In silico peptide-directed ligand design complements experimental peptide-directed binding for protein–protein interaction modulator discovery†
Received
10th August 2020
, Accepted 7th November 2020
First published on 19th November 2020
Abstract
Using the protein–protein interaction of Mcl-1/Noxa, two methods for efficient modulator discovery are directly compared. In silico peptide-directed ligand design is evaluated against experimental peptide-directed binding, allowing for the discovery of two new inhibitors of Mcl-1/Noxa with cellular activity. In silico peptide-directed ligand design demonstrates an in vitro hit rate of 80% (IC50 < 100 μM). The two rapid and efficient methods demonstrate complementary features for protein–protein interaction modulator discovery.
Introduction
Drug discovery for protein–protein interactions (PPIs) has made huge advances in the last decade, making strides to shed the “undruggable” label. The application of fragment based drug discovery1–3 and high throughput screening4,5 has resulted in many compounds undergoing clinical trials,6–9 and the approval of venetoclax in 2016.10 The methods have proven to be effective, but remain available only to pharmaceutical companies and specially equipped laboratories. In order to address this several innovative techniques have been reported to streamline PPI drug discovery.11 The development of constrained peptides12–15 and peptidomimetics,16–19 the application of new chemical modalities,20–22 and the demonstration of dynamic ligation screening23 has offered techniques for PPI modulation which utilise the shrewd application of commonly available resources and chemistries.
In an attempt to further improve the economy of drug discovery for PPIs we recently reported peptide-directed binding24 as a method to identify selective small molecule modulators. This technique extends on the REPLACE technique,25–27 utilising high affinity peptides as scaffolds for small molecule fragment identification. Using the PPI of apoptosis influencing Mcl-1/Noxa as a paradigm, we took the NoxaB-(75–93)-C75A peptide (AAQLRRIGDKVNLRQKLLN, IC50 650 nM) and divided it into two half peptides, amino acids 75–84 (AAQLRRIGD) and 85–93 (KVNLRQKLLN, Fig. 1: step 1), each containing two key binding residues (L78, I81, V85, and Q89). Reactive terminals were added to the termini of the half peptides, an alkyne on the C terminus of AAQLRRIGD and an azide on the N terminus of KVNLRQKLLN. These half peptides, with and without reactive termini, possessed no discernible binding affinity for Mcl-1. The reactive termini where used to “click” small molecule fragments to the peptides, with copper catalysed alkyne azide cycloaddition. The binding affinity of the peptide-small molecule hybrids was examined. The fragments which were shown to restore binding affinity in some way emulate the peptide segment they have replaced (Fig. 1: step 2). The small molecule fragments that appeared to emulate amino acids 75–84 where then clicked to the small molecule fragments which emulated amino acids 85–93, providing small molecules with a high probability of emulating the entire NoxaB peptide (Fig. 1: step 3). The choice of small molecule fragments to be clicked to peptides was guided by in silico modelling of the covalent reaction, allowing for a high efficiency.
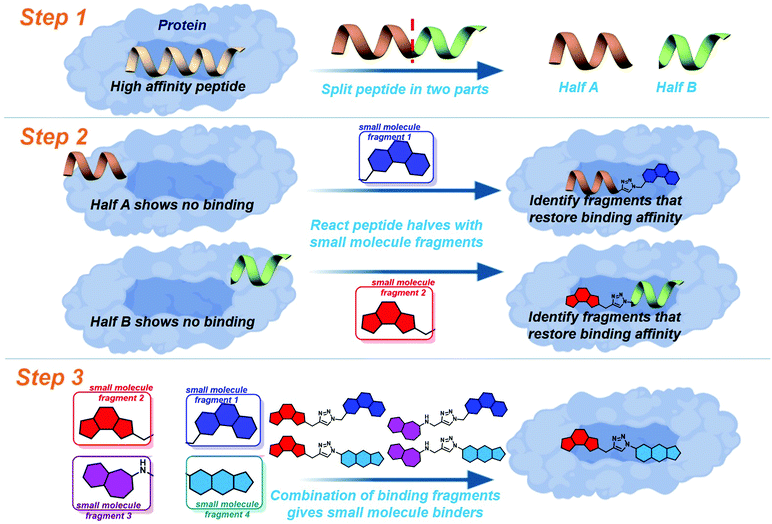 |
| Fig. 1 Schematic of peptide directed binding. The process can be completed experimentally, with the preparation of small molecule–peptide hybrids, identifying fragments which emulate half of the parent peptide. The identified small molecule fragments can be combined to create small molecules which control the target PPI. The process can also be performed virtually, identifying likely small molecule triazole candidates for synthesis. | |
To further improve the efficiency of this method we applied a fully computational peptide-directed ligand design to the PPIs of hDM2/hDMX and p53.28 This process mirrored that of peptide-directed binding, but performed steps 1–3 (Fig. 1) computationally, identifying a number of small molecules triazoles for synthesis. Analogous to our Mcl-1/Noxa example, the crystal structures of a modified p53 peptide bound to both hDM2 and hDMX were modified to generate two half peptides with reactive azide and alkyne termini. In silico covalent docking was performed to identify fragments which are likely to restore binding of the semi-peptide. Here the two methods diverge. Instead of preparing the identified peptide-small molecule hybrids, the top 10 azide fragments and top 10 alkyne fragments were combined virtually to identify 100 small molecule triazoles. This library was redocked to the protein target and the top 10 results were chosen for synthesis.
