition data: Interpretation with a new agricultural emissions inventory (MASAGE_NH3), J. Geophys. Res.: Atmos., 2014, 119, 4343–4364, DOI:10.1002/2013jd021130.
- E. A. Marais, A. K. Pandey, M. Van Damme, L. Clarisse, P. Coheur and M. W. Shephard,
et al., UK Ammonia Emissions Estimated With Satellite Observations and GEOS-Chem, J. Geophys. Res.: Atmos., 2021, 126 DOI:10.1029/2021jd035237.
-
I. N. Harman, J. J. Finnigan, S. E. Belcher and E. van Gorsel, Limits to accuracy and optimal instrument deployment for eddy flux measurement in complex terrain, in Proceedings of the 6th GEWEX and 2nd iLEAPS Science Conference, Melbourne, AU, 2009 Search PubMed.
- R. Hiller, M. J. Zeeman and W. Eugster, Eddy-Covariance Flux Measurements in the Complex Terrain of an Alpine Valley in Switzerland, Bound.-Layer Meteorol., 2008, 127, 449–467, DOI:10.1007/s10546-008-9267-0.
- B. B. Hicks and D. D. Baldocchi, Measurement of Fluxes Over Land: Capabilities, Origins, and Remaining Challenges, Bound.-Layer Meteorol., 2020, 177, 365–394, DOI:10.1007/s10546-020-00531-y.
- D. D. Baldocchi, B. B. Hincks and T. P. Meyers, Measuring Biosphere-Atmosphere Exchanges of Biologically Related Gases with Micrometeorological Methods, Ecology, 1988, 69, 1331–1340, DOI:10.2307/1941631.
- R. L. Desjardins and E. R. Lemon, Limitations of an eddy-correlation technique for the determination of the carbon dioxide and sensible heat fluxes, Bound.-Layer Meteorol., 1974, 5, 475–488, DOI:10.1007/BF00123493.
- J. A. Businger and S. P. Oncley, Flux Measurement with Conditional Sampling, J. Atmos. Ocean. Technol., 1990, 7, 349–352, DOI:10.1175/1520-0426(1990)007<0349:FMWCS>2.0.CO;2.
- A. J. Nelson, S. Koloutsou-Vakakis, M. J. Rood, L. Myles, C. Lehmann and C. Bernacchi,
et al., Season-long ammonia flux measurements above fertilized corn in central Illinois, USA, using relaxed eddy accumulation, Agric. For. Meteorol., 2017, 239, 202–212, DOI:10.1016/j.agrformet.2017.03.010.
- J. Duyzer, Dry deposition of ammonia and ammonium aerosols over heathland, J. Geophys. Res., 1994, 99, 18757, DOI:10.1029/94JD01210.
- U. Zöll, C. Brümmer, F. Schrader, C. Ammann, A. Ibrom and C. R. Flechard,
et al., Surface-atmosphere exchange of ammonia over peatland using QCL-based eddy-covariance measurements and inferential modeling, Atmos. Chem. Phys., 2016, 16, 11283–11299, DOI:10.5194/acp-16-11283-2016.
- A. Moravek, S. Singh, E. Pattey, L. Pelletier and J. G. Murphy, Measurements and quality control of ammonia eddy covariance fluxes: a new strategy for high-frequency attenuation correction, Atmos. Meas. Tech., 2019, 12, 6059–6078, DOI:10.5194/amt-12-6059-2019.
- K. Sun, L. Tao, D. J. Miller, M. A. Zondlo, K. B. Shonkwiler and C. Nash,
et al., Open-path eddy covariance measurements of ammonia fluxes from a beef cattle feedlot, Agric. For. Meteorol., 2015, 213, 193–202, DOI:10.1016/j.agrformet.2015.06.007.
- O. Marx, C. Brümmer, C. Ammann, V. Wolff and A. Freibauer, TRANC – a novel fast-response converter to measure total reactive atmospheric nitrogen, Atmos. Meas. Tech., 2012, 5, 1045–1057, DOI:10.5194/amt-5-1045-2012.
- C. Ammann, V. Wolff, O. Marx, C. Brümmer and A. Neftel, Measuring the biosphere-atmosphere exchange of total reactive nitrogen by eddy covariance, Biogeosciences, 2012, 9, 4247–4261, DOI:10.5194/bg-9-4247-2012.
- U. Zöll, A. M. Lucas-Moffat, P. Wintjen, F. Schrader, B. Beudert and C. Brümmer, Is the biosphere-atmosphere exchange of total reactive nitrogen above forest driven by the same factors as carbon dioxide? An analysis using artificial neural networks, Atmos. Environ., 2019, 206, 108–118, DOI:10.1016/j.atmosenv.2019.02.042.
- R. Ramsay, C. F. Di Marco, M. Sörgel, M. R. Heal, S. Carbone and P. Artaxo,
et al., Concentrations and biosphere–atmosphere fluxes of inorganic trace gases and associated ionic aerosol counterparts over the Amazon rainforest, Atmos. Chem. Phys., 2020, 20, 15551–15584, DOI:10.5194/acp-20-15551-2020.
-
D. Fowler, N. Cape, R. Smith, E. Nemitz, M. Sutton, T. Dore, M. Coyle, A. Crossley, R. Storeton-West, J. Muller, G. Phillips, R. Thomas, M. Vieno, S. Tang, D. Famulari, M. Twigg, B. Bealey, D. Benham, G. Hayman, H. Lawrence, K. Vincent, H. Fagerli and D. Simpson, Acid Deposition Processes. Final report to Defra. NERC/Centre for Ecology & Hydrology; 2007, p. 110 (CEH Project Number: C02379, RMP 2258), (Unpublished) Search PubMed.
- D. Famulari, D. Fowler, E. Nemitz, K. J. Hargreaves, R. L. Storeton-West and G. Rutherford,
et al., Development of a low-cost system for measuring conditional time-averaged gradients of SO2 and NH3, Environ. Monit. Assess., 2009, 161, 11–27, DOI:10.1007/s10661-008-0723-6.