Our report of in silico peptide-directed ligand design28 demonstrated a higher efficiency at discovering small molecule inhibitors of PPIs when compared to the analogous experimental peptide-directed binding.24 The in silico method required the preparation of only 20 compounds to obtain a 50% hit rate of compounds which demonstrated an IC50 < 100 μM in in vitro protein fluorescence anisotropy assays. The experimental method revealed 54% of the small molecule compounds prepared demonstrated an IC50 < 100 μM, slightly higher than the in silico only route, but also required the preparation of 60 peptide-small molecule hybrids, adding time and cost. The 60 hybrids prepared identified 23 hits (reducing the hit rate to 44% for total compounds synthesised), which suggested 104 possible small molecule triazoles, of which we chose to prepare 35, based on availability and cost of components. It may be by chance that our hit rate was as high as observed, but seems unlikely given the number of compounds observed. The question remained, is one method clearly superior to the other, or are they complementary?
Results and discussion
To examine this, we performed in silico peptide-directed ligand design on the Mcl-1/Noxa interaction, allowing for a direct comparison of the experimental versus computational approach. The crystal structure of Mcl-1/NoxaB (PDB ID 2NLA29) was modified to generate the virtual reactive semi peptide AAQLRRIGD-propargylglycine and covalent docking30,31 was used to model copper catalysed azide alkyne cycloaddition in silico allowing us to identify the top ten30 synthetically viable small molecule azides fragments, from a commercially available library of 214 azides, which fit into the site vacated by the other section of peptide. Similarly, the crystal structure was modified to generate α-azidoamide-KVNLRQKLLN, and covalent docking was performed to identify small molecule alkyne fragments from a commercially available library of 869 alkynes. To avoid bias the library was taken directly from the Merck catalogue of available reagents. The top 10 azide and top 10 alkyne fragments were used to create a virtual library of 100 triazoles which was docked to Mcl-1 in an induced fit manner to score and rank the library. The library was also evaluated to predict ADME properties (SwissADME evaluation prediciting lipophilicity, water solubility, pharmacokinetics, drug-likeness (Lipinski, Ghose, Veber) and PAINS and lead-likeness screen),32 to help ensure synthetic effort was most likely to generate results. The top 10 results were chosen for synthesis following published procedures with CuSO4 and sodium ascorbate in a tBuOH/H2O mix,24 followed by purification with reverse phase HPLC. Synthesised compounds were evaluated in the FITC-NoxaB Mcl-1 competitive binding fluorescence anisotropy (FA) assay (Table 1 and Fig. 2A).24
Table 1 IC50 values for the inhibition of the binding of FITC-NoxaB to Mcl-1 of small moleculesa
|
Structure |
FA IC50 (μM) |
|
Structure |
FA IC50 (μM) |
IC50 values determined by non-linear regression of at least three independent experiments (see ESI). Errors are 95% confidence intervals (CI). Fmoc, 9-fluorenylmethylcarbonyl.
|
1
|
|
0.17 [0.15–0.19] |
6
|
|
3.38 [2.56–4.47] |
2
|
|
0.36 [0.29–0.45] |
7
|
|
4.39 [3.95–4.87] |
3
|
|
0.62 [0.47–0.80] |
8
|
|
7.03 [6.20–7.97] |
4
|
|
1.01 [0.89–1.15] |
9
|
|
>100 |
5
|
|
1.14 [0.84–1.52] |
10
|
|
>100 |
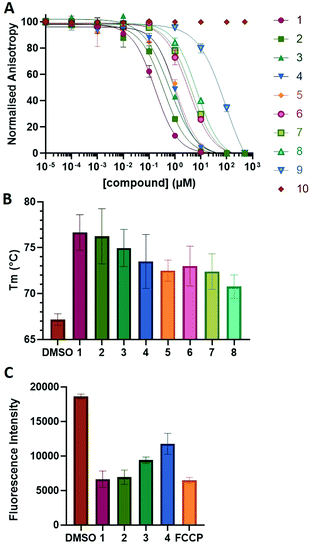 |
| Fig. 2 (A) Fluorescence anisotropy titration of compound 1–10 on 5 nm FITC-NoxaB peptide in the presence of 10 nm Mcl-1. (B) Thermal shift assay of compound 1–8 showing the change in melting temperature (Tm) of Mcl-1. (C) Mitochondrial depolarization assay of compound 1–4 and carbonyl cyanide 4-(trifluoromethoxy)phenylhydrazone (FCCP) demonstrating the fluorescence change of TMRE. | |
The NoxaB peptide (AAQLRRIGDKVNLRQKLLN) was employed as a positive control and to ensure the FA assay was performing adequately. Surprisingly, of the ten compounds prepared eight (80%) demonstrated binding in our assay, with an IC50 < 100 μM. Additionally, three of these compounds (1–3) demonstrated IC50 values less than 1 μM Selectivity for the Mcl-1/Noxa was evaluated by also examining the compounds in assays for Bcl-2/Bim, Bcl-xL/Bim, and Nrf2/Keap1. The ten compounds demonstrated excellent selectivity, with no appreciable inhibitory effect in these assays.
This very high hit rate demonstrates the power of peptide-directed ligand design for PPI modulators. Analysis of the compounds shows that four compounds were identified by experimental peptide-directed binding (1, 2, 4 & 6),24 with four new Mcl-1 binders identified with the in silico approach (3, 5, 7 & 8). Three of the top four binders (1, 2 & 4) were identified by the experimental peptide-directed binding, suggesting both experimental and computational methods are likely to find the most efficacious compounds. However, 1–6 have very similar structures, with the Fmoc-D-propargylglycine unit cyclised with a benzylazide, all of which demonstrated IC50 values <10 μM. The in silico method highlighted four new small molecule fragments which had not been highlighted in the experimental peptide-directed binding methodology24 (azide section of 3, 7, 8, and the alkyne section of 7 and 8). The binding of compounds to Mcl-1 was validated with a thermal shift assay33 using the hydrophobic dye Sypro orange.34 Compound 1–8 demonstrated the ability to increase the melting temperature of Mcl-1 compared to the DMSO vehicle control (Fig. 2B).
The compounds which demonstrated activity in the in vitro protein assays were evaluated in the MTS anti-proliferation assay,35 against pancreatic cancer cells lines. BxPC3 cells, which are dependent on Mcl-1, MiaPaCa2 cells, which are dependent on Mcl-1 and Bcl-2, and AsPC-1 cells, which do not overexpress Mcl-1,36–39 were treated with compound 1–8 (Table 2). Compound 5–8 showed no antiproliferative effect in all evaluated cell lines. Compound 1 and 2 demonstrated antiproliferative effects on all evaluated cell lines. Interestingly, compound 3 and 4 demonstrated efficacies in BxPC3 and MiaPaCa-2 cells, but no effect on Mcl-1 independent AsPC-1 cells, perhaps suggesting an Mcl-1 selective mode of action. 3 and 4 also suggest a para substituent of a certain size on the phenyl portion may offer selectivity. Compound 9 and 10 were highlighted by the ADME evaluation as unlikely to demonstrate cellular activity (c
log
P of 0.55 and −0.03 respectively), but were prepared nonetheless due to their high docking ranking. These results suggest that the subsequent ADME evaluation will offer increased efficiency if heeded.