- R. S. Massad, E. Nemitz and M. A. Sutton, Review and parameterisation of bi-directional ammonia exchange between vegetation and the atmosphere, Atmos. Chem. Phys., 2010, 10, 10359–10386, DOI:10.5194/acp-10-10359-2010.
- C. R. Flechard, D. Fowler, M. A. Sutton and J. N. Cape, A dynamic chemical model of bi-directional ammonia exchange between semi-natural vegetation and the atmosphere, Q. J. R. Metereol. Soc., 1999, 125, 2611–2641, DOI:10.1002/qj.49712555914.
- D. Fowler, K. Pilegaard, M. A. Sutton, P. Ambus, M. Raivonen and J. Duyzer,
et al., Atmospheric composition change: Ecosystems–Atmosphere interactions, Atmos. Environ., 2009, 43, 5193–5267, DOI:10.1016/j.atmosenv.2009.07.068.
- J. J. Finnigan and S. E. Belcher, Flow over a hill covered with a plant canopy, Q. J. R. Metereol. Soc., 2004, 130, 1–29, DOI:10.1256/qj.02.177.
- C. Feigenwinter, C. Bernhofer, U. Eichelmann, B. Heinesch, M. Hertel and D. Janous,
et al., Comparison of horizontal and vertical advective CO2 fluxes at three forest sites, Agric. For. Meteorol., 2008, 148, 12–24, DOI:10.1016/j.agrformet.2007.08.013.
- J. Mann, N. Angelou, J. Arnqvist, D. Callies, E. Cantero and R. C. Arroyo,
et al., Complex terrain experiments in the New European Wind Atlas, Philos. Trans. R. Soc., A, 2017, 375, 20160101, DOI:10.1098/rsta.2016.0101.
- R. Engelmann, U. Wandinger, A. Ansmann, D. Müller, E. Žeromskis and D. Althausen,
et al., Lidar Observations of the Vertical Aerosol Flux in the Planetary Boundary Layer, J. Atmos. Ocean. Technol., 2008, 25, 1296–1306, DOI:10.1175/2007jtecha967.1.
-
L. Järvi, L. A. Arboledas, R. Engelmann, H. Baars, A. Apituley, O. Makes, E. O'Connor, A. Manninen and V. Zdimal, Deliverable D12.6: Final report on the comparison and suitability of the different techniques to measure the aerosol particle fluxes at the ACTRIS-2 sites, 2019, available from: https://ec.europa.eu/research/participants/documents/downloadPublic?documentIds=080166e5c4f35196%26appId=PPGMS Search PubMed.
- X. Wang, G. Dai, S. Wu, K. Sun, X. Song and W. Chen,
et al., Retrieval and Calculation of Vertical Aerosol Mass Fluxes by a Coherent Doppler Lidar and a Sun Photometer, Remote Sens., 2021, 13, 3259, DOI:10.3390/rs13163259.
- F. Chiaravalloti, L. Brocca, A. Procopio, C. Massari and S. Gabriele, Assessment of GPM and SM2RAIN-ASCAT rainfall products over complex terrain in southern Italy, Atmos. Res., 2018, 206, 64–74, DOI:10.1016/j.atmosres.2018.02.019.
- H. W. Lean, J. F. Barlow and C. H. Halios, The impact of spin-up and resolution on the representation of a clear convective boundary layer over London in order 100 m grid-length versions of the Met Office Unified Model, Q. J. R. Metereol. Soc., 2019, 145, 1674–1689, DOI:10.1002/qj.3519.
- C. Liu, R. P. Allan, M. Brooks and S. Milton, Comparing Tropical Precipitation Simulated by the Met Office NWP and Climate Models with Satellite Observations, J. Appl. Meteorol. Climatol., 2014, 53, 200–214, DOI:10.1175/JAMC-D-13-082.1.
- Y. S. Tang, C. F. Braban, U. Dragosits, A. J. Dore, I. Simmons and N. van Dijk,
et al., Drivers for spatial, temporal and long-term trends in atmospheric ammonia and ammonium in the UK, Atmos. Chem. Phys., 2018, 18, 705–733, DOI:10.5194/acp-18-705-2018.
- D. Fowler, P. Brimblecombe, J. Burrows, M. R. Heal, P. Grennfelt and D. S. Stevenson,
et al., A chronology of global air quality, Philos. Trans. R. Soc., A, 2020, 378, 20190314, DOI:10.1098/rsta.2019.0314.
- J. Feng, R. Vet, A. Cole, L. Zhang, I. Cheng and J. O'Brien,
et al., Inorganic chemical components in precipitation in the eastern U.S. and Eastern Canada during 1989–2016: temporal and regional trends of wet concentration and wet deposition from the NADP and CAPMoN measurements, Atmos. Environ., 2021, 254, 118367, DOI:10.1016/j.atmosenv.2021.118367.
- Y. S. Tang, C. R. Flechard, U. Dämmgen, S. Vidic, V. Djuricic and M. Mitosinkova,
et al., Pan-European rural monitoring network shows dominance of NH3 gas and NH4NO3 aerosol in inorganic atmospheric pollution load, Atmos. Chem. Phys., 2021, 21, 875–914, DOI:10.5194/acp-21-875-2021.
- E. D. Palmes and R. H. Lindenboom, Ohm's law, Fick's law, and diffusion samplers for gases, Anal. Chem., 1979, 51, 2400–2401, DOI:10.1021/ac50050a026.
- M. Ferm and H. Rodhe, J. Atmos. Chem., 1997, 27, 17–29, DOI:10.1023/A:1005816621522.
- Y. S. Tang, J. N. Cape and M. A. Sutton, Development and Types of Passive Samplers for Monitoring Atmospheric NO2 and NH3 Concentrations, Sci. World J., 2001, 1, 513–529, DOI:10.1100/tsw.2001.82.
-
C. F. Braban, F. de Bree, S. Crunaire, M. Fröhlich, A. Fromage-Mariette, E. Goelen, T. Hafkenscheid, M. Hangartner, C. van Hoek, N. A. Martin, B. Michen, E. Noordijk, J. M. Stoll, M. M. Twigg, Y. S. Tang, N. Cowan and J. Poskitt, Literature review on the performance of diffusive samplers for the measurement of ammonia in ambient air and emissions to air, Edinburgh, Centre for Ecology & Hydrology, 2018, p. 85 (CEH Project no. C05204, C05967, C04544, C05952, C06942) Search PubMed.
- C. R. Flechard, E. Nemitz, R. I. Smith, D. Fowler, A. T. Vermeulen and A. Bleeker,
et al., Dry deposition of reactive nitrogen to European ecosystems: a comparison of inferential models across the NitroEurope network, Atmos. Chem. Phys., 2011, 11, 2703–2728, DOI:10.5194/acp-11-2703-2011.
- M. D. Castillo, J. Wagner, G. S. Casuccio, R. R. West, F. R. Freedman and H. M. Eisl,
et al., Field testing a low-cost passive aerosol sampler for long-term measurement of ambient PM2.5 concentrations and particle composition, Atmos. Environ., 2019, 216, 116905, DOI:10.1016/j.atmosenv.2019.116905.
- T. L. Sparks and J. Wagner, Composition of particulate matter during a wildfire smoke episode in an urban area, Aerosol Sci. Technol., 2021, 55, 734–747, DOI:10.1080/02786826.2021.1895429.
- A. Comerón, C. Muñoz-Porcar, F. Rocadenbosch, A. Rodríguez-Gómez and M. Sicard, Current Research in Lidar Technology Used for the Remote Sensing of Atmospheric Aerosols, Sensors, 2017, 17(6), 1450, DOI:10.3390/s17061450.
- O. Dubovik, Z. Li, M. I. Mishchenko, D. Tanré, Y. Karol and B. Bojkov,
et al., Polarimetric remote sensing of atmospheric aerosols: Instruments, methodologies, results, and perspectives, J. Quant. Spectrosc. Radiat. Transfer, 2019, 224, 474–511, DOI:10.1016/j.jqsrt.2018.11.024.
- T. I. A. N. Xiao-Min, L. Dong, X. U. Ji-Wei, W. A. N. G. Zhen-Zhu, W. A. N. G. Bang-Xin, W. U. De-Cheng, Z. H. O. N. G. Zhi-Qing, X. I. E. Chen-Bo and W. A. N. G. Ying-Jian, Review of lidar technology for atmosphere monitoring, J. Atmos. Environ. Opt., 2018, 13(5), 321 Search PubMed.
- P. Chazette, P. Couvert, H. Randriamiarisoa, J. Sanak, B. Bonsang and P. Moral,
et al., Three-dimensional survey of pollution during winter in French Alps valleys, Atmos. Environ., 2005, 39, 1035–1047, DOI:10.1016/j.atmosenv.2004.10.014.
- J. N. Cape, R. I. Smith, D. Fowler, K. Beswick and T. Choularton, Long-term trends in rain and cloud chemistry in a region of complex topography, Atmos. Res., 2015, 153, 335–347, DOI:10.1016/j.atmosres.2014.09.003.
- V. A. Mohnen and R. J. Vong, A climatology of cloud chemistry for the eastern United States derived from the mountain cloud chemistry project, Environ. Rev., 1993, 1, 38–54, DOI:10.1139/a93-005.
- M. Błaś, M. Sobik and R. Twarowski, Changes of cloud water chemical composition in the Western Sudety Mountains, Poland, Atmos. Res., 2008, 87, 224–231, DOI:10.1016/j.atmosres.2007.11.00.
- K. M. Beswick, K. J. Hargreaves, M. W. Gallagher, T. W. Choularton and D. Fowler, Size-resolved measurements of cloud droplet deposition velocity to a forest canopy using an eddy correlation technique, Q. J. R. Metereol. Soc., 1991, 117, 623–645, DOI:10.1002/qj.49711749910.
- D. Fowler, R. Mourne and D. Branford, The application of 210Pb inventories in soil to measure long-term average wet deposition of pollutants in complex terrain, Water, Air, Soil Pollut., 1995, 85, 2113–2118, DOI:10.1007/BF01186146.
- S. V. Hering, D. L. Blumenthal, R. L. Brewer, G. Alan, M. Hoffmann and J. A. Kadlecek,
et al., Field intercomparison of five types of fog water collectors, Environ. Sci. Technol., 1987, 21, 654–663, DOI:10.1021/es00161a006.
- S. L. Isil, T. Gebhart, K. Rogers and C. A. Wanta, Magnitude and Trends of High-elevation Cloud Water Pollutant Concentrations and Modeled Deposition Fluxes, J. Environ. Sci. Eng. B, 2017, 6, 127–143, DOI:10.17265/2162-5263/2017.03.003.
- E. M. Elliott, Z. Yu, A. S. Cole and J. G. Coughlin, Isotopic advances in understanding reactive nitrogen deposition and atmospheric processing, Sci. Total Environ., 2019, 662, 393–403, DOI:10.1016/j.scitotenv.2018.12.177.
- I. Bourgeois, J. Savarino, J. Némery, N. Caillon, S. Albertin and F. Delbart,
et al., Atmospheric nitrate export in streams along a montane to urban gradient, Sci. Total Environ., 2018, 633, 329–340, DOI:10.1016/j.scitotenv.2018.03.141.
- U. Tsunogai, T. Miyauchi, T. Ohyama, D. D. Komatsu, F. Nakagawa and Y. Obata,
et al., Accurate and precise quantification of atmospheric nitrate in streams draining land of various uses by using triple oxygen isotopes as tracers, Biogeosciences, 2016, 13, 3441–3459, DOI:10.5194/bg-13-3441-2016.