Table 2 IC50 values for the cell growth inhibition of compounds which demonstrated activity towards pancreatic cancer cell lines, demonstrated by the MTS assaya
|
BxPC3 (μM) |
MiaPaCa-2 (μM) |
AsPC-1 (μM) |
IC50 values determined by non-linear regression of at least three independent experiments (see ESI). Errors are 95% confidence intervals (CI). Table 2: IC50 results obtained for compound 3 and 5 to selected cancerous cell lines in the presence of jacalin or bovine serum albumin (BSA) at 10 μM. Errors are 95% confidence intervals.
|
1
|
9.16 [5.97–14.88] |
7.20 [6.15–8.45] |
1.73 [1.42–2.14] |
2
|
1.48 [1.20–1.87] |
1.12 [1.36–1.94] |
4.401 [3.86–5.02] |
3
|
2.74 [1.56–5.00] |
7.09 [5.50–9.09] |
>100 μM |
4
|
1.35 [1.23–1.49] |
1.14 [1.10–1.19] |
>100 μM |
A tetramethylrhodamine ethyl ester (TMRE) assay was employed to evaluate if compounds generated mitochondrial membrane depolarization, one of the first signs of Mcl-1 inhibition (Fig. 2C).40,41 BxPC-3 cells were incubated with compound 1–4 or positive control, carbonyl cyanide 4-(trifluoromethoxy)phenylhydrazone (FCCP), followed by TMRE. TMRE readily accumulates in the mitochondria resulting in a fluorescent signal. Depolarized mitochondria result in reduced fluorescence. Compound 1–4 showed significantly reduced fluorescence after 2 h of incubation, indicating depolarized mitochondria membrane and apoptosis.
Docking poses predicted by induced fit docking suggest compounds are able to bind in the key P2 and P3 pockets. Poses for compound 1 and 2 (Fig. 3), are representative of binding poses generated for compound 1–8. The aromatic groups create contacts with Phe270 in P2 and Phe228 in P3, and interaction with Arg263 either through a water bridge interaction or hydrogen bonding. In line with the design of peptide-small molecule hybrids, the azide fragment which interacts with Arg263 in the hybrids, also interacts with Arg263 in the predicted pose for compound 1. This motif is repeated in the majority of the binding poses, but is not necessarily the predicted lowest energy conformer. Voisin-Chiret and co-workers recently highlighted the importance of the P2/P3 binding pockets and Arg263 for binding of non-peptidic ligands.42 Representative 2D ligand interaction diagrams are presented in the ESI.†
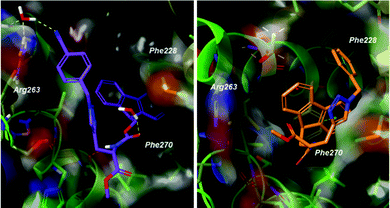 |
| Fig. 3 Predicted binding poses of 1 (left) and 2 (right). Compounds bind in the P2 and P3 pockets, making interactions with Phe228, Phe270 and Arg263. Poses are representative of the lowest energy predicted poses. | |
Conclusion
This study has achieved a direct comparison of peptide-directed binding and peptide-directed ligand design, using the Mcl-1/Noxa PPI as a paradigm. Both techniques have demonstrated 50% or higher hit rates previously, but experimental peptide-directed binding requires the synthesis of more molecules and therefore higher cost. The computational design has been further improved with the addition of ADME evaluation of highly ranked compounds, and in this example 80% of the prepared compounds demonstrated an IC50 < 100 μM in a fluorescence anisotropy assay. This application of peptide-directed ligand design to identify candidate triazoles is computationally 158 times more efficient than generating and screening a library of possible triazoles. The approach described here screened 1210 compounds in an induced fit manner – 214 azides, 896 alkynes and 100 triazoles – whereas screening the same fragment library as triazoles would have required the evaluation of 191
744 compounds. The results demonstrated here suggest that the techniques are complementary to each other. Both techniques appear to identity hits with promising potency, and both have identified compounds capable of demonstrating desired effects in cellular assays. Experimental peptide-directed binding has the advantage of identifying peptide-small molecule hybrids, which in themselves present possible leads for development. Computational peptide-directed ligand design allows for very few compounds to be prepared while still identifying potent, selective leads. While the experimental version requires more compounds to be prepared, it does provide a greater structural diversity, with computational methods highlighting one motif many times. While chemists may prefer a wider array of structures with which to learn from, biologists may prefer the limited synthesis required to generate selective modulators. Both of these techniques offer PPI researchers a rapid and efficient method to generate selective modulators of α-helical PPIs.
Conflicts of interest
There are no conflicts to declare.