- A. S. Likuku, Wet deposition of 210 Pb aerosols over two areas of contrasting topography, Environ. Res. Lett., 2006, 1, 014007, DOI:10.1088/1748-9326/1/1/014007.
- D. Branford, D. Fowler and M. V. Moghaddam, Study of Aerosol Deposition at a Wind Exposed Forest Edge Using 210Pb and 137Cs Soil Inventories, Water, Air, Soil Pollut., 2004, 157, 107–116, DOI:10.1023/B:WATE.0000038879.99600.69.
- D. Fowler, R. I. Smith, I. D. Leith, A. Crossley, R. W. Mourne and D. W. Branford,
et al.
, Water, Air, Soil Pollut., 1998, 105, 459–470, DOI:10.1023/A:1005043829181.
-
R. Mourne, Lead-210 as a tracer for acidic deposition in areas of complex topography, PhD Thesis, Univ. of Edinburgh, 1993.
-
M. V. Moghaddam, Study of surface roughness effects on deposition of atmospheric aerosols using 210Pb soil inventories, PhD Thesis, Univ. of Edinburgh, 1998.
-
F. Choubedar, Use of radio-nuclides (unsupported 210Pb, 7Be and 137Cs) in air, rain and undisturbed soil as environmental tools, PhD Thesis, Univ. of Edinburgh, 2000.
-
S. A. Likuku, The influence of topography and vegetation canopy on the deposition of atmospheric particulates studied with 210Pb and 137Cs in soil inventory measurements, PhD Thesis, Univ. of Edinburgh, 2003.
-
C. E. R. Pitcairn, I. D. Leith, D. Fowler, K. J. Hargreaves, M. Moghaddam, V. H. Kennedy, et al., Foliar Nitrogen as an Indicator of Nitrogen Deposition and Critical Loads Exceedance on a European Scale in, Acid rain 2000, Springer Netherlands, 2001, pp. 1037–1042, available from: DOI:10.1007/978-94-007-0810-5_20.
- C. Pitcairn, D. Fowler, I. Leith, L. Sheppard, S. Tang and M. Sutton,
et al., Diagnostic indicators of elevated nitrogen deposition, Environ. Pollut., 2006, 144, 941–950, DOI:10.1016/j.envpol.2006.01.049.
- S. Jovan, M. E. Fenn, M. Buhler, A. Bytnerowicz, A. Kovasi and M. Hutten,
et al., Challenges characterizing N deposition to high elevation protected areas: a case study integrating instrument, simulated, and lichen inventory datasets for the Devils Postpile National Monument and surrounding region, USA, Ecol. Indic., 2021, 122, 107311, DOI:10.1016/j.ecolind.2020.107311.
- J. G. Farmer, M. C. Graham, J. R. Bacon, S. M. Dunn, S. I. Vinogradoff and A. B. MacKenzie, Isotopic characterisation of the historical lead deposition record at Glensaugh, an organic-rich, upland catchment in rural N.E. Scotland, Sci. Total Environ., 2005, 346, 121–137, DOI:10.1016/j.scitotenv.2004.11.020.
- J. J. C. Dawson, D. Tetzlaff, A. M. Carey, A. Raab, C. Soulsby and K. Killham,
et al., Characterizing Pb Mobilization from Upland Soils to Streams Using 206Pb/207Pb Isotopic Ratios, Environ. Sci. Technol., 2009, 44, 243–249, DOI:10.1021/es902664d.
- D. N. Lipatov, A. I. Shcheglov and D. V. Manakhov, Spatial Distribution of Heavy Metals and 137Cs in Spruce Forest Soil under Conditions of Regional Pollution, Russ. J. Ecol., 2018, 49, 312–319, DOI:10.1134/s1067413618040100.
- Z. Zhong, H. Bing, Z. Xiang, Y. Wu, J. Zhou and S. Ding, Terrain-modulated deposition of atmospheric lead in the soils of alpine forest, central China, Sci. Total Environ., 2021, 790, 148106, DOI:10.1016/j.scitotenv.2021.148106.
- G. M. Lovett and S. E. Lindberg, Dry deposition of nitrate to a deciduous forest, Biogeochemistry, 1986, 2, 137–148, DOI:10.1007/BF02180191.
- C. S. Potter, H. L. Ragsdale and W. T. Swank, Atmospheric Deposition and Foliar Leaching in a Regenerating Southern Appalachian Forest Canopy, J. Ecol., 1991, 79, 97, DOI:10.2307/2260786.
- M. A. Sutton, J. K. Schjørring and G. P. Wyers, Plant—atmosphere exchange of ammonia, Philos. Trans. R. Soc., A, 1995, 351, 261–278, DOI:10.1098/rsta.1995.0033.
- J. Burkhardt, Microscopic processes governing the deposition of trace gases and particles to vegetation surfaces, Stud. Environ. Sci., 1995, 139–148, DOI:10.1016/s0166-1116(06)80280-3.
- G. P. J. Draaijers, R. Van Ek and W. Bleuten, Atmospheric deposition in complex forest landscapes, Bound.-Layer Meteorol., 1994, 69, 343–366, DOI:10.1007/BF00718124.
-
P. H. Schuepp, D. N. McGerrigle, H. G. Leighton, G. Paquette, R. S. Schemenauer and S. Kermasha, Observations on Wet and Dry Deposition to Foliage at a High Elevation Site, Acid Deposition at High Elevation Sites, Springer Netherlands, 1988, p. 615–637, available from: DOI:10.1007/978-94-009-3079-7_38.
- A. Avila, L. Aguillaume, S. Izquieta-Rojano, H. García-Gómez, D. Elustondo and J. M. Santamaría,
et al., Quantitative study on nitrogen deposition and canopy retention in Mediterranean evergreen forests, Environ. Sci. Pollut. Res., 2017, 24, 26213–26226, DOI:10.1007/s11356-017-8861-4.
- L. Aguillaume, S. Izquieta-Rojano, H. García-Gómez, D. Elustondo, J. M. Santamaría and R. Alonso,
et al., Dry deposition and canopy uptake in Mediterranean holm-oak forests estimated with a canopy budget model: a focus on N estimations, Atmos. Environ., 2017, 152, 191–200, DOI:10.1016/j.atmosenv.2016.12.038.
- W. Dai, C. I. Davidson, V. Etyemezian and M. Zufall, Wind Tunnel Studies of Particle Transport and Deposition in Turbulent Boundary Flows, Aerosol Sci. Technol., 2001, 35, 887–898, DOI:10.1080/02786820126851.
- J. M. Zufall, W. I. Dai and C. Davidson, Dry deposition of particles to wave surfaces: II. Wind tunnel experiments, Atmos. Environ., 1999, 33, 4283–4290, DOI:10.1016/S1352-2310(99)00178-8.
- T. A. Price, R. Stoll, J. M. Veranth and E. R. Pardyjak, A wind-tunnel study of the effect of turbulence on PM10 deposition onto vegetation, Atmos. Environ., 2017, 159, 117–125, DOI:10.1016/j.atmosenv.2017.03.043.
- P. Roupsard, M. Amielh, D. Maro, A. Coppalle, H. Branger and O. Connan,
et al., Measurement in a wind tunnel of dry deposition velocities of submicron aerosol with associated turbulence onto rough and smooth urban surfaces, J. Aerosol Sci., 2013, 55, 12–24, DOI:10.1016/j.jaerosci.2012.07.006.
- T. Ishihara, K. Hibi and S. Oikawa, A wind tunnel study of turbulent flow over a three-dimensional steep hill, J. Wind. Eng. Ind. Aerodyn., 1999, 83, 95–107, DOI:10.1016/S0167-6105(99)00064-1.
- S. T. Parker and R. P. Kinnersley, A computational and wind tunnel study of particle dry deposition in complex topography, Atmos. Environ., 2004, 38, 3867–3878, DOI:10.1016/j.atmosenv.2004.03.046.
-
S. T. Salesky, M. G. Giometto, M. Chamecki, M. Lehning and M. B. Parlange, The transport and deposition of heavy particles in complex terrain: insights from an Eulerian model for large eddy simulation, arXiv, 2019, available from: https://arxiv.org/abs/1903.03521.
- M. L. Wesely, Parameterization of surface resistances to gaseous dry deposition in regional-scale numerical models, Atmos. Environ., 1989, 23, 1293–1304, DOI:10.1016/0004-6981(89)90153-4.
-
B. B. Hicks and T. P. Meyers, Measuring and Modelling Dry Deposition in Mountainous Areas, Acid Deposition at High Elevation Sites, Springer Netherlands, 1988, pp. 541–552, available from: DOI:10.1007/978-94-009-3079-7_32.
- B. B. Hicks, On Estimating Dry Deposition Rates in Complex Terrain, J. Appl. Meteorol. Climatol., 2008, 47, 1651–1658, DOI:10.1175/2006JAMC1412.1.
- L. Giovannini, E. Ferrero, T. Karl, M. W. Rotach, C. Staquet and S. Trini Castelli,
et al., Atmospheric Pollutant Dispersion over Complex Terrain: Challenges and Needs for Improving Air Quality Measurements and Modeling, Atmosphere, 2020, 11, 646, DOI:10.3390/atmos11060646.
- W. Ruijgrok, H. Tieben and P. Eisinga, The dry deposition of particles to a forest canopy: a comparison of model and experimental results, Atmos. Environ., 1997, 31, 399–415, DOI:10.1016/S1352-2310(96)00089-1.
- H. Sievering, T. Tomaszewski and J. Torizzo, Canopy uptake of atmospheric N deposition at a conifer forest: part I -canopy N budget, photosynthetic efficiency and net ecosystem exchange, Tellus B, 2007, 59, 483–492, DOI:10.1111/j.1600-0889.2007.00264.x.
- A. Petroff, A. Mailliat, M. Amielh and F. Anselmet, Aerosol dry deposition on vegetative canopies. Part II: A new modelling approach and applications, Atmos. Environ., 2008, 42, 3654–3683, DOI:10.1016/j.atmosenv.2007.12.060.
- E. Nemitz, M. A. Sutton, J. K. Schjoerring, S. Husted and G. Paul Wyers, Resistance modelling of ammonia exchange over oilseed rape, Agric. For. Meteorol., 2000, 105, 405–425, DOI:10.1016/S0168-1923(00)00206-9.
-
R. T. McMillen, Estimating the spatial variability of trace-gas deposition velocities, Technical Memo, United States, 1990, available from: https://repository.library.noaa.gov/view/noaa/19718 Search PubMed.
-
C. M. Rogers, T. F. Lavery, M. O. Stewart, W. R. Barnard and H. K. Howell, CASTNET Methodology for Modeling Dry and Total Deposition, Air Pollution Modeling and its Application XXIII, Springer International Publishing, 2014, pp. 49–53, available from: DOI:10.1007/978-3-319-04379-1_8.
- J. R. Brook, F. Di-Giovanni, S. Cakmak and T. P. Meyers, Estimation of dry deposition velocity using inferential models and site-specific meteorology—uncertainty due to siting of meteorological towers, Atmos. Environ., 1997, 31, 3911–3919, DOI:10.1016/S1352-2310(97)00247-1.
- T. M. Thompson, M. A. Rodriguez, M. G. Barna, K. A. Gebhart, J. L. Hand and D. E. Day,
et al., Rocky Mountain National Park reduced nitrogen source apportionment, J. Geophys. Res.: Atmos., 2015, 120, 4370–4384, DOI:10.1002/2014JD022675.