Acknowledgements
This work was funded in part through a Royal Society Research Grant RGS/R1/201008 and EPSRC New Investigator Grant EP/M006379/1. We acknowledge the EPSRC UK National Mass Spectrometry Facility at Swansea University.
Notes and references
- E. Valkov, T. Sharpe, M. Marsh, S. Greive and M. Hyvönen, Top. Curr. Chem., 2012, 317, 145–179 CrossRef CAS.
-
F. Wang and S. W. Fesik, Fragment-based Drug Discovery: Lessons and Outlook, 2016, pp. 371–390 Search PubMed.
-
B. Xiong, Q. Wang and J. Shen, in Fragment-based Drug Discovery: Lessons and Outlook, ed. C. Sheng and G. I. Georg, Springer Singapore, Singapore, 2018, pp. 135–176 Search PubMed.
- A. G. Cochran, Chem. Biol., 2000, 7, R85–R94 CrossRef CAS.
- J. T. Heeres and P. J. Hergenrother, Chem. Soc. Rev., 2011, 40, 4398–4410 RSC.
- M. R. Arkin, Y. Tang and J. A. Wells, Chem. Biol., 2014, 21, 1102–1114 CrossRef CAS.
-
I. R. Hardcastle, Protein–Protein Interaction Inhibitors, in Cancer II, ed. M. J. Waring, Springer International Publishing, Cham, 2018, p. 399 Search PubMed.
- D. Bojadzic and P. Buchwald, Curr. Top. Med. Chem., 2018, 18, 674–699 CrossRef CAS.
- L. Mabonga and A. P. Kappo, Biophys. Rev., 2019, 11, 559–581 CrossRef CAS.
- E. D. Deeks, Drugs, 2016, 76, 979–987 CrossRef CAS.
- A. J. Wilson, Chem. Soc. Rev., 2009, 38, 3289–3300 RSC.
- L. Nevola and E. Giralt, Chem. Commun., 2015, 51, 3302–3315 RSC.
- D. M. Krüger, A. Glas, D. Bier, N. Pospiech, K. Wallraven, L. Dietrich, C. Ottmann, O. Koch, S. Hennig and T. N. Grossmann, J. Med. Chem., 2017, 60, 8982–8988 CrossRef.
- R. J. Steel, M. A. O’Connell and M. Searcey, Bioorg. Med. Chem. Lett., 2018, 28, 2728–2731 CrossRef CAS.
- D. Robertson and N. S. Spring, Molecules, 2018, 23, 959 CrossRef.
- L. R. Whitby and D. L. Boger, Acc. Chem. Res., 2012, 45, 1698–1709 CrossRef CAS.
- O. N. Akram, D. J. DeGraff, J. H. Sheehan, W. D. Tilley, R. J. Matusik, J.-M. Ahn and G. V. Raj, Mol. Cancer Res., 2014, 12, 967–978 CrossRef CAS.
- A. D. Cunningham, N. Qvit and D. Mochly-Rosen, Curr. Opin. Struct. Biol., 2017, 44, 59–66 CrossRef CAS.
- L. Mabonga and A. P. Kappo, Int. J. Pept. Res. Ther., 2020, 26, 225–241 CrossRef CAS.
- C. Sheng, G. Dong, Z. Miao, W. Zhang and W. Wang, Chem. Soc. Rev., 2015, 44, 8238–8259 RSC.
- E. Valeur, S. M. Guéret, H. Adihou, R. Gopalakrishnan, M. Lemurell, H. Waldmann, T. N. Grossmann and A. T. Plowright, Angew. Chem., Int. Ed., 2017, 56, 10294–10323 CrossRef CAS.
- E. Valeur, F. Narjes, C. Ottmann and A. T. Plowright, MedChemComm, 2019, 10, 1550–1568 RSC.
-
Z. Hegedüs, F. Hobor, D. K. Shoemark, S. Celis, L.-Y. Lian, C. Trinh, R. Sessions, T. Edwards and A. Wilson, ChemRxiv Prepr., DOI: 10.26434/chemrxiv.12429569.v1.
- A. M. Beekman, M. A. O’Connell and L. A. Howell, Angew. Chem., 2017, 56, 10446–10450 CrossRef CAS.
- M. J. I. Andrews, G. Kontopidis, C. McInnes, A. Plater, L. Innes, A. Cowan, P. Jewsbury and P. M. Fischer, ChemBioChem, 2006, 7, 1909–1915 CrossRef CAS.