- R. Zhang, T. M. Thompson, M. G. Barna, J. L. Hand, J. A. McMurray and M. D. Bell,
et al., Source regions contributing to excess reactive nitrogen deposition in the Greater Yellowstone Area (GYA) of the United States, Atmos. Chem. Phys., 2018, 18, 12991–13011, DOI:10.5194/acp-18-12991-2018.
- S. Aksoyoglu and A. S. H. Prévôt, Modelling nitrogen deposition: dry deposition velocities on various land-use types in Switzerland, Int. J. Environ. Pollut., 2018, 64, 230, DOI:10.1504/IJEP.2018.099159.
- C. Viatte, T. Lauvaux, J. K. Hedelius, H. Parker, J. Chen and T. Jones,
et al., Methane emissions from dairies in the Los Angeles Basin, Atmos. Chem. Phys., 2017, 17, 7509–7528, DOI:10.5194/acp-17-7509-2017.
- D. Sirithian and S. Thepanondh, Influence of Grid Resolution in Modeling of Air Pollution from Open Burning, Atmosphere, 2016, 7, 93, DOI:10.3390/atmos7070093.
- J. Tan, Y. Zhang, W. Ma, Q. Yu, J. Wang and L. Chen, Impact of spatial resolution on air quality simulation: a case study in a highly industrialized area in Shanghai, China, Atmos. Pollut. Res., 2015, 6, 322–333, DOI:10.5094/APR.2015.036.
- F. Garcia-Menendez, A. Yano, Y. Hu and M. Talat Odman, An adaptive grid version of CMAQ for improving the resolution of plumes, Atmos. Pollut. Res., 2010, 1, 239–249, DOI:10.5094/APR.2010.031.
-
K. W. Appel, J. O. Bash, K. M. Fahey, K. M. Foley, R. C. Gilliam, C. Hogrefe, et al., The Community Multiscale Air Quality (CMAQ) Model Versions 5.3 and 5.3.1: System Updates and Evaluation, Copernicus GmbH, 2020, available from: DOI:10.5194/gmd-2020-345.
- S. Reis, T. Liška, M. Vieno, E. J. Carnell, R. Beck and T. Clemens,
et al., The influence of residential and workday population mobility on exposure to air pollution in the UK, Environ. Int., 2018, 121, 803–813, DOI:10.1016/j.envint.2018.10.005.
- N. Azouz, J. L. Drouet, M. Beekmann, G. Siour, R. Wichink Kruit and P. Cellier, Comparison of spatial patterns of ammonia concentration and dry deposition flux between a regional Eulerian chemistry-transport model and a local Gaussian plume model, Air Qual., Atmos. Health, 2019, 12, 719–729, DOI:10.1007/s11869-019-00691-y.
- N. Azouz, M. Beekmann, G. Siour, P. Cellier and J. L. Drouet, Changes in spatial patterns of ammonia dry deposition flux and deposition threshold exceedance according to dispersion model formalism and horizontal resolution, Environ. Pollut., 2021, 268, 115823, DOI:10.1016/j.envpol.2020.115823.
-
A. Jones, D. Thomson, M. Hort and B. Devenish, The U.K. Met Office's Next-Generation Atmospheric Dispersion Model, NAME III, Air Pollution Modeling and Its Application XVII, Springer, US, 2007, 5809, available from: DOI:10.1007/978-0-387-68854-1_62.
- Y. Sanada, G. Katata, N. Kaneyasu, C. Nakanishi, Y. Urabe and Y. Nishizawa, Altitudinal characteristics of atmospheric deposition of aerosols in mountainous regions: Lessons from the Fukushima Daiichi Nuclear Power Station accident, Sci. Total Environ., 2018, 618, 881–890, DOI:10.1016/j.scitotenv.2017.08.246.
-
J. S. Scire, D. G. Strimaitis, R. J. Yamartino and X. Zhang, A user's guide for the CALPUFF dispersion model (Version 5), Earth Tech Inc., Concord, 2000, available from: https://www.src.com/calpuff/download/CALPUFF_UsersGuide.pdf Search PubMed.
- D. Giaiotti, D. Oshurok and O. Skrynyk, The Chernobyl nuclear accident 137Cs cumulative depositions simulated by means of the CALMET/CALPUFF modelling system, Atmos. Pollut. Res., 2018, 9, 502–512, DOI:10.1016/j.apr.2017.11.007.
- A. S. Rood, P. G. Voillequé, S. K. Rope, H. A. Grogan and J. E. Till, Reconstruction of atmospheric concentrations and deposition of uranium and decay products released from the former uranium mill at Uravan, Colorado, J. Environ. Radioact., 2008, 99, 1258–1278, DOI:10.1016/j.jenvrad.2008.03.009.
- L. Tositti, B. Moroni, E. Dinelli, P. Morozzi, E. Brattich and B. Sebastiani,
et al., Deposition processes over complex topographies: experimental data meets atmospheric modeling, Sci. Total Environ., 2020, 744, 140974, DOI:10.1016/j.scitotenv.2020.140974.
- N. B. Chang and D. Q. Chang, Long-term risk assessment of possible accidental release of nuclear power plants in complex terrains with respect to synoptic weather patterns, Front. Earth Sci. China, 2009, 4, 205–228, DOI:10.1007/s11707-010-0001-0.
- B. J. Viner and R. W. Arritt, Small-Scale Circulations Caused by Complex Terrain Affect Pollen Deposition, Crop Sci., 2012, 52, 904–913, DOI:10.2135/cropsci2011.07.0354.
- B. J. Viner, R. W. Arritt and M. E. Westgate, Examination of climatological wind patterns and simulated pollen dispersion in a complex island environment, Int. J. Biometeorol., 2017, 61, 1481–1492, DOI:10.1007/s00484-017-1325-1.
- Z. Y. Offer and D. Goossens, Wind tunnel experiments and field measurements of aeolian dust deposition on conical hills, Geomorphology, 1995, 14, 43–56, DOI:10.1016/0169-555X(95)00015-W.
-
S. T. Parker, An investigation into the effects of complex topography on particle dry deposition, PhD Thesis, School of Geography, Earth and Environmental Sciences, University of Birmingham, 2004.
- J. Finnigan, K. Ayotte, I. Harman, G. Katul, H. Oldroyd and E. Patton,
et al., Boundary-Layer Flow Over Complex Topography, Bound.-Layer Meteorol., 2020, 177, 247–313, DOI:10.1007/s10546-020-00564-3.
- S. A. Smith, S. B. Vosper and P. R. Field, Sensitivity of orographic precipitation enhancement to horizontal resolution in the operational Met Office Weather forecasts, Meteorol. Appl., 2012, 22, 14–24, DOI:10.1002/met.1352.
- N. M. Roberts, S. J. Cole, R. M. Forbes, R. J. Moore and D. Boswell, Use of high-resolution NWP rainfall and river flow forecasts for advance warning of the Carlisle flood, north-west England, Meteorol. Appl., 2009, 16, 23–34, DOI:10.1002/met.94.
- T. A. Hill, A. Jones and T. W. Choularton, Modelling Sulphate Deposition Onto Hills By Washout and Turbulence, Q. J. R. Metereol. Soc., 2007, 113, 1217–1236, DOI:10.1002/qj.49711347808.
- D. J. Carruthers and T. W. Choularton, Airflow over hills of moderate slope, Q. J. R. Metereol. Soc., 1982, 108, 603–624, DOI:10.1002/qj.49710845708.
- A. J. Dore, T. W. Choularton, D. Fowler and R. Storton-West, Field measurements of wet deposition in an extended region of complex topography, Q. J. R. Metereol. Soc., 1990, 116, 1193–1212, DOI:10.1002/qj.49711649510.
- M. Blaś, A. J. Dore and M. Sobik, Distribution of precipitation and wet deposition around an Island mountain in South-West Poland, Q. J. R. Metereol. Soc., 1999, 125, 253–270, DOI:10.1002/qj.49712555314.
- A. J. Dore, M. Mousavi-Baygi, R. I. Smith, J. Hall, D. Fowler and T. W. Choularton, A model of annual orographic precipitation and acid deposition and its application to Snowdonia, Atmos. Environ., 2006, 40, 3316–3326, DOI:10.1016/j.atmosenv.2006.01.043.
- J. E. Stout, Y. L. Lin and S. P. S. Arya, A Theoretical investigation of the Effects of Sinusoidal Topography on Particle Deposition, J. Atmos. Sci., 1993, 50, 2533–2541, DOI:10.1175/1520-0469(1993)050<2533:ATIOTE>2.0.CO;2.
- G. G. Katul and D. Poggi, The Influence of Hilly Terrain on Aerosol-Sized Particle Deposition into Forested Canopies, Bound.-Layer Meteorol., 2009, 135, 67–88, DOI:10.1007/s10546-009-9459-2.
- G. G. Katul and D. Poggi, A note on aerosol sized particle deposition onto dense and tall canopies
situated on gentle cosine hills, Tellus B, 2011, 63, 395–400, DOI:10.1111/j.1600-0889.2011.00528.x.
- D. Poggi and G. G. Katul, An experimental investigation of the mean momentum budget inside dense canopies on narrow gentle hilly terrain, Agric. For. Meteorol., 2007, 144, 1–13, DOI:10.1016/j.agrformet.2007.01.009.
- D. Poggi and G. G. Katul, Micro- and macro-dispersive fluxes in canopy flows, Acta Geophys., 2008, 56, 778–799, DOI:10.2478/s11600-008-0029-7.
-
I. N. Harman and J. J. Finnigan, The SCION conifer wilding analytical models: Overview. CSIRO Oceans and Atmosphere interim report, 2019, p. 16 Search PubMed.
-
I. N. Harman and J. J. Finnigan, Flow over a gentle Hill covered with a plant canopy: three-dimensional effects and extension to dispersal. CSIRO Oceans and Atmosphere interim report, 2019, p. 22 Search PubMed.
-
I. N. Harman and J. J. Finnigan, The SCION conifer wilding analytical models: Overview. CSIRO Oceans and Atmosphere interim report, 2019, p. 16 Search PubMed.
-
I. N. Harman, J. J. Finnigan and E. G. Patton, Comparing observations and models of wind over complex forested terrain. CSIRO Oceans and Atmosphere, 2020, p. 26 Search PubMed.
- T. Michioka, H. Takimoto, H. Ono and A. Sato, Numerical Simulations of Dry and Wet Deposition over Simplified Terrains, Asian J. Atmos. Environ., 2017, 11, 270–282, DOI:10.5572/ajae.2017.11.4.270.
-
D. J. Carruthers, J. C. R. Hunt and W. -S. Weng, A computational model of stratified turbulent airflow over hills—FLOWSTAR I. Proceedings of Envirosoft, in Computer Techniques in Environmental Studies, ed. P. Zanetti, Springer-Verlag, New York, NY, 1988, pp. 481–492 Search PubMed.
- P. S. Jackson and J. C. R. Hunt, Turbulent wind flow over a low hill, Q. J. R. Metereol. Soc., 1975, 101, 929–955, DOI:10.1002/qj.49710143015.
- J. C. R. Hunt, S. Leibovich and K. J. Richards, Turbulent shear flows over low hills, Q. J. R. Metereol. Soc., 1988, 114, 1435–1470, DOI:10.1002/qj.49711448405.
- H. Coe, T. W. Choularton, D. J. Carruthers, M. W. Gallagher and K. N. Bower, A model of occult deposition applicable to complex terrain, Q. J. R. Metereol. Soc., 1991, 117, 803–823, DOI:10.1002/qj.49711750008.