- G. Kontopidis, M. J. Andrews, C. McInnes, A. Plater, L. Innes, S. Renachowski, A. Cowan and P. M. Fischer, ChemMedChem, 2009, 4, 1120–1128 CrossRef CAS.
- P. N. Premnath, S. N. Craig, S. Liu, E. L. Anderson, A. I. Grigoroudis, G. Kontopidis, T. L. Perkins, M. D. Wyatt, D. L. Pittman and C. McInnes, J. Med. Chem., 2015, 58, 433–442 CrossRef CAS.
- A. M. Beekman, M. M. D. Cominetti, S. J. Walpole, S. Prabhu, M. A. O’Connell, J. Angulo and M. Searcey, Chem. Sci., 2019, 10, 4502–4508 RSC.
- P. E. Czabotar, E. F. Lee, M. F. van Delft, C. L. Day, B. J. Smith, D. C. S. Huang, W. D. Fairlie, M. G. Hinds and P. M. Colman, Proc. Natl. Acad. Sci. U. S. A., 2007, 104, 6217–6222 CrossRef CAS.
- K. Zhu, K. W. Borrelli, J. R. Greenwood, T. Day, R. Abel, R. S. Farid and E. Harder, J. Chem. Inf. Model., 2014, 54, 1932–1940 CrossRef CAS.
- D. Toledo Warshaviak, G. Golan, K. W. Borrelli, K. Zhu and O. Kalid, J. Chem. Inf. Model., 2014, 54, 1941–1950 CrossRef CAS.
- A. Daina, O. Michielin and V. Zoete, Sci. Rep., 2017, 7, 42717 CrossRef.
- G. Greaves, M. Milani, M. Butterworth, R. J. Carter, D. P. Byrne, P. A. Eyers, X. Luo, G. M. Cohen and S. Varadarajan, Cell Death Differ., 2019, 26, 1037–1047 CrossRef CAS.
- M.-C. Lo, A. Aulabaugh, G. Jin, R. Cowling, J. Bard, M. Malamas and G. Ellestad, Anal. Biochem., 2004, 332, 153–159 CrossRef CAS.
- T. M. Buttke, J. A. McCubrey and T. C. Owen, J. Immunol. Methods, 1993, 157, 233–240 CrossRef CAS.
- H. Takahashi, M. C. Chen, D. M. Harris, H. Pham, H. A. Reber, O. J. Hines, V. L. W. Go and G. Eibl, Gastroenterology, 2011, 140, S-684 CrossRef.
- H. Takahashi, M. C. Chen, H. Pham, Y. Matsuo, H. Ishiguro, H. A. Reber, H. Takeyama, O. J. Hines and G. Eibl, Biochim. Biophys. Acta, Mol. Cell Res., 2013, 1833, 2980–2987 CrossRef CAS.
- F. Abulwerdi, C. Liao, M. Liu, A. S. Azmi, A. Aboukameel, A. S. A. Mady, T. Gulappa, T. Cierpicki, S. Owens, T. Zhang, D. Sun, J. A. Stuckey, R. M. Mohammad and Z. Nikolovska-Coleska, Mol. Cancer Ther., 2014, 13, 565–575 CrossRef CAS.
- D. Wei, Q. Zhang, J. S. Schreiber, L. A. Parsels, F. A. Abulwerdi, T. Kausar, T. S. Lawrence, Y. Sun, Z. Nikolovska-Coleska and M. A. Morgan, Transl. Oncol., 2015, 8, 47–54 CrossRef.
- M. Chen, A. D. Guerrero, L. Huang, Z. Shabier, M. Pan, T.-H. Tan and J. Wang, J. Biol. Chem., 2007, 282, 33888–33895 CrossRef CAS.
- D. Popova, A. Forsblad, S. Hashemian and S. O. P. Jacobsson, PLoS One, 2016, 11, e0166750 CrossRef.
- C. Denis, J. Sopková-de Oliveira Santos, R. Bureau and A. S. Voisin-Chiret, J. Med. Chem., 2020, 63, 928–943 CrossRef CAS.
Footnote |
† Electronic supplementary information (ESI) available: Full experimental descriptions of synthesis and biological experiments, and supporting figures. See DOI: 10.1039/d0cb00148a |
|
This journal is © The Royal Society of Chemistry 2021 |
Click here to see how this site uses Cookies. View our privacy policy here.