- G. Ahmadi and A. Li, Computer simulation of particle transport and deposition near a small isolated building, J. Wind. Eng. Ind. Aerodyn., 2000, 84, 23–46, DOI:10.1016/S0167-6105(99)00048-3.
- R. W. Arritt, A Numerical Modeling Technique for Estimating Sulfur Dioxide Dry Deposition Due to Local Source Emissions, J. Air Waste Manage. Assoc., 1991, 41, 1341–1347, DOI:10.1080/10473289.1991.10466931.
- B. Blocken, A. van der Hout, J. Dekker and O. Weiler, CFD simulation of wind flow over natural complex terrain: case study with validation by field measurements for Ria de Ferrol, Galicia, Spain, J. Wind. Eng. Ind. Aerodyn., 2015, 147, 43–57, DOI:10.1016/j.jweia.2015.09.007.
- N. Wood, Wind Flow Over Complex Terrain: A Historical Perspective and the Prospect for Large-Eddy Modelling, Bound.-Layer Meteorol., 2000, 96, 11–32, DOI:10.1023/A:1002017732694.
- M. d. A. S. Bhuiyan and J. M. Alam, Scale-adaptive turbulence modeling for LES over complex terrain, Eng. Comput., 2020, 38, 1995–2007, DOI:10.1007/s00366-020-01190-w.
- Z. Liu, S. Cao, H. Liu and T. Ishihara, Large-Eddy Simulations of the Flow Over an Isolated Three-Dimensional Hill, Bound.-Layer Meteorol., 2018, 170, 415–441, DOI:10.1007/s10546-018-0410-2.
- Q. Yang, T. Zhou, B. Yan, P. Van Phuc and W. Hu, LES study of turbulent flow fields over hilly terrains—Comparisons of inflow turbulence generation methods and SGS models, J. Wind. Eng. Ind. Aerodyn., 2020, 204, 104230, DOI:10.1016/j.jweia.2020.104230.
- R. Buccolieri, J. L. Santiago, E. Rivas and B. Sanchez, Review on urban tree modelling in CFD simulations: aerodynamic, deposition and thermal effects, Urban Forestry and Urban Greening, 2018, 31, 212–220, DOI:10.1016/j.ufug.2018.03.003.
- C. Daly, M. Halbleib, J. I. Smith, W. P. Gibson, M. K. Doggett and G. H. Taylor,
et al., Physiographically sensitive mapping of climatological temperature and precipitation across the conterminous United States, Int. J. Climatol., 2008, 28, 2031–2064, DOI:10.1002/joc.1688.
- N. E. Latysh and G. A. Wetherbee, Improved mapping of National Atmospheric Deposition Program wet-deposition in complex terrain using PRISM-gridded data sets, Environ. Monit. Assess., 2011, 184, 913–928, DOI:10.1007/s10661-011-2009-7.
- A. J. Dore, T. W. Choularton and D. Fowler, An improved wet deposition map of the United Kingdom incorporating the seeder—feeder effect over mountainous terrain, Atmos. Environ., Part A, 1992, 26, 1375–1381, DOI:10.1016/0960-1686(92)90122-2.
- A. J. Dore, M. Sobik and K. Migala, Patterns of precipitation and pollutant deposition in the western Sudete mountains, Poland, Atmos. Environ., 1999, 33, 3301–3312, DOI:10.1016/S1352-2310(98)00294-5.
-
A. Argyraki, R. P. Kinnersley and M. H. Ramsey, Optimising emergency sampling strategy following a release of radioactive material into the environment, MAFF Research Contract No. RP 0249, 1999 Search PubMed.
- B. B. Hicks, On Estimating Dry Deposition Rates in Complex Terrain, J. Appl. Meteorol. Climatol., 2008, 47, 1651–1658, DOI:10.1175/2006JAMC1412.1.
- K. C. Weathers, S. M. Simkin, G. M. Lovett and S. E. Lindberg, Empirical modelling of atmospheric deposition in mountainous landscapes, Ecol. Appl., 2006, 16, 1590–1607, DOI:10.1890/1051-0761(2006)016[1590:EMOADI]2.0.CO;2.
- K. B. Benedict, C. M. Carrico, S. M. Kreidenweis, B. Schichtel, W. C. Malm and J. L. Collett Jr, A seasonal nitrogen deposition budget for Rocky Mountain National Park, Ecol. Appl., 2013, 23, 1156–1169, DOI:10.1890/12-1624.1.
- G. P. J. Draaijers, R. van Eak, W. Bleuten and R. Meijers, Measuring and modelling atmospheric dry deposition in complex forest terrain, Stud. Environ. Sci., 1992, 285–294, DOI:10.1016/S0166-1116(08)70123-7.
- L. J. Sheppard, I. D. Leith, T. Mizunuma, J. Neil Cape, A. Crossley and S. Leeson,
et al., Dry deposition of ammonia gas drives species change faster than wet deposition of ammonium ions: evidence from a long-term field manipulation, GCB Bioenergy, 2011, 17, 3589–3607, DOI:10.1111/j.1365-2486.2011.02478.x.
- T. W. Choularton, R. N. Colvile, K. N. Bower, M. W. Gallagher, M. Wells and K. M. Beswick,
et al., The great dun fell cloud experiment 1993: an overview, Atmos. Environ., 1997, 31, 2393–2405, DOI:10.1016/s1352-2310(96)00316-0.
-
K. N. Bower, T. W. Choularton, M. W. Gallagher, R. N. Colvile, K. M. Beswick, D. W. F. Inglis, et al., The Great Dun Fell Experiment 1995: an overview, Atmospheric Research, Elsevier BV, 1999, pp. 151–184, available from: DOI:10.1016/s0169-8095(98)00103-3.
|
This journal is © The Royal Society of Chemistry 2022 |
Click here to see how this site uses Cookies. View our privacy policy here.